You signed in with another tab or window. Reload to refresh your session.You signed out in another tab or window. Reload to refresh your session.You switched accounts on another tab or window. Reload to refresh your session.Dismiss alert
This issue is to document a first proof of concept of applying Self Supervised Learning on some HSC images. We'll of course want to tune this example to achieve our actual task of identifying tidal features but it already pretty much contains all the tools we'll need.
Demo notebook is here and should run nicely on Colab, no local install needed.
What does it do?
Very quickly, here is what this notebook does: it trains a neural network to encode HSC images into a 1D "code", and this code has the property that similar images should have similar code.
Once the model is trained, we can compute the code of a galaxy that looks interesting to us, and easily find all other galaxies which have similar code. So, for instance, if I find this galaxy interesting:
I can find a bunch of similar objects, just by keeping the ones that have the closest code:
For us it will be super useful, because once the code is trained on unlabeled data, if we know some galaxies with tidal features, we'll be able to interrogate the dataset to find all other similar galaxies, which hopefully should also display tidal features.
How does it work?
For this project we will be using Contrastive Learning, it is a class of ML models that try to train a neural network to react the same way to 2 different instances of the same image which has undergone different perturbations (like rotations, noise, etc...). This results in learning a high-level representation of the image that concentrates on the semantic information. And this doesn't need any labels. These representations can then be used for a bunch of downstream applications.
To read more on Contrastive Learning, this astro paper is great:
This issue is to document a first proof of concept of applying Self Supervised Learning on some HSC images. We'll of course want to tune this example to achieve our actual task of identifying tidal features but it already pretty much contains all the tools we'll need.
Demo notebook is here and should run nicely on Colab, no local install needed.
What does it do?
Very quickly, here is what this notebook does: it trains a neural network to encode HSC images into a 1D "code", and this code has the property that similar images should have similar code.
Once the model is trained, we can compute the code of a galaxy that looks interesting to us, and easily find all other galaxies which have similar code. So, for instance, if I find this galaxy interesting:
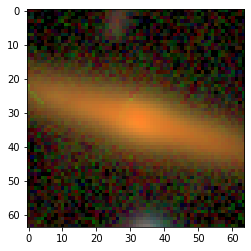
I can find a bunch of similar objects, just by keeping the ones that have the closest code:
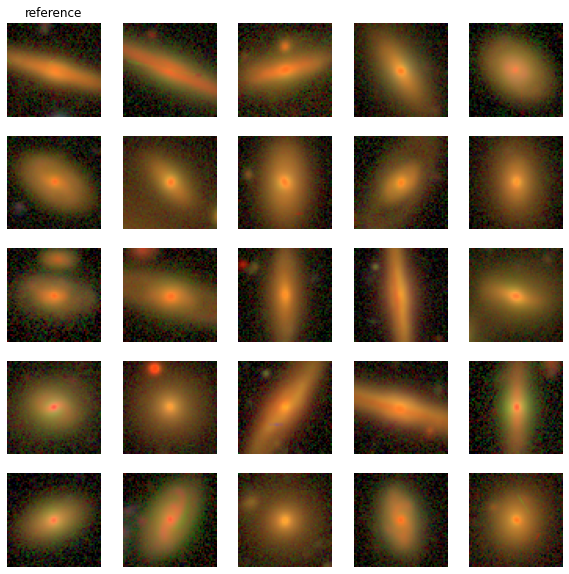
For us it will be super useful, because once the code is trained on unlabeled data, if we know some galaxies with tidal features, we'll be able to interrogate the dataset to find all other similar galaxies, which hopefully should also display tidal features.
How does it work?
For this project we will be using Contrastive Learning, it is a class of ML models that try to train a neural network to react the same way to 2 different instances of the same image which has undergone different perturbations (like rotations, noise, etc...). This results in learning a high-level representation of the image that concentrates on the semantic information. And this doesn't need any labels. These representations can then be used for a bunch of downstream applications.
To read more on Contrastive Learning, this astro paper is great:
And checkout this video from @mahayat and @georgestein: https://www.youtube.com/watch?v=LD4Zs8OCrOE
For starters, we'll be trying out the SimSiam model because it's super simple to implement and doesn't require a ton of computing power:
The text was updated successfully, but these errors were encountered: