You signed in with another tab or window. Reload to refresh your session.You signed out in another tab or window. Reload to refresh your session.You switched accounts on another tab or window. Reload to refresh your session.Dismiss alert
结合论文《Revisiting Deep Learning Models for Tabular Data》的观点,集成树模型通常擅长于表格数据这种异构数据集,是实打实的表格数据王者。集成树模型中的LightGBM是增强版的GBDT,支持了分类变量,在工程层面大大提高了训练效率。关于树模型的介绍,可见之前文章:一文讲透树模型
结合论文《Revisiting Deep Learning Models for Tabular Data》的观点,集成树模型通常擅长于表格数据这种异构数据集,是实打实的表格数据王者。集成树模型中的LightGBM是增强版的GBDT,支持了分类变量,在工程层面大大提高了训练效率。关于树模型的介绍,可见之前文章:一文讲透树模型
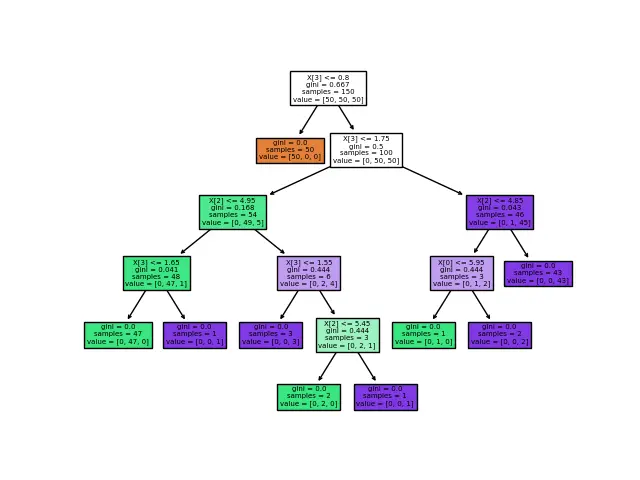
DNN深度神经网络擅长于同构的高维数据,从高维稀疏的表示中学习到低维致密的分布式表示,所以在自然语言、图像识别等领域基本上是称霸武林(神经网络的介绍及实践可见系列文章:一文搞定深度学习建模全流程)。对于异构致密的表格数据,个人实践来看,DNN模型的非线性能力没树模型来得高效。
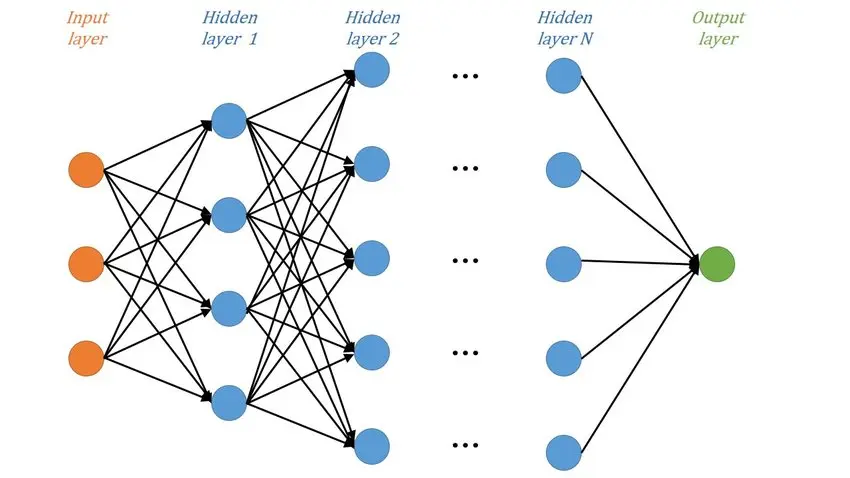
所以一个很朴素的想法是,结合这树模型+神经网络模型的优势。比如通过NN学习文本的嵌入特征后,输入树模型继续学习(如word2vec+LGB做文本分类,可见文章:NLP建模全流程)。 或者是,树模型学习表格数据后,输出样本的高维个叶子节点的特征表示,输入DNN模型。
接下来,我们使用LightGBM+DNN模型强强联手,验证其在信贷违约的表格数据预测分类效果。
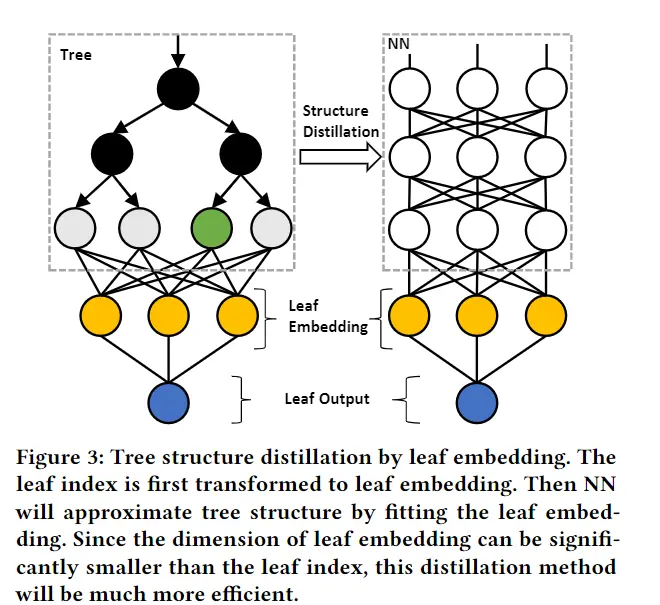
数据处理及树模型训练
lightgbm树模型,自带缺失、类别变量的处理,还有很强的非线性拟合能力,特征工程上面不用做很多处理,建模非常方便。
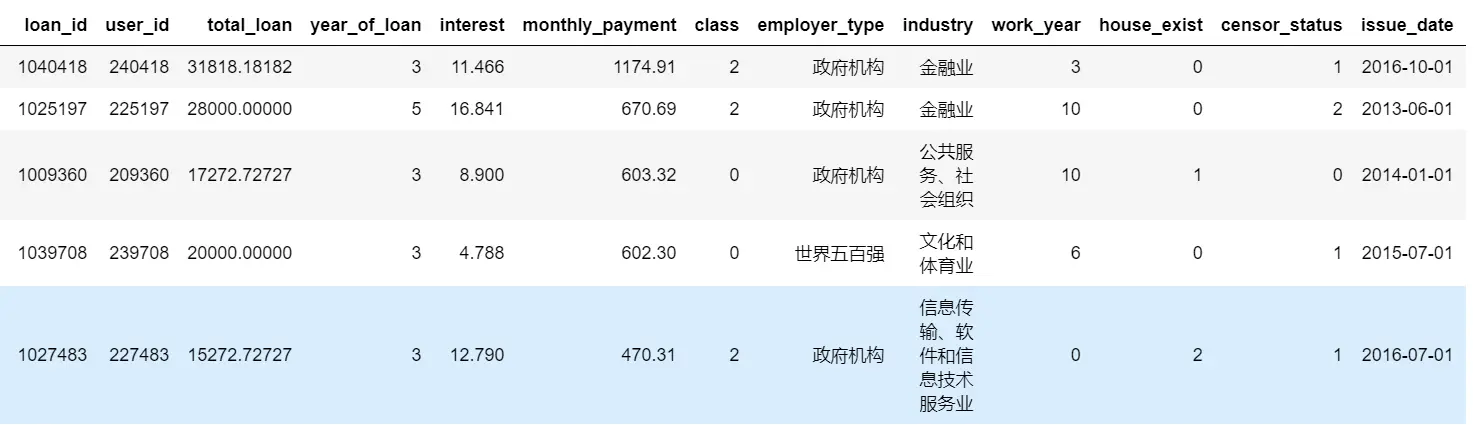
简单处理建模后test的AUC可以达到0.8656
树+神经网络
接下来我们将提取树模型的叶子节点的路径作为特征,并简单做下特征选择处理
将拼接好原特征及树节点路径特征输入神经网络模型,并使用网格搜索调优神经网络模型。
可见,在我们这个实验中,使用树模型+神经网络模型在test的auc得到一些不错的提升,树模型的AUC 0.8656,而树模型+神经网络的AUC 0.8776,提升了1.2%
其他试验结果
结合微软的试验,树+神经网络(DeepGBM),在不同的任务上也是可以带来一些的效果提升的。有兴趣可以阅读下文末参考文献。
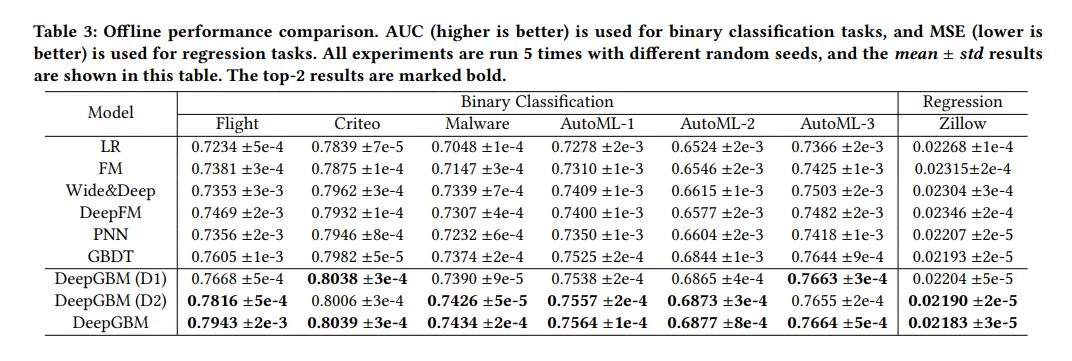
LGB+DNN(或者单层的LR)是一个很不错的想法,有提升模型的一些效果。但需要注意的是,这也会加重模型的落地及迭代的复杂度。总之,树+神经网络是一个好的故事,但是结局没有太惊艳。
The text was updated successfully, but these errors were encountered: