-
Notifications
You must be signed in to change notification settings - Fork 17
New issue
Have a question about this project? Sign up for a free GitHub account to open an issue and contact its maintainers and the community.
By clicking “Sign up for GitHub”, you agree to our terms of service and privacy statement. We’ll occasionally send you account related emails.
Already on GitHub? Sign in to your account
comparing single cell and single nuclei datasets #279
Comments
Update: |
For the second plot, I'd suggest looking at residual overdispersion rather than just overdispersion - if there's a global decrease in mean expression levels in the snRNAseq data relative to scRNAseq data (as you'd expect), this will tend to be accompanied by an increase in overdispersion. The offset correction of mean expression levels performed in |
Hi!
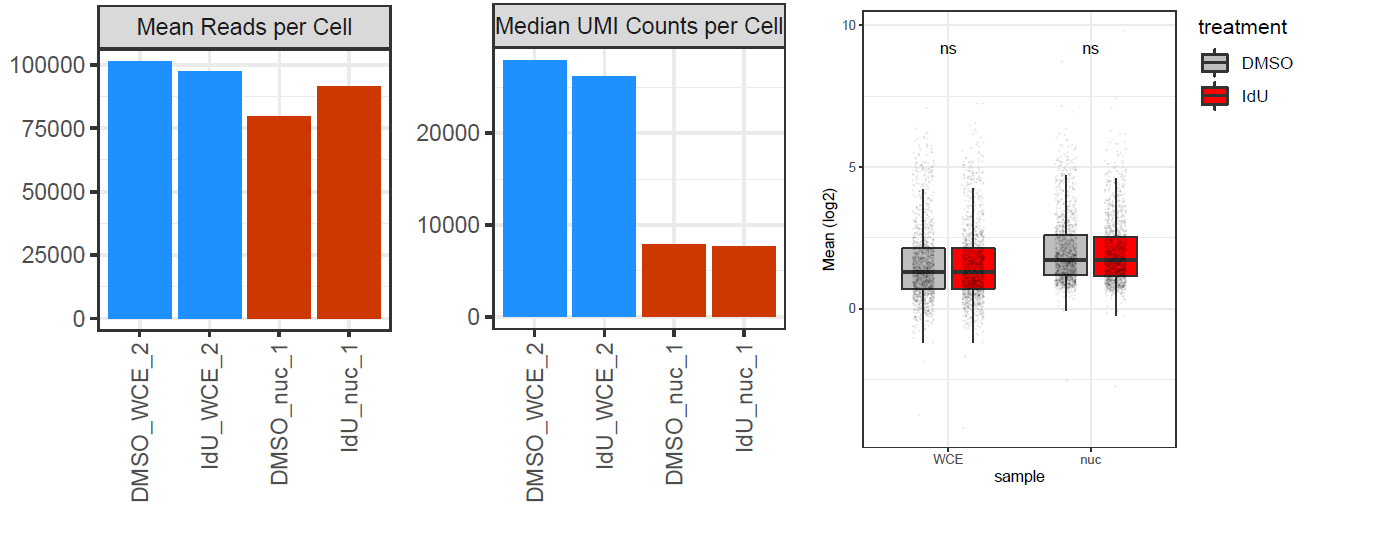
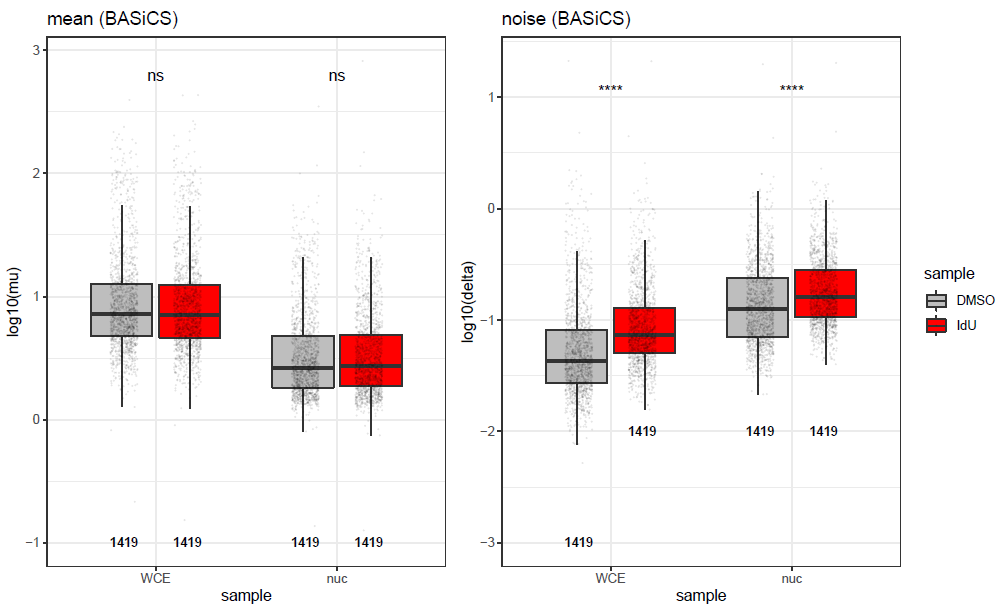
I am trying to compare mean and noise values between two datasets, one is single cell and one is single nuclei. both runs were performed on the same platform, with identical conditions, and the same cells, the only difference is the nuclei isolation process. The problem is that nuclei (nuc) and whole cells (WCE) have different RNA content, and the 10x methodology I used (droplet-based) doesn't allow spike-ins. So I end up with very different sequencing saturations - for WCE it's around 40%, while for nuc it's around 65%. Correspondingly, the read count to UMI count ratio is very different (see plot below, CellRanger output stats). Finally, mean expression values in WCE and nuc are not comparable (see right plot below, normalized means across cells).
I tried to apply BASiCS to the data, but obviously it doesn't help, as the difference in mean expression values is strongly increased (in the opposite direction), and overdispersion values are even less comparable:
It seems to me that a simple scaling factor applied to the count matrices can solve this problem and "normalize" the WCE and nuc datasets, however, I'm not sure what would be a good way to estimate this factor (maybe by just comparing median UMI counts in each sample?). What would you do to address this? Does BASiCS have a built-in option for such correction? Or would you normalize manually?
Thanks!
Binyamin
The text was updated successfully, but these errors were encountered: