diff --git a/.devcontainer/build_apple_silicon/devcontainer.json b/.devcontainer/build_apple_silicon/devcontainer.json
new file mode 100644
index 0000000000000..386e9b7d883e5
--- /dev/null
+++ b/.devcontainer/build_apple_silicon/devcontainer.json
@@ -0,0 +1,61 @@
+// For format details, see https://aka.ms/devcontainer.json. For config options, see the README at:
+// https://github.com/microsoft/vscode-dev-containers/tree/v0.236.0/containers/docker-existing-dockerfile
+{
+ "name": "Ivy Apple Silicon Development Environment (build)",
+
+ "build": {
+ "dockerfile": "../../docker/DockerfileAppleSilicon",
+ "context": "../..",
+ "args": {
+ "pycon": ["3.10"]
+ }
+ },
+
+ "customizations": {
+ "vscode": {
+ "extensions": [
+ "ms-python.python"
+ ],
+ "settings": {
+ "python.defaultInterpreterPath": "/opt/miniconda/envs/multienv/bin/python3"
+ }
+ }
+ },
+
+ "postCreateCommand": {
+ "post_create": "bash .devcontainer/post_create_commands.sh",
+ "bashrc": "echo \"alias python=python3\" >> ~/.bashrc"
+ },
+
+ // Use 'forwardPorts' to make a list of ports inside the container available locally.
+ // "forwardPorts": [],
+
+ // Uncomment the next line to run commands after the container is created - for example installing curl.
+
+ // Uncomment when using a ptrace-based debugger like C++, Go, and Rust
+ // "runArgs": [ "--cap-add=SYS_PTRACE", "--security-opt", "seccomp=unconfined" ],
+
+ // Uncomment to use the Docker CLI from inside the container. See https://aka.ms/vscode-remote/samples/docker-from-docker.
+ // "mounts": [ "source=/var/run/docker.sock,target=/var/run/docker.sock,type=bind" ],
+
+ // Uncomment to connect as a non-root user if you've added one. See https://aka.ms/vscode-remote/containers/non-root.
+ // "remoteUser": "vscode",
+ "features": {
+ "ghcr.io/devcontainers/features/common-utils:2": {
+ "installZsh": true,
+ "configureZshAsDefaultShell": true,
+ "installOhMyZsh": true,
+ "upgradePackages": false
+ },
+ "ghcr.io/devcontainers/features/docker-outside-of-docker:1": {
+ "moby": true,
+ "installDockerBuildx": true,
+ "version": "20.10",
+ "dockerDashComposeVersion": "v2"
+ },
+ "ghcr.io/devcontainers/features/github-cli:1": {
+ "installDirectlyFromGitHubRelease": true,
+ "version": "latest"
+ }
+ }
+}
diff --git a/.devcontainer/build_gpu/devcontainer.json b/.devcontainer/build_gpu/devcontainer.json
index 399fc8cf8d1b5..b74cb231c4a78 100644
--- a/.devcontainer/build_gpu/devcontainer.json
+++ b/.devcontainer/build_gpu/devcontainer.json
@@ -2,11 +2,11 @@
"name": "Ivy GPU Development Environment (build)",
"build": {
- "dockerfile": "../../docker/DockerfileGPUMultiCuda",
+ "dockerfile": "../../docker/DockerfileGPU",
"context": "../..",
"args": {
- "IMAGE_NAME": "unifyai/multicuda",
- "IMAGE_TAG": "base_and_requirements"
+ "IMAGE_NAME": "unifyai/ivy",
+ "IMAGE_TAG": "latest-gpu"
}
},
diff --git a/.devcontainer/image/devcontainer.json b/.devcontainer/devcontainer.json
similarity index 100%
rename from .devcontainer/image/devcontainer.json
rename to .devcontainer/devcontainer.json
diff --git a/.devcontainer/image_gpu/devcontainer.json b/.devcontainer/image_gpu/devcontainer.json
index 6824d7ca80037..ca899e132de7b 100644
--- a/.devcontainer/image_gpu/devcontainer.json
+++ b/.devcontainer/image_gpu/devcontainer.json
@@ -1,7 +1,7 @@
{
"name": "Ivy GPU Development Environment (image)",
- "image": "unifyai/multicuda:base_and_requirements",
+ "image": "unifyai/ivy:latest-gpu",
"customizations": {
"vscode": {
"extensions": [
diff --git a/.github/CODEOWNERS b/.github/CODEOWNERS
index 03da79e2b75fa..f24fc1e04f944 100644
--- a/.github/CODEOWNERS
+++ b/.github/CODEOWNERS
@@ -5,8 +5,7 @@ ivy/utils/backend @VedPatwardhan @CatB1t
ivy/utils/backend/ast_helpers.py @CatB1t
# Ivy Testing
-ivy_tests/test_ivy/helpers/ @sherry30 @CatB1t
-ivy_tests/array_api_testing/ @aarsh2001 @hirwa-nshuti
+ivy_tests/test_ivy/helpers/ @CatB1t
# Docs builder
docs/index.rst @KareemMAX
@@ -18,11 +17,5 @@ docs/overview/deep_dive/building_the_docs_pipeline.rst @KareemMAX
docs/_templates @KareemMAX
docs/demos @KareemMAX
-# Docker
-docker/* @ricksanchezstoic
-
-# Idea files
-.idea/* @Aarsh2001 @zaeemansari70
-
# README
README.md @guillesanbri
diff --git a/.github/pull_request_template.md b/.github/pull_request_template.md
index fae3cf3f9e5b8..f88501612a20e 100644
--- a/.github/pull_request_template.md
+++ b/.github/pull_request_template.md
@@ -16,7 +16,7 @@ Please use this format to link other issues with their numbers: Close #123
https://docs.github.com/en/issues/tracking-your-work-with-issues/linking-a-pull-request-to-an-issue#linking-a-pull-request-to-an-issue-using-a-keyword
-->
-Close #
+Closes #
## Checklist
diff --git a/.github/workflows/array-api-det-coverage.yml b/.github/workflows/array-api-det-coverage.yml
index ea913b3b17875..254fb28e548a0 100644
--- a/.github/workflows/array-api-det-coverage.yml
+++ b/.github/workflows/array-api-det-coverage.yml
@@ -1,11 +1,18 @@
name: array-api-determine-test-coverage
on:
workflow_dispatch:
+ schedule:
+ - cron: "30 20 * * 6"
+
permissions:
actions: read
jobs:
determine_coverage:
runs-on: ubuntu-latest
+ strategy:
+ fail-fast: false
+ matrix:
+ branch: [ 1, 2, 3, 4 ]
steps:
- name: Checkout Ivy π
uses: actions/checkout@v2
@@ -19,7 +26,7 @@ jobs:
run: |
pip install pydriller tqdm
cd ivy
- python run_tests_CLI/array_api_det_coverage.py
+ python scripts/determine_tests/array_api_det_coverage.py ${{ matrix.branch }}
cd ..
mkdir tests
cp ivy/tests.pbz2 tests/
@@ -32,6 +39,6 @@ jobs:
source-directory: tests/
destination-github-username: 'unifyai'
destination-repository-name: 'Mapping'
- user-email: rashul.chutani@gmail.com
+ user-email: ivy.branch@lets-unify.ai
commit-message: Update Array API Tests Mapping
- target-branch: main
+ target-branch: main${{ matrix.branch }}
diff --git a/.github/workflows/array-api-intelligent-tests-pr.yml b/.github/workflows/array-api-intelligent-tests-pr.yml
index ceea657f3d05a..2bb007f86ba61 100644
--- a/.github/workflows/array-api-intelligent-tests-pr.yml
+++ b/.github/workflows/array-api-intelligent-tests-pr.yml
@@ -5,33 +5,80 @@ on:
permissions:
actions: read
+
jobs:
+ display_test_results:
+ if: ${{ always() }}
+ runs-on: ubuntu-latest
+ needs:
+ - run_tests
+
+ steps:
+ - name: Download all test results
+ uses: actions/download-artifact@v3
+
+ - name: Combined Test Results
+ run: |
+ find . -name "test_results_*.txt" -exec cat {} + > combined_test_results.txt
+ echo "Test results summary:"
+ cat combined_test_results.txt
+
+ - name: New Failures Introduced
+ run: |
+ find . -name "new_failures_*.txt" -exec cat {} + > combined_failures.txt
+ if [ -s combined_failures.txt ]
+ then
+ echo "This PR introduces the following new failing tests:"
+ cat combined_failures.txt
+ else
+ echo "This PR does not introduce any new test failures! Yippee!"
+ fi
+
run_tests:
runs-on: ubuntu-latest
+ strategy:
+ fail-fast: false
+ matrix:
+ branch: [ 1, 2, 3, 4 ]
+
steps:
- name: Checkout Ivy π
- uses: actions/checkout@v2
+ uses: actions/checkout@v3
with:
path: ivy
persist-credentials: false
submodules: "recursive"
fetch-depth: 100
- - name: Determine Tests
+ - name: Get Job URL
+ uses: Tiryoh/gha-jobid-action@v0
+ id: jobs
+ with:
+ github_token: ${{ secrets.GITHUB_TOKEN }}
+ job_name: ${{ github.job }}
+
+ - name: Determine and Run Tests
run: |
- git clone -b main https://github.com/unifyai/Mapping.git --depth 1
+ git clone -b main${{ matrix.branch }} https://github.com/unifyai/Mapping.git --depth 1
pip install pydriller
cp Mapping/tests.pbz2 ivy/
cd ivy
- python run_tests_CLI/array_api_determine_tests.py
+ python scripts/determine_tests/array_api_determine_tests.py
+ python scripts/run_tests/array_api_run_tests_pr.py new_failures_${{ matrix.branch }}.txt | tee test_results_${{ matrix.branch }}.txt
+ cd ..
continue-on-error: true
- - name: Run Tests
- id: tests
- run: |
- cd ivy
- python run_tests_CLI/array_api_run_tests_pr.py
- continue-on-error: true
+ - name: Upload test results
+ uses: actions/upload-artifact@v3
+ with:
+ name: test_results_${{ matrix.branch }}
+ path: ivy/test_results_${{ matrix.branch }}.txt
+
+ - name: Upload New Failures
+ uses: actions/upload-artifact@v3
+ with:
+ name: new_failures_${{ matrix.branch }}
+ path: ivy/new_failures_${{ matrix.branch }}.txt
- name: Check on failures
if: steps.tests.outcome != 'success'
diff --git a/.github/workflows/array-api-intelligent-tests.yml b/.github/workflows/array-api-intelligent-tests.yml
index 5d18b3e64c9a3..576bbe9475e16 100644
--- a/.github/workflows/array-api-intelligent-tests.yml
+++ b/.github/workflows/array-api-intelligent-tests.yml
@@ -7,11 +7,32 @@ on:
permissions:
actions: read
jobs:
+ display_test_results:
+ if: ${{ always() }}
+ runs-on: ubuntu-latest
+ needs:
+ - run_tests
+
+ steps:
+ - name: Download all test results
+ uses: actions/download-artifact@v3
+
+ - name: Combined Test Results
+ run: |
+ find . -name "test_results_*.txt" -exec cat {} + > combined_test_results.txt
+ echo "Test results summary:"
+ cat combined_test_results.txt
+
run_tests:
runs-on: ubuntu-latest
+ strategy:
+ fail-fast: false
+ matrix:
+ branch: [ 1, 2, 3, 4 ]
+
steps:
- name: Checkout Ivy π
- uses: actions/checkout@v2
+ uses: actions/checkout@v3
with:
path: ivy
persist-credentials: false
@@ -29,26 +50,33 @@ jobs:
env:
SSH_DEPLOY_KEY: ${{ secrets.SSH_DEPLOY_KEY }}
run: |
- source ./ivy/clone_mapping.sh main
+ source ./ivy/scripts/shell/clone_mapping.sh main${{ matrix.branch }}
pip install pydriller pymongo
cp Mapping/tests.pbz2 ivy/
cd ivy
- python run_tests_CLI/array_api_determine_tests.py
+ python scripts/determine_tests/array_api_determine_tests.py ${{ matrix.branch }}
cd ..
cp ivy/tests.pbz2 Mapping/
cd Mapping
git add .
git commit -m "Update Mapping"
- git push origin main
+ git push origin main${{ matrix.branch }}
continue-on-error: true
- name: Run Tests
id: tests
run: |
cd ivy
- python run_tests_CLI/array_api_run_tests.py ${{ secrets.REDIS_CONNECTION_URL }} ${{ secrets.REDIS_PASSWORD }} ${{ secrets.MONGODB_PASSWORD }} ${{ github.run_id }} ${{ steps.jobs.outputs.html_url }}
+ set -o pipefail
+ python scripts/run_tests/array_api_run_tests.py ${{ secrets.REDIS_CONNECTION_URL }} ${{ secrets.REDIS_PASSWORD }} ${{ secrets.MONGODB_PASSWORD }} ${{ github.run_id }} ${{ steps.jobs.outputs.html_url }} | tee test_results_${{ matrix.branch }}.txt
continue-on-error: true
+ - name: Upload test results
+ uses: actions/upload-artifact@v3
+ with:
+ name: test_results_${{ matrix.branch }}
+ path: ivy/test_results_${{ matrix.branch }}.txt
+
- name: Check on failures
if: steps.tests.outcome != 'success'
run: exit 1
diff --git a/.github/workflows/binaries.yml b/.github/workflows/binaries.yml
index b02b0b8cba043..51dd639e700d0 100644
--- a/.github/workflows/binaries.yml
+++ b/.github/workflows/binaries.yml
@@ -1,18 +1,19 @@
-name: pypi
+name: release-binaries
on:
workflow_call:
jobs:
- runs-on: ubuntu-latest
- steps:
- - name: Checkout ποΈBinaries
- uses: actions/checkout@v2
- with:
- repository: unifyai/binaries
- path: binaries
- persist-credentials: false
+ release-binaries:
+ runs-on: ubuntu-latest
+ steps:
+ - name: Checkout ποΈBinaries
+ uses: actions/checkout@v2
+ with:
+ repository: unifyai/binaries
+ path: binaries
+ persist-credentials: false
- - name: Add Tag to Binaries
- run: |
- cd binaries
- git tag ${{ github.ref_name }}
- git push origin ${{ github.ref_name }}
+ - name: Add Tag to Binaries
+ run: |
+ cd binaries
+ git tag ${{ github.ref_name }}
+ git push origin ${{ github.ref_name }}
diff --git a/.github/workflows/det-test-coverage.yml b/.github/workflows/det-test-coverage.yml
index e8a560fe870f2..be26581eb0903 100644
--- a/.github/workflows/det-test-coverage.yml
+++ b/.github/workflows/det-test-coverage.yml
@@ -38,7 +38,7 @@ jobs:
run: |
pip install pydriller tqdm
cd ivy
- python determine_test_coverage.py ${{ matrix.branch }}
+ python scripts/determine_tests/determine_test_coverage.py ${{ matrix.branch }}
cd ..
mkdir tests
cp ivy/tests.pbz2 tests/
@@ -51,6 +51,6 @@ jobs:
source-directory: tests/
destination-github-username: 'unifyai'
destination-repository-name: 'Mapping'
- user-email: rashul.chutani@gmail.com
+ user-email: ivy.branch@lets-unify.ai
commit-message: Update Mapping
target-branch: master${{ matrix.branch }}
diff --git a/.github/workflows/dockerfile-multicuda-push.yml b/.github/workflows/dockerfile-gpu-push.yml
similarity index 60%
rename from .github/workflows/dockerfile-multicuda-push.yml
rename to .github/workflows/dockerfile-gpu-push.yml
index 79d774b11aee6..df978d2ee95a9 100644
--- a/.github/workflows/dockerfile-multicuda-push.yml
+++ b/.github/workflows/dockerfile-gpu-push.yml
@@ -1,4 +1,4 @@
-name: Dockerfile MultiCUDA Push
+name: GPU Dockerfile Push
on:
schedule:
@@ -20,8 +20,7 @@ jobs:
username: ${{ secrets.DOCKERHUB_USERNAME }}
password: ${{ secrets.DOCKERHUB_TOKEN }}
-
- - name: Build and push Dockerfile
+ - name: Build and push GPU image
run: |
- docker build --progress=plain --no-cache -t unifyai/multicuda:base_and_requirements -f docker/DockerfileGPUMultiCuda .
- docker push unifyai/multicuda:base_and_requirements
+ docker build --progress=plain --no-cache -t unifyai/ivy:latest-gpu -f docker/DockerfileGPU .
+ docker push unifyai/ivy:latest-gpu
diff --git a/.github/workflows/dockerfile-image.yml b/.github/workflows/dockerfile-image.yml
index 9c5be23f40475..3f82ea05ea63c 100644
--- a/.github/workflows/dockerfile-image.yml
+++ b/.github/workflows/dockerfile-image.yml
@@ -11,7 +11,7 @@ jobs:
build:
if: ${{(github.event_name == 'push') || contains(github.event.pull_request.labels.*.name, 'Exhaustive CI') || contains(github.event.pull_request.labels.*.name, 'Build Docker Files')}}
- runs-on: ubuntu-20.04
+ runs-on: ubuntu-latest
steps:
- uses: actions/checkout@v3
diff --git a/.github/workflows/dockerfile-push.yml b/.github/workflows/dockerfile-push.yml
index 06be15ea1e427..3a4e9959b6847 100644
--- a/.github/workflows/dockerfile-push.yml
+++ b/.github/workflows/dockerfile-push.yml
@@ -10,7 +10,7 @@ jobs:
build:
- runs-on: ubuntu-20.04
+ runs-on: ubuntu-latest
steps:
-
diff --git a/.github/workflows/duplication.yml b/.github/workflows/duplication.yml
index 3c647ec8d8968..4858881f132ef 100644
--- a/.github/workflows/duplication.yml
+++ b/.github/workflows/duplication.yml
@@ -21,7 +21,7 @@ jobs:
id: tests
run: |
cd ivy
- docker run --rm -v "$(pwd)":/ivy -v "$(pwd)"/.hypothesis:/.hypothesis unifyai/ivy:latest python3 duplicate.py
+ docker run --rm -v "$(pwd)":/ivy -v "$(pwd)"/.hypothesis:/.hypothesis unifyai/ivy:latest python3 scripts/duplicate.py
continue-on-error: true
- name: Check on failures
diff --git a/.github/workflows/intelligent-tests-pr.yml b/.github/workflows/intelligent-tests-pr.yml
index 5bee8006feb37..fa294f12f12fc 100644
--- a/.github/workflows/intelligent-tests-pr.yml
+++ b/.github/workflows/intelligent-tests-pr.yml
@@ -6,7 +6,6 @@ on:
permissions:
actions: read
- pull-requests: write
jobs:
display_test_results:
@@ -36,18 +35,6 @@ jobs:
echo "This PR does not introduce any new test failures! Yippee!"
fi
- - name: Write GitHub Comment
- uses: actions/github-script@v6
- with:
- github-token: ${{ secrets.GITHUB_TOKEN }}
- script: |
- github.rest.issues.createComment({
- issue_number: context.issue.number,
- owner: context.repo.owner,
- repo: context.repo.repo,
- body: 'π Thanks for the PR, the tests are ready to view!'
- })
-
run_tests:
runs-on: ubuntu-latest
strategy:
@@ -76,20 +63,34 @@ jobs:
submodules: "recursive"
fetch-depth: 100
+ - name: Install ivy and fetch binaries
+ run: |
+ cd ivy
+ pip3 install -e .
+ mkdir .ivy
+ touch .ivy/key.pem
+ echo -n ${{ secrets.USER_API_KEY }} > .ivy/key.pem
+ cd ..
+
+ - name: Get Job URL
+ uses: Tiryoh/gha-jobid-action@v0
+ id: jobs
+ with:
+ github_token: ${{ secrets.GITHUB_TOKEN }}
+ job_name: ${{ github.job }}
+
- name: Determine and Run Tests
id: tests
run: |
- git clone -b master${{ matrix.branch }} https://github.com/unifyai/Mapping.git --depth 200
+ git clone -b master${{ matrix.branch }} https://github.com/unifyai/Mapping.git --depth 1
pip install pydriller GitPython
- python ivy/run_tests_CLI/clone-mapping.py
+ python ivy/scripts/setup_tests/clone-mapping.py
cp Mapping/tests.pbz2 ivy/
cd ivy
- mkdir .ivy
- touch .ivy/key.pem
- echo -n ${{ secrets.USER_API_KEY }} > .ivy/key.pem
- python determine_tests.py ${{ matrix.branch }} pr
+ python scripts/determine_tests/determine_tests.py ${{ matrix.branch }} pr
set -o pipefail
- python run_tests_pr.py new_failures_${{ matrix.branch }}.txt | tee test_results_${{ matrix.branch }}.txt
+ python scripts/run_tests/run_tests_pr.py new_failures_${{ matrix.branch }}.txt | tee test_results_${{ matrix.branch }}.txt
+ cd ..
continue-on-error: true
- name: Upload test results
diff --git a/.github/workflows/intelligent-tests.yml b/.github/workflows/intelligent-tests.yml
index 41a8c4b7e7cbe..261cd5fd1014f 100644
--- a/.github/workflows/intelligent-tests.yml
+++ b/.github/workflows/intelligent-tests.yml
@@ -24,7 +24,7 @@ jobs:
cat combined_test_results.txt
run_tests:
- runs-on: ubuntu-20.04
+ runs-on: ubuntu-latest
strategy:
fail-fast: false
matrix:
@@ -51,6 +51,15 @@ jobs:
submodules: "recursive"
fetch-depth: 100
+ - name: Install ivy and fetch binaries
+ run: |
+ cd ivy
+ pip3 install -e .
+ mkdir .ivy
+ touch .ivy/key.pem
+ echo -n ${{ secrets.USER_API_KEY }} > .ivy/key.pem
+ cd ..
+
- name: Get Job URL
uses: Tiryoh/gha-jobid-action@v0
id: jobs
@@ -62,14 +71,11 @@ jobs:
env:
SSH_DEPLOY_KEY: ${{ secrets.SSH_DEPLOY_KEY }}
run: |
- source ./ivy/clone_mapping.sh master${{ matrix.branch }}
+ source ./ivy/scripts/shell/clone_mapping.sh master${{ matrix.branch }}
pip install pydriller pymongo
cp Mapping/tests.pbz2 ivy/
cd ivy
- mkdir .ivy
- touch .ivy/key.pem
- echo -n ${{ secrets.USER_API_KEY }} > .ivy/key.pem
- python determine_tests.py ${{ matrix.branch }}
+ python scripts/determine_tests/determine_tests.py ${{ matrix.branch }}
cd ..
cp ivy/tests.pbz2 Mapping/
cd Mapping
@@ -83,7 +89,7 @@ jobs:
run: |
cd ivy
set -o pipefail
- python run_tests.py ${{ secrets.REDIS_CONNECTION_URL }} ${{ secrets.REDIS_PASSWORD }} ${{ secrets.MONGODB_PASSWORD }} 'false' 'false' ${{ github.run_id }} 'false' ${{ steps.jobs.outputs.html_url }} | tee test_results_${{ matrix.branch }}.txt
+ python scripts/run_tests/run_tests.py ${{ secrets.REDIS_CONNECTION_URL }} ${{ secrets.REDIS_PASSWORD }} ${{ secrets.MONGODB_PASSWORD }} 'false' 'false' ${{ github.run_id }} 'false' ${{ steps.jobs.outputs.html_url }} | tee test_results_${{ matrix.branch }}.txt
continue-on-error: true
- name: Upload test results
diff --git a/.github/workflows/lint-bot.yml b/.github/workflows/lint-bot.yml
index 5136a5c1ad264..52ee85c1ae2d9 100644
--- a/.github/workflows/lint-bot.yml
+++ b/.github/workflows/lint-bot.yml
@@ -1,8 +1,9 @@
name: lint-bot
on:
- schedule:
- - cron: '0 8 * * *'
+ push:
+ branches:
+ - main
workflow_dispatch:
permissions:
diff --git a/.github/workflows/manual-tests-pr.yml b/.github/workflows/manual-tests-pr.yml
index 1e74fbff6d274..9f3534b2d357c 100644
--- a/.github/workflows/manual-tests-pr.yml
+++ b/.github/workflows/manual-tests-pr.yml
@@ -34,8 +34,8 @@ jobs:
mkdir .ivy
touch .ivy/key.pem
echo -n ${{ secrets.USER_API_KEY }} > .ivy/key.pem
- python setup_tests.py ${{ github.event.inputs.test }}
- python run_tests_pr.py new_failures.txt
+ python scripts/setup_tests/setup_tests.py ${{ github.event.inputs.test }}
+ python scripts/run_tests/run_tests_pr.py new_failures.txt
continue-on-error: true
- name: Check on failures
diff --git a/.github/workflows/manual-tests.yml b/.github/workflows/manual-tests.yml
index da0ec75f94cd0..819ff6cccc31e 100644
--- a/.github/workflows/manual-tests.yml
+++ b/.github/workflows/manual-tests.yml
@@ -30,12 +30,21 @@ jobs:
sudo rm -fr $GITHUB_WORKSPACE && mkdir $GITHUB_WORKSPACE
- name: Checkout Ivy π
- uses: actions/checkout@v2
+ uses: actions/checkout@v3
with:
path: ivy
persist-credentials: false
submodules: "recursive"
- set-safe-directory: false
+ fetch-depth: 100
+
+ - name: Install ivy and fetch binaries
+ run: |
+ cd ivy
+ pip3 install -e .
+ mkdir .ivy
+ touch .ivy/key.pem
+ echo -n ${{ secrets.USER_API_KEY }} > .ivy/key.pem
+ cd ..
- name: Get Job URL
uses: Tiryoh/gha-jobid-action@v0
@@ -49,11 +58,8 @@ jobs:
run: |
pip3 install pymongo
cd ivy
- mkdir .ivy
- touch .ivy/key.pem
- echo -n ${{ secrets.USER_API_KEY }} > .ivy/key.pem
- python3 setup_tests.py ${{ github.event.inputs.test }}
- python3 run_tests.py ${{ secrets.REDIS_CONNECTION_URL }} ${{ secrets.REDIS_PASSWORD }} ${{ secrets.MONGODB_PASSWORD }} 'false' ${{ github.event.inputs.gpu }} ${{ github.run_id }} 'false' ${{ steps.jobs.outputs.html_url }}
+ python3 scripts/setup_tests/setup_tests.py ${{ github.event.inputs.test }}
+ python3 scripts/run_tests/run_tests.py ${{ secrets.REDIS_CONNECTION_URL }} ${{ secrets.REDIS_PASSWORD }} ${{ secrets.MONGODB_PASSWORD }} 'false' ${{ github.event.inputs.gpu }} ${{ github.run_id }} 'false' ${{ steps.jobs.outputs.html_url }}
continue-on-error: true
- name: Check on failures
@@ -65,11 +71,21 @@ jobs:
runs-on: ubuntu-latest
steps:
- name: Checkout Ivy π
- uses: actions/checkout@v2
+ uses: actions/checkout@v3
with:
path: ivy
persist-credentials: false
submodules: "recursive"
+ fetch-depth: 100
+
+ - name: Install ivy and fetch binaries
+ run: |
+ cd ivy
+ pip3 install -e .
+ mkdir .ivy
+ touch .ivy/key.pem
+ echo -n ${{ secrets.USER_API_KEY }} > .ivy/key.pem
+ cd ..
- name: Get Job URL
uses: Tiryoh/gha-jobid-action@v0
@@ -83,11 +99,9 @@ jobs:
run: |
pip3 install pymongo
cd ivy
- mkdir .ivy
- touch .ivy/key.pem
- echo -n ${{ secrets.USER_API_KEY }} > .ivy/key.pem
- python setup_tests.py "${{ github.event.inputs.test }}"
- python run_tests.py ${{ secrets.REDIS_CONNECTION_URL }} ${{ secrets.REDIS_PASSWORD }} ${{ secrets.MONGODB_PASSWORD }} ${{ github.event.inputs.version}} 'false' ${{ github.run_id }} 'false' ${{ steps.jobs.outputs.html_url }}
+ pip3 install -e .
+ python scripts/setup_tests/setup_tests.py "${{ github.event.inputs.test }}"
+ python scripts/run_tests/run_tests.py ${{ secrets.REDIS_CONNECTION_URL }} ${{ secrets.REDIS_PASSWORD }} ${{ secrets.MONGODB_PASSWORD }} ${{ github.event.inputs.version}} 'false' ${{ github.run_id }} 'false' ${{ steps.jobs.outputs.html_url }}
continue-on-error: true
- name: Check on failures
diff --git a/.github/workflows/pre-release.yml b/.github/workflows/pre-release.yml
index f076990777952..483440add140b 100644
--- a/.github/workflows/pre-release.yml
+++ b/.github/workflows/pre-release.yml
@@ -7,10 +7,6 @@ permissions:
jobs:
run_tests:
runs-on: ubuntu-latest
- strategy:
- fail-fast: false
- matrix:
- file: [ivy.txt, torch.txt]
steps:
- name: Checkout Ivy π
uses: actions/checkout@v3
@@ -27,12 +23,21 @@ jobs:
github_token: ${{ secrets.GITHUB_TOKEN }}
job_name: ${{ github.job }}
- - name: Run Tests
+ - name: Setup Tests
run: |
pip3 install pymongo
cd ivy
mkdir .ivy
touch .ivy/key.pem
echo -n ${{ secrets.USER_API_KEY }} > .ivy/key.pem
- python run_tests_CLI/setup_priority_tests.py priority_tests/${{ matrix.file }}
- python run_tests.py ${{ secrets.REDIS_CONNECTION_URL }} ${{ secrets.REDIS_PASSWORD }} ${{ secrets.MONGODB_PASSWORD }} 'false' 'false' ${{ github.run_id }} 'true' ${{ steps.jobs.outputs.html_url }}
+ python scripts/setup_tests/setup_priority_tests.py ${{ secrets.MONGODB_PASSWORD }}
+
+ - name: Run CPU Tests
+ run: |
+ cd ivy
+ python scripts/run_tests/run_tests.py ${{ secrets.REDIS_CONNECTION_URL }} ${{ secrets.REDIS_PASSWORD }} ${{ secrets.MONGODB_PASSWORD }} 'false' 'false' ${{ github.run_id }} 'true' ${{ steps.jobs.outputs.html_url }}
+
+ - name: Run GPU Tests
+ run: |
+ cd ivy
+ python scripts/run_tests/run_tests.py ${{ secrets.REDIS_CONNECTION_URL }} ${{ secrets.REDIS_PASSWORD }} ${{ secrets.MONGODB_PASSWORD }} 'false' 'true' ${{ github.run_id }} 'true' ${{ steps.jobs.outputs.html_url }}
diff --git a/.github/workflows/pypi.yml b/.github/workflows/pypi.yml
index 7032f151da031..5515864a7fed7 100644
--- a/.github/workflows/pypi.yml
+++ b/.github/workflows/pypi.yml
@@ -29,4 +29,4 @@ jobs:
PYPI_PASSWORD: ${{ secrets.PYPI_PASSWORD }}
run: |
cd ivy
- bash deploy_pypi.sh
+ bash scripts/shell/deploy_pypi.sh
diff --git a/.github/workflows/run-all-tests.yml b/.github/workflows/run-all-tests.yml
index 1428280573130..64153d06ffa31 100644
--- a/.github/workflows/run-all-tests.yml
+++ b/.github/workflows/run-all-tests.yml
@@ -42,6 +42,15 @@ jobs:
submodules: "recursive"
fetch-depth: 100
+ - name: Install ivy and fetch binaries
+ run: |
+ cd ivy
+ pip3 install -e .
+ mkdir .ivy
+ touch .ivy/key.pem
+ echo -n ${{ secrets.USER_API_KEY }} > .ivy/key.pem
+ cd ..
+
- name: Get Job URL
uses: Tiryoh/gha-jobid-action@v0
id: jobs
@@ -53,12 +62,9 @@ jobs:
run: |
pip3 install pymongo
cd ivy
- mkdir .ivy
- touch .ivy/key.pem
- echo -n ${{ secrets.USER_API_KEY }} > .ivy/key.pem
- python run_tests_CLI/filter_tests.py ${{ matrix.branch }}
+ python scripts/setup_tests/filter_tests.py ${{ matrix.branch }}
set -o pipefail
- python run_tests.py ${{ secrets.REDIS_CONNECTION_URL }} ${{ secrets.REDIS_PASSWORD }} ${{ secrets.MONGODB_PASSWORD }} 'false' 'false' ${{ github.run_id }} 'false' ${{ steps.jobs.outputs.html_url }} | tee test_results_${{ matrix.branch }}.txt
+ python scripts/run_tests/run_tests.py ${{ secrets.REDIS_CONNECTION_URL }} ${{ secrets.REDIS_PASSWORD }} ${{ secrets.MONGODB_PASSWORD }} 'false' 'false' ${{ github.run_id }} 'false' ${{ steps.jobs.outputs.html_url }} | tee test_results_${{ matrix.branch }}.txt
continue-on-error: true
- name: Upload test results
diff --git a/.github/workflows/synchronize-db.yml b/.github/workflows/synchronize-db.yml
index 480e5c9a295f1..f5632e4f6ded0 100644
--- a/.github/workflows/synchronize-db.yml
+++ b/.github/workflows/synchronize-db.yml
@@ -19,4 +19,4 @@ jobs:
run: |
pip install pymongo
cd ivy
- python run_tests_CLI/synchronize_db.py ${{ secrets.MONGODB_PASSWORD }}
+ python scripts/setup_tests/synchronize_db.py ${{ secrets.MONGODB_PASSWORD }}
diff --git a/.github/workflows/test-array-api.yml b/.github/workflows/test-array-api.yml
index 2ba7d484492de..7db65e56a0379 100644
--- a/.github/workflows/test-array-api.yml
+++ b/.github/workflows/test-array-api.yml
@@ -55,7 +55,7 @@ jobs:
id: tests
run: |
cd ivy
- ./run_tests_CLI/test_array_api.sh ${{matrix.backends}} test_${{matrix.submodules}} ${{ secrets.REDIS_CONNECTION_URL }} ${{ secrets.REDIS_PASSWORD}}
+ ./scripts/shell/test_array_api.sh ${{matrix.backends}} test_${{matrix.submodules}} ${{ secrets.REDIS_CONNECTION_URL }} ${{ secrets.REDIS_PASSWORD}}
continue-on-error: true
- name: Zip Hypothesis Examples
diff --git a/.github/workflows/test-frontend-jax.yml b/.github/workflows/test-frontend-jax.yml
index 38b9944b9354e..7724876d33b7e 100644
--- a/.github/workflows/test-frontend-jax.yml
+++ b/.github/workflows/test-frontend-jax.yml
@@ -16,6 +16,15 @@ jobs:
persist-credentials: false
submodules: "recursive"
+ - name: Install ivy and fetch binaries
+ run: |
+ cd ivy
+ pip3 install -e .
+ mkdir .ivy
+ touch .ivy/key.pem
+ echo -n ${{ secrets.USER_API_KEY }} > .ivy/key.pem
+ cd ..
+
- name: Download artifact
if: github.event_name == 'pull_request'
uses: dawidd6/action-download-artifact@v2
diff --git a/.github/workflows/test-frontend-numpy.yml b/.github/workflows/test-frontend-numpy.yml
index 1a5a8f9bd75d6..d99000c459b95 100644
--- a/.github/workflows/test-frontend-numpy.yml
+++ b/.github/workflows/test-frontend-numpy.yml
@@ -16,6 +16,15 @@ jobs:
persist-credentials: false
submodules: "recursive"
+ - name: Install ivy and fetch binaries
+ run: |
+ cd ivy
+ pip3 install -e .
+ mkdir .ivy
+ touch .ivy/key.pem
+ echo -n ${{ secrets.USER_API_KEY }} > .ivy/key.pem
+ cd ..
+
- name: Download artifact
if: github.event_name == 'pull_request'
uses: dawidd6/action-download-artifact@v2
diff --git a/.github/workflows/test-frontend-tensorflow.yml b/.github/workflows/test-frontend-tensorflow.yml
index 52ae4ca44d9ef..f5a03dcef7e1a 100644
--- a/.github/workflows/test-frontend-tensorflow.yml
+++ b/.github/workflows/test-frontend-tensorflow.yml
@@ -16,6 +16,15 @@ jobs:
persist-credentials: false
submodules: "recursive"
+ - name: Install ivy and fetch binaries
+ run: |
+ cd ivy
+ pip3 install -e .
+ mkdir .ivy
+ touch .ivy/key.pem
+ echo -n ${{ secrets.USER_API_KEY }} > .ivy/key.pem
+ cd ..
+
- name: Download artifact
if: github.event_name == 'pull_request'
uses: dawidd6/action-download-artifact@v2
diff --git a/.github/workflows/test-frontend-torch.yml b/.github/workflows/test-frontend-torch.yml
index 6adeb7623a187..732958fd972a4 100644
--- a/.github/workflows/test-frontend-torch.yml
+++ b/.github/workflows/test-frontend-torch.yml
@@ -16,6 +16,15 @@ jobs:
persist-credentials: false
submodules: "recursive"
+ - name: Install ivy and fetch binaries
+ run: |
+ cd ivy
+ pip3 install -e .
+ mkdir .ivy
+ touch .ivy/key.pem
+ echo -n ${{ secrets.USER_API_KEY }} > .ivy/key.pem
+ cd ..
+
- name: Download artifact
if: github.event_name == 'pull_request'
uses: dawidd6/action-download-artifact@v2
diff --git a/.github/workflows/test-ivy-core.yml b/.github/workflows/test-ivy-core.yml
index 1a9d8a0f77956..f87a42a0abb43 100644
--- a/.github/workflows/test-ivy-core.yml
+++ b/.github/workflows/test-ivy-core.yml
@@ -27,6 +27,15 @@ jobs:
submodules: "recursive"
fetch-depth: 2
+ - name: Install ivy and fetch binaries
+ run: |
+ cd ivy
+ pip3 install -e .
+ mkdir .ivy
+ touch .ivy/key.pem
+ echo -n ${{ secrets.USER_API_KEY }} > .ivy/key.pem
+ cd ..
+
- name: Check Files Changed
if: ${{(github.event_name == 'push') || !contains(github.event.pull_request.labels.*.name, 'Exhaustive CI') }}
shell: pwsh
@@ -81,10 +90,7 @@ jobs:
if: steps.check_file_changed.outputs.changed == 'True' || steps.check_file_changed.conclusion == 'skipped'
run: |
cd ivy
- mkdir .ivy
- touch .ivy/key.pem
- echo -n ${{ secrets.USER_API_KEY }} > .ivy/key.pem
- ./run_tests_CLI/test_ivy_core.sh ${{ matrix.backends }} test_${{ matrix.submodules }} ${{ secrets.REDIS_CONNECTION_URL }} ${{ secrets.REDIS_PASSWORD}}
+ ./scripts/shell/test_ivy_core.sh ${{ matrix.backends }} test_${{ matrix.submodules }} ${{ secrets.REDIS_CONNECTION_URL }} ${{ secrets.REDIS_PASSWORD}}
continue-on-error: true
- name: Zip Hypothesis Examples
diff --git a/.github/workflows/test-ivy-cron-gpu.yml b/.github/workflows/test-ivy-cron-gpu.yml
index 83bc87e932cb8..fd1c3b592a06b 100644
--- a/.github/workflows/test-ivy-cron-gpu.yml
+++ b/.github/workflows/test-ivy-cron-gpu.yml
@@ -21,6 +21,15 @@ jobs:
submodules: "recursive"
set-safe-directory: false
+ - name: Install ivy and fetch binaries
+ run: |
+ cd ivy
+ pip3 install -e .
+ mkdir .ivy
+ touch .ivy/key.pem
+ echo -n ${{ secrets.USER_API_KEY }} > .ivy/key.pem
+ cd ..
+
- name: Get Job URL
uses: Tiryoh/gha-jobid-action@v0
id: jobs
@@ -33,11 +42,8 @@ jobs:
run: |
pip3 install pymongo
cd ivy
- mkdir .ivy
- touch .ivy/key.pem
- echo -n ${{ secrets.USER_API_KEY }} > .ivy/key.pem
- python3 run_tests_CLI/cron_tests.py ${{ github.run_number }}
- python3 run_tests.py ${{ secrets.REDIS_CONNECTION_URL }} ${{ secrets.REDIS_PASSWORD }} ${{ secrets.MONGODB_PASSWORD }} 'false' 'true' ${{ github.run_id }} 'false' ${{ steps.jobs.outputs.html_url }}
+ python3 scripts/setup_tests/cron_tests.py ${{ github.run_number }}
+ python3 scripts/run_tests/run_tests.py ${{ secrets.REDIS_CONNECTION_URL }} ${{ secrets.REDIS_PASSWORD }} ${{ secrets.MONGODB_PASSWORD }} 'false' 'true' ${{ github.run_id }} 'false' ${{ steps.jobs.outputs.html_url }}
continue-on-error: true
- name: Check on failures
diff --git a/.github/workflows/test-ivy-cron-multi-version.yml b/.github/workflows/test-ivy-cron-multi-version.yml
index 32a9560672ee5..fd36dd585d175 100644
--- a/.github/workflows/test-ivy-cron-multi-version.yml
+++ b/.github/workflows/test-ivy-cron-multi-version.yml
@@ -16,6 +16,15 @@ jobs:
persist-credentials: false
submodules: "recursive"
+ - name: Install ivy and fetch binaries
+ run: |
+ cd ivy
+ pip3 install -e .
+ mkdir .ivy
+ touch .ivy/key.pem
+ echo -n ${{ secrets.USER_API_KEY }} > .ivy/key.pem
+ cd ..
+
- name: Get Job URL
uses: Tiryoh/gha-jobid-action@v0
id: jobs
@@ -28,8 +37,8 @@ jobs:
run: |
cd ivy
pip3 install pymongo
- python run_tests_CLI/cron_tests_multi_version.py ${{ github.run_number }}
- python run_tests.py ${{ secrets.REDIS_CONNECTION_URL }} ${{ secrets.REDIS_PASSWORD }} ${{ secrets.MONGODB_PASSWORD }} 'true' 'false' ${{ github.run_id }} 'false' ${{ steps.jobs.outputs.html_url }}
+ python scripts/setup_tests/cron_tests_multi_version.py ${{ github.run_number }}
+ python scripts/run_tests/run_tests.py ${{ secrets.REDIS_CONNECTION_URL }} ${{ secrets.REDIS_PASSWORD }} ${{ secrets.MONGODB_PASSWORD }} 'true' 'false' ${{ github.run_id }} 'false' ${{ steps.jobs.outputs.html_url }}
continue-on-error: true
- name: Check on failures
diff --git a/.github/workflows/test-ivy-cron.yml b/.github/workflows/test-ivy-cron.yml
index 61476937d1259..c5ca96ab9dbfb 100644
--- a/.github/workflows/test-ivy-cron.yml
+++ b/.github/workflows/test-ivy-cron.yml
@@ -16,6 +16,15 @@ jobs:
persist-credentials: false
submodules: "recursive"
+ - name: Install ivy and fetch binaries
+ run: |
+ cd ivy
+ pip3 install -e .
+ mkdir .ivy
+ touch .ivy/key.pem
+ echo -n ${{ secrets.USER_API_KEY }} > .ivy/key.pem
+ cd ..
+
- name: Get Job URL
uses: Tiryoh/gha-jobid-action@v0
id: jobs
@@ -27,12 +36,9 @@ jobs:
id: tests
run: |
cd ivy
- mkdir .ivy
- touch .ivy/key.pem
- echo -n ${{ secrets.USER_API_KEY }} > .ivy/key.pem
pip3 install pymongo
- python run_tests_CLI/cron_tests.py ${{ github.run_number }}
- python run_tests.py ${{ secrets.REDIS_CONNECTION_URL }} ${{ secrets.REDIS_PASSWORD }} ${{ secrets.MONGODB_PASSWORD }} 'false' 'false' ${{ github.run_id }} 'false' ${{ steps.jobs.outputs.html_url }}
+ python scripts/setup_tests/cron_tests.py ${{ github.run_number }}
+ python scripts/run_tests/run_tests.py ${{ secrets.REDIS_CONNECTION_URL }} ${{ secrets.REDIS_PASSWORD }} ${{ secrets.MONGODB_PASSWORD }} 'false' 'false' ${{ github.run_id }} 'false' ${{ steps.jobs.outputs.html_url }}
continue-on-error: true
- name: Check on failures
diff --git a/.github/workflows/test-ivy-experimental-core.yml b/.github/workflows/test-ivy-experimental-core.yml
index 70062672504b2..8f8117978b2f5 100644
--- a/.github/workflows/test-ivy-experimental-core.yml
+++ b/.github/workflows/test-ivy-experimental-core.yml
@@ -26,6 +26,15 @@ jobs:
submodules: "recursive"
fetch-depth: 2
+ - name: Install ivy and fetch binaries
+ run: |
+ cd ivy
+ pip3 install -e .
+ mkdir .ivy
+ touch .ivy/key.pem
+ echo -n ${{ secrets.USER_API_KEY }} > .ivy/key.pem
+ cd ..
+
- name: Check Files Changed
shell: pwsh
id: check_file_changed
@@ -73,10 +82,7 @@ jobs:
id: tests
run: |
cd ivy
- mkdir .ivy
- touch .ivy/key.pem
- echo -n ${{ secrets.USER_API_KEY }} > .ivy/key.pem
- ./run_tests_CLI/test_experimental_core.sh ${{ matrix.backends }} test_${{ matrix.submodules }} ${{ secrets.REDIS_CONNECTION_URL }} ${{ secrets.REDIS_PASSWORD}}
+ ./scripts/shell/test_experimental_core.sh ${{ matrix.backends }} test_${{ matrix.submodules }} ${{ secrets.REDIS_CONNECTION_URL }} ${{ secrets.REDIS_PASSWORD}}
continue-on-error: true
- name: Zip Hypothesis Examples
diff --git a/.github/workflows/test-ivy-experimental-nn.yml b/.github/workflows/test-ivy-experimental-nn.yml
index 6fa4f2db7c0ca..ef5ebe1c294b1 100644
--- a/.github/workflows/test-ivy-experimental-nn.yml
+++ b/.github/workflows/test-ivy-experimental-nn.yml
@@ -24,6 +24,15 @@ jobs:
submodules: "recursive"
fetch-depth: 2
+ - name: Install ivy and fetch binaries
+ run: |
+ cd ivy
+ pip3 install -e .
+ mkdir .ivy
+ touch .ivy/key.pem
+ echo -n ${{ secrets.USER_API_KEY }} > .ivy/key.pem
+ cd ..
+
- name: Check Files Changed
shell: pwsh
id: check_file_changed
@@ -71,10 +80,7 @@ jobs:
id: tests
run: |
cd ivy
- mkdir .ivy
- touch .ivy/key.pem
- echo -n ${{ secrets.USER_API_KEY }} > .ivy/key.pem
- ./run_tests_CLI/test_experimental_nn.sh ${{ matrix.backends }} test_${{ matrix.submodules }} ${{ secrets.REDIS_CONNECTION_URL }} ${{ secrets.REDIS_PASSWORD}}
+ ./scripts/shell/test_experimental_nn.sh ${{ matrix.backends }} test_${{ matrix.submodules }} ${{ secrets.REDIS_CONNECTION_URL }} ${{ secrets.REDIS_PASSWORD}}
continue-on-error: true
- name: Zip Hypothesis Examples
diff --git a/.github/workflows/test-ivy-nn.yml b/.github/workflows/test-ivy-nn.yml
index 3a7898b889e5d..9f213e1e013bd 100644
--- a/.github/workflows/test-ivy-nn.yml
+++ b/.github/workflows/test-ivy-nn.yml
@@ -24,6 +24,15 @@ jobs:
submodules: "recursive"
fetch-depth: 2
+ - name: Install ivy and fetch binaries
+ run: |
+ cd ivy
+ pip3 install -e .
+ mkdir .ivy
+ touch .ivy/key.pem
+ echo -n ${{ secrets.USER_API_KEY }} > .ivy/key.pem
+ cd ..
+
- name: Check Files Changed
if: ${{ (github.event_name == 'push') || !contains(github.event.pull_request.labels.*.name, 'Exhaustive CI') }}
shell: pwsh
@@ -78,10 +87,7 @@ jobs:
if: steps.check_file_changed.outputs.changed == 'True' || steps.check_file_changed.conclusion == 'skipped'
run: |
cd ivy
- mkdir .ivy
- touch .ivy/key.pem
- echo -n ${{ secrets.USER_API_KEY }} > .ivy/key.pem
- ./run_tests_CLI/test_ivy_nn.sh ${{ matrix.backends }} test_${{ matrix.submodules }} ${{ secrets.REDIS_CONNECTION_URL }} ${{ secrets.REDIS_PASSWORD}}
+ ./scripts/shell/test_ivy_nn.sh ${{ matrix.backends }} test_${{ matrix.submodules }} ${{ secrets.REDIS_CONNECTION_URL }} ${{ secrets.REDIS_PASSWORD}}
continue-on-error: true
- name: Zip Hypothesis Examples
diff --git a/.github/workflows/test-ivy-stateful.yml b/.github/workflows/test-ivy-stateful.yml
index b51dbe2a7fabd..c4aeeb690f0b4 100644
--- a/.github/workflows/test-ivy-stateful.yml
+++ b/.github/workflows/test-ivy-stateful.yml
@@ -25,6 +25,15 @@ jobs:
submodules: "recursive"
fetch-depth: 2
+ - name: Install ivy and fetch binaries
+ run: |
+ cd ivy
+ pip3 install -e .
+ mkdir .ivy
+ touch .ivy/key.pem
+ echo -n ${{ secrets.USER_API_KEY }} > .ivy/key.pem
+ cd ..
+
- name: Check Files Changed
if: ${{ (github.event_name == 'push') || !contains(github.event.pull_request.labels.*.name, 'Exhaustive CI') }}
shell: pwsh
@@ -80,10 +89,7 @@ jobs:
if: steps.check_file_changed.outputs.changed == 'True' || steps.check_file_changed.conclusion == 'skipped'
run: |
cd ivy
- mkdir .ivy
- touch .ivy/key.pem
- echo -n ${{ secrets.USER_API_KEY }} > .ivy/key.pem
- ./run_tests_CLI/test_ivy_stateful.sh ${{ matrix.backends }} test_${{ matrix.submodules }} ${{ secrets.REDIS_CONNECTION_URL }} ${{ secrets.REDIS_PASSWORD}}
+ ./scripts/shell/test_ivy_stateful.sh ${{ matrix.backends }} test_${{ matrix.submodules }} ${{ secrets.REDIS_CONNECTION_URL }} ${{ secrets.REDIS_PASSWORD}}
continue-on-error: true
- name: Zip Hypothesis Examples
diff --git a/.github/workflows/welcome-message.yml b/.github/workflows/welcome-message.yml
index 2c2a8e0f037ad..a41cb471ed830 100644
--- a/.github/workflows/welcome-message.yml
+++ b/.github/workflows/welcome-message.yml
@@ -43,13 +43,14 @@ jobs:
repo-token: ${{ secrets.GITHUB_TOKEN }}
pr-message: |-
Congrats on making your first Pull Request and thanks for supporting Ivy! π
- Join the conversation in our [Discord](https://discord.com/invite/sXyFF8tDtm)
-
- Here are some notes to understand our tests:
- - We have merged all the tests in one file called \`display_test_results\` job. π It contains the following two sections:
- - **Combined Test Results:** This shows the results of all the ivy tests that ran on the PR. βοΈ
- - **New Failures Introduced:** This lists the tests that fails on this PR.
+ Join the conversation in our [Discord](https://discord.com/invite/sXyFF8tDtm).
+ For every PR opened, we run unit tests and comment the results in the PR to ensure the functionality remains intact.
Please make sure they are passing. πͺ
- Keep in mind that we will assign an engineer for this task and they will look at it based on the workload that they have, kindly be patient π.
+ If you want to check them from the action runners, you can open the `display_test_results` job. π
+ It contains the following two sections:
+ - **Combined Test Results:** This shows the results of all the ivy tests that ran on the PR. βοΈ
+ - **New Failures Introduced:** This lists the tests that fail on this PR.
+
+ Keep in mind that we will assign an engineer for this task and they will look at it based on the workload that they have, **kindly be patient π**.
diff --git a/.pre-commit-config.yaml b/.pre-commit-config.yaml
index 13bbede423d82..c58337243a79e 100644
--- a/.pre-commit-config.yaml
+++ b/.pre-commit-config.yaml
@@ -1,13 +1,13 @@
repos:
- repo: https://github.com/pre-commit/pre-commit-hooks
- rev: v4.4.0
+ rev: v4.5.0
hooks:
- id: check-yaml
- id: trailing-whitespace
- id: check-toml
- id: end-of-file-fixer
- repo: https://github.com/psf/black-pre-commit-mirror
- rev: 23.9.1
+ rev: 23.10.1
hooks:
- id: black
language_version: python3
@@ -32,11 +32,6 @@ repos:
- id: pydocstyle
# Exclude everything in frontends except __init__.py, and func_wrapper.py
exclude: 'ivy/functional/(frontends|backends)/(?!.*/func_wrapper\.py$).*(?!__init__\.py$)'
- - repo: https://github.com/asottile/pyupgrade
- rev: v3.13.0
- hooks:
- - id: pyupgrade
- args: [--py38-plus]
- repo: https://github.com/unifyai/lint-hook
rev: 2ea80bc854c7f74b09620151028579083ff92ec2
hooks:
diff --git a/CITATION.cff b/CITATION.cff
index a89af71e90500..7472e9bf6da27 100644
--- a/CITATION.cff
+++ b/CITATION.cff
@@ -39,5 +39,4 @@ preferred-citation:
- given-names: Ronald
family-names: Clark
doi: 10.48550/arXiv.2102.02886
- title: "Ivy: Templated deep learning for inter-framework
- portability"
+ title: "Ivy: Templated deep learning for inter-framework portability"
diff --git a/CONTRIBUTING.md b/CONTRIBUTING.md
index 3f92bf448b784..89d573f39917f 100644
--- a/CONTRIBUTING.md
+++ b/CONTRIBUTING.md
@@ -4,13 +4,13 @@ You can pick an open issue to contribute from our [ToDo list issues](https://git
Please, follow the next process when you work on your subtask:
-## Steps:
+## Steps
1. **Choosing a Task:**
- Choose a task to work on which:
- - is not marked as completed with a tick
- - does not have an issue created
+ - is not marked as completed with a tick.
+ - does not have an issue created.
- is not mentioned in the comments.
Currently, there are three open tasks:
@@ -37,9 +37,9 @@ Please, follow the next process when you work on your subtask:
- Every time you respond to our requested changes you must re-request a review in order for us to re-engage with the PR.
- Once the PR is in good shape, we will merge into main, and then you become an Ivy contributor!
-### Important Notes:
+### Important Notes
-- if your PR is not created within 7 days of creating the issue, then a warning message will appear on the issue, we do this in order to keep our ToDo lists moving quickly,
+- If your PR is not created within 7 days of creating the issue, then a warning message will appear on the issue, we do this in order to keep our ToDo lists moving quickly,
- Please don't take it personally if your issue or PR gets closed because of this 7-day inactivity time limit.
- Finally, we limit the maximum number of open and incomplete sub-task issues to three per person.
diff --git a/MANIFEST.in b/MANIFEST.in
index 7ac5320132b47..e0a363d10c6ec 100644
--- a/MANIFEST.in
+++ b/MANIFEST.in
@@ -2,3 +2,5 @@ include requirements/requirements.txt
include ivy/compiler/utils/*.so
include ivy/compiler/*.so
include ivy/compiler/*.py
+include binaries.json
+include available_configs.json
diff --git a/README.md b/README.md
index e4ce1c4e04438..91ae3f63899a5 100644
--- a/README.md
+++ b/README.md
@@ -1,1598 +1,1621 @@
-> π We are granting pilot access to **Ivy\'s Compiler and Transpiler**
-> to some users, [join the waitlist](https://console.unify.ai/) if you
-> want to test them out!
-
-
-
-
-
-------------------------------------------------------------------------
-
-
-
-------------------------------------------------------------------------
-
-# Status
-
-
-
-
-------------------------------------------------------------------------
-
-# Unified AI
-
-
-
-
-
-------------------------------------------------------------------------
-
-Ivy is both an ML transpiler and a framework, currently supporting JAX,
-TensorFlow, PyTorch, and Numpy.
-
-Ivy unifies all ML frameworks π₯ enabling you not only to **write code
-that can be used with any of these frameworks as the backend**, but also
-to **convert π any function, model, or library written in any of them to
-your preferred framework!**
-
-You can check out [Ivy as a transpiler](#ivy-as-a-transpiler) and [Ivy
-as a framework](#ivy-as-a-framework) to learn more about this, try out
-Ivy straight away going through the [Setting up Ivy](#setting-up-ivy)
-section, or dive deep into Ivy\'s [Documentation](#documentation) and
-[Examples](#examples)!
-
-If you would like to contribute, you can join our growing
-[Community](#community) π, check out our [Contributing](#contributing)
-guide, and take a look at the [open
-tasks](https://unify.ai/docs/ivy/overview/contributing/open_tasks.html)
-if you\'d like to dive straight in π§βπ»
-
-**Let\'s** [unify.ai](https://unify.ai) **together π¦Ύ**
-
-------------------------------------------------------------------------
-
-## Ivy as a transpiler
-
-Ivy\'s transpiler allows you to use code from any other framework (or
-from any other version of the same framework!) in your own code, by just
-adding one line of code. Under the hood, Ivy traces a computational
-graph and leverages the frontends and backends to link one framework to
-another.
-
-This way, Ivy makes all ML-related projects available for you,
-independently of the framework you want to use to research, develop, or
-deploy systems. Feel free to head over to the docs for the full API
-reference, but the functions you\'d most likely want to use are:
-
-``` python
-# Compiles a function into an efficient fully-functional graph, removing all wrapping and redundant code
-ivy.compile()
-
-# Converts framework-specific code to a different framework
-ivy.transpile()
-
-# Converts framework-specific code to Ivy
-ivy.unify()
-```
-
-These functions can be used eagerly or lazily. If you pass the necessary
-arguments for function tracing, the compilation/transpilation step will
-happen instantly (eagerly). Otherwise, the compilation/transpilation
-will happen only when the returned function is first invoked.
-
-``` python
-import ivy
-import jax
-ivy.set_backend("jax")
-
-# Simple JAX function to transpile
-def test_fn(x):
- return jax.numpy.sum(x)
-
-x1 = ivy.array([1., 2.])
-```
-
-``` python
-# Arguments are available -> transpilation happens eagerly
-eager_graph = ivy.transpile(test_fn, source="jax", to="torch", args=(x1,))
-
-# eager_graph is now torch code and runs efficiently
-ret = eager_graph(x1)
-```
-
-``` python
-# Arguments are not available -> transpilation happens lazily
-lazy_graph = ivy.transpile(test_fn, source="jax", to="torch")
-
-# The transpiled graph is initialized, transpilation will happen here
-ret = lazy_graph(x1)
-
-# lazy_graph is now torch code and runs efficiently
-ret = lazy_graph(x1)
-```
-
-If you want to learn more, you can find more information in the [Ivy as
-a transpiler section of the
-docs!](https://unify.ai/docs/ivy/overview/design/ivy_as_a_transpiler.html)
-
-### When should I use Ivy as a transpiler?
-
-If you want to use building blocks published in other frameworks (neural
-networks, layers, array computing libraries, training pipelines\...),
-you want to integrate code developed in various frameworks, or maybe
-straight up move code from one framework to another, the transpiler is
-definitely the tool π§ for the job! As the output of transpilation is
-native code in the target framework, you can use the converted code just
-as if it was code originally developed in that framework, applying
-framework-specific optimizations or tools, instantly exposing your
-project to all of the unique perks of a different framework.
-
-## Ivy as a framework
-
-The Ivy framework is built on top of various essential components,
-mainly the [Backend
-Handler](https://unify.ai/docs/ivy/overview/design/building_blocks.html#backend-handler),
-which manages what framework is being used behind the scenes and the
-[Backend Functional
-APIs](https://unify.ai/docs/ivy/overview/design/building_blocks.html#backend-functional-apis),
-which provide framework-specific implementations of the Ivy functions.
-Likewise, classes such as `ivy.Container` or `ivy.Array` are also
-available, facilitating the use of structured data and array-like
-objects (learn more about them
-[here!](https://unify.ai/docs/ivy/overview/design/ivy_as_a_framework.html)).
-
-All of the functionalities in Ivy are exposed through the
-`Ivy functional API` and the `Ivy stateful API`. All functions in the
-[Functional
-API](https://unify.ai/docs/ivy/overview/design/building_blocks.html#ivy-functional-api)
-are **Framework Agnostic Functions**, which means that we can use them
-like this:
-
-``` python
-import ivy
-import jax.numpy as jnp
-import tensorflow as tf
-import numpy as np
-import torch
-
-def mse_loss(y, target):
- return ivy.mean((y - target)**2)
-
-jax_mse = mse_loss(jnp.ones((5,)), jnp.ones((5,)))
-tf_mse = mse_loss(tf.ones((5,)), tf.ones((5,)))
-np_mse = mse_loss(np.ones((5,)), np.ones((5,)))
-torch_mse = mse_loss(torch.ones((5,)), torch.ones((5,)))
-```
-
-In the example above we show how Ivy\'s functions are compatible with
-tensors from different frameworks. This is the same for ALL Ivy
-functions. They can accept tensors from any framework and return the
-correct result.
-
-The [Ivy Stateful
-API](https://unify.ai/docs/ivy/overview/design/ivy_as_a_framework/ivy_stateful_api.html),
-on the other hand, allows you to define trainable modules and layers,
-which you can use alone or as a part of any other framework code!
-
-``` python
-import ivy
-
-
-class Regressor(ivy.Module):
- def __init__(self, input_dim, output_dim):
- self.input_dim = input_dim
- self.output_dim = output_dim
- super().__init__()
-
- def _build(self, *args, **kwargs):
- self.linear0 = ivy.Linear(self.input_dim, 128)
- self.linear1 = ivy.Linear(128, self.output_dim)
-
- def _forward(self, x):
- x = self.linear0(x)
- x = ivy.functional.relu(x)
- x = self.linear1(x)
- return x
-```
-
-If we put it all together, we\'ll have something like this. This example
-uses PyTorch as the backend, but this can easily be changed to your
-favorite frameworks, such as TensorFlow, or JAX.
-
-``` python
-import ivy
-
-
-class Regressor(ivy.Module):
- def __init__(self, input_dim, output_dim):
- self.input_dim = input_dim
- self.output_dim = output_dim
- super().__init__()
-
- def _build(self, *args, **kwargs):
- self.linear0 = ivy.Linear(self.input_dim, 128)
- self.linear1 = ivy.Linear(128, self.output_dim)
-
- def _forward(self, x):
- x = self.linear0(x)
- x = ivy.functional.relu(x)
- x = self.linear1(x)
- return x
-
-ivy.set_backend('torch') # set backend to PyTorch (or any other backend!)
-
-model = Regressor(input_dim=1, output_dim=1)
-optimizer = ivy.Adam(0.3)
-
-n_training_examples = 2000
-noise = ivy.random.random_normal(shape=(n_training_examples, 1), mean=0, std=0.1)
-x = ivy.linspace(-6, 3, n_training_examples).reshape((n_training_examples, 1))
-y = 0.2 * x ** 2 + 0.5 * x + 0.1 + noise
-
-
-def loss_fn(v, x, target):
- pred = model(x, v=v)
- return ivy.mean((pred - target) ** 2)
-
-for epoch in range(40):
- # forward pass
- pred = model(x)
-
- # compute loss and gradients
- loss, grads = ivy.execute_with_gradients(lambda params: loss_fn(*params), (model.v, x, y))
-
- # update parameters
- model.v = optimizer.step(model.v, grads)
-
- # print current loss
- print(f'Epoch: {epoch + 1:2d} --- Loss: {ivy.to_numpy(loss).item():.5f}')
-
-print('Finished training!')
-```
-
-The model\'s output can be visualized as follows:
-
-
-
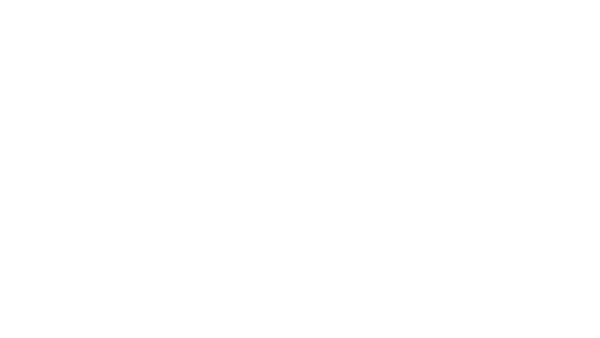
-
-
-
-As always, you can find more information about [Ivy as a framework in
-the
-docs!](https://unify.ai/docs/ivy/overview/design/ivy_as_a_framework.html)
-
-### When should I use Ivy as a framework?
-
-As Ivy supports multiple backends, writing code in Ivy breaks you free
-from framework limitations. If you want to publish highly flexible code
-for everyone to use, independently of the framework they are using, or
-you plan to develop ML-related tools and want them to be interoperable
-with not only the already existing frameworks, but also with future
-frameworks, then Ivy is for you!
-
-## Setting up Ivy
-
-There are various ways to use Ivy, depending on your preferred
-environment:
-
-### Installing using pip
-
-The easiest way to set up Ivy is to install it using pip with the
-following command:
-
-``` bash
-pip install ivy
-```
-
-or alternatively:
-
-``` bash
-python3 -m pip install ivy
-```
-
-### Docker
-
-If you prefer to use containers, we also have pre-built Docker images
-with all the supported frameworks and some relevant packages already
-installed, which you can pull from:
-
-``` bash
-docker pull unifyai/ivy:latest
-```
-
-If you are working on a GPU device, you can pull from:
-
-``` bash
-docker pull unifyai/ivy:latest-gpu
-```
-
-### Installing from source
-
-You can also install Ivy from source if you want to take advantage of
-the latest changes, but we can\'t ensure everything will work as
-expected. :sweat_smile:
-
-``` bash
-git clone https://github.com/unifyai/ivy.git
-cd ivy
-pip install --user -e .
-```
-
-or alternatively, for the last step:
-
-``` bash
-python3 -m pip install --user -e .
-```
-
-If you want to set up testing and various frameworks it\'s probably best
-to check out the [Contributing - Setting
-Up](https://unify.ai/docs/ivy/overview/contributing/setting_up.html#setting-up)
-page, where OS-specific and IDE-specific instructions and video
-tutorials to do so are available!
-
-### Using Ivy
-
-You can find quite a lot more examples in the corresponding section
-below, but using Ivy is as simple as:
-
-#### Multi-backend Support
-
-``` python
-import ivy
-import torch
-import jax
-
-ivy.set_backend("jax")
-
-x = jax.numpy.array([1, 2, 3])
-y = jax.numpy.array([3, 2, 1])
-z = ivy.add(x, y)
-
-ivy.set_backend('torch')
-
-x = torch.tensor([1, 2, 3])
-y = torch.tensor([3, 2, 1])
-z = ivy.add(x, y)
-```
-
-#### Transpilation API
-
-``` python
-import ivy
-import torch
-import jax
-
-def jax_fn(x):
- a = jax.numpy.dot(x, x)
- b = jax.numpy.mean(x)
- return x * a + b
-
-jax_x = jax.numpy.array([1, 2, 3])
-torch_x = torch.tensor([1, 2, 3])
-torch_fn = ivy.transpile(jax_fn, source="jax", to="torch", args=(jax_x,))
-ret = torch_fn(torch_x)
-```
-
-## Documentation
-
-The [Ivy Docs page](https://unify.ai/docs/ivy/) holds all the relevant
-information about Ivy and its framework API reference.
-
-There, you will find the
-[Design](https://unify.ai/docs/ivy/overview/design.html) page, which is
-a user-focused guide about the architecture and the building blocks of
-Ivy. Likewise, you can take a look at the [Deep
-dive](https://unify.ai/docs/ivy/overview/deep_dive.html), which is
-oriented towards potential contributors of the code base and explains
-the nuances of Ivy in full detail π
-
-Another important sections of the docs is
-[Background](https://unify.ai/docs/ivy/overview/background.html), which
-contextualises the problem Ivy is trying to solve and the current [ML
-Explosion](https://unify.ai/docs/ivy/overview/background/ml_explosion.html#ml-explosion),
-explaining both (1) why is important [to solve this
-problem](https://unify.ai/docs/ivy/overview/background/why_unify.html#why-unify)
-and (2) how we are adhering to existing
-[standards](https://unify.ai/docs/ivy/overview/background/standardization.html#standardization)
-to make this happen.
-
-Lastly, you can also find there the [Related
-Work](https://unify.ai/docs/ivy/overview/related_work.html) section,
-which paints a clear picture of the role Ivy plays in the ML stack,
-comparing it to other existing solutions in terms of functionalities and
-level.
-
-## Examples
-
-The [Examples page](https://unify.ai/demos/) features a wide range of
-demos and tutorials showcasing the functionalities of Ivy along with
-multiple use cases, but feel free to check out some shorter
-framework-specific examples here β¬οΈ
-
-
-I'm using PyTorch 
- You can use Ivy to get PyTorch code from:
-
- Any model
-
-
- From TensorFlow
-
-``` python
-import ivy
-import torch
-import tensorflow as tf
-
-# Get a pretrained keras model
-eff_encoder = tf.keras.applications.efficientnet_v2.EfficientNetV2B0(
- include_top=False, weights="imagenet", input_shape=(224, 224, 3)
-)
-
-# Transpile it into a torch.nn.Module with the corresponding parameters
-noise = tf.random.normal(shape=(1, 224, 224, 3))
-torch_eff_encoder = ivy.transpile(eff_encoder, to="torch", args=(noise,))
-
-# Build a classifier using the transpiled encoder
-class Classifier(torch.nn.Module):
- def __init__(self, num_classes=20):
- super(Classifier, self).__init__()
- self.encoder = torch_eff_encoder
- self.fc = torch.nn.Linear(1280, num_classes)
-
- def forward(self, x):
- x = self.encoder(x)
- return self.fc(x)
-
-# Initialize a trainable, customizable, torch.nn.Module
-classifier = Classifier()
-ret = classifier(torch.rand((1, 244, 244, 3)))
-```
-
-
-
- From JAX
-
-``` python
-import ivy
-import jax
-import torch
-
-# Get a pretrained haiku model
-# https://unify.ai/demos/scripts/deepmind_perceiver_io.py
-from deepmind_perceiver_io import key, perceiver_backbone
-
-# Transpile it into a torch.nn.Module with the corresponding parameters
-dummy_input = jax.random.uniform(key, shape=(1, 3, 224, 224))
-params = perceiver_backbone.init(rng=key, images=dummy_input)
-backbone = ivy.transpile(
- perceiver_backbone, to="torch", params_v=params, kwargs={"images": dummy_input}
-)
-
-# Build a classifier using the transpiled backbone
-class PerceiverIOClassifier(torch.nn.Module):
- def __init__(self, num_classes=20):
- super(PerceiverIOClassifier, self).__init__()
- self.backbone = backbone
- self.max_pool = torch.nn.MaxPool2d((512, 1))
- self.flatten = torch.nn.Flatten()
- self.fc = torch.nn.Linear(1024, num_classes)
-
- def forward(self, x):
- x = self.backbone(images=x)
- x = self.flatten(self.max_pool(x))
- return self.fc(x)
-
-# Initialize a trainable, customizable, torch.nn.Module
-classifier = PerceiverIOClassifier()
-ret = classifier(torch.rand((1, 3, 224, 224)))
-```
-
-
-
-
-
-
-Any library
-
-
- From Tensorflow
-
-``` python
-import ivy
-import torch
-import os
-os.environ["SM_FRAMEWORK"] = "tf.keras"
-import segmentation_models as sm
-
-# transpile sm from tensorflow to torch
-torch_sm = ivy.transpile(sm, source="tensorflow", to="torch")
-
-# get some image-like arrays
-output = torch.rand((1, 3, 512, 512))
-target = torch.rand((1, 3, 512, 512))
-
-# and use the transpiled version of any function from the library!
-out = torch_sm.metrics.iou_score(output, target)
-```
-
-
-
- From JAX
-
-``` python
-import ivy
-import rax
-import torch
-
-# transpile rax from jax to torch
-torch_rax = ivy.transpile(rax, source="jax", to="torch")
-
-# get some arrays
-scores = torch.tensor([2.2, 1.3, 5.4])
-labels = torch.tensor([1.0, 0.0, 0.0])
-
-# and use the transpiled version of any function from the library!
-out = torch_rax.poly1_softmax_loss(scores, labels)
-```
-
-
-
- From NumPy
-
-``` python
-import ivy
-import torch
-import madmom
-
-# transpile madmon from numpy to torch
-torch_madmom = ivy.transpile(madmom, source="numpy", to="torch")
-
-# get some arrays
-freqs = torch.arange(20) * 10
-
-# and use the transpiled version of any function from the library!
-out = torch_madmom.audio.filters.hz2midi(freqs)
-```
-
-
-
-
-
-
-Any function
-
-
- From Tensorflow
-
-``` python
-import ivy
-import tensorflow as tf
-import torch
-
-def loss(predictions, targets):
- return tf.sqrt(tf.reduce_mean(tf.square(predictions - targets)))
-
-# transpile any function from tf to torch
-torch_loss = ivy.transpile(loss, source="tensorflow", to="torch")
-
-# get some arrays
-p = torch.tensor([3.0, 2.0, 1.0])
-t = torch.tensor([0.0, 0.0, 0.0])
-
-# and use the transpiled version!
-out = torch_loss(p, t)
-```
-
-
-
- From JAX
-
-``` python
-import ivy
-import jax.numpy as jnp
-import torch
-
-def loss(predictions, targets):
- return jnp.sqrt(jnp.mean((predictions - targets) ** 2))
-
-# transpile any function from jax to torch
-torch_loss = ivy.transpile(loss, source="jax", to="torch")
-
-# get some arrays
-p = torch.tensor([3.0, 2.0, 1.0])
-t = torch.tensor([0.0, 0.0, 0.0])
-
-# and use the transpiled version!
-out = torch_loss(p, t)
-```
-
-
-
- From NumPy
-
-``` python
-import ivy
-import numpy as np
-import torch
-
-def loss(predictions, targets):
- return np.sqrt(np.mean((predictions - targets) ** 2))
-
-# transpile any function from numpy to torch
-torch_loss = ivy.transpile(loss, source="numpy", to="torch")
-
-# get some arrays
-p = torch.tensor([3.0, 2.0, 1.0])
-t = torch.tensor([0.0, 0.0, 0.0])
-
-# and use the transpiled version!
-out = torch_loss(p, t)
-```
-
-
-
-
-
-
-
-
-
-I'm using TensorFlow 
-You can use Ivy to get TensorFlow code from:
-
-Any model
-
-
- From PyTorch
-
-``` python
-import ivy
-import torch
-import timm
-import tensorflow as tf
-
-# Get a pretrained pytorch model
-mlp_encoder = timm.create_model("mixer_b16_224", pretrained=True, num_classes=0)
-
-# Transpile it into a keras.Model with the corresponding parameters
-noise = torch.randn(1, 3, 224, 224)
-mlp_encoder = ivy.transpile(mlp_encoder, to="tensorflow", args=(noise,))
-
-# Build a classifier using the transpiled encoder
-class Classifier(tf.keras.Model):
- def __init__(self):
- super(Classifier, self).__init__()
- self.encoder = mlp_encoder
- self.output_dense = tf.keras.layers.Dense(units=1000, activation="softmax")
-
- def call(self, x):
- x = self.encoder(x)
- return self.output_dense(x)
-
-# Transform the classifier and use it as a standard keras.Model
-x = tf.random.normal(shape=(1, 3, 224, 224))
-model = Classifier()
-ret = model(x)
-```
-
-
-
- From JAX
-
-``` python
-import ivy
-import jax
-import tensorflow as tf
-
-# Get a pretrained haiku model
-# https://unify.ai/demos/scripts/deepmind_perceiver_io.py
-from deepmind_perceiver_io import key, perceiver_backbone
-
-# Transpile it into a tf.keras.Model with the corresponding parameters
-dummy_input = jax.random.uniform(key, shape=(1, 3, 224, 224))
-params = perceiver_backbone.init(rng=key, images=dummy_input)
-backbone = ivy.transpile(
- perceiver_backbone, to="tensorflow", params_v=params, args=(dummy_input,)
-)
-
-# Build a classifier using the transpiled backbone
-class PerceiverIOClassifier(tf.keras.Model):
- def __init__(self, num_classes=20):
- super(PerceiverIOClassifier, self).__init__()
- self.backbone = backbone
- self.max_pool = tf.keras.layers.MaxPooling1D(pool_size=512)
- self.flatten = tf.keras.layers.Flatten()
- self.fc = tf.keras.layers.Dense(num_classes)
-
- def call(self, x):
- x = self.backbone(x)
- x = self.flatten(self.max_pool(x))
- return self.fc(x)
-
-# Initialize a trainable, customizable, tf.keras.Model
-x = tf.random.normal(shape=(1, 3, 224, 224))
-classifier = PerceiverIOClassifier()
-ret = classifier(x)
-```
-
-
-
-
-
-
-Any library
-
-
- From PyTorch
-
-``` python
-import ivy
-import kornia
-import requests
-import numpy as np
-import tensorflow as tf
-from PIL import Image
-
-# transpile kornia from torch to tensorflow
-tf_kornia = ivy.transpile(kornia, source="torch", to="tensorflow")
-
-# get an image
-url = "http://images.cocodataset.org/train2017/000000000034.jpg"
-raw_img = Image.open(requests.get(url, stream=True).raw)
-
-# convert it to the format expected by kornia
-img = np.array(raw_img)
-img = tf.transpose(tf.constant(img), (2, 0, 1))
-img = tf.expand_dims(img, 0) / 255
-
-# and use the transpiled version of any function from the library!
-out = tf_kornia.enhance.sharpness(img, 5)
-```
-
-
-
- From JAX
-
-``` python
-import ivy
-import rax
-import tensorflow as tf
-
-# transpile rax from jax to tensorflow
-tf_rax = ivy.transpile(rax, source="jax", to="tensorflow")
-
-# get some arrays
-scores = tf.constant([2.2, 1.3, 5.4])
-labels = tf.constant([1.0, 0.0, 0.0])
-
-# and use the transpiled version of any function from the library!
-out = tf_rax.poly1_softmax_loss(scores, labels)
-```
-
-
-
- From NumPy
-
-``` python
-import ivy
-import madmom
-import tensorflow as tf
-
-# transpile madmom from numpy to tensorflow
-tf_madmom = ivy.transpile(madmom, source="numpy", to="tensorflow")
-
-# get some arrays
-freqs = tf.range(20) * 10
-
-# and use the transpiled version of any function from the library!
-out = tf_madmom.audio.filters.hz2midi(freqs)
-```
-
-
-
-
-
-
-Any function
-
-
- From PyTorch
-
-``` python
-import ivy
-import torch
-import tensorflow as tf
-
-def loss(predictions, targets):
- return torch.sqrt(torch.mean((predictions - targets) ** 2))
-
-# transpile any function from torch to tensorflow
-tf_loss = ivy.transpile(loss, source="torch", to="tensorflow")
-
-# get some arrays
-p = tf.constant([3.0, 2.0, 1.0])
-t = tf.constant([0.0, 0.0, 0.0])
-
-# and use the transpiled version!
-out = tf_loss(p, t)
-```
-
-
-
- From JAX
-
-``` python
-import ivy
-import jax.numpy as jnp
-import tensorflow as tf
-
-def loss(predictions, targets):
- return jnp.sqrt(jnp.mean((predictions - targets) ** 2))
-
-# transpile any function from jax to tensorflow
-tf_loss = ivy.transpile(loss, source="jax", to="tensorflow")
-
-# get some arrays
-p = tf.constant([3.0, 2.0, 1.0])
-t = tf.constant([0.0, 0.0, 0.0])
-
-# and use the transpiled version!
-out = tf_loss(p, t)
-```
-
-
-
- From NumPy
-
-``` python
-import ivy
-import numpy as np
-import tensorflow as tf
-
-def loss(predictions, targets):
- return np.sqrt(np.mean((predictions - targets) ** 2))
-
-# transpile any function from numpy to tensorflow
-tf_loss = ivy.transpile(loss, source="numpy", to="tensorflow")
-
-# get some arrays
-p = tf.constant([3.0, 2.0, 1.0])
-t = tf.constant([0.0, 0.0, 0.0])
-
-# and use the transpiled version!
-out = tf_loss(p, t)
-```
-
-
-
-
-
-
-
-
-
-I'm using Jax 
-You can use Ivy to get JAX code from:
-
-Any model
-
-
- From PyTorch
-
-``` python
-import ivy
-import timm
-import torch
-import jax
-import haiku as hk
-
-# Get a pretrained pytorch model
-mlp_encoder = timm.create_model("mixer_b16_224", pretrained=True, num_classes=0)
-
-# Transpile it into a hk.Module with the corresponding parameters
-noise = torch.randn(1, 3, 224, 224)
-mlp_encoder = ivy.transpile(mlp_encoder, to="jax", args=(noise,))
-
-# Build a classifier using the transpiled encoder
-class Classifier(hk.Module):
- def __init__(self, num_classes=1000):
- super(Classifier, self).__init__()
- self.encoder = mlp_encoder()
- self.fc = hk.Linear(output_size=num_classes, with_bias=True)
-
- def __call__(self, x):
- x = self.encoder(x)
- x = self.fc(x)
- return x
-
-def _forward_classifier(x):
- module = Classifier()
- return module(x)
-
-# Transform the classifier and use it as a standard hk.Module
-rng_key = jax.random.PRNGKey(42)
-x = jax.random.uniform(key=rng_key, shape=(1, 3, 224, 224), dtype=jax.numpy.float32)
-forward_classifier = hk.transform(_forward_classifier)
-params = forward_classifier.init(rng=rng_key, x=x)
-
-ret = forward_classifier.apply(params, None, x)
-```
-
-
-
- From TensorFlow
-
-``` python
-import ivy
-import jax
-import haiku as hk
-import tensorflow as tf
-
-# Get a pretrained keras model
-eff_encoder = tf.keras.applications.efficientnet_v2.EfficientNetV2B0(
- include_top=False, weights="imagenet", input_shape=(224, 224, 3)
-)
-
-# Transpile it into a hk.Module with the corresponding parameters
-noise = tf.random.normal(shape=(1, 224, 224, 3))
-hk_eff_encoder = ivy.transpile(eff_encoder, to="jax", args=(noise,))
-
-# Build a classifier using the transpiled encoder
-class Classifier(hk.Module):
- def __init__(self, num_classes=1000):
- super(Classifier, self).__init__()
- self.encoder = hk_eff_encoder()
- self.fc = hk.Linear(output_size=num_classes, with_bias=True)
-
- def __call__(self, x):
- x = self.encoder(x)
- x = self.fc(x)
- return x
-
-def _forward_classifier(x):
- module = Classifier()
- return module(x)
-
-# Transform the classifier and use it as a standard hk.Module
-rng_key = jax.random.PRNGKey(42)
-dummy_x = jax.random.uniform(key=rng_key, shape=(1, 224, 224, 3))
-forward_classifier = hk.transform(_forward_classifier)
-params = forward_classifier.init(rng=rng_key, x=dummy_x)
-
-ret = forward_classifier.apply(params, None, dummy_x)
-```
-
-
-
-
-
-
-Any library
-
-
- From PyTorch
-
-``` python
-import ivy
-import kornia
-import requests
-import jax.numpy as jnp
-from PIL import Image
-
-# transpile kornia from torch to jax
-jax_kornia = ivy.transpile(kornia, source="torch", to="jax")
-
-# get an image
-url = "http://images.cocodataset.org/train2017/000000000034.jpg"
-raw_img = Image.open(requests.get(url, stream=True).raw)
-
-# convert it to the format expected by kornia
-img = jnp.transpose(jnp.array(raw_img), (2, 0, 1))
-img = jnp.expand_dims(img, 0) / 255
-
-# and use the transpiled version of any function from the library!
-out = jax_kornia.enhance.sharpness(img, 5)
-```
-
-
-
- From TensorFlow
-
-``` python
-import ivy
-import jax
-import os
-os.environ["SM_FRAMEWORK"] = "tf.keras"
-import segmentation_models as sm
-
-# transpile sm from tensorflow to jax
-jax_sm = ivy.transpile(sm, source="tensorflow", to="jax")
-
-# get some image-like arrays
-key = jax.random.PRNGKey(23)
-key1, key2 = jax.random.split(key)
-output = jax.random.uniform(key1, (1, 3, 512, 512))
-target = jax.random.uniform(key2, (1, 3, 512, 512))
-
-# and use the transpiled version of any function from the library!
-out = jax_sm.metrics.iou_score(output, target)
-```
-
-
-
- From NumPy
-
-``` python
-import ivy
-import madmom
-import jax.numpy as jnp
-
-# transpile madmon from numpy to jax
-jax_madmom = ivy.transpile(madmom, source="numpy", to="jax")
-
-# get some arrays
-freqs = jnp.arange(20) * 10
-
-# and use the transpiled version of any function from the library!
-out = jax_madmom.audio.filters.hz2midi(freqs)
-```
-
-
-
-
-
-
-Any function
-
-
- From PyTorch
-
-``` python
-import ivy
-import torch
-import jax.numpy as jnp
-
-def loss(predictions, targets):
- return torch.sqrt(torch.mean((predictions - targets) ** 2))
-
-# transpile any function from torch to jax
-jax_loss = ivy.transpile(loss, source="torch", to="jax")
-
-# get some arrays
-p = jnp.array([3.0, 2.0, 1.0])
-t = jnp.array([0.0, 0.0, 0.0])
-
-# and use the transpiled version!
-out = jax_loss(p, t)
-```
-
-
-
- From TensorFlow
-
-``` python
-import ivy
-import tensorflow as tf
-import jax.numpy as jnp
-
-def loss(predictions, targets):
- return tf.sqrt(tf.reduce_mean(tf.square(predictions - targets)))
-
-# transpile any function from tf to jax
-jax_loss = ivy.transpile(loss, source="tensorflow", to="jax")
-
-# get some arrays
-p = jnp.array([3.0, 2.0, 1.0])
-t = jnp.array([0.0, 0.0, 0.0])
-
-# and use the transpiled version!
-out = jax_loss(p, t)
-```
-
-
-
- From NumPy
-
-``` python
-import ivy
-import numpy as np
-import jax
-import jax.numpy as jnp
-jax.config.update('jax_enable_x64', True)
-
-def loss(predictions, targets):
- return np.sqrt(np.mean((predictions - targets) ** 2))
-
-# transpile any function from numpy to jax
-jax_loss = ivy.transpile(loss, source="numpy", to="jax")
-
-# get some arrays
-p = jnp.array([3.0, 2.0, 1.0])
-t = jnp.array([0.0, 0.0, 0.0])
-
-# and use the transpiled version!
-out = jax_loss(p, t)
-```
-
-
-
-
-
-
-
-
-
-I'm using NumPy 
-You can use Ivy to get NumPy code from:
-
-Any library
-
-
- From PyTorch
-
-``` python
-import ivy
-import kornia
-import requests
-import numpy as np
-from PIL import Image
-
-# transpile kornia from torch to np
-np_kornia = ivy.transpile(kornia, source="torch", to="numpy")
-
-# get an image
-url = "http://images.cocodataset.org/train2017/000000000034.jpg"
-raw_img = Image.open(requests.get(url, stream=True).raw)
-
-# convert it to the format expected by kornia
-img = np.transpose(np.array(raw_img), (2, 0, 1))
-img = np.expand_dims(img, 0) / 255
-
-# and use the transpiled version of any function from the library!
-out = np_kornia.enhance.sharpness(img, 5)
-```
-
-
-
- From TensorFlow
-
-``` python
-import ivy
-import numpy as np
-import os
-os.environ["SM_FRAMEWORK"] = "tf.keras"
-import segmentation_models as sm
-
-# transpile sm from tensorflow to numpy
-np_sm = ivy.transpile(sm, source="tensorflow", to="numpy")
-
-# get some image-like arrays
-output = np.random.rand(1, 3, 512, 512).astype(dtype=np.float32)
-target = np.random.rand(1, 3, 512, 512).astype(dtype=np.float32)
-
-# and use the transpiled version of any function from the library!
-out = np_sm.metrics.iou_score(output, target)
-```
-
-
-
- From Jax
-
-``` python
-import ivy
-import rax
-import numpy as np
-
-# transpile rax from jax to numpy
-np_rax = ivy.transpile(rax, source="jax", to="numpy")
-
-# get some arrays
-scores = np.array([2.2, 1.3, 5.4])
-labels = np.array([1.0, 0.0, 0.0])
-
-# and use the transpiled version of any function from the library!
-out = np_rax.poly1_softmax_loss(scores, labels)
-```
-
-
-
-
-
-
-Any function
-
-
- From PyTorch
-
-``` python
-import ivy
-import torch
-import numpy as np
-
-def loss(predictions, targets):
- return torch.sqrt(torch.mean((predictions - targets) ** 2))
-
-# transpile any function from torch to numpy
-np_loss = ivy.transpile(loss, source="torch", to="numpy")
-
-# get some arrays
-p = np.array([3.0, 2.0, 1.0])
-t = np.array([0.0, 0.0, 0.0])
-
-# and use the transpiled version!
-out = np_loss(p, t)
-```
-
-
-
- From TensorFlow
-
-``` python
-import ivy
-import tensorflow as tf
-import numpy as np
-
-def loss(predictions, targets):
- return tf.sqrt(tf.reduce_mean(tf.square(predictions - targets)))
-
-# transpile any function from tf to numpy
-np_loss = ivy.transpile(loss, source="tensorflow", to="numpy")
-
-# get some arrays
-p = np.array([3.0, 2.0, 1.0])
-t = np.array([0.0, 0.0, 0.0])
-
-# and use the transpiled version!
-out = np_loss(p, t)
-```
-
-
-
- From JAX
-
-``` python
-import ivy
-import jax.numpy as jnp
-import numpy as np
-
-def loss(predictions, targets):
- return jnp.sqrt(jnp.mean((predictions - targets) ** 2))
-
-# transpile any function from jax to numpy
-np_loss = ivy.transpile(loss, source="jax", to="numpy")
-
-# get some arrays
-p = np.array([3.0, 2.0, 1.0])
-t = np.array([0.0, 0.0, 0.0])
-
-# and use the transpiled version!
-out = np_loss(p, t)
-```
-
-
-
-
-
-
-
-
-I'm using Ivy 
-
-Or you can use Ivy as a framework, breaking yourself (and your code)
-free from deciding which community to support, allowing anyone to run
-your code in their framework of choice!
-
-``` python
-import ivy
-
-# A simple image classification model
-class IvyNet(ivy.Module):
- def __init__(
- self,
- h_w=(32, 32),
- input_channels=3,
- output_channels=512,
- num_classes=2,
- data_format="NCHW",
- device="cpu",
- ):
- self.h_w = h_w
- self.input_channels = input_channels
- self.output_channels = output_channels
- self.num_classes = num_classes
- self.data_format = data_format
- self.device = device
- super().__init__()
-
- def _build(self, *args, **kwargs):
- self.extractor = ivy.Sequential(
- ivy.Conv2D(self.input_channels, 6, [5, 5], 1, "SAME", data_format=self.data_format),
- ivy.GELU(),
- ivy.Conv2D(6, 16, [5, 5], 1, "SAME", data_format=self.data_format),
- ivy.GELU(),
- ivy.Conv2D(16, self.output_channels, [5, 5], 1, "SAME", data_format=self.data_format),
- ivy.GELU(),
- )
-
- self.classifier = ivy.Sequential(
- # Since the padding is "SAME", this would be image_height x image_width x output_channels
- ivy.Linear(self.h_w[0] * self.h_w[1] * self.output_channels, 512),
- ivy.GELU(),
- ivy.Linear(512, self.num_classes),
- )
-
- def _forward(self, x):
- x = self.extractor(x)
- # flatten all dims except batch dim
- x = ivy.flatten(x, start_dim=1, end_dim=-1)
- logits = self.classifier(x)
- probs = ivy.softmax(logits)
- return logits, probs
-```
-
-After building your model in Ivy, you can set your favourite framework
-as the backend to use its operations under the hood!
-
-``` python
-ivy.set_backend("torch")
-model = IvyNet()
-x = torch.randn(1, 3, 32, 32)
-logits, probs = model(x)
-```
-
-``` python
-ivy.set_backend("tensorflow")
-model = IvyNet()
-x = tf.random.uniform(shape=(1, 3, 32, 32))
-logits, probs = model(x)
-```
-
-``` python
-ivy.set_backend("jax")
-model = IvyNet()
-x = jax.random.uniform(key, shape=(1, 3, 32, 32))
-logits, probs = model(x)
-```
-
-``` python
-ivy.set_backend("numpy")
-model = IvyNet()
-x = np.random.uniform(size=(1, 3, 32, 32))
-logits, probs = model(x)
-```
-
-Last but not least, we can also build the training pipeline in pure ivy
-β¬οΈ
-
-
-Let's define some helper functions first
-
-``` python
-# helper function for loading the dataset in batches
-def generate_batches(images, classes, dataset_size, batch_size=32):
- targets = {k: v for v, k in enumerate(np.unique(classes))}
- y_train = [targets[classes[i]] for i in range(len(classes))]
- if batch_size > dataset_size:
- raise ivy.utils.exceptions.IvyError("Use a smaller batch size")
- for idx in range(0, dataset_size, batch_size):
- yield ivy.stack(images[idx : min(idx + batch_size, dataset_size)]), ivy.array(
- y_train[idx : min(idx + batch_size, dataset_size)]
- )
-
-
-# helper function to get the number of current predictions
-def num_correct(preds, labels):
- return (preds.argmax() == labels).sum().to_numpy().item()
-
-
-# define a loss function
-def loss_fn(params):
- v, model, x, y = params
- y_pred, probs = model(x)
- return ivy.cross_entropy(y, probs), probs
-```
-
-
-
-
-And train this model!
-
-``` python
-# train the model on gpu if it's available
-device = "cuda:0" if ivy.gpu_is_available() else "cpu"
-
-# training hyperparams
-optimizer= ivy.Adam(1e-4)
-batch_size = 64
-num_epochs = 20
-num_classes = 10
-
-model = IvyNet(
- h_w=(28, 28),
- input_channels=1,
- output_channels=120,
- num_classes=num_classes,
- device=device,
-)
-model_name = type(model).__name__.lower()
-
-
-# training loop
-def train(images, classes, epochs, model, device, num_classes=10, batch_size=32):
- # training metrics
- epoch_loss = 0.0
- running_loss = 0.0
- fields = ["epoch", "epoch_loss", "training_accuracy"]
- metrics = []
- dataset_size = len(images)
-
- for epoch in range(epochs):
- train_loss, train_correct = 0, 0
- train_loop = tqdm(
- generate_batches(images, classes, len(images), batch_size=batch_size),
- total=dataset_size // batch_size,
- position=0,
- leave=True,
- )
-
- for xbatch, ybatch in train_loop:
- if device != "cpu":
- xbatch, ybatch = xbatch.to_device("gpu:0"), ybatch.to_device("gpu:0")
-
- # Since the cross entropy function expects the target classes to be in one-hot encoded format
- ybatch_encoded = ivy.one_hot(ybatch, num_classes)
-
- # update model params
- loss_probs, grads = ivy.execute_with_gradients(
- loss_fn,
- (model.v, model, xbatch, ybatch_encoded),
- )
-
- model.v = optimizer.step(model.v, grads["0"])
-
- batch_loss = ivy.to_numpy(loss_probs[0]).mean().item() # batch mean loss
- epoch_loss += batch_loss * xbatch.shape[0]
- train_correct += num_correct(loss_probs[1], ybatch)
-
- train_loop.set_description(f"Epoch [{epoch + 1:2d}/{epochs}]")
- train_loop.set_postfix(
- running_loss=batch_loss,
- accuracy_percentage=(train_correct / dataset_size) * 100,
- )
-
- epoch_loss = epoch_loss / dataset_size
- training_accuracy = train_correct / dataset_size
-
- metrics.append([epoch, epoch_loss, training_accuracy])
-
- train_loop.write(
- f"\nAverage training loss: {epoch_loss:.6f}, Train Correct: {train_correct}",
- end="\n",
- )
-
- # write metrics for plotting
- with open(f"/{model_name}_train_summary.csv", "w") as f:
- f = csv.writer(f)
- f.writerow(fields)
- f.writerows(metrics)
-
-
-# assuming the dataset(images and classes) are already prepared in a folder
-train(images, classes, num_epochs, model, device, num_classes = num_classes, batch_size = batch_size)
-```
-
-
-
-## Contributing
-
-We believe that everyone can contribute and make a difference. Whether
-it\'s writing code π», fixing bugs π, or simply sharing feedback π¬,
-your contributions are definitely welcome and appreciated π
-
-Check out all of our open tasks, and find out more info in our
-[Contributing
-guide](https://unify.ai/docs/ivy/overview/contributing.html) in the
-docs!
-
-Join our amazing community as a code contributor, and help accelerate
-our journey to unify all ML frameworks!
-
-
-
-
-
-## Community
-
-In order to achieve the ambitious goal of unifying AI we definitely need
-as many hands as possible on it! Whether you are a seasoned developer or
-just starting out, you\'ll find a place here! Join the Ivy community in
-our [Discord](https://discord.gg/sXyFF8tDtm) πΎ server, which is the
-perfect place to ask questions, share ideas, and get help from both
-fellow developers and the Ivy Team directly!
-
-Also! Feel free to follow us on
-[Twitter](https://twitter.com/letsunifyai) π¦ as well, we use it to
-share updates, sneak peeks, and all sorts of relevant news, certainly a
-great way to stay in the loop π
-
-Can\'t wait to see you there!
-
-## Citation
-
-If you use Ivy for your work, please don\'t forget to give proper credit
-by including the accompanying [paper](https://arxiv.org/abs/2102.02886)
-π in your references. It\'s a small way to show appreciation and help
-to continue to support this and other open source projects π
-
- @article{lenton2021ivy,
- title={Ivy: Templated deep learning for inter-framework portability},
- author={Lenton, Daniel and Pardo, Fabio and Falck, Fabian and James, Stephen and Clark, Ronald},
- journal={arXiv preprint arXiv:2102.02886},
- year={2021}
- }
+> π We are granting pilot access to **Ivy\'s Tracer and Transpiler**
+> to some users, [join the waitlist](https://console.unify.ai/) if you
+> want to test them out!
+
+
+
+
+
+------------------------------------------------------------------------
+
+
+
+------------------------------------------------------------------------
+
+# Status
+
+
+
+
+------------------------------------------------------------------------
+
+# Unified AI
+
+
+
+
+
+------------------------------------------------------------------------
+
+Ivy is an open-source machine learning framework that
+enables you to:
+
+- π₯ **Autotune your model**: Automatically find the optimal framework, compiler infrastructure and hardware for your specific use case using `ivy.autotune`.
+- π **Convert code into any framework**: Use and build on top of any model, library, or device by converting any code from one framework to another using `ivy.transpile`.
+- βοΈ **Write framework-agnostic code**: Write your code once in ivy and then choose the most appropriate ML framework as the backend to leverage all the benefits and tools.
+
+[Join our growing community](https://discord.com/invite/sXyFF8tDtm) π to connect with people using Ivy. **Let\'s** [unify.ai](https://unify.ai) **together π¦Ύ**
+
+------------------------------------------------------------------------
+
+# Getting started
+
+The best way to get familiar with Ivy is to go through the [Demos](https://unify.ai/docs/ivy/demos/examples_and_demos.html), a good starting point is [Learn The Basics](https://unify.ai/docs/ivy/demos/learn_the_basics.html).
+
+The most important notebooks are:
+
+- [How to convert your code between frameworks?](https://unify.ai/docs/ivy/demos/learn_the_basics/04_transpile_code.html)
+- [How to write framework-agnostic code?](https://unify.ai/docs/ivy/demos/learn_the_basics/01_write_ivy_code.html)
+- Accelerate your development (WIP)
+- Autotune and optimize models (WIP)
+
+------------------------------------------------------------------------
+
+## Installing ivy
+
+There are various ways to use Ivy, depending on your preferred
+environment:
+
+### Installing using pip
+
+The easiest way to set up Ivy is to install it using pip with the
+following command:
+
+``` bash
+pip install ivy
+```
+
+or alternatively:
+
+``` bash
+python3 -m pip install ivy
+```
+
+
+Docker
+
+If you prefer to use containers, we also have pre-built Docker images
+with all the supported frameworks and some relevant packages already
+installed, which you can pull from:
+
+``` bash
+docker pull unifyai/ivy:latest
+```
+
+If you are working on a GPU device, you can pull from:
+
+``` bash
+docker pull unifyai/ivy:latest-gpu
+```
+
+
+
+From Source
+
+You can also install Ivy from source if you want to take advantage of
+the latest changes, but we can\'t ensure everything will work as
+expected. :sweat_smile:
+
+``` bash
+git clone https://github.com/unifyai/ivy.git
+cd ivy
+pip install --user -e .
+```
+
+or alternatively, for the last step:
+
+``` bash
+python3 -m pip install --user -e .
+```
+
+
+If you want to set up testing and various frameworks it\'s probably best
+to check out the [Contributing - Setting
+Up](https://unify.ai/docs/ivy/overview/contributing/setting_up. html#setting-up)
+page, where OS-specific and IDE-specific instructions and video
+tutorials to do so are available!
+
+
+
+------------------------------------------------------------------------
+
+## Using Ivy
+
+After installing Ivy, you can start using it straight away, for example:
+
+
+ Transpiling any code from one framework to another
+
+ ``` python
+ import ivy
+ import torch
+ import jax
+
+ def jax_fn(x):
+ a = jax.numpy.dot(x, x)
+ b = jax.numpy.mean(x)
+ return x * a + b
+
+ jax_x = jax.numpy.array([1, 2, 3])
+ torch_x = torch.tensor([1, 2, 3])
+ torch_fn = ivy.transpile(jax_fn, source="jax", to="torch", args=(jax_x,))
+ ret = torch_fn(torch_x)
+ ```
+
+
+
+
+ Running your code with any backend
+
+ ``` python
+ import ivy
+ import torch
+ import jax
+
+ ivy.set_backend("jax")
+
+ x = jax.numpy.array([1, 2, 3])
+ y = jax.numpy.array([3, 2, 1])
+ z = ivy.add(x, y)
+
+ ivy.set_backend('torch')
+
+ x = torch.tensor([1, 2, 3])
+ y = torch.tensor([3, 2, 1])
+ z = ivy.add(x, y)
+ ```
+
+
+
+------------------------------------------------------------------------
+
+# Documentation
+
+You can find Ivy's documentation on the [Docs page](https://unify.ai/docs/ivy/), which includes:
+- [Motivation](https://unify.ai/docs/ivy/overview/background.html): This contextualizes the problem Ivy is trying to solve by going over
+ - The current [ML Explosion](https://unify.ai/docs/ivy/overview/background/ml_explosion.html#ml-explosion).
+ - Explaining why it is important [to solve this problem](https://unify.ai/docs/ivy/overview/background/why_unify.html#why-unify).
+ - Explaining how we adhere to existing [standards](https://unify.ai/docs/ivy/overview/background/standardization.html#standardization) to make this happen.
+- [Related Work](https://unify.ai/docs/ivy/overview/related_work.html): Which paints a picture of the role Ivy plays in the ML stack, comparing it to other existing solutions in terms of functionalities and abstraction level.
+- [Design](https://unify.ai/docs/ivy/overview/design.html): A user-focused guide about the design decision behind the architecture and the main building blocks of Ivy.
+- [Deep Dive](https://unify.ai/docs/ivy/overview/deep_dive.html): Which delves deeper into the implementation details of Ivy and is oriented towards potential contributors to the code base.
+
+
+------------------------------------------------------------------------
+
+# Examples
+
+The [Examples page](https://unify.ai/demos/) features a wide range of
+demos and tutorials showcasing the functionalities of Ivy along with
+multiple use cases, but feel free to check out some shorter
+framework-specific examples here β¬οΈ
+
+
+I'm using PyTorch 
+ You can use Ivy to get PyTorch code from:
+
+ Any model
+
+
+ From TensorFlow
+
+``` python
+import ivy
+import torch
+import tensorflow as tf
+
+# Get a pretrained keras model
+eff_encoder = tf.keras.applications.efficientnet_v2.EfficientNetV2B0(
+ include_top=False, weights="imagenet", input_shape=(224, 224, 3)
+)
+
+# Transpile it into a torch.nn.Module with the corresponding parameters
+noise = tf.random.normal(shape=(1, 224, 224, 3))
+torch_eff_encoder = ivy.transpile(eff_encoder, to="torch", args=(noise,))
+
+# Build a classifier using the transpiled encoder
+class Classifier(torch.nn.Module):
+ def __init__(self, num_classes=20):
+ super().__init__()
+ self.encoder = torch_eff_encoder
+ self.fc = torch.nn.Linear(1280, num_classes)
+
+ def forward(self, x):
+ x = self.encoder(x)
+ return self.fc(x)
+
+# Initialize a trainable, customizable, torch.nn.Module
+classifier = Classifier()
+ret = classifier(torch.rand((1, 244, 244, 3)))
+```
+
+
+
+ From JAX
+
+``` python
+import ivy
+import jax
+import torch
+
+# Get a pretrained haiku model
+# https://unify.ai/demos/scripts/deepmind_perceiver_io.py
+from deepmind_perceiver_io import key, perceiver_backbone
+
+# Transpile it into a torch.nn.Module with the corresponding parameters
+dummy_input = jax.random.uniform(key, shape=(1, 3, 224, 224))
+params = perceiver_backbone.init(rng=key, images=dummy_input)
+backbone = ivy.transpile(
+ perceiver_backbone, to="torch", params_v=params, kwargs={"images": dummy_input}
+)
+
+# Build a classifier using the transpiled backbone
+class PerceiverIOClassifier(torch.nn.Module):
+ def __init__(self, num_classes=20):
+ super().__init__()
+ self.backbone = backbone
+ self.max_pool = torch.nn.MaxPool2d((512, 1))
+ self.flatten = torch.nn.Flatten()
+ self.fc = torch.nn.Linear(1024, num_classes)
+
+ def forward(self, x):
+ x = self.backbone(images=x)
+ x = self.flatten(self.max_pool(x))
+ return self.fc(x)
+
+# Initialize a trainable, customizable, torch.nn.Module
+classifier = PerceiverIOClassifier()
+ret = classifier(torch.rand((1, 3, 224, 224)))
+```
+
+
+
+
+
+
+Any library
+
+
+ From Tensorflow
+
+``` python
+import ivy
+import torch
+import os
+os.environ["SM_FRAMEWORK"] = "tf.keras"
+import segmentation_models as sm
+
+# transpile sm from tensorflow to torch
+torch_sm = ivy.transpile(sm, source="tensorflow", to="torch")
+
+# get some image-like arrays
+output = torch.rand((1, 3, 512, 512))
+target = torch.rand((1, 3, 512, 512))
+
+# and use the transpiled version of any function from the library!
+out = torch_sm.metrics.iou_score(output, target)
+```
+
+
+
+ From JAX
+
+``` python
+import ivy
+import rax
+import torch
+
+# transpile rax from jax to torch
+torch_rax = ivy.transpile(rax, source="jax", to="torch")
+
+# get some arrays
+scores = torch.tensor([2.2, 1.3, 5.4])
+labels = torch.tensor([1.0, 0.0, 0.0])
+
+# and use the transpiled version of any function from the library!
+out = torch_rax.poly1_softmax_loss(scores, labels)
+```
+
+
+
+ From NumPy
+
+``` python
+import ivy
+import torch
+import madmom
+
+# transpile madmon from numpy to torch
+torch_madmom = ivy.transpile(madmom, source="numpy", to="torch")
+
+# get some arrays
+freqs = torch.arange(20) * 10
+
+# and use the transpiled version of any function from the library!
+out = torch_madmom.audio.filters.hz2midi(freqs)
+```
+
+
+
+
+
+
+Any function
+
+
+ From Tensorflow
+
+``` python
+import ivy
+import tensorflow as tf
+import torch
+
+def loss(predictions, targets):
+ return tf.sqrt(tf.reduce_mean(tf.square(predictions - targets)))
+
+# transpile any function from tf to torch
+torch_loss = ivy.transpile(loss, source="tensorflow", to="torch")
+
+# get some arrays
+p = torch.tensor([3.0, 2.0, 1.0])
+t = torch.tensor([0.0, 0.0, 0.0])
+
+# and use the transpiled version!
+out = torch_loss(p, t)
+```
+
+
+
+ From JAX
+
+``` python
+import ivy
+import jax.numpy as jnp
+import torch
+
+def loss(predictions, targets):
+ return jnp.sqrt(jnp.mean((predictions - targets) ** 2))
+
+# transpile any function from jax to torch
+torch_loss = ivy.transpile(loss, source="jax", to="torch")
+
+# get some arrays
+p = torch.tensor([3.0, 2.0, 1.0])
+t = torch.tensor([0.0, 0.0, 0.0])
+
+# and use the transpiled version!
+out = torch_loss(p, t)
+```
+
+
+
+ From NumPy
+
+``` python
+import ivy
+import numpy as np
+import torch
+
+def loss(predictions, targets):
+ return np.sqrt(np.mean((predictions - targets) ** 2))
+
+# transpile any function from numpy to torch
+torch_loss = ivy.transpile(loss, source="numpy", to="torch")
+
+# get some arrays
+p = torch.tensor([3.0, 2.0, 1.0])
+t = torch.tensor([0.0, 0.0, 0.0])
+
+# and use the transpiled version!
+out = torch_loss(p, t)
+```
+
+
+
+
+
+
+
+
+
+I'm using TensorFlow 
+You can use Ivy to get TensorFlow code from:
+
+Any model
+
+
+ From PyTorch
+
+``` python
+import ivy
+import torch
+import timm
+import tensorflow as tf
+
+# Get a pretrained pytorch model
+mlp_encoder = timm.create_model("mixer_b16_224", pretrained=True, num_classes=0)
+
+# Transpile it into a keras.Model with the corresponding parameters
+noise = torch.randn(1, 3, 224, 224)
+mlp_encoder = ivy.transpile(mlp_encoder, to="tensorflow", args=(noise,))
+
+# Build a classifier using the transpiled encoder
+class Classifier(tf.keras.Model):
+ def __init__(self):
+ super().__init__()
+ self.encoder = mlp_encoder
+ self.output_dense = tf.keras.layers.Dense(units=1000, activation="softmax")
+
+ def call(self, x):
+ x = self.encoder(x)
+ return self.output_dense(x)
+
+# Transform the classifier and use it as a standard keras.Model
+x = tf.random.normal(shape=(1, 3, 224, 224))
+model = Classifier()
+ret = model(x)
+```
+
+
+
+ From JAX
+
+``` python
+import ivy
+import jax
+import tensorflow as tf
+
+# Get a pretrained haiku model
+# https://unify.ai/demos/scripts/deepmind_perceiver_io.py
+from deepmind_perceiver_io import key, perceiver_backbone
+
+# Transpile it into a tf.keras.Model with the corresponding parameters
+dummy_input = jax.random.uniform(key, shape=(1, 3, 224, 224))
+params = perceiver_backbone.init(rng=key, images=dummy_input)
+backbone = ivy.transpile(
+ perceiver_backbone, to="tensorflow", params_v=params, args=(dummy_input,)
+)
+
+# Build a classifier using the transpiled backbone
+class PerceiverIOClassifier(tf.keras.Model):
+ def __init__(self, num_classes=20):
+ super().__init__()
+ self.backbone = backbone
+ self.max_pool = tf.keras.layers.MaxPooling1D(pool_size=512)
+ self.flatten = tf.keras.layers.Flatten()
+ self.fc = tf.keras.layers.Dense(num_classes)
+
+ def call(self, x):
+ x = self.backbone(x)
+ x = self.flatten(self.max_pool(x))
+ return self.fc(x)
+
+# Initialize a trainable, customizable, tf.keras.Model
+x = tf.random.normal(shape=(1, 3, 224, 224))
+classifier = PerceiverIOClassifier()
+ret = classifier(x)
+```
+
+
+
+
+
+
+Any library
+
+
+ From PyTorch
+
+``` python
+import ivy
+import kornia
+import requests
+import numpy as np
+import tensorflow as tf
+from PIL import Image
+
+# transpile kornia from torch to tensorflow
+tf_kornia = ivy.transpile(kornia, source="torch", to="tensorflow")
+
+# get an image
+url = "http://images.cocodataset.org/train2017/000000000034.jpg"
+raw_img = Image.open(requests.get(url, stream=True).raw)
+
+# convert it to the format expected by kornia
+img = np.array(raw_img)
+img = tf.transpose(tf.constant(img), (2, 0, 1))
+img = tf.expand_dims(img, 0) / 255
+
+# and use the transpiled version of any function from the library!
+out = tf_kornia.enhance.sharpness(img, 5)
+```
+
+
+
+ From JAX
+
+``` python
+import ivy
+import rax
+import tensorflow as tf
+
+# transpile rax from jax to tensorflow
+tf_rax = ivy.transpile(rax, source="jax", to="tensorflow")
+
+# get some arrays
+scores = tf.constant([2.2, 1.3, 5.4])
+labels = tf.constant([1.0, 0.0, 0.0])
+
+# and use the transpiled version of any function from the library!
+out = tf_rax.poly1_softmax_loss(scores, labels)
+```
+
+
+
+ From NumPy
+
+``` python
+import ivy
+import madmom
+import tensorflow as tf
+
+# transpile madmom from numpy to tensorflow
+tf_madmom = ivy.transpile(madmom, source="numpy", to="tensorflow")
+
+# get some arrays
+freqs = tf.range(20) * 10
+
+# and use the transpiled version of any function from the library!
+out = tf_madmom.audio.filters.hz2midi(freqs)
+```
+
+
+
+
+
+
+Any function
+
+
+ From PyTorch
+
+``` python
+import ivy
+import torch
+import tensorflow as tf
+
+def loss(predictions, targets):
+ return torch.sqrt(torch.mean((predictions - targets) ** 2))
+
+# transpile any function from torch to tensorflow
+tf_loss = ivy.transpile(loss, source="torch", to="tensorflow")
+
+# get some arrays
+p = tf.constant([3.0, 2.0, 1.0])
+t = tf.constant([0.0, 0.0, 0.0])
+
+# and use the transpiled version!
+out = tf_loss(p, t)
+```
+
+
+
+ From JAX
+
+``` python
+import ivy
+import jax.numpy as jnp
+import tensorflow as tf
+
+def loss(predictions, targets):
+ return jnp.sqrt(jnp.mean((predictions - targets) ** 2))
+
+# transpile any function from jax to tensorflow
+tf_loss = ivy.transpile(loss, source="jax", to="tensorflow")
+
+# get some arrays
+p = tf.constant([3.0, 2.0, 1.0])
+t = tf.constant([0.0, 0.0, 0.0])
+
+# and use the transpiled version!
+out = tf_loss(p, t)
+```
+
+
+
+ From NumPy
+
+``` python
+import ivy
+import numpy as np
+import tensorflow as tf
+
+def loss(predictions, targets):
+ return np.sqrt(np.mean((predictions - targets) ** 2))
+
+# transpile any function from numpy to tensorflow
+tf_loss = ivy.transpile(loss, source="numpy", to="tensorflow")
+
+# get some arrays
+p = tf.constant([3.0, 2.0, 1.0])
+t = tf.constant([0.0, 0.0, 0.0])
+
+# and use the transpiled version!
+out = tf_loss(p, t)
+```
+
+
+
+
+
+
+
+
+
+I'm using Jax 
+You can use Ivy to get JAX code from:
+
+Any model
+
+
+ From PyTorch
+
+``` python
+import ivy
+import timm
+import torch
+import jax
+import haiku as hk
+
+# Get a pretrained pytorch model
+mlp_encoder = timm.create_model("mixer_b16_224", pretrained=True, num_classes=0)
+
+# Transpile it into a hk.Module with the corresponding parameters
+noise = torch.randn(1, 3, 224, 224)
+mlp_encoder = ivy.transpile(mlp_encoder, to="jax", args=(noise,))
+
+# Build a classifier using the transpiled encoder
+class Classifier(hk.Module):
+ def __init__(self, num_classes=1000):
+ super().__init__()
+ self.encoder = mlp_encoder()
+ self.fc = hk.Linear(output_size=num_classes, with_bias=True)
+
+ def __call__(self, x):
+ x = self.encoder(x)
+ x = self.fc(x)
+ return x
+
+def _forward_classifier(x):
+ module = Classifier()
+ return module(x)
+
+# Transform the classifier and use it as a standard hk.Module
+rng_key = jax.random.PRNGKey(42)
+x = jax.random.uniform(key=rng_key, shape=(1, 3, 224, 224), dtype=jax.numpy.float32)
+forward_classifier = hk.transform(_forward_classifier)
+params = forward_classifier.init(rng=rng_key, x=x)
+
+ret = forward_classifier.apply(params, None, x)
+```
+
+
+
+ From TensorFlow
+
+``` python
+import ivy
+import jax
+import haiku as hk
+import tensorflow as tf
+
+# Get a pretrained keras model
+eff_encoder = tf.keras.applications.efficientnet_v2.EfficientNetV2B0(
+ include_top=False, weights="imagenet", input_shape=(224, 224, 3)
+)
+
+# Transpile it into a hk.Module with the corresponding parameters
+noise = tf.random.normal(shape=(1, 224, 224, 3))
+hk_eff_encoder = ivy.transpile(eff_encoder, to="jax", args=(noise,))
+
+# Build a classifier using the transpiled encoder
+class Classifier(hk.Module):
+ def __init__(self, num_classes=1000):
+ super().__init__()
+ self.encoder = hk_eff_encoder()
+ self.fc = hk.Linear(output_size=num_classes, with_bias=True)
+
+ def __call__(self, x):
+ x = self.encoder(x)
+ x = self.fc(x)
+ return x
+
+def _forward_classifier(x):
+ module = Classifier()
+ return module(x)
+
+# Transform the classifier and use it as a standard hk.Module
+rng_key = jax.random.PRNGKey(42)
+dummy_x = jax.random.uniform(key=rng_key, shape=(1, 224, 224, 3))
+forward_classifier = hk.transform(_forward_classifier)
+params = forward_classifier.init(rng=rng_key, x=dummy_x)
+
+ret = forward_classifier.apply(params, None, dummy_x)
+```
+
+
+
+
+
+
+Any library
+
+
+ From PyTorch
+
+``` python
+import ivy
+import kornia
+import requests
+import jax.numpy as jnp
+from PIL import Image
+
+# transpile kornia from torch to jax
+jax_kornia = ivy.transpile(kornia, source="torch", to="jax")
+
+# get an image
+url = "http://images.cocodataset.org/train2017/000000000034.jpg"
+raw_img = Image.open(requests.get(url, stream=True).raw)
+
+# convert it to the format expected by kornia
+img = jnp.transpose(jnp.array(raw_img), (2, 0, 1))
+img = jnp.expand_dims(img, 0) / 255
+
+# and use the transpiled version of any function from the library!
+out = jax_kornia.enhance.sharpness(img, 5)
+```
+
+
+
+ From TensorFlow
+
+``` python
+import ivy
+import jax
+import os
+os.environ["SM_FRAMEWORK"] = "tf.keras"
+import segmentation_models as sm
+
+# transpile sm from tensorflow to jax
+jax_sm = ivy.transpile(sm, source="tensorflow", to="jax")
+
+# get some image-like arrays
+key = jax.random.PRNGKey(23)
+key1, key2 = jax.random.split(key)
+output = jax.random.uniform(key1, (1, 3, 512, 512))
+target = jax.random.uniform(key2, (1, 3, 512, 512))
+
+# and use the transpiled version of any function from the library!
+out = jax_sm.metrics.iou_score(output, target)
+```
+
+
+
+ From NumPy
+
+``` python
+import ivy
+import madmom
+import jax.numpy as jnp
+
+# transpile madmon from numpy to jax
+jax_madmom = ivy.transpile(madmom, source="numpy", to="jax")
+
+# get some arrays
+freqs = jnp.arange(20) * 10
+
+# and use the transpiled version of any function from the library!
+out = jax_madmom.audio.filters.hz2midi(freqs)
+```
+
+
+
+
+
+
+Any function
+
+
+ From PyTorch
+
+``` python
+import ivy
+import torch
+import jax.numpy as jnp
+
+def loss(predictions, targets):
+ return torch.sqrt(torch.mean((predictions - targets) ** 2))
+
+# transpile any function from torch to jax
+jax_loss = ivy.transpile(loss, source="torch", to="jax")
+
+# get some arrays
+p = jnp.array([3.0, 2.0, 1.0])
+t = jnp.array([0.0, 0.0, 0.0])
+
+# and use the transpiled version!
+out = jax_loss(p, t)
+```
+
+
+
+ From TensorFlow
+
+``` python
+import ivy
+import tensorflow as tf
+import jax.numpy as jnp
+
+def loss(predictions, targets):
+ return tf.sqrt(tf.reduce_mean(tf.square(predictions - targets)))
+
+# transpile any function from tf to jax
+jax_loss = ivy.transpile(loss, source="tensorflow", to="jax")
+
+# get some arrays
+p = jnp.array([3.0, 2.0, 1.0])
+t = jnp.array([0.0, 0.0, 0.0])
+
+# and use the transpiled version!
+out = jax_loss(p, t)
+```
+
+
+
+ From NumPy
+
+``` python
+import ivy
+import numpy as np
+import jax
+import jax.numpy as jnp
+jax.config.update('jax_enable_x64', True)
+
+def loss(predictions, targets):
+ return np.sqrt(np.mean((predictions - targets) ** 2))
+
+# transpile any function from numpy to jax
+jax_loss = ivy.transpile(loss, source="numpy", to="jax")
+
+# get some arrays
+p = jnp.array([3.0, 2.0, 1.0])
+t = jnp.array([0.0, 0.0, 0.0])
+
+# and use the transpiled version!
+out = jax_loss(p, t)
+```
+
+
+
+
+
+
+
+
+
+I'm using NumPy 
+You can use Ivy to get NumPy code from:
+
+Any library
+
+
+ From PyTorch
+
+``` python
+import ivy
+import kornia
+import requests
+import numpy as np
+from PIL import Image
+
+# transpile kornia from torch to np
+np_kornia = ivy.transpile(kornia, source="torch", to="numpy")
+
+# get an image
+url = "http://images.cocodataset.org/train2017/000000000034.jpg"
+raw_img = Image.open(requests.get(url, stream=True).raw)
+
+# convert it to the format expected by kornia
+img = np.transpose(np.array(raw_img), (2, 0, 1))
+img = np.expand_dims(img, 0) / 255
+
+# and use the transpiled version of any function from the library!
+out = np_kornia.enhance.sharpness(img, 5)
+```
+
+
+
+ From TensorFlow
+
+``` python
+import ivy
+import numpy as np
+import os
+os.environ["SM_FRAMEWORK"] = "tf.keras"
+import segmentation_models as sm
+
+# transpile sm from tensorflow to numpy
+np_sm = ivy.transpile(sm, source="tensorflow", to="numpy")
+
+# get some image-like arrays
+output = np.random.rand(1, 3, 512, 512).astype(dtype=np.float32)
+target = np.random.rand(1, 3, 512, 512).astype(dtype=np.float32)
+
+# and use the transpiled version of any function from the library!
+out = np_sm.metrics.iou_score(output, target)
+```
+
+
+
+ From Jax
+
+``` python
+import ivy
+import rax
+import numpy as np
+
+# transpile rax from jax to numpy
+np_rax = ivy.transpile(rax, source="jax", to="numpy")
+
+# get some arrays
+scores = np.array([2.2, 1.3, 5.4])
+labels = np.array([1.0, 0.0, 0.0])
+
+# and use the transpiled version of any function from the library!
+out = np_rax.poly1_softmax_loss(scores, labels)
+```
+
+
+
+
+
+
+Any function
+
+
+ From PyTorch
+
+``` python
+import ivy
+import torch
+import numpy as np
+
+def loss(predictions, targets):
+ return torch.sqrt(torch.mean((predictions - targets) ** 2))
+
+# transpile any function from torch to numpy
+np_loss = ivy.transpile(loss, source="torch", to="numpy")
+
+# get some arrays
+p = np.array([3.0, 2.0, 1.0])
+t = np.array([0.0, 0.0, 0.0])
+
+# and use the transpiled version!
+out = np_loss(p, t)
+```
+
+
+
+ From TensorFlow
+
+``` python
+import ivy
+import tensorflow as tf
+import numpy as np
+
+def loss(predictions, targets):
+ return tf.sqrt(tf.reduce_mean(tf.square(predictions - targets)))
+
+# transpile any function from tf to numpy
+np_loss = ivy.transpile(loss, source="tensorflow", to="numpy")
+
+# get some arrays
+p = np.array([3.0, 2.0, 1.0])
+t = np.array([0.0, 0.0, 0.0])
+
+# and use the transpiled version!
+out = np_loss(p, t)
+```
+
+
+
+ From JAX
+
+``` python
+import ivy
+import jax.numpy as jnp
+import numpy as np
+
+def loss(predictions, targets):
+ return jnp.sqrt(jnp.mean((predictions - targets) ** 2))
+
+# transpile any function from jax to numpy
+np_loss = ivy.transpile(loss, source="jax", to="numpy")
+
+# get some arrays
+p = np.array([3.0, 2.0, 1.0])
+t = np.array([0.0, 0.0, 0.0])
+
+# and use the transpiled version!
+out = np_loss(p, t)
+```
+
+
+
+
+
+
+
+
+
+
+I'm using Ivy
+
+Or you can use Ivy as a framework, breaking yourself (and your code)
+free from deciding which community to support, allowing anyone to run
+your code in their framework of choice!
+
+``` python
+import ivy
+
+# A simple image classification model
+class IvyNet(ivy.Module):
+ def __init__(
+ self,
+ h_w=(32, 32),
+ input_channels=3,
+ output_channels=512,
+ num_classes=2,
+ data_format="NCHW",
+ device="cpu",
+ ):
+ self.h_w = h_w
+ self.input_channels = input_channels
+ self.output_channels = output_channels
+ self.num_classes = num_classes
+ self.data_format = data_format
+ self.device = device
+ super().__init__()
+
+ def _build(self, *args, **kwargs):
+ self.extractor = ivy.Sequential(
+ ivy.Conv2D(self.input_channels, 6, [5, 5], 1, "SAME", data_format=self.data_format),
+ ivy.GELU(),
+ ivy.Conv2D(6, 16, [5, 5], 1, "SAME", data_format=self.data_format),
+ ivy.GELU(),
+ ivy.Conv2D(16, self.output_channels, [5, 5], 1, "SAME", data_format=self.data_format),
+ ivy.GELU(),
+ )
+
+ self.classifier = ivy.Sequential(
+ # Since the padding is "SAME", this would be image_height x image_width x output_channels
+ ivy.Linear(self.h_w[0] * self.h_w[1] * self.output_channels, 512),
+ ivy.GELU(),
+ ivy.Linear(512, self.num_classes),
+ )
+
+ def _forward(self, x):
+ x = self.extractor(x)
+ # flatten all dims except batch dim
+ x = ivy.flatten(x, start_dim=1, end_dim=-1)
+ logits = self.classifier(x)
+ probs = ivy.softmax(logits)
+ return logits, probs
+```
+
+After building your model in Ivy, you can set your favourite framework
+as the backend to use its operations under the hood!
+
+``` python
+ivy.set_backend("torch")
+model = IvyNet()
+x = torch.randn(1, 3, 32, 32)
+logits, probs = model(x)
+```
+
+``` python
+ivy.set_backend("tensorflow")
+model = IvyNet()
+x = tf.random.uniform(shape=(1, 3, 32, 32))
+logits, probs = model(x)
+```
+
+``` python
+ivy.set_backend("jax")
+model = IvyNet()
+x = jax.random.uniform(key, shape=(1, 3, 32, 32))
+logits, probs = model(x)
+```
+
+``` python
+ivy.set_backend("numpy")
+model = IvyNet()
+x = np.random.uniform(size=(1, 3, 32, 32))
+logits, probs = model(x)
+```
+
+Last but not least, we can also build the training pipeline in pure ivy
+β¬οΈ
+
+
+Let's define some helper functions first
+
+``` python
+# helper function for loading the dataset in batches
+def generate_batches(images, classes, dataset_size, batch_size=32):
+ targets = {k: v for v, k in enumerate(np.unique(classes))}
+ y_train = [targets[classes[i]] for i in range(len(classes))]
+ if batch_size > dataset_size:
+ raise ivy.utils.exceptions.IvyError("Use a smaller batch size")
+ for idx in range(0, dataset_size, batch_size):
+ yield ivy.stack(images[idx : min(idx + batch_size, dataset_size)]), ivy.array(
+ y_train[idx : min(idx + batch_size, dataset_size)]
+ )
+
+
+# helper function to get the number of current predictions
+def num_correct(preds, labels):
+ return (preds.argmax() == labels).sum().to_numpy().item()
+
+
+# define a loss function
+def loss_fn(params):
+ v, model, x, y = params
+ y_pred, probs = model(x)
+ return ivy.cross_entropy(y, probs), probs
+```
+
+
+
+
+And train this model!
+
+``` python
+# train the model on gpu if it's available
+device = "cuda:0" if ivy.gpu_is_available() else "cpu"
+
+# training hyperparams
+optimizer= ivy.Adam(1e-4)
+batch_size = 64
+num_epochs = 20
+num_classes = 10
+
+model = IvyNet(
+ h_w=(28, 28),
+ input_channels=1,
+ output_channels=120,
+ num_classes=num_classes,
+ device=device,
+)
+model_name = type(model).__name__.lower()
+
+
+# training loop
+def train(images, classes, epochs, model, device, num_classes=10, batch_size=32):
+ # training metrics
+ epoch_loss = 0.0
+ running_loss = 0.0
+ fields = ["epoch", "epoch_loss", "training_accuracy"]
+ metrics = []
+ dataset_size = len(images)
+
+ for epoch in range(epochs):
+ train_loss, train_correct = 0, 0
+ train_loop = tqdm(
+ generate_batches(images, classes, len(images), batch_size=batch_size),
+ total=dataset_size // batch_size,
+ position=0,
+ leave=True,
+ )
+
+ for xbatch, ybatch in train_loop:
+ if device != "cpu":
+ xbatch, ybatch = xbatch.to_device("gpu:0"), ybatch.to_device("gpu:0")
+
+ # Since the cross entropy function expects the target classes to be in one-hot encoded format
+ ybatch_encoded = ivy.one_hot(ybatch, num_classes)
+
+ # update model params
+ loss_probs, grads = ivy.execute_with_gradients(
+ loss_fn,
+ (model.v, model, xbatch, ybatch_encoded),
+ )
+
+ model.v = optimizer.step(model.v, grads["0"])
+
+ batch_loss = ivy.to_numpy(loss_probs[0]).mean().item() # batch mean loss
+ epoch_loss += batch_loss * xbatch.shape[0]
+ train_correct += num_correct(loss_probs[1], ybatch)
+
+ train_loop.set_description(f"Epoch [{epoch + 1:2d}/{epochs}]")
+ train_loop.set_postfix(
+ running_loss=batch_loss,
+ accuracy_percentage=(train_correct / dataset_size) * 100,
+ )
+
+ epoch_loss = epoch_loss / dataset_size
+ training_accuracy = train_correct / dataset_size
+
+ metrics.append([epoch, epoch_loss, training_accuracy])
+
+ train_loop.write(
+ f"\nAverage training loss: {epoch_loss:.6f}, Train Correct: {train_correct}",
+ end="\n",
+ )
+
+ # write metrics for plotting
+ with open(f"/{model_name}_train_summary.csv", "w") as f:
+ f = csv.writer(f)
+ f.writerow(fields)
+ f.writerows(metrics)
+
+
+# assuming the dataset(images and classes) are already prepared in a folder
+train(images, classes, num_epochs, model, device, num_classes = num_classes, batch_size = batch_size)
+```
+
+
+
+
+------------------------------------------------------------------------
+
+# Diving deeper
+
+Although the [Docs](https://unify.ai/docs/ivy/) are the best place to learn more, in the next section we will take a look at how Ivy works both as a transpiler and a framework in a bit more detail to get an idea of why and where to use it.
+
+
+Ivy as a transpiler
+
+Ivy\'s transpiler allows you to use code from any other framework (or
+from any other version of the same framework!) in your own code, by just
+adding one line of code. Under the hood, Ivy traces a computational
+graph and leverages the frontends and backends to link one framework to
+another.
+
+This way, Ivy makes all ML-related projects available for you,
+independently of the framework you want to use to research, develop, or
+deploy systems. Feel free to head over to the docs for the full API
+reference, but the functions you\'d most likely want to use are:
+
+``` python
+# Traces an efficient fully-functional graph from a function, removing all wrapping and redundant code
+ivy.trace_graph()
+
+# Converts framework-specific code to a different framework
+ivy.transpile()
+
+# Converts framework-specific code to Ivy
+ivy.unify()
+```
+
+These functions can be used eagerly or lazily. If you pass the necessary
+arguments for function tracing, the graph tracing/transpilation step will
+happen instantly (eagerly). Otherwise, the graph tracing/transpilation
+will happen only when the returned function is first invoked.
+
+``` python
+import ivy
+import jax
+ivy.set_backend("jax")
+
+# Simple JAX function to transpile
+def test_fn(x):
+ return jax.numpy.sum(x)
+
+x1 = ivy.array([1., 2.])
+```
+
+``` python
+# Arguments are available -> transpilation happens eagerly
+eager_graph = ivy.transpile(test_fn, source="jax", to="torch", args=(x1,))
+
+# eager_graph is now torch code and runs efficiently
+ret = eager_graph(x1)
+```
+
+``` python
+# Arguments are not available -> transpilation happens lazily
+lazy_graph = ivy.transpile(test_fn, source="jax", to="torch")
+
+# The transpiled graph is initialized, transpilation will happen here
+ret = lazy_graph(x1)
+
+# lazy_graph is now torch code and runs efficiently
+ret = lazy_graph(x1)
+```
+
+If you want to learn more, you can find more information in the [Ivy as
+a transpiler section of the
+docs!](https://unify.ai/docs/ivy/overview/design/ivy_as_a_transpiler.html)
+
+## When should I use Ivy as a transpiler?
+
+If you want to use building blocks published in other frameworks (neural
+networks, layers, array computing libraries, training pipelines\...),
+you want to integrate code developed in various frameworks, or maybe
+straight up move code from one framework to another, the transpiler is
+definitely the tool π§ for the job! As the output of transpilation is
+native code in the target framework, you can use the converted code just
+as if it was code originally developed in that framework, applying
+framework-specific optimizations or tools, instantly exposing your
+project to all of the unique perks of a different framework.
+
+
+
+
+Ivy as a framework
+
+The Ivy framework is built on top of various essential components,
+mainly the [Backend
+Handler](https://unify.ai/docs/ivy/overview/design/building_blocks.html#backend-handler),
+which manages what framework is being used behind the scenes and the
+[Backend Functional
+APIs](https://unify.ai/docs/ivy/overview/design/building_blocks.html#backend-functional-apis),
+which provide framework-specific implementations of the Ivy functions.
+Likewise, classes such as `ivy.Container` or `ivy.Array` are also
+available, facilitating the use of structured data and array-like
+objects (learn more about them
+[here!](https://unify.ai/docs/ivy/overview/design/ivy_as_a_framework.html)).
+
+All of the functionalities in Ivy are exposed through the
+`Ivy functional API` and the `Ivy stateful API`. All functions in the
+[Functional
+API](https://unify.ai/docs/ivy/overview/design/building_blocks.html#ivy-functional-api)
+are **Framework Agnostic Functions**, which means that we can use them
+like this:
+
+``` python
+import ivy
+import jax.numpy as jnp
+import tensorflow as tf
+import numpy as np
+import torch
+
+def mse_loss(y, target):
+ return ivy.mean((y - target)**2)
+
+jax_mse = mse_loss(jnp.ones((5,)), jnp.ones((5,)))
+tf_mse = mse_loss(tf.ones((5,)), tf.ones((5,)))
+np_mse = mse_loss(np.ones((5,)), np.ones((5,)))
+torch_mse = mse_loss(torch.ones((5,)), torch.ones((5,)))
+```
+
+In the example above we show how Ivy\'s functions are compatible with
+tensors from different frameworks. This is the same for ALL Ivy
+functions. They can accept tensors from any framework and return the
+correct result.
+
+The [Ivy Stateful
+API](https://unify.ai/docs/ivy/overview/design/ivy_as_a_framework/ivy_stateful_api.html),
+on the other hand, allows you to define trainable modules and layers,
+which you can use alone or as a part of any other framework code!
+
+``` python
+import ivy
+
+
+class Regressor(ivy.Module):
+ def __init__(self, input_dim, output_dim):
+ self.input_dim = input_dim
+ self.output_dim = output_dim
+ super().__init__()
+
+ def _build(self, *args, **kwargs):
+ self.linear0 = ivy.Linear(self.input_dim, 128)
+ self.linear1 = ivy.Linear(128, self.output_dim)
+
+ def _forward(self, x):
+ x = self.linear0(x)
+ x = ivy.functional.relu(x)
+ x = self.linear1(x)
+ return x
+```
+
+If we put it all together, we\'ll have something like this. This example
+uses PyTorch as the backend, but this can easily be changed to your
+favorite frameworks, such as TensorFlow, or JAX.
+
+``` python
+import ivy
+
+
+class Regressor(ivy.Module):
+ def __init__(self, input_dim, output_dim):
+ self.input_dim = input_dim
+ self.output_dim = output_dim
+ super().__init__()
+
+ def _build(self, *args, **kwargs):
+ self.linear0 = ivy.Linear(self.input_dim, 128)
+ self.linear1 = ivy.Linear(128, self.output_dim)
+
+ def _forward(self, x):
+ x = self.linear0(x)
+ x = ivy.functional.relu(x)
+ x = self.linear1(x)
+ return x
+
+ivy.set_backend('torch') # set backend to PyTorch (or any other backend!)
+
+model = Regressor(input_dim=1, output_dim=1)
+optimizer = ivy.Adam(0.3)
+
+n_training_examples = 2000
+noise = ivy.random.random_normal(shape=(n_training_examples, 1), mean=0, std=0.1)
+x = ivy.linspace(-6, 3, n_training_examples).reshape((n_training_examples, 1))
+y = 0.2 * x ** 2 + 0.5 * x + 0.1 + noise
+
+
+def loss_fn(v, x, target):
+ pred = model(x, v=v)
+ return ivy.mean((pred - target) ** 2)
+
+for epoch in range(40):
+ # forward pass
+ pred = model(x)
+
+ # compute loss and gradients
+ loss, grads = ivy.execute_with_gradients(lambda params: loss_fn(*params), (model.v, x, y))
+
+ # update parameters
+ model.v = optimizer.step(model.v, grads)
+
+ # print current loss
+ print(f'Epoch: {epoch + 1:2d} --- Loss: {ivy.to_numpy(loss).item():.5f}')
+
+print('Finished training!')
+```
+
+The model\'s output can be visualized as follows:
+
+
+
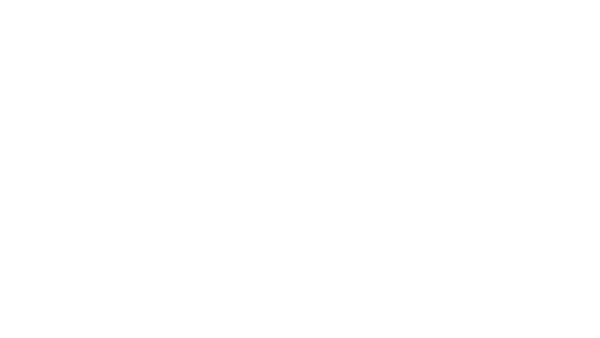
+
+
+As always, you can find more information about [Ivy as a framework in
+the
+docs!](https://unify.ai/docs/ivy/overview/design/ivy_as_a_framework.html)
+
+ When should I use Ivy as a framework?
+
+As Ivy supports multiple backends, writing code in Ivy breaks you free
+from framework limitations. If you want to publish highly flexible code
+for everyone to use, independently of the framework they are using, or
+you plan to develop ML-related tools and want them to be interoperable
+with not only the already existing frameworks, but also with future
+frameworks, then Ivy is for you!
+
+
+
+------------------------------------------------------------------------
+
+# Contributing
+
+
+We believe that everyone can contribute and make a difference. Whether
+it\'s writing code π», fixing bugs π, or simply sharing feedback π¬,
+your contributions are definitely welcome and appreciated π
+
+Check out all of our open tasks, and find out more info in our
+[Contributing
+guide](https://unify.ai/docs/ivy/overview/contributing.html) in the
+docs!
+
+Join our amazing community as a code contributor, and help accelerate
+our journey to unify all ML frameworks!
+
+
+
+
+
+------------------------------------------------------------------------
+
+# Community
+
+
+In order to achieve the ambitious goal of unifying AI, we definitely need
+as many hands as possible on it! Whether you are a seasoned developer or
+just starting out, you\'ll find a place here! Join the Ivy community on
+our [Discord](https://discord.gg/sXyFF8tDtm) πΎ server, which is the
+perfect place to ask questions, share ideas, and get help from both
+fellow developers and the Ivy Team directly!
+
+Also! Feel free to follow us on
+[Twitter](https://twitter.com/letsunifyai) π¦ as well, we use it to
+share updates, sneak peeks, and all sorts of relevant news, certainly a
+great way to stay in the loop π
+
+Can\'t wait to see you there!
+
+------------------------------------------------------------------------
+
+# Citation
+
+If you use Ivy for your work, please don\'t forget to give proper credit
+by including the accompanying [paper](https://arxiv.org/abs/2102.02886)
+π in your references. It\'s a small way to show appreciation and help
+to continue to support this and other open source projects π
+
+
+ @article{lenton2021ivy,
+ title={Ivy: Templated deep learning for inter-framework portability},
+ author={Lenton, Daniel and Pardo, Fabio and Falck, Fabian and James, Stephen and Clark, Ronald},
+ journal={arXiv preprint arXiv:2102.02886},
+ year={2021}
+ }
diff --git a/available_configs.json b/available_configs.json
index ec5cb7c7a81a8..da04c67cda05a 100644
--- a/available_configs.json
+++ b/available_configs.json
@@ -1,9 +1,11 @@
{
"compiler": [
- "cp38-none-manylinux_2_17_x86_64",
- "cp310-none-manylinux_2_17_x86_64"
+ "cp38-cp38-manylinux_2_17_x86_64",
+ "cp39-cp39-manylinux_2_17_x86_64",
+ "cp310-cp310-manylinux_2_17_x86_64",
+ "cp311-cp311-manylinux_2_17_x86_64"
],
"engines": [
- "cp310-none-manylinux_2_17_x86_64"
+ "cp310-cp310-manylinux_2_17_x86_64"
]
}
diff --git a/binaries.json b/binaries.json
index b63806177aaee..f77586c81524f 100644
--- a/binaries.json
+++ b/binaries.json
@@ -76,6 +76,7 @@
"creation.so",
"elementwise.so",
"general.so",
+ "ivy2xla.so",
"layers.so",
"linear_algebra.so",
"manipulation.so",
diff --git a/deploy_pypi.sh b/deploy_pypi.sh
deleted file mode 100644
index cf7548a06023a..0000000000000
--- a/deploy_pypi.sh
+++ /dev/null
@@ -1,2 +0,0 @@
-python3 -m build
-python3 -m twine upload dist/* -u "__token__" -p "$PYPI_PASSWORD" --verbose
diff --git a/docker/Dockerfile b/docker/Dockerfile
index 3accb0508e2a4..173982af80053 100644
--- a/docker/Dockerfile
+++ b/docker/Dockerfile
@@ -61,7 +61,7 @@ COPY /docker/requirement_mappings.json .
SHELL ["/bin/bash", "-c"]
# installing requirements based on mappings in location /opt/fw/$framework
-RUN jq -r 'to_entries[] | select(.value != [""]) | .key as $dir | .value[] | @sh "/opt/fw/\($dir) \(.)"' requirement_mappings.json | xargs -I {} sh -c 'printf "Installing %s\n" $2 && pip install --ignore-installed --target $1 $2' sh {}
+RUN jq -r 'to_entries[] | select(.value != [""]) | .key as $dir | .value[] | @sh "/opt/fw/\($dir) \(.)"' requirement_mappings.json | xargs -I {} sh -c 'printf "Installing %s\n" $2 && pip install --ignore-installed --target $1 $2 --extra-index-url https://download.pytorch.org/whl/cpu --no-cache-dir' sh {}
# install the requirements.txt, optional.txt with the mapped dependencies filtered out
RUN pip install --upgrade -r requirements.txt &&\
@@ -70,9 +70,9 @@ RUN pip install --upgrade -r requirements.txt &&\
# add all the directories to environment path so that python knows where to find them
-ENV PYTHONPATH "/opt/fw/mxnet:/opt/fw/numpy:/opt/fw/jax:/opt/fw/torch:/opt/fw/tensorflow:/opt/fw/paddle:/opt/miniconda/envs/multienv/bin"
+ENV PYTHONPATH "/opt/fw/mxnet:/opt/fw/numpy:/opt/fw/tensorflow:/opt/fw/jax:/opt/fw/torch:/opt/fw/paddle:/opt/miniconda/envs/multienv/bin"
-COPY run_tests_CLI/test_dependencies.py .
+COPY scripts/test_dependencies.py .
RUN python3 test_dependencies.py -fp requirements.txt,optional.txt && \
rm -rf requirements.txt && \
rm -rf optional.txt && \
diff --git a/docker/DockerfileAppleSilicon b/docker/DockerfileAppleSilicon
index 46fcb8f3c7618..b0d258ad19646 100644
--- a/docker/DockerfileAppleSilicon
+++ b/docker/DockerfileAppleSilicon
@@ -226,7 +226,7 @@ RUN pip install --upgrade -r requirements.txt &&\
# add all the directories to environment path so that python knows where to find them
ENV PYTHONPATH "/opt/fw/mxnet:/opt/fw/numpy:/opt/fw/jax:/opt/fw/torch:/opt/fw/tensorflow:/opt/fw/paddle"
-COPY run_tests_CLI/test_dependencies.py .
+COPY scripts/test_dependencies.py .
RUN python3 test_dependencies.py -fp requirements.txt && \
rm -rf requirements.txt && \
rm -rf optional_apple_silicon_1.txt && \
@@ -234,9 +234,3 @@ RUN python3 test_dependencies.py -fp requirements.txt && \
rm -rf tmp.txt && \
rm -rf test_dependencies.py && \
rm -rf requirement_mappings_apple_silicon.json
-
-# Post installation steps
-COPY .devcontainer/post_create_commands.sh .
-RUN chmod +x post_create_commands.sh && \
- bash post_create_commands.sh && \
- rm -rf post_create_commands.sh
diff --git a/docker/DockerfileGPU b/docker/DockerfileGPU
index 091bc9d04076c..5eca7bab1a6cc 100644
--- a/docker/DockerfileGPU
+++ b/docker/DockerfileGPU
@@ -1,129 +1,100 @@
-# BASE CUDA IMAGE #
-# ----------------#
-
-ARG UBUNTU_VERSION=20.04
-ARG CUDA=11.2
-FROM nvidia/cuda:${CUDA}.2-base-ubuntu${UBUNTU_VERSION} as base
+# installs multiple versions of cuda and cudnn and then installs the
+# latest frameworks and the requirements
+FROM debian:buster
WORKDIR /ivy
-# For TensorFlow #
-# ---------------#
-# Adapted from
-# https://github.com/tensorflow/tensorflow/blob/master/tensorflow/tools/dockerfiles/dockerfiles/gpu.Dockerfile
-
-# CUDA is specified again because the FROM directive resets ARGs
-# (but their default value is retained if set previously)
-ARG CUDA
-ARG CUDNN=8.1.0.77-1
-ARG CUDNN_MAJOR_VERSION=8
-ARG LIB_DIR_PREFIX=x86_64
-ARG LIBNVINFER=7.2.2-1
-ARG LIBNVINFER_MAJOR_VERSION=7
-
-# Let us install tzdata painlessly
-ENV DEBIAN_FRONTEND=noninteractive
+# arguments
+ARG fw
+ARG pycon=3.10
-# Needed for string substitution
-SHELL ["/bin/bash", "-c"]
-
-# taken from https://github.com/Kaggle/docker-python/commit/f1a3cfc6ee71899b1af8d76598b42a2da280448d
-RUN \
- # Temporarily swap the NVIDIA GPG key. Remove once new base image with new GPG key is released.
- rm /etc/apt/sources.list.d/cuda.list && \
- apt-key del 7fa2af80 && \
- apt-key adv --fetch-keys https://developer.download.nvidia.com/compute/cuda/repos/ubuntu2004/x86_64/7fa2af80.pub && \
- apt-get update
-
-# Refer to https://forums.developer.nvidia.com/t/notice-cuda-linux-repository-key-rotation/212772
-RUN apt-get update && apt-get install -y wget
-RUN wget https://developer.download.nvidia.com/compute/cuda/repos/ubuntu2004/x86_64/cuda-keyring_1.0-1_all.deb
-RUN dpkg -i cuda-keyring_1.0-1_all.deb
-RUN apt-get update
-
-# Pick up some TF dependencies
-RUN apt-get update && apt-get install -y --no-install-recommends \
- build-essential \
- cuda-command-line-tools-${CUDA/./-} \
- libcublas-${CUDA/./-} \
- cuda-nvrtc-${CUDA/./-} \
- libcufft-${CUDA/./-} \
- libcurand-${CUDA/./-} \
- libcusolver-${CUDA/./-} \
- libcusparse-${CUDA/./-} \
- curl \
- libcudnn8=${CUDNN}+cuda${CUDA} \
- libfreetype6-dev \
- libhdf5-serial-dev \
- libzmq3-dev \
- pkg-config \
- software-properties-common \
- unzip
-
-# Install TensorRT if not building for PowerPC
+# environment variables
+ENV DEBIAN_FRONTEND=noninteractive
+ENV TZ=Europe/Moscow
+ENV CONDA_DIR /opt/miniconda/
+
+
+# install base libraries
+RUN grep security /etc/apt/sources.list | tee /etc/apt/security.sources.list && \
+ apt-get update && \
+ apt-get upgrade -o Dir::Etc::SourceList=/etc/apt/security.sources.list -y &&\
+ apt-get -y update && \
+ apt-get install -y gnupg \
+ curl \
+ wget \
+ software-properties-common \
+ gcc \
+ nano
+
+
+# install miniconda
+RUN apt clean && \
+ rm -rf /var/lib/apt/lists/* && \
+ apt-get update && \
+ apt-get install -y wget && \
+ apt-get install -y jq && \
+ apt-get install git -y && \
+ wget --quiet https://repo.anaconda.com/miniconda/Miniconda3-latest-Linux-x86_64.sh -O ~/miniconda.sh && \
+ /bin/bash ~/miniconda.sh -b -p /opt/miniconda
+
+
+# create conda environment
+ENV PATH=$CONDA_DIR/bin:$PATH
+RUN conda create --name multienv python==$pycon -y
+
+
+# fix protobuf conflicts
+ENV PROTOCOL_BUFFERS_PYTHON_IMPLEMENTATION python
+ENV PATH=/opt/miniconda/envs/multienv/bin:$PATH
RUN apt-get update && \
- apt-key adv --fetch-keys https://developer.download.nvidia.com/compute/machine-learning/repos/ubuntu1804/x86_64/7fa2af80.pub && \
- echo "deb https://developer.download.nvidia.com/compute/machine-learning/repos/ubuntu1804/x86_64 /" > /etc/apt/sources.list.d/tensorRT.list && \
- apt-get update && \
- apt-get install -y --no-install-recommends libnvinfer${LIBNVINFER_MAJOR_VERSION}=${LIBNVINFER}+cuda11.0 \
- libnvinfer-plugin${LIBNVINFER_MAJOR_VERSION}=${LIBNVINFER}+cuda11.0 \
- && apt-get clean \
- && rm -rf /var/lib/apt/lists/*
-
-# For CUDA profiling, TensorFlow requires CUPTI.
-ENV LD_LIBRARY_PATH /usr/local/cuda-11.0/targets/x86_64-linux/lib:/usr/local/cuda/extras/CUPTI/lib64:/usr/local/cuda/lib64:$LD_LIBRARY_PATH
-
-# Link the libcuda stub to the location where tensorflow is searching for it and reconfigure
-# dynamic linker run-time bindings
-RUN ln -s /usr/local/cuda/lib64/stubs/libcuda.so /usr/local/cuda/lib64/stubs/libcuda.so.1 \
- && echo "/usr/local/cuda/lib64/stubs" > /etc/ld.so.conf.d/z-cuda-stubs.conf \
- && ldconfig
+ apt-get install -y python3-pip python3-tk && \
+ apt-get install -y libsm6 libxext6 libxrender-dev libgl1-mesa-glx && \
+ apt-get install -y git && \
+ apt-get install -y rsync && \
+ apt-get install -y libusb-1.0-0 && \
+ apt-get install -y libglib2.0-0 && \
+ pip3 install --upgrade pip && \
+ pip3 install setuptools==58.5.3
+
+
+# install Ivy Upstream
+RUN git clone --progress --recurse-submodules https://github.com/unifyai/ivy --depth 1 && \
+ cd ivy && \
+ cd ivy_tests/array_api_testing/test_array_api && \
+ pip3 install --no-cache-dir -r requirements.txt
-# See http://bugs.python.org/issue19846
-ENV LANG C.UTF-8
-RUN apt-get update && apt-get install -y \
- python3 \
- python3-pip
-RUN pip3 install --upgrade pip
+# copy library files to workdir
+COPY docker/gpu_framework_directory.py .
+COPY requirements/optional_gpu.txt .
+COPY requirements/requirements.txt .
-RUN python3 -m pip --no-cache-dir install --upgrade \
- pip \
- setuptools==58.5.3
-# Some TF tools expect a "python" binary
-RUN ln -s $(which python3) /usr/local/bin/python
+# setting torch path early on because torch-scatter needs it
+ENV PYTHONPATH "/opt/fw/torch:/opt/miniconda/envs/multienv/bin"
-RUN apt-get install -y git
-RUN apt-get install -y python-opengl
-# Ivy #
-# ----#
+# requirement mappings directs which dependency to be installed and where
+COPY /docker/requirement_mappings_gpu.json .
+SHELL ["/bin/bash", "-c"]
-# Make sure torch installed before torch-scatter (in optional_gpu.txt)
-RUN pip install --no-cache-dir torch==1.11.0+cu113 --extra-index-url https://download.pytorch.org/whl/cu113
-# Install Ivy Upstream
-RUN git clone --recurse-submodules https://github.com/unifyai/ivy --depth 1 && \
- cd ivy && \
- cat requirements/requirements.txt | grep -v "ivy-" | pip3 install --no-cache-dir -r /dev/stdin && \
- cat requirements/optional_gpu.txt | grep -v "ivy-" | pip3 install --no-cache-dir -r /dev/stdin && \
- python3 -m pip install --user -e . && \
- cd ivy_tests/array_api_testing/test_array_api && \
- pip3 install --no-cache-dir -r requirements.txt
+# install all libraries based on the mappings
+RUN python3 gpu_framework_directory.py $fw &&\
+ jq -r 'to_entries[] | select(.value != [""]) | .key as $dir | .value[] | @sh "/opt/fw/\($dir) \(.)"' requirement_mappings_gpu.json | xargs -I {} sh -c 'printf "Installing %s\n" $2 && pip install --ignore-installed --target $1 $2 --extra-index-url https://download.pytorch.org/whl/cu118' sh {}
+RUN sed -i '/numpy/d' requirements.txt &&\
+ pip install -r requirements.txt &&\
+ cp ./optional_gpu.txt tmp.txt &&\
+ jq -r 'to_entries[] | [.key] + .value | select(length > 0 or (. == "")) | .[]' requirement_mappings_gpu.json | sort -u | xargs -I {} sed -i '/{}/d;/jaxlib/d' tmp.txt && pip install -r tmp.txt
-# Install local requirements
-COPY requirements/requirements.txt .
-RUN pip3 install --no-cache-dir -r requirements.txt
-# Install local optional
-COPY requirements/optional_gpu.txt .
-RUN pip3 install --no-cache-dir -r optional_gpu.txt
+# add all the directories to environment path so that python knows where to find them
+ENV PYTHONPATH "/opt/fw/mxnet:/opt/fw/numpy:/opt/fw/tensorflow:/opt/fw/jax:/opt/fw/torch:/opt/fw/paddle:/opt/miniconda/envs/multienv/bin"
-# Install jax cuda after optional_gpu.txt, otherwise cpu version will override
-RUN pip install --no-cache-dir jaxlib -f https://storage.googleapis.com/jax-releases/jax_cuda_releases.html
-COPY run_tests_CLI/test_dependencies.py .
+# test dependencies
+COPY scripts/test_dependencies.py .
RUN python3 test_dependencies.py -fp requirements.txt,optional_gpu.txt && \
rm -rf requirements.txt && \
+ rm -rf tmp.txt && \
rm -rf optional_gpu.txt && \
rm -rf test_dependencies.py
diff --git a/docker/DockerfileGPUMultiCuda b/docker/DockerfileGPUMultiCuda
deleted file mode 100644
index cee4cbf731a9e..0000000000000
--- a/docker/DockerfileGPUMultiCuda
+++ /dev/null
@@ -1,84 +0,0 @@
-# uses the base image which has cuda and cudnn installed(multiple versions) and then installs the
-# latest frameworks and the requirements
-FROM unifyai/multicuda:base
-WORKDIR /ivy
-ARG fw
-
-ARG pycon=3.10
-
-ENV DEBIAN_FRONTEND=noninteractive
-
-# Install miniconda
-ENV CONDA_DIR /opt/miniconda/
-
-RUN apt clean && \
- rm -rf /var/lib/apt/lists/* && \
- apt-get update && \
- apt-get install -y wget && \
- apt-get install -y jq && \
- apt-get install git -y && \
- wget --quiet https://repo.anaconda.com/miniconda/Miniconda3-latest-Linux-x86_64.sh -O ~/miniconda.sh && \
- /bin/bash ~/miniconda.sh -b -p /opt/miniconda
-
-
-ENV PATH=$CONDA_DIR/bin:$PATH
-RUN conda create --name multienv python==$pycon -y
-
-# to fix protobuf conflicts
-ENV PROTOCOL_BUFFERS_PYTHON_IMPLEMENTATION python
-ENV PATH=/opt/miniconda/envs/multienv/bin:$PATH
-RUN apt-get update && \
- apt-get install -y python3-pip python3-tk && \
- apt-get install -y libsm6 libxext6 libxrender-dev libgl1-mesa-glx && \
- apt-get install -y git && \
- apt-get install -y rsync && \
- apt-get install -y libusb-1.0-0 && \
- apt-get install -y libglib2.0-0 && \
- pip3 install --upgrade pip && \
- pip3 install setuptools==58.5.3
-
-
-# Install Ivy Upstream
-RUN git clone --progress --recurse-submodules https://github.com/unifyai/ivy --depth 1 && \
- cd ivy && \
- cd ivy_tests/array_api_testing/test_array_api && \
- pip3 install --no-cache-dir -r requirements.txt
-
-# Install local optional
-COPY /docker/multicuda_framework_directory.py .
-COPY requirements/optional_gpu.txt .
-COPY requirements/requirements.txt .
-
-
-#setting torch path early on because torch-scatter needs it
-ENV PYTHONPATH "/opt/fw/torch:/opt/miniconda/envs/multienv/bin"
-
-# requirement mappings directs which dependency to be installed and where
-COPY /docker/requirement_mappings_gpu.json .
-SHELL ["/bin/bash", "-c"]
-
-
-
-RUN python3 multicuda_framework_directory.py $fw &&\
- jq -r 'to_entries[] | select(.value != [""]) | .key as $dir | .value[] | @sh "/opt/fw/\($dir) \(.)"' requirement_mappings_gpu.json | xargs -I {} sh -c 'printf "Installing %s\n" $2 && pip install --ignore-installed --target $1 $2' sh {}
-
-
-
-RUN sed -i '/numpy/d' requirements.txt &&\
- pip install -r requirements.txt &&\
- cp ./optional_gpu.txt tmp.txt &&\
- jq -r 'to_entries[] | [.key] + .value | select(length > 0 or (. == "")) | .[]' requirement_mappings_gpu.json | sort -u | xargs -I {} sed -i '/{}/d;/jaxlib/d' tmp.txt && pip install -r tmp.txt
-
-
-
-
-# add all the directories to environment path so that python knows where to find them
-ENV PYTHONPATH "/opt/fw/mxnet:/opt/fw/numpy:/opt/fw/jax:/opt/fw/torch:/opt/fw/tensorflow:/opt/fw/paddle:/opt/miniconda/envs/multienv/bin"
-
-
-COPY run_tests_CLI/test_dependencies.py .
-RUN python3 test_dependencies.py -fp requirements.txt,optional_gpu.txt && \
- rm -rf requirements.txt && \
- rm -rf tmp.txt && \
- rm -rf optional_gpu.txt && \
- rm -rf test_dependencies.py
diff --git a/docker/DockerfileGPUMultiCuda-base b/docker/DockerfileGPUMultiCuda-base
deleted file mode 100644
index fa8c4c9895f53..0000000000000
--- a/docker/DockerfileGPUMultiCuda-base
+++ /dev/null
@@ -1,20 +0,0 @@
-# is used to create a base image where we then manually install cuda and cudnn
-FROM debian:buster
-WORKDIR /ivy
-
-
-COPY ../docker/multicuda_framework_directory.py .
-COPY ../docker/multicuda_requirements.txt .
-
-ENV DEBIAN_FRONTEND=noninteractive
-ENV TZ=Europe/Moscow
-RUN grep security /etc/apt/sources.list | tee /etc/apt/security.sources.list && \
- apt-get update && \
- apt-get upgrade -o Dir::Etc::SourceList=/etc/apt/security.sources.list -y &&\
- apt-get -y update && \
- apt-get install -y gnupg \
- curl \
- wget \
- software-properties-common \
- gcc \
- nano
diff --git a/docker/build_DockerfileGPUMultiCuda.sh b/docker/build_DockerfileGPUMultiCuda.sh
deleted file mode 100644
index f9e0ff9da5d9f..0000000000000
--- a/docker/build_DockerfileGPUMultiCuda.sh
+++ /dev/null
@@ -1 +0,0 @@
-docker build --progress=plain --no-cache -t unifyai/multicuda:base_and_requirements -f DockerfileGPUMultiCuda ..
diff --git a/docker/build_gpu_dockerfile.sh b/docker/build_gpu_dockerfile.sh
new file mode 100644
index 0000000000000..ec946c8b97e89
--- /dev/null
+++ b/docker/build_gpu_dockerfile.sh
@@ -0,0 +1 @@
+docker build --progress=plain --no-cache -t unifyai/ivy:latest-gpu -f DockerfileGPU ..
diff --git a/docker/multicuda_framework_directory.py b/docker/gpu_framework_directory.py
similarity index 99%
rename from docker/multicuda_framework_directory.py
rename to docker/gpu_framework_directory.py
index 5c1ca2034c35e..e4e185475ea85 100644
--- a/docker/multicuda_framework_directory.py
+++ b/docker/gpu_framework_directory.py
@@ -45,7 +45,7 @@ def install_pkg(path, pkg, base="fw/"):
)
elif pkg.split("==")[0] if "==" in pkg else pkg == "jax":
subprocess.run(
- f"yes |pip install --upgrade --target {path} 'jax[cuda11_local]' -f"
+ f"yes |pip install --upgrade --target {path} 'jax[cuda11_pip]' -f"
" https://storage.googleapis.com/jax-releases/jax_cuda_releases.html "
" --no-cache-dir",
shell=True,
diff --git a/docker/multiversion_framework_directory.py b/docker/multiversion_framework_directory.py
index c29d525da9f36..8b071b1d252d7 100644
--- a/docker/multiversion_framework_directory.py
+++ b/docker/multiversion_framework_directory.py
@@ -44,7 +44,7 @@ def install_deps(pkgs, path_to_json, base="/opt/fw/"):
fw, ver = fw.split("/")
path = base + fw + "/" + ver
# check to see if this pkg has specific version dependencies
- with open(path_to_json) as file:
+ with open(path_to_json, "r") as file:
json_data = json.load(file)
for keys in json_data[fw]:
# check if key is dict
diff --git a/docker/requirement_mappings.json b/docker/requirement_mappings.json
index f18918623b96f..61b4ad87e17a5 100644
--- a/docker/requirement_mappings.json
+++ b/docker/requirement_mappings.json
@@ -1,6 +1,6 @@
{
"tensorflow": ["tensorflow-cpu", "tensorflow-probability"],
- "jax": ["jax[cpu]","dm-haiku", "flax", "jaxlib"],
+ "jax": ["ml-dtypes","jax[cpu]","dm-haiku", "flax", "jaxlib"],
"numpy": ["numpy"],
"paddle": ["paddlepaddle"],
"mxnet": ["mxnet"],
diff --git a/docker/requirement_mappings_multiversion.json b/docker/requirement_mappings_multiversion.json
index 85dd54daaa0cb..7eaa8101d5730 100644
--- a/docker/requirement_mappings_multiversion.json
+++ b/docker/requirement_mappings_multiversion.json
@@ -11,16 +11,18 @@
"jax": [
"dm-haiku",
"flax",
-
{
"jaxlib": {
+ "0.4.17": "0.4.17",
"0.4.14": "0.4.14",
"0.4.10": "0.4.10",
"0.4.8": "0.4.7"
}
},
- {"ml_dtypes":{"0.4.10":"0.2.0"}
-
+ {
+ "ml_dtypes": {
+ "0.4.10": "0.2.0"
+ }
}
],
"numpy": [
@@ -33,6 +35,7 @@
"mxnet"
],
"torch": [
- "torch-scatter", "torchvision"
+ "torch-scatter",
+ "torchvision"
]
}
diff --git a/docs/demos b/docs/demos
index 4f73be9fe9b27..2bf43d222424b 160000
--- a/docs/demos
+++ b/docs/demos
@@ -1 +1 @@
-Subproject commit 4f73be9fe9b271dfee6b37e0d551afb848baf781
+Subproject commit 2bf43d222424bacfe88ed5746870d5ba5528aed1
diff --git a/docs/overview/contributing/building_the_docs.rst b/docs/overview/contributing/building_the_docs.rst
index 5ce33253bd572..c695e0accd5d4 100644
--- a/docs/overview/contributing/building_the_docs.rst
+++ b/docs/overview/contributing/building_the_docs.rst
@@ -5,6 +5,21 @@ This document describes how to build the Ivy docs. If you want to know more abou
our custom building pipeline work, check our `Building the Docs Pipeline
<../deep_dive/building_the_docs_pipeline.rst>`_ deep dive
+.. warning::
+
+ Be aware that the doc-builder was developed originally for Linux, although, in theory, you can run
+ it on any platform (supporting either docker or windows), it's only tested it on
+ Linux. If you find any windows related issues, feel free to open an issue for that to review it.
+
+.. note::
+
+ Recommendation:
+ You can use the convenience script if you build the docs regularly,
+ as it will not re-download the dependencies.
+
+ If you have a slow internet connection, you can use GitHub Codespaces since it will help you to build the
+ docs faster since our script downloads large dependency files.
+
Building the Docs using Docker
------------------------------
diff --git a/docs/overview/contributing/error_handling.rst b/docs/overview/contributing/error_handling.rst
index be30a9796d38c..2ddfa6be2c4ca 100644
--- a/docs/overview/contributing/error_handling.rst
+++ b/docs/overview/contributing/error_handling.rst
@@ -26,7 +26,7 @@ This section, "Error Handling" aims to assist you in navigating through some com
E with_out=False,
E instance_method=False,
E test_gradients=False,
- E test_compile=None,
+ E test_trace=None,
E as_variable=[False],
E native_arrays=[False],
E container=[False],
@@ -65,7 +65,7 @@ This section, "Error Handling" aims to assist you in navigating through some com
E with_out=False,
E instance_method=False,
E test_gradients=True,
- E test_compile=None,
+ E test_trace=None,
E as_variable=[False],
E native_arrays=[False],
E container=[False],
@@ -129,7 +129,7 @@ This section, "Error Handling" aims to assist you in navigating through some com
E with_out=False,
E instance_method=False,
E test_gradients=False,
- E test_compile=None,
+ E test_trace=None,
E as_variable=[False],
E native_arrays=[False],
E container=[False],
diff --git a/docs/overview/contributing/setting_up.rst b/docs/overview/contributing/setting_up.rst
index adbf2319b2a57..0b6c0535dbc93 100644
--- a/docs/overview/contributing/setting_up.rst
+++ b/docs/overview/contributing/setting_up.rst
@@ -9,7 +9,9 @@ Setting Up
.. _`pip packages channel`: https://discord.com/channels/799879767196958751/942114789642080317
.. _`miniconda`: https://docs.conda.io/en/latest/miniconda.html
.. _`venv`: https://docs.python.org/3/library/venv.html
-.. _`ivy/run_tests_CLI`: https://github.com/unifyai/ivy/tree/f71a414417646e1dfecb5de27fb555f80333932c/run_tests_CLI
+.. _`ivy/scripts`: https://github.com/unifyai/ivy/tree/bcddc79978afe447958dfa3ea660716845c85846/scripts
+.. _`platform compatibility tags`: https://packaging.python.org/en/latest/specifications/platform-compatibility-tags/
+.. _`logging level`: https://docs.python.org/3/library/logging.html#logging.Logger.setLevel
We're really happy you'd like to learn how to contribute towards Ivy π
@@ -120,7 +122,7 @@ Using miniconda
.. code-block:: none
- pip install e .
+ pip install -e .
#. Setup the interpreter by:
@@ -430,7 +432,7 @@ Ubuntu
d. Choosing "Docker" from the left panel.
Type python3 (with the number) in python interpreter path and press ok.
-**Docker Connection not Successfull**
+**Docker Connection not Successful**
This is a common error which you might face. If you are not successfully able to connect docker with Pycharm(point 4a) and your docker is also running, the issue is that you are not able to use your docker socket. So, executing the below two commands should solve this.
@@ -486,31 +488,31 @@ Click this and you should see a progress bar of all the tests running in the fil
:width: 420
It is also possible to run the entire set of ivy tests or the array api test suite using pre-written shell scripts that can be run from the 'Terminal' tab in PyCharm.
-There are a number of such shell scripts in `ivy/run_tests_CLI`_:
+There are a number of such shell scripts in `ivy/scripts`_:
.. code-block:: bash
:emphasize-lines: 4,5,8,9,10
- run_ivy_core_test.py
- run_ivy_nn_test.py
- run_ivy_stateful_test.py
- run_tests.sh
- test_array_api.sh
- test_dependencies.py
- test_dependencies.sh
- test_ivy_core.sh
- test_ivy_nn.sh
- test_ivy_stateful.sh
+ scripts/setup_tests/run_ivy_core_test.py
+ scripts/setup_tests/run_ivy_nn_test.py
+ scripts/setup_tests/run_ivy_stateful_test.py
+ scripts/shell/run_tests.sh
+ scripts/shell/test_array_api.sh
+ scripts/test_dependencies.py
+ scripts/shell/test_dependencies.sh
+ scripts/shell/test_ivy_core.sh
+ scripts/shell/test_ivy_nn.sh
+ scripts/shell/test_ivy_stateful.sh
**For Unix-based systems (Linux and macOS):**
-* :code:`run_tests.sh` is run by typing :code:`./run_tests_CLI/run_tests.sh` in the :code:`/ivy` directory.
+* :code:`scripts/shell/run_tests.sh` is run by typing :code:`./scripts/shell/run_tests.sh` in the :code:`/ivy` directory.
This runs all tests in :code:`ivy/ivy_tests`.
-* :code:`test_array_api.sh` is run by typing :code:`./test_array_api.sh [backend] test_[submodule]`.
+* :code:`scripts/shell/test_array_api.sh` is run by typing :code:`./scripts/shell/test_array_api.sh [backend] test_[submodule]`.
This runs all array-api tests for a certain submodule in a certain backend.
-* :code:`test_ivy_core.sh` is run by typing :code:`./run_tests_CLI/test_ivy_core.sh [backend] test_[submodule]` in the ivy directory.
+* :code:`scripts/shell/test_ivy_core.sh` is run by typing :code:`./scripts/shell/test_ivy_core.sh [backend] test_[submodule]` in the ivy directory.
This runs all ivy tests for a certain submodule in a certain backend in :code:`test_ivy/test_functional/test_core`.
-* :code:`test_ivy_nn.sh`, :code:`test_ivy_stateful.sh` are run in a similar manner to :code:`test_ivy_core.sh`.
+* :code:`scripts/shell/test_ivy_nn.sh`, :code:`scripts/shell/test_ivy_stateful.sh` are run in a similar manner to :code:`scripts/shell/test_ivy_core.sh`.
Make sure to check the submodule names in the source code before running.
.. image:: https://github.com/unifyai/unifyai.github.io/blob/main/img/externally_linked/contributing/setting_up/setting_up_testing/pycharm_run_array_api_tests.png?raw=true
@@ -522,19 +524,19 @@ There are a number of such shell scripts in `ivy/run_tests_CLI`_:
For Windows users, you may need to specify that the shell scripts should be run by :code:`sh`, which comes with Git. In the Terminal, prepend sh to the script commands like so:
-* To run :code:`run_tests.sh` on Windows, type :code:`sh ./run_tests_CLI/run_tests.sh` in the :code:`/ivy` directory.
+* To run :code:`scripts/shell/run_tests.sh` on Windows, type :code:`sh ./scripts/shell/run_tests.sh` in the :code:`/ivy` directory.
This runs all tests in :code:`ivy/ivy_tests`.
-* To run :code:`test_array_api.sh` on Windows, type :code:`sh ./test_array_api.sh [backend] test_[submodule]`.
+* To run :code:`scripts/shell/test_array_api.sh` on Windows, type :code:`sh ./scripts/shell/test_array_api.sh [backend] test_[submodule]`.
This runs all array-api tests for a certain submodule in a certain backend.
-* To run :code:`test_ivy_core.sh` on Windows, type :code:`sh ./run_tests_CLI/test_ivy_core.sh [backend] test_[submodule]` in the ivy directory.
+* To run :code:`scripts/shell/test_ivy_core.sh` on Windows, type :code:`sh ./scripts/shell/test_ivy_core.sh [backend] test_[submodule]` in the ivy directory.
This runs all ivy tests for a certain submodule in a certain backend in :code:`test_ivy/test_functional/test_core`.
-* :code:`test_ivy_nn.sh`, :code:`test_ivy_stateful.sh` are run in a similar manner to :code:`test_ivy_core.sh` on Windows.
+* :code:`scripts/shell/test_ivy_nn.sh`, :code:`scripts/shell/test_ivy_stateful.sh` are run in a similar manner to :code:`scripts/shell/test_ivy_core.sh` on Windows.
Make sure to check the submodule names in the source code before running.
The above instructions for running tests on Windows assume that you have installed Git and have access to the Git Bash terminal. If you do not have Git Bash, you can download it from the `official Git website `_.
-If you wish to run tests of all submodules of `ivy_core`, `ivy_nn` or `ivy_stateful`, there are :code:`.py` available in :code:`run_tests_CLI`.
-All are run like: :code:`python run_tests_CLI/run_ivy_nn_test.py 1`, where 1 = numpy, 2 = torch, 3 = jax, and 4 = tensorflow.
+If you wish to run tests of all submodules of `ivy_core`, `ivy_nn` or `ivy_stateful`, there are :code:`.py` available in :code:`scripts/shell`.
+All are run like: :code:`python scripts/setup_tests/run_ivy_nn_test.py 1`, where 1 = numpy, 2 = torch, 3 = jax, and 4 = tensorflow.
More Detailed Hypothesis Logs in PyCharm
@@ -760,7 +762,7 @@ If you want to setup a GPU instance on codespaces and also have access to it, ki
.. image:: https://raw.githubusercontent.com/unifyai/unifyai.github.io/main/img/externally_linked/contributing/setting_up/github_codespaces/Selecting_the_GPU.png?raw=true
:width: 420
-2. Refer to the ref:`Setting up Codespaces` section for the other configurations such as the "Dev conatiner configuration". Your Machine Type section will look like the following image shown below. Feel free to click on the green button to create the instance.
+2. Refer to the ref:`Setting up Codespaces` section for the other configurations such as the "Dev container configuration". Your Machine Type section will look like the following image shown below. Feel free to click on the green button to create the instance.
.. image:: https://raw.githubusercontent.com/unifyai/unifyai.github.io/main/img/externally_linked/contributing/setting_up/github_codespaces/Interface_after_selecting_the_GPU_1.png?raw=true
:width: 420
@@ -835,6 +837,49 @@ The steps are as following to setup testing on VS Code when using a new Codespac
Note: Currently you do not need to comment out the :code:`conftest.py` file in the :code:`array_api_tests` directory.
+
+The Binaries
+------------
+
+Some features in :code:`ivy` are served as compiled binaries, such as the transpiler.
+These binaries aren't maintained in the :code:`ivy` repository directly, but on a separate :code:`binaries` repository.
+All the binaries that are required to make use of the full potential of :code:`ivy` are recorded in the :code:`binaries.json`.
+The supported configurations (Python version - OS - Architecture) are recorded in the :code:`available_configs.json`.
+
+The format of representing a configuration is based on PyPI's `platform compatibility tags`_,
+meaning :code:`cp310-none-manylinux_2_17_x86_64` represents a configuration that can be used in a Python 3.10 environment on a linux system with x86-64.
+We continue to add support to many more supported configurations to our binaries to work with various python versions, OS and architecture.
+
+On installing :code:`ivy` with :code:`pip install -e .` all the required binaries with a supported configuration to your system get downloaded.
+Just to have another check on whether all binaries are present, there's a warning that gets thrown when you :code:`import ivy` if any binaries are missing of the form,
+
+.. code-block:: none
+
+ WARNING:root: Some binaries seem to be missing in your system. This could be either because we don't have compatible binaries for your system or that newer binaries were available.
+ In the latter case, calling ivy.utils.cleanup_and_fetch_binaries() should fetch the binaries binaries. Feel free to create an issue on https://github.com/unifyai/ivy.git in case of the former
+
+ WARNING:root:
+ Following are the supported configurations :
+ compiler : cp38-none-manylinux_2_17_x86_64, cp310-none-manylinux_2_17_x86_64
+ engines : cp310-none-manylinux_2_17_x86_64
+
+ WARNING:root: /workspaces/ivy/ivy/compiler/_compiler.so not found.
+
+In case there are no supported binaries for your configuration, then feel free to create an issue on the :code:`ivy` repo asking for adding support to the same.
+Feel free to ignore the warning in the meantime, set a `logging level`_ to avoid receiving the warning.
+In case the you are using a supported configuration and still receiving this warning, it indicates that you are yet to do a :code:`pip install -e .` as mentioned in the previous sections.
+Running a :code:`pip install -e .` is sufficient to download the binaries if they're supported but the :func:`ivy.utils.cleanup_and_fetch_binaries` function is provided just in case you want to download the binaries without a local installation.
+
+.. code-block:: python
+
+ import ivy
+
+ ivy.utils.cleanup_and_fetch_binaries()
+
+
+.. note:: Bear in mind that the binaries are **not** required for working on the open tasks for the most part, so it's totally fine to not have the binaries downloaded on your system for working on any of the open tasks.
+
+
**Video**
.. raw:: html
diff --git a/docs/overview/contributing/the_basics.rst b/docs/overview/contributing/the_basics.rst
index 96de0bfc02e9c..dd43ce0ae0a19 100644
--- a/docs/overview/contributing/the_basics.rst
+++ b/docs/overview/contributing/the_basics.rst
@@ -129,9 +129,9 @@ This can be done using:
The main branch then simply has the role of being kept up to date with upstream.
You *can* create PRs based on the main branch of your fork, but this will make things more complicated if you would then like to create additional PRs in the future.
-For keeping any branch on your fork up to date, there is a script in the root folder of the repo `merge_with_upstream.sh `_.
-To update your fork's branch to the upstream main branch, simply run :code:`./merge_with_upstream.sh name_of_your_branch`.
-To update the main branch, this would then be: :code:`./merge_with_upstream.sh main`.
+For keeping any branch on your fork up to date, there is a script in the root folder of the repo `scripts/shell/merge_with_upstream.sh `_.
+To update your fork's branch to the upstream main branch, simply run :code:`./scripts/shell/merge_with_upstream.sh name_of_your_branch`.
+To update the main branch, this would then be: :code:`./scripts/shell/merge_with_upstream.sh main`.
When making a PR (explained in the next sub-section), sometimes you will see that changes to upstream have caused conflicts with your PR.
In this case, you will need to either resolve these conflicts in the browser, or clone your fork and make changes locally in the terminal and push once resolved.
diff --git a/docs/overview/deep_dive.rst b/docs/overview/deep_dive.rst
index 024dfd599ad43..aaa63055ea4b5 100644
--- a/docs/overview/deep_dive.rst
+++ b/docs/overview/deep_dive.rst
@@ -115,3 +115,4 @@ We're excited for you to get involved! π¦Ύ
deep_dive/gradients.rst
deep_dive/operating_modes.rst
deep_dive/building_the_docs_pipeline.rst
+ deep_dive/fix_failing_tests.rst
diff --git a/docs/overview/deep_dive/array_api_tests.rst b/docs/overview/deep_dive/array_api_tests.rst
index e9225fedc5c83..3a6aa29a8d67b 100644
--- a/docs/overview/deep_dive/array_api_tests.rst
+++ b/docs/overview/deep_dive/array_api_tests.rst
@@ -8,7 +8,7 @@ Array API Tests
.. _`repo`: https://github.com/unifyai/ivy
.. _`discord`: https://discord.gg/sXyFF8tDtm
.. _`array api tests channel`: https://discord.com/channels/799879767196958751/982738404611592256
-.. _`test_array_api.sh`: https://github.com/unifyai/ivy/blob/d76f0f5ab02d608864eb2c4012af2404da5806c2/test_array_api.sh
+.. _`scripts/shell/test_array_api.sh`: https://github.com/unifyai/ivy/blob/bcddc79978afe447958dfa3ea660716845c85846/scripts/shell/test_array_api.sh
.. _`array-api test repository`: https://github.com/data-apis/array-api/tree/main
.. _`issue`: https://github.com/numpy/numpy/issues/21213
.. _`ivy_tests/array_api_testing/test_array_api/array_api_tests/test_special_cases.py`: https://github.com/data-apis/array-api-tests/blob/ddd3b7a278cd0c0b68c0e4666b2c9f4e67b7b284/array_api_tests/test_special_cases.py
@@ -30,7 +30,7 @@ Instead, the change must be made to the array-api repository directly and then o
.. code-block:: none
- # to initialise local config file and fetch + checkout submodule (not needed everytime)
+ # to initialise local config file and fetch + checkout submodule (not needed every time)
git submodule update --init --recursive
# pulls changes from the upstream remote repo and merges them
@@ -62,12 +62,12 @@ There are two ways to do this: using the terminal or using your IDE.
Using Terminal
**************
-Using the terminal, you can run all array-api tests in a given file for a certain backend using the bash file `test_array_api.sh`_:
+Using the terminal, you can run all array-api tests in a given file for a certain backend using the bash file `scripts/shell/test_array_api.sh`_:
.. code-block:: none
# /ivy
- /bin/bash -e ./run_tests_CLI/test_array_api.sh jax test_linalg
+ /bin/bash -e ./scripts/shell/scripts/shell/test_array_api.sh jax test_linalg
You can change the argument with any of our supported frameworks - tensorflow, numpy, torch, or jax - and the individual test function categories in :code:`ivy/ivy_tests/array_api_testing/test_array_api/array_api_tests`, e.g. *test_set_functions*, *test_signatures* etc.
diff --git a/docs/overview/deep_dive/backend_setting.rst b/docs/overview/deep_dive/backend_setting.rst
index b2be3a2f0a7a5..6a7565ec335f0 100644
--- a/docs/overview/deep_dive/backend_setting.rst
+++ b/docs/overview/deep_dive/backend_setting.rst
@@ -57,22 +57,19 @@ In addition, all the previously set backends can be cleared by calling :func:`iv
Dynamic Backend Setting
-----------------------
-.. _`ivy.set_dynamic_backend`: https://github.com/unifyai/ivy/blob/main/ivy/__init__.py#L1134.
-.. _`ivy.unset_dynamic_backend`: https://github.com/unifyai/ivy/blob/main/ivy/__init__.py#L1143.
-.. _`ivy.dynamic_backend_as`: https://github.com/unifyai/ivy/blob/main/ivy/__init__.py#L1174.
-.. _`ivy.Array`: https://github.com/unifyai/ivy/blob/main/ivy/data_classes/array/array.py#L186.
-.. _`ivy.Container`: https://github.com/unifyai/ivy/blob/main/ivy/data_classes/container/base.py#L4166.
-.. _`converted to numpy`: https://github.com/unifyai/ivy/blob/main/ivy/utils/backend/handler.py#L283.
-.. _`converted from numpy`: https://github.com/unifyai/ivy/blob/main/ivy/utils/backend/handler.py#L363.
+.. _`ivy.set_dynamic_backend`: https://github.com/unifyai/ivy/blob/e2b0b1d7fcd454f12bfae94b03213457460276c8/ivy/__init__.py#L1150.
+.. _`ivy.unset_dynamic_backend`: https://github.com/unifyai/ivy/blob/e2b0b1d7fcd454f12bfae94b03213457460276c8/ivy/__init__.py#L1187.
+.. _`ivy.dynamic_backend_as`: https://github.com/unifyai/ivy/blob/e2b0b1d7fcd454f12bfae94b03213457460276c8/ivy/__init__.py#L1190.
+.. _`ivy.Array`: https://github.com/unifyai/ivy/blob/e2b0b1d7fcd454f12bfae94b03213457460276c8/ivy/data_classes/array/array.py#L190.
+.. _`ivy.Container`: https://github.com/unifyai/ivy/blob/e2b0b1d7fcd454f12bfae94b03213457460276c8/ivy/data_classes/container/base.py#L4285.
+.. _`dynamic_backend_converter`: https://github.com/unifyai/ivy/blob/e2b0b1d7fcd454f12bfae94b03213457460276c8/ivy/utils/backend/handler.py#L252.
Working with different backends in Ivy can be challenging, especially when you need to switch between backends frequently.
To make this easier, users can make use of the dynamic backend attribute of :class:`ivy.Array` and :class:`ivy.Container` classes which allow you to automatically convert ivy arrays to the new backend whenever the backend is changed.
Essentially, when the user calls :code:`ivy.set_backend(, dynamic=True)`, the following steps are performed:
#. First, all live objects in the current project scope are found and then filtered to only include :class:`ivy.Array`/:class:`ivy.Container` objects.
-#. Then, these objects are iterated through and `converted to numpy`_ as an intermediary using the current backend.
-#. Next, the global :code:`ivy.__dict__` is updated to the new backend as mentioned in the Backend Setting section above.
-#. Finally, the objects are `converted from numpy`_ to the target backend using the newly set backend.
+#. Then, these objects are iterated through and converted to the target backend using DLPack or numpy as an intermediary.
By default, the dynamic backend attribute is set to True when you create an ivy array (e.g., :code:`x = ivy.array([1,2,3])`), but the attribute is mutable and can be changed after the ivy array is created (e.g., :code:`x.dynamic_backend= True`).
Here's an example to illustrate how this works in practice:
@@ -91,7 +88,7 @@ Here's an example to illustrate how this works in practice:
x.data # will be a jax array
y.data # will still be a torch tensor since dynamic_backend=False
-In addition to setting the dynamic backend attribute for individual ivy arrays, you can also set or unset the dynamic backend feature globally for all such instances using `ivy.set_dynamic_backend`_ and `ivy.unset_dynamic_backend`_ respectively.
+Setting the attribute to True converts the array to the current backend even if the backend was set with `dynamic=False`. In addition to setting the dynamic backend attribute for individual ivy arrays, you can also set or unset the dynamic backend feature globally for all such instances using `ivy.set_dynamic_backend`_ and `ivy.unset_dynamic_backend`_ respectively.
Another useful feature of the dynamic backend is the `ivy.dynamic_backend_as`_ context manager. This allows you to write code like this:
@@ -107,25 +104,6 @@ Another useful feature of the dynamic backend is the `ivy.dynamic_backend_as`_ c
This makes it easy to define different sections of your project with different settings, without having to explicitly call :code:`ivy.set_` and :code:`ivy.unset_` etc.
-There is one technical point to keep in mind when using the dynamic backend attribute. Consider the following example:
-
-.. code-block:: python
-
- ivy.set_backend("tensorflow")
- arr = ivy.array([1,2,3])
- arr.dynamic_backend= False
-
- ivy.set_backend("torch")
-
- # arr.data should still be a tf.Tensor
-
- arr.dynamic_backend = True
-
- ivy.set_backend("jax")
-
- # This would cause a problem since the conversion goes from TF -> JAX, whereas the backend stack goes from Torch -> Jax.
-
-To avoid the above issue, we update the :attr:`.data` attribute to be a native array for the current set backend framework in the setter method for dynamic_backend attribute for `ivy.Array`_ and `ivy.Container`_ classes. So after the line :code:`arr.dynamic_backend = True` in the example above, then :attr:`arr.data` would be a torch.Tensor and not a tf.Tensor.
Backend and Frontend Version Support
------------------------------------
diff --git a/docs/overview/deep_dive/building_the_docs_pipeline.rst b/docs/overview/deep_dive/building_the_docs_pipeline.rst
index e38f01b892941..3cfc6d2e10840 100644
--- a/docs/overview/deep_dive/building_the_docs_pipeline.rst
+++ b/docs/overview/deep_dive/building_the_docs_pipeline.rst
@@ -6,6 +6,21 @@ Building the Docs Pipeline
.. _autosummary: https://www.sphinx-doc.org/en/master/usage/extensions/autosummary.html
.. _doc-builder repository: https://github.com/unifyai/doc-builder
+.. warning::
+
+ Be aware that the doc-builder was developed originally for Linux, although, in theory, you can run
+ it on any platform (supporting either docker or windows), it's only tested it on
+ Linux. If you find any windows related issues, feel free to open an issue for that to review it.
+
+.. note::
+
+ Recommendation:
+ You can use the convenience script if you build the docs regularly,
+ as it will not re-download the dependencies.
+
+ If you have a slow internet connection, you can use GitHub Codespaces since it will help you to build the
+ docs faster since our script downloads large dependency files.
+
To build our docs, we use `Sphinx`_. Sphinx is an extendable documentation generator
for Python. As our building pipeline is complex, we heavily customize Sphinx using
custom and third party extensions. As well as having a convenience script to build
@@ -348,8 +363,8 @@ This is a custom documenter for ``autodoc`` that documents Ivy data attributes t
in ``ivy.functional.ivy``, it will replace the module to ``ivy.`` instead of
``ivy.functional.ivy.``.
-It's used instead of simply using ``ivy.`` because data attributes have
-no ``__doc__`` atribute, instead docs are discovered by parsing the source code itself.
+It's used instead of simply using ``ivy.`` because data attributes have
+no ``__doc__`` attribute, instead docs are discovered by parsing the source code itself.
So for Sphinx to find the required docs, it needs to be supplied the full module name,
then using the ``autoivydata`` directive will replace the module name to ``ivy.``.
diff --git a/docs/overview/deep_dive/containers.rst b/docs/overview/deep_dive/containers.rst
index 13521ec772f17..bfcc94e048bbe 100644
--- a/docs/overview/deep_dive/containers.rst
+++ b/docs/overview/deep_dive/containers.rst
@@ -252,8 +252,8 @@ There may be some compositional functions which are not implicitly nestable for
One such example is the :func:`ivy.linear` function which is not implicitly nestable despite being compositional. This is because of the use of special functions like :func:`__len__` and :func:`__list__` which, among other functions, are not nestable and can't be made nestable.
But we should try to avoid this, in order to make the flow of computation as intuitive to the user as possible.
-When compiling the code, the computation graph is **identical** in either case, and there will be no implications on performance whatsoever.
-The implicit nestable solution may be slightly less efficient in eager mode, as the leaves of the container are traversed multiple times rather than once, but if performance is of concern then the code should always be compiled in any case.
+When tracing the code, the computation graph is **identical** in either case, and there will be no implications on performance whatsoever.
+The implicit nestable solution may be slightly less efficient in eager mode, as the leaves of the container are traversed multiple times rather than once, but if performance is of concern then the code should always be traced in any case.
The distinction is only really relevant when stepping through and debugging with eager mode execution, and for the reasons outlined above, the preference is to keep compositional functions implicitly nestable where possible.
**Shared Nested Structure**
diff --git a/docs/overview/deep_dive/continuous_integration.rst b/docs/overview/deep_dive/continuous_integration.rst
index e639efa7bcfca..9653ddd175da9 100644
--- a/docs/overview/deep_dive/continuous_integration.rst
+++ b/docs/overview/deep_dive/continuous_integration.rst
@@ -152,7 +152,7 @@ Once the Mapping has been updated, the βDetermine & Run Testsβ Logic works a
tests_to_run = determine_tests_line(tests_file, line, tests_to_run)
4. Further, All the new tests added in a commit are collected (up to a max limit of 10, any more tests added are taken up in subsequent commits).
-5. Finally, All the collected tests are triggered by the run_tests.py script, and the corresponding entry in the MongoDB Database is updated with the Test Result (Details on this in the Dashboard Section below).
+5. Finally, All the collected tests are triggered by the scripts/run_tests/run_tests.py script, and the corresponding entry in the MongoDB Database is updated with the Test Result (Details on this in the Dashboard Section below).
Storing (and retrieving) the Mapping
------------------------------------
@@ -174,7 +174,7 @@ For Push triggered testing (intelligent-tests.yml Workflow), we use the SSH Clon
.. code-block::
- source ./ivy/clone_mapping.sh master
+ source ./ivy/scripts/shell/clone_mapping.sh master
Determine and Run Tests, and Update the Mapping ...
git add .
git commit -m "Update Mapping"
@@ -186,8 +186,8 @@ Now, that the SSH key of the Runner has permissions to push and clone the Mappin
.. code-block::
- USER_EMAIL="rashul.chutani@gmail.com"
- USER_NAME="Rashul Chutani"
+ USER_EMAIL="ivy.branch@lets-unify.ai"
+ USER_NAME="ivy-branch"
TARGET_BRANCH=$1
GITHUB_SERVER="github.com"
mkdir --parents "$HOME/.ssh"
@@ -314,8 +314,7 @@ follow the following steps:
Manual Tests are also available for PRs.
You can also run the Manual Tests Workflow on a Fork Repository (while reviewing PRs), as follows:
-1. Visit https://github.com/RashulChutani/ivy/actions/workflows/manual-tests-pr.yml by going to the
-βActionsβ Tab on the Fork, and selecting the manual-tests-pr workflow from the left pane.
+1. Visit the βActionsβ Tab on the Fork, and selecting the manual-tests-pr workflow from the left pane.
2. Trigger the Workflow by following Steps 2-4 described above.
This might take some time to run as the Fork may have limited runners.
diff --git a/docs/overview/deep_dive/data_types.rst b/docs/overview/deep_dive/data_types.rst
index f5dc74853c04a..f3cb6defae24b 100644
--- a/docs/overview/deep_dive/data_types.rst
+++ b/docs/overview/deep_dive/data_types.rst
@@ -339,7 +339,7 @@ Only one of these decorators can be specified for any given function.
In the case of :attr:`@with_supported_dtypes` it is assumed that all unmentioned data types are unsupported, and in the case of :attr:`@with_unsupported_dtypes` it is assumed that all unmentioned data types are supported.
The decorators take two arguments, a dictionary with the unsupported dtypes mapped to the corresponding version of the backend framework and the current version of the backend framework on the user's system.
-Based on that, the version specific unsupported dtypes and devices are set for the given function everytime the function is called.
+Based on that, the version specific unsupported dtypes and devices are set for the given function every time the function is called.
For Backend Functions:
@@ -423,7 +423,7 @@ set of dtypes is not supported by a certain device.
.. code-block:: python
- @with_unsupported_device_and_dtypes({"2.5.1 and below": {"cpu": ("int8", "int16", "uint8")}}, backend_version)
+ @with_unsupported_device_and_dtypes({"2.5.2 and below": {"cpu": ("int8", "int16", "uint8")}}, backend_version)
def gcd(
x1: Union[paddle.Tensor, int, list, tuple],
x2: Union[paddle.Tensor, float, list, tuple],
@@ -528,7 +528,7 @@ The attributes are set for functions that don't have a specific backend implemen
An example of an ivy function which does not have a specific backend implementation for each backend is the :attr:`einops_reduce` function. `This function `_ , makes use of a third-party library :attr:`einops` which has its own backend-agnostic implementations.
-The :attr:`unsupported_dtypes` and :attr:`supported_dtypes` attributes take two arguments, a dictionary with the unsupported dtypes mapped to the corresponding backend framework. Based on that, the specific unsupported dtypes are set for the given function everytime the function is called.
+The :attr:`unsupported_dtypes` and :attr:`supported_dtypes` attributes take two arguments, a dictionary with the unsupported dtypes mapped to the corresponding backend framework. Based on that, the specific unsupported dtypes are set for the given function every time the function is called.
For example, we use the :attr:`unsupported_dtypes` attribute for the :attr:`einops_reduce` function within the ivy functional API as shown below:
.. code-block:: python
@@ -539,7 +539,7 @@ For example, we use the :attr:`unsupported_dtypes` attribute for the :attr:`eino
"paddle": ("complex", "uint8", "int8", "int16", "float16"),
}
-With the above aproach, we ensure that anytime the backend is set to torch, the :attr:`einops_reduce` function does not support float16, likewise, complex dtypes are not supported with a tensorflow backend and
+With the above approach, we ensure that anytime the backend is set to torch, the :attr:`einops_reduce` function does not support float16, likewise, complex dtypes are not supported with a tensorflow backend and
complex, uint8, int8, int16, float16 are not supported with a paddle backend.
Backend Data Type Bugs
diff --git a/docs/overview/deep_dive/devices.rst b/docs/overview/deep_dive/devices.rst
index 1159535728bdb..2b68f1bbabec1 100644
--- a/docs/overview/deep_dive/devices.rst
+++ b/docs/overview/deep_dive/devices.rst
@@ -214,7 +214,7 @@ This is the exception you will get while running the code above:
File "/content/ivy/ivy/func_wrapper.py", line 863, in _handle_device_shifting
raise ivy.utils.exceptions.IvyException(
During the handling of the above exception, another exception occurred:
- Expected all input arrays to be on the same device, but found atleast two devices - ('cpu', 'gpu:0'),
+ Expected all input arrays to be on the same device, but found at least two devices - ('cpu', 'gpu:0'),
set `ivy.set_soft_device_mode(True)` to handle this problem.
b. If all the input arrays are on the same device, the operation is executed without raising any device exceptions.
diff --git a/docs/overview/deep_dive/docstring_examples.rst b/docs/overview/deep_dive/docstring_examples.rst
index b315d10c0e63a..1debbc8d67963 100644
--- a/docs/overview/deep_dive/docstring_examples.rst
+++ b/docs/overview/deep_dive/docstring_examples.rst
@@ -221,7 +221,7 @@ Let's start with the functional examples, with :class:`ivy.Array` instances in t
These examples cover points 1, 2, 3, 4 and 5.
-Please note that in the above case of `x` having multi-line input, it is necessary for each line of the input to be seperated by a '...\' so that they can be parsed by the script that tests the examples in the docstrings.
+Please note that in the above case of `x` having multi-line input, it is necessary for each line of the input to be separated by a '...\' so that they can be parsed by the script that tests the examples in the docstrings.
Point 1 is simple to satisfy.
Ignoring the union over :class:`ivy.Array` and :class:`ivy.NativeArray` which is covered by points 6 and 7, and ignoring the *nestable* nature of the function which is covered by points 8 and 9, then as far as point 1 is concerned, the input :code:`x` only has one possible variation.
@@ -349,7 +349,7 @@ Let's start with the functional examples, with :class:`ivy.Array` instances in t
These examples cover points 1, 2, 3, 4 and 5.
-Again, please note that in the above case of `x` having multi-line input, it is necessary for each line of the input to be seperated by a '...\' so that they can be parsed by the script that tests the examples in the docstrings.
+Again, please note that in the above case of `x` having multi-line input, it is necessary for each line of the input to be separated by a '...\' so that they can be parsed by the script that tests the examples in the docstrings.
Point 1 is a bit less trivial to satisfy than it was for :func:`ivy.tan` above.
While :code:`x` again only has one variation (for the same reason as explained in the :func:`ivy.tan` example above), :code:`shift` has two variations (:code:`int` or sequence of :code:`int`), and :code:`axis` has three variations (:code:`int`, :sequence of :code:`int`, or :code:`None`).
@@ -497,7 +497,7 @@ Let's start with the functional examples, with :class:`ivy.Array` instances in t
These examples cover points 1, 2, 3, 4 and 5.
-Again, please note that in the above case of `x` having multi-line input, it is necessary for each line of the input to be seperated by a '...\' so that they can be parsed by the script that tests the examples in the docstrings.
+Again, please note that in the above case of `x` having multi-line input, it is necessary for each line of the input to be separated by a '...\' so that they can be parsed by the script that tests the examples in the docstrings.
Point 1 is again trivial to satisfy, as was the case for :func:`ivy.tan`.
Ignoring the union over :class:`ivy.Array` and :class:`ivy.NativeArray` which is covered by points 6 and 7, and also ignoring the *nestable* nature of the function which is covered by points 8 and 9, then as far as point 1 is concerned, inputs :code:`x1` and :code:`x2` both only have one possible variation.
@@ -533,7 +533,7 @@ We then also add an example with an :class:`ivy.Container` for one of the inputs
[8.1, 9.3, 3.4]])
}
-Again, unlike :func:`ivy.tan`, point 7 is relevant in this case, as there are two function inputs in total (exluding :code:`out`).
+Again, unlike :func:`ivy.tan`, point 7 is relevant in this case, as there are two function inputs in total (excluding :code:`out`).
We can therefore add an example with multiple :class:`ivy.Container` inputs, in order to satisfy point 7.
.. parsed-literal::
diff --git a/docs/overview/deep_dive/exception_handling.rst b/docs/overview/deep_dive/exception_handling.rst
index 8695c318fe42d..55313c46b00a4 100644
--- a/docs/overview/deep_dive/exception_handling.rst
+++ b/docs/overview/deep_dive/exception_handling.rst
@@ -511,14 +511,16 @@ Let's look at an example!
# less_equal
if allow_equal and ivy.any(x1 > x2):
raise ivy.exceptions.IvyException(
- "{} must be lesser than or equal to {}".format(x1, x2)
+ f"{x1} must be lesser than or equal to {x2}"
if message == ""
else message
)
# less
elif not allow_equal and ivy.any(x1 >= x2):
raise ivy.exceptions.IvyException(
- "{} must be lesser than {}".format(x1, x2) if message == "" else message
+ f"{x1} must be lesser than {x2}"
+ if message == ""
+ else message
)
**ivy.set_split_factor**
diff --git a/docs/overview/deep_dive/fix_failing_tests.rst b/docs/overview/deep_dive/fix_failing_tests.rst
new file mode 100644
index 0000000000000..c2dc97832636c
--- /dev/null
+++ b/docs/overview/deep_dive/fix_failing_tests.rst
@@ -0,0 +1,310 @@
+Fix Failing Tests:
+==============================
+
+.. _`repo`: https://github.com/unifyai/ivy
+.. _`issues`: https://github.com/unifyai/ivy/issues?q=is%3Aopen+is%3Aissue+label%3A%22Failing+Test%22
+.. _`issue`: https://github.com/unifyai/ivy/issues/25849
+.. _`discord`: https://discord.gg/sXyFF8tDtm
+.. _`docker channel`: https://discord.com/channels/799879767196958751/942114744691740772
+.. _`miniconda`: https://docs.conda.io/en/latest/miniconda.html
+.. _`venv`: https://docs.python.org/3/library/venv.html
+.. _`ivy/scripts/shell`: https://github.com/unifyai/ivy/tree/f71a414417646e1dfecb5de27fb555f80333932c/scripts/shell
+.. _`platform compatibility tags`: https://packaging.python.org/en/latest/specifications/platform-compatibility-tags/
+.. _`logging level`: https://docs.python.org/3/library/logging.html#logging.Logger.setLevel
+.. _`pycharm channel`: https://discord.com/channels/799879767196958751/942114831039856730
+.. _`pre-commit channel`: https://discord.com/channels/799879767196958751/982725464110034944
+.. _`pip packages channel`: https://discord.com/channels/799879767196958751/942114789642080317
+.. _`ivy tests channel`: https://discord.com/channels/799879767196958751/982738436383445073
+.. _`ivy frontend tests channel`: https://discord.com/channels/799879767196958751/1028267758028337193
+
+We're really happy you'd like to learn how to contribute towards Ivy π
+
+This page explains the main steps to get started with fixing failing tests!
+
+Prerequirement:
+**************************
+
+Before you start with this you should have:
+
+#. `Git `_
+#. `Visual Studio Code here `_
+#. `Docker Desktop `_
+
+
+Setting Up
+***********
+
+**Forking and cloning the repo**
+
+#. `Fork Ivy Repo `_
+#. `Clone `_ the fork with it's submoodules locally or on codespaces
+
+ .. dropdown:: If you are new to Git:
+
+ Depending on your preferred mode of cloning, any of the below should work:
+
+ .. code-block:: bash
+
+ git clone --recurse-submodules git@github.com:YOUR_USERNAME/ivy.git
+
+ .. code-block:: bash
+
+ git clone --recurse-submodules https://github.com/YOUR_USERNAME/ivy.git
+
+ .. code-block:: bash
+
+ gh repo clone YOUR_USERNAME/ivy your_folder -- --recurse-submodules
+
+ Then enter into your cloned ivy folder, for example :code:`cd ~/ivy` and add Ivy original repository as upstream, to easily sync with the latest changes.
+
+ .. code-block:: bash
+
+ git remote add upstream https://github.com/unifyai/ivy.git
+
+.. dropdown:: **Windows, docker and VsCode**
+
+ #. Open the Docker desktop, make sure it's running in the background while following the process below.
+ #. Open Ivy repo folder with Visual Studio Code, and follow the next steps:
+ a. At the bottom right a window will pop up asking for "Dev Containers" extension, install that.
+ In case the window doesn't pop up, search for the "Dev Containers" extension in the Visual Studio Code and install that.
+ b. Install the "Docker" extension for Visual Studio Code, you'll easily find that by searching "docker" in the extensions tab.
+ c. Once done, restart Visual Studio Code, at the bottom left corner there would be an icon similar to " >< " overlapped on each other.
+ d. Clicking on that will open a bar at the top which will give you an option "Open Folder in Container...", click on that.
+ e. Run tests with the next command "pytest test_file_path::test_fn_name". You are inside the container now, and you can locally run the tests that you've modified.
+
+ .. warning::
+ Opening the container may take a long time, as the Docker image is very large (5+ GB).
+
+
+How to run tests
+****************
+To find tests which are currently failing, open the `issues`_ in our GitHub.,
+
+You can notice :code:`test_jax_transpose` is failing in this `issue`_, this function is in the Jax frontends in the manipulaiton submodule.
+
+To run test locally, you need to run the following command:
+
+:code:`pytest test_file_path::test_fn_name`
+
+In the case of :code:`test_jax_transpose`, the command will be
+
+.. code-block:: bash
+
+ pytest ivy_tests/test_ivy/test_frontends/test_jax/test_numpy/test_manipulations.py::test_jax_transpose
+
+You will need to read through the errors in the terminal and use the common errors in the list at the end of this page to solve the test.
+
+.. dropdown:: **Setting Up Testing for VsCode**
+
+ The steps are as following to setup testing on VS Code.
+
+ 1. In the left toolbar menu, click on the flask Icon and select "Configure Python Tests" and select PyTest as the test framework.
+
+ .. image:: https://github.com/unifyai/unifyai.github.io/blob/main/img/externally_linked/contributing/setting_up/vs_code_testing_setup/vs_testing_01.png?raw=true
+ :width: 420
+
+ 1. Select ivy_tests as the root directory for testing.
+
+ .. image:: https://github.com/unifyai/unifyai.github.io/blob/main/img/externally_linked/contributing/setting_up/vs_code_testing_setup/vs_testing_02.png?raw=true
+ :width: 420
+
+ 1. Configure the _array_module.py file in the array_api_tests to be set to one of the supported frameworks.
+
+ .. image:: https://github.com/unifyai/unifyai.github.io/blob/main/img/externally_linked/contributing/setting_up/vs_code_testing_setup/vs_testing_03.png?raw=true
+ :width: 420
+
+ 1. Following all of this, you should refresh the test suite and you should now be able to run tests right from VS Code!
+
+ 2. To simply run the tests using the play button in the toolbar, you will need to add the .vscode folder to your workspace. Then add the ``settings.json`` file containing the following:
+
+ .. code-block:: json
+
+ {
+ "python.testing.pytestArgs": [
+ "./ivy_tests/test_ivy/",
+ "./ivy_tests/array_api_testing/test_array_api/",
+ "--continue-on-collection-errors",
+ ],
+ "python.testing.unittestEnabled": false,
+ "python.testing.pytestEnabled": true,
+ "python.testing.autoTestDiscoverOnSaveEnabled": true,
+ }
+
+Common Errors
+*************
+
+This section aims to assist you in navigating through some common errors you might encounter while working with the Ivy's Functional API. We'll go through :code:`test_jax_transpose` and then some common errors which you might encounter while working as a contributor or a developer.
+
+#. Starting off with :code:`test_jax_transpose`, it throws an Assertion error because the shape returned by ground truth is different from the shape returned by the target backend.
+
+ .. code-block:: python
+
+ E ivy.utils.exceptions.IvyBackendException: paddle: to_numpy: paddle: default_device: paddle: dev: (PreconditionNotMet) Tensor not initialized yet when DenseTensor::place() is called.
+ E [Hint: holder_ should not be null.] (at /paddle/paddle/phi/core/dense_tensor_impl.cc:61)
+ E
+ E Falsifying example: test_jax_transpose(
+ E on_device='cpu',
+ E frontend='jax',
+ E backend_fw='paddle',
+ E array_and_axes=(array([], shape=(1, 0), dtype=complex64),
+ E ['complex64'],
+ E None),
+ E test_flags=FrontendFunctionTestFlags(
+ E num_positional_args=0,
+ E with_out=False,
+ E inplace=False,
+ E as_variable=[False],
+ E native_arrays=[False],
+ E test_trace=False,
+ E generate_frontend_arrays=False,
+ E transpile=False,
+ E precision_mode=True,
+ E ),
+ E fn_tree='ivy.functional.frontends.jax.numpy.transpose',
+ E )
+ E
+ E You can reproduce this example by temporarily adding @reproduce_failure('6.87.3', b'AAEGBAEGAQAAAAAAAAAAAAAB') as a decorator on your test case
+
+ **Solution:**
+
+ As it is failing for torch backend and its producing a different shape than the ground truth, it is most likely a bug in the :code:`permute_dims` in torch backend which is being used in this frontend function.
+
+ Now lets explore some other common errors you might face.
+
+#. This is the case where we pass in a dtype to `torch` which is not actually supported by the torch's native framework itself.
+
+ .. code-block:: python
+
+ E RuntimeError: "logaddexp2_cpu" not implemented for 'Half'
+ E Falsifying example: test_logaddexp2(
+ E backend_fw='torch',
+ E on_device='cpu',
+ E dtype_and_x=(['float16', 'float16'],
+ E [array([-1.], dtype=float16), array([-1.], dtype=float16)]),
+ E test_flags=FunctionTestFlags(
+ E ground_truth_backend='tensorflow',
+ E num_positional_args=2,
+ E with_out=False,
+ E instance_method=False,
+ E test_gradients=False,
+ E test_trace=None,
+ E as_variable=[False],
+ E native_arrays=[False],
+ E container=[False],
+ E ),
+ E fn_name='logaddexp2',
+ E )
+ E
+ E You can reproduce this example by temporarily adding @reproduce_failure('6.82.4', b'AXicY2BkAAMoBaaR2WAAAACVAAY=') as a decorator on your test case
+
+
+ **Solution:**
+
+ As we are explicitly passing in a `dtype` which is not supported in the torch framework itself so torch backend fails here, a possible fix is adding the dtype in the unsupported dtype decoartor which would look something like this.
+
+ .. code-block:: python
+
+ @with_unsupported_dtypes({"2.0.1 and below": ("float16",)}, backend_version)
+
+ and place it above the function definition.
+
+#. This is the case where the value from the ground-truth backend(tensorflow) does not match the value of the backend(jax) we are testing for this case.
+
+ .. code-block:: python
+
+ E AssertionError: the results from backend jax and ground truth framework tensorflow do not match
+ E 0.25830078125!=0.258544921875
+ E
+ E
+ E Falsifying example: test_acosh(
+ E backend_fw='jax',
+ E on_device='cpu',
+ E dtype_and_x=(['float16'], [array(4., dtype=float16)]),
+ E test_flags=FunctionTestFlags(
+ E ground_truth_backend='tensorflow',
+ E num_positional_args=1,
+ E with_out=False,
+ E instance_method=False,
+ E test_gradients=True,
+ E test_trace=None,
+ E as_variable=[False],
+ E native_arrays=[False],
+ E container=[False],
+ E ),
+ E fn_name='acosh',
+ E )
+ E
+ E You can reproduce this example by temporarily adding @reproduce_failure('6.82.4', b'AXicY2BAABYQwQgiAABDAAY=') as a decorator on your test case
+
+ **Solution:**
+
+ As both the results are pretty close to each others in this case, adding an `rtol = 10^-3` and `atol = 10^-3` would fix the failing tests here.
+
+ .. code-block:: python
+
+ @handle_test(
+ fn_tree="functional.ivy.acosh",
+ dtype_and_x=helpers.dtype_and_values(
+ available_dtypes=helpers.get_dtypes("float"),
+ min_value=1,
+ large_abs_safety_factor=4,
+ small_abs_safety_factor=4,
+ ),
+ )
+ def test_acosh(*, dtype_and_x, test_flags, backend_fw, fn_name, on_device):
+ input_dtype, x = dtype_and_x
+ helpers.test_function(
+ input_dtypes=input_dtype,
+ test_flags=test_flags,
+ backend_to_test=backend_fw,
+ fn_name=fn_name,
+ on_device=on_device,
+ rtol_=1e-2,
+ atol_=1e-2,
+ x=x[0],
+ )
+
+#. This is a similar assertion as stated in point 2 but with torch and ground-truth tensorflow not matching but the matrices are quite different so there should be an issue in the backends rather than a numerical instability here.
+
+ .. code-block:: python
+
+ E AssertionError: the results from backend torch and ground truth framework tensorflow do not match
+ E [[1.41421356 1.41421356 1.41421356]
+ E [1.41421356 1.41421356 1.41421356]
+ E [1.41421356 inf 1.41421356]]!=[[1.41421356e+000 1.41421356e+000 1.41421356e+000]
+ E [1.41421356e+000 1.41421356e+000 1.41421356e+000]
+ E [1.41421356e+000 1.34078079e+154 1.41421356e+000]]
+ E
+ E
+ E Falsifying example: test_abs(
+ E backend_fw='torch',
+ E on_device='cpu',
+ E dtype_and_x=(['complex128'],
+ E [array([[-1.-1.00000000e+000j, -1.-1.00000000e+000j, -1.-1.00000000e+000j],
+ E [-1.-1.00000000e+000j, -1.-1.00000000e+000j, -1.-1.00000000e+000j],
+ E [-1.-1.00000000e+000j, -1.-1.34078079e+154j, -1.-1.00000000e+000j]])]),
+ E fn_name='abs',
+ E test_flags=FunctionTestFlags(
+ E ground_truth_backend='tensorflow',
+ E num_positional_args=1,
+ E with_out=False,
+ E instance_method=False,
+ E test_gradients=False,
+ E test_trace=None,
+ E as_variable=[False],
+ E native_arrays=[False],
+ E container=[False],
+ E ),
+ E )
+ E
+ E You can reproduce this example by temporarily adding @reproduce_failure('6.82.4', b'AXicY2ZkYAIiBiBgZIAAxqHEXsAAB7jUQAAAMtEAzQ==') as a decorator on your test case
+
+ **Solution:**
+
+ If this is passing for all other backends and just failing for torch, and the result matrices are also different which states there is not a numerical instability, the issue is with the torch backend. The best approach in this case is to see the torch backend, there should be an issue in the implementation. You have to correct the backend implementation for torch.
+
+
+Where to ask for Help
+*********************
+
+The best place to ask for help is our `discord`_ server in the relevant channels. For instance, lets say you're facing an issue with :code:`test_jax_transpose` function, in this case you should post your query in the `ivy frontend tests channel`_.
diff --git a/docs/overview/deep_dive/formatting.rst b/docs/overview/deep_dive/formatting.rst
index a5d51ffcedbd9..f970f8e0c24d2 100644
--- a/docs/overview/deep_dive/formatting.rst
+++ b/docs/overview/deep_dive/formatting.rst
@@ -185,7 +185,7 @@ be applied by the ``ivy-gardener`` properly.
On the other hand, ``ivy-gardener`` itself can fail if the bot handling it (ivy-branch) can not apply the changes
suggested by the linters, for example, when it does not have access to edit the target branch. In this case, you
should try to give the maintainer bot the access to your branch (which is an option shown in GitHub UI) and give it
-another try, or manually resolve the formatting errors by commiting the changes yourself.
+another try, or manually resolve the formatting errors by committing the changes yourself.
**Round Up**
diff --git a/docs/overview/deep_dive/function_types.rst b/docs/overview/deep_dive/function_types.rst
index aba496df485d1..d2bc16af271f8 100644
--- a/docs/overview/deep_dive/function_types.rst
+++ b/docs/overview/deep_dive/function_types.rst
@@ -103,7 +103,7 @@ For example, the implementation of :func:`ivy.cross_entropy` in :mod:`ivy/functi
*,
axis: int = -1,
epsilon: float = 1e-7,
- reduction: str = "sum",
+ reduction: str = "mean",
out: Optional[ivy.Array] = None
) -> ivy.Array:
ivy.utils.assertions.check_elem_in_list(reduction, ["none", "sum", "mean"])
diff --git a/docs/overview/deep_dive/inplace_updates.rst b/docs/overview/deep_dive/inplace_updates.rst
index 42df6520a9668..a2a4019a8e106 100644
--- a/docs/overview/deep_dive/inplace_updates.rst
+++ b/docs/overview/deep_dive/inplace_updates.rst
@@ -408,7 +408,7 @@ We'll use :func:`ivy.cross_entropy` as an example:
*,
axis: int = -1,
epsilon: float = 1e-7,
- reduction: str = "sum",
+ reduction: str = "mean",
out: Optional[ivy.Array] = None,
) -> ivy.Array:
ivy.utils.assertions.check_elem_in_list(reduction, ["none", "sum", "mean"])
diff --git a/docs/overview/deep_dive/ivy_frontends.rst b/docs/overview/deep_dive/ivy_frontends.rst
index 8214423af7e3b..ac7f1aab1ea14 100644
--- a/docs/overview/deep_dive/ivy_frontends.rst
+++ b/docs/overview/deep_dive/ivy_frontends.rst
@@ -92,12 +92,12 @@ The former set of functions map very closely to the API for the Accelerated Line
The latter set of functions map very closely to NumPy's well known API.
In general, all functions in the :mod:`jax.numpy` namespace are themselves implemented as a composition of the lower-level functions in the :mod:`jax.lax` namespace.
-When transpiling between frameworks, the first step is to compile the computation graph into low level python functions for the source framework using Ivy's graph compiler, before then replacing these nodes with the associated functions in Ivy's frontend API.
+When transpiling between frameworks, the first step is to trace a computation graph of low level python functions for the source framework using Ivy's tracer, before then replacing these nodes with the associated functions in Ivy's frontend API.
Given that all jax code can be decomposed into :mod:`jax.lax` function calls, when transpiling JAX code it should always be possible to express the computation graph as a composition of only :mod:`jax.lax` functions.
Therefore, arguably these are the *only* functions we should need to implement in the JAX frontend.
-However, in general we wish to be able to compile a graph in the backend framework with varying levels of dynamicism.
+However, in general we wish to be able to trace a graph in the backend framework with varying levels of dynamicism.
A graph of only :mod:`jax.lax` functions chained together in general is more *static* and less *dynamic* than a graph which chains :mod:`jax.numpy` functions together.
-We wish to enable varying extents of dynamicism when compiling a graph with our graph compiler, and therefore we also implement the functions in the :mod:`jax.numpy` namespace in our frontend API for JAX.
+We wish to enable varying extents of dynamicism when creating a graph with our tracer, and therefore we also implement the functions in the :mod:`jax.numpy` namespace in our frontend API for JAX.
Thus, both :mod:`lax` and :mod:`numpy` modules are created in the JAX frontend API.
We start with the function :func:`lax.add` as an example.
diff --git a/docs/overview/deep_dive/ivy_frontends_tests.rst b/docs/overview/deep_dive/ivy_frontends_tests.rst
index 97a6f7a6ab2c2..6cdad3a87772d 100644
--- a/docs/overview/deep_dive/ivy_frontends_tests.rst
+++ b/docs/overview/deep_dive/ivy_frontends_tests.rst
@@ -61,7 +61,7 @@ Frontend Test Examples
-----------------------
Before you begin writing a frontend test, make sure you are placing it in the correct location.
-See the 'Where to place a frontend function' sub-section of the frontend APIs `open task`_ for more details.
+See the :ref:`/overview/contributing/open_tasks:Where to place a frontend function` sub-section of the frontend APIs `open task`_ for more details.
ivy.tan()
^^^^^^^^^
@@ -619,7 +619,7 @@ Frontend Instance Method Tests
The frontend instance method tests are similar to the frontend function test, but instead of testing the function directly we test the instance method of the frontend class.
major difference is that we have more flags to pass now, most initialization functions take an array as an input. also some methods may take an array as input,
-for example, :code:`ndarray.__add__` would expect an array as input, despite the :code:`self.array`. and to make our test **complete** we need to generate seperate flags for each.
+for example, :code:`ndarray.__add__` would expect an array as input, despite the :code:`self.array`. and to make our test **complete** we need to generate separate flags for each.
**Important Helper Functions**
@@ -630,8 +630,8 @@ for example, :code:`ndarray.__add__` would expect an array as input, despite the
:func:`helpers.test_frontend_method` is used to test frontend instance methods. It is used in the same way as :func:`helpers.test_frontend_function`. A few important arguments for this function are following:
- :code:`init_input_dtypes` Input dtypes of the arguments on which we are initializing the array on.
- - :code:`init_all_as_kwargs_np` The data to be passed when intializing, this will be a dictionary in which the numpy array which will contain the data will be passed in the :code:`data` key.
- - :code:`method_input_dtypes` The input dtypes of the argument which are to be passed to the instance method after the intialization of the array.
+ - :code:`init_all_as_kwargs_np` The data to be passed when initializing, this will be a dictionary in which the numpy array which will contain the data will be passed in the :code:`data` key.
+ - :code:`method_input_dtypes` The input dtypes of the argument which are to be passed to the instance method after the initialization of the array.
- :code:`method_all_as_kwargs_np` All the arguments which are to be passed to the instance method.
diff --git a/docs/overview/deep_dive/navigating_the_code.rst b/docs/overview/deep_dive/navigating_the_code.rst
index 1bd9b0ae9dd7d..1fe0e6f256cd7 100644
--- a/docs/overview/deep_dive/navigating_the_code.rst
+++ b/docs/overview/deep_dive/navigating_the_code.rst
@@ -192,9 +192,7 @@ To have a better idea on this, let's look at an example!
)
):
raise ivy.utils.exceptions.IvyException(
- "the fill_value: {} and data type: {} are not compatible".format(
- fill_value, dtype
- )
+ f"the fill_value: {fill_value} and data type: {dtype} are not compatible"
)
diff --git a/docs/overview/deep_dive/operating_modes.rst b/docs/overview/deep_dive/operating_modes.rst
index e74d4d21a1349..921ae6fd38ede 100644
--- a/docs/overview/deep_dive/operating_modes.rst
+++ b/docs/overview/deep_dive/operating_modes.rst
@@ -28,7 +28,7 @@ Some of them are:
#. `warning_level`_: Determines the warning level to be shown when one occurs.
#. `nan_policy`_: Determines the policy of handling related to ``nan``.
#. `dynamic_backend`_: Determines if the global dynamic backend setting is active or not.
-#. `precise_mode`_: Determines whether to use a promotion table that avoids any precision loss or a compute effecient table that avoids most wider-than-necessary promotions.
+#. `precise_mode`_: Determines whether to use a promotion table that avoids any precision loss or a compute efficient table that avoids most wider-than-necessary promotions.
#. `array_mode`_: Determines the mode of whether to convert inputs to ``ivy.NativeArray``, then convert the outputs back to ``ivy.Array``.
#. `nestable_mode`_: Determines the mode of whether to check if function inputs are ``ivy.Container``.
#. `exception_trace_mode`_: Determines how much details of the ivy exception traces to be shown in the log.
diff --git a/docs/overview/deep_dive/superset_behaviour.rst b/docs/overview/deep_dive/superset_behaviour.rst
index 5e232c7ceabd3..6ccccad5696f6 100644
--- a/docs/overview/deep_dive/superset_behaviour.rst
+++ b/docs/overview/deep_dive/superset_behaviour.rst
@@ -47,7 +47,7 @@ We've already explained that we should not duplicate arguments in the Ivy functi
Does this mean, provided that the proposed argument is not a duplicate, that we should always add this backend-specific argument to the Ivy function?
The answer is **no**.
When determining the superset, we are only concerned with the pure **mathematics** of the function, and nothing else.
-For example, the :code:`name` argument is common to many TensorFlow functions, such as `tf.concat `_, and is used for uniquely identifying parts of the compiled computation graph during logging and debugging.
+For example, the :code:`name` argument is common to many TensorFlow functions, such as `tf.concat `_, and is used for uniquely identifying parts of the traced computation graph during logging and debugging.
This has nothing to do with the mathematics of the function, and so is *not* included in the superset considerations when implementing Ivy functions.
Similarly, in NumPy the argument :code:`subok` controls whether subclasses of the :class:`numpy.ndarray` class should be permitted, which is included in many functions, such as `numpy.ndarray.astype `_.
Finally, in JAX the argument :code:`precision` is quite common, which controls the precision of the return values, as used in `jax.lax.conv `_ for example.
@@ -129,8 +129,8 @@ The following would be a much better solution:
return res
You will notice that this implementation involves more lines of code, but this should not be confused with added complexity.
-All Ivy code should be graph compiled for efficiency, and in this case all the :code:`if` and :code:`else` statements are removed, and all that remains is the backend functions which were executed.
-This new implementation will be compiled to a graph of either one, three, four, or six functions depending on the values of :code:`beta` and :code:`threshold`, while the previous implementation would *always* compile to six functions.
+All Ivy code should be traced for efficiency, and in this case all the :code:`if` and :code:`else` statements are removed, and all that remains is the backend functions which were executed.
+This new implementation will be traced to a graph of either one, three, four, or six functions depending on the values of :code:`beta` and :code:`threshold`, while the previous implementation would *always* traces to six functions.
This does mean we do not adopt the default values used by PyTorch, but that's okay.
Implementing the superset does not mean adopting the same default values for arguments, it simply means equipping the Ivy function with the capabilities to execute the superset of behaviours.
@@ -167,7 +167,7 @@ With regards to both of these points, Ivy provides the generalized superset impl
However, as discussed above, :func:`np.logical_and` also supports the :code:`where` argument, which we opt to **not** support in Ivy.
This is because the behaviour can easily be created as a composition like so :code:`ivy.where(mask, ivy.logical_and(x, y), ivy.zeros_like(mask))`, and we prioritize the simplicity, clarity, and function uniqueness in Ivy's API in this case, which comes at the cost of reduced runtime efficiency for some functions when using a NumPy backend.
-However, in future releases our automatic graph compilation and graph simplification processes will alleviate these minor inefficiencies entirely from the final computation graph, by fusing multiple operations into one at the API level where possible.
+However, in future releases our automatic graph tracing and graph simplification processes will alleviate these minor inefficiencies entirely from the final computation graph, by fusing multiple operations into one at the API level where possible.
Maximizing Usage of Native Functionality
----------------------------------------
@@ -241,7 +241,7 @@ Ivy allows this using the `partial_mixed_handler`_ attribute on the backend-spec
interpolate.partial_mixed_handler = lambda *args, mode="linear", **kwargs: mode not in [
"tf_area",
- "bicubic_tensorflow",
+ "tf_bicubic",
"mitchellcubic",
"lanczos3",
"lanczos5",
diff --git a/docs/overview/design.rst b/docs/overview/design.rst
index ea32c66512596..a8cc4e382338b 100644
--- a/docs/overview/design.rst
+++ b/docs/overview/design.rst
@@ -29,7 +29,7 @@ If that sounds like you, feel free to check out the `Deep Dive`_ section after y
| back-end functional APIs β
| Ivy functional API β
| Framework Handler β
-| Ivy Compiler π§
+| Ivy Tracer π§
|
| (b) `Ivy as a Transpiler `_
| front-end functional APIs π§
diff --git a/docs/overview/design/building_blocks.rst b/docs/overview/design/building_blocks.rst
index 0d1e2b22250d8..249e48050e006 100644
--- a/docs/overview/design/building_blocks.rst
+++ b/docs/overview/design/building_blocks.rst
@@ -210,14 +210,14 @@ The contents of this function are as follows:
if backend_stack:
f = backend_stack[-1]
if verbosity.level > 0:
- verbosity.cprint("Using backend from stack: {}".format(f))
+ verbosity.cprint(f"Using backend from stack: {f}")
return f
# if no global backend exists, we try to infer the backend from the arguments
f = _determine_backend_from_args(list(args) + list(kwargs.values()))
if f is not None:
if verbosity.level > 0:
- verbosity.cprint("Using backend from type: {}".format(f))
+ verbosity.cprint(f"Using backend from type: {f}")
implicit_backend = f.current_backend_str()
return f
return importlib.import_module(_backend_dict[implicit_backend])
@@ -254,7 +254,8 @@ The following is a slightly simplified version of this code for illustration, wh
# maybe log to the terminal
if verbosity.level > 0:
verbosity.cprint(
- 'Backend stack: {}'.format(backend_stack))
+ f'Backend stack: {backend_stack}'
+ )
The functions implemented by the backend-specific backend such as :code:`ivy.functional.backends.torch` only constitute a subset of the full Ivy API.
This is because many higher level functions are written as a composition of lower level Ivy functions.
@@ -321,7 +322,7 @@ A good example is :func:`ivy.lstm_update`, as shown:
ct = init_c
# lstm outputs
- hts_list = list()
+ hts_list = []
# unrolled time dimension with lstm steps
for Wii_xt, Wif_xt, Wig_xt, Wio_xt in zip(
@@ -354,26 +355,26 @@ A good example is :func:`ivy.lstm_update`, as shown:
We *could* find and wrap the functional LSTM update methods for each backend framework which might bring a small performance improvement, but in this case there are no functional LSTM methods exposed in the official functional APIs of the backend frameworks, and therefore the functional LSTM code which does exist for the backends is much less stable and less reliable for wrapping into Ivy.
Generally, we have made decisions so that Ivy is as stable and scalable as possible, minimizing dependencies to backend framework code where possible with minimal sacrifices in performance.
-Graph Compiler π§
+Tracer π§
-----------------
βWhat about performance?β I hear you ask.
This is a great point to raise!
With the design as currently presented, there would be a small performance hit every time we call an Ivy function by virtue of the added Python wrapping.
-One reason we created the graph compiler was to address this issue.
+One reason we created the tracer was to address this issue.
-The compiler takes in any Ivy function, backend function, or composition, and returns the computation graph using the backend functional API only.
+The tracer takes in any Ivy function, backend function, or composition, and returns the computation graph using the backend functional API only.
The dependency graph for this process looks like this:
.. image:: https://github.com/unifyai/unifyai.github.io/blob/main/img/externally_linked/design/compiler_dependency_graph.png?raw=true
:align: center
:width: 75%
-Let's look at a few examples, and observe the compiled graph of the Ivy code against the native backend code.
+Let's look at a few examples, and observe the traced graph of the Ivy code against the native backend code.
First, let's set our desired backend as PyTorch.
-When we compile the three functions below, despite the fact that each
-has a different mix of Ivy and PyTorch code, they all compile to the same graph:
+When we trace the three functions below, despite the fact that each
+has a different mix of Ivy and PyTorch code, they all trace to the same graph:
+----------------------------------------+-----------------------------------------+-----------------------------------------+
|.. code-block:: python |.. code-block:: python |.. code-block:: python |
@@ -392,7 +393,7 @@ has a different mix of Ivy and PyTorch code, they all compile to the same graph:
| x = ivy.array([[1., 2., 3.]]) | x = torch.tensor([[1., 2., 3.]]) | x = ivy.array([[1., 2., 3.]]) |
| | | |
| # create graph | # create graph | # create graph |
-| graph = ivy.compile_graph( | graph = ivy.compile_graph( | graph = ivy.compile_graph( |
+| graph = ivy.trace_graph( | graph = ivy.trace_graph( | graph = ivy.trace_graph( |
| pure_ivy, x) | pure_torch, x) | mix, x) |
| | | |
| # call graph | # call graph | # call graph |
@@ -407,7 +408,7 @@ For all existing ML frameworks, the functional API is the backbone that underpin
This means that under the hood, any code can be expressed as a composition of ops in the functional API.
The same is true for Ivy.
Therefore, when compiling the graph with Ivy, any higher-level classes or extra code which does not directly contribute towards the computation graph is excluded.
-For example, the following 3 pieces of code all compile to the exact same computation graph as shown:
+For example, the following 3 pieces of code all result in the exact same computation graph when traced as shown:
+----------------------------------------+-----------------------------------------+-----------------------------------------+
|.. code-block:: python |.. code-block:: python |.. code-block:: python |
@@ -426,9 +427,9 @@ For example, the following 3 pieces of code all compile to the exact same comput
| | -1, 1, (3, 3)) | -1, 1, (3, 3)) |
| # input | b = ivy.zeros((3,)) | b = ivy.zeros((3,)) |
| x = ivy.array([1., 2., 3.]) | | |
-| | # compile graph | # compile graph |
-| # compile graph | graph = ivy.compile_graph( | graph = ivy.compile_graph( |
-| net.compile_graph(x) | clean, x, w, b) | unclean, x, w, b) |
+| | # trace graph | # trace graph |
+| # trace graph | graph = ivy.trace_graph( | graph = ivy.trace_graph( |
+| net.trace_graph(x) | clean, x, w, b) | unclean, x, w, b) |
| | | |
| # execute graph | # execute graph | # execute graph |
| net(x) | graph(x, w, b) | graph(x, w, b) |
@@ -438,8 +439,8 @@ For example, the following 3 pieces of code all compile to the exact same comput
:align: center
:width: 75%
-This compilation is not restricted to just PyTorch.
-Let's take another example, but compile to Tensorflow, NumPy, and JAX:
+This tracing is not restricted to just PyTorch.
+Let's take another example, but trace to Tensorflow, NumPy, and JAX:
+------------------------------------+
|.. code-block:: python |
@@ -453,7 +454,7 @@ Let's take another example, but compile to Tensorflow, NumPy, and JAX:
| x = ivy.array([[1., 2., 3.]]) |
| y = ivy.array([[2., 3., 4.]]) |
| # create graph |
-| graph = ivy.compile_graph( |
+| graph = ivy.trace_graph( |
| ivy_func, x, y) |
| |
| # call graph |
@@ -485,13 +486,13 @@ Jax:
:width: 75%
|
-The example above further emphasizes that the graph compiler creates a computation graph consisting of backend functions, not Ivy functions.
-Specifically, the same Ivy code compiles to different graphs depending on the selected backend.
-However, when compiling native framework code, we are only able to compile a graph for that same framework.
-For example, we cannot take torch code and compile this into tensorflow code.
+The example above further emphasizes that the tracer creates a computation graph consisting of backend functions, not Ivy functions.
+Specifically, the same Ivy code is traced to different graphs depending on the selected backend.
+However, when compiling native framework code, we are only able to trace a graph for that same framework.
+For example, we cannot take torch code and trace this into tensorflow code.
However, we can transpile torch code into tensorflow code (see `Ivy as a Transpiler `_ for more details).
-The graph compiler does not compile to C++, CUDA, or any other lower level language.
+The tracer is not a compiler and does not compile to C++, CUDA, or any other lower level language.
It simply traces the backend functional methods in the graph, stores this graph, and then efficiently traverses this graph at execution time, all in Python.
Compiling to lower level languages (C++, CUDA, TorchScript etc.) is supported for most backend frameworks via :func:`ivy.compile`, which wraps backend-specific compilation code, for example:
@@ -523,6 +524,6 @@ Therefore, the backend code can always be run with maximal efficiency by compili
**Round Up**
-Hopefully, this has painted a clear picture of the fundamental building blocks underpinning the Ivy framework, being the backend functional APIs, Ivy functional API, backend handler, and graph compiler π
+Hopefully, this has painted a clear picture of the fundamental building blocks underpinning the Ivy framework, being the Backend functional APIs, Ivy functional API, Backend handler, and Tracer π
Please reach out on `discord `_ if you have any questions!
diff --git a/docs/overview/design/ivy_as_a_framework.rst b/docs/overview/design/ivy_as_a_framework.rst
index bf1201048a94b..fd88a46f8113c 100644
--- a/docs/overview/design/ivy_as_a_framework.rst
+++ b/docs/overview/design/ivy_as_a_framework.rst
@@ -1,7 +1,7 @@
Ivy as a Framework
==================
-On the `Building Blocks `_ page, we explored the role of the backend functional APIs, the Ivy functional API, the framework handler, and the graph compiler.
+On the `Building Blocks `_ page, we explored the role of the Backend functional APIs, the Ivy functional API, the Backend handler, and the Tracer.
These are parts labeled as (a) in the image below.
On the `Ivy as a Transpiler `_ page, we explained the role of the backend-specific frontends in Ivy, and how these enable automatic code conversions between different ML frameworks.
diff --git a/docs/overview/design/ivy_as_a_framework/ivy_array.rst b/docs/overview/design/ivy_as_a_framework/ivy_array.rst
index 96d0ba2e6ef76..2e13902a7ec4c 100644
--- a/docs/overview/design/ivy_as_a_framework/ivy_array.rst
+++ b/docs/overview/design/ivy_as_a_framework/ivy_array.rst
@@ -70,7 +70,7 @@ Letβs dive straight in and check out what the :class:`ivy.Array` constructor l
self._dev_str = ivy.as_ivy_dev(self._device)
self._pre_repr = "ivy."
if "gpu" in self._dev_str:
- self._post_repr = ", dev={})".format(self._dev_str)
+ self._post_repr = f", dev={self._dev_str})"
else:
self._post_repr = ")"
self.framework_str = ivy.current_backend_str()
diff --git a/docs/overview/design/ivy_as_a_framework/ivy_container.rst b/docs/overview/design/ivy_as_a_framework/ivy_container.rst
index fad13c67f9f26..3e17db699dc8a 100644
--- a/docs/overview/design/ivy_as_a_framework/ivy_container.rst
+++ b/docs/overview/design/ivy_as_a_framework/ivy_container.rst
@@ -636,8 +636,7 @@ The following code is possible thanks to the recursive operation of the containe
loss, grads = ivy.execute_with_gradients(
loss_fn, model.v)
model.v = model.v - lr * grads
- print('step {} loss {}'.format(
- step, ivy.to_numpy(loss).item()))
+ print(f'step {step} loss {ivy.to_numpy(loss).item()}')
print(model.v)
diff --git a/docs/overview/design/ivy_as_a_framework/ivy_stateful_api.rst b/docs/overview/design/ivy_as_a_framework/ivy_stateful_api.rst
index 3c6574b884d04..c98bb5e860de5 100644
--- a/docs/overview/design/ivy_as_a_framework/ivy_stateful_api.rst
+++ b/docs/overview/design/ivy_as_a_framework/ivy_stateful_api.rst
@@ -427,18 +427,18 @@ The implementation is as follows:
def __init__(self, lr=1e-4, beta1=0.9, beta2=0.999,
epsilon=1e-07, inplace=None,
- stop_gradients=True, compile_on_next_step=False,
+ stop_gradients=True, trace_on_next_step=False,
dev=None):
ivy.Optimizer.__init__(
self, lr, inplace, stop_gradients, True,
- compile_on_next_step, dev)
+ trace_on_next_step, dev)
self._beta1 = beta1
self._beta2 = beta2
self._epsilon = epsilon
self._mw = None
self._vw = None
self._first_pass = True
- self._should_compile = False
+ self._should_trace = False
# Custom Step
diff --git a/docs/overview/design/ivy_as_a_transpiler.rst b/docs/overview/design/ivy_as_a_transpiler.rst
index 50dd33d747ada..d17c45cc78c92 100644
--- a/docs/overview/design/ivy_as_a_transpiler.rst
+++ b/docs/overview/design/ivy_as_a_transpiler.rst
@@ -1,7 +1,7 @@
Ivy as a Transpiler
===================
-On the `Building Blocks `_ page, we explored the role of the backend functional APIs, the Ivy functional API, the backend handler, and the graph compiler.
+On the `Building Blocks `_ page, we explored the role of the Backend functional APIs, the Ivy functional API, the Backend handler, and the Tracer.
These parts are labelled (a) in the image below.
Here, we explain the role of the backend-specific frontends in Ivy, and how these enable automatic code conversions between different ML frameworks.
@@ -164,11 +164,11 @@ Again, by chaining these methods together, we can now call :func:`tf.math.cumpro
x = torch.tensor([[0., 1., 2.]])
ret = tf.math.cumprod(x, -1)
-Role of the Graph Compiler π§
+Role of the Tracer π§
-----------------------------
-The very simple example above worked well, but what about even more complex PyTorch code involving Modules, Optimizers, and other higher level objects? This is where the graph compiler plays a vital role.
-The graph compiler can convert any code into its constituent functions at the functional API level for any ML framework.
+The very simple example above worked well, but what about even more complex PyTorch code involving Modules, Optimizers, and other higher level objects? This is where the tracer plays a vital role.
+The tracer can convert any code into its constituent functions at the functional API level for any ML framework.
For example, letβs take the following PyTorch code and run it using JAX:
@@ -179,7 +179,7 @@ For example, letβs take the following PyTorch code and run it using JAX:
class Network(torch.nn.Module):
def __init__(self):
- super(Network, self).__init__()
+ super().__init__()
self._linear = torch.nn.Linear(3, 3)
def forward(self, x):
@@ -192,7 +192,7 @@ For example, letβs take the following PyTorch code and run it using JAX:
We cannot simply :code:`import ivy.frontends.torch` in place of :code:`import torch` as we did in the previous examples.
This is because the Ivy frontend only supports the functional API for each framework, whereas the code above makes use of higher level classes through the use of the :mod:`torch.nn` namespace.
-In general, the way we convert code is by first compiling the code into its constituent functions in the core API using Ivyβs graph compiler, and then we convert this executable graph into the new framework.
+In general, the way we convert code is by first decomposing the code into its constituent functions in the core API using Ivyβs tracer, and then we convert this executable graph into the new framework.
For the example above, this would look like:
.. code-block:: python
@@ -200,11 +200,11 @@ For the example above, this would look like:
import jax
import ivy
- jax_graph = ivy.compile_graph(net, x).to_backend('jax')
+ jax_graph = ivy.trace_graph(net, x).to_backend('jax')
x = jax.numpy.array([1., 2., 3.])
jax_graph(x)
-However, when calling :func:`ivy.compile_graph` the graph only connects the inputs to the outputs.
+However, when calling :func:`ivy.trace` the graph only connects the inputs to the outputs.
Any other tensors or variables which are not listed in the inputs are treated as constants in the graph.
In this case, this means the learnable weights in the Module will be treated as constants.
This works fine if we only care about running inference on our graph post-training, but this wonβt enable training of the Module in JAX.
@@ -219,15 +219,15 @@ In order to convert a model from PyTorch to JAX, we first must convert the :clas
net = ivy.to_ivy_module(net)
In its current form, the :class:`ivy.Module` instance thinly wraps the PyTorch model into the :class:`ivy.Module` interface, whilst preserving the pure PyTorch backend.
-We can compile this network into a graph using Ivyβs graph compiler like so:
+We can trace a graph of this network using Ivyβs tracer like so:
.. code-block:: python
- net = net.compile_graph()
+ net = net.trace_graph()
In this case, the learnable weights are treated as inputs to the graph rather than constants.
-Now, with a compiled graph under the hood of our model, we can call :meth:`to_backend` directly on the :class:`ivy.Module` instance to convert it to any backend of our choosing, like so:
+Now, with a traced graph under the hood of our model, we can call :meth:`to_backend` directly on the :class:`ivy.Module` instance to convert it to any backend of our choosing, like so:
.. code-block:: python
diff --git a/docs/overview/faq.rst b/docs/overview/faq.rst
index e74df1b21dff7..6cb113df6a2f7 100644
--- a/docs/overview/faq.rst
+++ b/docs/overview/faq.rst
@@ -38,17 +38,17 @@ TensorFlow and PyTorch do allow dynamic sizes, but only on certain backends.
Dynamic sizes require a dynamic memory manager, which CPUs/GPUs have, but XLA currently doesn't.
How does Ivy deal with all of this?
-**A:** Ivy assumes dynamic shapes are supported, but an error will be thrown if/when the function is compiled with dynamic shapes enabled, but the backend does not support dynamic shapes in the compiled graph.
-For now, fully framework-agnostic compiled graphs are only possible for static graphs.
+**A:** Ivy assumes dynamic shapes are supported, but an error will be thrown if/when the function is traced with dynamic shapes enabled, but the backend does not support dynamic shapes in the traced graph.
+For now, fully framework-agnostic traced graphs are only possible for static graphs.
Type and Shape Checking
-----------------------
**Q:** What kind of type system does Ivy use? Does it do shape-checking of tensors? If so, how does it handle dynamic sizes? The gold standard here is a fully dependent type system, but this is very rare, with the exception of `dex`_.
-**A:** The checks performed during graph compilation will remain backend-specific.
-The function :func:`ivy.compile` wraps the backend compilation functions, for example :func:`jax.jit`, :func:`tf.function`, :func:`torch.jit.script` and :func:`torch.jit.trace`.
-For some backends, shape-checking will be performed during the compilation phase and for others it will not.
+**A:** The checks performed during compiling will remain backend-specific.
+The function :func:`ivy.compile` wraps the backend tracing functions, for example :func:`jax.jit`, :func:`tf.function`, :func:`torch.jit.script` and :func:`torch.jit.trace`.
+For some backends, shape-checking will be performed during the tracing phase and for others it will not.
GPU handling
------------
@@ -62,7 +62,7 @@ Model Deployment
**Q:** Does Ivy support model deployment?
**A:** Yes, Ivy will support efficient model deployment.
-However, currently this feature is not yet supported as the graph compiler module is still under development, and will be released soon with ivy version 1.2.0.
+However, currently this feature is not yet supported as the tracer module is still under development, and will be released soon with ivy version 1.2.0.
Dynamic Control Flow
@@ -78,9 +78,9 @@ How will Ivy handle dynamic control flow?
Will Ivy parse python ASTs?
**A:** For now, Ivy will not support dynamic control flow by parsing ASTs.
-The dynamism of :code:`for` loops and :code:`while` loops will be ignored during compilation, and just the static trace which chains the array operations performed during the forward pass at compile time will be preserved.
+The dynamism of :code:`for` loops and :code:`while` loops will be ignored during tracing, and just the static trace which chains the array operations performed during the forward pass at tracing time will be preserved.
-However, Ivy will support the compilation of looping and branching methods such as :code:`lax.scan`, :code:`lax.while`, :code:`tf.while`, :code:`tf.cond` etc.
+However, Ivy will support the tracing of looping and branching methods such as :code:`lax.scan`, :code:`lax.while`, :code:`tf.while`, :code:`tf.cond` etc.
In cases where there is not an associated compilable method in other backends, we will strive to implement this as a composition of existing compilable operations.
If such a composition is not possible, then we will instead convert these to compositions of pure Python :code:`for`, :code:`while` and :code:`if` statements (when using a PyTorch backend for example).
@@ -121,7 +121,7 @@ Weβre very happy in either case!
Support for Functions
---------------------
-**Q:** Is it possible to compile tensor code into a reusable and differentiable function? If you can't, then it will be difficult to apply any fancy kernel fusion algorithms, and you can expect to lose a lot of performance.
+**Q:** Is it possible to trace tensor code into a reusable and differentiable function? If you can't, then it will be difficult to apply any fancy kernel fusion algorithms, and you can expect to lose a lot of performance.
What about higher-order operations, like :code:`jax.vmap` and :code:`jax.pmap`?
**A:** Most functions in Ivy are *primary* functions, which are generally implemented as light wrapping around a near-identical backend-specific function, which itself will likely map to an efficient kernel.
@@ -137,7 +137,7 @@ Alternative Data Structures
**Q:** Will Ivy support data structures such as tuples, dictionaries, lists etc.? For example, JAX code is full of them.
**A:** We will of course support these structures in pure python code, but we will not support backend-specific alternative compilable data structures.
-While Ivy will not provide an interface to these data structures directly, Ivy code can easily supplement JAX code which does contain these data structures, and both can be compiled together without issue.
+While Ivy will not provide an interface to these data structures directly, Ivy code can easily supplement JAX code which does contain these data structures, and both can be traced together without issue.
Ivy can act as a supplementary framework if/when some of the more unique backend-specific data structures are required.
Custom Operations
diff --git a/docs/overview/get_started.rst b/docs/overview/get_started.rst
index 9d891f143e5c6..42b3e1e1f12c3 100644
--- a/docs/overview/get_started.rst
+++ b/docs/overview/get_started.rst
@@ -3,8 +3,8 @@ Get Started
..
- If you want to use **Ivy's compiler and transpiler**, make sure to follow the
- :ref:`setting up instructions for the API key `
+ If you want to use **Ivy's tracer and transpiler**, make sure to follow the
+ :ref:`setting up instructions for the API key `
after installing Ivy!
@@ -56,10 +56,10 @@ the `Contributing - Setting Up `_ page,
where OS-specific and IDE-specific instructions and video tutorials to install Ivy are available!
-Ivy's compiler and transpiler
+Ivy's tracer and transpiler
-----------------------------
-To use Ivy's compiler and transpiler, you'll need an **API key**. We are starting to
+To use Ivy's tracer and transpiler, you'll need an **API key**. We are starting to
grant pilot access to certain users, so you can `join the waitlist `_
if you want to get one!
@@ -84,8 +84,8 @@ For reference, this would be equivalent to:
Issues and Questions
~~~~~~~~~~~~~~~~~~~~
-If you find any issue or bug while using the compiler and/or the transpiler, please
-raise an `issue in GitHub `_ and add the ``compiler``
+If you find any issue or bug while using the tracer and/or the transpiler, please
+raise an `issue in GitHub `_ and add the ``tracer``
or the ``transpiler`` label accordingly. A member of the team will get back to you ASAP!
Otherwise, if you haven't found a bug but want to ask a question, suggest something, or get help
diff --git a/docs/overview/glossary.rst b/docs/overview/glossary.rst
index a7fec1b41f195..e00facf819e3b 100644
--- a/docs/overview/glossary.rst
+++ b/docs/overview/glossary.rst
@@ -30,10 +30,10 @@ All of these new words can get confusing! We've created a glossary to help nail
A wrapper function around native compiler functions, which uses lower level compilers such as XLA to compile to lower level languages such as C++, CUDA, TorchScript, etc.
Graph Compiler
- Graph compilers map the high-level computational graph coming from frameworks to operations that are executable on a specific device.
+ Graph Compilers map the high-level computational graph coming from frameworks to operations that are executable on a specific device.
- Ivy Graph Compiler
- Ivy's Graph Compiler traces the graph as a composition of functions in the functional API in Python.
+ Ivy Tracer
+ Ivy's Tracer creates a graph as a composition of functions in the functional API in Python.
Ivy Functional API
Is used for defining complex models, the Ivy functional API does not implement its own backend but wraps around other frameworks functional APIs and brings them into alignment.
diff --git a/docs/overview/one_liners.rst b/docs/overview/one_liners.rst
index 0b11527b0b132..e3c53cbff6e47 100644
--- a/docs/overview/one_liners.rst
+++ b/docs/overview/one_liners.rst
@@ -4,10 +4,10 @@ One liners
.. grid:: 1 1 3 3
:gutter: 4
- .. grid-item-card:: ``ivy.compile()``
- :link: one_liners/compile.rst
+ .. grid-item-card:: ``ivy.trace_graph()``
+ :link: one_liners/trace.rst
- Compiles a ``Callable`` or set of them into an Ivy graph.
+ Traces a ``Callable`` or set of them into an Ivy graph.
.. grid-item-card:: ``ivy.transpile()``
:link: one_liners/transpile.rst
@@ -25,6 +25,6 @@ One liners
:hidden:
:maxdepth: -1
- one_liners/compile.rst
+ one_liners/trace.rst
one_liners/transpile.rst
one_liners/unify.rst
diff --git a/docs/overview/one_liners/compile.rst b/docs/overview/one_liners/trace.rst
similarity index 69%
rename from docs/overview/one_liners/compile.rst
rename to docs/overview/one_liners/trace.rst
index 98d3cfd826a3a..05000be5870d2 100644
--- a/docs/overview/one_liners/compile.rst
+++ b/docs/overview/one_liners/trace.rst
@@ -1,35 +1,35 @@
-``ivy.compile()``
-=================
+``ivy.trace_graph()``
+=====================
..
- β οΈ **Warning**: The compiler and the transpiler are not publicly available yet, so certain parts of this doc won't work as expected as of now!
+ β οΈ **Warning**: The tracer and the transpiler are not publicly available yet, so certain parts of this doc won't work as expected as of now!
When we call an Ivy function, there is always a small performance hit due to added
Python wrapping. This overhead becomes increasingly noticeable when we use large
-models with multiple function calls. The Graph Compiler improves the performance of
+models with multiple function calls. The Tracer improves the performance of
Ivy by removing the extra wrapping around each function call.
-The Graph Compiler takes in any Ivy function, framework-specific (backend) function,
+The Tracer takes in any Ivy function, framework-specific (backend) function,
or composition of both, and produces a simplified executable computation graph composed
of functions from the backend functional API only, which results in:
-- Simplified code: The Graph Compiler simplifies the code by removing all the wrapping
+- Simplified code: The Tracer simplifies the code by removing all the wrapping
and functions that don't contribute to the output: print statements, loggers, etc.
-- Improved performance: The compiled graph has no performance overhead due to Ivy's
+- Improved performance: The created graph has no performance overhead due to Ivy's
function wrapping, likewise, redundant operations from the original function are also
removed, increasing its overall performance.
-Compiler API
+Tracer API
------------
-.. py:function:: ivy.compile(*objs, stateful = None, arg_stateful_idxs = None, kwarg_stateful_idxs = None, to = None, include_generators = True, array_caching = True, return_backend_compiled_fn = False, static_argnums = None, static_argnames = None, args = None, kwargs = None,)
+.. py:function:: ivy.trace_graph(*objs, stateful = None, arg_stateful_idxs = None, kwarg_stateful_idxs = None, to = None, include_generators = True, array_caching = True, return_backend_traced_fn = False, static_argnums = None, static_argnames = None, args = None, kwargs = None,)
- Compiles a ``Callable`` or set of them into an Ivy graph. If ``args`` or ``kwargs`` are specified,
+ Creates a ``Callable`` or set of them into an Ivy graph. If ``args`` or ``kwargs`` are specified,
compilation is performed eagerly, otherwise, compilation will happen lazily.
- :param objs: Callable(s) to compile and create a graph of.
+ :param objs: Callable(s) to trace and create a graph of.
:type objs: ``Callable``
:param stateful: List of instances to be considered stateful during the graph compilation.
:type stateful: ``Optional[List]``
@@ -37,14 +37,14 @@ Compiler API
:type arg_stateful_idxs: ``Optional[List]``
:param kwarg_stateful_idxs: Keyword arguments to be considered stateful during the graph compilation.
:type kwarg_stateful_idxs: ``Optional[List]``
- :param to: Backend that the graph will be compiled to. If not specified, the current backend will be used.
+ :param to: Backend that the graph will be traced to. If not specified, the current backend will be used.
:type to: ``Optional[str]``
:param include_generators: Include array creation/generation functions as part of the graph.
:type include_generators: ``bool``
:param array_caching: Cache the constant arrays that appear as arguments to the functions in the graph.
:type array_caching: ``bool``
- :param return_backend_compiled_fn: Whether to apply the native compilers, i.e. tf.function, after ivy's compilation.
- :type return_backend_compiled_fn: ``bool``
+ :param return_backend_traced_fn: Whether to apply the native compilers, i.e. tf.function, after ivy's compilation.
+ :type return_backend_traced_fn: ``bool``
:param static_argnums: For jax's jit compilation.
:type static_argnums: ``Optional[Union[int, Iterable[int]]]``
:param static_argnames: For jax's jit compilation.
@@ -54,12 +54,12 @@ Compiler API
:param kwargs: Keyword arguments for obj.
:type kwargs: ``Optional[dict]``
:rtype: ``Union[Graph, LazyGraph, ivy.Module, ModuleType]``
- :return: A compiled ``Graph`` or a non-initialized ``LazyGraph``. If the object is an ``ivy.Module``, the forward pass will be compiled and the same module will be returned. If the object is a ``ModuleType``, the function will return a copy of the module with every method lazily compiled.
+ :return: A ``Graph`` or a non-initialized ``LazyGraph``. If the object is an ``ivy.Module``, the forward pass will be traced and the same module will be returned. If the object is a ``ModuleType``, the function will return a copy of the module with every method lazily traced.
-Using the compiler
+Using the tracer
------------------
-To use the ``ivy.compile()`` function, you need to pass a callable object and the corresponding inputs
+To use the ``ivy.trace_graph()`` function, you need to pass a callable object and the corresponding inputs
to the function.
Let's start with a simple function:
@@ -81,10 +81,10 @@ Let's start with a simple function:
x = ivy.array([1, 2, 3])
y = ivy.array([2, 3, 4])
- # Compile the function
- compiled_fn = ivy.compile(fn, args=(x, y))
+ # Trace the function
+ traced_fn = ivy.trace_graph(fn, args=(x, y))
-In this case, the compiled graph would be:
+In this case, the created graph would be:
.. image:: https://raw.githubusercontent.com/unifyai/unifyai.github.io/main/img/externally_linked/compiler/figure1.png
@@ -93,49 +93,49 @@ From the graph, we can observe that:
1. As ``x`` and ``y`` are the only variables used when calculating the returned value ``z``,
the non-contributing variable(s), ``k`` was not included in the graph. Function calls that
don't contribute to the output like the ``print`` function were also excluded.
-2. As we set the backend to ``torch`` during the compilation process, the compiled
+2. As we set the backend to ``torch`` during the compilation process, the traced
functions are torch functions, and the input and output types are torch tensors.
3. The tensor shape in the graph only indicates the shape of the inputs the graph was
- traced with. The compiler doesn't impose additional restrictions on the shape or
+ traced with. The tracer doesn't impose additional restrictions on the shape or
datatype of the input array(s).
.. code-block:: python
# Original set of inputs
- out = compiled_fn(x, y)
+ out = traced_fn(x, y)
# Inputs of different shape
a = ivy.array([[1., 2.]])
b = ivy.array([[2., 3.]])
# New set of inputs
- out = compiled_fn(a, b)
+ out = traced_fn(a, b)
Eager vs lazy Compilation
~~~~~~~~~~~~~~~~~~~~~~~~~
-The graph compiler runs the original function under the hood and tracks its computation
-to create the compiled graph. The **eager compilation** method traces the graph in the
-corresponding function call with the specified inputs before we use the compiled
+The Tracer runs the original function under the hood and tracks its computation
+to create the created graph. The **eager compilation** method traces the graph in the
+corresponding function call with the specified inputs before we use the traced
function.
-Instead of compiling functions before using them, Ivy also allows you to compile the
+Instead of compiling functions before using them, Ivy also allows you to trace the
function dynamically. This can be done by passing only the function to the
-compile method and not including the function arguments. In this case, the output will be a
+trace method and not including the function arguments. In this case, the output will be a
``LazyGraph`` instead of a ``Graph`` instance. When this ``LazyGraph`` object is first invoked with
-function arguments, it compiles the function and returns the output of the compiled
+function arguments, it Creates the function and returns the output of the traced
function. Once the graph has been initialized, calls to the ``LazyGraph`` object will
-use the compiled function to compute the outputs directly.
+use the traced function to compute the outputs directly.
.. code-block:: python
- # Compile the function eagerly (compilation happens here)
- eager_graph = ivy.compile(fn, args=(x, y))
+ # Trace the function eagerly (compilation happens here)
+ eager_graph = ivy.trace_graph(fn, args=(x, y))
- # Compile the function lazily (compilation does not happen here)
- lazy_graph = ivy.compile(fn)
+ # Trace the function lazily (compilation does not happen here)
+ lazy_graph = ivy.trace_graph(fn)
- # Compile and return the output
+ # Trace and return the output
out = lazy_graph(x, y)
To sum up, lazy compilation enables you to delay the compilation process until you have
@@ -144,12 +144,12 @@ compiling libraries, where itβs not feasible to provide valid arguments for ev
function call.
Now let's look at additional functionalities that you can find in the
-compiler.
+tracer.
Array caching
~~~~~~~~~~~~~
-The compiler is able to cache constant arrays and their operations through the
+The tracer is able to cache constant arrays and their operations through the
``array_caching`` flag, reducing computation time after compilation.
.. code-block:: python
@@ -164,9 +164,9 @@ The compiler is able to cache constant arrays and their operations through the
z = x ** (a + b)
return z
- comp_func = ivy.compile(fn, args=(x,))
+ comp_func = ivy.trace_graph(fn, args=(x,))
-When calling ``ivy.compile()``, the ``array_caching`` argument is set to ``True`` by
+When calling ``ivy.trace_graph()``, the ``array_caching`` argument is set to ``True`` by
default, which returns the following graph.
.. image:: https://raw.githubusercontent.com/unifyai/unifyai.github.io/main/img/externally_linked/compiler/figure2.png
@@ -196,7 +196,7 @@ are included as nodes or "baked" into the graph.
z = x ** a
return z + torch.rand([1])
- comp_func = ivy.compile(fn, include_generators=True, args=(x,))
+ comp_func = ivy.trace_graph(fn, include_generators=True, args=(x,))
Returns:
@@ -215,7 +215,7 @@ And instead,
z = x * a
return z + torch.rand([1])
- comp_func = ivy.compile(fn, include_generators=False, args=(x,))
+ comp_func = ivy.trace_graph(fn, include_generators=False, args=(x,))
Returns:
@@ -241,32 +241,32 @@ arbitrary classes using the ``stateful`` parameters.
cont = ivy.Container(x=x)
args = (cont.cont_deep_copy(), x)
- comp_func = ivy.compile(fn, arg_stateful_idxs=[[0]], args=args)
+ comp_func = ivy.trace_graph(fn, arg_stateful_idxs=[[0]], args=args)
.. image:: https://raw.githubusercontent.com/unifyai/unifyai.github.io/main/img/externally_linked/compiler/figure6.png
Sharp bits
----------
-As some parts of the graph compiler are still under development, there are some sharp
+As some parts of the Tracer are still under development, there are some sharp
bits to take into account when using it. All of these points are WIP, so they'll be
removed soon!
-1. **Dynamic control flow**: The compiled graph is built using function tracing at the
+1. **Dynamic control flow**: The created graph is built using function tracing at the
moment, so dynamic control flow such as conditional branches or conditional loops
will not be registered correctly. As an example, if there is a while loop in your
code that depends on a changing value, the number of iterations in the final graph
will be the same as the number of iterations performed with the input passed to the
- compile function.
-2. **Non-framework-specific code**: As the compiler traces the function using the
+ trace function.
+2. **Non-framework-specific code**: As the tracer traces the function using the
functional API of the underlying framework, any piece of code inside the model that
is not from the said framework will not be correctly registered, this includes other
frameworks code (such as NumPy statements inside a torch model) or python statements
such as len().
3. **Incorrectly cached parts of the graph**: There are certain cases where compilation
can succeed but hide some cached parts of the graph which shouldn't really be cached.
- To check this, it's recommended to compile with a noise array of the same shape and
- then check if the output of the original function and the compiled graph with another
+ To check this, it's recommended to trace with a noise array of the same shape and
+ then check if the output of the original function and the created graph with another
input is the same. If you find out that the graph is not right, feel free to open an
`issue `_ with a minimal example and we'll look
into it!
@@ -274,7 +274,7 @@ removed soon!
Examples
--------
-Below, we compile a ResNet50 model from
+Below, we trace a ResNet50 model from
`Hugging Face `_ and use it to classify the
breed of a cat.
@@ -306,15 +306,15 @@ Normally, we would then feed these inputs to the model itself without compiling
with torch.no_grad():
logits = model(**inputs).logits
-With ivy, you can compile your model to a computation graph for increased performance.
+With ivy, you can trace your model to a computation graph for increased performance.
.. code-block:: python
# Compiling the model
- compiled_graph = ivy.compile(model, args=(**inputs,))
+ traced_graph = ivy.trace_graph(model, args=(**inputs,))
- # Using the compiled function
- logits = compiled_graph(**inputs).logits
+ # Using the traced function
+ logits = traced_graph(**inputs).logits
Time for the final output of our computation graph.
diff --git a/docs/overview/one_liners/transpile.rst b/docs/overview/one_liners/transpile.rst
index 701be359e3165..eebd3d99806d2 100644
--- a/docs/overview/one_liners/transpile.rst
+++ b/docs/overview/one_liners/transpile.rst
@@ -1,9 +1,9 @@
``ivy.transpile()``
-=================
+===================
..
- β οΈ **Warning**: The compiler and the transpiler are not publicly available yet, so certain parts of this doc won't work as expected as of now!
+ β οΈ **Warning**: The tracer and the transpiler are not publicly available yet, so certain parts of this doc won't work as expected as of now!
Ivy's Transpiler converts a function written in any framework into your framework of
@@ -24,10 +24,10 @@ want to use to research, develop, or deploy systems. So if you want to:
Ivy's Transpiler is definitely the tool for the job π§
-To convert the code, it traces a computational graph using the Graph Compiler and
+To convert the code, it traces a computational graph using the Tracer and
leverages Ivy's frontends and backends to link one framework to another. After swapping
each function node in the computational graph with their equivalent Ivy frontend
-functions, the compiler removes all the wrapping in the frontends and replaces them with the native
+functions, the tracer removes all the wrapping in the frontends and replaces them with the native
functions of the target framework.
@@ -61,7 +61,7 @@ Transpiler API
Using the transpiler
--------------------
-Similar to the ``ivy.compile`` function, ``ivy.unify`` and ``ivy.transpile`` can be used
+Similar to the ``ivy.trace`` function, ``ivy.unify`` and ``ivy.transpile`` can be used
eagerly and lazily. If you pass the necessary arguments, the function will be called
instantly, otherwise, transpilation will happen the first time you invoke the function
with the proper arguments.
@@ -154,7 +154,7 @@ another, at the moment we support ``torch.nn.Module`` when ``to="torch"``,
# Build a classifier using the transpiled encoder
class Classifier(hk.Module):
def __init__(self, num_classes=1000):
- super(Classifier, self).__init__()
+ super().__init__()
self.encoder = mlp_encoder()
self.fc = hk.Linear(output_size=num_classes, with_bias=True)
@@ -178,7 +178,7 @@ another, at the moment we support ``torch.nn.Module`` when ``to="torch"``,
Sharp bits
----------
-In a similar fashion to the compiler, the transpiler is under development and we are
+In a similar fashion to the trace, the transpiler is under development and we are
still working on some rough edges. These include:
1. **Keras model subclassing**: If a model is transpiled to keras, the resulting
@@ -195,15 +195,15 @@ still working on some rough edges. These include:
3. **Haiku transform with state**: As of now, we only support the transpilation of
transformed Haiku modules, this means that ``transformed_with_state`` objects will
not be correctly transpiled.
-4. **Array format between frameworks**: As the compiler outputs a 1-to-1 mapping of the
- compiled function, the format of the tensors is preserved when transpiling from a
+4. **Array format between frameworks**: As the tracer outputs a 1-to-1 mapping of the
+ traced function, the format of the tensors is preserved when transpiling from a
framework to another. As an example, if you transpile a convolutional block from
PyTorch (which uses ``N, C, H, W``) to TensorFlow (which uses ``N, H, W, C``) and want
to use it as part of a bigger (TensorFlow) model, you'll need to include a permute statement for
the inference to be correct.
-Keep in mind that the transpiler uses the graph compiler under the hood, so the
-:ref:`sharp bits of the compiler `
+Keep in mind that the transpiler uses the Tracer under the hood, so the
+:ref:`sharp bits of the tracer `
apply here as well!
Examples
diff --git a/docs/overview/one_liners/unify.rst b/docs/overview/one_liners/unify.rst
index a07ac2fbf5b40..687ab07293f1f 100644
--- a/docs/overview/one_liners/unify.rst
+++ b/docs/overview/one_liners/unify.rst
@@ -1,9 +1,9 @@
``ivy.unify()``
-================
+===============
..
- β οΈ **Warning**: The compiler and the transpiler are not publicly available yet, so certain parts of this doc won't work as expected as of now!
+ β οΈ **Warning**: The tracer and the transpiler are not publicly available yet, so certain parts of this doc won't work as expected as of now!
Ivy's Unify function is an alias for ``ivy.transpile(..., to="ivy", ...)``. You can know
more about the transpiler in the `transpile() `_ page.
diff --git a/docs/overview/related_work/what_does_ivy_add.rst b/docs/overview/related_work/what_does_ivy_add.rst
index 14a407d24a751..7bd10805ce8ea 100644
--- a/docs/overview/related_work/what_does_ivy_add.rst
+++ b/docs/overview/related_work/what_does_ivy_add.rst
@@ -51,11 +51,11 @@ It therefore extends what is possible in any of the specific individual framewor
Graph Tracers
-------------
-Ivyβs `Graph Compiler <../one_liners/compile>`_ exhibits similar properties to many of the framework-specific graph tracers.
-Ivyβs graph compiler employs function tracing for computing the graph, and uses this graph as an intermediate representation during the transpilation process.
-Of all the graph tracers, Ivyβs graph compiler is most similar to `torch.fx`_.
+Ivyβs `Tracer <../one_liners/trace>`_ exhibits similar properties to many of the framework-specific graph tracers.
+Ivyβs tracer employs function tracing for computing the graph, and uses this graph as an intermediate representation during the transpilation process.
+Of all the graph tracers, Ivyβs tracer is most similar to `torch.fx`_.
This is because :code:`torch.fx` also operates entirely in Python, without deferring to lower level languages for tracing and extracting the computation graph or the intermediate representation.
-The main difference is that Ivyβs graph compiler is fully framework-agnostic; Ivyβs compiler is able to compile graphs from any framework, while framework-specific compilers are of course bound to their particular framework.
+The main difference is that Ivyβs tracer is fully framework-agnostic; Ivyβs tracer is able to trace graphs from any framework, while framework-specific tracers are of course bound to their particular framework.
Exchange Formats
----------------
@@ -105,6 +105,6 @@ Firstly, we are adhering to the `Array API Standard`_ defined by Quansight.
In essence, they have written the standard and we have implemented it, which is pretty much as complementary as it gets.
Similarly, OctoML makes it easy for anyone to *deploy* their model anywhere, while Ivy makes it easy for anyone to mix and match any code from any frameworks and versions to *train* their model anywhere.
Again very complementary objectives.
-Finally, Modular will perhaps make it possible for developers to make changes at various levels of the stack when creating ML models using their "", and this would also be a great addition to the field.
+Finally, Modular will perhaps make it possible for developers to make changes at various levels of the stack when creating ML models using their infrastructure, and this would also be a great addition to the field.
Compared to Modular which focuses on the lower levels of the stack, Ivy instead unifies the ML frameworks at the functional API level, enabling code conversions to and from the user-facing APIs themselves, without diving into any of the lower level details.
All of these features are entirely complementary, and together would form a powerful suite of unifying tools for ML practitioners.
diff --git a/install_dependencies.sh b/install_dependencies.sh
index 628ae1ff2e9a0..d5dbcc660307b 100755
--- a/install_dependencies.sh
+++ b/install_dependencies.sh
@@ -1,3 +1,6 @@
+# This shell script is required by the doc-builder. Moving it might break
+# the doc-building pipeline
+
sudo apt-get update
sudo apt-get install pandoc -y
pip install -r requirements/requirements.txt
diff --git a/ivy/__init__.py b/ivy/__init__.py
index 01a621b5a3a3b..1faf53a31acdf 100644
--- a/ivy/__init__.py
+++ b/ivy/__init__.py
@@ -2,6 +2,7 @@
import copy
import re
import warnings
+import logging
import builtins
import numpy as np
import sys
@@ -258,6 +259,11 @@ def __repr__(self):
f"ivy.Shape({shape_repr})" if self._shape is not None else "ivy.Shape(None)"
)
+ def __deepcopy__(self, memo):
+ ret = self.__class__.__new__(self.__class__)
+ ret._shape = self.shape
+ return ret
+
def __iter__(self):
return iter(self._shape)
@@ -398,10 +404,6 @@ def index(self, index):
else:
return self._shape[index]
- @property
- def shape(self):
- return self._shape
-
def as_dimension(self):
if isinstance(self._shape, Shape):
return self._shape
@@ -439,7 +441,7 @@ def unknown_shape(rank=None, **kwargs):
def with_rank(self, rank):
try:
- return self.merge_with(unknown_shape(rank=rank))
+ return self.merge_with(self.unknown_shape(rank=rank))
except ValueError:
raise ValueError(f"Shape {self} must have rank {rank}")
@@ -478,8 +480,7 @@ def is_fully_defined(self):
shape is not None for shape in self._shape
)
- property
-
+ @property
def num_elements(self):
if not self.is_fully_defined():
return None
@@ -789,12 +790,12 @@ class Node(str):
try:
from .engines import XLA as xla
from .engines import ivy2xla
-except:
+except: # noqa: E722
pass
try:
from .compiler.compiler import transpile, trace_graph, unify
except: # noqa: E722
- pass # Added for the finally statment
+ pass # Added for the finally statement
finally:
# Skip framework imports done by Ivy compiler for now
for backend_framework in _not_imported_backends.copy():
@@ -992,7 +993,7 @@ def _assert_array_significant_figures_formatting(sig_figs):
ivy.utils.assertions.check_greater(sig_figs, 0, as_array=False)
-# ToDo: SF formating for complex number
+# ToDo: SF formatting for complex number
def vec_sig_fig(x, sig_fig=3):
if isinstance(x, np.bool_):
return x
@@ -1502,7 +1503,7 @@ def __setattr__(self, name, value, internal=False):
if (
- "ivy" in sys.modules.keys()
+ "ivy" in sys.modules
and sys.modules["ivy"].utils._importlib.IS_COMPILING_WITH_BACKEND
):
# Required for ivy.with_backend internal compilation
diff --git a/ivy/_version.py b/ivy/_version.py
index 96f906838bd10..d1bfff206d3b1 100644
--- a/ivy/_version.py
+++ b/ivy/_version.py
@@ -1 +1 @@
-__version__ = "0.0.3.0"
+__version__ = "0.0.4.0"
diff --git a/ivy/compiler/compiler.py b/ivy/compiler/compiler.py
index 672394c3c28c2..97f8ada162541 100644
--- a/ivy/compiler/compiler.py
+++ b/ivy/compiler/compiler.py
@@ -1,14 +1,4 @@
-from typing import Callable, Optional, List, Union, Iterable, Tuple, Any
-
-
-# TODO: create meaningful types for Graph and LazyGraph,
-# will probably need a seperate file for that
-class Graph:
- pass
-
-
-class LazyGraph:
- pass
+from typing import Callable, Optional, List, Union, Iterable, Tuple, Mapping
def trace_graph(
@@ -26,8 +16,10 @@ def trace_graph(
mode: Optional[str] = None,
graph_caching: bool = False,
args: Optional[Tuple] = None,
- kwargs: Optional[dict] = None,
-) -> Union[Graph, LazyGraph]:
+ kwargs: Optional[Mapping] = None,
+ params_v=None,
+ v=None
+):
"""
Take `fn` and traces it into a more efficient composition of backend operations.
@@ -36,17 +28,17 @@ def trace_graph(
objs
callable(s) to trace and create a graph of
stateful
- list of instances to be considered stateful during the graph compilation
+ list of instances to be considered stateful during the graph tracing
arg_stateful_idxs
- positional arguments to be considered stateful during the graph compilation
+ positional arguments to be considered stateful during the graph tracing
kwarg_stateful_idxs
- keyword arguments to be considered stateful during the graph compilation
+ keyword arguments to be considered stateful during the graph tracing
include_generators
include array creation/generation functions as part of the graph
array_caching
cache the constant arrays that appear as arguments to the functions in the graph
backend_compile
- whether to apply the native compilers, i.e. tf.function, after ivy's compilation
+ whether to apply the native compilers, i.e. tf.function, after ivy's tracing
static_argnums
for jax's jit compilation
static_argnames
@@ -67,7 +59,7 @@ def trace_graph(
Examples
--------
>>> import ivy, time
- >>> from ivy import compile
+ >>> from ivy import trace_graph
>>> ivy.set_backend("torch")
>>> x = ivy.array([1.])
@@ -98,7 +90,7 @@ def trace_graph(
>>> print(time.time() - start)
0.0001785755157470703
"""
- from ._compiler import compile as _trace_graph
+ from ._compiler import trace_graph as _trace_graph
return _trace_graph(
*objs,
@@ -116,6 +108,8 @@ def trace_graph(
graph_caching=graph_caching,
args=args,
kwargs=kwargs,
+ params_v=params_v,
+ v=v,
)
@@ -133,10 +127,10 @@ def transpile(
arg_stateful_idxs: Optional[List] = None,
kwarg_stateful_idxs: Optional[List] = None,
args: Optional[Tuple] = None,
- kwargs: Optional[Any] = None,
+ kwargs: Optional[Mapping] = None,
params_v=None,
- v=None, # Make this cleaner
-) -> Union[Graph, LazyGraph]:
+ v=None
+):
"""
Transpiles Callable objects passed as arguments. If args and kwargs are specified,
transpilation is performed eagerly, otherwise, transpilation will happen lazily.
@@ -185,10 +179,10 @@ def unify(
source: Optional[str] = None,
graph_caching: bool = False,
args: Optional[Tuple] = None,
- kwargs: Optional[dict] = None,
+ kwargs: Optional[Mapping] = None,
with_numpy: bool = True,
- **transpile_kwargs,
-) -> Callable:
+ **transpile_kwargs
+):
from ._compiler import unify as _unify
return _unify(
diff --git a/ivy/data_classes/array/array.py b/ivy/data_classes/array/array.py
index d290ac8f4071e..46aa785c67afe 100644
--- a/ivy/data_classes/array/array.py
+++ b/ivy/data_classes/array/array.py
@@ -160,7 +160,7 @@ def _init(self, data, dynamic_backend=None):
self._dev_str = None
self._pre_repr = None
self._post_repr = None
- self._backend = ivy.backend
+ self._backend = ivy.current_backend(self._data).backend
if dynamic_backend is not None:
self._dynamic_backend = dynamic_backend
else:
@@ -188,28 +188,27 @@ def dynamic_backend(self):
@dynamic_backend.setter
def dynamic_backend(self, value):
- from ivy.functional.ivy.gradients import _variable, _is_variable, _variable_data
- from ivy.utils.backend.handler import _determine_backend_from_args
+ from ivy.functional.ivy.gradients import _variable
+ from ivy.utils.backend.handler import _data_to_new_backend, _get_backend_for_arg
- if value == False:
- self._backend = _determine_backend_from_args(self).backend
-
- else:
+ if value:
ivy_backend = ivy.with_backend(self._backend)
- to_numpy = ivy_backend.to_numpy
- if _is_variable(self.data) and not self._backend in ["jax", "numpy"]:
- native_data = _variable_data(self.data)
- np_data = to_numpy(native_data)
- new_arr = ivy.array(np_data)
- self._data = _variable(new_arr).data
+ if ivy_backend.gradients._is_variable(self.data):
+ native_var = ivy_backend.gradients._variable_data(
+ self,
+ )
+ data = _data_to_new_backend(native_var, ivy_backend).data
+ self._data = _variable(data).data
else:
- np_data = to_numpy(self.data)
- self._data = ivy.array(np_data).data
+ self._data = _data_to_new_backend(self, ivy_backend).data
self._backend = ivy.backend
+ else:
+ self._backend = _get_backend_for_arg(self.data.__class__.__module__).backend
+
self._dynamic_backend = value
@property
@@ -401,13 +400,7 @@ def __repr__(self):
self._post_repr = ")"
sig_fig = ivy.array_significant_figures
dec_vals = ivy.array_decimal_values
- if self.backend == "" or ivy.is_local():
- # If the array was constructed using implicit backend
- backend = ivy.current_backend()
- else:
- # Requirerd in the case that backend is different
- # from the currently set backend
- backend = ivy.with_backend(self.backend)
+ backend = ivy.with_backend(self.backend)
arr_np = backend.to_numpy(self._data)
rep = (
np.array(ivy.vec_sig_fig(arr_np, sig_fig))
@@ -446,7 +439,7 @@ def __contains__(self, key):
return self._data.__contains__(key)
def __getstate__(self):
- data_dict = dict()
+ data_dict = {}
# only pickle the native array
data_dict["data"] = self.data
@@ -671,10 +664,10 @@ def __imod__(self, other):
return ivy.remainder(self._data, other)
def __divmod__(self, other):
- return tuple([ivy.divide(self._data, other), ivy.remainder(self._data, other)])
+ return ivy.divide(self._data, other), ivy.remainder(self._data, other)
def __rdivmod__(self, other):
- return tuple([ivy.divide(other, self._data), ivy.remainder(other, self._data)])
+ return ivy.divide(other, self._data), ivy.remainder(other, self._data)
def __truediv__(self, other):
"""
diff --git a/ivy/data_classes/array/creation.py b/ivy/data_classes/array/creation.py
index 795b09c517eb1..94e2ab7a096d3 100644
--- a/ivy/data_classes/array/creation.py
+++ b/ivy/data_classes/array/creation.py
@@ -292,7 +292,7 @@ def empty_like(
input array from which to derive the output array shape.
dtype
output array data type. If dtype is None, the output array data type must be
- inferred from ``self``. Deafult: ``None``.
+ inferred from ``self``. Default: ``None``.
device
device on which to place the created array. If device is None, the output
array device must be inferred from ``self``. Default: ``None``.
diff --git a/ivy/data_classes/array/data_type.py b/ivy/data_classes/array/data_type.py
index d20696c600aef..01cdedea04c58 100644
--- a/ivy/data_classes/array/data_type.py
+++ b/ivy/data_classes/array/data_type.py
@@ -19,7 +19,7 @@ def astype(
out: Optional[ivy.Array] = None,
) -> ivy.Array:
"""
- Copy an array to a specified data type irrespective of :ref:`type-promotion`
+ Copy an array to a specified data type irrespective of :ref:`type- promotion`
rules.
.. note::
diff --git a/ivy/data_classes/array/elementwise.py b/ivy/data_classes/array/elementwise.py
index c9ce05589e45b..f33601eac27da 100644
--- a/ivy/data_classes/array/elementwise.py
+++ b/ivy/data_classes/array/elementwise.py
@@ -1438,6 +1438,27 @@ def log2(self: ivy.Array, *, out: Optional[ivy.Array] = None) -> ivy.Array:
an array containing the evaluated base ``2`` logarithm for each element
in ``self``. The returned array must have a real-valued floating-point
data type determined by :ref:`type-promotion`.
+
+ Examples
+ --------
+ Using :code:`ivy.Array` instance method:
+
+ >>> x = ivy.array([5.0, 1, -0.0, -6.0])
+ >>> y = ivy.log2(x)
+ >>> print(y)
+ ivy.array([2.32, 0., -inf, nan])
+
+ >>> x = ivy.array([float('nan'), -5.0, -0.0, 1.0, 5.0, float('+inf')])
+ >>> y = x.log2()
+ >>> print(y)
+ ivy.array([nan, nan, -inf, 0., 2.32, inf])
+
+ >>> x = ivy.array([[float('nan'), 1, 5.0, float('+inf')],\
+ [+0, -2.0, -5, float('-inf')]])
+ >>> y = x.log2()
+ >>> print(y)
+ ivy.array([[nan, 0., 2.32, inf],
+ [-inf, nan, nan, nan]])
"""
return ivy.log2(self._data, out=out)
diff --git a/ivy/data_classes/array/experimental/activations.py b/ivy/data_classes/array/experimental/activations.py
index 50db0fbbdca1a..24e38a9dc52ff 100644
--- a/ivy/data_classes/array/experimental/activations.py
+++ b/ivy/data_classes/array/experimental/activations.py
@@ -25,7 +25,7 @@ def logit(
self
Input array.
eps
- When eps is None the function outpus NaN where x < 0 or x > 1.
+ When eps is None the function outputs NaN where x < 0 or x > 1.
and inf or -inf where x = 1 or x = 0, respectively.
Otherwise if eps is defined, x is clamped to [eps, 1 - eps]
complex_mode
@@ -337,3 +337,235 @@ def hardtanh(
ivy.array([-1., 1., 1.])
"""
return ivy.hardtanh(self._data, min_val=min_val, max_val=max_val, out=out)
+
+ def tanhshrink(self: ivy.Array, /, *, out: Optional[ivy.Array] = None) -> ivy.Array:
+ """
+ ivy.Array instance method variant of ivy.tanhshrink. This method simply wraps
+ the function, and so the docstring for ivy.tanhshrink also applies to this
+ method with minimal changes.
+
+ Parameters
+ ----------
+ self
+ input array.
+ out
+ optional output array, for writing the result to. It must have a shape
+ that the inputs broadcast to.
+
+ Examples
+ --------
+ >>> x = ivy.array([-1., 0., 1.])
+ >>> y = x.tanhshrink()
+ >>> print(y)
+ ivy.array([-0.23840582, 0. , 0.23840582])
+ """
+ return ivy.tanhshrink(self._data, out=out)
+
+ def threshold(
+ self: ivy.Array,
+ /,
+ *,
+ threshold: Union[int, float],
+ value: Union[int, float],
+ out: Optional[ivy.Array] = None,
+ ) -> ivy.Array:
+ """
+ ivy.Array instance method variant of ivy.threshold. This method simply wraps the
+ function, and so the docstring for ivy.threshold also applies to this method
+ with minimal changes.
+
+ Parameters
+ ----------
+ self
+ input array.
+ threshold
+ threshold value for thresholding operation.
+ value
+ value to replace with if thresholding condition is not met.
+ out
+ optional output array, for writing the result to. It must have a shape
+ that the inputs broadcast to.
+
+ Returns
+ -------
+ ret
+ an array with the thresholding function applied element-wise.
+
+ Examples
+ --------
+ >>> x = ivy.array([-1., 0., 1.])
+ >>> y = x.hreshold(threshold=0.5, value=0.0)
+ >>> print(y)
+ ivy.array([0.5, 0.5 , 1. ])
+ """
+ return ivy.threshold(self._data, threshold=threshold, value=value, out=out)
+
+ def softshrink(
+ self: ivy.Array,
+ /,
+ *,
+ lambd: float = 0.5,
+ out: Optional[ivy.Array] = None,
+ ) -> ivy.Array:
+ """
+ ivy.Array instance method variant of ivy.softshrink. This method simply wraps
+ the function, and so the docstring for ivy.softshrink also applies to this
+ method with minimal changes.
+
+ Parameters
+ ----------
+ self
+ input array.
+ lambd
+ the value of the lower bound of the linear region range.
+ out
+ optional output array, for writing the result to. It must have a shape
+ that the inputs broadcast to.
+
+ Returns
+ -------
+ ret
+ an array with the softshrink activation function applied element-wise.
+
+ Examples
+ --------
+ >>> x = ivy.array([-1., 0., 1.])
+ >>> y = x.softshrink()
+ >>> print(y)
+ ivy.array([-0.5, 0. , 0.5])
+ >>> x = ivy.array([-1., 0., 1.])
+ >>> y = x.softshrink(lambd=1.0)
+ >>> print(y)
+ ivy.array([0., 0., 0.])
+ """
+ return ivy.softshrink(self._data, lambd=lambd, out=out)
+
+ def celu(
+ self: ivy.Array,
+ /,
+ *,
+ alpha: float = 1.0,
+ complex_mode: Literal["split", "magnitude", "jax"] = "jax",
+ out: Optional[ivy.Array] = None,
+ ) -> ivy.Array:
+ """
+ ivy.Array instance method variant of ivy.celu. This method simply wraps the
+ function, and so the docstring for ivy.celu also applies to this method with
+ minimal changes.
+
+ Parameters
+ ----------
+ self
+ input array.
+ alpha
+ the alpha (negative slope) value for CELU formulation.
+ complex_mode
+ optional specifier for how to handle complex data types. See
+ ``ivy.func_wrapper.handle_complex_input`` for more detail.
+ out
+ optional output array, for writing the result to. It must have a shape
+ that the inputs broadcast to.
+
+ Returns
+ -------
+ ret
+ an array with the celu activation function applied element-wise.
+
+ Examples
+ --------
+ >>> x = ivy.array([0.39, -0.85])
+ >>> y = x.celu()
+ >>> print(y)
+ ivy.array([ 0.39, -0.57])
+ """
+ return ivy.celu(self._data, alpha=alpha, complex_mode=complex_mode, out=out)
+
+ def scaled_tanh(
+ self: ivy.Array,
+ /,
+ *,
+ alpha: float = 1.7159,
+ beta: float = 0.67,
+ out: Optional[ivy.Array] = None,
+ ) -> ivy.Array:
+ """
+ ivy.Array instance method variant of ivy.scaled_tanh. This method simply wraps
+ the function, and so the docstring for ivy.scaled_tanh also applies to this
+ method with minimal changes.
+
+ Parameters
+ ----------
+ self
+ input array.
+ alpha
+ The scaling parameter for the output.
+ Determines the amplitude of the tanh function.
+ Default: 1.7159
+ beta
+ The scaling parameter for the input.
+ Determines the slope of the tanh function.
+ Default: 0.67
+ out
+ optional output array, for writing the result to. It must have a shape
+ that the inputs broadcast to.
+
+ Returns
+ -------
+ ret
+ an array after applying the scaled_tanh activation.
+
+ Examples
+ --------
+ >>> x = ivy.array([-3., 2., 3.])
+ >>> x.scaled_tanh()
+ ivy.array([-1.65537548, 1.49570239, 1.65537548])
+
+ >>> x = ivy.array([2., 2., 2.])
+ >>> x.scaled_tanh(alpha=9, beta=0.1)
+ ivy.array([1.77637792, 1.77637792, 1.77637792])
+
+ >>> x = ivy.array([2., 2., 2.])
+ >>> x.scaled_tanh(alpha=0.1, beta=9)
+ ivy.array([0.1, 0.1, 0.1])
+ """
+ return ivy.scaled_tanh(self._data, alpha=alpha, beta=beta, out=out)
+
+ def hardshrink(
+ self: ivy.Array,
+ /,
+ *,
+ lambd: float = 0.5,
+ out: Optional[ivy.Array] = None,
+ ) -> ivy.Array:
+ """
+ ivy.Array instance method variant of ivy.hardshrink. This method simply wraps
+ the function, and so the docstring for ivy.hardshrink also applies to this
+ method with minimal changes.
+
+ Parameters
+ ----------
+ self
+ input array.
+ lambd
+ the lambd value for the Hardshrink formulation
+ out
+ optional output array, for writing the result to. It must have a shape
+ that the inputs broadcast to.
+
+ Returns
+ -------
+ ret
+ an array with the hardshrink activation function applied element-wise.
+
+ Examples
+ --------
+ >>> x = ivy.array([-1., 0., 1.])
+ >>> y = x.hardshrink()
+ >>> print(y)
+ ivy.array([-1., 0., 1.])
+ >>> x = ivy.array([-1., 0., 1.])
+ >>> y = x.hardshrink(lambd=1.0)
+ >>> print(y)
+ ivy.array([0., 0., 0.])
+ """
+ return ivy.hardshrink(self._data, lambd=lambd, out=out)
diff --git a/ivy/data_classes/array/experimental/creation.py b/ivy/data_classes/array/experimental/creation.py
index 1bd85ba5170c3..fdca1bcffabf3 100644
--- a/ivy/data_classes/array/experimental/creation.py
+++ b/ivy/data_classes/array/experimental/creation.py
@@ -264,3 +264,89 @@ def mel_weight_matrix(
lower_edge_hertz,
upper_edge_hertz,
)
+
+ def unsorted_segment_mean(
+ self: ivy.Array,
+ segment_ids: ivy.Array,
+ num_segments: Union[int, ivy.Array],
+ ) -> ivy.Array:
+ """
+ Compute the mean of values in the array 'self' based on segment identifiers.
+
+ Parameters
+ ----------
+ self : ivy.Array
+ The array from which to gather values.
+ segment_ids : ivy.Array
+ Must be in the same size with the first dimension of `self`. Has to be
+ of integer data type. The index-th element of `segment_ids` array is
+ the segment identifier for the index-th element of `self`.
+ num_segments : Union[int, ivy.Array]
+ An integer or array representing the total number of distinct segment IDs.
+
+ Returns
+ -------
+ ret : ivy.Array
+ The output array, representing the result of a segmented mean operation.
+ For each segment, it computes the mean of values in `self` where
+ `segment_ids` equals to segment ID.
+
+ Examples
+ --------
+ >>> data = ivy.array([1.0, 2.0, 3.0, 4.0])
+ >>> segment_ids = ivy.array([0, 0, 0, 0])
+ >>> num_segments = 1
+ >>> result = ivy.unsorted_segment_mean(data, segment_ids, num_segments)
+ >>> result
+ ivy.array([2.5])
+
+ >>> data = ivy.array([1.0, 2.0, 3.0, 4.0, 5.0, 6.0])
+ >>> segment_ids = ivy.array([0, 0, 1, 1, 2, 2])
+ >>> num_segments = 3
+ >>> result = ivy.unsorted_segment_mean(data, segment_ids, num_segments)
+ >>> result
+ ivy.array([[1.5, 3.5, 5.5],[1.5, 3.5, 5.5],[1.5, 3.5, 5.5]])
+ """
+ return ivy.unsorted_segment_mean(self._data, segment_ids, num_segments)
+
+
+def polyval(
+ coeffs=ivy.Array,
+ x=Union[ivy.Array, ivy.NativeArray, int, float],
+ /,
+ *,
+ dtype: Optional[Union[ivy.Dtype, ivy.NativeDtype]] = None,
+ device: Optional[Union[ivy.Device, ivy.NativeDevice]] = None,
+) -> ivy.Array:
+ """
+ ivy.Array instance method of polyval. This method simply wraps the function, and so
+ the docstring for ivy.polyval also applies to this method with minimal changes.
+
+ Evaluate and return a polynomial at specific given values.
+
+ Parameters
+ ----------
+ coeffs
+ Input array containing polynomial coefficients (including zero)
+ from highest degree to constant term.
+ x
+ The value of the indeterminate variable at which to evaluate the polynomial.
+
+ Returns
+ -------
+ ret
+ Simplified result of substituing x in the coefficients - final value of
+ polynomial.
+
+ Examples
+ --------
+ >>> x = ivy.array([[0, 0, 0])
+ >>> x.polyval([3, 0, 1], 5)
+ ivy.array(76)
+ """
+ return ivy.polyval(
+ coeffs,
+ x,
+ dtype=dtype,
+ device=device,
+ )
diff --git a/ivy/data_classes/array/experimental/elementwise.py b/ivy/data_classes/array/experimental/elementwise.py
index efabeb1617b63..31e4245b01f15 100644
--- a/ivy/data_classes/array/experimental/elementwise.py
+++ b/ivy/data_classes/array/experimental/elementwise.py
@@ -1,6 +1,6 @@
# global
import abc
-from typing import Optional, Union, Tuple, List
+from typing import Optional, Union, Tuple, List, Sequence
from numbers import Number
# local
@@ -8,6 +8,130 @@
class _ArrayWithElementWiseExperimental(abc.ABC):
+ def amax(
+ self: ivy.Array,
+ /,
+ *,
+ axis: Optional[Union[int, Sequence[int]]] = None,
+ keepdims: bool = False,
+ out: Optional[ivy.Array] = None,
+ ) -> ivy.Array:
+ """
+ ivy.Array instance method variant of ivy.amax. This method simply wraps the
+ function, and so the docstring for ivy.amax also applies to this method with
+ minimal changes.
+
+ Parameters
+ ----------
+ self
+ input array. Should have a real-valued data type.
+ axis
+ axis or axes along which maximum values must be computed. By default, the
+ maximum value must be computed over the entire array. If a tuple of
+ integers, maximum values must be computed over multiple axes.
+ Default: ``None``.
+ keepdims
+ optional boolean, if ``True``, the reduced axes (dimensions) must be
+ included in the result as singleton dimensions, and, accordingly, the
+ result must be compatible with the input array
+ (see `broadcasting`_).
+ Otherwise, if ``False``, the reduced axes (dimensions)
+ must not be included in the result.
+ Default: ``False``.
+ out
+ optional output array, for writing the result to.
+
+ Returns
+ -------
+ ret
+ if the maximum value was computed over the entire array, a zero-dimensional
+ array containing the maximum value; otherwise, a non-zero-dimensional array
+ containing the maximum values. The returned array must have the same
+ data type as ``x``.
+
+ Examples
+ --------
+ >>> x = ivy.array([3., 4., 5.])
+ >>> y = x.amax()
+ >>> print(y)
+ ivy.array(5.)
+
+ >>> x = ivy.array([[-1, 0, 1], [2, 3, 4]])
+ >>> y = x.amax(axis=1)
+ >>> print(y)
+ ivy.array([1, 4])
+
+ >>> x = ivy.array([0.1, 1.1, 2.1])
+ >>> y = ivy.array(0.)
+ >>> x.amax(out=y)
+ >>> print(y)
+ ivy.array(2.1)
+ """
+ return ivy.amax(self._data, axis=axis, keepdims=keepdims, out=out)
+
+ def amin(
+ self: ivy.Array,
+ /,
+ *,
+ axis: Optional[Union[int, Sequence[int]]] = None,
+ keepdims: bool = False,
+ out: Optional[ivy.Array] = None,
+ ) -> ivy.Array:
+ """
+ ivy.Array instance method variant of ivy.amin. This method simply wraps the
+ function, and so the docstring for ivy.amin also applies to this method with
+ minimal changes.
+
+ Parameters
+ ----------
+ self
+ input array. Should have a real-valued data type.
+ axis
+ axis or axes along which minimum values must be computed. By default, the
+ minimum value must be computed over the entire array. If a tuple of
+ integers, minimum values must be computed over multiple axes.
+ Default: ``None``.
+ keepdims
+ optional boolean, if ``True``, the reduced axes (dimensions) must be
+ included in the result as singleton dimensions, and, accordingly, the
+ result must be compatible with the input array
+ (see `broadcasting`_). Otherwise,
+ if ``False``, the reduced axes (dimensions)
+ must not be included in the result.
+ Default: ``False``.
+ out
+ optional output array, for writing the result to.
+
+ Returns
+ -------
+ ret
+ if the minimum value was computed over the entire array, a zero-dimensional
+ array containing the minimum value; otherwise, a non-zero-dimensional array
+ containing the minimum values. The returned array must have the same
+ data type as ``x``.
+
+ Examples
+ --------
+ >>> x = ivy.array([3., 4., 5.])
+ >>> y = x.amin()
+ >>> print(y)
+ ivy.array(3.)
+
+ >>> x = ivy.array([[-1, 0, 1], [2, 3, 4]])
+ >>> y = x.amin(axis=1)
+ >>> print(y)
+ ivy.array([-1, 2])
+
+ >>> x = ivy.array([0.1, 1.1, 2.1])
+ >>> y = ivy.array(0.)
+ >>> x.amin(out=y)
+ >>> print(y)
+ ivy.array(0.1)
+ """
+ return ivy.amin(self._data, axis=axis, keepdims=keepdims, out=out)
+
def lgamma(self: ivy.Array, *, out: Optional[ivy.Array] = None) -> ivy.Array:
"""
ivy.Array instance method variant of ivy.lgamma. This method simply wraps the
@@ -705,7 +829,7 @@ def gradient(
Note: jax supports edge_order=1 case only
axis
dimension(s) to approximate the gradient over
- by default partial gradient is computed in every dimention
+ by default partial gradient is computed in every dimension
Returns
diff --git a/ivy/data_classes/array/experimental/layers.py b/ivy/data_classes/array/experimental/layers.py
index 0466030de549f..eefb667184fb4 100644
--- a/ivy/data_classes/array/experimental/layers.py
+++ b/ivy/data_classes/array/experimental/layers.py
@@ -455,7 +455,7 @@ def dct(
type
The type of the dct. Must be 1, 2, 3 or 4.
n
- The lenght of the transform. If n is less than the input signal lenght,
+ The length of the transform. If n is less than the input signal length,
then x is truncated, if n is larger than x is zero-padded.
norm
The type of normalization to be applied. Must be either None or "ortho".
@@ -1086,6 +1086,62 @@ def ifftn(
"""
return ivy.ifftn(self._data, s=s, axes=axes, norm=norm, out=out)
+ def rfft(
+ self: ivy.Array,
+ /,
+ *,
+ n: Optional[int] = None,
+ axis: int = -1,
+ norm: Literal["backward", "ortho", "forward"] = "backward",
+ out: Optional[ivy.Array] = None,
+ ) -> ivy.Array:
+ """
+ ivy.Array instance method variant of ivy.rfft. This method simply wraps the
+ function, and so the docstring for ivy.rfft also applies to this method with
+ minimal changes.
+
+ Parameters
+ ----------
+ self
+ input array. Must have a real-valued floating-point data type.
+ n
+ length of the transformed axis of the input. If
+ - n is greater than the length of the input array, the input array
+ is zero-padded to length n.
+ - n is less than the length of the input array, the input array is
+ trimmed to length n.
+ - n is not provided, the length of the transformed axis of the
+ output must equal the length of the input along the axis specified
+ by axis. Default is ``None``.
+ axis
+ axis (dimension) over which to compute the Fourier transform.
+ If not set, the last axis (dimension) is used. Default is ``-1``.
+ norm
+ normalization mode. Should be one of the following modes:
+ - 'backward': no normalization.
+ - 'ortho': normalize by 1/sqrt(n) (i.e., make the FFT orthonormal).
+ - 'forward': normalize by 1/n.
+ Default is ``backward``.
+ out
+ Optional output array, for writing the result to. It must
+ have a shape that the inputs broadcast to.
+
+ Returns
+ -------
+ ret
+ an array transformed along the axis (dimension) indicated by axis.
+ The returned array must have a complex-valued floating-point
+ data type determined by Type Promotion Rules.
+
+ Examples
+ --------
+ >>> x = ivy.array([0,1,2])
+ >>> y = x.rfft()
+ >>> print(y)
+ ivy.array([ 3. +0.j , -1.5+0.8660254j])
+ """
+ return ivy.rfft(self, n=n, axis=axis, norm=norm, out=out)
+
def rfftn(
self: ivy.Array,
s: Sequence[int] = None,
diff --git a/ivy/data_classes/array/experimental/linear_algebra.py b/ivy/data_classes/array/experimental/linear_algebra.py
index a9b1a648b111b..0fe58b0dc3513 100644
--- a/ivy/data_classes/array/experimental/linear_algebra.py
+++ b/ivy/data_classes/array/experimental/linear_algebra.py
@@ -411,6 +411,37 @@ def make_svd_non_negative(
"""
return ivy.make_svd_non_negative(self._data, U, S, V, nntype=nntype)
+ def tensor_train(
+ self: Union[ivy.Array, ivy.NativeArray],
+ rank: Union[int, Sequence[int]],
+ /,
+ svd: Optional[Literal["truncated_svd"]] = "truncated_svd",
+ verbose: Optional[bool] = False,
+ ) -> ivy.TTTensor:
+ """
+ ivy.Array instance method variant of ivy.tensor_train. This method simply wraps
+ the function, and so the docstring for ivy.tensor_train also applies to this
+ method with minimal changes.
+
+ Parameters
+ ----------
+ self
+ input tensor
+ rank
+ maximum allowable TT rank of the factors
+ if int, then this is the same for all the factors
+ if int list, then rank[k] is the rank of the kth factor
+ svd
+ function to use to compute the SVD
+ verbose
+ level of verbosity
+
+ Returns
+ -------
+ ivy.TTTensor
+ """
+ return ivy.tensor_train(self._data, rank, svd=svd, verbose=verbose)
+
def truncated_svd(
self: Union[ivy.Array, ivy.NativeArray],
/,
diff --git a/ivy/data_classes/array/experimental/manipulation.py b/ivy/data_classes/array/experimental/manipulation.py
index e11d6df634fe0..017df89bd4b6b 100644
--- a/ivy/data_classes/array/experimental/manipulation.py
+++ b/ivy/data_classes/array/experimental/manipulation.py
@@ -294,7 +294,7 @@ def top_k(
self
The array to compute top_k for.
k
- Number of top elements to retun must not exceed the array size.
+ Number of top elements to return must not exceed the array size.
axis
The axis along which we must return the top elements default value is 1.
largest
@@ -1076,6 +1076,129 @@ def fill_diagonal(
"""
return ivy.fill_diagonal(self._data, v, wrap=wrap)
+ def take(
+ self: ivy.Array,
+ indices: Union[int, ivy.Array, ivy.NativeArray],
+ /,
+ *,
+ axis: Optional[int] = None,
+ mode: str = "fill",
+ fill_value: Optional[Number] = None,
+ out: Optional[ivy.Array] = None,
+ ) -> ivy.Array:
+ """
+ ivy.Array instance method variant of ivy.take.
+
+ This method simply wraps the function, and so the docstring for
+ ivy.take also applies to this method with minimal changes.
+
+ Parameters
+ ----------
+ self
+ input array
+ indices
+ array indices. Must have an integer data type.
+ axis
+ axis over which to select values. If `axis` is negative,
+ the function must determine the axis along which to select values
+ by counting from the last dimension.
+ By default, the flattened input array is used.
+ mode
+ specifies how out-of-bounds `indices` will behave.
+ - βraiseβ β raise an error
+ - βwrapβ β wrap around
+ - βclipβ β clip to the range (all indices that are too large are
+ replaced by the index that addresses the last element along that axis.
+ Note that this disables indexing with negative numbers.)
+ - 'fill' (default) = returns invalid values (e.g. NaN)
+ for out-of bounds indices (see also fill_value below)
+ fill_value
+ fill value to return for out-of-bounds slices
+ (Defaults to NaN for inexact types,
+ the largest negative value for signed types,
+ the largest positive value for unsigned types, and True for booleans.)
+ out
+ optional output array, for writing the result to. It must
+ have a shape that the inputs broadcast to.
+
+ Returns
+ -------
+ ret
+ an array having the same data type as `x`.
+ The output array must have the same rank
+ (i.e., number of dimensions) as `x` and
+ must have the same shape as `x`, except
+ for the axis specified by `axis`
+ whose size must equal the number of elements in `indices`.
+
+ Examples
+ --------
+ With `ivy.Array` input:
+
+ >>> x = ivy.array([4,5,6])
+ >>> indices = ivy.array([2,1,0])
+ >>> y = x.take(indices)
+ >>> print(y)
+ ivy.array([6, 5, 4])
+
+ >>> x = ivy.array([4.7,5.2,6.5])
+ >>> indices = ivy.array([[0,1]])
+ >>> y = ivy.zeros_like(indices, dtype=x.dtype)
+ >>> x.take(indices, out=y)
+ >>> print(y)
+ ivy.array([[4.7, 5.2]])
+
+ >>> x = ivy.array([False, False, True])
+ >>> indices = ivy.array([[4,3,2]])
+ >>> y = ivy.zeros_like(indices, dtype=x.dtype)
+ >>> x.take(indices, out=y, mode="wrap")
+ >>> print(y)
+ ivy.array([[False, False, True]])
+ """
+ return ivy.take(
+ self, indices, axis=axis, mode=mode, fill_value=fill_value, out=out
+ )
+
+ def trim_zeros(
+ self: ivy.Array,
+ /,
+ *,
+ trim: Optional[str] = "fb",
+ ) -> ivy.Array:
+ """
+ ivy.Array instance method variant of ivy.trim_zeros.
+
+ This method simply wraps the function, and so the docstring for
+ ivy.trim_zeros also applies to this method with minimal changes.
+
+ Parameters
+ ----------
+ self : 1-D array
+ Input array.
+ trim : str, optional
+ A string with 'f' representing trim from front and 'b' to trim from
+ back. Default is 'fb', trim zeros from both front and back of the
+ array.
+
+ Returns
+ -------
+ 1-D array
+ The result of trimming the input. The input data type is preserved.
+
+ Examples
+ --------
+ >>> a = ivy.array([0, 0, 0, 0, 8, 3, 0, 0, 7, 1, 0])
+ >>> ivy.trim_zeros(a)
+ array([8, 3, 0, 0, 7, 1])
+
+ >>> ivy.trim_zeros(a, 'b')
+ array([0, 0, 0, 0, 8, 3, 0, 0, 7, 1])
+
+ >>> ivy.trim_zeros([0, 8, 3, 0, 0])
+ [8, 3]
+ """
+ return ivy.trim_zeros(self, trim=trim)
+
def unfold(
self: Union[ivy.Array, ivy.NativeArray],
/,
@@ -1352,7 +1475,7 @@ def column_stack(
Parameters
----------
self
- Array that will be stacked at the begining of the provided array iterable.
+ Array that will be stacked at the beginning of the provided array iterable.
arrays
Arrays to be stacked.
out
diff --git a/ivy/data_classes/array/experimental/random.py b/ivy/data_classes/array/experimental/random.py
index 69966e65f4760..cd067cd304ba2 100644
--- a/ivy/data_classes/array/experimental/random.py
+++ b/ivy/data_classes/array/experimental/random.py
@@ -177,7 +177,7 @@ def poisson(
Parameters
----------
self
- Input Array of rate paramter(s). It must have a shape that is broadcastable
+ Input Array of rate parameter(s). It must have a shape that is broadcastable
to the requested shape
shape
If the given shape is, e.g '(m, n, k)', then 'm * n * k' samples are drawn.
diff --git a/ivy/data_classes/array/experimental/statistical.py b/ivy/data_classes/array/experimental/statistical.py
index 0a2ab13449157..d206ff796fae0 100644
--- a/ivy/data_classes/array/experimental/statistical.py
+++ b/ivy/data_classes/array/experimental/statistical.py
@@ -180,6 +180,61 @@ def nanmean(
self._data, axis=axis, keepdims=keepdims, dtype=dtype, out=out
)
+ def nanmin(
+ self: ivy.Array,
+ /,
+ *,
+ axis: Optional[Union[Tuple[int], int]] = None,
+ keepdims: Optional[bool] = False,
+ initial: Optional[Union[int, float, complex]] = None,
+ where: Optional[ivy.Array] = None,
+ out: Optional[ivy.Array] = None,
+ ) -> ivy.Array:
+ """
+ ivy.Array instance method variant of ivy.nanmin. This method simply wraps the
+ function, and so the docstring for ivy.min also applies to this method with
+ minimal changes.
+
+ Parameters
+ ----------
+ self
+ Input array.
+ axis
+ Axis or axes along which the minimum is computed.
+ The default is to compute the minimum of the flattened array.
+ out
+ optional output array, for writing the result to.
+ keepdims
+ If this is set to True, the axes which are reduced are left in the result
+ as dimensions with size one. With this option, the result will broadcast
+ correctly against the original a.
+ initial
+ The maximum value of an output element
+ where
+ Elements to compare for the minimum
+
+ Returns
+ -------
+ ret
+ Return minimum of an array or minimum along an axis, ignoring any NaNs.
+
+ Examples
+ --------
+ >>> a = ivy.array([[1, 2], [3, ivy.nan]])
+ >>> a.nanmin(a)
+ 1.0
+ >>> a.nanmin(a, axis=0)
+ ivy.array([1., 2.])
+ """
+ return ivy.nanmin(
+ self._data,
+ axis=axis,
+ keepdims=keepdims,
+ out=out,
+ initial=initial,
+ where=where,
+ )
+
def nanprod(
self: ivy.Array,
/,
diff --git a/ivy/data_classes/array/general.py b/ivy/data_classes/array/general.py
index 6320790c8b13d..b17c29e0168d5 100644
--- a/ivy/data_classes/array/general.py
+++ b/ivy/data_classes/array/general.py
@@ -905,7 +905,7 @@ def fourier_encode(
Default is ``False``.
concat
Whether to concatenate the position, sin and cos values, or return
- seperately. Default is ``True``.
+ separately. Default is ``True``.
flatten
Whether to flatten the position dimension into the batch dimension.
Default is ``False``.
diff --git a/ivy/data_classes/array/layers.py b/ivy/data_classes/array/layers.py
index e9169b2ee0cbd..fd1a9a22dc968 100644
--- a/ivy/data_classes/array/layers.py
+++ b/ivy/data_classes/array/layers.py
@@ -80,7 +80,7 @@ def dropout(
) -> ivy.Array:
"""
ivy.Array instance method variant of ivy.dropout. This method simply wraps the
- function, and so the docstring for ivy.droput also applies to this method with
+ function, and so the docstring for ivy.dropout also applies to this method with
minimal changes.
Parameters
@@ -327,7 +327,7 @@ def scaled_dot_product_attention(
Default is None. The shape of mask input should be in
*[batch_shape,num_queries,num_keys]*.
dropout_p
- Specifies the dropout probablity, if greater than 0.0, dropout is applied
+ Specifies the dropout probability, if greater than 0.0, dropout is applied
is_causal
If true, assumes causal attention masking and errors if both `mask` and
`is_causal` are set.
diff --git a/ivy/data_classes/array/linear_algebra.py b/ivy/data_classes/array/linear_algebra.py
index 300d22b5e2804..a25df9d4cabb7 100644
--- a/ivy/data_classes/array/linear_algebra.py
+++ b/ivy/data_classes/array/linear_algebra.py
@@ -1,4 +1,5 @@
# global
+
import abc
from typing import Union, Optional, Literal, Tuple, List, Sequence
@@ -759,6 +760,19 @@ def qr(
is 'complete', the array must have shape (..., M, N). If mode is 'reduced',
the array must have shape (..., K, N), where K = min(M, N). The first
x.ndim-2 dimensions must have the same size as those of the input x.
+
+ Examples
+ --------
+ >>> x = ivy.array([[1.,2.,3.],[4.,5.,6.],[7.,8.,9.]])
+ >>> q, r = x.qr(mode='reduced')
+ >>> print(q)
+ ivy.array([[-0.12309149, 0.90453403, 0.40824829],
+ [-0.49236596, 0.30151134, -0.81649658],
+ [-0.86164044, -0.30151134, 0.40824829]])
+ >>> print(r)
+ ivy.array([[-8.12403841e+00,-9.60113630e+00, -1.10782342e+01],
+ [ 0.00000000e+00, 9.04534034e-01, 1.80906807e+00],
+ [ 0.00000000e+00, 0.00000000e+00, -8.88178420e-16]])
"""
return ivy.qr(self._data, mode=mode, out=out)
@@ -922,6 +936,14 @@ def trace(
offset
Offset of the diagonal from the main diagonal. Can be both positive and
negative. Defaults to 0.
+ axis1
+ axis to be used as the first axis of the 2-D sub-arrays from which the
+ diagonals should be taken.
+ Defaults to ``0.`` .
+ axis2
+ axis to be used as the second axis of the 2-D sub-arrays from which the
+ diagonals should be taken.
+ Defaults to ``1.`` .
out
optional output array, for writing the result to. It must have a shape that
the inputs broadcast to.
diff --git a/ivy/data_classes/array/losses.py b/ivy/data_classes/array/losses.py
index 71011a82f2f96..214c05ac5a189 100644
--- a/ivy/data_classes/array/losses.py
+++ b/ivy/data_classes/array/losses.py
@@ -14,7 +14,7 @@ def cross_entropy(
*,
axis: int = -1,
epsilon: float = 1e-7,
- reduction: str = "sum",
+ reduction: str = "mean",
out: Optional[ivy.Array] = None,
) -> ivy.Array:
"""
@@ -64,7 +64,7 @@ def binary_cross_entropy(
*,
from_logits: bool = False,
epsilon: float = 0.0,
- reduction: str = "none",
+ reduction: str = "mean",
pos_weight: Optional[Union[ivy.Array, ivy.NativeArray]] = None,
axis: Optional[int] = None,
out: Optional[ivy.Array] = None,
@@ -131,7 +131,7 @@ def sparse_cross_entropy(
*,
axis: int = -1,
epsilon: float = 1e-7,
- reduction: str = "sum",
+ reduction: str = "mean",
out: Optional[ivy.Array] = None,
) -> ivy.Array:
"""
diff --git a/ivy/data_classes/array/searching.py b/ivy/data_classes/array/searching.py
index eade8e99125c5..15537fa4b2005 100644
--- a/ivy/data_classes/array/searching.py
+++ b/ivy/data_classes/array/searching.py
@@ -29,7 +29,7 @@ def argmax(
input array. Should have a numeric data type.
axis
axis along which to search. If None, the function must return the index of
- the maximum value of the flattened array. Deafult: ``None``.
+ the maximum value of the flattened array. Default: ``None``.
keepdims
If this is set to True, the axes which are reduced are left in the result as
dimensions with size one. With this option, the result will broadcast
diff --git a/ivy/data_classes/container/base.py b/ivy/data_classes/container/base.py
index a401c5c2cd16e..26f4623eceaca 100644
--- a/ivy/data_classes/container/base.py
+++ b/ivy/data_classes/container/base.py
@@ -134,7 +134,7 @@ def __init__(
"list_join": self.cont_list_join,
"concat": lambda conts: self.concat(conts, 0),
}[self._container_combine_method]
- self._loaded_containers_from_queues = dict()
+ self._loaded_containers_from_queues = {}
self._queue_load_sizes_cum = np.cumsum(queue_load_sizes)
self._queue_timeout = ivy.default(queue_timeout, ivy.queue_timeout)
if dynamic_backend is not None:
@@ -145,26 +145,26 @@ def __init__(
if kwargs:
dict_in = dict(**kwargs)
else:
- dict_in = dict()
+ dict_in = {}
elif kwargs:
raise ivy.utils.exceptions.IvyException(
"dict_in and **kwargs cannot both be specified for ivy.Container "
"constructor, please specify one or the other, not both."
)
- self._config_in = dict(
- print_limit=print_limit,
- print_indent=print_indent,
- key_length_limit=key_length_limit,
- print_line_spacing=print_line_spacing,
- ivyh=ivyh,
- default_key_color=default_key_color,
- keyword_color_dict=keyword_color_dict,
- rebuild_child_containers=rebuild_child_containers,
- build_callable=build_callable,
- types_to_iteratively_nest=types_to_iteratively_nest,
- alphabetical_keys=alphabetical_keys,
- )
- self._config = dict()
+ self._config_in = {
+ "print_limit": print_limit,
+ "print_indent": print_indent,
+ "key_length_limit": key_length_limit,
+ "print_line_spacing": print_line_spacing,
+ "ivyh": ivyh,
+ "default_key_color": default_key_color,
+ "keyword_color_dict": keyword_color_dict,
+ "rebuild_child_containers": rebuild_child_containers,
+ "build_callable": build_callable,
+ "types_to_iteratively_nest": types_to_iteratively_nest,
+ "alphabetical_keys": alphabetical_keys,
+ }
+ self._config = {}
self.cont_inplace_update(dict_in, **self._config_in)
# Class Methods #
@@ -316,9 +316,9 @@ def cont_list_join(containers, config=None):
)
if isinstance(container0, ivy.Container):
- return_dict = dict()
+ return_dict = {}
for key in container0.keys():
- new_list = list()
+ new_list = []
for container in containers:
new_list.append(container[key])
return_dict[key] = ivy.Container.cont_list_join(new_list, config)
@@ -351,7 +351,7 @@ def cont_list_stack(containers, dim, config=None):
)
if isinstance(container0, ivy.Container):
- return_dict = dict()
+ return_dict = {}
for key in container0.keys():
return_dict[key] = ivy.Container.cont_list_stack(
[container[key] for container in containers], dim, config
@@ -369,7 +369,7 @@ def _cont_concat_unify(containers, device, axis=0):
@staticmethod
def _cont_sum_unify(containers, device, _=None, _1=None):
return sum(
- [cont.to_device(device) for cont in containers.values()],
+ (cont.to_device(device) for cont in containers.values()),
start=ivy.zeros([]),
)
@@ -442,7 +442,7 @@ def cont_combine(*containers, config=None):
# otherwise, check that the keys are aligned between each container, and apply
# this method recursively
- return_dict = dict()
+ return_dict = {}
all_keys = {
item
for sublist in [list(cont.keys()) for cont in containers]
@@ -529,9 +529,9 @@ def cont_diff(
return ivy.Container(**config)
else:
cont_range = range(num_containers)
- diff_dict = dict()
+ diff_dict = {}
cont_dict = dict(zip(cont_range, containers))
- idxs_added = list()
+ idxs_added = []
for idx in cont_range:
if idx not in idxs_added:
idxs_to_add = ivy.argwhere(equal_mat[idx])
@@ -539,15 +539,13 @@ def cont_diff(
ivy.to_numpy(idxs_to_add).reshape(-1).tolist()
)
if isinstance(diff_keys, str):
- key = diff_keys + "_" + str(idxs_to_add_list)[1:-1]
+ key = f"{diff_keys}_{str(idxs_to_add_list)[1:-1]}"
elif isinstance(diff_keys, (list, tuple)):
key = diff_keys[idx]
else:
raise ivy.utils.exceptions.IvyException(
"diff_keys must be either a string or list of strings,"
- "but found {} of type {}".format(
- diff_keys, type(diff_keys)
- )
+ f" but found {diff_keys} of type {type(diff_keys)}"
)
diff_dict[key] = cont_dict[idx]
idxs_added += idxs_to_add_list
@@ -555,7 +553,7 @@ def cont_diff(
# otherwise, check that the keys are aligned between each container, and apply
# this method recursively
- return_dict = dict()
+ return_dict = {}
all_keys = {
item
for sublist in [list(cont.keys()) for cont in containers]
@@ -589,7 +587,7 @@ def cont_diff(
if mode == "all":
return_dict[key] = containers[keys_present.index(True)][key]
continue
- diff_dict = dict()
+ diff_dict = {}
for i, (key_present, cont) in enumerate(zip(keys_present, containers)):
if detect_key_diffs:
if key_present and mode != "same_only":
@@ -600,9 +598,7 @@ def cont_diff(
else:
raise ivy.utils.exceptions.IvyException(
"diff_keys must be either a string or list of strings,"
- "but found {} of type {}".format(
- diff_keys, type(diff_keys)
- )
+ f" but found {diff_keys} of type {type(diff_keys)}"
)
if diff_dict:
return_dict[key] = diff_dict
@@ -702,7 +698,7 @@ def cont_multi_map(
Container
"""
# retrieve all keys and the first container if it exists
- keys = set()
+ keys = set([])
container0 = None
for container in containers:
if isinstance(container, ivy.Container):
@@ -718,7 +714,7 @@ def cont_multi_map(
config = (
container0.cont_config if isinstance(container0, ivy.Container) else {}
)
- return_dict = dict()
+ return_dict = {}
for key in keys:
values = []
@@ -851,7 +847,8 @@ def cont_identical(
Chain of keys for this dict entry (Default value = '')
assert_and_assign
if true, then the container being compared with is updated with the value
- in the container being compared to given that the strucutres are congruent
+ in the container being compared to given that the structures are congruent
+
Returns
-------
Boolean
@@ -867,7 +864,7 @@ def cont_identical(
# noinspection PyProtectedMember
for key in keys:
- if not min([key in cont for cont in containers]):
+ if not min(key in cont for cont in containers):
return False
for cont in containers:
if cont.cont_config["build_callable"]:
@@ -876,19 +873,19 @@ def cont_identical(
value_0 = values[0]
type_0 = type(value_0)
types = [type(val) for val in values]
- if not min([type_n is type_0 for type_n in types]):
+ if not min(type_n is type_0 for type_n in types):
if isinstance(value_0, ivy.Container) or check_types:
return False
if ivy.is_array(value_0):
if check_shapes:
shape_0 = value_0.shape
shapes = [val.shape for val in values]
- if not min([shape_n == shape_0 for shape_n in shapes]):
+ if not min(shape_n == shape_0 for shape_n in shapes):
return False
if same_arrays:
id_0 = id(value_0)
ids = [id(val) for val in values]
- if not min([id_n == id_0 for id_n in ids]):
+ if not min(id_n == id_0 for id_n in ids):
return False
elif arrays_equal:
if not ivy.all_equal(*values):
@@ -897,7 +894,7 @@ def cont_identical(
containers[0].cont_set_at_key_chain(
key, containers[1][key], inplace=True
)
- this_key_chain = key if key_chain == "" else (key_chain + "/" + key)
+ this_key_chain = key if key_chain == "" else f"{key_chain}/{key}"
if isinstance(value_0, ivy.Container):
ret = ivy.Container.cont_identical(
values,
@@ -1012,7 +1009,8 @@ def cont_identical_structure(
Chain of keys for this dict entry (Default value = '')
assert_and_assign
if true, then the container being compared with is updated with the value in
- the container being compared to given that the strucutres are congruent
+ the container being compared to given that the structures are congruent
+
Returns
-------
Boolean
@@ -1064,7 +1062,7 @@ def cont_assert_identical_structure(
Default is ``False``.
assert_and_assign
if true, then the container being compared with is updated with the value in
- the container being compared to given that the strucutres are congruent
+ the container being compared to given that the structures are congruent
"""
ivy.utils.assertions.check_true(
ivy.Container.cont_identical_structure(
@@ -1076,9 +1074,8 @@ def cont_assert_identical_structure(
partial,
assert_and_assign=assert_and_assign,
),
- "Containers did not have identical structure:\n\n{}".format(
- ivy.Container.cont_structural_diff(*containers)
- ),
+ "Containers did not have identical"
+ f" structure:\n\n{ivy.Container.cont_structural_diff(*containers)}",
)
@staticmethod
@@ -1095,10 +1092,9 @@ def cont_identical_configs(containers):
ivy.utils.assertions.check_greater(len(containers), 1, as_array=False)
configs = [cont.cont_config for cont in containers]
config0 = configs[0]
- for k, v in config0.items():
- if not min([config[k] == v for config in configs]):
- return False
- return True
+ return all(
+ min(config[k] == v for config in configs) for k, v in config0.items()
+ )
@staticmethod
def cont_identical_array_shapes(containers, exclusive=False):
@@ -1125,10 +1121,8 @@ def cont_identical_array_shapes(containers, exclusive=False):
if len(array_cont) != array_cont0_len:
return False
elif not min(
- [
- a.shape == a0.shape
- for a, a0 in zip(array_cont.values(), array_cont0.values())
- ]
+ a.shape == a0.shape
+ for a, a0 in zip(array_cont.values(), array_cont0.values())
):
return False
return True
@@ -1176,7 +1170,7 @@ def cont_from_disk_as_hdf5(
"files from disk into a container."
),
)
- container_dict = dict()
+ container_dict = {}
if type(h5_obj_or_filepath) is str:
h5_obj = h5py.File(h5_obj_or_filepath, "r")
else:
@@ -1349,7 +1343,7 @@ def cont_reduce(containers, reduction, config=None):
)
if isinstance(container0, ivy.Container):
- return_dict = dict()
+ return_dict = {}
for key in container0.keys():
return_dict[key] = ivy.Container.cont_reduce(
[container[key] for container in containers], reduction
@@ -1384,10 +1378,10 @@ def cont_flatten_key_chain(
(Default value = '__')
"""
# noinspection RegExpSingleCharAlternation
- flat_keys = re.split(r"/|\.", key_chain) # noqa
+ flat_keys = re.split("/|\.", key_chain) # noqa
num_keys = len(flat_keys)
- pre_keys = list()
- post_keys = list()
+ pre_keys = []
+ post_keys = []
if above_height and num_keys > above_height:
post_keys = flat_keys[-above_height:]
del flat_keys[-above_height:]
@@ -1517,7 +1511,7 @@ def _cont_get_shape(self):
]
if not sub_shapes:
return sub_shapes
- min_num_dims = min([len(sub_shape) for sub_shape in sub_shapes])
+ min_num_dims = min(len(sub_shape) for sub_shape in sub_shapes)
sub_shapes_array = np.asarray(
[sub_shape[0:min_num_dims] for sub_shape in sub_shapes]
)
@@ -1558,7 +1552,7 @@ def _cont_get_dev(self, as_native=False):
return None
def _cont_at_key_chains_input_as_seq(self, key_chains, ignore_key_errors=False):
- return_cont = ivy.Container(dict(), **self._config)
+ return_cont = ivy.Container({}, **self._config)
for kc in key_chains:
val = self.cont_at_key_chain(kc, ignore_key_errors=ignore_key_errors)
if ignore_key_errors and not ivy.exists(val):
@@ -1569,7 +1563,7 @@ def _cont_at_key_chains_input_as_seq(self, key_chains, ignore_key_errors=False):
def _cont_at_key_chains_input_as_dict(
self, key_chains, current_chain="", ignore_key_errors=False
):
- return_dict = dict()
+ return_dict = {}
for k, v in key_chains.items():
if current_chain == "":
new_current_chain = k
@@ -1631,9 +1625,9 @@ def cont_duplicate_array_keychains(self):
return duplciates
def cont_update_config(self, **config):
- new_config = dict()
+ new_config = {}
for k, v in config.items():
- att_name = "_" + k
+ att_name = f"_{k}"
if k in self._config_in:
if k == "types_to_iteratively_nest":
v = ivy.default(lambda: tuple(v), (), catch_exceptions=True)
@@ -1690,11 +1684,10 @@ def cont_inplace_update(
)
) or isinstance(value, tuple(self._types_to_iteratively_nest)):
self[key] = ivy.Container(value, **self._config)
+ elif key in self and isinstance(self[key], ivy.Container):
+ self[key].cont_inplace_update(value)
else:
- if key in self and isinstance(self[key], ivy.Container):
- self[key].cont_inplace_update(value)
- else:
- self[key] = value
+ self[key] = value
def cont_all_true(
self,
@@ -1803,7 +1796,7 @@ def cont_slice_via_key(self, slice_key):
-------
Container object sliced at desired key.
"""
- return_dict = dict()
+ return_dict = {}
for key, value in self.items():
if key == slice_key:
return value
@@ -2061,9 +2054,9 @@ def cont_to_disk_as_hdf5(
value_shape = value_as_np.shape
this_batch_size = value_shape[0]
max_bs = (
- starting_index + this_batch_size
- if not max_batch_size
- else max_batch_size
+ max_batch_size
+ if max_batch_size
+ else starting_index + this_batch_size
)
if key not in h5_obj.keys():
dataset_shape = [max_bs] + list(value_shape[1:])
@@ -2121,7 +2114,7 @@ def cont_to_disk_as_json(self, json_filepath):
json.dump(self.cont_to_jsonable().cont_to_dict(), json_data_file, indent=4)
def cont_to_nested_list(self):
- return_list = list()
+ return_list = []
for key, value in self.items():
if isinstance(value, ivy.Container):
return_list.append(value.cont_to_nested_list())
@@ -2138,17 +2131,15 @@ def cont_to_raw(self):
ret
Container data in its raw form.
"""
- return_item = dict()
- for i, (key, value) in enumerate(self.items()):
+ return_item = {}
+ for key, value in self.items():
if isinstance(value, ivy.Container):
return_item[key] = value.cont_to_raw()
elif key[0:3] == "it_" and tuple(self._types_to_iteratively_nest):
- return_item = list(
- [
- v.cont_to_raw() if isinstance(v, ivy.Container) else v
- for v in self.values()
- ]
- )
+ return_item = [
+ v.cont_to_raw() if isinstance(v, ivy.Container) else v
+ for v in self.values()
+ ]
break
else:
return_item[key] = value
@@ -2162,7 +2153,7 @@ def cont_to_dict(self):
-------
ret Container as nested dict.
"""
- return_dict = dict()
+ return_dict = {}
for key, value in self.items():
if isinstance(value, ivy.Container):
return_dict[key] = value.cont_to_dict()
@@ -2191,7 +2182,7 @@ def cont_to_iterator(self, key_chain="", leaf_keys_only=False, include_empty=Fal
if leaf_keys_only:
kc = key
else:
- kc = key_chain + "/" + key if key_chain != "" else key
+ kc = f"{key_chain}/{key}" if key_chain != "" else key
if isinstance(value, ivy.Container) and (not include_empty or value):
yield from value.cont_to_iterator(kc, leaf_keys_only, include_empty)
else:
@@ -2240,7 +2231,7 @@ def cont_to_iterator_keys(
if leaf_keys_only:
kc = key
else:
- kc = key_chain + "/" + key if key_chain != "" else key
+ kc = f"{key_chain}/{key}" if key_chain != "" else key
if isinstance(value, ivy.Container) and (not include_empty or value):
# noinspection PyCompatibility
yield from value.cont_to_iterator_keys(
@@ -2258,7 +2249,7 @@ def cont_to_flat_list(self):
ret
Container as flat list.
"""
- return list([item for key, item in self.cont_to_iterator()])
+ return [item for key, item in self.cont_to_iterator()]
def cont_from_flat_list(self, flat_list):
"""
@@ -2274,7 +2265,7 @@ def cont_from_flat_list(self, flat_list):
-------
Container.
"""
- new_dict = dict()
+ new_dict = {}
for key, value in self.items():
if isinstance(value, ivy.Container):
new_value = value.cont_from_flat_list(flat_list)
@@ -2343,7 +2334,7 @@ def cont_has_key_chain(self, key_chain):
def cont_find_sub_container(self, sub_cont_to_find, partial=False):
"""
- Find the sub-container in the current container if it exsits.
+ Find the sub-container in the current container if it exists.
Parameters
----------
@@ -2390,11 +2381,7 @@ def cont_contains_sub_container(self, sub_cont, partial=False):
-------
Bool
"""
- return (
- True
- if isinstance(self.cont_find_sub_container(sub_cont, partial), str)
- else False
- )
+ return isinstance(self.cont_find_sub_container(sub_cont, partial), str)
def cont_assert_contains_sub_container(self, sub_cont, partial=False):
"""
@@ -2421,16 +2408,15 @@ def cont_assert_contains_sub_container(self, sub_cont, partial=False):
key_chain = ""
# noinspection PyTypeChecker
raise ivy.utils.exceptions.IvyException(
- "Containers did not have identical structure and values:\n\n{}".format(
- ivy.Container.cont_diff(self[key_chain], sub_cont)
- )
+ "Containers did not have identical structure and"
+ f" values:\n\n{ivy.Container.cont_diff(self[key_chain], sub_cont)}"
)
def cont_find_sub_structure(
self, sub_struc_to_find, check_shapes=True, partial=False
):
"""
- Find the sub-container structure in the current container if it exsits.
+ Find the sub-container structure in the current container if it exists.
Parameters
----------
@@ -2487,12 +2473,8 @@ def cont_contains_sub_structure(self, sub_cont, check_shapes=True, partial=False
Whether to also check for partially complete sub-containers.
Default is ``False``.
"""
- return (
- True
- if isinstance(
- self.cont_find_sub_structure(sub_cont, check_shapes, partial), str
- )
- else False
+ return isinstance(
+ self.cont_find_sub_structure(sub_cont, check_shapes, partial), str
)
def cont_assert_contains_sub_structure(
@@ -2560,7 +2542,7 @@ def cont_at_keys(
"""
if queries is None and ignore_none:
return self
- key_chains_to_keep = list()
+ key_chains_to_keep = []
if isinstance(queries, str):
queries = [queries]
@@ -2568,8 +2550,10 @@ def map_fn(x, kc):
nonlocal key_chains_to_keep
kc_split = re.split("[/.]", kc)
for query_key in queries:
- if query_key in kc_split or (
- containing and min([query_key in k for k in kc_split])
+ if (
+ query_key in kc_split
+ or containing
+ and min(query_key in k for k in kc_split)
):
key_chains_to_keep.append(kc)
return x
@@ -2641,7 +2625,7 @@ def cont_at_key_chains(self, key_chains, ignore_none=True, ignore_key_errors=Fal
else:
raise ivy.utils.exceptions.IvyException(
"Invalid type for input key_chains, must either be a list, tuple, dict"
- " or ivy.Container, but found type {}".format(type(key_chains))
+ f" or ivy.Container, but found type {type(key_chains)}"
)
def cont_all_key_chains(self, include_empty=False):
@@ -2686,7 +2670,7 @@ def cont_set_at_keys(self, target_dict):
type
new container with updated value at each key
"""
- return_dict = dict()
+ return_dict = {}
for key, val in self.items():
if key in target_dict:
return_dict[key] = target_dict[key]
@@ -2863,7 +2847,7 @@ def cont_prune_keys(self, query_keys, ignore_none=True):
"""
if query_keys is None and ignore_none:
return self
- key_chains_to_prune = list()
+ key_chains_to_prune = []
if isinstance(query_keys, str):
query_keys = [query_keys]
@@ -2900,7 +2884,7 @@ def cont_prune_key_chain(self, key_chain):
Container with keys in key chain pruned.
"""
keys_in_chain = re.split("[/.]", key_chain)
- out_dict = dict()
+ out_dict = {}
for key, value in self.items():
if isinstance(value, ivy.Container):
if key == keys_in_chain[0]:
@@ -2947,8 +2931,8 @@ def cont_prune_key_chains(self, key_chains, ignore_none=True):
return self._cont_prune_key_chains_input_as_seq([key_chains])
else:
raise ivy.utils.exceptions.IvyException(
- "Invalid type for input key_chains, must either be a list, tuple, dict "
- "or ivy.Container, but found type {}".format(type(key_chains))
+ "Invalid type for input key_chains, must either be a list, tuple, dict"
+ f" or ivy.Container, but found type {type(key_chains)}"
)
def cont_format_key_chains(self, format_fn):
@@ -2968,7 +2952,7 @@ def cont_format_key_chains(self, format_fn):
return ivy.Container({format_fn(k): v for k, v in self.cont_to_iterator()})
def cont_sort_by_key(self):
- new_dict = dict()
+ new_dict = {}
for k, v in self.items():
if isinstance(v, ivy.Container):
v_back = v.cont_sort_by_key()
@@ -2994,7 +2978,7 @@ def cont_prune_empty(self, keep_nones=False, base=True):
ret
Container with empty keys pruned.
"""
- out_dict = dict()
+ out_dict = {}
for key, value in self.items():
if isinstance(value, ivy.Container):
new_value = value.cont_prune_empty(keep_nones, False)
@@ -3079,8 +3063,10 @@ def cont_prune_keys_from_key_chains(self, absolute=None, containing=None):
)
out_cont = ivy.Container(**self._config)
for key, value in self.items():
- if (absolute and key in absolute) or (
- containing and max([con in key for con in containing])
+ if (
+ (absolute and key in absolute)
+ or containing
+ and max(con in key for con in containing)
):
if isinstance(value, ivy.Container):
out_cont = ivy.Container.cont_combine(out_cont, value)
@@ -3245,11 +3231,9 @@ def cont_map(
-------
New container following the function mapped to each sub-array.
"""
- return_dict = self if inplace else dict()
+ return_dict = self if inplace else {}
for key, value in self.items():
- this_key_chain = (
- key if key_chain == "" else (str(key_chain) + "/" + str(key))
- )
+ this_key_chain = key if key_chain == "" else f"{str(key_chain)}/{str(key)}"
if isinstance(value, ivy.Container):
ret = value.cont_map(
func,
@@ -3316,16 +3300,16 @@ def cont_map_sub_conts(
key_chain
Chain of keys for this dict entry (Default value = '')
include_self
- Whether to also apply the (possiby in-place) function to this container.
+ Whether to also apply the (possibly in-place) function to this container.
Default is ``True``.
Returns
-------
New container following the function mapped to each sub-container.
"""
- return_dict = self if inplace else dict()
+ return_dict = self if inplace else {}
for key, value in self.items():
- this_key_chain = key if key_chain == "" else (key_chain + "/" + key)
+ this_key_chain = key if key_chain == "" else f"{key_chain}/{key}"
if isinstance(value, ivy.Container):
ret = value.cont_map_sub_conts(
func, key_chains, to_apply, prune_unapplied, inplace, this_key_chain
@@ -3334,16 +3318,12 @@ def cont_map_sub_conts(
continue
if not inplace:
return_dict[key] = ret
- else:
- if (
- key_chains is not None
- and (
- (this_key_chain in key_chains and not to_apply)
- or (this_key_chain not in key_chains and to_apply)
- )
- and prune_unapplied
- ):
- continue
+ elif (
+ key_chains is None
+ or (this_key_chain not in key_chains or to_apply)
+ and (this_key_chain in key_chains or not to_apply)
+ or not prune_unapplied
+ ):
return_dict[key] = value
ret = return_dict if inplace else ivy.Container(return_dict, **self._config)
if key_chain != "" or include_self:
@@ -3380,7 +3360,7 @@ def cont_reshape_like(self, target_dict, leading_shape=None, return_cont=None):
ret
new container with values of updated shapes
"""
- leading_shape = self._cont_ivy.default(leading_shape, list())
+ leading_shape = self._cont_ivy.default(leading_shape, [])
if return_cont is None:
return_cont = self.cont_copy()
for (_, v_shape), (k, v) in zip(target_dict.items(), return_cont.items()):
@@ -3495,8 +3475,8 @@ def _cont_slice_keys(self, key_slice):
ivy.utils.assertions.check_true(self._alphabetical_keys)
start_char = key_slice[0]
end_char = key_slice[2]
- start_idx = min([i for i, k in enumerate(keys) if k[0] == start_char])
- end_idx = max([i for i, k in enumerate(keys) if k[0] == end_char]) + 1
+ start_idx = min(i for i, k in enumerate(keys) if k[0] == start_char)
+ end_idx = max(i for i, k in enumerate(keys) if k[0] == end_char) + 1
key_slice = slice(start_idx, end_idx, 1)
ret = self.cont_copy()
desired_keys = keys[key_slice]
@@ -3823,7 +3803,7 @@ def _pre_pad_alpha_line(str_in):
s[0].isnumeric()
or s[0] == "-"
or s[0:3] == "..."
- or max([ss in s[0:6] for ss in ["nan, ", "inf, "]])
+ or max(ss in s[0:6] for ss in ["nan, ", "inf, "])
)
else (
indent_str + indented_key_str + s
@@ -3854,7 +3834,7 @@ def _align_arrays(str_in):
]
return ("\n" + indent_str).join(chunks)
- new_dict = dict()
+ new_dict = {}
for k, v in self.items():
if isinstance(v, ivy.Container):
# noinspection PyArgumentList
@@ -4065,7 +4045,7 @@ def _get_queue_item(self, query):
queue_idxs = {
np.sum(q >= self._queue_load_sizes_cum).item() for q in queue_queries
}
- conts = list()
+ conts = []
for i in queue_idxs:
if i not in self._loaded_containers_from_queues:
cont = ivy.Container(
@@ -4084,10 +4064,7 @@ def _get_queue_item(self, query):
shifted_query = slice(query.start - offset, query.stop - offset, query.step)
elif isinstance(query, (list, tuple)):
shifted_query = tuple(
- [
- slice(slc.start - offset, slc.stop - offset, slc.step)
- for slc in query
- ]
+ slice(slc.start - offset, slc.stop - offset, slc.step) for slc in query
)
# noinspection PyUnboundLocalVariable
return combined_cont[shifted_query]
@@ -4116,13 +4093,13 @@ def __getitem__(self, query):
elif ivy.exists(self._queues):
ret = self._get_queue_item(query)
return ret
- return_dict = dict()
+ return_dict = {}
for key, value in self.items():
if isinstance(value, ivy.Container):
return_dict[key] = value[query]
else:
# noinspection PyBroadException
- if isinstance(value, list) or isinstance(value, tuple):
+ if isinstance(value, (list, tuple)):
if len(value) == 0:
return_dict[key] = value
else:
@@ -4205,31 +4182,26 @@ def __getstate__(self):
return state_dict
def __setstate__(self, state_dict):
- if "_local_ivy" in state_dict:
- if ivy.exists(state_dict["_local_ivy"]):
- if len(state_dict["_local_ivy"]) > 0:
- state_dict["_local_ivy"] = ivy.with_backend(
- state_dict["_local_ivy"]
- )
- else:
- state_dict["_local_ivy"] = ivy
+ if "_local_ivy" in state_dict and ivy.exists(state_dict["_local_ivy"]):
+ if len(state_dict["_local_ivy"]) > 0:
+ state_dict["_local_ivy"] = ivy.with_backend(state_dict["_local_ivy"])
+ else:
+ state_dict["_local_ivy"] = ivy
if "_config_in" in state_dict:
config_in = copy.copy(state_dict["_config_in"])
- if "ivyh" in config_in:
- if ivy.exists(config_in["ivyh"]):
- if len(config_in["ivyh"]) > 0:
- config_in["ivyh"] = ivy.with_backend(config_in["ivyh"])
- else:
- config_in["ivyh"] = ivy
+ if "ivyh" in config_in and ivy.exists(config_in["ivyh"]):
+ if len(config_in["ivyh"]) > 0:
+ config_in["ivyh"] = ivy.with_backend(config_in["ivyh"])
+ else:
+ config_in["ivyh"] = ivy
state_dict["_config_in"] = config_in
if "_config" in state_dict:
config = copy.copy(state_dict["_config"])
- if "ivyh" in config:
- if ivy.exists(config["ivyh"]):
- if len(config["ivyh"]) > 0:
- config["ivyh"] = ivy.with_backend(config["ivyh"])
- else:
- config["ivyh"] = ivy
+ if "ivyh" in config and ivy.exists(config["ivyh"]):
+ if len(config["ivyh"]) > 0:
+ config["ivyh"] = ivy.with_backend(config["ivyh"])
+ else:
+ config["ivyh"] = ivy
state_dict["_config"] = config
self.__dict__.update(state_dict)
@@ -4307,7 +4279,7 @@ def cont_max_depth(self):
kcs = [kc for kc in self.cont_to_iterator_keys(include_empty=True)]
if not kcs:
return 0
- return max([len(kc.split("/")) for kc in kcs])
+ return max(len(kc.split("/")) for kc in kcs)
@property
def dynamic_backend(self):
diff --git a/ivy/data_classes/container/data_type.py b/ivy/data_classes/container/data_type.py
index c5c6264937faa..c8d7533d64006 100644
--- a/ivy/data_classes/container/data_type.py
+++ b/ivy/data_classes/container/data_type.py
@@ -23,7 +23,7 @@ def _static_astype(
out: Optional[ivy.Container] = None,
) -> ivy.Container:
"""
- Copy an array to a specified data type irrespective of :ref:`type-promotion`
+ Copy an array to a specified data type irrespective of :ref:`type- promotion`
rules.
.. note::
@@ -94,7 +94,7 @@ def astype(
out: Optional[ivy.Container] = None,
) -> ivy.Container:
"""
- Copy an array to a specified data type irrespective of :ref:`type-promotion`
+ Copy an array to a specified data type irrespective of :ref:`type- promotion`
rules.
.. note::
@@ -156,7 +156,7 @@ def astype(
@staticmethod
def _static_broadcast_arrays(
- *arrays: Union[ivy.Container, ivy.Array, ivy.NativeArray, ivy.Container],
+ *arrays: Union[ivy.Container, ivy.Array, ivy.NativeArray],
key_chains: Optional[Union[List[str], Dict[str, str], ivy.Container]] = None,
to_apply: Union[bool, ivy.Container] = True,
prune_unapplied: Union[bool, ivy.Container] = False,
@@ -232,7 +232,7 @@ def _static_broadcast_arrays(
def broadcast_arrays(
self: ivy.Container,
- *arrays: Union[ivy.Container, ivy.Array, ivy.NativeArray, ivy.Container],
+ *arrays: Union[ivy.Container, ivy.Array, ivy.NativeArray],
key_chains: Optional[Union[List[str], Dict[str, str], ivy.Container]] = None,
to_apply: Union[bool, ivy.Container] = True,
prune_unapplied: Union[bool, ivy.Container] = False,
diff --git a/ivy/data_classes/container/elementwise.py b/ivy/data_classes/container/elementwise.py
index 2c4432952b979..c52a3f42ba646 100644
--- a/ivy/data_classes/container/elementwise.py
+++ b/ivy/data_classes/container/elementwise.py
@@ -9,7 +9,7 @@
class _ContainerWithElementwise(ContainerBase):
@staticmethod
def _static_abs(
- x: Union[ivy.Container, ivy.Array, ivy.NativeArray, ivy.Container],
+ x: Union[ivy.Container, ivy.Array, ivy.NativeArray],
/,
*,
key_chains: Optional[Union[List[str], Dict[str, str], ivy.Container]] = None,
@@ -59,7 +59,6 @@ def _static_abs(
b: ivy.array([4.5, 5.3, 0, 2.3])
}
"""
-
return ContainerBase.cont_multi_map_in_function(
"abs",
x,
@@ -5167,6 +5166,21 @@ def _static_log2(
a container containing the evaluated base ``2`` logarithm for
each element in ``x``. The returned array must have a real-valued
floating-point data type determined by :ref:`type-promotion`.
+
+ Examples
+ --------
+ Using :code:`ivy.Container` input:
+
+ >>> x = ivy.Container(a=ivy.array([0.0, float('nan')]),\
+ b=ivy.array([-0., -4.9, float('+inf')]),\
+ c=ivy.array([8.9, 2.1, 1.]))
+ >>> y = ivy.Container.static_log2(x)
+ >>> print(y)
+ {
+ a: ivy.array([-inf, nan]),
+ b: ivy.array([-inf, nan, inf]),
+ c: ivy.array([3.15, 1.07, 0.])
+ }
"""
return ContainerBase.cont_multi_map_in_function(
"log2",
@@ -5217,6 +5231,21 @@ def log2(
a container containing the evaluated base ``2`` logarithm for each
element in ``self``. The returned array must have a real-valued
floating-point data type determined by :ref:`type-promotion`.
+
+ Examples
+ --------
+ Using :code:`ivy.Container` instance method:
+
+ >>> x = ivy.Container(a=ivy.array([0.0, float('nan')]),
+ ... b=ivy.array([-0., -5.9, float('+inf')]),
+ ... c=ivy.array([8.9, 2.1, 1.]))
+ >>> y = ivy.log2(x)
+ >>> print(y)
+ {
+ a: ivy.array([-inf, nan]),
+ b: ivy.array([-inf, nan, inf]),
+ c: ivy.array([3.15, 1.07, 0.])
+ }
"""
return self._static_log2(
self,
diff --git a/ivy/data_classes/container/experimental/activations.py b/ivy/data_classes/container/experimental/activations.py
index f8067dadcd632..1d1cb87018641 100644
--- a/ivy/data_classes/container/experimental/activations.py
+++ b/ivy/data_classes/container/experimental/activations.py
@@ -26,14 +26,14 @@ def static_logit(
x
Input container.
eps
- When eps is None the function outpus NaN where x < 0 or x > 1.
+ When eps is None the function outputs NaN where x < 0 or x > 1.
and inf or -inf where x = 1 or x = 0, respectively.
Otherwise if eps is defined, x is clamped to [eps, 1 - eps]
complex_mode
optional specifier for how to handle complex data types. See
``ivy.func_wrapper.handle_complex_input`` for more detail.
out
- Optional output Contaner.
+ Optional output Container.
Returns
-------
@@ -88,14 +88,14 @@ def logit(
self
Input container.
eps
- When eps is None the function outpus NaN where x < 0 or x > 1.
+ When eps is None the function outputs NaN where x < 0 or x > 1.
and inf or -inf where x = 1 or x = 0, respectively.
Otherwise if eps is defined, x is clamped to [eps, 1 - eps]
complex_mode
optional specifier for how to handle complex data types. See
``ivy.func_wrapper.handle_complex_input`` for more detail.
out
- Optional output Contaner.
+ Optional output Container.
Returns
-------
@@ -935,7 +935,7 @@ def elu(
)
@staticmethod
- def _static_hardtaanh(
+ def _static_hardtanh(
x: Union[ivy.Array, ivy.NativeArray, ivy.Container],
/,
*,
@@ -1057,7 +1057,7 @@ def hardtanh(
b: ivy.array([1., -0.2])
}
"""
- return self._static_hardtaanh(
+ return self._static_hardtanh(
self,
max_val=max_val,
min_val=min_val,
@@ -1067,3 +1067,802 @@ def hardtanh(
map_sequences=map_sequences,
out=out,
)
+
+ @staticmethod
+ def _static_tanhshrink(
+ x: Union[ivy.Array, ivy.NativeArray, ivy.Container],
+ /,
+ *,
+ key_chains: Optional[Union[List[str], Dict[str, str], ivy.Container]] = None,
+ to_apply: Union[bool, ivy.Container] = True,
+ prune_unapplied: Union[bool, ivy.Container] = False,
+ map_sequences: Union[bool, ivy.Container] = False,
+ out: Optional[ivy.Container] = None,
+ ) -> ivy.Container:
+ """
+ ivy.Container static method variant of ivy.tanhshrink. This method simply wraps
+ the function, and so the docstring for ivy.tanhshrink also applies to this
+ method with minimal changes.
+
+ Parameters
+ ----------
+ x
+ input container.
+ key_chains
+ The key-chains to apply or not apply the method to. Default is ``None``.
+ to_apply
+ If True, the method will be applied to key_chains, otherwise key_chains
+ will be skipped. Default is ``True``.
+ prune_unapplied
+ Whether to prune key_chains for which the function was not applied.
+ Default is ``False``.
+ map_sequences
+ Whether to also map method to sequences (lists, tuples).
+ Default is ``False``.
+ out
+ optional output container, for writing the result to. It must have a shape
+ that the inputs broadcast to.
+
+ Returns
+ -------
+ ret
+ a container with the tanhshrink activation unit function
+ applied element-wise.
+
+ Examples
+ --------
+ >>> x = ivy.Container(a=ivy.array([1.0, -1.2]), b=ivy.array([0.4, -0.2]))
+ >>> y = ivy.Container._static_tanhshrink(x)
+ >>> print(y)
+ {
+ a: ivy.array([0.23840582, -0.36634541]),
+ b: ivy.array([0.02005103, -0.00262468])
+ }
+ """
+ return ContainerBase.cont_multi_map_in_function(
+ "tanhshrink",
+ x,
+ key_chains=key_chains,
+ to_apply=to_apply,
+ prune_unapplied=prune_unapplied,
+ map_sequences=map_sequences,
+ out=out,
+ )
+
+ def tanhshrink(
+ self: ivy.Container,
+ /,
+ *,
+ key_chains: Optional[Union[List[str], Dict[str, str], ivy.Container]] = None,
+ to_apply: Union[bool, ivy.Container] = True,
+ prune_unapplied: Union[bool, ivy.Container] = False,
+ map_sequences: Union[bool, ivy.Container] = False,
+ out: Optional[ivy.Container] = None,
+ ) -> ivy.Container:
+ """
+ ivy.Container instance method variant of ivy.tanhshrink. This method simply
+ wraps the function, and so the docstring for ivy.tanhshrink also applies to this
+ method with minimal changes.
+
+ Parameters
+ ----------
+ self
+ input container.
+ key_chains
+ The key-chains to apply or not apply the method to. Default is ``None``.
+ to_apply
+ If True, the method will be applied to key_chains, otherwise key_chains
+ will be skipped. Default is ``True``.
+ prune_unapplied
+ Whether to prune key_chains for which the function was not applied.
+ Default is ``False``.
+ map_sequences
+ Whether to also map method to sequences (lists, tuples).
+ Default is ``False``.
+ out
+ optional output container, for writing the result to. It must have a shape
+ that the inputs broadcast to.
+
+ Returns
+ -------
+ ret
+ a container with the tanhshrink activation unit function
+ applied element-wise.
+
+ Examples
+ --------
+ >>> x = ivy.Container(a=ivy.array([1.0, -1.2]), b=ivy.array([0.4, -0.2]))
+ >>> y = x.tanhshrink()
+ >>> print(y)
+ {
+ a: ivy.array([0.23840582, -0.36634541]),
+ b: ivy.array([0.02005103, -0.00262468])
+ }
+ """
+ return self._static_tanhshrink(
+ self,
+ key_chains=key_chains,
+ to_apply=to_apply,
+ prune_unapplied=prune_unapplied,
+ map_sequences=map_sequences,
+ out=out,
+ )
+
+ @staticmethod
+ def _static_threshold(
+ x: Union[ivy.Array, ivy.NativeArray, ivy.Container],
+ /,
+ *,
+ threshold: ivy.Container,
+ value: ivy.Container,
+ key_chains: Optional[Union[List[str], Dict[str, str], ivy.Container]] = None,
+ to_apply: Union[bool, ivy.Container] = True,
+ prune_unapplied: Union[bool, ivy.Container] = False,
+ map_sequences: Union[bool, ivy.Container] = False,
+ out: Optional[ivy.Container] = None,
+ ) -> ivy.Container:
+ """
+ ivy.Container static method variant of ivy.threshold. This method simply wraps
+ the function, and so the docstring for ivy.threshold also applies to this method
+ with minimal changes.
+
+ Parameters
+ ----------
+ x
+ input container.
+ threshold
+ threshold value for thresholding operation.
+ value
+ value to replace with if thresholding condition is not met.
+ key_chains
+ The key-chains to apply or not apply the method to.
+ to_apply
+ If True, the method will be applied to key_chains, otherwise key_chains
+ will be skipped.
+ prune_unapplied
+ Whether to prune key_chains for which the function was not applied.
+ map_sequences
+ Whether to also map method to sequences (lists, tuples).
+ out
+ optional output container, for writing the result to. It must have a shape
+ that the inputs broadcast to.
+
+ Returns
+ -------
+ ret
+ a container with the threshold activation unit function
+ applied element-wise.
+
+ Examples
+ --------
+ >>> x = ivy.Container(a=ivy.array([1.0, -1.2]), b=ivy.array([0.4, -0.2]))
+ >>> y = x._static_threshold(threshold=0.5, value=0.0)
+ >>> print(y)
+ {
+ a: ivy.array([1., 0.]),
+ b: ivy.array([0., 0.])
+ }
+ """
+ return ContainerBase.cont_multi_map_in_function(
+ "threshold",
+ x,
+ threshold=threshold,
+ value=value,
+ key_chains=key_chains,
+ to_apply=to_apply,
+ prune_unapplied=prune_unapplied,
+ map_sequences=map_sequences,
+ out=out,
+ )
+
+ def threshold(
+ self: ivy.Container,
+ /,
+ *,
+ threshold: ivy.Container,
+ value: ivy.Container,
+ key_chains: Optional[Union[List[str], Dict[str, str], ivy.Container]] = None,
+ to_apply: Union[bool, ivy.Container] = True,
+ prune_unapplied: Union[bool, ivy.Container] = False,
+ map_sequences: Union[bool, ivy.Container] = False,
+ out: Optional[ivy.Container] = None,
+ ) -> ivy.Container:
+ """
+ ivy.Container instance method variant of ivy.threshold. This method simply wraps
+ the function, and so the docstring for ivy.threshold also applies to this method
+ with minimal changes.
+
+ Parameters
+ ----------
+ self
+ input container.
+ threshold
+ threshold value for thresholding operation.
+ value
+ value to replace with if thresholding condition is not met.
+ key_chains
+ The key-chains to apply or not apply the method to.
+ to_apply
+ If True, the method will be applied to key_chains, otherwise key_chains
+ will be skipped.
+ prune_unapplied
+ Whether to prune key_chains for which the function was not applied.
+ map_sequences
+ Whether to also map method to sequences (lists, tuples).
+ out
+ optional output container, for writing the result to. It must have a shape
+ that the inputs broadcast to.
+
+ Returns
+ -------
+ ret
+ a container with the threshold activation unit function
+ applied element-wise.
+
+ Examples
+ --------
+ >>> x = ivy.Container(a=ivy.array([1.0, -1.2]), b=ivy.array([0.4, -0.2]))
+ >>> y = x.threshold(threshold=0.5, value=0.0)
+ >>> print(y)
+ {
+ a: ivy.array([1., 0.]),
+ b: ivy.array([0., 0.])
+ }
+ """
+ return self._static_threshold(
+ self,
+ threshold=threshold,
+ value=value,
+ key_chains=key_chains,
+ to_apply=to_apply,
+ prune_unapplied=prune_unapplied,
+ map_sequences=map_sequences,
+ out=out,
+ )
+
+ @staticmethod
+ def _static_softshrink(
+ x: Union[ivy.Array, ivy.NativeArray, ivy.Container],
+ /,
+ *,
+ lambd: ivy.Container = 0.5,
+ key_chains: Optional[Union[List[str], Dict[str, str], ivy.Container]] = None,
+ to_apply: Union[bool, ivy.Container] = False,
+ prune_unapplied: Union[bool, ivy.Container] = True,
+ map_sequences: Union[bool, ivy.Container] = False,
+ out: Optional[ivy.Container] = None,
+ ) -> ivy.Container:
+ """
+ ivy.Container static method variant of ivy.softshrink. This method simply wraps
+ the function, and so the docstring for ivy.softshrink also applies to this
+ method with minimal changes.
+
+ Parameters
+ ----------
+ x
+ input container.
+ lambd
+ Lambda value for soft shrinkage calculation.
+ key_chains
+ The key-chains to apply or not apply the method to.
+ to_apply
+ If True, the method will be applied to key_chains, otherwise key_chains
+ will be skipped.
+ prune_unapplied
+ Whether to prune key_chains for which the function was not applied.
+ map_sequences
+ Whether to also map method to sequences (lists, tuples).
+ out
+ optional output container, for writing the result to. It must have a shape
+ that the inputs broadcast to.
+
+ Returns
+ -------
+ ret
+ Container with soft shrinkage applied to the leaves.
+
+ Examples
+ --------
+ >>> x = ivy.Container(a=ivy.array([1., -2.]), b=ivy.array([0.4, -0.2]))
+ >>> y = ivy.Container._static_softshrink(x)
+ >>> print(y)
+ {
+ a: ivy.array([0.5, -1.5]),
+ b: ivy.array([0., 0.])
+ }
+ """
+ return ContainerBase.cont_multi_map_in_function(
+ "softshrink",
+ x,
+ lambd=lambd,
+ key_chains=key_chains,
+ to_apply=to_apply,
+ prune_unapplied=prune_unapplied,
+ map_sequences=map_sequences,
+ out=out,
+ )
+
+ def softshrink(
+ self: ivy.Container,
+ /,
+ *,
+ lambd: ivy.Container = 0.5,
+ key_chains: Optional[Union[List[str], Dict[str, str], ivy.Container]] = None,
+ to_apply: Union[bool, ivy.Container] = False,
+ prune_unapplied: Union[bool, ivy.Container] = True,
+ map_sequences: Union[bool, ivy.Container] = False,
+ out: Optional[ivy.Container] = None,
+ ) -> ivy.Container:
+ """
+ Apply the soft shrinkage function element-wise.
+
+ Parameters
+ ----------
+ self
+ Input container.
+ lambd
+ Lambda value for soft shrinkage calculation.
+ key_chains
+ The key-chains to apply or not apply the method to.
+ to_apply
+ If True, the method will be applied to key_chains, otherwise key_chains
+ will be skipped.
+ prune_unapplied
+ Whether to prune key_chains for which the function was not applied.
+ map_sequences
+ Whether to also map method to sequences (lists, tuples).
+ out
+ optional output container, for writing the result to. It must have a shape
+ that the inputs broadcast to.
+
+ Returns
+ -------
+ ret
+ Container with soft shrinkage applied to the leaves.
+
+ Examples
+ --------
+ >>> import ivy.numpy as np
+ >>> x = ivy.Container(a=np.array([1., -2.]), b=np.array([0.4, -0.2]))
+ >>> y = ivy.Container.softshrink(x)
+ >>> print(y)
+ {
+ a: ivy.array([0.5, -1.5]),
+ b: ivy.array([0., 0.])
+ }
+ """
+ return self._static_softshrink(
+ self,
+ lambd=lambd,
+ key_chains=key_chains,
+ to_apply=to_apply,
+ prune_unapplied=prune_unapplied,
+ map_sequences=map_sequences,
+ out=out,
+ )
+
+ @staticmethod
+ def _static_celu(
+ x: Union[ivy.Array, ivy.NativeArray, ivy.Container],
+ /,
+ *,
+ alpha: ivy.Container = 1.0,
+ key_chains: Optional[Union[List[str], Dict[str, str], ivy.Container]] = None,
+ to_apply: Union[bool, ivy.Container] = True,
+ prune_unapplied: Union[bool, ivy.Container] = False,
+ map_sequences: Union[bool, ivy.Container] = False,
+ complex_mode: Literal["split", "magnitude", "jax"] = "jax",
+ out: Optional[ivy.Container] = None,
+ ) -> ivy.Container:
+ """
+ ivy.Container static method variant of ivy.celu. This method simply wraps the
+ function, and so the docstring for ivy.celu also applies to this method with
+ minimal changes.
+
+ Parameters
+ ----------
+ x
+ input container.
+ alpha
+ array or scalar specifying the alpha value for CELU formlation.
+ key_chains
+ The key-chains to apply or not apply the method to. Default is ``None``.
+ to_apply
+ If True, the method will be applied to key_chains, otherwise key_chains
+ will be skipped. Default is ``True``.
+ prune_unapplied
+ Whether to prune key_chains for which the function was not applied.
+ Default is ``False``.
+ map_sequences
+ Whether to also map method to sequences (lists, tuples).
+ Default is ``False``.
+ complex_mode
+ optional specifier for how to handle complex data types. See
+ ``ivy.func_wrapper.handle_complex_input`` for more detail.
+ out
+ optional output container, for writing the result to. It must have a shape
+ that the inputs broadcast to.
+
+ Returns
+ -------
+ ret
+ a container with the celu unit function applied element-wise.
+
+ Examples
+ --------
+ >>> x = x = ivy.Container(a=ivy.array([0.39, -0.85]), b=ivy.array([1., -0.2]))
+ >>> y = ivy.Container.static_celu(x)
+ >>> print(y)
+ {
+ a: ivy.array([0.38999999, -0.17]),
+ b: ivy.array([1., -0.04])
+ }
+ """
+ return ContainerBase.cont_multi_map_in_function(
+ "celu",
+ x,
+ alpha=alpha,
+ key_chains=key_chains,
+ to_apply=to_apply,
+ prune_unapplied=prune_unapplied,
+ map_sequences=map_sequences,
+ complex_mode=complex_mode,
+ out=out,
+ )
+
+ def celu(
+ self: ivy.Container,
+ /,
+ *,
+ alpha: ivy.Container = 1.0,
+ key_chains: Optional[Union[List[str], Dict[str, str], ivy.Container]] = None,
+ to_apply: Union[bool, ivy.Container] = True,
+ prune_unapplied: Union[bool, ivy.Container] = False,
+ map_sequences: Union[bool, ivy.Container] = False,
+ complex_mode: Literal["split", "magnitude", "jax"] = "jax",
+ out: Optional[ivy.Container] = None,
+ ) -> ivy.Container:
+ """
+ ivy.Container instance method variant of ivy.leaky_relu. This method simply
+ wraps the function, and so the docstring for ivy.leaky_relu also applies to this
+ method with minimal changes.
+
+ Parameters
+ ----------
+ self
+ input container.
+ alpha
+ array or scalar specifying alpha (negative slope) value for CELU
+ formulation.
+ key_chains
+ The key-chains to apply or not apply the method to. Default is ``None``.
+ to_apply
+ If True, the method will be applied to key_chains, otherwise key_chains
+ will be skipped. Default is ``True``.
+ prune_unapplied
+ Whether to prune key_chains for which the function was not applied.
+ Default is ``False``.
+ map_sequences
+ Whether to also map method to sequences (lists, tuples).
+ Default is ``False``.
+ complex_mode
+ optional specifier for how to handle complex data types. See
+ ``ivy.func_wrapper.handle_complex_input`` for more detail.
+ out
+ optional output container, for writing the result to. It must have a shape
+ that the inputs broadcast to.
+
+ Returns
+ -------
+ ret
+ a container with the celu unit function applied element-wise.
+
+ Examples
+ --------
+ >>> x = ivy.Container(a=ivy.array([0.39, -0.85]), b=ivy.array([1., -0.2]))
+ >>> y = x.celu()
+ >>> print(y)
+ {
+ a: ivy.array([0.38999999, -0.57]),
+ b: ivy.array([1., -0.18])
+ }
+ """
+ return self._static_celu(
+ self,
+ alpha=alpha,
+ key_chains=key_chains,
+ to_apply=to_apply,
+ prune_unapplied=prune_unapplied,
+ map_sequences=map_sequences,
+ complex_mode=complex_mode,
+ out=out,
+ )
+
+ @staticmethod
+ def _static_scaled_tanh(
+ x: Union[ivy.Array, ivy.NativeArray, ivy.Container],
+ /,
+ *,
+ alpha: Union[float, ivy.Container] = 1.7159,
+ beta: Union[float, ivy.Container] = 0.67,
+ key_chains: Optional[Union[List[str], Dict[str, str], ivy.Container]] = None,
+ to_apply: Union[bool, ivy.Container] = True,
+ prune_unapplied: Union[bool, ivy.Container] = False,
+ map_sequences: Union[bool, ivy.Container] = False,
+ out: Optional[ivy.Container] = None,
+ ) -> ivy.Container:
+ """
+ ivy.Container static method variant of ivy.scaled_tanh. This method simply wraps
+ the function, and so the docstring for ivy.scaled_tanh also applies to this
+ method with minimal changes.
+
+ Parameters
+ ----------
+ x
+ input container.
+ alpha
+ The scaling parameter for the output.
+ Determines the amplitude of the tanh function.
+ Default: 1.7159
+ beta
+ The scaling parameter for the input.
+ Determines the slope of the tanh function.
+ Default: 0.67
+ key_chains
+ The key-chains to apply or not apply the method to. Default is ``None``.
+ to_apply
+ If True, the method will be applied to key_chains, otherwise key_chains
+ will be skipped. Default is ``True``.
+ prune_unapplied
+ Whether to prune key_chains for which the function was not applied.
+ Default is ``False``.
+ map_sequences
+ Whether to also map method to sequences (lists, tuples).
+ Default is ``False``.
+ out
+ optional output container, for writing the result to. It must have a shape
+ that the inputs broadcast to.
+
+ Returns
+ -------
+ ret
+ a container with the scaled_tanh function applied.
+
+ Examples
+ --------
+ >>> x = ivy.Container(a=ivy.array([8.931, -0.85]), b=ivy.array([1., -0.2])))
+ >>> y = ivy.Container._static_scaled_tanh(x)
+ >>> y
+ {
+ a: ivy.array([1.71587813, -0.88367474]),
+ b: ivy.array([1.00376701, -0.2285642])
+ }
+
+ >>> x = ivy.Container(a=ivy.array([8.9, -8.9]), b=ivy.array([3., 33.2]))
+ >>> y = ivy.Container._static_scaled_tanh(x, alpha=2, beta=2.5)
+ >>> y
+ {
+ a: ivy.array([2., -2.]),
+ b: ivy.array([1.99999881, 2.])
+ }
+
+ >>> x = ivy.Container(a=ivy.array([0.3, -0.3]), b=ivy.array([33.0, -33.0]))
+ >>> y = ivy.Container._static_scaled_tanh(x, alpha=1.5, beta=25)
+ >>> y
+ {
+ a: ivy.array([1.49999905, -1.49999905]),
+ b: ivy.array([1.5, -1.5])
+ }
+ """
+ return ContainerBase.cont_multi_map_in_function(
+ "scaled_tanh",
+ x,
+ alpha=alpha,
+ beta=beta,
+ key_chains=key_chains,
+ to_apply=to_apply,
+ prune_unapplied=prune_unapplied,
+ map_sequences=map_sequences,
+ out=out,
+ )
+
+ def scaled_tanh(
+ self: ivy.Container,
+ /,
+ *,
+ alpha: Union[float, ivy.Container] = 1.7159,
+ beta: Union[float, ivy.Container] = 0.67,
+ key_chains: Optional[Union[List[str], Dict[str, str], ivy.Container]] = None,
+ to_apply: Union[bool, ivy.Container] = True,
+ prune_unapplied: Union[bool, ivy.Container] = False,
+ map_sequences: Union[bool, ivy.Container] = False,
+ out: Optional[ivy.Container] = None,
+ ) -> ivy.Container:
+ """
+ ivy.Container instance method variant of ivy.scaled_tanh. This method
+ simplywraps the function, and so the docstring for ivy.scaled_tanh also applies
+ to this method with minimal changes.
+
+ Parameters
+ ----------
+ x
+ input container.
+ alpha
+ The scaling parameter for the output.
+ Determines the amplitude of the tanh function.
+ Default: 1.7159
+ beta
+ The scaling parameter for the input.
+ Determines the slope of the tanh function.
+ Default: 0.67
+ key_chains
+ The key-chains to apply or not apply the method to. Default is ``None``.
+ to_apply
+ If True, the method will be applied to key_chains, otherwise key_chains
+ will be skipped. Default is ``True``.
+ prune_unapplied
+ Whether to prune key_chains for which the function was not applied.
+ Default is ``False``.
+ map_sequences
+ Whether to also map method to sequences (lists, tuples).
+ Default is ``False``.
+ out
+ optional output container, for writing the result to. It must have a shape
+ that the inputs broadcast to.
+
+ Returns
+ -------
+ ret
+ a container with the scaled_tanh function applied.
+
+ Examples
+ --------
+ >>> x = ivy.Container(a=ivy.array([2., 3.]), b=ivy.array([1., 2.]))
+ >>> x.scaled_tanh()
+ {
+ a: ivy.array([1.49570239, 1.65537548]),
+ b: ivy.array([1.00376701, 1.49570239])
+ }
+
+ >>> x = ivy.Container(a=ivy.array([1., 1.]), b=ivy.array([1., 1.]))
+ >>> x.scaled_tanh(alpha=30)
+ {
+ a: ivy.array([17.54939651, 17.54939651]),
+ b: ivy.array([17.54939651, 17.54939651])
+ }
+
+ >>> x = ivy.Container(a=ivy.array([20., 21.]), b=ivy.array([3., 1.]))
+ >>> x.scaled_tanh(alpha=0.1, beta=-0.4)
+ {
+ a: ivy.array([-0.09999998, -0.09999999]),
+ b: ivy.array([-0.08336546, -0.0379949])
+ }
+ """
+ return self._static_scaled_tanh(
+ self,
+ alpha=alpha,
+ beta=beta,
+ key_chains=key_chains,
+ to_apply=to_apply,
+ prune_unapplied=prune_unapplied,
+ map_sequences=map_sequences,
+ out=out,
+ )
+
+ @staticmethod
+ def _static_hardshrink(
+ x: Union[ivy.Array, ivy.NativeArray, ivy.Container],
+ /,
+ *,
+ lambd: ivy.Container = 0.5,
+ key_chains: Optional[Union[List[str], Dict[str, str], ivy.Container]] = None,
+ to_apply: Union[bool, ivy.Container] = False,
+ prune_unapplied: Union[bool, ivy.Container] = True,
+ map_sequences: Union[bool, ivy.Container] = False,
+ out: Optional[ivy.Container] = None,
+ ) -> ivy.Container:
+ """
+ ivy.Container static method variant of ivy.hardshrink. This method simply wraps
+ the function, and so the docstring for ivy.hardshrink also applies to this
+ method with minimal changes.
+
+ Parameters
+ ----------
+ x
+ input container.
+ lambd
+ Lambda value for hard shrinkage calculation.
+ key_chains
+ The key-chains to apply or not apply the method to.
+ to_apply
+ If True, the method will be applied to key_chains, otherwise key_chains
+ will be skipped.
+ prune_unapplied
+ Whether to prune key_chains for which the function was not applied.
+ map_sequences
+ Whether to also map method to sequences (lists, tuples).
+
+ Returns
+ -------
+ ret
+ Container with hard shrinkage applied to the leaves.
+
+ Examples
+ --------
+ >>> x = ivy.Container(a=ivy.array([1., -2.]), b=ivy.array([0.4, -0.2]))
+ >>> y = ivy.Container._static_hardshrink(x)
+ >>> print(y)
+ {
+ a: ivy.array([1., -2.]),
+ b: ivy.array([0., 0.])
+ }
+ """
+ return ContainerBase.cont_multi_map_in_function(
+ "hardshrink",
+ x,
+ lambd=lambd,
+ key_chains=key_chains,
+ to_apply=to_apply,
+ prune_unapplied=prune_unapplied,
+ map_sequences=map_sequences,
+ out=out,
+ )
+
+ def hardshrink(
+ self: ivy.Container,
+ /,
+ *,
+ lambd: ivy.Container = 0.5,
+ key_chains: Optional[Union[List[str], Dict[str, str], ivy.Container]] = None,
+ to_apply: Union[bool, ivy.Container] = False,
+ prune_unapplied: Union[bool, ivy.Container] = True,
+ map_sequences: Union[bool, ivy.Container] = False,
+ out: Optional[ivy.Container] = None,
+ ) -> ivy.Container:
+ """
+ Apply the hard shrinkage function element-wise.
+
+ Parameters
+ ----------
+ self
+ Input container.
+ lambd
+ Lambda value for hard shrinkage calculation.
+ key_chains
+ The key-chains to apply or not apply the method to.
+ to_apply
+ If True, the method will be applied to key_chains, otherwise key_chains
+ will be skipped.
+ prune_unapplied
+ Whether to prune key_chains for which the function was not applied.
+ map_sequences
+ Whether to also map method to sequences (lists, tuples).
+ out
+ optional output container, for writing the result to. It must have a shape
+ that the inputs broadcast to.
+
+ Returns
+ -------
+ ret
+ Container with hard shrinkage applied to the leaves.
+
+ Examples
+ --------
+ >>> import ivy.numpy as np
+ >>> x = ivy.Container(a=np.array([1., -2.]), b=np.array([0.4, -0.2]))
+ >>> y = ivy.Container.hardshrink(x)
+ >>> print(y)
+ {
+ a: ivy.array([1., -2.]),
+ b: ivy.array([0., 0.])
+ }
+ """
+ return self._static_hardshrink(
+ self,
+ lambd=lambd,
+ key_chains=key_chains,
+ to_apply=to_apply,
+ prune_unapplied=prune_unapplied,
+ map_sequences=map_sequences,
+ out=out,
+ )
diff --git a/ivy/data_classes/container/experimental/creation.py b/ivy/data_classes/container/experimental/creation.py
index 63eb416191d7b..b760f16ddde3f 100644
--- a/ivy/data_classes/container/experimental/creation.py
+++ b/ivy/data_classes/container/experimental/creation.py
@@ -127,7 +127,7 @@ def static_kaiser_window(
Parameters
----------
window_length
- input container including window lenghts.
+ input container including window lengths.
periodic
If True, returns a periodic window suitable for use in spectral analysis.
If False, returns a symmetric window suitable for use in filter design.
@@ -185,7 +185,7 @@ def kaiser_window(
Parameters
----------
self
- input container including window lenghts.
+ input container including window lengths.
periodic
If True, returns a periodic window suitable for use in spectral analysis.
If False, returns a symmetric window suitable for use in filter design.
@@ -244,7 +244,7 @@ def static_kaiser_bessel_derived_window(
Parameters
----------
x
- input container including window lenghts.
+ input container including window lengths.
periodic
If True, returns a periodic window suitable for use in spectral analysis.
If False, returns a symmetric window suitable for use in filter design.
@@ -303,7 +303,7 @@ def kaiser_bessel_derived_window(
Parameters
----------
self
- input container including window lenghts.
+ input container including window lengths.
periodic
If True, returns a periodic window suitable for use in spectral analysis.
If False, returns a symmetric window suitable for use in filter design.
@@ -363,7 +363,7 @@ def static_hamming_window(
Parameters
----------
x
- input container including window lenghts.
+ input container including window lengths.
periodic
If True, returns a window to be used as periodic function.
If False, return a symmetric window.
@@ -425,7 +425,7 @@ def hamming_window(
Parameters
----------
self
- input container including window lenghts.
+ input container including window lengths.
periodic
If True, returns a window to be used as periodic function.
If False, return a symmetric window.
@@ -476,7 +476,7 @@ def static_vorbis_window(
Parameters
----------
x
- input container including window lenghts.
+ input container including window lengths.
dtype
data type of the returned arrays.
@@ -528,7 +528,7 @@ def vorbis_window(
Parameters
----------
self
- input container including window lenghts.
+ input container including window lengths.
dtype
data type of the returned arrays.
out
@@ -1200,3 +1200,193 @@ def mel_weight_matrix(
lower_edge_hertz,
upper_edge_hertz,
)
+
+ @staticmethod
+ def static_unsorted_segment_mean(
+ data: ivy.Container,
+ segment_ids: Union[ivy.Array, ivy.Container],
+ num_segments: Union[int, ivy.Container],
+ *,
+ key_chains: Optional[Union[List[str], Dict[str, str], ivy.Container]] = None,
+ to_apply: Union[bool, ivy.Container] = True,
+ prune_unapplied: Union[bool, ivy.Container] = False,
+ map_sequences: Union[bool, ivy.Container] = False,
+ ) -> ivy.Container:
+ """
+ Compute the mean of values in the input data based on segment identifiers.
+
+ Parameters
+ ----------
+ data : ivy.Container
+ Input array or container from which to gather the input.
+ segment_ids : ivy.Container
+ An array of integers indicating the segment identifier for each element in
+ 'data'.
+ num_segments : Union[int, ivy.Container]
+ An integer or array representing the total number of distinct segment IDs.
+ key_chains : Optional[Union[List[str], Dict[str, str], ivy.Container]], optional
+ The key-chains to apply or not apply the method to. Default is None.
+ to_apply : Union[bool, ivy.Container], optional
+ If True, the method will be applied to key-chains, otherwise key-chains will
+ be skipped. Default is True.
+ prune_unapplied : Union[bool, ivy.Container], optional
+ Whether to prune key-chains for which the function was not applied.
+ Default is False.
+ map_sequences : Union[bool, ivy.Container], optional
+ Whether to also map method to sequences (lists, tuples). Default is False.
+
+ Returns
+ -------
+ ivy.Container
+ A container representing the result of a segmented mean operation.
+ For each segment, it computes the mean of values in 'data' where
+ 'segment_ids' equals the segment ID.
+ """
+ return ContainerBase.cont_multi_map_in_function(
+ "unsorted_segment_mean",
+ data,
+ segment_ids,
+ num_segments,
+ key_chains=key_chains,
+ to_apply=to_apply,
+ prune_unapplied=prune_unapplied,
+ map_sequences=map_sequences,
+ )
+
+ def static_polyval(
+ coeffs: ivy.Container,
+ x: Union[ivy.Container, int, float],
+ *,
+ key_chains: Optional[Union[List[str], Dict[str, str]]] = None,
+ to_apply: bool = True,
+ prune_unapplied: bool = False,
+ map_sequences: bool = False,
+ ) -> ivy.Container:
+ r"""
+ ivy.Container static method variant of ivy.polyval. This method simply wraps the
+ function, and so the docstring for ivy.polyval also applies to this method with
+ minimal changes.
+
+ Evaluate and return a polynomial at specific given values.
+
+ Parameters
+ ----------
+ coeffs
+ Polynomial coefficients (including zero) from highest degree
+ to constant term.
+ x
+ The value of the indeterminate variable at which to evaluate the polynomial.
+ key_chains
+ The key-chains to apply or not apply the method to. Default is ``None``.
+ to_apply
+ If True, the method will be applied to key_chains, otherwise key_chains
+ will be skipped. Default is ``True``.
+ prune_unapplied
+ Whether to prune key_chains for which the function was not applied.
+ Default is ``False``.
+ map_sequences
+ Whether to also map method to sequences (lists, tuples).
+ Default is ``False``.
+
+ Returns
+ -------
+ ret
+ Output container containing simplified result of substituing x in the
+ coefficients - final value of polynomial.
+ """
+ return ContainerBase.cont_multi_map_in_function(
+ "polyval",
+ coeffs,
+ x,
+ key_chains=key_chains,
+ to_apply=to_apply,
+ prune_unapplied=prune_unapplied,
+ map_sequences=map_sequences,
+ )
+
+ def unsorted_segment_mean(
+ self: ivy.Container,
+ segment_ids: Union[ivy.Array, ivy.Container],
+ num_segments: Union[int, ivy.Container],
+ ) -> ivy.Container:
+ """
+ Compute the mean of values in the input array or container based on segment
+ identifiers.
+
+ Parameters
+ ----------
+ self : ivy.Container
+ Input array or container from which to gather the input.
+ segment_ids : ivy.Container
+ An array of integers indicating the segment identifier for each element
+ in 'self'.
+ num_segments : Union[int, ivy.Container]
+ An integer or array representing the total number of distinct segment IDs.
+
+ Returns
+ -------
+ ivy.Container
+ A container representing the result of a segmented mean operation.
+ For each segment, it computes the mean of values in 'self' where
+ 'segment_ids' equals the segment ID.
+
+ Example
+ --------
+ >>> data = ivy.Container(a=ivy.array([0., 1., 2., 4.]),
+ ... b=ivy.array([3., 4., 5., 6.]))
+ >>> segment_ids = ivy.array([0, 0, 1, 1])
+ >>> num_segments = 2
+ >>> result = ivy.unsorted_segment_mean(data, segment_ids, num_segments)
+ >>> print(result)
+ {
+ a: ivy.array([0.5, 3.0]),
+ b: ivy.array([3.5, 5.5])
+ }
+
+ >>> data = ivy.Container(a=ivy.array([0., 1., 2., 4., 5., 6.]),
+ ... b=ivy.array([3., 4., 5., 6., 7., 8.]))
+ >>> segment_ids = ivy.array([0, 0, 1, 1, 2, 2])
+ >>> num_segments = 3
+ >>> result = ivy.unsorted_segment_mean(data, segment_ids, num_segments)
+ >>> print(result)
+ {
+ a: ivy.array([0.5, 3.0, 5.5]),
+ b: ivy.array([3.5, 5.5, 7.5])
+ }
+ """
+ return self.static_unsorted_segment_mean(
+ self,
+ segment_ids,
+ num_segments,
+ )
+
+ def polyval(
+ self: ivy.Container,
+ coeffs: ivy.Container,
+ x: ivy.Container,
+ ) -> ivy.Container:
+ r"""
+ ivy.Container instance method variant of ivy.polyval. This method simply wraps
+ the function, and so the docstring for ivy.polyval also applies to this method
+ with minimal changes.
+
+ Evaluate and return a polynomial at specific given values.
+
+ Parameters
+ ----------
+ self
+ Arbitrary input container
+ coeffs
+ Polynomial coefficients (including zero) from highest degree to
+ constant term.
+ x
+ The value of the indeterminate variable at which to
+ evaluate the polynomial.
+
+ Returns
+ -------
+ ret
+ Output container containing simplified result of substituing x in the
+ coefficients - final value of polynomial.
+ """
+ return self.static_polyval(self, coeffs, x)
diff --git a/ivy/data_classes/container/experimental/elementwise.py b/ivy/data_classes/container/experimental/elementwise.py
index d25e8973c157c..45cf7674adbb0 100644
--- a/ivy/data_classes/container/experimental/elementwise.py
+++ b/ivy/data_classes/container/experimental/elementwise.py
@@ -1,5 +1,5 @@
# global
-from typing import Optional, Union, List, Dict, Tuple
+from typing import Optional, Union, List, Dict, Tuple, Sequence
from numbers import Number
# local
@@ -8,6 +8,388 @@
class _ContainerWithElementWiseExperimental(ContainerBase):
+ @staticmethod
+ def static_amax(
+ x: ivy.Container,
+ /,
+ *,
+ axis: Optional[Union[int, Sequence[int], ivy.Container]] = None,
+ keepdims: Union[bool, ivy.Container] = False,
+ key_chains: Optional[Union[List[str], Dict[str, str], ivy.Container]] = None,
+ to_apply: Union[bool, ivy.Container] = True,
+ prune_unapplied: Union[bool, ivy.Container] = False,
+ map_sequences: Union[bool, ivy.Container] = False,
+ out: Optional[ivy.Container] = None,
+ ) -> ivy.Container:
+ """
+ ivy.Container static method variant of ivy.amax. This method simply wraps the
+ function, and so the docstring for ivy.amax also applies to this method with
+ minimal changes.
+
+ Parameters
+ ----------
+ x
+ input container. Should have a real-valued data type.
+ axis
+ axis or axes along which maximum values must be computed.
+ By default, the maximum value must be computed over the
+ entire array. If a tuple of integers, maximum values must
+ be computed over multiple axes. Default: ``None``.
+ keepdims
+ optional boolean, if ``True``, the reduced axes
+ (dimensions) must be included in the result as singleton
+ dimensions, and, accordingly, the result must be
+ compatible with the input array
+ (see `broadcasting`_).
+ Otherwise, if ``False``, the reduced axes (dimensions)
+ must not be included in the result.
+ Default: ``False``.
+ key_chains
+ The key-chains to apply or not apply the method to.
+ Default is ``None``.
+ to_apply
+ If True, the method will be applied to key_chains,
+ otherwise key_chains will be skipped. Default is ``True``.
+ prune_unapplied
+ Whether to prune key_chains for which the function was
+ not applied. Default is ``False``.
+ map_sequences
+ Whether to also map method to sequences (lists, tuples).
+ Default is ``False``.
+ out
+ optional output, for writing the result to.
+ It must have a shape that the inputs broadcast to.
+
+ Returns
+ -------
+ ret
+ container, if the maximum value was computed over the entire array,
+ a zero-dimensional array containing the maximum value;
+ otherwise, a non-zero-dimensional array containing the
+ maximum values. The returned array must have the same data type
+ as ``x``.
+
+ Examples
+ --------
+ With :class:`ivy.Container` input:
+
+ >>> x = ivy.Container(a=ivy.array([1, 2, 3]),
+ ... b=ivy.array([2, 3, 4]))
+ >>> y = ivy.Container.static_amax(x)
+ >>> print(y)
+ {
+ a: ivy.array(3),
+ b: ivy.array(4)
+ }
+
+ >>> x = ivy.Container(a=ivy.array([[1, 2, 3], [-1, 0, 2]]),
+ ... b=ivy.array([[2, 3, 4], [0, 1, 2]]))
+ >>> y = ivy.Container.static_amax(x, axis=1)
+ >>> print(y)
+ {
+ a:ivy.array([3, 2]),
+ b:ivy.array([4, 2])
+ }
+ """
+ return ContainerBase.cont_multi_map_in_function(
+ "amax",
+ x,
+ axis=axis,
+ keepdims=keepdims,
+ key_chains=key_chains,
+ to_apply=to_apply,
+ prune_unapplied=prune_unapplied,
+ map_sequences=map_sequences,
+ out=out,
+ )
+
+ def amax(
+ self: ivy.Container,
+ /,
+ *,
+ axis: Optional[Union[int, Sequence[int], ivy.Container]] = None,
+ keepdims: Union[bool, ivy.Container] = False,
+ key_chains: Optional[Union[List[str], Dict[str, str], ivy.Container]] = None,
+ to_apply: Union[bool, ivy.Container] = True,
+ prune_unapplied: Union[bool, ivy.Container] = False,
+ map_sequences: Union[bool, ivy.Container] = False,
+ out: Optional[ivy.Container] = None,
+ ) -> ivy.Container:
+ """
+ ivy.Container instance method variant of ivy.amax. This method simply wraps the
+ function, and so the docstring for ivy.amax also applies to this method with
+ minimal changes.
+
+ Parameters
+ ----------
+ self
+ input container. Should have a real-valued data type.
+ axis
+ axis or axes along which maximum values must be computed.
+ By default, the maximum value must be computed over the
+ entire array. If a tuple of integers, maximum values must
+ be computed over multiple axes. Default: ``None``.
+ keepdims
+ optional boolean, if ``True``, the reduced axes
+ (dimensions) must be included in the result as singleton
+ dimensions, and, accordingly, the result must be
+ compatible with the input array
+ (see `broadcasting`_).
+ Otherwise, if ``False``, the reduced axes (dimensions)
+ must not be included in the result.
+ Default: ``False``.
+ key_chains
+ The key-chains to apply or not apply the method to.
+ Default is ``None``.
+ to_apply
+ If True, the method will be applied to key_chains,
+ otherwise key_chains will be skipped. Default is ``True``.
+ prune_unapplied
+ Whether to prune key_chains for which the function was
+ not applied. Default is ``False``.
+ map_sequences
+ Whether to also map method to sequences (lists, tuples).
+ Default is ``False``.
+ out
+ optional output, for writing the result to.
+ It must have a shape that the inputs broadcast to.
+
+ Returns
+ -------
+ ret
+ container, if the maximum value was computed over the entire array,
+ a zero-dimensional array containing the maximum value;
+ otherwise, a non-zero-dimensional array containing the
+ maximum values. The returned array must have the same data type
+ as ``x``.
+
+ Examples
+ --------
+ With :class:`ivy.Container` input:
+
+ >>> x = ivy.Container(a=ivy.array([1, 2, 3]),
+ ... b=ivy.array([2, 3, 4]))
+ >>> y = x.amax()
+ >>> print(y)
+ {
+ a: ivy.array(3),
+ b: ivy.array(4)
+ }
+
+ >>> x = ivy.Container(a=ivy.array([[1, 2, 3], [-1, 0, 2]]),
+ ... b=ivy.array([[2, 3, 4], [0, 1, 2]]))
+ >>> y = x.amax(axis=1)
+ >>> print(y)
+ {
+ a:ivy.array([3, 2]),
+ b:ivy.array([4, 2])
+ }
+ """
+ return self.static_amax(
+ self,
+ axis=axis,
+ keepdims=keepdims,
+ key_chains=key_chains,
+ to_apply=to_apply,
+ prune_unapplied=prune_unapplied,
+ map_sequences=map_sequences,
+ out=out,
+ )
+
+ @staticmethod
+ def static_amin(
+ x: ivy.Container,
+ /,
+ *,
+ axis: Optional[Union[int, Sequence[int], ivy.Container]] = None,
+ keepdims: Union[bool, ivy.Container] = False,
+ key_chains: Optional[Union[List[str], Dict[str, str], ivy.Container]] = None,
+ to_apply: Union[bool, ivy.Container] = True,
+ prune_unapplied: Union[bool, ivy.Container] = False,
+ map_sequences: Union[bool, ivy.Container] = False,
+ out: Optional[ivy.Container] = None,
+ ) -> ivy.Container:
+ """
+ ivy.Container static method variant of ivy.amin. This method simply wraps the
+ function, and so the docstring for ivy.amin also applies to this method with
+ minimal changes.
+
+ Parameters
+ ----------
+ x
+ input container. Should have a real-valued data type.
+ axis
+ axis or axes along which minimum values must be computed.
+ By default, the minimum value must be computed over the
+ entire array. If a tuple of integers, minimum values must
+ be computed over multiple axes. Default: ``None``.
+ keepdims
+ optional boolean, if ``True``, the reduced axes
+ (dimensions) must be included in the result as
+ singleton dimensions, and, accordingly, the
+ result must be compatible with the input array
+ (see `broadcasting`_). Otherwise,
+ if ``False``, the reduced axes (dimensions)
+ must not be included in the result.
+ Default: ``False``.
+ key_chains
+ The key-chains to apply or not apply the method to.
+ Default is ``None``.
+ to_apply
+ If True, the method will be applied to key_chains,
+ otherwise key_chains will be skipped. Default is ``True``.
+ prune_unapplied
+ Whether to prune key_chains for which the function was
+ not applied. Default is ``False``.
+ map_sequences
+ Whether to also map method to sequences (lists, tuples).
+ Default is ``False``.
+ out
+ optional output, for writing the result to.
+ It must have a shape that the inputs broadcast to.
+
+ Returns
+ -------
+ ret
+ container, if the minimum value was computed over the entire array,
+ a zero-dimensional array containing the minimum value;
+ otherwise, a non-zero-dimensional array containing the
+ minimum values. The returned array must have the same data type
+ as ``x``.
+
+ Examples
+ --------
+ With :class:`ivy.Container` input:
+
+ >>> x = ivy.Container(a=ivy.array([1, 2, 3]),
+ ... b=ivy.array([2, 3, 4]))
+ >>> y = ivy.Container.static_amin(x)
+ >>> print(y)
+ {
+ a: ivy.array(1),
+ b: ivy.array(2)
+ }
+
+ >>> x = ivy.Container(a=ivy.array([[1, 2, 3], [-1, 0, 2]]),
+ ... b=ivy.array([[2, 3, 4], [0, 1, 2]]))
+ >>> y = ivy.Container.static_amin(x, axis=1)
+ >>> print(y)
+ {
+ a:ivy.array([1, -1]),
+ b:ivy.array([2, 0])
+ }
+ """
+ return ContainerBase.cont_multi_map_in_function(
+ "amin",
+ x,
+ axis=axis,
+ keepdims=keepdims,
+ key_chains=key_chains,
+ to_apply=to_apply,
+ prune_unapplied=prune_unapplied,
+ map_sequences=map_sequences,
+ out=out,
+ )
+
+ def amin(
+ self: ivy.Container,
+ /,
+ *,
+ axis: Optional[Union[int, Sequence[int], ivy.Container]] = None,
+ keepdims: Union[bool, ivy.Container] = False,
+ key_chains: Optional[Union[List[str], Dict[str, str], ivy.Container]] = None,
+ to_apply: Union[bool, ivy.Container] = True,
+ prune_unapplied: Union[bool, ivy.Container] = False,
+ map_sequences: Union[bool, ivy.Container] = False,
+ out: Optional[ivy.Container] = None,
+ ) -> ivy.Container:
+ """
+ ivy.Container instance method variant of ivy.amin. This method simply wraps the
+ function, and so the docstring for ivy.amin also applies to this method with
+ minimal changes.
+
+ Parameters
+ ----------
+ self
+ input container. Should have a real-valued data type.
+ axis
+ axis or axes along which minimum values must be computed.
+ By default, the minimum value must be computed over the
+ entire array. If a tuple of integers, minimum values must
+ be computed over multiple axes. Default: ``None``.
+ keepdims
+ optional boolean, if ``True``, the reduced axes
+ (dimensions) must be included in the result as
+ singleton dimensions, and, accordingly, the
+ result must be compatible with the input array
+ (see `broadcasting`_). Otherwise,
+ if ``False``, the reduced axes (dimensions)
+ must not be included in the result.
+ Default: ``False``.
+ key_chains
+ The key-chains to apply or not apply the method to.
+ Default is ``None``.
+ to_apply
+ If True, the method will be applied to key_chains,
+ otherwise key_chains will be skipped. Default is ``True``.
+ prune_unapplied
+ Whether to prune key_chains for which the function was
+ not applied. Default is ``False``.
+ map_sequences
+ Whether to also map method to sequences (lists, tuples).
+ Default is ``False``.
+ out
+ optional output, for writing the result to.
+ It must have a shape that the inputs broadcast to.
+
+ Returns
+ -------
+ ret
+ container, if the minimum value was computed over the entire array,
+ a zero-dimensional array containing the minimum value;
+ otherwise, a non-zero-dimensional array containing the
+ minimum values. The returned array must have the same data type
+ as ``x``.
+
+ Examples
+ --------
+ With :class:`ivy.Container` input:
+
+ >>> x = ivy.Container(a=ivy.array([1, 2, 3]),
+ ... b=ivy.array([2, 3, 4]))
+ >>> y = x.amin()
+ >>> print(y)
+ {
+ a: ivy.array(1),
+ b: ivy.array(2)
+ }
+
+ >>> x = ivy.Container(a=ivy.array([[1, 2, 3], [-1, 0, 2]]),
+ ... b=ivy.array([[2, 3, 4], [0, 1, 2]]))
+ >>> y = x.amin(axis=1)
+ >>> print(y)
+ {
+ a:ivy.array([1, -1]),
+ b:ivy.array([2, 0])
+ }
+ """
+ return self.static_amin(
+ self,
+ axis=axis,
+ keepdims=keepdims,
+ key_chains=key_chains,
+ to_apply=to_apply,
+ prune_unapplied=prune_unapplied,
+ map_sequences=map_sequences,
+ out=out,
+ )
+
@staticmethod
def static_sinc(
x: ivy.Container,
@@ -2896,6 +3278,7 @@ def static_digamma(
-------
ret
container including the digamma function computed element-wise
+
Examples
--------
>>> x = ivy.Container(a=ivy.array([1, 0.5]),\
@@ -2958,6 +3341,7 @@ def digamma(
-------
ret
container including the digamma function computed element-wise
+
Examples
--------
>>> x = ivy.Container(a=ivy.array([1, 0.5]), b=ivy.array([2.0, 3.0])
@@ -3018,6 +3402,7 @@ def static_sparsify_tensor(
-------
ret
container including the sparsified tensor computed element-wise
+
Examples
--------
>>> x = ivy.Container(
diff --git a/ivy/data_classes/container/experimental/layers.py b/ivy/data_classes/container/experimental/layers.py
index d9f26cf0ce328..fc61b0caa19dd 100644
--- a/ivy/data_classes/container/experimental/layers.py
+++ b/ivy/data_classes/container/experimental/layers.py
@@ -971,7 +971,7 @@ def static_dct(
type
The type of the dct. Must be 1, 2, 3 or 4.
n
- The lenght of the transform. If n is less than the input signal lenght,
+ The length of the transform. If n is less than the input signal length,
then x is truncated, if n is larger than x is zero-padded.
norm
The type of normalization to be applied. Must be either None or "ortho".
@@ -1047,7 +1047,7 @@ def dct(
type
The type of the dct. Must be 1, 2, 3 or 4.
n
- The lenght of the transform. If n is less than the input signal lenght,
+ The length of the transform. If n is less than the input signal length,
then x is truncated, if n is larger then x is zero-padded.
norm
The type of normalization to be applied. Must be either None or "ortho".
@@ -2172,6 +2172,185 @@ def ifftn(
out=out,
)
+ @staticmethod
+ def static_rfft(
+ x: ivy.Container,
+ /,
+ *,
+ n: Optional[Union[int, ivy.Container]] = None,
+ axis: Union[int, ivy.Container] = -1,
+ norm: Union[
+ Literal["backward", "ortho", "forward"], ivy.Container
+ ] = "backward",
+ out: Optional[Union[ivy.Array, ivy.Container]] = None,
+ key_chains: Optional[Union[List[str], Dict[str, str], ivy.Container]] = None,
+ to_apply: Union[bool, ivy.Container] = True,
+ prune_unapplied: Union[bool, ivy.Container] = False,
+ map_sequences: Union[bool, ivy.Container] = False,
+ ) -> ivy.Container:
+ """
+ ivy.Container static method variant of ivy.rfft.
+
+ This method simply wraps the function, and so the docstring for
+ ivy.rfft also applies to this method with minimal changes.
+
+ Parameters
+ ----------
+ x
+ input array. Must have a real-valued floating-point data type.
+ n
+ length of the transformed axis of the input. If
+ - n is greater than the length of the input array, the input array
+ is zero-padded to length n.
+ - n is less than the length of the input array, the input array is
+ trimmed to length n.
+ - n is not provided, the length of the transformed axis of the
+ output must equal the length of the input along the axis specified
+ by axis. Default is ``None``.
+ axis
+ axis (dimension) over which to compute the Fourier transform.
+ If not set, the last axis (dimension) is used. Default is ``-1``.
+ norm
+ normalization mode. Should be one of the following modes:
+ - 'backward': no normalization.
+ - 'ortho': normalize by 1/sqrt(n) (i.e., make the FFT orthonormal).
+ - 'forward': normalize by 1/n.
+ Default is ``backward``.
+ out
+ Optional output array, for writing the result to. It must
+ have a shape that the inputs broadcast to.
+ key_chains
+ The key-chains to apply or not apply the method to.
+ Default is ``None``.
+ to_apply
+ If True, the method will be applied to key_chains,
+ otherwise key_chains will be skipped. Default is ``True``.
+ prune_unapplied
+ Whether to prune key_chains for which the function was
+ not applied. Default is ``False``.
+ map_sequences
+ Whether to also map method to sequences (lists, tuples).
+ Default is ``False``.
+
+ Returns
+ -------
+ ret
+ an array transformed along the axis (dimension) indicated by axis.
+ The returned array must have a complex-valued floating-point
+ data type determined by Type Promotion Rules.
+
+ Examples
+ --------
+ >>> x = ivy.Container(a=ivy.array([0.,1.,2.]),
+ ... b=ivy.array([3.,4.,5.]))
+ >>> y = ivy.Container.static_rfft(x)
+ >>> print(y)
+ {
+ a: ivy.array([3.+0.j, -1.5+0.8660254j]),
+ b: ivy.array([12.+0.j, -1.5+0.8660254j])
+ }
+ """
+ return ContainerBase.cont_multi_map_in_function(
+ "rfft",
+ x,
+ n=n,
+ axis=axis,
+ norm=norm,
+ out=out,
+ key_chains=key_chains,
+ to_apply=to_apply,
+ prune_unapplied=prune_unapplied,
+ map_sequences=map_sequences,
+ )
+
+ def rfft(
+ self: ivy.Container,
+ /,
+ *,
+ n: Optional[Union[int, ivy.Container]] = None,
+ axis: Union[int, ivy.Container] = -1,
+ norm: Union[
+ Literal["backward", "ortho", "forward"], ivy.Container
+ ] = "backward",
+ out: Optional[Union[ivy.Array, ivy.Container]] = None,
+ key_chains: Optional[Union[List[str], Dict[str, str], ivy.Container]] = None,
+ to_apply: Union[bool, ivy.Container] = True,
+ prune_unapplied: Union[bool, ivy.Container] = False,
+ map_sequences: Union[bool, ivy.Container] = False,
+ ):
+ """
+ ivy.Container instance method variant of ivy.rfft. This method simply wraps the
+ function, and so the docstring for ivy.rfft also applies to this method with
+ minimal changes.
+
+ Parameters
+ ----------
+ self
+ input array. Must have a real-valued floating-point data type.
+ n
+ length of the transformed axis of the input. If
+ - n is greater than the length of the input array, the input array
+ is zero-padded to length n.
+ - n is less than the length of the input array, the input array is
+ trimmed to length n.
+ - n is not provided, the length of the transformed axis of the
+ output must equal the length of the input along the axis specified
+ by axis. Default is ``None``.
+ axis
+ axis (dimension) over which to compute the Fourier transform.
+ If not set, the last axis (dimension) is used. Default is ``-1``.
+ norm
+ normalization mode. Should be one of the following modes:
+ - 'backward': no normalization.
+ - 'ortho': normalize by 1/sqrt(n) (i.e., make the FFT orthonormal).
+ - 'forward': normalize by 1/n.
+ Default is ``backward``.
+ out
+ Optional output array, for writing the result to. It must
+ have a shape that the inputs broadcast to.
+ key_chains
+ The key-chains to apply or not apply the method to.
+ Default is ``None``.
+ to_apply
+ If True, the method will be applied to key_chains,
+ otherwise key_chains will be skipped. Default is ``True``.
+ prune_unapplied
+ Whether to prune key_chains for which the function was
+ not applied. Default is ``False``.
+ map_sequences
+ Whether to also map method to sequences (lists, tuples).
+ Default is ``False``.
+
+ Returns
+ -------
+ ret
+ an array transformed along the axis (dimension) indicated by axis.
+ The returned array must have a complex-valued floating-point
+ data type determined by Type Promotion Rules.
+
+ Examples
+ --------
+ >>> x = ivy.Container(a=ivy.array([0.,1.,2.]),
+ ... b=ivy.array([3.,4.,5.]))
+ >>> y = x.rfft()
+ >>> print(y)
+ {
+ a: ivy.array([3.+0.j, -1.5+0.8660254j]),
+ b: ivy.array([12.+0.j, -1.5+0.8660254j])
+ }
+ """
+ return self.static_rfft(
+ self,
+ n=n,
+ axis=axis,
+ norm=norm,
+ out=out,
+ key_chains=key_chains,
+ to_apply=to_apply,
+ prune_unapplied=prune_unapplied,
+ map_sequences=map_sequences,
+ )
+
@staticmethod
def static_rfftn(
x: ivy.Container,
diff --git a/ivy/data_classes/container/experimental/linear_algebra.py b/ivy/data_classes/container/experimental/linear_algebra.py
index b39d5434e3402..b7f2d2ae67435 100644
--- a/ivy/data_classes/container/experimental/linear_algebra.py
+++ b/ivy/data_classes/container/experimental/linear_algebra.py
@@ -1296,6 +1296,86 @@ def make_svd_non_negative(
map_sequences=map_sequences,
)
+ @staticmethod
+ def static_tensor_train(
+ input_tensor: Union[ivy.Array, ivy.NativeArray, ivy.Container],
+ rank: Union[Sequence[int], ivy.Container],
+ /,
+ *,
+ svd: Optional[Union[Literal["truncated_svd"], ivy.Container]] = "truncated_svd",
+ verbose: Optional[Union[bool, ivy.Container]] = False,
+ key_chains: Optional[Union[List[str], Dict[str, str], ivy.Container]] = None,
+ to_apply: Union[bool, ivy.Container] = True,
+ prune_unapplied: Union[bool, ivy.Container] = False,
+ map_sequences: Union[bool, ivy.Container] = False,
+ ) -> Tuple[ivy.Container, Sequence[ivy.Container]]:
+ """
+ ivy.Container static method variant of ivy.tensor_train. This method simply
+ wraps the function, and so the docstring for ivy.tensor_train also applies to
+ this method with minimal changes.
+
+ Parameters
+ ----------
+ input_tensor
+ tensor to be decomposed.
+ rank
+ maximum allowable TT-ranks of the decomposition.
+ svd
+ SVD method to use.
+ verbose
+ level of verbosity.
+ """
+ return ContainerBase.cont_multi_map_in_function(
+ "tensor_train",
+ input_tensor,
+ rank,
+ svd=svd,
+ verbose=verbose,
+ key_chains=key_chains,
+ to_apply=to_apply,
+ prune_unapplied=prune_unapplied,
+ map_sequences=map_sequences,
+ )
+
+ def tensor_train(
+ self: Union[ivy.Array, ivy.NativeArray, ivy.Container],
+ rank: Union[Sequence[int], ivy.Container],
+ /,
+ *,
+ svd: Optional[Union[Literal["truncated_svd"], ivy.Container]] = "truncated_svd",
+ verbose: Optional[Union[bool, ivy.Container]] = False,
+ key_chains: Optional[Union[List[str], Dict[str, str], ivy.Container]] = None,
+ to_apply: Union[bool, ivy.Container] = True,
+ prune_unapplied: Union[bool, ivy.Container] = False,
+ map_sequences: Union[bool, ivy.Container] = False,
+ ) -> Tuple[ivy.Container, Sequence[ivy.Container]]:
+ """
+ ivy.Container instance method variant of ivy.tensor_train. This method simply
+ wraps the function, and so the docstring for ivy.tensor_train also applies to
+ this method with minimal changes.
+
+ Parameters
+ ----------
+ input_tensor
+ tensor to be decomposed.
+ rank
+ maximum allowable TT-ranks of the decomposition.
+ svd
+ SVD method to use.
+ verbose
+ level of verbosity.
+ """
+ return self.static_tensor_train(
+ self,
+ rank,
+ svd=svd,
+ verbose=verbose,
+ key_chains=key_chains,
+ to_apply=to_apply,
+ prune_unapplied=prune_unapplied,
+ map_sequences=map_sequences,
+ )
+
@staticmethod
def static_truncated_svd(
x: Union[ivy.Array, ivy.NativeArray, ivy.Container],
diff --git a/ivy/data_classes/container/experimental/manipulation.py b/ivy/data_classes/container/experimental/manipulation.py
index a71fcd08dce9a..0621ae4be3267 100644
--- a/ivy/data_classes/container/experimental/manipulation.py
+++ b/ivy/data_classes/container/experimental/manipulation.py
@@ -682,7 +682,7 @@ def static_top_k(
x
The container to compute top_k for.
k
- Number of top elements to retun must not exceed the array size.
+ Number of top elements to return must not exceed the array size.
axis
The axis along which we must return the top elements default value is 1.
largest
@@ -765,7 +765,7 @@ def top_k(
self
The container to compute top_k for.
k
- Number of top elements to retun must not exceed the array size.
+ Number of top elements to return must not exceed the array size.
axis
The axis along which we must return the top elements default value is 1.
largest
@@ -1652,7 +1652,7 @@ def static_atleast_1d(
-------
ret
container or list of container where each elements within container is
- atleast 1d. Copies are made only if necessary.
+ at least 1d. Copies are made only if necessary.
Examples
--------
@@ -1718,7 +1718,7 @@ def atleast_1d(
-------
ret
container or list of container where each elements within container is
- atleast 1d. Copies are made only if necessary.
+ at least 1d. Copies are made only if necessary.
Examples
--------
@@ -1874,7 +1874,7 @@ def static_atleast_2d(
-------
ret
container or list of container where each elements within container is
- atleast 2D. Copies are made only if necessary.
+ at least 2D. Copies are made only if necessary.
Examples
--------
@@ -1940,7 +1940,7 @@ def atleast_2d(
-------
ret
container or list of container where each elements within container is
- atleast 2D. Copies are made only if necessary.
+ at least 2D. Copies are made only if necessary.
Examples
--------
@@ -2010,7 +2010,7 @@ def static_atleast_3d(
-------
ret
container or list of container where each elements within container is
- atleast 3D. Copies are made only if necessary. For example, a 1-D array
+ at least 3D. Copies are made only if necessary. For example, a 1-D array
of shape (N,) becomes a view of shape (1, N, 1), and a 2-D array of shape
(M, N) becomes a view of shape (M, N, 1).
@@ -2074,7 +2074,7 @@ def atleast_3d(
-------
ret
container or list of container where each elements within container is
- atleast 3D. Copies are made only if necessary. For example, a 1-D array
+ at least 3D. Copies are made only if necessary. For example, a 1-D array
of shape (N,) becomes a view of shape (1, N, 1), and a 2-D array of shape
(M, N) becomes a view of shape (M, N, 1).
@@ -3859,3 +3859,370 @@ def put_along_axis(
map_sequences=map_sequences,
out=out,
)
+
+ @staticmethod
+ def _static_take(
+ x: Union[int, ivy.Array, ivy.NativeArray, ivy.Container],
+ indices: Union[int, ivy.Array, ivy.NativeArray, ivy.Container],
+ /,
+ *,
+ axis: Optional[Union[int, ivy.Container]] = None,
+ mode: Union[str, ivy.Container] = "fill",
+ fill_value: Optional[Union[Number, ivy.Container]] = None,
+ out: Optional[Union[ivy.Array, ivy.Container]] = None,
+ key_chains: Optional[Union[List[str], Dict[str, str], ivy.Container]] = None,
+ to_apply: Union[bool, ivy.Container] = True,
+ prune_unapplied: Union[bool, ivy.Container] = False,
+ map_sequences: Union[bool, ivy.Container] = False,
+ ) -> ivy.Container:
+ """
+ ivy.Container static method variant of ivy.take.
+
+ This method simply wraps the function, and so the docstring for
+ ivy.take also applies to this method with minimal changes.
+
+ Parameters
+ ----------
+ x
+ input array
+ indices
+ array indices. Must have an integer data type.
+ axis
+ axis over which to select values. If `axis` is negative,
+ the function must determine the axis along which to select values
+ by counting from the last dimension.
+ By default, the flattened input array is used.
+ mode
+ specifies how out-of-bounds `indices` will behave.
+ - βraiseβ β raise an error
+ - βwrapβ β wrap around
+ - βclipβ β clip to the range (all indices that are too large are
+ replaced by the index that addresses the last element along that axis.
+ Note that this disables indexing with negative numbers.)
+ - 'fill' (default) = returns invalid values (e.g. NaN)
+ for out-of bounds indices (see also fill_value below)
+ fill_value
+ fill value to return for out-of-bounds slices
+ (Defaults to NaN for inexact types,
+ the largest negative value for signed types,
+ the largest positive value for unsigned types, and True for booleans.)
+ out
+ optional output array, for writing the result to. It must
+ have a shape that the inputs broadcast to.
+ key_chains
+ The key-chains to apply or not apply the method to.
+ Default is ``None``.
+ to_apply
+ If True, the method will be applied to key_chains,
+ otherwise key_chains will be skipped. Default is ``True``.
+ prune_unapplied
+ Whether to prune key_chains for which the function was
+ not applied. Default is ``False``.
+ map_sequences
+ Whether to also map method to sequences (lists, tuples).
+ Default is ``False``.
+
+ Returns
+ -------
+ ret
+ an array having the same data type as `x`.
+ The output array must have the same rank
+ (i.e., number of dimensions) as `x` and
+ must have the same shape as `x`,
+ except for the axis specified by `axis`
+ whose size must equal the number of elements in `indices`.
+
+ Examples
+ --------
+ With `ivy.Container` input:
+
+ >>> x = ivy.Container(a=ivy.array([True,False,False]),
+ ... b=ivy.array([2.3,4.5,6.7]),
+ ... c=ivy.array([1,2,3]))
+ >>> indices = ivy.array([[1,9,2]])
+ >>> y = ivy.Container._static_take(x, indices)
+ >>> print(y)
+ {
+ a: ivy.array([[False, True, False]]),
+ b: ivy.array([[4.5, nan, 6.69999981]]),
+ c: ivy.array([[2, -2147483648, 3]])
+ }
+ """
+ return ContainerBase.cont_multi_map_in_function(
+ "take",
+ x,
+ indices,
+ axis=axis,
+ mode=mode,
+ fill_value=fill_value,
+ out=out,
+ key_chains=key_chains,
+ to_apply=to_apply,
+ prune_unapplied=prune_unapplied,
+ map_sequences=map_sequences,
+ )
+
+ def take(
+ self: Union[int, ivy.Array, ivy.NativeArray, ivy.Container],
+ indices: Union[int, ivy.Array, ivy.NativeArray, ivy.Container],
+ /,
+ *,
+ axis: Optional[Union[int, ivy.Container]] = None,
+ mode: Union[str, ivy.Container] = "fill",
+ fill_value: Optional[Union[Number, ivy.Container]] = None,
+ out: Optional[Union[ivy.Array, ivy.Container]] = None,
+ key_chains: Optional[Union[List[str], Dict[str, str], ivy.Container]] = None,
+ to_apply: Union[bool, ivy.Container] = True,
+ prune_unapplied: Union[bool, ivy.Container] = False,
+ map_sequences: Union[bool, ivy.Container] = False,
+ ) -> ivy.Container:
+ """
+ ivy.Container instance method variant of ivy.take.
+
+ This method simply wraps the function, and so the docstring for
+ ivy.take also applies to this method with minimal changes.
+
+ Parameters
+ ----------
+ self
+ input array
+ indices
+ array indices. Must have an integer data type.
+ axis
+ axis over which to select values. If `axis` is negative,
+ the function must determine the axis along which to select values
+ by counting from the last dimension.
+ By default, the flattened input array is used.
+ mode
+ specifies how out-of-bounds `indices` will behave.
+ - βraiseβ β raise an error
+ - βwrapβ β wrap around
+ - βclipβ β clip to the range (all indices that are too large are
+ replaced by the index that addresses the last element along that axis.
+ Note that this disables indexing with negative numbers.)
+ - 'fill' (default) = returns invalid values (e.g. NaN)
+ for out-of bounds indices (see also fill_value below)
+ fill_value
+ fill value to return for out-of-bounds slices
+ (Defaults to NaN for inexact types,
+ the largest negative value for signed types,
+ the largest positive value for unsigned types, and True for booleans.)
+ out
+ optional output array, for writing the result to. It must
+ have a shape that the inputs broadcast to.
+ key_chains
+ The key-chains to apply or not apply the method to.
+ Default is ``None``.
+ to_apply
+ If True, the method will be applied to key_chains,
+ otherwise key_chains will be skipped. Default is ``True``.
+ prune_unapplied
+ Whether to prune key_chains for which the function was
+ not applied. Default is ``False``.
+ map_sequences
+ Whether to also map method to sequences (lists, tuples).
+ Default is ``False``.
+
+ Returns
+ -------
+ ret
+ an array having the same data type as `x`.
+ The output array must have the same rank
+ (i.e., number of dimensions) as `x` and
+ must have the same shape as `x`,
+ except for the axis specified by `axis`
+ whose size must equal the number of elements in `indices`.
+
+ Examples
+ --------
+ With `ivy.Container` input:
+
+ >>> x = ivy.Container(a=ivy.array([True,False,False]),
+ ... b=ivy.array([2.3,4.5,6.7]),
+ ... c=ivy.array([1,2,3]))
+ >>> indices = ivy.array([[1,9,2]])
+ >>> y = x.take(indices)
+ >>> print(y)
+ {
+ a: ivy.array([[False, True, False]]),
+ b: ivy.array([[4.5, nan, 6.69999981]]),
+ c: ivy.array([[2, -2147483648, 3]])
+ }
+ """
+ return self._static_take(
+ self,
+ indices,
+ axis=axis,
+ mode=mode,
+ fill_value=fill_value,
+ out=out,
+ key_chains=key_chains,
+ to_apply=to_apply,
+ prune_unapplied=prune_unapplied,
+ map_sequences=map_sequences,
+ )
+
+ @staticmethod
+ def _static_trim_zeros(
+ a: Union[ivy.Array, ivy.NativeArray, ivy.Container],
+ /,
+ *,
+ trim: Optional[str] = "fb",
+ ) -> ivy.Container:
+ """
+ ivy.Container static method variant of ivy.trim_zeros. This method simply wraps
+ the function, and so the docstring for ivy.trim_zeros also applies to this
+ method with minimal changes.
+
+ Parameters
+ ----------
+ self : 1-D array
+ Input array.
+ trim : str, optional
+ A string with 'f' representing trim from front and 'b' to trim from
+ back. Default is 'fb', trim zeros from both front and back of the
+ array.
+
+ Returns
+ -------
+ 1-D array
+ The result of trimming the input. The input data type is preserved.
+
+ Examples
+ --------
+ >>> a = ivy.array([0, 0, 0, 0, 8, 3, 0, 0, 7, 1, 0])
+ >>> ivy.trim_zeros(a)
+ array([8, 3, 0, 0, 7, 1])
+ >>> ivy.trim_zeros(a, 'b')
+ array([0, 0, 0, 0, 8, 3, 0, 0, 7, 1])
+ >>> ivy.trim_zeros([0, 8, 3, 0, 0])
+ [8, 3]
+ """
+ return ContainerBase.cont_multi_map_in_function(a, trim)
+
+ def trim_zeros(
+ self: ivy.Container,
+ /,
+ *,
+ trim: Optional[str] = "fb",
+ ) -> ivy.Array:
+ """
+ ivy.Container instance method variant of ivy.trim_zeros. This method simply
+ wraps the function, and so the docstring for ivy.trim_zeros also applies to this
+ method with minimal changes.
+
+ Parameters
+ ----------
+ self : 1-D array
+ Input array.
+ trim : str, optional
+ A string with 'f' representing trim from front and 'b' to trim from
+ back. Default is 'fb', trim zeros from both front and back of the
+ array.
+
+ Returns
+ -------
+ 1-D array
+ The result of trimming the input. The input data type is preserved.
+
+ Examples
+ --------
+ >>> a = ivy.array([0, 0, 0, 0, 8, 3, 0, 0, 7, 1, 0])
+ >>> ivy.trim_zeros(a)
+ array([8, 3, 0, 0, 7, 1])
+ >>> ivy.trim_zeros(a, 'b')
+ array([0, 0, 0, 0, 8, 3, 0, 0, 7, 1])
+ >>> ivy.trim_zeros([0, 8, 3, 0, 0])
+ [8, 3]
+ """
+ return self._static_trim_zeros(self, trim=trim)
+
+
+def concat_from_sequence(
+ self: ivy.Container,
+ /,
+ input_sequence: Union[
+ Tuple[Union[ivy.Array, ivy.NativeArray, ivy.Container]],
+ List[Union[ivy.Array, ivy.NativeArray, ivy.Container]],
+ ],
+ *,
+ new_axis: Union[int, ivy.Container] = 0,
+ axis: Union[int, ivy.Container] = 0,
+ key_chains: Optional[Union[List[str], Dict[str, str], ivy.Container]] = None,
+ to_apply: Union[bool, ivy.Container] = True,
+ prune_unapplied: Union[bool, ivy.Container] = False,
+ map_sequences: Union[bool, ivy.Container] = False,
+ out: Optional[ivy.Container] = None,
+) -> ivy.Container:
+ """
+ ivy.Container instance method variant of ivy.stack. This method simply wraps the
+ function, and so the docstring for ivy.stack also applies to this method with
+ minimal changes.
+
+ Parameters
+ ----------
+ self
+ Container with leaves to join with leaves of other arrays/containers.
+ Each array leave must have the same shape.
+ input_sequence
+ Container with other leaves to join.
+ Each array leave must have the same shape.
+ new_axis
+ Insert and concatenate on a new axis or not,
+ default 0 means do not insert new axis.
+ new_axis = 0: concatenate
+ new_axis = 1: stack
+ axis
+ axis along which the array leaves will be concatenated. More details can be found in
+ the docstring for ivy.stack.
+ key_chains
+ The key-chains to apply or not apply the method to. Default is ``None``.
+ to_apply
+ If True, the method will be applied to key_chains, otherwise key_chains
+ will be skipped. Default is ``True``.
+ prune_unapplied
+ Whether to prune key_chains for which the function was not applied.
+ Default is ``False``.
+ map_sequences
+ Whether to also map method to sequences (lists, tuples).
+ Default is ``False``.
+ out
+ optional output array, for writing the result to. It must have a shape
+ that the inputs broadcast to.
+
+ Returns
+ -------
+ ret
+ an output container with the results.
+
+ Examples
+ --------
+ >>> x = ivy.Container(a=ivy.array([[0, 1], [2,3]]), b=ivy.array([[4, 5]]))
+ >>> y = ivy.Container(a=ivy.array([[3, 2], [1,0]]), b=ivy.array([[1, 0]]))
+ >>> z = ivy.Container.static_concat_from_sequence([x,y],axis=1)
+ >>> print(z)
+ {
+ 'a': ivy.array([[[0, 1],
+ [3, 2]],
+ [[2, 3],
+ [1, 0]]]),
+ 'b': ivy.array([[[4, 5],
+ [1, 0]]])
+ }
+ """
+ new_input_sequence = (
+ input_sequence.cont_copy()
+ if ivy.is_ivy_container(input_sequence)
+ else input_sequence.copy()
+ )
+ new_input_sequence.insert(0, self.cont_copy())
+ return self.concat_from_sequence(
+ new_input_sequence,
+ new_axis=new_axis,
+ axis=axis,
+ key_chains=key_chains,
+ to_apply=to_apply,
+ prune_unapplied=prune_unapplied,
+ map_sequences=map_sequences,
+ out=out,
+ )
diff --git a/ivy/data_classes/container/experimental/statistical.py b/ivy/data_classes/container/experimental/statistical.py
index edf20317bbdc9..d7fa55b44c924 100644
--- a/ivy/data_classes/container/experimental/statistical.py
+++ b/ivy/data_classes/container/experimental/statistical.py
@@ -444,6 +444,130 @@ def nanmean(
self, axis=axis, keepdims=keepdims, dtype=dtype, out=out
)
+ @staticmethod
+ def _static_nanmin(
+ x: ivy.Container,
+ /,
+ *,
+ axis: Optional[Union[Tuple[int], int, ivy.Container]] = None,
+ keepdims: Optional[Union[bool, ivy.Container]] = False,
+ key_chains: Optional[Union[List[str], Dict[str, str]]] = None,
+ to_apply: bool = True,
+ prune_unapplied: bool = False,
+ map_sequences: bool = False,
+ initial: Optional[Union[int, float, complex, ivy.Container]] = None,
+ where: Optional[Union[ivy.Array, ivy.Container]] = None,
+ out: Optional[ivy.Array] = None,
+ ) -> ivy.Container:
+ """
+ ivy.Container static method variant of ivy.nanmin. This method simply wraps the
+ function, and so the docstring for ivy.nanmin also applies to this method with
+ minimal changes.
+
+ Parameters
+ ----------
+ input
+ Input container including arrays.
+ axis
+ Axis or axes along which the minimum is computed.
+ The default is to compute the minimum of the flattened array.
+ out
+ optional output array, for writing the result to.
+ keepdims
+ If this is set to True, the axes which are reduced are left in the result
+ as dimensions with size one. With this option, the result will broadcast
+ correctly against the original a.
+ initial
+ The maximum value of an output element
+ where
+ Elements to compare for the minimum
+
+ Returns
+ -------
+ ret
+ Return minimum of an array or minimum along an axis, ignoring any NaNs.
+
+ Examples
+ --------
+ >>> a = ivy.Container(x=ivy.array([[1, 2], [3, ivy.nan]]),\
+ y=ivy.array([[ivy.nan, 1, 2], [1, 2, 3]])
+ >>> ivy.Container.static_nanmin(a)
+ {
+ x: 1.
+ y: 1.
+ }
+ """
+ return ContainerBase.cont_multi_map_in_function(
+ "nanmin",
+ x,
+ axis=axis,
+ keepdims=keepdims,
+ key_chains=key_chains,
+ to_apply=to_apply,
+ prune_unapplied=prune_unapplied,
+ map_sequences=map_sequences,
+ out=out,
+ initial=initial,
+ where=where,
+ )
+
+ def nanmin(
+ self: ivy.Container,
+ /,
+ *,
+ axis: Optional[Union[Tuple[int], int, ivy.Container]] = None,
+ keepdims: Optional[Union[bool, ivy.Container]] = False,
+ out: Optional[ivy.Container] = None,
+ initial: Optional[Union[int, float, complex, ivy.Container]] = None,
+ where: Optional[Union[ivy.Array, ivy.Container]] = None,
+ ) -> ivy.Container:
+ """
+ ivy.Container instance method variant of ivy.nanmin. This method simply wraps
+ the function, and so the docstring for ivy.nanmin also applies to this method
+ with minimal changes.
+
+ Parameters
+ ----------
+ self
+ Input container including arrays.
+ axis
+ Axis or axes along which the minimum is computed.
+ The default is to compute the minimum of the flattened array.
+ out
+ optional output array, for writing the result to.
+ keepdims
+ If this is set to True, the axes which are reduced are left in the result
+ as dimensions with size one. With this option, the result will broadcast
+ correctly against the original a.
+ initial
+ The maximum value of an output element.
+ where
+ Elements to compare for the minimum.
+
+ Returns
+ -------
+ ret
+ Return minimum of an array or minimum along an axis, ignoring any NaNs
+
+ Examples
+ --------
+ >>> a = ivy.Container(x=ivy.array([[1, 2], [3, ivy.nan]]),\
+ y=ivy.array([[ivy.nan, 1, 2], [1, 2, 3]])
+ >>> a.nanmin()
+ {
+ x: 12.0
+ y: 12.0
+ }
+ """
+ return self._static_nanmin(
+ self,
+ axis=axis,
+ keepdims=keepdims,
+ out=out,
+ initial=initial,
+ where=where,
+ )
+
@staticmethod
def static_nanprod(
input: ivy.Container,
diff --git a/ivy/data_classes/container/general.py b/ivy/data_classes/container/general.py
index 32c021edc72a4..4dff39400124c 100644
--- a/ivy/data_classes/container/general.py
+++ b/ivy/data_classes/container/general.py
@@ -1019,6 +1019,7 @@ def assert_supports_inplace(
ret
An ivy.Container instance of True bool values if nodes of the Container \
support in-place operations, raises IvyBackendException otherwise
+
Examples
--------
>>> ivy.set_backend("numpy")
@@ -4291,7 +4292,7 @@ def _static_exists(
Returns
-------
ret
- A boolean container detaling if any of the leaf nodes are None.
+ A boolean container detailing if any of the leaf nodes are None.
True if not None, False if None.
Examples
@@ -4353,7 +4354,7 @@ def exists(
Returns
-------
ret
- A boolean container detaling if any of the leaf nodes are None.
+ A boolean container detailing if any of the leaf nodes are None.
True if not None, False if None.
Examples
diff --git a/ivy/data_classes/container/layers.py b/ivy/data_classes/container/layers.py
index d071b6fa7be89..b2740bd33dbe2 100644
--- a/ivy/data_classes/container/layers.py
+++ b/ivy/data_classes/container/layers.py
@@ -807,7 +807,7 @@ def _static_scaled_dot_product_attention(
Default is None. The shape of mask input array leaves should be in
*[batch_shape,num_queries,num_keys]*.
dropout_p
- Specifies the dropout probablity, if greater than 0.0, dropout is applied
+ Specifies the dropout probability, if greater than 0.0, dropout is applied
is_causal
If true, assumes causal attention masking and errors if both `mask` and
`is_causal` are set.
@@ -930,7 +930,7 @@ def scaled_dot_product_attention(
Default is None. The shape of mask input array leaves should be in
*[batch_shape,num_queries,num_keys]*.
dropout_p
- Specifies the dropout probablity, if greater than 0.0, dropout is applied
+ Specifies the dropout probability, if greater than 0.0, dropout is applied
is_causal
If true, assumes causal attention masking and errors if both `mask` and
`is_causal` are set.
diff --git a/ivy/data_classes/container/linear_algebra.py b/ivy/data_classes/container/linear_algebra.py
index d9b7fa142b492..20a30dc92b22e 100644
--- a/ivy/data_classes/container/linear_algebra.py
+++ b/ivy/data_classes/container/linear_algebra.py
@@ -1,4 +1,5 @@
# global
+
from typing import Union, Optional, Tuple, Literal, List, Dict, Sequence
# local
@@ -2074,7 +2075,7 @@ def _static_qr(
prune_unapplied: Union[bool, ivy.Container] = False,
map_sequences: Union[bool, ivy.Container] = False,
out: Optional[Tuple[ivy.Container, ivy.Container]] = None,
- ) -> ivy.Container:
+ ) -> Tuple[ivy.Container, ivy.Container]:
"""
ivy.Container static method variant of ivy.qr. This method simply wraps the
function, and so the docstring for ivy.qr also applies to this method with
@@ -2128,6 +2129,26 @@ def _static_qr(
'reduced', the container must have shape (..., K, N), where K = min(M, N).
The first x.ndim-2 dimensions must have the same size as those of the input
x.
+
+ Examples
+ --------
+ >>> x = ivy.Container(a = ivy.native_array([[1., 2.], [3., 4.]]),
+ ... b = ivy.array([[2., 3.], [4. ,5.]]))
+ >>> q,r = ivy.Container.static_qr(x, mode='complete')
+ >>> print(q)
+ {
+ a: ivy.array([[-0.31622777, -0.9486833],
+ [-0.9486833, 0.31622777]]),
+ b: ivy.array([[-0.4472136, -0.89442719],
+ [-0.89442719, 0.4472136]])
+ }
+ >>> print(r)
+ {
+ a: ivy.array([[-3.16227766, -4.42718872],
+ [0., -0.63245553]]),
+ b: ivy.array([[-4.47213595, -5.81377674],
+ [0., -0.4472136]])
+ }
"""
return ContainerBase.cont_multi_map_in_function(
"qr",
@@ -2204,6 +2225,26 @@ def qr(
'reduced', the container must have shape (..., K, N), where K = min(M, N).
The first x.ndim-2 dimensions must have the same size as those of the input
x.
+
+ Examples
+ --------
+ >>> x = ivy.Container(a = ivy.native_array([[1., 2.], [3., 4.]]),
+ ... b = ivy.array([[2., 3.], [4. ,5.]]))
+ >>> q,r = x.qr(mode='complete')
+ >>> print(q)
+ {
+ a: ivy.array([[-0.31622777, -0.9486833],
+ [-0.9486833, 0.31622777]]),
+ b: ivy.array([[-0.4472136, -0.89442719],
+ [-0.89442719, 0.4472136]])
+ }
+ >>> print(r)
+ {
+ a: ivy.array([[-3.16227766, -4.42718872],
+ [0., -0.63245553]]),
+ b: ivy.array([[-4.47213595, -5.81377674],
+ [0., -0.4472136]])
+ }
"""
return self._static_qr(
self,
@@ -2720,6 +2761,14 @@ def _static_trace(
offset
Offset of the diagonal from the main diagonal. Can be both positive and
negative. Defaults to 0.
+ axis1
+ axis to be used as the first axis of the 2-D sub-arrays from which the
+ diagonals should be taken.
+ Defaults to ``0.`` .
+ axis2
+ axis to be used as the second axis of the 2-D sub-arrays from which the
+ diagonals should be taken.
+ Defaults to ``1.`` .
key_chains
The key-chains to apply or not apply the method to. Default is ``None``.
to_apply
@@ -2805,6 +2854,14 @@ def trace(
offset
Offset of the diagonal from the main diagonal. Can be both positive and
negative. Defaults to 0.
+ axis1
+ axis to be used as the first axis of the 2-D sub-arrays from which the
+ diagonals should be taken.
+ Defaults to ``0.`` .
+ axis2
+ axis to be used as the second axis of the 2-D sub-arrays from which the
+ diagonals should be taken.
+ Defaults to ``1.`` .
key_chains
The key-chains to apply or not apply the method to. Default is ``None``.
to_apply
diff --git a/ivy/data_classes/container/losses.py b/ivy/data_classes/container/losses.py
index fb91996775907..4f3b65b47a39f 100644
--- a/ivy/data_classes/container/losses.py
+++ b/ivy/data_classes/container/losses.py
@@ -15,7 +15,7 @@ def _static_cross_entropy(
*,
axis: Union[int, ivy.Container] = -1,
epsilon: Union[float, ivy.Container] = 1e-7,
- reduction: Union[str, ivy.Container] = "sum",
+ reduction: Union[str, ivy.Container] = "mean",
key_chains: Optional[Union[List[str], Dict[str, str], ivy.Container]] = None,
to_apply: Union[bool, ivy.Container] = True,
prune_unapplied: Union[bool, ivy.Container] = False,
@@ -106,7 +106,7 @@ def cross_entropy(
*,
axis: Union[int, ivy.Container] = -1,
epsilon: Union[float, ivy.Container] = 1e-7,
- reduction: Union[str, ivy.Container] = "sum",
+ reduction: Union[str, ivy.Container] = "mean",
key_chains: Optional[Union[List[str], Dict[str, str], ivy.Container]] = None,
to_apply: Union[bool, ivy.Container] = True,
prune_unapplied: Union[bool, ivy.Container] = False,
@@ -184,7 +184,7 @@ def _static_binary_cross_entropy(
*,
from_logits: Union[bool, ivy.Container] = False,
epsilon: Union[float, ivy.Container] = 0.0,
- reduction: Union[str, ivy.Container] = "none",
+ reduction: Union[str, ivy.Container] = "mean",
pos_weight: Optional[Union[ivy.Container, ivy.Array, ivy.NativeArray]] = None,
axis: Optional[Union[int, ivy.Container]] = None,
key_chains: Optional[Union[List[str], Dict[str, str], ivy.Container]] = None,
@@ -286,7 +286,7 @@ def binary_cross_entropy(
*,
from_logits: Union[bool, ivy.Container] = False,
epsilon: Union[float, ivy.Container] = 0.0,
- reduction: Union[str, ivy.Container] = "none",
+ reduction: Union[str, ivy.Container] = "mean",
pos_weight: Optional[Union[ivy.Container, ivy.Array, ivy.NativeArray]] = None,
axis: Optional[Union[int, ivy.Container]] = None,
key_chains: Optional[Union[List[str], Dict[str, str], ivy.Container]] = None,
@@ -377,7 +377,7 @@ def _static_sparse_cross_entropy(
*,
axis: Union[int, ivy.Container] = -1,
epsilon: Union[float, ivy.Container] = 1e-7,
- reduction: Union[str, ivy.Container] = "sum",
+ reduction: Union[str, ivy.Container] = "mean",
key_chains: Optional[Union[List[str], Dict[str, str], ivy.Container]] = None,
to_apply: Union[bool, ivy.Container] = True,
prune_unapplied: Union[bool, ivy.Container] = False,
@@ -467,7 +467,7 @@ def sparse_cross_entropy(
*,
axis: Union[int, ivy.Container] = -1,
epsilon: Union[float, ivy.Container] = 1e-7,
- reduction: Union[str, ivy.Container] = "sum",
+ reduction: Union[str, ivy.Container] = "mean",
key_chains: Optional[Union[List[str], Dict[str, str], ivy.Container]] = None,
to_apply: Union[bool, ivy.Container] = True,
prune_unapplied: Union[bool, ivy.Container] = False,
diff --git a/ivy/data_classes/container/searching.py b/ivy/data_classes/container/searching.py
index 8e6dd9e269c28..c007d1f67cc8e 100644
--- a/ivy/data_classes/container/searching.py
+++ b/ivy/data_classes/container/searching.py
@@ -31,7 +31,7 @@ def _static_argmax(
input array or container. Should have a numeric data type.
axis
axis along which to search. If None, the function must return the index of
- the maximum value of the flattened array. Deafult: ``None``.
+ the maximum value of the flattened array. Default: ``None``.
keepdims
If this is set to True, the axes which are reduced are left in the result as
dimensions with size one. With this option, the result will broadcast
@@ -92,7 +92,7 @@ def argmax(
input array or container. Should have a numeric data type.
axis
axis along which to search. If None, the function must return the index of
- the maximum value of the flattened array. Deafult: ``None``.
+ the maximum value of the flattened array. Default: ``None``.
keepdims
If this is set to True, the axes which are reduced are left in the result as
dimensions with size one. With this option, the result will broadcast
diff --git a/ivy/data_classes/container/statistical.py b/ivy/data_classes/container/statistical.py
index 39b39e7dbd2fe..2d527a7a46b09 100644
--- a/ivy/data_classes/container/statistical.py
+++ b/ivy/data_classes/container/statistical.py
@@ -371,7 +371,7 @@ def var(
Returns
-------
ret
- a container contianing different arrays depends on parameters. see below
+ a container containing different arrays depends on parameters. see below
for the types of arrays in the returned container if the variance was
computed over the entire array, a zero-dimensional array containing the
variance; otherwise, a non-zero-dimensional array containing the variances.
diff --git a/ivy/data_classes/factorized_tensor/cp_tensor.py b/ivy/data_classes/factorized_tensor/cp_tensor.py
index 57b93a4fff3a3..72d72d7242641 100644
--- a/ivy/data_classes/factorized_tensor/cp_tensor.py
+++ b/ivy/data_classes/factorized_tensor/cp_tensor.py
@@ -735,7 +735,7 @@ def cp_norm(cp_tensor):
# -------
# permuted_tensors : permuted cp tensor or list of cp tensors
# permutation : list
- # list of permuted indices. Lenght is equal to rank of cp_tensors.
+ # list of permuted indices. Length is equal to rank of cp_tensors.
# """
# if not isinstance(tensors_to_permute, list):
# permuted_tensors = [tensors_to_permute.cp_copy()]
diff --git a/ivy/data_classes/factorized_tensor/parafac2_tensor.py b/ivy/data_classes/factorized_tensor/parafac2_tensor.py
index 466b98220055c..c2a211ee5924f 100644
--- a/ivy/data_classes/factorized_tensor/parafac2_tensor.py
+++ b/ivy/data_classes/factorized_tensor/parafac2_tensor.py
@@ -104,7 +104,7 @@ def from_CPTensor(cls, cp_tensor, parafac2_tensor_ok=False):
Returns
-------
- Parafac2Tensor with factor matrices and weigths extracted from a CPTensor
+ Parafac2Tensor with factor matrices and weights extracted from a CPTensor
"""
if parafac2_tensor_ok and len(cp_tensor) == 3:
return Parafac2Tensor(cp_tensor)
diff --git a/ivy/data_classes/factorized_tensor/tr_tensor.py b/ivy/data_classes/factorized_tensor/tr_tensor.py
index ac7f76f27b4e8..8670d46963c9c 100644
--- a/ivy/data_classes/factorized_tensor/tr_tensor.py
+++ b/ivy/data_classes/factorized_tensor/tr_tensor.py
@@ -75,7 +75,7 @@ def validate_tr_tensor(factors):
current_rank, current_shape, next_rank = ivy.shape(factor)
# Check that factors are third order tensors
- if not len(factor.shape) == 3:
+ if len(factor.shape) != 3:
raise ValueError(
"TR expresses a tensor as third order factors (tr-cores).\n"
f"However, ivy.ndim(factors[{index}]) = {len(factor.shape)}"
diff --git a/ivy/data_classes/factorized_tensor/tt_tensor.py b/ivy/data_classes/factorized_tensor/tt_tensor.py
index 03f2bf7602971..176f3b1fb5b23 100644
--- a/ivy/data_classes/factorized_tensor/tt_tensor.py
+++ b/ivy/data_classes/factorized_tensor/tt_tensor.py
@@ -69,7 +69,7 @@ def validate_tt_tensor(tt_tensor):
for index, factor in enumerate(factors):
current_rank, current_shape, next_rank = ivy.shape(factor)
- if not len(ivy.shape(factor)) == 3:
+ if len(ivy.shape(factor)) != 3:
raise ValueError(
"TT expresses a tensor as third order factors"
f" (tt-cores).\nHowever, len(ivy.shape(factors[{index}])) ="
@@ -139,7 +139,7 @@ def tt_to_tensor(factors):
@staticmethod
def tt_to_unfolded(factors, mode):
"""
- Return the unfolding matrix of a tensor given in TT (or Tensor-Train) format.
+ Return the unfolding matrix of a tensor given in TT (or Tensor- Train) format.
Reassembles a full tensor from 'factors' and returns its unfolding matrix
with mode given by 'mode'
@@ -291,9 +291,7 @@ def validate_tt_rank(
delta = ivy.sqrt(b**2 - 4 * a * c)
fraction_param = (-b + delta) / (2 * a)
- rank = tuple(
- [max(int(rounding_fn(d * fraction_param)), 1) for d in avg_dim]
- )
+ rank = tuple(max(int(rounding_fn(d * fraction_param)), 1) for d in avg_dim)
rank = (1,) + rank + (1,)
else:
@@ -310,14 +308,14 @@ def validate_tt_rank(
if rank[0] != 1:
message = (
- "Provided rank[0] == {} but boundary conditions dictate rank[0] =="
- " rank[-1] == 1.".format(rank[0])
+ f"Provided rank[0] == {rank[0]} but boundary conditions dictate"
+ " rank[0] == rank[-1] == 1."
)
raise ValueError(message)
if rank[-1] != 1:
message = (
- "Provided rank[-1] == {} but boundary conditions dictate rank[0] =="
- " rank[-1] == 1.".format(rank[-1])
+ f"Provided rank[-1] == {rank[-1]} but boundary conditions dictate"
+ " rank[0] == rank[-1] == 1."
)
raise ValueError(message)
diff --git a/ivy/data_classes/nested_array/base.py b/ivy/data_classes/nested_array/base.py
index f76d78097490f..188f1c731cfa2 100644
--- a/ivy/data_classes/nested_array/base.py
+++ b/ivy/data_classes/nested_array/base.py
@@ -32,7 +32,7 @@ def nested_array(
device = ivy.default_device(device, item=data)
# convert all the leaf lists to ivy arrays, determine inner_shape and depth
- det_inner_shape = list()
+ det_inner_shape = []
# ToDo: add check for depth being the same for all nests
def _seq_to_ivy(x, depth=0):
@@ -42,7 +42,7 @@ def _seq_to_ivy(x, depth=0):
if x.ndim > 1:
det_inner_shape.append(list(x.shape[1:]))
else:
- det_inner_shape.append(list())
+ det_inner_shape.append([])
elif (
isinstance(x, (list, tuple))
and len(x) != 0
@@ -59,7 +59,7 @@ def _seq_to_ivy(x, depth=0):
if x.ndim > 1:
det_inner_shape.append(list(x.shape[1:]))
else:
- det_inner_shape.append(list())
+ det_inner_shape.append([])
return x, depth
if isinstance(data, (list, tuple)):
@@ -70,7 +70,7 @@ def _seq_to_ivy(x, depth=0):
if [det_inner_shape[0]] * len(det_inner_shape) != det_inner_shape:
raise ValueError(
"All the elements of the nested array must have the same "
- "inner shape, got: {}".format(det_inner_shape)
+ f"inner shape, got: {det_inner_shape}"
)
det_inner_shape = det_inner_shape[0]
@@ -80,7 +80,7 @@ def _seq_to_ivy(x, depth=0):
if inner_shape is None
else max(0, depth - 1 - len(inner_shape))
)
- default_inner_shape = list() if nested_rank is None else det_inner_shape
+ default_inner_shape = [] if nested_rank is None else det_inner_shape
# determining actual values for nested_rank and inner_shape
nested_rank = (
@@ -134,10 +134,9 @@ def map_fn(vals):
@staticmethod
def ragged_multi_map(fn, ragged_arrays):
- args = list()
+ args = []
for ragged in ragged_arrays:
args.append(ivy.copy_nest(ragged.data))
- ragged_arrays[0]
ret = ivy.nested_multi_map(lambda x, _: fn(x), args)
# infer dtype, shape, and device from the first array in the ret data
broadcasted_shape = ivy.NestedArray.broadcast_shapes(
@@ -170,7 +169,7 @@ def replace_ivy_arrays(ragged_array, arrays):
@staticmethod
def broadcast_shapes(shapes):
z = []
- max_length = max([len(x) for x in shapes])
+ max_length = max(len(x) for x in shapes)
shape_list = list(shapes)
# making every shape the same length
for i, shape in enumerate(shapes):
@@ -192,20 +191,19 @@ def broadcast_shapes(shapes):
z.append(dims)
if dim_exist:
raise ValueError(
- "Shapes {} and {} are not broadcastable".format(
- shapes[0], shapes[1]
- )
+ f"Shapes {shapes[0]} and {shapes[1]} are not"
+ " broadcastable"
)
- dim_exist = True
+ else:
+ dim_exist = True
if not dim_exist:
z.append(1)
+ elif len(set(x)) == 1:
+ z.append(x[0])
else:
- if len(set(x)) == 1:
- z.append(x[0])
- else:
- raise ValueError(
- f"Shapes {shapes[0]} and {shapes[1]} are not broadcastable"
- )
+ raise ValueError(
+ f"Shapes {shapes[0]} and {shapes[1]} are not broadcastable"
+ )
return z
def ragged_map(self, fn):
diff --git a/ivy/data_classes/nested_array/nested_array.py b/ivy/data_classes/nested_array/nested_array.py
index 2bfe8120c8a8b..52105ac1c29ed 100644
--- a/ivy/data_classes/nested_array/nested_array.py
+++ b/ivy/data_classes/nested_array/nested_array.py
@@ -10,7 +10,7 @@ def __init__(self, data, nested_rank, inner_shape, dtype, device, internal=False
@classmethod
def from_row_lengths(cls, values, row_lengths):
- ivy_arrays = list()
+ ivy_arrays = []
for i in range(len(row_lengths)):
ivy_arrays.append(values[: row_lengths[i]])
values = values[row_lengths[i] :]
@@ -18,7 +18,7 @@ def from_row_lengths(cls, values, row_lengths):
@classmethod
def from_row_splits(cls, values, row_splits):
- row_lengths = list()
+ row_lengths = []
for i in range(1, len(row_splits)):
row_lengths.append(row_splits[i] - row_splits[i - 1])
return cls.from_row_lengths(values, row_lengths)
diff --git a/ivy/engines/XLA/__init__.py b/ivy/engines/XLA/__init__.py
index 45a9584091f50..f5b736bcb5bec 100644
--- a/ivy/engines/XLA/__init__.py
+++ b/ivy/engines/XLA/__init__.py
@@ -7,7 +7,6 @@
# from .rust_api.python_frontend.sequential_handler import *
from .rust_api.python_frontend.general import *
-from .rust_api.python_frontend.manipulation import *
from .rust_api.python_frontend.creation import *
from .rust_api.python_frontend.linear_algebra import *
from .rust_api.python_frontend.elementwise import *
diff --git a/ivy/func_wrapper.py b/ivy/func_wrapper.py
index 6aa3369a35fb0..d13bdf675606e 100644
--- a/ivy/func_wrapper.py
+++ b/ivy/func_wrapper.py
@@ -33,6 +33,7 @@
"handle_nestable",
"handle_ragged",
"handle_backend_invalid",
+ "temp_asarray_wrapper",
"handle_exceptions",
"handle_nans",
]
@@ -187,10 +188,10 @@ def cross_caster(intersect):
valid_float = sorted(ivy.valid_float_dtypes)
valid_int = sorted(ivy.valid_int_dtypes)
intersect = sorted(intersect)
- if intersect == valid_int:
+ if set(valid_int).issubset(intersect):
# make dtype equal to default float
dtype = ivy.default_float_dtype()
- elif intersect == valid_float:
+ elif set(valid_float).issubset(intersect):
# make dtype equal to default int
dtype = ivy.default_int_dtype()
@@ -203,7 +204,7 @@ def try_array_function_override(func, overloaded_args, types, args, kwargs):
for overloaded_arg in overloaded_args:
# Note that we're only calling __ivy_array_function__ on the *first*
- # occurence of each argument type. This is necessary for reasonable
+ # occurrence of each argument type. This is necessary for reasonable
# performance with a possibly long list of overloaded arguments, for
# which each __ivy_array_function__ implementation might reasonably need to
# check all argument types.
@@ -817,7 +818,7 @@ def _handle_device(*args, **kwargs):
elif len(unique_devices) > 1:
raise ivy.utils.exceptions.IvyException(
"Expected all input arrays to be on the same device, "
- f"but found atleast two devices - {devices}, "
+ f"but found at least two devices - {devices}, "
"set `ivy.set_soft_device_mode(True)` to handle this problem."
)
return fn(*args, **kwargs)
@@ -1028,6 +1029,40 @@ def _handle_partial_mixed_function(*args, **kwargs):
return _handle_partial_mixed_function
+# Temporary asarray wrapper (Please request my review before removing)
+
+
+def temp_asarray_wrapper(fn: Callable) -> Callable:
+ @functools.wraps(fn)
+ def _temp_asarray_wrapper(*args, **kwargs):
+ """
+ Convert `Tensor` into `ivy.Array` instances.
+
+ Convert all `Tensor` instances in both the positional and keyword arguments
+ into `ivy.Array` instances, and then call the function with the updated
+ arguments.
+ """
+
+ def _to_ivy_array(x):
+ # if x is a frontend torch Tensor (or any frontend "Tensor" actually) return the wrapped ivy array # noqa: E501
+ if hasattr(x, "ivy_array"):
+ return x.ivy_array
+ # else just return x
+ return x
+
+ # convert all input arrays to ivy.Array instances
+ new_args = ivy.nested_map(
+ _to_ivy_array, args, include_derived={"tuple": True}, shallow=False
+ )
+ new_kwargs = ivy.nested_map(
+ _to_ivy_array, kwargs, include_derived={"tuple": True}, shallow=False
+ )
+ return fn(*new_args, **new_kwargs)
+
+ _temp_asarray_wrapper.temp_asarray_wrapper = True
+ return _temp_asarray_wrapper
+
+
# Functions #
@@ -1125,9 +1160,13 @@ def _wrap_function(
return to_wrap
-def casting_modes_ops(fn):
+def casting_modes_ops(fn, ret_dtype_target=None):
@functools.wraps(fn)
def method(*args, **kwargs):
+ # Get the function signature
+ signature = inspect.signature(fn)
+ # Extract argument names
+ arg_names = [param.name for param in signature.parameters.values()]
# we first check if it has unsupported/supported dtypes uniquely added to it
intersect = set(ivy.function_unsupported_dtypes(fn)).difference(
set(ivy.invalid_dtypes)
@@ -1144,7 +1183,10 @@ def method(*args, **kwargs):
# no unsupported dtype specified
return fn(*args, **kwargs)
+ # specifies which dtype to cast the output to
+ to_cast = None
if "dtype" in kwargs and kwargs["dtype"] is not None:
+ to_cast = kwargs["dtype"]
dtype = caster(kwargs["dtype"], intersect)
if dtype:
kwargs["dtype"] = ivy.as_native_dtype(dtype)
@@ -1159,7 +1201,36 @@ def mini_helper(x):
args = ivy.nested_map(mini_helper, args, include_derived=True)
kwargs = ivy.nested_map(mini_helper, kwargs)
- return fn(*args, **kwargs)
+
+ if not to_cast and ret_dtype_target:
+ for arg in ret_dtype_target:
+ if arg:
+ to_cast, arg_mod = ivy.promote_types_of_inputs(
+ to_cast,
+ (
+ args[arg_names.index(arg)]
+ if arg not in kwargs
+ else kwargs[arg]
+ ),
+ )
+ if arg not in kwargs:
+ args[arg_names.index(arg)] = (
+ arg_mod
+ if not ivy.is_array(args[arg_names.index(arg)])
+ else args[arg_names.index(arg)]
+ )
+ else:
+ kwargs[arg] = (
+ arg_mod
+ if not ivy.is_array(args[arg_names.index(arg)])
+ else kwargs[arg]
+ )
+
+ return (
+ ivy.astype(fn(*args, **kwargs), ivy.to_native(to_cast))
+ if to_cast
+ else fn(*args, **kwargs)
+ )
return method
@@ -1217,7 +1288,7 @@ def __init__(self):
self.attribute_function = attribute_function
def __get__(self, instance=None, owner=None):
- # version dtypes recalculated everytime it's accessed
+ # version dtypes recalculated every time it's accessed
return self.attribute_function()
def __iter__(self):
@@ -1227,6 +1298,9 @@ def __iter__(self):
def __repr__(self):
return repr(self.__get__())
+ def __bool__(self):
+ return bool(self.__get__())
+
return VersionedAttributes()
@@ -1249,7 +1323,7 @@ def _dtype_device_wrapper_creator(attrib, t):
A wrapper function for the attribute.
"""
- def _wrapper_outer(version_dict, version, exclusive=True):
+ def _wrapper_outer(version_dict, version, exclusive=True, ret_dtype_target=None):
def _wrapped(func):
val = _versioned_attribute_factory(
lambda: _dtype_from_version(version_dict, version), t
@@ -1259,7 +1333,7 @@ def _wrapped(func):
return func
if not exclusive:
# exclusive attribute comes into existence
- # only when exlusive is passed as true
+ # only when exclusive is passed as true
setattr(func, "exclusive", True)
# set the attribute on the function and return the function as is
@@ -1295,12 +1369,16 @@ def _wrapped(func):
# for conflicting ones we do nothing
pass
else:
- setattr(func, attrib, val)
+ if not val and attrib.startswith("supported"):
+ setattr(func, f"un{attrib}", val)
+ else:
+ setattr(func, attrib, val)
setattr(func, "dictionary_info", (version_dict, version))
if "frontends" in func.__module__:
# it's a frontend func, no casting modes for this
return func
- return casting_modes_ops(func)
+
+ return casting_modes_ops(func, ret_dtype_target=ret_dtype_target)
return _wrapped
@@ -1316,7 +1394,7 @@ def _leaf_has_nans(x):
return x.has_nans()
elif ivy.is_array(x):
return ivy.isnan(x).any()
- elif x == float("nan"):
+ elif np.isnan(x):
return True
return False
@@ -1355,7 +1433,7 @@ def _handle_nans(*args, **kwargs):
if nan_policy == "nothing":
return fn(*args, **kwargs)
- # check all args and kwards for presence of nans
+ # check all args and kwargs for presence of nans
result = _nest_has_nans(args) or _nest_has_nans(kwargs)
if result:
@@ -1757,9 +1835,7 @@ def __init__(self, *args, **kwargs):
dicti[key]["all"]
)
else:
- nested_dic[nested_key] = dicti[key].get(nested_key, ()) + tuple(
- dicti[key][nested_key]
- )
+ nested_dic[nested_key] = tuple(dicti[key][nested_key])
dicti[key] = nested_dic
args = (dicti, args[1])
@@ -1817,9 +1893,7 @@ def __init__(self, *args, **kwargs):
dicti[key]["all"]
)
else:
- nested_dic[nested_key] = dicti[key].get(nested_key, ()) + tuple(
- dicti[key][nested_key]
- )
+ nested_dic[nested_key] = tuple(dicti[key][nested_key])
dicti[key] = nested_dic
args = (dicti, args[1])
diff --git a/ivy/functional/backends/jax/__init__.py b/ivy/functional/backends/jax/__init__.py
index 04f554eb9d583..74d697ec9be9c 100644
--- a/ivy/functional/backends/jax/__init__.py
+++ b/ivy/functional/backends/jax/__init__.py
@@ -16,11 +16,15 @@
backend_version = {"version": jax.__version__}
-register_pytree_node(
- ivy.Container,
- lambda c: tree_flatten(c.cont_to_dict()),
- lambda a, c: ivy.Container(tree_unflatten(a, c)),
-)
+try:
+ register_pytree_node(
+ ivy.Container,
+ lambda c: tree_flatten(c.cont_to_dict()),
+ lambda a, c: ivy.Container(tree_unflatten(a, c)),
+ )
+except Exception as e:
+ if "Duplicate custom PyTreeDef type registration" not in str(e):
+ raise
# make ivy.Array compatible with jax pytree traversal
@@ -34,7 +38,12 @@ def _array_unflatten(aux_data, children):
return ivy.Array(*children)
-register_pytree_node(ivy.Array, _array_flatten, _array_unflatten)
+try:
+ register_pytree_node(ivy.Array, _array_flatten, _array_unflatten)
+except Exception as e:
+ if "Duplicate custom PyTreeDef type registration" not in str(e):
+ raise
+
# noinspection PyUnresolvedReferences
if not ivy.is_local():
@@ -92,7 +101,7 @@ def _array_unflatten(aux_data, children):
# update these to add new dtypes
valid_dtypes = {
- "0.4.16 and below": (
+ "0.4.19 and below": (
ivy.int8,
ivy.int16,
ivy.int32,
@@ -111,7 +120,7 @@ def _array_unflatten(aux_data, children):
)
}
valid_numeric_dtypes = {
- "0.4.16 and below": (
+ "0.4.19 and below": (
ivy.int8,
ivy.int16,
ivy.int32,
@@ -130,7 +139,7 @@ def _array_unflatten(aux_data, children):
}
valid_int_dtypes = {
- "0.4.16 and below": (
+ "0.4.19 and below": (
ivy.int8,
ivy.int16,
ivy.int32,
@@ -143,12 +152,12 @@ def _array_unflatten(aux_data, children):
}
valid_uint_dtypes = {
- "0.4.16 and below": (ivy.uint8, ivy.uint16, ivy.uint32, ivy.uint64)
+ "0.4.19 and below": (ivy.uint8, ivy.uint16, ivy.uint32, ivy.uint64)
}
valid_float_dtypes = {
- "0.4.16 and below": (ivy.bfloat16, ivy.float16, ivy.float32, ivy.float64)
+ "0.4.19 and below": (ivy.bfloat16, ivy.float16, ivy.float32, ivy.float64)
}
-valid_complex_dtypes = {"0.4.16 and below": (ivy.complex64, ivy.complex128)}
+valid_complex_dtypes = {"0.4.19 and below": (ivy.complex64, ivy.complex128)}
# leave these untouched
@@ -163,12 +172,12 @@ def _array_unflatten(aux_data, children):
# invalid data types
# update these to add new dtypes
-invalid_dtypes = {"0.4.16 and below": ()}
-invalid_numeric_dtypes = {"0.4.16 and below": ()}
-invalid_int_dtypes = {"0.4.16 and below": ()}
-invalid_float_dtypes = {"0.4.16 and below": ()}
-invalid_uint_dtypes = {"0.4.16 and below": ()}
-invalid_complex_dtypes = {"0.4.16 and below": ()}
+invalid_dtypes = {"0.4.19 and below": ()}
+invalid_numeric_dtypes = {"0.4.19 and below": ()}
+invalid_int_dtypes = {"0.4.19 and below": ()}
+invalid_float_dtypes = {"0.4.19 and below": ()}
+invalid_uint_dtypes = {"0.4.19 and below": ()}
+invalid_complex_dtypes = {"0.4.19 and below": ()}
# leave these untouched
invalid_dtypes = _dtype_from_version(invalid_dtypes, backend_version)
diff --git a/ivy/functional/backends/jax/activations.py b/ivy/functional/backends/jax/activations.py
index 2dc0643665959..1120af0466f64 100644
--- a/ivy/functional/backends/jax/activations.py
+++ b/ivy/functional/backends/jax/activations.py
@@ -1,6 +1,5 @@
"""Collection of Jax activation functions, wrapped to fit Ivy syntax and signature."""
-
# global
diff --git a/ivy/functional/backends/jax/creation.py b/ivy/functional/backends/jax/creation.py
index ee3804bab9759..17ade3b74b32e 100644
--- a/ivy/functional/backends/jax/creation.py
+++ b/ivy/functional/backends/jax/creation.py
@@ -12,6 +12,7 @@
import ivy
from ivy import as_native_dtype
from ivy.functional.backends.jax import JaxArray
+from ivy.functional.backends.jax.device import dev
from ivy.functional.ivy.creation import (
_asarray_to_native_arrays_and_back,
_asarray_infer_device,
@@ -73,10 +74,13 @@ def asarray(
out: Optional[JaxArray] = None,
) -> JaxArray:
ivy.utils.assertions._check_jax_x64_flag(dtype)
- if copy is True:
- return jnp.array(obj, dtype=dtype, copy=True)
- else:
- return jnp.asarray(obj, dtype=dtype)
+ ret = jnp.asarray(obj, dtype=dtype)
+ # jnp.copy is used to ensure correct device placement
+ # it's slower than jax.device_put before JIT, but it's necessary to use since
+ # jax device objects aren't serializable and prevent saving transpiled graphs
+ # this workaround only works because we are inside jax.default_device context
+ # invoked in @handle_device decorator
+ return jnp.copy(ret) if (dev(ret, as_native=True) != device or copy) else ret
def empty(
diff --git a/ivy/functional/backends/jax/device.py b/ivy/functional/backends/jax/device.py
index 7cd30e045142e..e12164e617440 100644
--- a/ivy/functional/backends/jax/device.py
+++ b/ivy/functional/backends/jax/device.py
@@ -41,14 +41,11 @@ def dev(
) -> Union[ivy.Device, jaxlib.xla_extension.Device]:
if isinstance(x, jax.interpreters.partial_eval.DynamicJaxprTracer):
return ""
- try:
- dv = _to_array(x).device_buffer.device
- dv = dv()
- except Exception:
+ if hasattr(x, "device_buffer"):
+ dv = _to_array(x).device_buffer.device()
+ else:
dv = jax.devices()[0]
- if as_native:
- return dv
- return as_ivy_dev(dv)
+ return dv if as_native else as_ivy_dev(dv)
def to_device(
diff --git a/ivy/functional/backends/jax/elementwise.py b/ivy/functional/backends/jax/elementwise.py
index 39e72b3cf5854..f00c00b4443bf 100644
--- a/ivy/functional/backends/jax/elementwise.py
+++ b/ivy/functional/backends/jax/elementwise.py
@@ -72,7 +72,7 @@ def atanh(x: JaxArray, /, *, out: Optional[JaxArray] = None) -> JaxArray:
return jnp.arctanh(x)
-@with_unsupported_dtypes({"0.4.16 and below": ("complex",)}, backend_version)
+@with_unsupported_dtypes({"0.4.19 and below": ("complex",)}, backend_version)
def bitwise_and(
x1: Union[int, JaxArray],
x2: Union[int, JaxArray],
@@ -84,14 +84,14 @@ def bitwise_and(
return jnp.bitwise_and(x1, x2)
-@with_unsupported_dtypes({"0.4.16 and below": ("complex",)}, backend_version)
+@with_unsupported_dtypes({"0.4.19 and below": ("complex",)}, backend_version)
def bitwise_invert(
x: Union[int, JaxArray], /, *, out: Optional[JaxArray] = None
) -> JaxArray:
return jnp.bitwise_not(x)
-@with_unsupported_dtypes({"0.4.16 and below": ("complex",)}, backend_version)
+@with_unsupported_dtypes({"0.4.19 and below": ("complex",)}, backend_version)
def bitwise_left_shift(
x1: Union[int, JaxArray],
x2: Union[int, JaxArray],
@@ -103,7 +103,7 @@ def bitwise_left_shift(
return jnp.left_shift(x1, x2)
-@with_unsupported_dtypes({"0.4.16 and below": ("complex",)}, backend_version)
+@with_unsupported_dtypes({"0.4.19 and below": ("complex",)}, backend_version)
def bitwise_or(
x1: Union[int, JaxArray],
x2: Union[int, JaxArray],
@@ -115,7 +115,7 @@ def bitwise_or(
return jnp.bitwise_or(x1, x2)
-@with_unsupported_dtypes({"0.4.16 and below": ("complex",)}, backend_version)
+@with_unsupported_dtypes({"0.4.19 and below": ("complex",)}, backend_version)
def bitwise_right_shift(
x1: Union[int, JaxArray],
x2: Union[int, JaxArray],
@@ -127,7 +127,7 @@ def bitwise_right_shift(
return jnp.right_shift(x1, x2)
-@with_unsupported_dtypes({"0.4.16 and below": ("complex",)}, backend_version)
+@with_unsupported_dtypes({"0.4.19 and below": ("complex",)}, backend_version)
def bitwise_xor(
x1: Union[int, JaxArray],
x2: Union[int, JaxArray],
@@ -139,7 +139,7 @@ def bitwise_xor(
return jnp.bitwise_xor(x1, x2)
-@with_unsupported_dtypes({"0.4.16 and below": ("complex",)}, backend_version)
+@with_unsupported_dtypes({"0.4.19 and below": ("complex",)}, backend_version)
def ceil(x: JaxArray, /, *, out: Optional[JaxArray] = None) -> JaxArray:
if "int" in str(x.dtype):
return x
@@ -151,7 +151,7 @@ def cos(x: JaxArray, /, *, out: Optional[JaxArray] = None) -> JaxArray:
return jnp.cos(x)
-@with_unsupported_dtypes({"0.4.16 and below": ("float16",)}, backend_version)
+@with_unsupported_dtypes({"0.4.19 and below": ("float16",)}, backend_version)
def cosh(x: JaxArray, /, *, out: Optional[JaxArray] = None) -> JaxArray:
return jnp.cosh(x)
@@ -191,7 +191,7 @@ def expm1(x: JaxArray, /, *, out: Optional[JaxArray] = None) -> JaxArray:
return jnp.expm1(x)
-@with_unsupported_dtypes({"0.4.16 and below": ("complex",)}, backend_version)
+@with_unsupported_dtypes({"0.4.19 and below": ("complex",)}, backend_version)
def floor(x: JaxArray, /, *, out: Optional[JaxArray] = None) -> JaxArray:
if "int" in str(x.dtype):
return x
@@ -199,7 +199,7 @@ def floor(x: JaxArray, /, *, out: Optional[JaxArray] = None) -> JaxArray:
return jnp.floor(x)
-@with_unsupported_dtypes({"0.4.16 and below": ("complex",)}, backend_version)
+@with_unsupported_dtypes({"0.4.19 and below": ("complex",)}, backend_version)
def floor_divide(
x1: Union[float, JaxArray],
x2: Union[float, JaxArray],
@@ -427,7 +427,7 @@ def pow(
return jnp.power(x1, x2)
-@with_unsupported_dtypes({"0.4.16 and below": ("complex",)}, backend_version)
+@with_unsupported_dtypes({"0.4.19 and below": ("complex",)}, backend_version)
def remainder(
x1: Union[float, JaxArray],
x2: Union[float, JaxArray],
@@ -524,7 +524,7 @@ def tanh(
return jnp.tanh(x)
-@with_unsupported_dtypes({"0.4.16 and below": ("complex",)}, backend_version)
+@with_unsupported_dtypes({"0.4.19 and below": ("complex",)}, backend_version)
def trunc(x: JaxArray, /, *, out: Optional[JaxArray] = None) -> JaxArray:
if "int" in str(x.dtype):
return x
@@ -564,7 +564,7 @@ def angle(
# ------#
-@with_unsupported_dtypes({"0.4.16 and below": ("complex",)}, backend_version)
+@with_unsupported_dtypes({"0.4.19 and below": ("complex",)}, backend_version)
def erf(x: JaxArray, /, *, out: Optional[JaxArray] = None) -> JaxArray:
return jax.scipy.special.erf(x)
@@ -615,7 +615,7 @@ def isreal(x: JaxArray, /, *, out: Optional[JaxArray] = None) -> JaxArray:
return jnp.isreal(x)
-@with_unsupported_dtypes({"0.4.16 and below": ("complex",)}, backend_version)
+@with_unsupported_dtypes({"0.4.19 and below": ("complex",)}, backend_version)
def fmod(
x1: JaxArray,
x2: JaxArray,
diff --git a/ivy/functional/backends/jax/experimental/activations.py b/ivy/functional/backends/jax/experimental/activations.py
index 22ee33f220d0f..f2e298cf4400c 100644
--- a/ivy/functional/backends/jax/experimental/activations.py
+++ b/ivy/functional/backends/jax/experimental/activations.py
@@ -35,7 +35,7 @@ def relu6(
# https://github.com/google/jax/pull/14682
def custom_grad_func(x_and_grad, one):
return lax.select(
- (6 > x_and_grad[0]) & (x_and_grad[0] > 0), one, lax.full_like(one, 0)
+ (x_and_grad[0] < 6) & (x_and_grad[0] > 0), one, lax.full_like(one, 0)
)
new_func = ivy.bind_custom_gradient_function(relu6_func, custom_grad_func)
@@ -82,6 +82,17 @@ def elu(
return ret
+def celu(
+ x: JaxArray,
+ /,
+ *,
+ alpha: float = 1.0,
+ complex_mode="jax",
+ out: Optional[JaxArray] = None,
+) -> JaxArray:
+ return jax.nn.celu(x, alpha=alpha)
+
+
@with_unsupported_dtypes({"0.4.14 and below": ("float16", "bfloat16")}, backend_version)
def hardtanh(
x: JaxArray,
@@ -95,3 +106,56 @@ def hardtanh(
if ivy.exists(out):
return ivy.inplace_update(out, ret).astype(x.dtype)
return ivy.astype(ret, x.dtype)
+
+
+def tanhshrink(x: JaxArray, /, *, out: Optional[JaxArray] = None) -> JaxArray:
+ ret = jnp.subtract(x, jax.nn.tanh(x))
+ if ivy.exists(out):
+ return ivy.inplace_update(out, ret).astype(x.dtype)
+ return ret
+
+
+def threshold(
+ x: JaxArray,
+ /,
+ *,
+ threshold: Union[int, float],
+ value: Union[int, float],
+ out: Optional[JaxArray] = None,
+) -> JaxArray:
+ ret = jnp.where(x > threshold, x, value).astype(x.dtype)
+ if ivy.exists(out):
+ return ivy.inplace_update(out, ret).astype(x.dtype) # type: ignore
+ return ret
+
+
+@with_unsupported_dtypes({"0.4.16 and below": ("float16", "bfloat16")}, backend_version)
+def softshrink(
+ x: JaxArray, /, *, lambd: float = 0.5, out: Optional[JaxArray] = None
+) -> JaxArray:
+ ret = jnp.where(x > lambd, x - lambd, jnp.where(x < -lambd, x + lambd, 0))
+ if ivy.exists(out):
+ return ivy.inplace_update(out, ret).astype(x.dtype)
+ return ret
+
+
+@with_unsupported_dtypes({"0.4.17 and below": ("float64",)}, backend_version)
+def scaled_tanh(
+ x: JaxArray,
+ /,
+ *,
+ alpha: float = 1.7159,
+ beta: float = 0.67,
+ out: Optional[JaxArray] = None,
+) -> JaxArray:
+ return alpha * jax.nn.tanh(beta * x)
+
+
+@with_unsupported_dtypes({"0.4.16 and below": ("float16", "bfloat16")}, backend_version)
+def hardshrink(
+ x: JaxArray, /, *, lambd: float = 0.5, out: Optional[JaxArray] = None
+) -> JaxArray:
+ ret = jnp.where(x > lambd, x, jnp.where(x < -lambd, x, 0))
+ if ivy.exists(out):
+ return ivy.inplace_update(out, ret).astype(x.dtype)
+ return ret
diff --git a/ivy/functional/backends/jax/experimental/creation.py b/ivy/functional/backends/jax/experimental/creation.py
index 32a11dd83406c..19e1833db5e4f 100644
--- a/ivy/functional/backends/jax/experimental/creation.py
+++ b/ivy/functional/backends/jax/experimental/creation.py
@@ -83,7 +83,7 @@ def unsorted_segment_min(
num_segments: int,
) -> JaxArray:
# added this check to keep the same behaviour as tensorflow
- ivy.utils.assertions.check_unsorted_segment_min_valid_params(
+ ivy.utils.assertions.check_unsorted_segment_valid_params(
data, segment_ids, num_segments
)
return jax.ops.segment_min(data, segment_ids, num_segments)
@@ -98,7 +98,7 @@ def unsorted_segment_sum(
# the check should be same
# Might require to change the assertion function name to
# check_unsorted_segment_valid_params
- ivy.utils.assertions.check_unsorted_segment_min_valid_params(
+ ivy.utils.assertions.check_unsorted_segment_valid_params(
data, segment_ids, num_segments
)
return jax.ops.segment_sum(data, segment_ids, num_segments)
@@ -118,9 +118,10 @@ def blackman_window(
count = jnp.arange(size) / size
else:
count = jnp.linspace(start=0, stop=size, num=size)
- return (0.42 - 0.5 * jnp.cos(2 * jnp.pi * count)) + (
- 0.08 * jnp.cos(2 * jnp.pi * 2 * count)
- )
+ return (
+ (0.42 - 0.5 * jnp.cos(2 * jnp.pi * count))
+ + (0.08 * jnp.cos(2 * jnp.pi * 2 * count))
+ ).astype(dtype)
def trilu(
@@ -155,10 +156,41 @@ def hz_to_mel(f):
dtype=jnp.float32,
)
mel_edges = jnp.stack([mel_edges[i : i + 3] for i in range(num_mel_bins)])
- lower_edge_mel, center_mel, upper_edge_mel = (
+ lower_edge_mel, center_mel, upper_edge_mel = [
t.reshape((1, num_mel_bins)) for t in jnp.split(mel_edges, 3, axis=1)
- )
+ ]
lower_slopes = (spec_bin_mels - lower_edge_mel) / (center_mel - lower_edge_mel)
upper_slopes = (upper_edge_mel - spec_bin_mels) / (upper_edge_mel - center_mel)
mel_weights = jnp.maximum(zero, jnp.minimum(lower_slopes, upper_slopes))
return jnp.pad(mel_weights, [[1, 0], [0, 0]])
+
+
+def unsorted_segment_mean(
+ data: JaxArray,
+ segment_ids: JaxArray,
+ num_segments: int,
+) -> JaxArray:
+ ivy.utils.assertions.check_unsorted_segment_valid_params(
+ data, segment_ids, num_segments
+ )
+ segment_sum = jax.ops.segment_sum(data, segment_ids, num_segments)
+
+ segment_count = jax.ops.segment_sum(jnp.ones_like(data), segment_ids, num_segments)
+
+ segment_mean = segment_sum / segment_count
+
+ return segment_mean
+
+
+def polyval(
+ coeffs: JaxArray,
+ x: JaxArray,
+) -> JaxArray:
+ with ivy.PreciseMode(True):
+ promoted_type = ivy.promote_types(ivy.dtype(coeffs[0]), ivy.dtype(x[0]))
+ coeffs, x = ivy.promote_types_of_inputs(coeffs, x)
+ y = jnp.zeros_like(x)
+ for pv in coeffs:
+ y = y * x + pv
+ y = jnp.array(y, dtype=jnp.dtype(promoted_type))
+ return y
diff --git a/ivy/functional/backends/jax/experimental/elementwise.py b/ivy/functional/backends/jax/experimental/elementwise.py
index 1dbcf2db2aab9..6ee0c250ecb32 100644
--- a/ivy/functional/backends/jax/experimental/elementwise.py
+++ b/ivy/functional/backends/jax/experimental/elementwise.py
@@ -1,5 +1,5 @@
import operator
-from typing import Optional, Union, Tuple, List
+from typing import Optional, Union, Tuple, List, Sequence
from numbers import Number
from ivy import (
@@ -19,12 +19,38 @@
jax_ArrayLike = Union[JaxArray, Number]
+def amax(
+ x: JaxArray,
+ /,
+ *,
+ axis: Optional[Union[int, Sequence[int]]] = None,
+ keepdims: bool = False,
+ out: Optional[JaxArray] = None,
+) -> JaxArray:
+ axis = tuple(axis) if isinstance(axis, list) else axis
+ ret = jnp.amax(a=jnp.asarray(x), axis=axis, keepdims=keepdims)
+ return jnp.asarray(ret) if jnp.isscalar(ret) else ret
+
+
+def amin(
+ x: JaxArray,
+ /,
+ *,
+ axis: Optional[Union[int, Sequence[int]]] = None,
+ keepdims: bool = False,
+ out: Optional[JaxArray] = None,
+) -> JaxArray:
+ axis = tuple(axis) if isinstance(axis, list) else axis
+ ret = jnp.amin(a=jnp.asarray(x), axis=axis, keepdims=keepdims)
+ return jnp.asarray(ret) if jnp.isscalar(ret) else ret
+
+
def sinc(x: JaxArray, /, *, out: Optional[JaxArray] = None) -> JaxArray:
return jnp.sinc(x)
@with_supported_dtypes(
- {"0.4.16 and below": ("float16", "float32", "float64")}, backend_version
+ {"0.4.19 and below": ("float16", "float32", "float64")}, backend_version
)
def lgamma(x: JaxArray, /, *, out: Optional[JaxArray] = None) -> JaxArray:
return jlax.lgamma(x)
@@ -227,7 +253,7 @@ def _normalize_axis_tuple(axis: Union[int, list, tuple], ndim: int) -> Tuple[int
axis = [operator.index(axis)]
except TypeError:
pass
- axis = tuple([_normalize_axis_index(ax, ndim) for ax in axis])
+ axis = tuple(_normalize_axis_index(ax, ndim) for ax in axis)
if len(set(axis)) != len(axis):
raise ValueError("repeated axis")
return axis
diff --git a/ivy/functional/backends/jax/experimental/gradients.py b/ivy/functional/backends/jax/experimental/gradients.py
index c4c5d7d4fd8d1..5b191d4c18da1 100644
--- a/ivy/functional/backends/jax/experimental/gradients.py
+++ b/ivy/functional/backends/jax/experimental/gradients.py
@@ -1,5 +1,6 @@
# global
import jax
+from typing import Callable
# local
import ivy
@@ -17,3 +18,36 @@ def custom_backward(*args):
func = jax.custom_vjp(func)
func.defvjp(custom_forward, custom_backward)
return inputs_to_native_arrays(func)
+
+
+def vjp(func: Callable, *primals):
+ def grad_fn(*x_in):
+ return ivy.to_native(
+ func(*ivy.to_ivy(x_in, nested=True)), nested=True, include_derived=True
+ )
+
+ primals_out, _vjpfun = ivy.outputs_to_ivy_arrays(jax.vjp)(
+ grad_fn, *ivy.to_native(primals, nested=True)
+ )
+
+ def vjpfun(x_in):
+ return ivy.to_ivy(
+ _vjpfun(ivy.to_native(x_in, nested=True)), nested=True, include_derived=True
+ )
+
+ return (primals_out, vjpfun)
+
+
+def jvp(func: Callable, primals, tangents):
+ def grad_fn(*x_in):
+ return ivy.to_native(
+ func(*ivy.to_ivy(x_in, nested=True)), nested=True, include_derived=True
+ )
+
+ primals_out, tangents_out = ivy.outputs_to_ivy_arrays(jax.jvp)(
+ grad_fn,
+ ivy.to_native(primals, nested=True),
+ ivy.to_native(tangents, nested=True),
+ )
+
+ return (primals_out, tangents_out)
diff --git a/ivy/functional/backends/jax/experimental/layers.py b/ivy/functional/backends/jax/experimental/layers.py
index b5051290aa94a..c409337ed7df0 100644
--- a/ivy/functional/backends/jax/experimental/layers.py
+++ b/ivy/functional/backends/jax/experimental/layers.py
@@ -83,10 +83,8 @@ def general_pool(
# shape of window after dilation
new_window_shape = tuple(
- [
- window_shape[i - 1] + (dilation[i] - 1) * (window_shape[i - 1] - 1)
- for i in range(1, len(dims) - 1)
- ]
+ window_shape[i - 1] + (dilation[i] - 1) * (window_shape[i - 1] - 1)
+ for i in range(1, len(dims) - 1)
)
inputs, window_shape, strides, depth_pooling = _determine_depth_max_pooling(
inputs, window_shape, strides, dim, data_format="channel_last"
@@ -136,20 +134,20 @@ def general_pool(
# because they are counted in average calculation
inputs = jnp.pad(inputs, pad_list, mode="constant", constant_values=1.0)
pad_list = [(0, 0)] * len(pad_list)
+ elif isinstance(padding, list) and any(
+ item != 0 for sublist in padding for item in sublist
+ ):
+ raise NotImplementedError(
+ "Nonzero explicit padding is not supported for depthwise max pooling"
+ )
else:
- if isinstance(padding, list) and any(
- [item != 0 for sublist in padding for item in sublist]
- ):
- raise NotImplementedError(
- "Nonzero explicit padding is not supported for depthwise max pooling"
- )
pad_list = [(0, 0)] * (dim + 2)
if not ivy.is_array(inputs):
# if dtype is not set here, jax casts it to float64
inputs = jnp.array(inputs, dtype=jnp.float32)
if not ivy.is_array(init):
- init = jnp.array(init, dtype=jnp.float32)
+ init = jnp.array(init, dtype=inputs.dtype)
promoted_type = jnp.promote_types(inputs.dtype, init.dtype)
inputs = inputs.astype(promoted_type)
init = init.astype(promoted_type)
@@ -175,7 +173,7 @@ def max_pool1d(
) -> JaxArray:
dims = 1
kernel, strides, padding, dilation = _validate_max_pool_params(
- kernel, strides, padding, dilation, ceil_mode, dims=dims
+ kernel, strides, padding, dilation, ceil_mode, dims, data_format
)
if data_format == "NCW":
@@ -214,7 +212,7 @@ def max_pool2d(
dims = 2
odtype = x.dtype
kernel, strides, padding, dilation = _validate_max_pool_params(
- kernel, strides, padding, dilation, ceil_mode, dims=dims
+ kernel, strides, padding, dilation, ceil_mode, dims, data_format
)
if data_format == "NCHW":
@@ -257,7 +255,7 @@ def max_pool3d(
) -> JaxArray:
dims = 3
kernel, strides, padding, dilation = _validate_max_pool_params(
- kernel, strides, padding, dilation, ceil_mode, dims=dims
+ kernel, strides, padding, dilation, ceil_mode, dims, data_format
)
if data_format == "NCDHW":
x = jnp.transpose(x, (0, 2, 3, 4, 1))
@@ -291,7 +289,7 @@ def avg_pool1d(
x: JaxArray,
kernel: Union[int, Tuple[int]],
strides: Union[int, Tuple[int]],
- padding: str,
+ padding: Union[str, int, List[Tuple[int, int]]],
/,
*,
data_format: str = "NWC",
@@ -341,7 +339,7 @@ def avg_pool2d(
x: JaxArray,
kernel: Union[int, Tuple[int], Tuple[int, int]],
strides: Union[int, Tuple[int], Tuple[int, int]],
- padding: str,
+ padding: Union[str, int, List[Tuple[int, int]]],
/,
*,
data_format: str = "NHWC",
@@ -393,7 +391,7 @@ def avg_pool3d(
x: JaxArray,
kernel: Union[int, Tuple[int], Tuple[int, int, int]],
strides: Union[int, Tuple[int], Tuple[int, int, int]],
- padding: str,
+ padding: Union[str, int, List[Tuple[int, int]]],
/,
*,
data_format: str = "NDHWC",
@@ -441,7 +439,7 @@ def avg_pool3d(
return res
-@with_supported_dtypes({"0.4.16 and below": ("float32", "float64")}, backend_version)
+@with_supported_dtypes({"0.4.19 and below": ("float32", "float64")}, backend_version)
def dct(
x: JaxArray,
/,
@@ -455,7 +453,7 @@ def dct(
if norm not in (None, "ortho"):
raise ValueError("Norm must be either None or 'ortho'")
if axis < 0:
- axis = axis + len(x.shape)
+ axis += len(x.shape)
if n is not None:
signal_len = x.shape[axis]
if n <= signal_len:
@@ -558,7 +556,7 @@ def fft(
raise ivy.utils.exceptions.IvyError(
f"Invalid data points {n}, expecting more than 1"
)
- if norm != "backward" and norm != "ortho" and norm != "forward":
+ if norm not in {"backward", "ortho", "forward"}:
raise ivy.utils.exceptions.IvyError(f"Unrecognized normalization mode {norm}")
return jnp.fft.fft(x, n, dim, norm)
@@ -670,7 +668,7 @@ def ifft(
raise ivy.utils.exceptions.IvyError(
f"Invalid data points {n}, expecting more than 1"
)
- if norm != "backward" and norm != "ortho" and norm != "forward":
+ if norm not in {"backward", "ortho", "forward"}:
raise ivy.utils.exceptions.IvyError(f"Unrecognized normalization mode {norm}")
return jnp.fft.ifft(x, n, dim, norm)
@@ -689,7 +687,8 @@ def interpolate(
"area",
"nearest_exact",
"tf_area",
- "bicubic_tensorflow" "bicubic",
+ "tf_bicubic",
+ "bicubic",
"mitchellcubic",
"lanczos3",
"lanczos5",
@@ -697,29 +696,35 @@ def interpolate(
] = "linear",
scale_factor: Optional[Union[Sequence[int], int]] = None,
recompute_scale_factor: Optional[bool] = None,
- align_corners: Optional[bool] = None,
+ align_corners: bool = False,
antialias: bool = False,
out: Optional[JaxArray] = None,
):
- dims = len(x.shape) - 2
- size = _get_size(scale_factor, size, dims, x.shape)
- mode = (
- "nearest"
- if mode == "nearest-exact"
- else "bicubic" if mode == "bicubic_tensorflow" else mode
- )
+ input_size = ivy.shape(x)[2:]
+ dims = len(input_size)
+ size, _ = _get_size(scale_factor, size, dims, input_size)
+ if all(a == b for a, b in zip(size, input_size)):
+ ret = x
+ else:
+ mode = (
+ "nearest"
+ if mode == "nearest-exact"
+ else "bicubic" if mode == "tf_bicubic" else mode
+ )
- size = [x.shape[0], *size, x.shape[1]]
- x = jnp.transpose(x, (0, *range(2, dims + 2), 1))
- return jnp.transpose(
- jax.image.resize(x, shape=size, method=mode, antialias=antialias),
- (0, dims + 1, *range(1, dims + 1)),
- )
+ size = [x.shape[0], *size, x.shape[1]]
+ x = jnp.transpose(x, (0, *range(2, dims + 2), 1))
+ ret = jnp.transpose(
+ jax.image.resize(x, shape=size, method=mode, antialias=antialias),
+ (0, dims + 1, *range(1, dims + 1)),
+ )
+ if ivy.exists(out):
+ return ivy.inplace_update(out, ret)
+ return ret
-interpolate.partial_mixed_handler = lambda *args, mode="linear", scale_factor=None, recompute_scale_factor=None, align_corners=None, **kwargs: ( # noqa: E501
- (align_corners is None or not align_corners)
- and mode
+interpolate.partial_mixed_handler = (
+ lambda *args, mode="linear", recompute_scale_factor=None, align_corners=None, **kwargs: mode # noqa: E501
not in [
"area",
"nearest",
@@ -729,6 +734,8 @@ def interpolate(
"gaussian",
"bicubic",
]
+ and not align_corners
+ and recompute_scale_factor
)
@@ -814,7 +821,7 @@ def ifftn(
@with_unsupported_dtypes(
- {"0.4.16 and below": ("bfloat16", "float16", "complex")}, backend_version
+ {"0.4.19 and below": ("bfloat16", "float16", "complex")}, backend_version
)
def embedding(
weights: JaxArray,
@@ -840,7 +847,29 @@ def embedding(
return embeddings
-@with_unsupported_dtypes({"0.4.16 and below": ("float16", "complex")}, backend_version)
+def rfft(
+ x: JaxArray,
+ /,
+ *,
+ n: Optional[int] = None,
+ axis: int = -1,
+ norm: Literal["backward", "ortho", "forward"] = "backward",
+ out: Optional[JaxArray] = None,
+) -> JaxArray:
+ x = x.real
+ if x.dtype == jnp.float16:
+ x = x.astype(jnp.float32)
+
+ ret = jnp.fft.rfft(x, n=n, axis=axis, norm=norm)
+
+ if x.dtype != jnp.float64:
+ ret = ret.astype(jnp.complex64)
+ if ivy.exists(out):
+ return ivy.inplace_update(out, ret)
+ return ret
+
+
+@with_unsupported_dtypes({"0.4.19 and below": ("float16", "complex")}, backend_version)
def rfftn(
x: JaxArray,
s: Sequence[int] = None,
@@ -868,7 +897,7 @@ def rfftn(
raise ivy.utils.exceptions.IvyError(
f"Invalid data points {s}, expecting s points larger than 1"
)
- if norm != "backward" and norm != "ortho" and norm != "forward":
+ if norm not in {"backward", "ortho", "forward"}:
raise ivy.utils.exceptions.IvyError(f"Unrecognized normalization mode {norm}")
return jnp.fft.rfftn(x, s, axes, norm).astype(jnp.complex128)
diff --git a/ivy/functional/backends/jax/experimental/linear_algebra.py b/ivy/functional/backends/jax/experimental/linear_algebra.py
index f4fd65d9f9a10..0f1841e41f1a6 100644
--- a/ivy/functional/backends/jax/experimental/linear_algebra.py
+++ b/ivy/functional/backends/jax/experimental/linear_algebra.py
@@ -136,6 +136,25 @@ def adjoint(
return jnp.conjugate(jnp.transpose(x, axes=axes))
+def solve_triangular(
+ x1: JaxArray,
+ x2: JaxArray,
+ /,
+ *,
+ upper: bool = True,
+ adjoint: bool = False,
+ unit_diagonal: bool = False,
+ out: Optional[JaxArray] = None,
+) -> JaxArray:
+ return jla.solve_triangular(
+ x1,
+ x2,
+ lower=not upper,
+ trans="C" if adjoint else "N",
+ unit_diagonal=unit_diagonal,
+ )
+
+
def multi_dot(
x: Sequence[JaxArray],
/,
diff --git a/ivy/functional/backends/jax/experimental/losses.py b/ivy/functional/backends/jax/experimental/losses.py
index 6a96f2cbd010e..bb7e3e3020d50 100644
--- a/ivy/functional/backends/jax/experimental/losses.py
+++ b/ivy/functional/backends/jax/experimental/losses.py
@@ -64,11 +64,11 @@ def soft_margin_loss(
return loss
-def _apply_loss_reduction(loss: JaxArray, reduction: str) -> JaxArray:
+def _apply_loss_reduction(loss: JaxArray, reduction: str, axis=None) -> JaxArray:
if reduction == "sum":
- return jnp.sum(loss)
+ return jnp.sum(loss, axis=axis)
elif reduction == "mean":
- return jnp.mean(loss)
+ return jnp.mean(loss, axis=axis)
else: # reduction == "none"
return loss
diff --git a/ivy/functional/backends/jax/experimental/manipulation.py b/ivy/functional/backends/jax/experimental/manipulation.py
index d7721db9f02aa..fc760a9929655 100644
--- a/ivy/functional/backends/jax/experimental/manipulation.py
+++ b/ivy/functional/backends/jax/experimental/manipulation.py
@@ -391,3 +391,79 @@ def unique_consecutive(
inverse_indices,
counts,
)
+
+
+def fill_diagonal(
+ a: JaxArray,
+ v: Union[int, float],
+ /,
+ *,
+ wrap: bool = False,
+) -> JaxArray:
+ shape = jnp.array(a.shape)
+ end = None
+ if len(shape) == 2:
+ step = shape[1] + 1
+ if not wrap:
+ end = shape[1] * shape[1]
+ else:
+ step = 1 + (jnp.cumprod(shape[:-1])).sum()
+ a = jnp.reshape(a, (-1,))
+ a = a.at[:end:step].set(jnp.array(v).astype(a.dtype))
+ a = jnp.reshape(a, shape)
+ return a
+
+
+def take(
+ x: Union[int, JaxArray],
+ indices: Union[int, JaxArray],
+ /,
+ *,
+ axis: Optional[int] = None,
+ mode: str = "fill",
+ fill_value: Optional[Number] = None,
+ out: Optional[JaxArray] = None,
+) -> JaxArray:
+ if mode not in ["raise", "wrap", "clip", "fill"]:
+ raise ValueError("mode must be one of 'clip', 'raise', 'wrap', or 'fill'")
+ if not isinstance(x, JaxArray):
+ x = jnp.array(x)
+ if len(x.shape) == 0:
+ x = jnp.array([x])
+ if not isinstance(indices, JaxArray):
+ indices = jnp.array(indices)
+ if jnp.issubdtype(indices.dtype, jnp.floating):
+ indices = indices.astype(jnp.int64)
+
+ # raise
+ if mode == "raise":
+ mode = "fill"
+ if ivy.exists(axis):
+ try:
+ x_shape = x.shape[axis]
+ except Exception:
+ raise ValueError(
+ f"axis {axis} is out of bounds for array of dimension"
+ f" {len(x.shape)}"
+ )
+ else:
+ x_shape = jnp.prod(x.shape)
+
+ bound_check = (indices < -x_shape) | (indices >= x_shape)
+ if jnp.any(bound_check):
+ if len(indices.shape) != 0:
+ indices = indices[bound_check].flatten()[0]
+ raise IndexError(
+ f"index {indices} is out of bounds for axis "
+ f"{axis if axis else 0} with size {x_shape}"
+ )
+
+ # clip, wrap, fill
+ ret = jnp.take(x, indices, axis=axis, mode=mode, fill_value=fill_value)
+ if ivy.exists(out):
+ ivy.inplace_update(out)
+ return ret
+
+
+def trim_zeros(a: JaxArray, /, *, trim: Optional[str] = "bf") -> JaxArray:
+ return jnp.trim_zeros(a, trim=trim)
diff --git a/ivy/functional/backends/jax/experimental/random.py b/ivy/functional/backends/jax/experimental/random.py
index aa0557545a983..b0148cc1ca30b 100644
--- a/ivy/functional/backends/jax/experimental/random.py
+++ b/ivy/functional/backends/jax/experimental/random.py
@@ -56,7 +56,7 @@ def beta(
return jax.random.beta(rng_input, a, b, shape, dtype)
-@with_unsupported_dtypes({"0.4.16 and below": ("bfloat16",)}, backend_version)
+@with_unsupported_dtypes({"0.4.19 and below": ("bfloat16",)}, backend_version)
def gamma(
alpha: Union[float, JaxArray],
beta: Union[float, JaxArray],
@@ -124,6 +124,6 @@ def bernoulli(
_setRNG(RNG_)
if logits is not None:
probs = jax.nn.softmax(logits, axis=-1)
- if not _check_shapes_broadcastable(shape, probs.shape):
+ if hasattr(probs, "shape") and not _check_shapes_broadcastable(shape, probs.shape):
shape = probs.shape
return jax.random.bernoulli(rng_input, probs, shape=shape)
diff --git a/ivy/functional/backends/jax/experimental/sorting.py b/ivy/functional/backends/jax/experimental/sorting.py
index 376c3f6be365d..27f7994429c51 100644
--- a/ivy/functional/backends/jax/experimental/sorting.py
+++ b/ivy/functional/backends/jax/experimental/sorting.py
@@ -23,7 +23,7 @@ def invert_permutation(
# lexsort
-@with_unsupported_dtypes({"0.4.16 and below": ("bfloat16",)}, backend_version)
+@with_unsupported_dtypes({"0.4.19 and below": ("bfloat16",)}, backend_version)
def lexsort(
keys: JaxArray,
/,
diff --git a/ivy/functional/backends/jax/experimental/statistical.py b/ivy/functional/backends/jax/experimental/statistical.py
index d6250e4be4c9d..305eb9649829c 100644
--- a/ivy/functional/backends/jax/experimental/statistical.py
+++ b/ivy/functional/backends/jax/experimental/statistical.py
@@ -10,7 +10,7 @@
@with_unsupported_dtypes(
- {"0.4.16 and below": ("bfloat16",)},
+ {"0.4.19 and below": ("bfloat16",)},
backend_version,
)
def histogram(
@@ -121,7 +121,7 @@ def histogram(
@with_unsupported_dtypes(
- {"0.4.16 and below": ("complex64", "complex128")}, backend_version
+ {"0.4.19 and below": ("complex64", "complex128")}, backend_version
)
def median(
input: JaxArray,
@@ -162,6 +162,23 @@ def nanmean(
return jnp.nanmean(a, axis=axis, keepdims=keepdims, dtype=dtype, out=out)
+def nanmin(
+ x: JaxArray,
+ /,
+ *,
+ axis: Optional[Union[int, Tuple[int]]] = None,
+ keepdims: Optional[bool] = False,
+ initial: Optional[Union[int, float, complex]] = None,
+ where: Optional[JaxArray] = None,
+ out: Optional[JaxArray] = None,
+) -> JaxArray:
+ if isinstance(axis, list):
+ axis = tuple(axis)
+ return jnp.nanmin(
+ x, axis=axis, keepdims=keepdims, initial=initial, where=where, out=out
+ )
+
+
def nanprod(
a: JaxArray,
/,
@@ -389,7 +406,7 @@ def __get_index(lst, indices=None, prefix=None):
@with_unsupported_dtypes(
{
- "0.4.16 and below": (
+ "0.4.19 and below": (
"bfloat16",
"bool",
)
diff --git a/ivy/functional/backends/jax/general.py b/ivy/functional/backends/jax/general.py
index 6bc3efbec82d3..e783eb26fbcbb 100644
--- a/ivy/functional/backends/jax/general.py
+++ b/ivy/functional/backends/jax/general.py
@@ -101,7 +101,7 @@ def array_equal(x0: JaxArray, x1: JaxArray, /) -> bool:
return bool(jnp.array_equal(x0, x1))
-@with_unsupported_dtypes({"0.4.16 and below": ("bfloat16",)}, backend_version)
+@with_unsupported_dtypes({"0.4.19 and below": ("bfloat16",)}, backend_version)
def to_numpy(x: JaxArray, /, *, copy: bool = True) -> np.ndarray:
if copy:
return np.array(_to_array(x))
@@ -129,8 +129,8 @@ def gather(
batch_dims: int = 0,
out: Optional[JaxArray] = None,
) -> JaxArray:
- axis = axis % len(params.shape)
- batch_dims = batch_dims % len(params.shape)
+ axis %= len(params.shape)
+ batch_dims %= len(params.shape)
ivy.utils.assertions.check_gather_input_valid(params, indices, axis, batch_dims)
result = []
if batch_dims == 0:
@@ -334,8 +334,8 @@ def scatter_flat(
target = target.at[indices].max(updates)
else:
raise ivy.utils.exceptions.IvyException(
- "reduction is {}, but it must be one of "
- '"sum", "min", "max" or "replace"'.format(reduction)
+ f'reduction is {reduction}, but it must be one of "sum", "min", "max" or'
+ ' "replace"'
)
if target_given:
return ivy.inplace_update(out, target)
@@ -386,8 +386,8 @@ def scatter_nd(
target = target.at[indices_tuple].mul(updates)
else:
raise ivy.utils.exceptions.IvyException(
- "reduction is {}, but it must be one of "
- '"sum", "min", "max", "mul" or "replace"'.format(reduction)
+ f'reduction is {reduction}, but it must be one of "sum", "min", "max",'
+ ' "mul" or "replace"'
)
if ivy.exists(out):
return ivy.inplace_update(out, target)
@@ -420,7 +420,7 @@ def vmap(
)
-@with_unsupported_dtypes({"0.4.16 and below": ("float16", "bfloat16")}, backend_version)
+@with_unsupported_dtypes({"0.4.19 and below": ("float16", "bfloat16")}, backend_version)
def isin(
elements: JaxArray,
test_elements: JaxArray,
diff --git a/ivy/functional/backends/jax/gradients.py b/ivy/functional/backends/jax/gradients.py
index 1475ba81baa76..13ee80730c2b4 100644
--- a/ivy/functional/backends/jax/gradients.py
+++ b/ivy/functional/backends/jax/gradients.py
@@ -48,7 +48,7 @@ def _forward_fn(
ivy.index_nest(xs, grad_idx), ivy.is_array
)
for idx in xs_grad_arr_idx:
- xs_grad_arr_idxs.append(grad_idx + idx)
+ xs_grad_arr_idxs.append(list(grad_idx) + idx)
ivy.set_nest_at_indices(xs, xs_grad_arr_idxs, x_arr_values)
elif ivy.is_array(xs):
xs = x
@@ -74,8 +74,8 @@ def execute_with_gradients(
/,
*,
retain_grads: bool = False,
- xs_grad_idxs: Optional[Sequence[Sequence[Union[str, int]]]] = [[0]],
- ret_grad_idxs: Optional[Sequence[Sequence[Union[str, int]]]] = [[0]],
+ xs_grad_idxs: Sequence[Sequence[Union[str, int]]] = ((0,),),
+ ret_grad_idxs: Sequence[Sequence[Union[str, int]]] = ((0,),),
):
# Conversion of required arrays to float variables and duplicate index chains
(
diff --git a/ivy/functional/backends/jax/layers.py b/ivy/functional/backends/jax/layers.py
index ff4c264439da7..001de4a03d0ff 100644
--- a/ivy/functional/backends/jax/layers.py
+++ b/ivy/functional/backends/jax/layers.py
@@ -1,6 +1,5 @@
"""Collection of Jax network layers, wrapped to fit Ivy syntax and signature."""
-
# global
import jax.lax as jlax
import jax.numpy as jnp
@@ -64,7 +63,7 @@ def _get_new_padding_before_conv(
dilations,
x_dilations,
):
- if not len(x_dilations) == x_dilations.count(1):
+ if len(x_dilations) != x_dilations.count(1):
new_pad = [0] * dims
x_shape = (
list(x.shape[1 : dims + 1])
@@ -333,11 +332,11 @@ def conv3d_transpose(
def _get_filter_dataformat(dims: int = 2, filter_format: str = "channel_last"):
first = True if filter_format == "channel_first" else False
if dims == 1:
- return "WIO" if not first else "OIW"
+ return "OIW" if first else "WIO"
if dims == 2:
- return "HWIO" if not first else "OIHW"
+ return "OIHW" if first else "HWIO"
elif dims == 3:
- return "DHWIO" if not first else "OIDHW"
+ return "OIDHW" if first else "DHWIO"
def conv_general_dilated(
@@ -362,7 +361,7 @@ def conv_general_dilated(
if isinstance(padding, int):
padding = [(padding, padding)] * dims
filter_df = _get_filter_dataformat(dims, filter_format)
- if not len(x_dilations) == x_dilations.count(1):
+ if len(x_dilations) != x_dilations.count(1):
new_pad = [0] * dims
x_shape = (
list(x.shape[1 : dims + 1])
@@ -455,3 +454,74 @@ def conv_general_transpose(
if data_format == "channel_first":
return jnp.transpose(res, (0, dims + 1, *range(1, dims + 1)))
return res
+
+
+def nms(
+ boxes,
+ scores=None,
+ iou_threshold=0.5,
+ max_output_size=None,
+ score_threshold=float("-inf"),
+):
+ change_id = False
+ if score_threshold != float("-inf") and scores is not None:
+ keep_idx = scores > score_threshold
+ boxes = boxes[keep_idx]
+ scores = scores[keep_idx]
+ change_id = True
+ nonzero = jnp.nonzero(keep_idx)[0].flatten()
+ if scores is None:
+ scores = jnp.ones((boxes.shape[0],), dtype=boxes.dtype)
+
+ if len(boxes) < 2:
+ if len(boxes) == 1:
+ ret = jnp.array([0], dtype=ivy.int64)
+ else:
+ ret = jnp.array([], dtype=ivy.int64)
+ else:
+ areas = jnp.prod(boxes[:, 2:4] - boxes[:, :2], axis=1)
+ order = jnp.argsort(-1 * scores) # get boxes with more ious first
+ boxes = boxes[order]
+ areas = areas[order]
+ size = order.size
+ pad_width = 1 if size == 0 else 2 ** (size - 1).bit_length()
+
+ order = jnp.pad(order, [0, pad_width - size], constant_values=pad_width)
+ boxes = jnp.pad(boxes, [[0, pad_width - size], [0, 0]])
+ areas = jnp.pad(areas, [0, pad_width - size])
+ keep = jnp.zeros((size,), dtype=jnp.int64)
+ keep_idx = 0
+
+ while jnp.unique(order).size > 1:
+ max_iou_idx = order[0]
+ keep = keep.at[keep_idx].set(max_iou_idx)
+ keep_idx += 1
+ boxes1 = jnp.maximum(boxes[0, :2], boxes[1:, :2])
+ boxes2 = jnp.minimum(boxes[0, 2:4], boxes[1:, 2:4])
+ boxes_intersection = jnp.maximum(0.0, boxes2 - boxes1)
+ intersection = jnp.prod(
+ jnp.where(boxes_intersection != 0, boxes_intersection, 1), axis=1
+ )
+ iou = intersection / (areas[0] + areas[1:] - intersection)
+ condition = jnp.pad(iou <= iou_threshold, [1, 0], constant_values=False)
+ order = jnp.where(condition, order, pad_width)
+ boxes = jnp.where(jnp.expand_dims(condition, axis=1), boxes, 0)
+ areas = jnp.where(condition, areas, 0)
+ first = jnp.argwhere(order < pad_width, size=pad_width)[0][0]
+ forward = jnp.array([0, first])
+ order = order.at[forward].set(order[forward[::-1]])
+ boxes = boxes.at[forward].set(boxes[forward[::-1]])
+ areas = areas.at[forward].set(areas[forward[::-1]])
+
+ ret = jnp.array(keep[:keep_idx], dtype=jnp.int64)
+
+ if len(ret) > 1 and scores is not None:
+ ret = sorted(
+ ret.flatten().tolist(), reverse=True, key=lambda x: (scores[x], -x)
+ )
+ ret = jnp.array(ret, dtype=jnp.int64).flatten()
+
+ if change_id and len(ret) > 0:
+ ret = jnp.array(nonzero[ret], dtype=jnp.int64).flatten()
+
+ return ret.flatten()[:max_output_size]
diff --git a/ivy/functional/backends/jax/linear_algebra.py b/ivy/functional/backends/jax/linear_algebra.py
index 72ad54a0b619e..68aeb20ed53bf 100644
--- a/ivy/functional/backends/jax/linear_algebra.py
+++ b/ivy/functional/backends/jax/linear_algebra.py
@@ -20,7 +20,7 @@
@with_unsupported_dtypes(
- {"0.4.16 and below": ("bfloat16", "float16", "complex")},
+ {"0.4.19 and below": ("bfloat16", "float16", "complex")},
backend_version,
)
def cholesky(
@@ -34,7 +34,7 @@ def cholesky(
return ret
-@with_unsupported_dtypes({"0.4.16 and below": ("complex",)}, backend_version)
+@with_unsupported_dtypes({"0.4.19 and below": ("complex",)}, backend_version)
def cross(
x1: JaxArray,
x2: JaxArray,
@@ -51,14 +51,14 @@ def cross(
@with_unsupported_dtypes(
- {"0.4.16 and below": ("bfloat16", "float16", "complex")},
+ {"0.4.19 and below": ("bfloat16", "float16", "complex")},
backend_version,
)
def det(x: JaxArray, /, *, out: Optional[JaxArray] = None) -> JaxArray:
return jnp.linalg.det(x)
-@with_unsupported_dtypes({"0.4.16 and below": ("float16", "bfloat16")}, backend_version)
+@with_unsupported_dtypes({"0.4.19 and below": ("float16", "bfloat16")}, backend_version)
def eig(x: JaxArray, /, *, out: Optional[JaxArray] = None) -> Tuple[JaxArray]:
result_tuple = NamedTuple(
"eig", [("eigenvalues", JaxArray), ("eigenvectors", JaxArray)]
@@ -67,7 +67,7 @@ def eig(x: JaxArray, /, *, out: Optional[JaxArray] = None) -> Tuple[JaxArray]:
return result_tuple(eigenvalues, eigenvectors)
-@with_unsupported_dtypes({"0.4.16 and below": ("complex",)}, backend_version)
+@with_unsupported_dtypes({"0.4.19 and below": ("complex",)}, backend_version)
def diagonal(
x: JaxArray,
/,
@@ -77,7 +77,7 @@ def diagonal(
axis2: int = -1,
out: Optional[JaxArray] = None,
) -> JaxArray:
- if not x.dtype == bool and not jnp.issubdtype(x.dtype, jnp.integer):
+ if x.dtype != bool and not jnp.issubdtype(x.dtype, jnp.integer):
ret = jnp.diagonal(x, offset=offset, axis1=axis1, axis2=axis2)
ret_edited = jnp.diagonal(
x.at[1 / x == -jnp.inf].set(-jnp.inf),
@@ -104,7 +104,7 @@ def tensorsolve(
@with_unsupported_dtypes(
- {"0.4.16 and below": ("bfloat16", "float16", "complex")},
+ {"0.4.19 and below": ("bfloat16", "float16", "complex")},
backend_version,
)
def eigh(
@@ -118,7 +118,7 @@ def eigh(
@with_unsupported_dtypes(
- {"0.4.16 and below": ("bfloat16", "float16", "complex")},
+ {"0.4.19 and below": ("bfloat16", "float16", "complex")},
backend_version,
)
def eigvalsh(
@@ -127,14 +127,14 @@ def eigvalsh(
return jnp.linalg.eigvalsh(x, UPLO=UPLO)
-@with_unsupported_dtypes({"0.4.16 and below": ("complex",)}, backend_version)
+@with_unsupported_dtypes({"0.4.19 and below": ("complex",)}, backend_version)
def inner(x1: JaxArray, x2: JaxArray, /, *, out: Optional[JaxArray] = None) -> JaxArray:
x1, x2 = ivy.promote_types_of_inputs(x1, x2)
return jnp.inner(x1, x2)
@with_unsupported_dtypes(
- {"0.4.16 and below": ("bfloat16", "float16", "complex")},
+ {"0.4.19 and below": ("bfloat16", "float16", "complex")},
backend_version,
)
def inv(
@@ -155,7 +155,7 @@ def inv(
@with_unsupported_dtypes(
- {"0.4.16 and below": ("bfloat16", "float16", "complex")},
+ {"0.4.19 and below": ("bfloat16", "float16", "complex")},
backend_version,
)
def matmul(
@@ -181,7 +181,7 @@ def matmul(
@with_unsupported_dtypes(
- {"0.4.16 and below": ("bfloat16", "float16", "complex")},
+ {"0.4.19 and below": ("bfloat16", "float16", "complex")},
backend_version,
)
def matrix_norm(
@@ -202,13 +202,13 @@ def matrix_norm(
return jnp.linalg.norm(x, ord=ord, axis=axis, keepdims=keepdims)
-@with_unsupported_dtypes({"0.4.16 and below": ("complex",)}, backend_version)
+@with_unsupported_dtypes({"0.4.19 and below": ("complex",)}, backend_version)
def matrix_power(x: JaxArray, n: int, /, *, out: Optional[JaxArray] = None) -> JaxArray:
return jnp.linalg.matrix_power(x, n)
@with_unsupported_dtypes(
- {"0.4.16 and below": ("bfloat16", "float16", "complex")},
+ {"0.4.19 and below": ("bfloat16", "float16", "complex")},
backend_version,
)
def matrix_rank(
@@ -239,7 +239,7 @@ def matrix_rank(
@with_unsupported_dtypes(
- {"0.4.16 and below": ("int", "float16", "complex")},
+ {"0.4.19 and below": ("int", "float16", "complex")},
backend_version,
)
def matrix_transpose(
@@ -251,7 +251,7 @@ def matrix_transpose(
@with_unsupported_dtypes(
- {"0.4.16 and below": ("bfloat16", "float16", "complex")},
+ {"0.4.19 and below": ("bfloat16", "float16", "complex")},
backend_version,
)
def outer(
@@ -266,7 +266,7 @@ def outer(
@with_unsupported_dtypes(
- {"0.4.16 and below": ("bfloat16", "float16", "complex")},
+ {"0.4.19 and below": ("bfloat16", "float16", "complex")},
backend_version,
)
def pinv(
@@ -284,7 +284,7 @@ def pinv(
@with_unsupported_dtypes(
- {"0.4.16 and below": ("bfloat16", "float16", "complex")},
+ {"0.4.19 and below": ("bfloat16", "float16", "complex")},
backend_version,
)
def qr(
@@ -296,7 +296,7 @@ def qr(
@with_unsupported_dtypes(
- {"0.4.16 and below": ("bfloat16", "float16", "complex")},
+ {"0.4.19 and below": ("bfloat16", "float16", "complex")},
backend_version,
)
def slogdet(
@@ -309,7 +309,7 @@ def slogdet(
@with_unsupported_dtypes(
- {"0.4.16 and below": ("bfloat16", "float16", "complex")},
+ {"0.4.19 and below": ("bfloat16", "float16", "complex")},
backend_version,
)
def solve(
@@ -351,7 +351,7 @@ def solve(
@with_unsupported_dtypes(
- {"0.4.16 and below": ("bfloat16", "float16", "complex")},
+ {"0.4.19 and below": ("bfloat16", "float16", "complex")},
backend_version,
)
def svd(
@@ -368,14 +368,17 @@ def svd(
@with_unsupported_dtypes(
- {"0.4.16 and below": ("bfloat16", "float16", "complex")},
+ {"0.4.19 and below": ("bfloat16", "float16", "complex")},
backend_version,
)
-def svdvals(x: JaxArray, /, *, out: Optional[JaxArray] = None) -> JaxArray:
+def svdvals(
+ x: JaxArray, /, *, driver: Optional[str] = None, out: Optional[JaxArray] = None
+) -> JaxArray:
+ # TODO: handling the driver argument
return jnp.linalg.svd(x, compute_uv=False)
-@with_unsupported_dtypes({"0.4.16 and below": ("complex",)}, backend_version)
+@with_unsupported_dtypes({"0.4.19 and below": ("complex",)}, backend_version)
def tensordot(
x1: JaxArray,
x2: JaxArray,
@@ -389,7 +392,7 @@ def tensordot(
@with_unsupported_dtypes(
- {"0.4.16 and below": ("bfloat16", "float16", "complex")},
+ {"0.4.19 and below": ("bfloat16", "float16", "complex")},
backend_version,
)
def trace(
@@ -404,7 +407,7 @@ def trace(
return jnp.trace(x, offset=offset, axis1=axis1, axis2=axis2, out=out)
-@with_unsupported_dtypes({"0.4.16 and below": ("complex",)}, backend_version)
+@with_unsupported_dtypes({"0.4.19 and below": ("complex",)}, backend_version)
def vecdot(
x1: JaxArray, x2: JaxArray, /, *, axis: int = -1, out: Optional[JaxArray] = None
) -> JaxArray:
@@ -412,7 +415,7 @@ def vecdot(
return jnp.tensordot(x1, x2, axes=(axis, axis))
-@with_unsupported_dtypes({"0.4.16 and below": ("complex",)}, backend_version)
+@with_unsupported_dtypes({"0.4.19 and below": ("complex",)}, backend_version)
def vector_norm(
x: JaxArray,
/,
@@ -442,7 +445,7 @@ def vector_norm(
# ------#
-@with_unsupported_dtypes({"0.4.16 and below": ("complex",)}, backend_version)
+@with_unsupported_dtypes({"0.4.19 and below": ("complex",)}, backend_version)
def diag(
x: JaxArray,
/,
@@ -454,7 +457,7 @@ def diag(
@with_unsupported_dtypes(
- {"0.4.16 and below": ("bfloat16", "float16", "complex")},
+ {"0.4.19 and below": ("bfloat16", "float16", "complex")},
backend_version,
)
def vander(
@@ -470,7 +473,7 @@ def vander(
@with_unsupported_dtypes(
{
- "0.4.16 and below": (
+ "0.4.19 and below": (
"complex",
"unsigned",
)
diff --git a/ivy/functional/backends/jax/manipulation.py b/ivy/functional/backends/jax/manipulation.py
index 5b84e5c591bd4..f2e54c5ddfd51 100644
--- a/ivy/functional/backends/jax/manipulation.py
+++ b/ivy/functional/backends/jax/manipulation.py
@@ -162,9 +162,8 @@ def split(
if x.shape == ():
if num_or_size_splits is not None and num_or_size_splits != 1:
raise ivy.utils.exceptions.IvyException(
- "input array had no shape, but num_sections specified was {}".format(
- num_or_size_splits
- )
+ "input array had no shape, but num_sections specified was"
+ f" {num_or_size_splits}"
)
return [x]
if isinstance(num_or_size_splits, jnp.ndarray):
@@ -227,7 +226,7 @@ def clip(
return x
-@with_unsupported_dtypes({"0.4.16 and below": ("uint64",)}, backend_version)
+@with_unsupported_dtypes({"0.4.19 and below": ("uint64",)}, backend_version)
def constant_pad(
x: JaxArray,
/,
diff --git a/ivy/functional/backends/jax/random.py b/ivy/functional/backends/jax/random.py
index 82847594fe2bc..9abba4d7bb617 100644
--- a/ivy/functional/backends/jax/random.py
+++ b/ivy/functional/backends/jax/random.py
@@ -82,7 +82,7 @@ def random_normal(
return jax.random.normal(rng_input, shape, dtype=dtype) * std + mean
-@with_unsupported_dtypes({"0.4.16 and below": ("bfloat16",)}, backend_version)
+@with_unsupported_dtypes({"0.4.19 and below": ("bfloat16",)}, backend_version)
def multinomial(
population_size: int,
num_samples: int,
diff --git a/ivy/functional/backends/jax/searching.py b/ivy/functional/backends/jax/searching.py
index a62d3f10e0af8..ffdd22b03e098 100644
--- a/ivy/functional/backends/jax/searching.py
+++ b/ivy/functional/backends/jax/searching.py
@@ -12,7 +12,7 @@
# ------------------ #
-@with_unsupported_dtypes({"0.4.16 and below": ("complex",)}, backend_version)
+@with_unsupported_dtypes({"0.4.19 and below": ("complex",)}, backend_version)
def argmax(
x: JaxArray,
/,
@@ -38,7 +38,7 @@ def argmax(
return ret
-@with_unsupported_dtypes({"0.4.16 and below": ("complex",)}, backend_version)
+@with_unsupported_dtypes({"0.4.19 and below": ("complex",)}, backend_version)
def argmin(
x: JaxArray,
/,
diff --git a/ivy/functional/backends/jax/set.py b/ivy/functional/backends/jax/set.py
index a0bd51052f61b..d8b1864435912 100644
--- a/ivy/functional/backends/jax/set.py
+++ b/ivy/functional/backends/jax/set.py
@@ -85,13 +85,19 @@ def unique_counts(
def unique_inverse(
x: JaxArray,
/,
+ *,
+ axis: Optional[int] = None,
) -> Tuple[JaxArray, JaxArray]:
Results = namedtuple("Results", ["values", "inverse_indices"])
- values, inverse_indices = jnp.unique(x, return_inverse=True)
+ values, inverse_indices = jnp.unique(x, return_inverse=True, axis=axis)
+
nan_count = jnp.count_nonzero(jnp.isnan(x))
if nan_count > 1:
- values = jnp.append(values, jnp.full(nan_count - 1, jnp.nan)).astype(x.dtype)
- inverse_indices = jnp.reshape(inverse_indices, x.shape)
+ values = jnp.append(values, jnp.full(nan_count - 1, jnp.nan), axis=0).astype(
+ x.dtype
+ )
+ inverse_indices = jnp.reshape(inverse_indices, x.shape, axis=0)
+
return Results(values, inverse_indices)
diff --git a/ivy/functional/backends/jax/sorting.py b/ivy/functional/backends/jax/sorting.py
index 9643dfa9167b2..4edfc258954ab 100644
--- a/ivy/functional/backends/jax/sorting.py
+++ b/ivy/functional/backends/jax/sorting.py
@@ -80,7 +80,7 @@ def searchsorted(
# msort
-@with_unsupported_dtypes({"0.4.16 and below": ("complex",)}, backend_version)
+@with_unsupported_dtypes({"0.4.19 and below": ("complex",)}, backend_version)
def msort(
a: Union[JaxArray, list, tuple],
/,
diff --git a/ivy/functional/backends/jax/statistical.py b/ivy/functional/backends/jax/statistical.py
index 8e45fa667d212..43b7d097136d9 100644
--- a/ivy/functional/backends/jax/statistical.py
+++ b/ivy/functional/backends/jax/statistical.py
@@ -37,7 +37,10 @@ def max(
return jnp.max(a=jnp.asarray(x), axis=axis, keepdims=keepdims)
-@with_unsupported_dtypes({"0.4.14 and below": "bfloat16"}, backend_version)
+@with_unsupported_dtypes(
+ {"0.4.19 and below": "bfloat16"},
+ backend_version,
+)
def mean(
x: JaxArray,
/,
@@ -47,7 +50,7 @@ def mean(
out: Optional[JaxArray] = None,
) -> JaxArray:
axis = tuple(axis) if isinstance(axis, list) else axis
- return jnp.mean(x, axis=axis, keepdims=keepdims)
+ return jnp.mean(x, axis=axis, keepdims=keepdims, dtype=x.dtype)
def _infer_dtype(dtype: jnp.dtype):
@@ -140,7 +143,7 @@ def var(
# ------#
-@with_unsupported_dtypes({"0.4.16 and below": "bfloat16"}, backend_version)
+@with_unsupported_dtypes({"0.4.19 and below": "bfloat16"}, backend_version)
def cumprod(
x: JaxArray,
/,
diff --git a/ivy/functional/backends/mxnet/activations.py b/ivy/functional/backends/mxnet/activations.py
index 3bb8f87e1b7b7..96bd23c3ca96f 100644
--- a/ivy/functional/backends/mxnet/activations.py
+++ b/ivy/functional/backends/mxnet/activations.py
@@ -4,6 +4,7 @@
Collection of MXNet activation functions, wrapped to fit Ivy syntax and
signature.
"""
+
import mxnet as mx
import numpy as np
@@ -20,9 +21,7 @@ def gelu(
out: Optional[None] = None,
) -> None:
if approximate:
- return (
- 0.5 * x * (1 + mx.nd.tanh(((2 / np.pi) ** 0.5) * (x + 0.044715 * x**3)))
- )
+ return 0.5 * x * (1 + mx.nd.tanh(((2 / np.pi) ** 0.5) * (x + 0.044715 * x**3)))
return mx.nd.LeakyReLU(x, act_type="gelu")
diff --git a/ivy/functional/backends/mxnet/creation.py b/ivy/functional/backends/mxnet/creation.py
index 11599037f8172..d32d312efc394 100644
--- a/ivy/functional/backends/mxnet/creation.py
+++ b/ivy/functional/backends/mxnet/creation.py
@@ -4,7 +4,7 @@
from numbers import Number
from typing import Union, List, Optional, Sequence, Tuple
-# lcoal
+# local
import ivy
from ivy.utils.exceptions import IvyNotImplementedException
from ivy.functional.ivy.creation import (
diff --git a/ivy/functional/backends/mxnet/data_type.py b/ivy/functional/backends/mxnet/data_type.py
index 1ec0e1174ceec..74ab7b6cb71dd 100644
--- a/ivy/functional/backends/mxnet/data_type.py
+++ b/ivy/functional/backends/mxnet/data_type.py
@@ -129,9 +129,7 @@ def iinfo(type: Union[str, mx.ndarray.NDArray, np.dtype], /) -> np.iinfo:
return np.iinfo(ivy.as_native_dtype(type))
-def result_type(
- *arrays_and_dtypes: Union[(None, mx.ndarray.NDArray, None)]
-) -> ivy.Dtype:
+def result_type(*arrays_and_dtypes: Union[(None, mx.ndarray.NDArray)]) -> ivy.Dtype:
raise IvyNotImplementedException()
diff --git a/ivy/functional/backends/mxnet/device.py b/ivy/functional/backends/mxnet/device.py
index c204921117f8f..ece458d2d7c20 100644
--- a/ivy/functional/backends/mxnet/device.py
+++ b/ivy/functional/backends/mxnet/device.py
@@ -4,6 +4,7 @@
Collection of MXNet general functions, wrapped to fit Ivy syntax and
signature.
"""
+
import mxnet as mx
from typing import Union, Optional
import ivy
@@ -45,9 +46,9 @@ def as_ivy_dev(device):
def as_native_dev(device: str, /):
if isinstance(device, mx.Context):
return device
- if device is None or device.find("cpu") != -1:
+ if device is None or "cpu" in device:
mx_dev = "cpu"
- elif device.find("gpu") != -1:
+ elif "gpu" in device:
mx_dev = "gpu"
else:
raise Exception(f"dev input {device} not supported.")
diff --git a/ivy/functional/backends/mxnet/experimental/activations.py b/ivy/functional/backends/mxnet/experimental/activations.py
index 2ab8f7443e0af..87fa83a7c8ce5 100644
--- a/ivy/functional/backends/mxnet/experimental/activations.py
+++ b/ivy/functional/backends/mxnet/experimental/activations.py
@@ -34,3 +34,9 @@ def selu(x: None, /, *, out: Optional[None] = None) -> None:
def silu(x: None, /, *, out: Optional[None] = None) -> None:
raise IvyNotImplementedException()
+
+
+def celu(
+ x: None, /, *, alpha: float = 0.2, complex_mode="jax", out: Optional[None] = None
+) -> None:
+ return mx.nd.maximum(0, x) + alpha * mx.nd.expm1(mx.nd.minimum(0, x) / alpha)
diff --git a/ivy/functional/backends/mxnet/experimental/elementwise.py b/ivy/functional/backends/mxnet/experimental/elementwise.py
index ce69fe9e91ed3..01287f61474f6 100644
--- a/ivy/functional/backends/mxnet/experimental/elementwise.py
+++ b/ivy/functional/backends/mxnet/experimental/elementwise.py
@@ -1,4 +1,4 @@
-from typing import Union, Optional, Tuple, List
+from typing import Union, Optional, Tuple, List, Sequence
from numbers import Number
import mxnet as mx
@@ -7,6 +7,28 @@
from .. import backend_version
+def amax(
+ x: Union[(None, mx.ndarray.NDArray)],
+ /,
+ *,
+ axis: Optional[Union[int, Sequence[int]]] = None,
+ keepdims: bool = False,
+ out: Optional[Union[(None, mx.ndarray.NDArray)]] = None,
+) -> Union[(None, mx.ndarray.NDArray)]:
+ raise IvyNotImplementedException()
+
+
+def amin(
+ x: Union[(None, mx.ndarray.NDArray)],
+ /,
+ *,
+ axis: Optional[Union[int, Sequence[int]]] = None,
+ keepdims: bool = False,
+ out: Optional[Union[(None, mx.ndarray.NDArray)]] = None,
+) -> Union[(None, mx.ndarray.NDArray)]:
+ raise IvyNotImplementedException()
+
+
@with_supported_dtypes(
{"1.9.1 and below": ("float16", "float32", "float64")},
backend_version,
diff --git a/ivy/functional/backends/mxnet/experimental/gradients.py b/ivy/functional/backends/mxnet/experimental/gradients.py
index 7e1a70137aec5..e952f2264ccbb 100644
--- a/ivy/functional/backends/mxnet/experimental/gradients.py
+++ b/ivy/functional/backends/mxnet/experimental/gradients.py
@@ -1,5 +1,56 @@
+# global
+from typing import Callable
+import mxnet as mx
+
+# local
+import ivy
+from ivy.functional.ivy.gradients import (
+ _flatten_containers,
+ _rebuild_flattened_containers,
+)
from ivy.utils.exceptions import IvyNotImplementedException
def bind_custom_gradient_function(func, custom_grad_fn):
raise IvyNotImplementedException()
+
+
+def vjp(func: Callable, *primals):
+ flattened_primals, ret_idxs = _flatten_containers(primals)
+
+ def grad_fn(*x_in):
+ return _flatten_containers(
+ ivy.to_native(
+ func(
+ *ivy.to_ivy(
+ _rebuild_flattened_containers(x_in, ret_idxs), nested=True
+ )
+ ),
+ nested=True,
+ include_derived=True,
+ )
+ )
+
+ with mx.autograd.record():
+ flat_primals_out, func_ret_idxs = grad_fn(
+ *ivy.to_native(flattened_primals, nested=True)
+ )
+
+ primals_out = _rebuild_flattened_containers(flat_primals_out, func_ret_idxs)
+
+ def vjpfun(x_in):
+ grads = mx.autograd.grad(
+ flat_primals_out,
+ ivy.to_native(flattened_primals, nested=True),
+ head_grads=ivy.to_native(_flatten_containers(x_in)[0], nested=True),
+ )
+
+ return _rebuild_flattened_containers(
+ ivy.to_ivy(grads, nested=True, include_derived=True)
+ )
+
+ return (ivy.to_ivy(primals_out, nested=True, include_derived=True), vjpfun)
+
+
+def jvp(func: Callable, primals, tangents):
+ raise IvyNotImplementedException()
diff --git a/ivy/functional/backends/mxnet/experimental/layers.py b/ivy/functional/backends/mxnet/experimental/layers.py
index 84fab7a4ba311..618f365a0e65b 100644
--- a/ivy/functional/backends/mxnet/experimental/layers.py
+++ b/ivy/functional/backends/mxnet/experimental/layers.py
@@ -1,9 +1,8 @@
# global
-from typing import Optional, Union, Tuple, Literal, Sequence
+from typing import List, Optional, Union, Tuple, Literal, Sequence
import mxnet as mx
# local
-from ivy.func_wrapper import handle_partial_mixed_function
from ivy.utils.exceptions import IvyNotImplementedException
@@ -75,7 +74,7 @@ def avg_pool1d(
x: mx.nd.NDArray,
kernel: Union[int, Tuple[int]],
strides: Union[int, Tuple[int]],
- padding: str,
+ padding: Union[str, int, List[Tuple[int, int]]],
/,
*,
data_format: str = "NWC",
@@ -90,7 +89,7 @@ def avg_pool2d(
x: mx.nd.NDArray,
kernel: Union[int, Tuple[int], Tuple[int, int]],
strides: Union[int, Tuple[int], Tuple[int, int]],
- padding: str,
+ padding: Union[str, int, List[Tuple[int, int]]],
/,
*,
data_format: str = "NHWC",
@@ -106,7 +105,7 @@ def avg_pool3d(
x: mx.nd.NDArray,
kernel: Union[int, Tuple[int], Tuple[int, int, int]],
strides: Union[int, Tuple[int], Tuple[int, int, int]],
- padding: str,
+ padding: Union[str, int, List[Tuple[int, int]]],
/,
*,
data_format: str = "NDHWC",
@@ -190,21 +189,6 @@ def ifft(
raise IvyNotImplementedException()
-@handle_partial_mixed_function(
- lambda *args, mode="linear", scale_factor=None, recompute_scale_factor=None, align_corners=None, **kwargs: ( # noqa: E501
- not align_corners
- and mode
- not in [
- "area",
- "nearest",
- "tf_area",
- "mitchellcubic",
- "gaussian",
- "bicubic",
- ]
- and recompute_scale_factor
- )
-)
def interpolate(
x: mx.nd.NDArray,
size: Union[Sequence[int], int],
@@ -214,11 +198,13 @@ def interpolate(
"linear",
"bilinear",
"trilinear",
+ "nd",
"nearest",
"area",
"nearest_exact",
"tf_area",
- "bicubic_tensorflow" "bicubic",
+ "tf_bicubic",
+ "bicubic",
"mitchellcubic",
"lanczos3",
"lanczos5",
@@ -226,8 +212,20 @@ def interpolate(
] = "linear",
scale_factor: Optional[Union[Sequence[int], int]] = None,
recompute_scale_factor: Optional[bool] = None,
- align_corners: Optional[bool] = None,
+ align_corners: bool = False,
antialias: bool = False,
out: Optional[mx.nd.NDArray] = None,
):
raise IvyNotImplementedException()
+
+
+def rfft(
+ x: mx.nd.NDArray,
+ /,
+ *,
+ n: Optional[int] = None,
+ axis: int = -1,
+ norm: Literal["backward", "ortho", "forward"] = "backward",
+ out: Optional[mx.nd.NDArray] = None,
+) -> mx.nd.NDArray:
+ raise IvyNotImplementedException()
diff --git a/ivy/functional/backends/mxnet/experimental/linear_algebra.py b/ivy/functional/backends/mxnet/experimental/linear_algebra.py
index 8212bc3c54f76..dd31f5eeb070d 100644
--- a/ivy/functional/backends/mxnet/experimental/linear_algebra.py
+++ b/ivy/functional/backends/mxnet/experimental/linear_algebra.py
@@ -82,6 +82,25 @@ def adjoint(
raise IvyNotImplementedException()
+def solve_triangular(
+ x1: Union[(None, mx.ndarray.NDArray)],
+ x2: Union[(None, mx.ndarray.NDArray)],
+ /,
+ *,
+ upper: bool = True,
+ adjoint: bool = False,
+ unit_diagonal: bool = False,
+ out: Optional[Union[(None, mx.ndarray.NDArray)]] = None,
+) -> Union[(None, mx.ndarray.NDArray)]:
+ # Multiplying with a mask matrix can stop gradients on the diagonal.
+ if unit_diagonal:
+ w = mx.eye(x1.shape[-2], batch_shape=x1.shape[:-2], dtype=x1.dtype)
+ x1 = w + (1 - w) * x1
+ # MXNet does not support complex tensors for this operation,
+ # so adjoint always equals transpose.
+ return mx.nd.linalg.trsm(x1, x2, lower=not upper, transpose=adjoint)
+
+
def multi_dot(
x: Sequence[Union[(None, mx.ndarray.NDArray)]],
/,
diff --git a/ivy/functional/backends/mxnet/experimental/manipulation.py b/ivy/functional/backends/mxnet/experimental/manipulation.py
index 66b46e813ae47..45acc1adedc60 100644
--- a/ivy/functional/backends/mxnet/experimental/manipulation.py
+++ b/ivy/functional/backends/mxnet/experimental/manipulation.py
@@ -146,6 +146,19 @@ def atleast_3d(
raise IvyNotImplementedException()
+def take(
+ x: Union[int, List, Union[(None, mx.ndarray.NDArray)]],
+ indices: Union[int, List, Union[(None, mx.ndarray.NDArray)]],
+ /,
+ *,
+ axis: Optional[int] = None,
+ mode: str = "clip",
+ fill_value: Optional[Number] = None,
+ out: Optional[Union[(None, mx.ndarray.NDArray)]] = None,
+) -> Union[(None, mx.ndarray.NDArray)]:
+ raise IvyNotImplementedException()
+
+
def take_along_axis(
arr: Union[(None, mx.ndarray.NDArray)],
indices: Union[(None, mx.ndarray.NDArray)],
diff --git a/ivy/functional/backends/mxnet/general.py b/ivy/functional/backends/mxnet/general.py
index c469b517c47c1..9f8e0e707cc8f 100644
--- a/ivy/functional/backends/mxnet/general.py
+++ b/ivy/functional/backends/mxnet/general.py
@@ -18,7 +18,7 @@ def is_native_array(
if exclusive:
return isinstance(x, mx.ndarray.NDArray)
else:
- return isinstance(x, mx.ndarray.NDArray) or isinstance(x, np.ndarray)
+ return isinstance(x, (mx.ndarray.NDArray, np.ndarray))
def to_numpy(x: mx.ndarray.NDArray, /, *, copy: bool = True) -> np.ndarray:
diff --git a/ivy/functional/backends/mxnet/gradients.py b/ivy/functional/backends/mxnet/gradients.py
index dd3e9041601be..97577e8634e6f 100644
--- a/ivy/functional/backends/mxnet/gradients.py
+++ b/ivy/functional/backends/mxnet/gradients.py
@@ -1,7 +1,7 @@
"""Collection of MXNet gradient functions, wrapped to fit Ivy syntax and signature."""
# global
-from typing import Optional, Sequence, Union
+from typing import Sequence, Union
import mxnet as mx
# local
@@ -26,8 +26,8 @@ def execute_with_gradients(
/,
*,
retain_grads: bool = False,
- xs_grad_idxs: Optional[Sequence[Sequence[Union[str, int]]]] = [[0]],
- ret_grad_idxs: Optional[Sequence[Sequence[Union[str, int]]]] = [[0]],
+ xs_grad_idxs: Sequence[Sequence[Union[str, int]]] = ((0,),),
+ ret_grad_idxs: Sequence[Sequence[Union[str, int]]] = ((0,),),
):
raise IvyNotImplementedException()
diff --git a/ivy/functional/backends/mxnet/layers.py b/ivy/functional/backends/mxnet/layers.py
index 1ae0560d0c356..a30c494799fbd 100644
--- a/ivy/functional/backends/mxnet/layers.py
+++ b/ivy/functional/backends/mxnet/layers.py
@@ -1,4 +1,5 @@
"""Collection of MXNet network layers, wrapped to fit Ivy syntax and signature."""
+
# global
import mxnet as mx
from typing import Optional, Tuple, Union, Sequence
diff --git a/ivy/functional/backends/mxnet/linear_algebra.py b/ivy/functional/backends/mxnet/linear_algebra.py
index 5077fd3a24bc2..e7717406c1a23 100644
--- a/ivy/functional/backends/mxnet/linear_algebra.py
+++ b/ivy/functional/backends/mxnet/linear_algebra.py
@@ -1,6 +1,11 @@
+# global
+
import mxnet as mx
-from typing import Union, Optional, Tuple, Literal, List, NamedTuple, Sequence
+from typing import Union, Optional, Tuple, Literal, List, Sequence
+from collections import namedtuple
+
+# local
from ivy import inf
from ivy.utils.exceptions import IvyNotImplementedException
@@ -184,8 +189,10 @@ def qr(
out: Optional[
Tuple[(Union[(None, mx.ndarray.NDArray)], Union[(None, mx.ndarray.NDArray)])]
] = None,
-) -> NamedTuple:
- raise IvyNotImplementedException()
+) -> Tuple[(Union[(None, mx.ndarray.NDArray)], Union[(None, mx.ndarray.NDArray)])]:
+ res = namedtuple("qr", ["Q", "R"])
+ q, r = mx.np.linalg.qr(x, mode=mode)
+ return res(q, r)
def slogdet(
@@ -221,8 +228,10 @@ def svdvals(
x: Union[(None, mx.ndarray.NDArray)],
/,
*,
+ driver: Optional[str] = None,
out: Optional[Union[(None, mx.ndarray.NDArray)]] = None,
) -> Union[(None, mx.ndarray.NDArray)]:
+ # TODO: handling the driver argument
raise IvyNotImplementedException()
diff --git a/ivy/functional/backends/mxnet/random.py b/ivy/functional/backends/mxnet/random.py
index 4f1e25f4763d5..875fc1ce304cb 100644
--- a/ivy/functional/backends/mxnet/random.py
+++ b/ivy/functional/backends/mxnet/random.py
@@ -4,6 +4,7 @@
Collection of MXNet random functions, wrapped to fit Ivy syntax and
signature.
"""
+
import mxnet as mx
from typing import Optional, Union, Sequence
import ivy
diff --git a/ivy/functional/backends/mxnet/set.py b/ivy/functional/backends/mxnet/set.py
index 5b72d506eb490..cb9a9cc9906e6 100644
--- a/ivy/functional/backends/mxnet/set.py
+++ b/ivy/functional/backends/mxnet/set.py
@@ -24,7 +24,7 @@ def unique_counts(
def unique_inverse(
- x: Union[(None, mx.ndarray.NDArray)], /
+ x: Union[(None, mx.ndarray.NDArray)], /, *, axis: Optional[int] = None
) -> Tuple[(Union[(None, mx.ndarray.NDArray)], Union[(None, mx.ndarray.NDArray)])]:
raise IvyNotImplementedException()
diff --git a/ivy/functional/backends/numpy/__init__.py b/ivy/functional/backends/numpy/__init__.py
index 8df8e90dbf269..163da57f6a834 100644
--- a/ivy/functional/backends/numpy/__init__.py
+++ b/ivy/functional/backends/numpy/__init__.py
@@ -34,12 +34,10 @@ def rep_method(self, ufunc, method, *inputs, **kwargs):
"bitwise_and": "bitwise_and",
"matmul": "matmul",
"power": "pow",
- "divide": "divide",
"subtract": "subtract",
"add": "add",
- "not_equal": "not_equal",
}
- if ufunc.__name__ in methods.keys():
+ if ufunc.__name__ in methods:
return eval("ivy." + methods[ufunc.__name__] + "(*inputs, **kwargs)")
return func(self, ufunc, method, *inputs, **kwargs)
@@ -83,7 +81,7 @@ def rep_method(self, ufunc, method, *inputs, **kwargs):
# update these to add new dtypes
valid_dtypes = {
- "1.26.0 and below": (
+ "1.26.1 and below": (
ivy.int8,
ivy.int16,
ivy.int32,
@@ -101,7 +99,7 @@ def rep_method(self, ufunc, method, *inputs, **kwargs):
)
}
valid_numeric_dtypes = {
- "1.26.0 and below": (
+ "1.26.1 and below": (
ivy.int8,
ivy.int16,
ivy.int32,
@@ -118,7 +116,7 @@ def rep_method(self, ufunc, method, *inputs, **kwargs):
)
}
valid_int_dtypes = {
- "1.26.0 and below": (
+ "1.26.1 and below": (
ivy.int8,
ivy.int16,
ivy.int32,
@@ -129,11 +127,11 @@ def rep_method(self, ufunc, method, *inputs, **kwargs):
ivy.uint64,
)
}
-valid_float_dtypes = {"1.26.0 and below": (ivy.float16, ivy.float32, ivy.float64)}
+valid_float_dtypes = {"1.26.1 and below": (ivy.float16, ivy.float32, ivy.float64)}
valid_uint_dtypes = {
- "1.26.0 and below": (ivy.uint8, ivy.uint16, ivy.uint32, ivy.uint64)
+ "1.26.1 and below": (ivy.uint8, ivy.uint16, ivy.uint32, ivy.uint64)
}
-valid_complex_dtypes = {"1.26.0 and below": (ivy.complex64, ivy.complex128)}
+valid_complex_dtypes = {"1.26.1 and below": (ivy.complex64, ivy.complex128)}
# leave these untouched
valid_dtypes = _dtype_from_version(valid_dtypes, backend_version)
@@ -145,12 +143,12 @@ def rep_method(self, ufunc, method, *inputs, **kwargs):
# invalid data types
# update these to add new dtypes
-invalid_dtypes = {"1.26.0 and below": (ivy.bfloat16,)}
-invalid_numeric_dtypes = {"1.26.0 and below": (ivy.bfloat16,)}
-invalid_int_dtypes = {"1.26.0 and below": ()}
-invalid_float_dtypes = {"1.26.0 and below": (ivy.bfloat16,)}
-invalid_uint_dtypes = {"1.26.0 and below": ()}
-invalid_complex_dtypes = {"1.26.0 and below": ()}
+invalid_dtypes = {"1.26.1 and below": (ivy.bfloat16,)}
+invalid_numeric_dtypes = {"1.26.1 and below": (ivy.bfloat16,)}
+invalid_int_dtypes = {"1.26.1 and below": ()}
+invalid_float_dtypes = {"1.26.1 and below": (ivy.bfloat16,)}
+invalid_uint_dtypes = {"1.26.1 and below": ()}
+invalid_complex_dtypes = {"1.26.1 and below": ()}
# leave these untouched
diff --git a/ivy/functional/backends/numpy/creation.py b/ivy/functional/backends/numpy/creation.py
index 1e7ad6d3aaa0d..4ce48b5332d57 100644
--- a/ivy/functional/backends/numpy/creation.py
+++ b/ivy/functional/backends/numpy/creation.py
@@ -6,7 +6,6 @@
# local
import ivy
-from ivy.functional.backends.numpy.device import _to_device
from ivy.functional.ivy.creation import (
_asarray_to_native_arrays_and_back,
_asarray_infer_device,
@@ -35,7 +34,7 @@ def arange(
) -> np.ndarray:
if dtype:
dtype = as_native_dtype(dtype)
- res = _to_device(np.arange(start, stop, step, dtype=dtype), device=device)
+ res = np.arange(start, stop, step, dtype=dtype)
if not dtype:
if res.dtype == np.float64:
return res.astype(np.float32)
@@ -60,7 +59,7 @@ def asarray(
device: str = None,
out: Optional[np.ndarray] = None,
) -> np.ndarray:
- ret = _to_device(np.asarray(obj, dtype=dtype), device=device)
+ ret = np.asarray(obj, dtype=dtype)
return np.copy(ret) if copy else ret
@@ -71,7 +70,7 @@ def empty(
device: str = None,
out: Optional[np.ndarray] = None,
) -> np.ndarray:
- return _to_device(np.empty(shape, dtype), device=device)
+ return np.empty(shape, dtype)
def empty_like(
@@ -82,7 +81,7 @@ def empty_like(
device: str = None,
out: Optional[np.ndarray] = None,
) -> np.ndarray:
- return _to_device(np.empty_like(x, dtype=dtype), device=device)
+ return np.empty_like(x, dtype=dtype)
def eye(
@@ -100,20 +99,32 @@ def eye(
n_cols = n_rows
i = np.eye(n_rows, n_cols, k, dtype)
if batch_shape is None:
- return _to_device(i, device=device)
+ return i
else:
reshape_dims = [1] * len(batch_shape) + [n_rows, n_cols]
tile_dims = list(batch_shape) + [1, 1]
return_mat = np.tile(np.reshape(i, reshape_dims), tile_dims)
- return _to_device(return_mat, device=device)
+ return return_mat
def to_dlpack(x, /, *, out: Optional[np.ndarray] = None):
return x.__dlpack__()
+class _dlpack_wrapper:
+ def __init__(self, capsule) -> None:
+ self.capsule = capsule
+
+ def dlpack(self):
+ return self.capsule
+
+
def from_dlpack(x, /, *, out: Optional[np.ndarray] = None):
- return np.from_dlpack(x)
+ if not hasattr(x, "__dlpack__"):
+ capsule = _dlpack_wrapper(x)
+ else:
+ capsule = x
+ return np.from_dlpack(capsule)
def full(
@@ -125,10 +136,7 @@ def full(
out: Optional[np.ndarray] = None,
) -> np.ndarray:
dtype = ivy.default_dtype(dtype=dtype, item=fill_value, as_native=True)
- return _to_device(
- np.full(shape, fill_value, dtype),
- device=device,
- )
+ return np.full(shape, fill_value, dtype)
def full_like(
@@ -140,7 +148,7 @@ def full_like(
device: str = None,
out: Optional[np.ndarray] = None,
) -> np.ndarray:
- return _to_device(np.full_like(x, fill_value, dtype=dtype), device=device)
+ return np.full_like(x, fill_value, dtype=dtype)
def linspace(
@@ -165,7 +173,7 @@ def linspace(
and (not isinstance(stop, np.ndarray))
):
ans[0] = start
- return _to_device(ans, device=device)
+ return ans
def meshgrid(
@@ -184,7 +192,7 @@ def ones(
device: str = None,
out: Optional[np.ndarray] = None,
) -> np.ndarray:
- return _to_device(np.ones(shape, dtype), device=device)
+ return np.ones(shape, dtype)
def ones_like(
@@ -195,7 +203,7 @@ def ones_like(
device: str = None,
out: Optional[np.ndarray] = None,
) -> np.ndarray:
- return _to_device(np.ones_like(x, dtype=dtype), device=device)
+ return np.ones_like(x, dtype=dtype)
def tril(
@@ -217,7 +225,7 @@ def zeros(
device: str = None,
out: Optional[np.ndarray] = None,
) -> np.ndarray:
- return _to_device(np.zeros(shape, dtype), device=device)
+ return np.zeros(shape, dtype)
def zeros_like(
@@ -228,7 +236,7 @@ def zeros_like(
device: str = None,
out: Optional[np.ndarray] = None,
) -> np.ndarray:
- return _to_device(np.zeros_like(x, dtype=dtype), device=device)
+ return np.zeros_like(x, dtype=dtype)
# Extra #
@@ -304,6 +312,4 @@ def triu_indices(
*,
device: str = None,
) -> Tuple[np.ndarray]:
- return tuple(
- _to_device(np.asarray(np.triu_indices(n=n_rows, k=k, m=n_cols)), device=device)
- )
+ return tuple(np.asarray(np.triu_indices(n=n_rows, k=k, m=n_cols)))
diff --git a/ivy/functional/backends/numpy/data_type.py b/ivy/functional/backends/numpy/data_type.py
index 7d167cc2280d7..4246f695a2493 100644
--- a/ivy/functional/backends/numpy/data_type.py
+++ b/ivy/functional/backends/numpy/data_type.py
@@ -133,7 +133,7 @@ def broadcast_arrays(*arrays: np.ndarray) -> List[np.ndarray]:
raise ivy.utils.exceptions.IvyBroadcastShapeError(e)
-@with_unsupported_dtypes({"1.26.0 and below": ("complex",)}, backend_version)
+@with_unsupported_dtypes({"1.26.1 and below": ("complex",)}, backend_version)
def broadcast_to(
x: np.ndarray,
/,
@@ -216,7 +216,7 @@ def as_ivy_dtype(
)
-@with_unsupported_dtypes({"1.26.0 and below": ("bfloat16",)}, backend_version)
+@with_unsupported_dtypes({"1.26.1 and below": ("bfloat16",)}, backend_version)
def as_native_dtype(dtype_in: Union[np.dtype, str, bool, int, float], /) -> np.dtype:
if dtype_in is int:
return ivy.default_int_dtype(as_native=True)
diff --git a/ivy/functional/backends/numpy/device.py b/ivy/functional/backends/numpy/device.py
index abf1cc2c9156b..d636feb8d8f25 100644
--- a/ivy/functional/backends/numpy/device.py
+++ b/ivy/functional/backends/numpy/device.py
@@ -3,6 +3,7 @@
# global
import os
import time
+import logging
import numpy as np
from typing import Union, Optional, Any
@@ -18,10 +19,18 @@ def dev(x: np.ndarray, /, *, as_native: bool = False) -> Union[ivy.Device, str]:
def as_ivy_dev(device: str, /):
+ if "gpu" in device:
+ logging.warning(
+ "Native Numpy does not support GPU placement, consider using Jax instead"
+ )
return ivy.Device("cpu")
def as_native_dev(device: str, /):
+ if "gpu" in device:
+ logging.warning(
+ "Native Numpy does not support GPU placement, consider using Jax instead"
+ )
return "cpu"
@@ -41,25 +50,6 @@ def gpu_is_available() -> bool:
return False
-# private version of to_device to be used in backend implementations
-def _to_device(x: np.ndarray, device=None) -> np.ndarray:
- """Private version of `to_device` to be used in backend implementations."""
- if device is not None:
- if "gpu" in device:
- raise ivy.utils.exceptions.IvyException(
- "Native Numpy does not support GPU placement, "
- "consider using Jax instead"
- )
- elif "cpu" in device:
- pass
- else:
- raise ivy.utils.exceptions.IvyException(
- "Invalid device specified, must be in the form "
- "[ 'cpu:idx' | 'gpu:idx' ], but found {}".format(device)
- )
- return x
-
-
def to_device(
x: np.ndarray,
device: str,
@@ -70,18 +60,6 @@ def to_device(
) -> np.ndarray:
if device is not None:
device = as_native_dev(device)
- if "gpu" in device:
- raise ivy.utils.exceptions.IvyException(
- "Native Numpy does not support GPU placement, "
- "consider using Jax instead"
- )
- elif "cpu" in device:
- pass
- else:
- raise ivy.utils.exceptions.IvyException(
- "Invalid device specified, must be in the form "
- "[ 'cpu:idx' | 'gpu:idx' ], but found {}".format(device)
- )
return x
diff --git a/ivy/functional/backends/numpy/elementwise.py b/ivy/functional/backends/numpy/elementwise.py
index 92bcc24579ead..9a6dfde82b98a 100644
--- a/ivy/functional/backends/numpy/elementwise.py
+++ b/ivy/functional/backends/numpy/elementwise.py
@@ -83,7 +83,7 @@ def atan(x: np.ndarray, /, *, out: Optional[np.ndarray] = None) -> np.ndarray:
@_scalar_output_to_0d_array
-@with_unsupported_dtypes({"1.26.0 and below": ("complex",)}, backend_version)
+@with_unsupported_dtypes({"1.26.1 and below": ("complex",)}, backend_version)
def atan2(
x1: np.ndarray, x2: np.ndarray, /, *, out: Optional[np.ndarray] = None
) -> np.ndarray:
@@ -103,7 +103,7 @@ def atanh(x: np.ndarray, /, *, out: Optional[np.ndarray] = None) -> np.ndarray:
@_scalar_output_to_0d_array
-@with_unsupported_dtypes({"1.26.0 and below": ("complex",)}, backend_version)
+@with_unsupported_dtypes({"1.26.1 and below": ("complex",)}, backend_version)
def bitwise_and(
x1: Union[int, bool, np.ndarray],
x2: Union[int, bool, np.ndarray],
@@ -119,7 +119,7 @@ def bitwise_and(
@_scalar_output_to_0d_array
-@with_unsupported_dtypes({"1.26.0 and below": ("complex",)}, backend_version)
+@with_unsupported_dtypes({"1.26.1 and below": ("complex",)}, backend_version)
def bitwise_invert(
x: Union[int, bool, np.ndarray], /, *, out: Optional[np.ndarray] = None
) -> np.ndarray:
@@ -130,7 +130,7 @@ def bitwise_invert(
@_scalar_output_to_0d_array
-@with_unsupported_dtypes({"1.26.0 and below": ("complex",)}, backend_version)
+@with_unsupported_dtypes({"1.26.1 and below": ("complex",)}, backend_version)
def bitwise_left_shift(
x1: Union[int, bool, np.ndarray],
x2: Union[int, bool, np.ndarray],
@@ -146,7 +146,7 @@ def bitwise_left_shift(
@_scalar_output_to_0d_array
-@with_unsupported_dtypes({"1.26.0 and below": ("complex",)}, backend_version)
+@with_unsupported_dtypes({"1.26.1 and below": ("complex",)}, backend_version)
def bitwise_or(
x1: Union[int, bool, np.ndarray],
x2: Union[int, bool, np.ndarray],
@@ -162,7 +162,7 @@ def bitwise_or(
@_scalar_output_to_0d_array
-@with_unsupported_dtypes({"1.26.0 and below": ("complex",)}, backend_version)
+@with_unsupported_dtypes({"1.26.1 and below": ("complex",)}, backend_version)
def bitwise_right_shift(
x1: Union[int, bool, np.ndarray],
x2: Union[int, bool, np.ndarray],
@@ -178,7 +178,7 @@ def bitwise_right_shift(
@_scalar_output_to_0d_array
-@with_unsupported_dtypes({"1.26.0 and below": ("complex",)}, backend_version)
+@with_unsupported_dtypes({"1.26.1 and below": ("complex",)}, backend_version)
def bitwise_xor(
x1: Union[int, bool, np.ndarray],
x2: Union[int, bool, np.ndarray],
@@ -193,7 +193,7 @@ def bitwise_xor(
bitwise_xor.support_native_out = True
-@with_unsupported_dtypes({"1.26.0 and below": ("complex",)}, backend_version)
+@with_unsupported_dtypes({"1.26.1 and below": ("complex",)}, backend_version)
@_scalar_output_to_0d_array
def ceil(x: np.ndarray, /, *, out: Optional[np.ndarray] = None) -> np.ndarray:
if "int" in str(x.dtype):
@@ -216,7 +216,7 @@ def cos(x: np.ndarray, /, *, out: Optional[np.ndarray] = None) -> np.ndarray:
cos.support_native_out = True
-@with_unsupported_dtypes({"1.26.0 and below": ("float16",)}, backend_version)
+@with_unsupported_dtypes({"1.26.1 and below": ("float16",)}, backend_version)
@_scalar_output_to_0d_array
def cosh(x: np.ndarray, /, *, out: Optional[np.ndarray] = None) -> np.ndarray:
return np.cosh(x, out=out)
@@ -289,7 +289,7 @@ def expm1(x: np.ndarray, /, *, out: Optional[np.ndarray] = None) -> np.ndarray:
@_scalar_output_to_0d_array
-@with_unsupported_dtypes({"1.26.0 and below": ("complex",)}, backend_version)
+@with_unsupported_dtypes({"1.26.1 and below": ("complex",)}, backend_version)
def floor(x: np.ndarray, /, *, out: Optional[np.ndarray] = None) -> np.ndarray:
if "int" in str(x.dtype):
ret = np.copy(x)
@@ -304,7 +304,7 @@ def floor(x: np.ndarray, /, *, out: Optional[np.ndarray] = None) -> np.ndarray:
@_scalar_output_to_0d_array
-@with_unsupported_dtypes({"1.26.0 and below": ("complex",)}, backend_version)
+@with_unsupported_dtypes({"1.26.1 and below": ("complex",)}, backend_version)
def floor_divide(
x1: Union[float, np.ndarray],
x2: Union[float, np.ndarray],
@@ -486,7 +486,7 @@ def log2(x: np.ndarray, /, *, out: Optional[np.ndarray] = None) -> np.ndarray:
@_scalar_output_to_0d_array
-@with_unsupported_dtypes({"1.26.0 and below": ("complex",)}, backend_version)
+@with_unsupported_dtypes({"1.26.1 and below": ("complex",)}, backend_version)
def logaddexp(
x1: np.ndarray, x2: np.ndarray, /, *, out: Optional[np.ndarray] = None
) -> np.ndarray:
@@ -623,7 +623,7 @@ def pow(
@_scalar_output_to_0d_array
-@with_unsupported_dtypes({"1.26.0 and below": ("complex",)}, backend_version)
+@with_unsupported_dtypes({"1.26.1 and below": ("complex",)}, backend_version)
def remainder(
x1: Union[float, np.ndarray],
x2: Union[float, np.ndarray],
@@ -865,7 +865,7 @@ def reciprocal(
@_scalar_output_to_0d_array
-@with_unsupported_dtypes({"1.26.0 and below": ("complex",)}, backend_version)
+@with_unsupported_dtypes({"1.26.1 and below": ("complex",)}, backend_version)
def deg2rad(x: np.ndarray, /, *, out: Optional[np.ndarray] = None) -> np.ndarray:
return np.deg2rad(x, out=out)
@@ -874,7 +874,7 @@ def deg2rad(x: np.ndarray, /, *, out: Optional[np.ndarray] = None) -> np.ndarray
@_scalar_output_to_0d_array
-@with_unsupported_dtypes({"1.26.0 and below": ("complex",)}, backend_version)
+@with_unsupported_dtypes({"1.26.1 and below": ("complex",)}, backend_version)
def rad2deg(x: np.ndarray, /, *, out: Optional[np.ndarray] = None) -> np.ndarray:
return np.rad2deg(x, out=out)
@@ -891,7 +891,7 @@ def isreal(x: np.ndarray, /, *, out: Optional[np.ndarray] = None) -> np.ndarray:
@_scalar_output_to_0d_array
-@with_unsupported_dtypes({"1.26.0 and below": ("complex",)}, backend_version)
+@with_unsupported_dtypes({"1.26.1 and below": ("complex",)}, backend_version)
def fmod(
x1: np.ndarray,
x2: np.ndarray,
diff --git a/ivy/functional/backends/numpy/experimental/activations.py b/ivy/functional/backends/numpy/experimental/activations.py
index 52019b6adf626..721365d16b7b5 100644
--- a/ivy/functional/backends/numpy/experimental/activations.py
+++ b/ivy/functional/backends/numpy/experimental/activations.py
@@ -6,7 +6,9 @@
# local
import ivy
from ivy.functional.backends.numpy.helpers import _scalar_output_to_0d_array
-from ivy.func_wrapper import with_unsupported_dtypes
+from ivy.func_wrapper import (
+ with_unsupported_dtypes,
+)
from . import backend_version
@@ -53,7 +55,7 @@ def relu6(
relu6.support_native_out = True
-@with_unsupported_dtypes({"1.26.0 and below": ("bool",)}, backend_version)
+@with_unsupported_dtypes({"1.26.1 and below": ("bool",)}, backend_version)
@_scalar_output_to_0d_array
def logsigmoid(
input: np.ndarray, /, *, complex_mode="jax", out: Optional[np.ndarray] = None
@@ -102,6 +104,20 @@ def elu(
elu.support_native_out = True
+@_scalar_output_to_0d_array
+def celu(
+ x: np.ndarray,
+ /,
+ *,
+ alpha: float = 1.0,
+ complex_mode="jax",
+ out: Optional[np.ndarray] = None,
+) -> np.ndarray:
+ return (np.maximum(0, x) + alpha * np.expm1(np.minimum(0, x) / alpha)).astype(
+ x.dtype
+ )
+
+
@with_unsupported_dtypes({"1.25.2 and below": ("float16", "bfloat16")}, backend_version)
@_scalar_output_to_0d_array
def hardtanh(
@@ -119,3 +135,70 @@ def hardtanh(
hardtanh.support_native_out = True
+
+
+@_scalar_output_to_0d_array
+def tanhshrink(x: np.ndarray, /, *, out: Optional[np.ndarray] = None) -> np.ndarray:
+ ret = np.subtract(x, np.tanh(x))
+ if ivy.exists(out):
+ return ivy.inplace_update(out, ret).astype(x.dtype)
+ return ivy.astype(ret, x.dtype)
+
+
+tanhshrink.support_native_out = True
+
+
+@_scalar_output_to_0d_array
+def threshold(
+ x: np.ndarray,
+ /,
+ *,
+ threshold: float,
+ value: float,
+ out: Optional[np.ndarray] = None,
+) -> np.ndarray:
+ ret = np.where(x > threshold, x, value)
+ if ivy.exists(out):
+ return ivy.inplace_update(out, ret).astype(x.dtype)
+ return ivy.astype(ret, x.dtype)
+
+
+threshold.support_native_out = True
+
+
+@_scalar_output_to_0d_array
+def softshrink(
+ x: np.ndarray, /, *, lambd: float = 0.5, out: Optional[np.ndarray] = None
+) -> np.ndarray:
+ ret = np.where(x > lambd, x - lambd, np.where(x < -lambd, x + lambd, 0))
+ if ivy.exists(out):
+ return ivy.inplace_update(out, ret).astype(x.dtype)
+ return ivy.astype(ret, x.dtype)
+
+
+softshrink.support_native_out = True
+
+
+@_scalar_output_to_0d_array
+def scaled_tanh(
+ x: np.ndarray,
+ /,
+ *,
+ alpha: float = 1.7159,
+ beta: float = 0.67,
+ out: Optional[np.ndarray] = None,
+) -> np.ndarray:
+ return alpha * np.tanh(beta * x)
+
+
+@_scalar_output_to_0d_array
+def hardshrink(
+ x: np.ndarray, /, *, lambd: float = 0.5, out: Optional[np.ndarray] = None
+) -> np.ndarray:
+ ret = np.where(x > lambd, x, np.where(x < -lambd, x, 0))
+ if ivy.exists(out):
+ return ivy.inplace_update(out, ret).astype(x.dtype)
+ return ivy.astype(ret, x.dtype)
+
+
+hardshrink.support_native_out = True
diff --git a/ivy/functional/backends/numpy/experimental/creation.py b/ivy/functional/backends/numpy/experimental/creation.py
index fd53b780a2802..e6c4b5a064779 100644
--- a/ivy/functional/backends/numpy/experimental/creation.py
+++ b/ivy/functional/backends/numpy/experimental/creation.py
@@ -4,7 +4,6 @@
import numpy as np
# local
-from ivy.functional.backends.numpy.device import _to_device
import ivy
# Array API Standard #
@@ -35,9 +34,7 @@ def tril_indices(
*,
device: str = None,
) -> Tuple[np.ndarray, ...]:
- return tuple(
- _to_device(np.asarray(np.tril_indices(n=n_rows, k=k, m=n_cols)), device=device)
- )
+ return tuple(np.asarray(np.tril_indices(n=n_rows, k=k, m=n_cols)))
def hann_window(
@@ -92,7 +89,7 @@ def unsorted_segment_min(
segment_ids: np.ndarray,
num_segments: int,
) -> np.ndarray:
- ivy.utils.assertions.check_unsorted_segment_min_valid_params(
+ ivy.utils.assertions.check_unsorted_segment_valid_params(
data, segment_ids, num_segments
)
@@ -146,7 +143,7 @@ def unsorted_segment_sum(
# check should be same
# Might require to change the assertion function name to
# check_unsorted_segment_valid_params
- ivy.utils.assertions.check_unsorted_segment_min_valid_params(
+ ivy.utils.assertions.check_unsorted_segment_valid_params(
data, segment_ids, num_segments
)
@@ -197,10 +194,46 @@ def hz_to_mel(f):
dtype=np.float32,
)
mel_edges = np.stack([mel_edges[i : i + 3] for i in range(num_mel_bins)])
- lower_edge_mel, center_mel, upper_edge_mel = (
+ lower_edge_mel, center_mel, upper_edge_mel = [
t.reshape((1, num_mel_bins)) for t in np.split(mel_edges, 3, axis=1)
- )
+ ]
lower_slopes = (spec_bin_mels - lower_edge_mel) / (center_mel - lower_edge_mel)
upper_slopes = (upper_edge_mel - spec_bin_mels) / (upper_edge_mel - center_mel)
mel_weights = np.maximum(zero, np.minimum(lower_slopes, upper_slopes))
return np.pad(mel_weights, [[1, 0], [0, 0]])
+
+
+def unsorted_segment_mean(
+ data: np.ndarray,
+ segment_ids: np.ndarray,
+ num_segments: int,
+) -> np.ndarray:
+ ivy.utils.assertions.check_unsorted_segment_valid_params(
+ data, segment_ids, num_segments
+ )
+
+ if len(segment_ids) == 0:
+ # If segment_ids is empty, return an empty array of the correct shape
+ return np.zeros((num_segments,) + data.shape[1:], dtype=data.dtype)
+
+ # Initialize an array to store the sum of elements for each segment
+ res = np.zeros((num_segments,) + data.shape[1:], dtype=data.dtype)
+
+ # Initialize an array to keep track of the number of elements in each segment
+ counts = np.zeros(num_segments, dtype=np.int64)
+
+ for i in range(len(segment_ids)):
+ seg_id = segment_ids[i]
+ if seg_id < num_segments:
+ res[seg_id] += data[i]
+ counts[seg_id] += 1
+
+ return res / counts[:, np.newaxis]
+
+
+def polyval(coeffs: np.ndarray, x: np.ndarray) -> np.ndarray:
+ with ivy.PreciseMode(True):
+ promoted_type = ivy.promote_types(ivy.dtype(coeffs[0]), ivy.dtype(x[0]))
+ result = np.polyval(coeffs, x)
+ result = np.asarray(result, np.dtype(promoted_type))
+ return result
diff --git a/ivy/functional/backends/numpy/experimental/elementwise.py b/ivy/functional/backends/numpy/experimental/elementwise.py
index bb93091034706..ca0ecc4bad310 100644
--- a/ivy/functional/backends/numpy/experimental/elementwise.py
+++ b/ivy/functional/backends/numpy/experimental/elementwise.py
@@ -1,4 +1,4 @@
-from typing import Optional, Union, Tuple, List
+from typing import Optional, Union, Tuple, List, Sequence
import numpy as np
import numpy.typing as npt
@@ -9,8 +9,40 @@
from . import backend_version
+def amax(
+ x: np.ndarray,
+ /,
+ *,
+ axis: Optional[Union[int, Sequence[int]]] = None,
+ keepdims: bool = False,
+ out: Optional[np.ndarray] = None,
+) -> np.ndarray:
+ axis = tuple(axis) if isinstance(axis, list) else axis
+ ret = np.amax(a=x, axis=axis, out=out, keepdims=keepdims)
+ return np.asarray(ret) if np.isscalar(ret) else ret
+
+
+amax.support_native_out = True
+
+
+def amin(
+ x: np.ndarray,
+ /,
+ *,
+ axis: Optional[Union[int, Sequence[int]]] = None,
+ keepdims: bool = False,
+ out: Optional[np.ndarray] = None,
+) -> np.ndarray:
+ axis = tuple(axis) if isinstance(axis, list) else axis
+ ret = np.amin(a=x, axis=axis, out=out, keepdims=keepdims)
+ return np.asarray(ret) if np.isscalar(ret) else ret
+
+
+amin.support_native_out = True
+
+
@_scalar_output_to_0d_array
-@with_unsupported_dtypes({"1.26.0 and below": ("bfloat16",)}, backend_version)
+@with_unsupported_dtypes({"1.26.1 and below": ("bfloat16",)}, backend_version)
def sinc(x: np.ndarray, /, *, out: Optional[np.ndarray] = None) -> np.ndarray:
return np.sinc(x).astype(x.dtype)
@@ -406,7 +438,7 @@ def digamma(
def sinpi(x):
y = np.abs(x) % 2.0
n = np.round(2.0 * y)
- assert 0 <= n and n <= 4
+ assert n >= 0 and n <= 4
if n == 0:
r = np.sin(np.pi * y)
@@ -543,7 +575,7 @@ def _EvaluatePolynomial(x, coefficients):
return poly
-# TODO: Remove this once native function is avilable.
+# TODO: Remove this once native function is available.
# Compute an approximation of the error function complement (1 - erf(x)).
def erfc(
x: np.ndarray,
diff --git a/ivy/functional/backends/numpy/experimental/general.py b/ivy/functional/backends/numpy/experimental/general.py
index 5dbddeeaa2821..74fe96dd63423 100644
--- a/ivy/functional/backends/numpy/experimental/general.py
+++ b/ivy/functional/backends/numpy/experimental/general.py
@@ -7,7 +7,7 @@
from ivy import with_unsupported_dtypes
-@with_unsupported_dtypes({"1.26.0 and below": ("complex",)}, backend_version)
+@with_unsupported_dtypes({"1.26.1 and below": ("complex",)}, backend_version)
def reduce(
operand: np.ndarray,
init_value: Union[int, float],
diff --git a/ivy/functional/backends/numpy/experimental/gradients.py b/ivy/functional/backends/numpy/experimental/gradients.py
index eeeb12269933d..e673c5cbdf025 100644
--- a/ivy/functional/backends/numpy/experimental/gradients.py
+++ b/ivy/functional/backends/numpy/experimental/gradients.py
@@ -1,5 +1,8 @@
# global
import logging
+from typing import Callable
+
+# local
def bind_custom_gradient_function(func, custom_grad_fn):
@@ -8,3 +11,18 @@ def bind_custom_gradient_function(func, custom_grad_fn):
"has no effect on the array, as gradients are not supported in the first place."
)
return func
+
+
+def vjp(func: Callable, *primals):
+ logging.warning(
+ "NumPy does not support autograd, 'vjp' returns None in place of `vjpfun`."
+ )
+ return func(*primals), None
+
+
+def jvp(func: Callable, primals, tangents):
+ logging.warning(
+ "NumPy does not support autograd, "
+ "'jvp' returns None in place of `tangents_out`."
+ )
+ return func(*primals), None
diff --git a/ivy/functional/backends/numpy/experimental/layers.py b/ivy/functional/backends/numpy/experimental/layers.py
index 6ba201c5f189e..7f11ed19c4848 100644
--- a/ivy/functional/backends/numpy/experimental/layers.py
+++ b/ivy/functional/backends/numpy/experimental/layers.py
@@ -44,7 +44,7 @@ def max_pool1d(
) -> np.ndarray:
dims = 1
kernel, strides, padding, dilation = _validate_max_pool_params(
- kernel, strides, padding, dilation, ceil_mode, dims=dims
+ kernel, strides, padding, dilation, ceil_mode, dims, data_format
)
if data_format == "NCW":
@@ -97,7 +97,7 @@ def max_pool1d(
)
else:
if isinstance(padding, list) and any(
- [item != 0 for sublist in padding for item in sublist]
+ item != 0 for sublist in padding for item in sublist
):
raise NotImplementedError(
"Nonzero explicit padding is not supported for depthwise max pooling"
@@ -146,7 +146,7 @@ def max_pool2d(
) -> np.ndarray:
dims = 2
kernel, strides, padding, dilation = _validate_max_pool_params(
- kernel, strides, padding, dilation, ceil_mode, dims=dims
+ kernel, strides, padding, dilation, ceil_mode, dims, data_format
)
if data_format == "NCHW":
@@ -203,7 +203,7 @@ def max_pool2d(
)
else:
if isinstance(padding, list) and any(
- [item != 0 for sublist in padding for item in sublist]
+ item != 0 for sublist in padding for item in sublist
):
raise NotImplementedError(
"Nonzero explicit padding is not supported for depthwise max pooling"
@@ -256,7 +256,7 @@ def max_pool3d(
) -> np.ndarray:
dims = 3
kernel, strides, padding, dilation = _validate_max_pool_params(
- kernel, strides, padding, dilation, ceil_mode, dims=dims
+ kernel, strides, padding, dilation, ceil_mode, dims, data_format
)
if data_format == "NCDHW":
@@ -317,7 +317,7 @@ def max_pool3d(
)
else:
if isinstance(padding, list) and any(
- [item != 0 for sublist in padding for item in sublist]
+ item != 0 for sublist in padding for item in sublist
):
raise NotImplementedError(
"Nonzero explicit padding is not supported for depthwise max pooling"
@@ -386,7 +386,7 @@ def avg_pool1d(
x: np.ndarray,
kernel: Union[int, Tuple[int]],
strides: Union[int, Tuple[int]],
- padding: str,
+ padding: Union[str, int, List[Tuple[int, int]]],
/,
*,
data_format: str = "NWC",
@@ -470,7 +470,7 @@ def avg_pool2d(
x: np.ndarray,
kernel: Union[int, Tuple[int], Tuple[int, int]],
strides: Union[int, Tuple[int], Tuple[int, int]],
- padding: str,
+ padding: Union[str, int, List[Tuple[int, int]]],
/,
*,
data_format: str = "NHWC",
@@ -577,7 +577,7 @@ def avg_pool3d(
x: np.ndarray,
kernel: Union[int, Tuple[int], Tuple[int, int, int]],
strides: Union[int, Tuple[int], Tuple[int, int, int]],
- padding: str,
+ padding: Union[str, int, List[Tuple[int, int]]],
/,
*,
data_format: str = "NDHWC",
@@ -717,7 +717,7 @@ def fft(
raise ivy.utils.exceptions.IvyError(
f"Invalid data points {n}, expecting more than 1"
)
- if norm != "backward" and norm != "ortho" and norm != "forward":
+ if norm not in {"backward", "ortho", "forward"}:
raise ivy.utils.exceptions.IvyError(f"Unrecognized normalization mode {norm}")
if x.dtype in [np.uint64, np.int64, np.float64, np.complex128]:
out_dtype = np.complex128
@@ -726,7 +726,7 @@ def fft(
return np.fft.fft(x, n, dim, norm).astype(out_dtype)
-@with_supported_dtypes({"1.26.0 and below": ("float32", "float64")}, backend_version)
+@with_supported_dtypes({"1.26.1 and below": ("float32", "float64")}, backend_version)
def dct(
x: np.ndarray,
/,
@@ -940,7 +940,7 @@ def ifft(
raise ivy.utils.exceptions.IvyError(
f"Invalid data points {n}, expecting more than 1"
)
- if norm != "backward" and norm != "ortho" and norm != "forward":
+ if norm not in {"backward", "ortho", "forward"}:
raise ivy.utils.exceptions.IvyError(f"Unrecognized normalization mode {norm}")
return np.asarray(np.fft.ifft(x, n, dim, norm), dtype=x.dtype)
@@ -991,7 +991,7 @@ def ifftn(
return np.fft.ifftn(x, s, axes, norm).astype(x.dtype)
-@with_unsupported_dtypes({"1.26.0 and below": ("complex",)}, backend_version)
+@with_unsupported_dtypes({"1.26.1 and below": ("complex",)}, backend_version)
def embedding(
weights: np.ndarray,
indices: np.ndarray,
@@ -1016,6 +1016,26 @@ def embedding(
return embeddings
+def rfft(
+ x: np.ndarray,
+ /,
+ *,
+ n: Optional[int] = None,
+ axis: int = -1,
+ norm: Literal["backward", "ortho", "forward"] = "backward",
+ out: Optional[np.ndarray] = None,
+) -> np.ndarray:
+ x = x.real
+
+ ret = np.fft.rfft(x, n=n, axis=axis, norm=norm)
+
+ if x.dtype != np.float64:
+ ret = ret.astype(np.complex64)
+ if ivy.exists(out):
+ return ivy.inplace_update(out, ret)
+ return ret
+
+
def rfftn(
x: np.ndarray,
s: Sequence[int] = None,
@@ -1043,7 +1063,7 @@ def rfftn(
raise ivy.utils.exceptions.IvyError(
f"Invalid data points {s}, expecting s points larger than 1"
)
- if norm != "backward" and norm != "ortho" and norm != "forward":
+ if norm not in {"backward", "ortho", "forward"}:
raise ivy.utils.exceptions.IvyError(f"Unrecognized normalization mode {norm}")
return np.fft.rfftn(x, s, axes, norm).astype(np.complex128)
diff --git a/ivy/functional/backends/numpy/experimental/linear_algebra.py b/ivy/functional/backends/numpy/experimental/linear_algebra.py
index 5beee3d93b32f..2f054e1cb586b 100644
--- a/ivy/functional/backends/numpy/experimental/linear_algebra.py
+++ b/ivy/functional/backends/numpy/experimental/linear_algebra.py
@@ -68,15 +68,7 @@ def diagflat(
diagonal_to_add = np.diag(x - np.full_like(x, padding_value), k=offset)
diagonal_to_add = diagonal_to_add[tuple(slice(0, n) for n in output_array.shape)]
- output_array += np.pad(
- diagonal_to_add.astype(output_array.dtype),
- [
- (0, max([output_array.shape[0] - diagonal_to_add.shape[0], 0])),
- (0, max([output_array.shape[1] - diagonal_to_add.shape[1], 0])),
- ],
- mode="constant",
- )
- ret = output_array.astype(out_dtype)
+ ret = diagonal_to_add.astype(out_dtype)
if ivy.exists(out):
ivy.inplace_update(out, ret)
@@ -98,7 +90,7 @@ def kron(
@with_supported_dtypes(
- {"1.26.0 and below": ("float32", "float64", "complex64", "complex128")},
+ {"1.26.1 and below": ("float32", "float64", "complex64", "complex128")},
backend_version,
)
def matrix_exp(
@@ -114,7 +106,7 @@ def matrix_exp(
return exp_mat.astype(x.dtype)
-@with_unsupported_dtypes({"1.26.0 and below": ("float16",)}, backend_version)
+@with_unsupported_dtypes({"1.26.1 and below": ("float16",)}, backend_version)
def eig(
x: np.ndarray,
/,
@@ -128,7 +120,7 @@ def eig(
eig.support_native_out = False
-@with_unsupported_dtypes({"1.26.0 and below": ("float16",)}, backend_version)
+@with_unsupported_dtypes({"1.26.1 and below": ("float16",)}, backend_version)
def eigvals(x: np.ndarray, /) -> np.ndarray:
e = np.linalg.eigvals(x)
return e.astype(complex)
@@ -149,6 +141,47 @@ def adjoint(
return np.conjugate(np.transpose(x, axes=axes))
+_adjoint = adjoint
+
+
+def solve_triangular(
+ x1: np.ndarray,
+ x2: np.ndarray,
+ /,
+ *,
+ upper: bool = True,
+ adjoint: bool = False,
+ unit_diagonal: bool = False,
+ out: Optional[np.ndarray] = None,
+) -> np.ndarray:
+ # NumPy does not expose an API for `trsm`, so we have to implement substitution
+ # in Python. There is no need to support gradients for this backend.
+ # Pre: `x1` is square, `x1` and `x2` have the same number `n` of rows.
+ n = x1.shape[-2]
+ ret = x2.copy()
+
+ if adjoint:
+ x1 = _adjoint(x1)
+ upper = not upper
+
+ if unit_diagonal:
+ for i in range(n):
+ x1[..., i, i] = 1
+
+ if upper:
+ for i in reversed(range(n)):
+ ret[..., i, :] /= x1[..., i, np.newaxis, i]
+ ret[..., :i, :] -= x1[..., :i, np.newaxis, i] * ret[..., np.newaxis, i, :]
+ else:
+ for i in range(n):
+ ret[..., i, :] /= x1[..., i, np.newaxis, i]
+ ret[..., i + 1 :, :] -= (
+ x1[..., i + 1 :, np.newaxis, i] * ret[..., np.newaxis, i, :]
+ )
+
+ return ret
+
+
def multi_dot(
x: Sequence[np.ndarray],
/,
diff --git a/ivy/functional/backends/numpy/experimental/losses.py b/ivy/functional/backends/numpy/experimental/losses.py
index da8a56154dad3..363916ca62353 100644
--- a/ivy/functional/backends/numpy/experimental/losses.py
+++ b/ivy/functional/backends/numpy/experimental/losses.py
@@ -8,7 +8,7 @@
from . import backend_version
-@with_unsupported_dtypes({"1.26.0 and below": ("bool",)}, backend_version)
+@with_unsupported_dtypes({"1.26.1 and below": ("bool",)}, backend_version)
@_scalar_output_to_0d_array
def huber_loss(
input: np.ndarray,
@@ -32,7 +32,7 @@ def huber_loss(
# Implementation of smooth_l1_loss in the given format
-@with_unsupported_dtypes({"1.26.0 and below": ("bool",)}, backend_version)
+@with_unsupported_dtypes({"1.26.1 and below": ("bool",)}, backend_version)
@_scalar_output_to_0d_array
def smooth_l1_loss(
input: np.ndarray,
@@ -56,7 +56,7 @@ def smooth_l1_loss(
return loss
-@with_unsupported_dtypes({"1.26.0 and below": ("bool",)}, backend_version)
+@with_unsupported_dtypes({"1.26.1 and below": ("bool",)}, backend_version)
@_scalar_output_to_0d_array
def soft_margin_loss(
input: np.ndarray,
@@ -75,12 +75,15 @@ def soft_margin_loss(
return loss
-def _apply_loss_reduction(loss: np.ndarray, reduction: str) -> np.ndarray:
+def _apply_loss_reduction(loss: np.ndarray, reduction: str, axis, out) -> np.ndarray:
if reduction == "sum":
- return np.sum(loss)
+ return np.sum(loss, axis=axis, out=out)
elif reduction == "mean":
- return np.mean(loss)
+ return np.mean(loss, axis=axis, out=out)
else: # reduction == "none"
+ if out is not None:
+ out[...] = loss
+ return out
return loss
diff --git a/ivy/functional/backends/numpy/experimental/manipulation.py b/ivy/functional/backends/numpy/experimental/manipulation.py
index bcbd4d28aaecb..cefc371fedb26 100644
--- a/ivy/functional/backends/numpy/experimental/manipulation.py
+++ b/ivy/functional/backends/numpy/experimental/manipulation.py
@@ -403,7 +403,7 @@ def expand(
shape = list(shape)
for i, dim in enumerate(shape):
if dim < 0:
- shape[i] = int(np.prod(x.shape) / np.prod([s for s in shape if s > 0]))
+ shape[i] = x.shape[i]
return np.broadcast_to(x, tuple(shape))
@@ -483,6 +483,94 @@ def fill_diagonal(
return a
+@_scalar_output_to_0d_array
+def take(
+ x: Union[int, List, np.ndarray],
+ indices: Union[int, List, np.ndarray],
+ /,
+ *,
+ axis: Optional[int] = None,
+ mode: str = "raise",
+ fill_value: Optional[Number] = None,
+ out: Optional[np.ndarray] = None,
+) -> np.ndarray:
+ if mode not in ["raise", "wrap", "clip", "fill"]:
+ raise ValueError("mode must be one of 'clip', 'raise', 'wrap', or 'fill'")
+
+ # raise, clip, wrap
+ if mode != "fill":
+ return np.take(x, indices, axis=axis, mode=mode, out=out)
+
+ if not isinstance(x, np.ndarray):
+ x = np.array(x)
+ if len(x.shape) == 0:
+ x = np.array([x])
+ if not isinstance(indices, np.ndarray):
+ indices = np.array(indices)
+ if np.issubdtype(indices.dtype, np.floating):
+ indices = indices.astype(np.int64)
+
+ # fill
+ x_dtype = x.dtype
+ if fill_value is None:
+ # set according to jax behaviour
+ # https://tinyurl.com/66jn68uj
+ # NaN for inexact types (let fill_value as None)
+ if not np.issubdtype(x_dtype, np.inexact):
+ if np.issubdtype(x_dtype, np.bool_):
+ # True for booleans
+ fill_value = True
+ elif np.issubdtype(x_dtype, np.unsignedinteger):
+ # the largest positive value for unsigned types
+ fill_value = np.iinfo(x_dtype).max
+ else:
+ # the largest negative value for signed types
+ fill_value = np.iinfo(x_dtype).min
+
+ fill_value = np.array(fill_value, dtype=x_dtype)
+ x_shape = x.shape
+ ret = np.take(x, indices, axis=axis, mode="wrap")
+
+ if len(ret.shape) == 0:
+ # if scalar, scalar fill (replace)
+ if np.any(indices != 0):
+ ret = fill_value
+ else:
+ if ivy.exists(axis):
+ rank = len(x.shape)
+ axis = ((axis % rank) + rank) % rank
+ x_shape = x_shape[axis]
+ else:
+ axis = 0
+ x_shape = np.prod(x_shape)
+
+ bound_check = (indices < -x_shape) | (indices >= x_shape)
+
+ if np.any(bound_check):
+ if axis > 0:
+ bound_check = np.broadcast_to(
+ bound_check, (*x.shape[:axis], *bound_check.shape)
+ )
+ ret[bound_check] = fill_value
+
+ if ivy.exists(out):
+ ivy.inplace_update(out, ret)
+
+ return ret
+
+
+take.support_native_out = True
+
+
+def trim_zeros(
+ a: np.ndarray,
+ /,
+ *,
+ trim: Optional[str] = "fb",
+) -> np.ndarray:
+ return np.trim_zeros(a, trim=trim)
+
+
def column_stack(
arrays: Sequence[np.ndarray], /, *, out: Optional[np.ndarray] = None
) -> np.ndarray:
diff --git a/ivy/functional/backends/numpy/experimental/norms.py b/ivy/functional/backends/numpy/experimental/norms.py
index 56419015f7587..3d1b549283d13 100644
--- a/ivy/functional/backends/numpy/experimental/norms.py
+++ b/ivy/functional/backends/numpy/experimental/norms.py
@@ -4,7 +4,7 @@
from . import backend_version
-@with_unsupported_dtypes({"1.26.0 and below": ("float16",)}, backend_version)
+@with_unsupported_dtypes({"1.26.1 and below": ("float16",)}, backend_version)
def l1_normalize(
x: np.ndarray,
/,
diff --git a/ivy/functional/backends/numpy/experimental/searching.py b/ivy/functional/backends/numpy/experimental/searching.py
index a16fe58a84043..ce66a03b0b2b9 100644
--- a/ivy/functional/backends/numpy/experimental/searching.py
+++ b/ivy/functional/backends/numpy/experimental/searching.py
@@ -7,7 +7,7 @@
from . import backend_version
-@with_supported_dtypes({"1.26.0 and below": ("int32", "int64")}, backend_version)
+@with_supported_dtypes({"1.26.1 and below": ("int32", "int64")}, backend_version)
def unravel_index(
indices: np.ndarray,
shape: Tuple[int],
diff --git a/ivy/functional/backends/numpy/experimental/statistical.py b/ivy/functional/backends/numpy/experimental/statistical.py
index 084eed1b97545..b150ff89ff775 100644
--- a/ivy/functional/backends/numpy/experimental/statistical.py
+++ b/ivy/functional/backends/numpy/experimental/statistical.py
@@ -9,7 +9,7 @@
@with_unsupported_dtypes(
- {"1.26.0 and below": ("bfloat16",)},
+ {"1.26.1 and below": ("bfloat16",)},
backend_version,
)
def histogram(
@@ -167,6 +167,32 @@ def nanmean(
nanmean.support_native_out = True
+def nanmin(
+ a: np.ndarray,
+ /,
+ *,
+ axis: Optional[Union[int, Tuple[int]]] = None,
+ keepdims: Optional[bool] = False,
+ initial: Optional[Union[int, float, complex]] = None,
+ where: Optional[np.ndarray] = True,
+ out: Optional[np.ndarray] = None,
+) -> np.ndarray:
+ axis = tuple(axis) if isinstance(axis, list) else axis
+ if where is None:
+ where = True
+ return np.nanmin(
+ a=a,
+ axis=axis,
+ keepdims=keepdims,
+ out=out,
+ initial=initial,
+ where=where,
+ )
+
+
+nanmin.support_native_out = True
+
+
def nanprod(
a: np.ndarray,
/,
@@ -200,7 +226,7 @@ def _validate_quantile(q):
if not (0.0 <= q[i] <= 1.0):
return False
else:
- if not (np.all(0 <= q) and np.all(q <= 1)):
+ if not (np.all(q >= 0) and np.all(q <= 1)):
return False
return True
@@ -508,7 +534,7 @@ def __get_index(lst, indices=None, prefix=None):
return indices
-@with_unsupported_dtypes({"1.26.0 and below": "bfloat16"}, backend_version)
+@with_unsupported_dtypes({"1.26.1 and below": "bfloat16"}, backend_version)
def cummin(
x: np.ndarray,
/,
diff --git a/ivy/functional/backends/numpy/general.py b/ivy/functional/backends/numpy/general.py
index 758b2a68bf4ba..b984c8ab8dec8 100644
--- a/ivy/functional/backends/numpy/general.py
+++ b/ivy/functional/backends/numpy/general.py
@@ -10,7 +10,6 @@
# local
import ivy
-from ivy.functional.backends.numpy.device import _to_device
from ivy.functional.backends.numpy.helpers import _scalar_output_to_0d_array
from ivy.func_wrapper import with_unsupported_dtypes
from . import backend_version
@@ -81,8 +80,8 @@ def gather(
batch_dims: int = 0,
out: Optional[np.ndarray] = None,
) -> np.ndarray:
- axis = axis % len(params.shape)
- batch_dims = batch_dims % len(params.shape)
+ axis %= len(params.shape)
+ batch_dims %= len(params.shape)
ivy.utils.assertions.check_gather_input_valid(params, indices, axis, batch_dims)
result = []
if batch_dims == 0:
@@ -101,7 +100,7 @@ def gather(
result.append(r)
result = np.array(result)
result = result.reshape([*params.shape[0:batch_dims], *result.shape[1:]])
- return _to_device(result)
+ return result
def gather_nd_helper(params, indices):
@@ -144,7 +143,7 @@ def gather_nd(
out: Optional[np.ndarray] = None,
) -> np.ndarray:
ivy.utils.assertions.check_gather_nd_input_valid(params, indices, batch_dims)
- batch_dims = batch_dims % len(params.shape)
+ batch_dims %= len(params.shape)
result = []
if batch_dims == 0:
result = gather_nd_helper(params, indices)
@@ -162,7 +161,7 @@ def gather_nd(
result.append(r)
result = np.array(result)
result = result.reshape([*params.shape[0:batch_dims], *result.shape[1:]])
- return _to_device(result)
+ return result
def get_num_dims(x, /, *, as_array=False):
@@ -280,8 +279,8 @@ def scatter_flat(
np.maximum.at(target, indices, updates)
else:
raise ivy.utils.exceptions.IvyException(
- "reduction is {}, but it must be one of "
- '"sum", "min", "max" or "replace"'.format(reduction)
+ f'reduction is {reduction}, but it must be one of "sum", "min", "max" or'
+ ' "replace"'
)
if target_given:
return ivy.inplace_update(out, target)
@@ -326,12 +325,12 @@ def scatter_nd(
np.multiply.at(target, indices_tuple, updates)
else:
raise ivy.utils.exceptions.IvyException(
- "reduction is {}, but it must be one of "
- '"sum", "min", "max", "mul" or "replace"'.format(reduction)
+ f'reduction is {reduction}, but it must be one of "sum", "min", "max",'
+ ' "mul" or "replace"'
)
if ivy.exists(out):
- return ivy.inplace_update(out, _to_device(target))
- return _to_device(target)
+ return ivy.inplace_update(out, target)
+ return target
scatter_nd.support_native_out = True
@@ -401,7 +400,7 @@ def _vmap(*args):
# Handling None in in_axes by broadcasting the axis_size
if isinstance(in_axes, (tuple, list)) and None in in_axes:
- none_axis_index = list()
+ none_axis_index = []
for index, axis in enumerate(in_axes):
if axis is None:
none_axis_index.append(index)
@@ -435,7 +434,7 @@ def _vmap(*args):
return _vmap
-@with_unsupported_dtypes({"1.26.0 and below": ("bfloat16",)}, backend_version)
+@with_unsupported_dtypes({"1.26.1 and below": ("bfloat16",)}, backend_version)
def isin(
elements: np.ndarray,
test_elements: np.ndarray,
diff --git a/ivy/functional/backends/numpy/gradients.py b/ivy/functional/backends/numpy/gradients.py
index dbe9dbcbee98f..1f930d0ebe687 100644
--- a/ivy/functional/backends/numpy/gradients.py
+++ b/ivy/functional/backends/numpy/gradients.py
@@ -2,7 +2,7 @@
# global
import logging
-from typing import Optional, Sequence, Union
+from typing import Sequence, Union
import ivy
@@ -30,8 +30,8 @@ def execute_with_gradients(
/,
*,
retain_grads: bool = False,
- xs_grad_idxs: Optional[Sequence[Sequence[Union[str, int]]]] = [[0]],
- ret_grad_idxs: Optional[Sequence[Sequence[Union[str, int]]]] = [[0]],
+ xs_grad_idxs: Sequence[Sequence[Union[str, int]]] = ((0,),),
+ ret_grad_idxs: Sequence[Sequence[Union[str, int]]] = ((0,),),
):
logging.warning(
"NumPy does not support autograd, "
diff --git a/ivy/functional/backends/numpy/linear_algebra.py b/ivy/functional/backends/numpy/linear_algebra.py
index fc423ebd48bab..26257efbba19f 100644
--- a/ivy/functional/backends/numpy/linear_algebra.py
+++ b/ivy/functional/backends/numpy/linear_algebra.py
@@ -18,7 +18,7 @@
# -------------------#
-@with_unsupported_dtypes({"1.26.0 and below": ("float16", "complex")}, backend_version)
+@with_unsupported_dtypes({"1.26.1 and below": ("float16", "complex")}, backend_version)
def cholesky(
x: np.ndarray, /, *, upper: bool = False, out: Optional[np.ndarray] = None
) -> np.ndarray:
@@ -30,7 +30,7 @@ def cholesky(
return ret
-@with_unsupported_dtypes({"1.26.0 and below": ("float16",)}, backend_version)
+@with_unsupported_dtypes({"1.26.1 and below": ("float16",)}, backend_version)
def cross(
x1: np.ndarray,
x2: np.ndarray,
@@ -46,7 +46,7 @@ def cross(
@_scalar_output_to_0d_array
-@with_unsupported_dtypes({"1.26.0 and below": ("float16",)}, backend_version)
+@with_unsupported_dtypes({"1.26.1 and below": ("float16",)}, backend_version)
def det(x: np.ndarray, /, *, out: Optional[np.ndarray] = None) -> np.ndarray:
return np.linalg.det(x)
@@ -63,7 +63,7 @@ def diagonal(
return np.diagonal(x, offset=offset, axis1=axis1, axis2=axis2)
-@with_unsupported_dtypes({"1.26.0 and below": ("float16",)}, backend_version)
+@with_unsupported_dtypes({"1.26.1 and below": ("float16",)}, backend_version)
def eigh(
x: np.ndarray, /, *, UPLO: str = "L", out: Optional[np.ndarray] = None
) -> Tuple[np.ndarray]:
@@ -74,7 +74,7 @@ def eigh(
return result_tuple(eigenvalues, eigenvectors)
-@with_unsupported_dtypes({"1.26.0 and below": ("float16",)}, backend_version)
+@with_unsupported_dtypes({"1.26.1 and below": ("float16",)}, backend_version)
def eigvalsh(
x: np.ndarray, /, *, UPLO: str = "L", out: Optional[np.ndarray] = None
) -> np.ndarray:
@@ -90,7 +90,7 @@ def inner(
@with_unsupported_dtypes(
- {"1.26.0 and below": ("bfloat16", "float16", "complex")},
+ {"1.26.1 and below": ("bfloat16", "float16", "complex")},
backend_version,
)
def inv(
@@ -110,7 +110,7 @@ def inv(
return np.linalg.inv(x)
-@with_unsupported_dtypes({"1.26.0 and below": ("float16", "bfloat16")}, backend_version)
+@with_unsupported_dtypes({"1.26.1 and below": ("float16", "bfloat16")}, backend_version)
def matmul(
x1: np.ndarray,
x2: np.ndarray,
@@ -140,7 +140,7 @@ def matmul(
@_scalar_output_to_0d_array
-@with_unsupported_dtypes({"1.26.0 and below": ("float16", "bfloat16")}, backend_version)
+@with_unsupported_dtypes({"1.26.1 and below": ("float16", "bfloat16")}, backend_version)
def matrix_norm(
x: np.ndarray,
/,
@@ -162,7 +162,7 @@ def matrix_power(
@with_unsupported_dtypes(
- {"1.26.0 and below": ("float16", "bfloat16", "complex")},
+ {"1.26.1 and below": ("float16", "bfloat16", "complex")},
backend_version,
)
@_scalar_output_to_0d_array
@@ -201,7 +201,7 @@ def matrix_transpose(
return np.swapaxes(x, -1, -2)
-@with_unsupported_dtypes({"1.26.0 and below": ("float16",)}, backend_version)
+@with_unsupported_dtypes({"1.26.1 and below": ("float16",)}, backend_version)
def outer(
x1: np.ndarray,
x2: np.ndarray,
@@ -216,7 +216,7 @@ def outer(
outer.support_native_out = True
-@with_unsupported_dtypes({"1.26.0 and below": ("float16",)}, backend_version)
+@with_unsupported_dtypes({"1.26.1 and below": ("float16",)}, backend_version)
def pinv(
x: np.ndarray,
/,
@@ -230,20 +230,20 @@ def pinv(
return np.linalg.pinv(x, rtol)
-@with_unsupported_dtypes({"1.26.0 and below": ("float16",)}, backend_version)
+@with_unsupported_dtypes({"1.26.1 and below": ("float16",)}, backend_version)
def qr(
x: np.ndarray,
/,
*,
mode: str = "reduced",
out: Optional[Tuple[np.ndarray, np.ndarray]] = None,
-) -> NamedTuple:
+) -> Tuple[np.ndarray, np.ndarray]:
res = namedtuple("qr", ["Q", "R"])
q, r = np.linalg.qr(x, mode=mode)
return res(q, r)
-@with_unsupported_dtypes({"1.26.0 and below": ("float16",)}, backend_version)
+@with_unsupported_dtypes({"1.26.1 and below": ("float16",)}, backend_version)
def slogdet(
x: np.ndarray,
/,
@@ -258,7 +258,7 @@ def slogdet(
return results(sign, logabsdet)
-@with_unsupported_dtypes({"1.26.0 and below": ("float16",)}, backend_version)
+@with_unsupported_dtypes({"1.26.1 and below": ("float16",)}, backend_version)
def solve(
x1: np.ndarray,
x2: np.ndarray,
@@ -283,7 +283,7 @@ def solve(
return ret
-@with_unsupported_dtypes({"1.26.0 and below": ("float16",)}, backend_version)
+@with_unsupported_dtypes({"1.26.1 and below": ("float16",)}, backend_version)
def svd(
x: np.ndarray, /, *, compute_uv: bool = True, full_matrices: bool = True
) -> Union[np.ndarray, Tuple[np.ndarray, ...]]:
@@ -297,8 +297,11 @@ def svd(
return results(D)
-@with_unsupported_dtypes({"1.26.0 and below": ("float16",)}, backend_version)
-def svdvals(x: np.ndarray, /, *, out: Optional[np.ndarray] = None) -> np.ndarray:
+@with_unsupported_dtypes({"1.26.1 and below": ("float16",)}, backend_version)
+def svdvals(
+ x: np.ndarray, /, *, driver: Optional[str] = None, out: Optional[np.ndarray] = None
+) -> np.ndarray:
+ # TODO: handling the driver argument
return np.linalg.svd(x, compute_uv=False)
@@ -327,7 +330,7 @@ def tensordot(
@_scalar_output_to_0d_array
-@with_unsupported_dtypes({"1.26.0 and below": ("float16", "bfloat16")}, backend_version)
+@with_unsupported_dtypes({"1.26.1 and below": ("float16", "bfloat16")}, backend_version)
def trace(
x: np.ndarray,
/,
@@ -355,7 +358,7 @@ def vecdot(
return np.tensordot(x1, x2, axes=(axis, axis))
-@with_unsupported_dtypes({"1.26.0 and below": ("float16",)}, backend_version)
+@with_unsupported_dtypes({"1.26.1 and below": ("float16",)}, backend_version)
def eig(x: np.ndarray, /, *, out: Optional[np.ndarray] = None) -> Tuple[np.ndarray]:
result_tuple = NamedTuple(
"eig", [("eigenvalues", np.ndarray), ("eigenvectors", np.ndarray)]
@@ -432,7 +435,7 @@ def vander(
@with_unsupported_dtypes(
{
- "1.26.0 and below": (
+ "1.26.1 and below": (
"complex",
"unsigned",
)
diff --git a/ivy/functional/backends/numpy/manipulation.py b/ivy/functional/backends/numpy/manipulation.py
index 5a98379486828..2bc593bfcbe37 100644
--- a/ivy/functional/backends/numpy/manipulation.py
+++ b/ivy/functional/backends/numpy/manipulation.py
@@ -63,11 +63,10 @@ def flip(
axis: Optional[Union[int, Sequence[int]]] = None,
out: Optional[np.ndarray] = None,
) -> np.ndarray:
+ if copy:
+ x = x.copy()
num_dims = len(x.shape)
if not num_dims:
- if copy:
- newarr = x.copy()
- return newarr
return x
if axis is None:
axis = list(range(num_dims))
@@ -166,9 +165,8 @@ def split(
if x.shape == ():
if num_or_size_splits is not None and num_or_size_splits != 1:
raise ivy.utils.exceptions.IvyException(
- "input array had no shape, but num_sections specified was {}".format(
- num_or_size_splits
- )
+ "input array had no shape, but num_sections specified was"
+ f" {num_or_size_splits}"
)
return [x]
if num_or_size_splits is None:
@@ -191,7 +189,7 @@ def split(
return np.split(x, num_or_size_splits, axis)
-@with_unsupported_dtypes({"1.26.0 and below": ("uint64",)}, backend_version)
+@with_unsupported_dtypes({"1.26.1 and below": ("uint64",)}, backend_version)
def repeat(
x: np.ndarray,
/,
diff --git a/ivy/functional/backends/numpy/random.py b/ivy/functional/backends/numpy/random.py
index 2150f96fc2453..a398c6a63db41 100644
--- a/ivy/functional/backends/numpy/random.py
+++ b/ivy/functional/backends/numpy/random.py
@@ -51,7 +51,7 @@ def random_normal(
return np.asarray(np.random.normal(mean, std, shape), dtype=dtype)
-@with_unsupported_dtypes({"1.26.0 and below": ("bfloat16",)}, backend_version)
+@with_unsupported_dtypes({"1.26.1 and below": ("bfloat16",)}, backend_version)
def multinomial(
population_size: int,
num_samples: int,
diff --git a/ivy/functional/backends/numpy/set.py b/ivy/functional/backends/numpy/set.py
index 1986224d438d6..18911bebd6402 100644
--- a/ivy/functional/backends/numpy/set.py
+++ b/ivy/functional/backends/numpy/set.py
@@ -75,13 +75,17 @@ def unique_counts(
def unique_inverse(
x: np.ndarray,
/,
+ *,
+ axis: Optional[int] = None,
) -> Tuple[np.ndarray, np.ndarray]:
Results = namedtuple("Results", ["values", "inverse_indices"])
- values, inverse_indices = np.unique(x, return_inverse=True)
+ values, inverse_indices = np.unique(x, return_inverse=True, axis=axis)
nan_count = np.count_nonzero(np.isnan(x))
if nan_count > 1:
- values = np.append(values, np.full(nan_count - 1, np.nan)).astype(x.dtype)
- inverse_indices = inverse_indices.reshape(x.shape)
+ values = np.append(values, np.full(nan_count - 1, np.nan), axis=axis).astype(
+ x.dtype
+ )
+ inverse_indices = np.reshape(inverse_indices, x.shape, axis=0)
return Results(values, inverse_indices)
diff --git a/ivy/functional/backends/numpy/sorting.py b/ivy/functional/backends/numpy/sorting.py
index a072eb6206b54..3edced7e06096 100644
--- a/ivy/functional/backends/numpy/sorting.py
+++ b/ivy/functional/backends/numpy/sorting.py
@@ -42,7 +42,7 @@ def sort(
# msort
-@with_unsupported_dtypes({"1.26.0 and below": ("complex",)}, backend_version)
+@with_unsupported_dtypes({"1.26.1 and below": ("complex",)}, backend_version)
def msort(
a: Union[np.ndarray, list, tuple], /, *, out: Optional[np.ndarray] = None
) -> np.ndarray:
diff --git a/ivy/functional/backends/numpy/statistical.py b/ivy/functional/backends/numpy/statistical.py
index c2c9b91a662da..d63277c355bb0 100644
--- a/ivy/functional/backends/numpy/statistical.py
+++ b/ivy/functional/backends/numpy/statistical.py
@@ -54,9 +54,7 @@ def mean(
out: Optional[np.ndarray] = None,
) -> np.ndarray:
axis = tuple(axis) if isinstance(axis, list) else axis
- return ivy.astype(
- np.mean(x, axis=axis, keepdims=keepdims, out=out), x.dtype, copy=False
- )
+ return np.mean(x, axis=axis, keepdims=keepdims, dtype=x.dtype, out=out)
mean.support_native_out = True
@@ -171,7 +169,7 @@ def var(
# ------#
-@with_unsupported_dtypes({"1.26.0 and below": ("bfloat16",)}, backend_version)
+@with_unsupported_dtypes({"1.26.1 and below": ("bfloat16",)}, backend_version)
def cumprod(
x: np.ndarray,
/,
diff --git a/ivy/functional/backends/paddle/__init__.py b/ivy/functional/backends/paddle/__init__.py
index bd0f7259b687c..e63c5c3183202 100644
--- a/ivy/functional/backends/paddle/__init__.py
+++ b/ivy/functional/backends/paddle/__init__.py
@@ -175,20 +175,27 @@ def rep_method(*args, **kwargs):
),
}
valid_int_dtypes = {
- "2.5.1 and below": (
+ "2.5.2 and below": (
ivy.int8,
ivy.int16,
ivy.int32,
ivy.int64,
ivy.uint8,
- )
+ ),
+ "2.5.2 and above": (
+ ivy.int8,
+ ivy.int16,
+ ivy.int32,
+ ivy.int64,
+ ivy.uint8,
+ ),
}
valid_float_dtypes = {
"2.4.0 and below": (ivy.float16, ivy.float32, ivy.float64),
"2.4.1 and above": (ivy.bfloat16, ivy.float16, ivy.float32, ivy.float64),
}
-valid_uint_dtypes = {"2.5.1 and below": (ivy.uint8,)}
-valid_complex_dtypes = {"2.5.1 and below": (ivy.complex64, ivy.complex128)}
+valid_uint_dtypes = {"2.5.2 and below": (ivy.uint8,)}
+valid_complex_dtypes = {"2.5.2 and below": (ivy.complex64, ivy.complex128)}
# leave these untouched
valid_dtypes = _dtype_from_version(valid_dtypes, backend_version)
@@ -228,10 +235,10 @@ def rep_method(*args, **kwargs):
),
}
-invalid_int_dtypes = {"2.5.1 and below": (ivy.uint16, ivy.uint32, ivy.uint64)}
+invalid_int_dtypes = {"2.5.2 and below": (ivy.uint16, ivy.uint32, ivy.uint64)}
invalid_float_dtypes = {"2.4.0 and below": (ivy.bfloat16,), "2.4.1 and above": ()}
-invalid_uint_dtypes = {"2.5.1 and below": (ivy.uint16, ivy.uint32, ivy.uint64)}
-invalid_complex_dtypes = {"2.5.1 and below": ()}
+invalid_uint_dtypes = {"2.5.2 and below": (ivy.uint16, ivy.uint32, ivy.uint64)}
+invalid_complex_dtypes = {"2.5.2 and below": ()}
# leave these untouched
invalid_dtypes = _dtype_from_version(invalid_dtypes, backend_version)
diff --git a/ivy/functional/backends/paddle/activations.py b/ivy/functional/backends/paddle/activations.py
index ac1343e86aa9f..8182b0ef2263a 100644
--- a/ivy/functional/backends/paddle/activations.py
+++ b/ivy/functional/backends/paddle/activations.py
@@ -4,6 +4,7 @@
Collection of Paddle activation functions, wrapped to fit Ivy syntax and
signature.
"""
+
from typing import Optional, Union, Literal
# global
@@ -13,35 +14,27 @@
# local
import ivy.functional.backends.paddle as paddle_backend
import ivy
-from ivy.func_wrapper import with_unsupported_device_and_dtypes
+from ivy.func_wrapper import (
+ with_unsupported_device_and_dtypes,
+ with_supported_dtypes,
+ with_supported_device_and_dtypes,
+)
from . import backend_version
-unsupported_dtypes = [
- paddle.int8,
- paddle.int16,
- paddle.int32,
- paddle.int64,
- paddle.uint8,
- paddle.float16,
- paddle.complex64,
- paddle.complex128,
- paddle.bool,
-]
-
-
-def relu(
- x: paddle.Tensor, /, *, complex_mode="jax", out: Optional[paddle.Tensor] = None
-) -> paddle.Tensor:
- if x.dtype in unsupported_dtypes:
- if paddle.is_complex(x):
- return paddle.complex(F.relu(x.real()), F.relu(x.imag()))
- return F.relu(x.cast("float32")).cast(x.dtype)
+@with_supported_dtypes(
+ {"2.5.1 and below": ("float32", "float64", "complex")},
+ backend_version,
+)
+def relu(x: paddle.Tensor, /, *, out: Optional[paddle.Tensor] = None) -> paddle.Tensor:
+ if paddle.is_complex(x):
+ return paddle.complex(F.relu(x.real()), F.relu(x.imag()))
return F.relu(x)
-@with_unsupported_device_and_dtypes(
- {"2.5.1 and below": {"cpu": ("bfloat16",)}}, backend_version
+@with_supported_device_and_dtypes(
+ {"2.5.2 and below": {"cpu": ("float32", "float64", "complex")}},
+ backend_version,
)
def leaky_relu(
x: paddle.Tensor,
@@ -51,18 +44,17 @@ def leaky_relu(
complex_mode="jax",
out: Optional[paddle.Tensor] = None,
) -> paddle.Tensor:
- if x.dtype in unsupported_dtypes:
- if paddle.is_complex(x):
- return paddle.complex(
- F.leaky_relu(x.real(), negative_slope=alpha),
- F.leaky_relu(x.imag(), negative_slope=alpha),
- )
- return F.leaky_relu(x.cast("float32"), negative_slope=alpha).cast(x.dtype)
+ if paddle.is_complex(x):
+ return paddle.complex(
+ F.leaky_relu(x.real(), negative_slope=alpha),
+ F.leaky_relu(x.imag(), negative_slope=alpha),
+ )
return F.leaky_relu(x, negative_slope=alpha)
-@with_unsupported_device_and_dtypes(
- {"2.5.1 and below": {"cpu": ("bfloat16",)}}, backend_version
+@with_supported_device_and_dtypes(
+ {"2.5.2 and below": {"cpu": ("float32", "float64", "complex")}},
+ backend_version,
)
def gelu(
x: paddle.Tensor,
@@ -81,26 +73,23 @@ def gelu(
* x
* (1 + paddle_backend.tanh(sqrt_2_over_pi * (x + 0.044715 * x * x * x)))
)
- if x.dtype in unsupported_dtypes:
- return F.gelu(x.cast("float32"), approximate=approximate).cast(x.dtype)
return F.gelu(x, approximate=approximate)
-@with_unsupported_device_and_dtypes(
- {"2.5.1 and below": {"cpu": ("bfloat16",)}}, backend_version
+@with_supported_device_and_dtypes(
+ {"2.5.2 and below": {"cpu": ("float32", "float64", "complex")}},
+ backend_version,
)
def sigmoid(
x: paddle.Tensor, /, *, complex_mode="jax", out: Optional[paddle.Tensor] = None
) -> paddle.Tensor:
if paddle.is_complex(x):
return 1.0 / (1.0 + paddle_backend.exp(-x))
- if x.dtype in unsupported_dtypes:
- return F.sigmoid(x.cast("float32")).cast(x.dtype)
return F.sigmoid(x)
@with_unsupported_device_and_dtypes(
- {"2.5.1 and below": {"cpu": ("float16", "bfloat16")}}, backend_version
+ {"2.5.2 and below": {"cpu": ("bfloat16", "float16")}}, backend_version
)
def softmax(
x: paddle.Tensor,
@@ -150,7 +139,7 @@ def softplus(
# Softsign
@with_unsupported_device_and_dtypes(
- {"2.5.1 and below": {"cpu": ("float16", "bfloat16")}}, backend_version
+ {"2.5.2 and below": {"cpu": ("float16", "bfloat16")}}, backend_version
)
def softsign(
x: paddle.Tensor,
@@ -164,7 +153,7 @@ def softsign(
@with_unsupported_device_and_dtypes(
- {"2.5.1 and below": {"cpu": ("float16", "bfloat16")}}, backend_version
+ {"2.5.2 and below": {"cpu": ("float16", "bfloat16")}}, backend_version
)
def log_softmax(
x: paddle.Tensor,
@@ -182,8 +171,9 @@ def log_softmax(
return ret
-@with_unsupported_device_and_dtypes(
- {"2.5.1 and below": {"cpu": ("bfloat16",)}}, backend_version
+@with_supported_device_and_dtypes(
+ {"2.5.2 and below": {"cpu": ("float32", "float64", "complex")}},
+ backend_version,
)
def mish(
x: paddle.Tensor,
@@ -192,15 +182,13 @@ def mish(
complex_mode: Literal["split", "magnitude", "jax"] = "jax",
out: Optional[paddle.Tensor] = None,
) -> paddle.Tensor:
- if x.dtype in unsupported_dtypes:
- if paddle.is_complex(x):
- return x * paddle_backend.tanh(paddle_backend.log1p(paddle_backend.exp(x)))
- return F.mish(x.cast("float32")).cast(x.dtype)
+ if paddle.is_complex(x):
+ return x * paddle_backend.tanh(paddle_backend.log1p(paddle_backend.exp(x)))
return F.mish(x)
@with_unsupported_device_and_dtypes(
- {"2.5.1 and below": {"cpu": ("float16",)}}, backend_version
+ {"2.5.2 and below": {"cpu": ("float16",)}}, backend_version
)
def hardswish(
x: paddle.Tensor,
diff --git a/ivy/functional/backends/paddle/creation.py b/ivy/functional/backends/paddle/creation.py
index c6922d3690032..e91fcc479ba5d 100644
--- a/ivy/functional/backends/paddle/creation.py
+++ b/ivy/functional/backends/paddle/creation.py
@@ -95,15 +95,24 @@ def asarray(
ret = obj.clone().detach()
ret.stop_gradient = obj.stop_gradient
else:
- ret = obj
+ ret = paddle.to_tensor(
+ obj.detach(),
+ dtype=dtype,
+ place=device,
+ stop_gradient=obj.stop_gradient,
+ )
else:
ret = obj
- return ret.astype(dtype)
+ ret = ret.astype(dtype) if ret.dtype != obj.dtype else ret
+ return paddle_backend.to_device(ret, device)
elif isinstance(obj, (Number, bool, complex)):
- return paddle_backend.squeeze(
- paddle.to_tensor(obj, dtype=dtype, place=device), axis=0
- )
+ ret = paddle.to_tensor(obj, dtype=dtype, place=device)
+
+ if ret.ndim != 0: # for versions <2.5.0
+ return ret.squeeze()
+ else:
+ return ret
obj = ivy.nested_map(_remove_np_bfloat16, obj, shallow=False)
return paddle.to_tensor(obj, dtype=dtype, place=device)
@@ -133,7 +142,7 @@ def empty_like(
@with_unsupported_device_and_dtypes(
{
- "2.5.1 and below": {
+ "2.5.2 and below": {
"cpu": (
"uint8",
"int8",
@@ -198,7 +207,11 @@ def to_dlpack(x, /, *, out: Optional[paddle.Tensor] = None):
def from_dlpack(x, /, *, out: Optional[paddle.Tensor] = None):
- return paddle.utils.dlpack.from_dlpack(x)
+ if hasattr(x, "__dlpack__"):
+ capsule = x.__dlpack__()
+ else:
+ capsule = x
+ return paddle.utils.dlpack.from_dlpack(capsule)
def full(
@@ -263,7 +276,10 @@ def _linspace_helper(start, stop, num, axis=None, *, dtype=None):
sos_shape = stop_shape
if num == 1:
return (
- paddle_backend.ones(stop_shape[:axis] + [1] + stop_shape[axis:]) * start
+ paddle_backend.ones(
+ stop_shape[:axis] + [1] + stop_shape[axis:], dtype=dtype
+ )
+ * start
)
stop = stop.reshape((-1,))
linspace_method = (
@@ -340,7 +356,7 @@ def _slice_at_axis(sl, axis):
@with_unsupported_device_and_dtypes(
- {"2.5.1 and below": {"cpu": ("uint16", "bfloat16", "float16")}}, backend_version
+ {"2.5.2 and below": {"cpu": ("uint16", "bfloat16", "float16")}}, backend_version
)
def linspace(
start: Union[paddle.Tensor, float],
@@ -398,7 +414,7 @@ def linspace(
@with_unsupported_device_and_dtypes(
{
- "2.5.1 and below": {
+ "2.5.2 and below": {
"cpu": (
"int8",
"int16",
@@ -468,7 +484,7 @@ def ones_like(
@with_unsupported_device_and_dtypes(
{
- "2.5.1 and below": {
+ "2.5.2 and below": {
"cpu": (
"int8",
"int16",
@@ -487,7 +503,7 @@ def tril(
@with_unsupported_device_and_dtypes(
{
- "2.5.1 and below": {
+ "2.5.2 and below": {
"cpu": (
"int8",
"int16",
@@ -600,7 +616,7 @@ def one_hot(
@with_unsupported_device_and_dtypes(
- {"2.5.1 and below": {"cpu": ("complex64", "complex128")}},
+ {"2.5.2 and below": {"cpu": ("complex64", "complex128")}},
backend_version,
)
def frombuffer(
diff --git a/ivy/functional/backends/paddle/data_type.py b/ivy/functional/backends/paddle/data_type.py
index 1dfcf49e4104f..2aca8cca43f87 100644
--- a/ivy/functional/backends/paddle/data_type.py
+++ b/ivy/functional/backends/paddle/data_type.py
@@ -5,7 +5,9 @@
import ivy.functional.backends.paddle as paddle_backend
import numpy as np
import ivy
+from ivy.func_wrapper import with_unsupported_dtypes
from ivy.functional.ivy.data_type import _handle_nestable_dtype_info
+from . import backend_version
ivy_dtype_dict = {
@@ -97,8 +99,9 @@ def __init__(self):
self.tiny = 1.17549e-38
def __repr__(self):
- return "finfo(resolution={}, min={}, max={}, dtype={})".format(
- self.resolution, self.min, self.max, "bfloat16"
+ return (
+ f"finfo(resolution={self.resolution}, min={self.min}, max={self.max},"
+ " dtype=bfloat16)"
)
@@ -116,8 +119,8 @@ def astype(
) -> paddle.Tensor:
dtype = ivy.as_native_dtype(dtype)
if x.dtype == dtype:
- return paddle_backend.copy_array(x).data if copy else x
- return x.cast(dtype)
+ return x.clone() if copy else x
+ return x.clone().cast(dtype) if copy else x.cast(dtype)
def broadcast_arrays(*arrays: paddle.Tensor) -> List[paddle.Tensor]:
@@ -138,6 +141,18 @@ def broadcast_arrays(*arrays: paddle.Tensor) -> List[paddle.Tensor]:
return result
+@with_unsupported_dtypes(
+ {
+ "2.5.1 and below": (
+ "uint8",
+ "int8",
+ "int16",
+ "float16",
+ "bfloat16",
+ )
+ },
+ backend_version,
+)
def broadcast_to(
x: paddle.Tensor,
/,
@@ -156,15 +171,7 @@ def broadcast_to(
if x.ndim > len(shape):
x = x.reshape([-1])
- if x.dtype in [
- paddle.int8,
- paddle.int16,
- paddle.uint8,
- paddle.float16,
- paddle.bfloat16,
- ]:
- return paddle.broadcast_to(x.cast("float32"), shape).cast(x.dtype)
- elif x.dtype in [paddle.complex64, paddle.complex128]:
+ if x.dtype in [paddle.complex64, paddle.complex128]:
x_real = paddle.broadcast_to(x.real(), shape)
x_imag = paddle.broadcast_to(x.imag(), shape)
return paddle.complex(x_real, x_imag)
@@ -236,7 +243,7 @@ def as_native_dtype(
return paddle.bool
if not isinstance(dtype_in, str):
return dtype_in
- if dtype_in in native_dtype_dict.keys():
+ if dtype_in in native_dtype_dict:
return native_dtype_dict[ivy.Dtype(dtype_in)]
else:
raise ivy.utils.exceptions.IvyException(
diff --git a/ivy/functional/backends/paddle/device.py b/ivy/functional/backends/paddle/device.py
index 3865f9ec3a3d6..0270ccd9d1d5b 100644
--- a/ivy/functional/backends/paddle/device.py
+++ b/ivy/functional/backends/paddle/device.py
@@ -32,10 +32,16 @@ def to_device(
out: Optional[paddle.Tensor] = None,
) -> paddle.Tensor:
device = as_native_dev(device)
- if device.is_cpu_place():
+ if device.is_cpu_place() and not x.place.is_cpu_place():
return x.cpu()
- elif device.is_gpu_place():
+ elif (device.is_gpu_place() and not x.place.is_gpu_place()) or (
+ x.place.is_gpu_place()
+ and device.is_gpu_place()
+ and x.place.gpu_device_id() != device.gpu_device_id()
+ ):
return x.cuda(device.gpu_device_id())
+ else:
+ return x
def as_ivy_dev(device: core.Place, /):
@@ -48,7 +54,7 @@ def as_ivy_dev(device: core.Place, /):
return ivy.Device("cpu")
elif device.is_gpu_place():
dev_idx = device.gpu_device_id()
- return ivy.Device("gpu:" + str(dev_idx))
+ return ivy.Device(f"gpu:{str(dev_idx)}")
def as_native_dev(
diff --git a/ivy/functional/backends/paddle/elementwise.py b/ivy/functional/backends/paddle/elementwise.py
index 852d95e6487be..146d7e53ee102 100644
--- a/ivy/functional/backends/paddle/elementwise.py
+++ b/ivy/functional/backends/paddle/elementwise.py
@@ -1,23 +1,36 @@
# global
-from typing import Union, Optional, Tuple, Type
+from typing import Union, Optional
-import paddle
import math
+import paddle
import ivy.functional.backends.paddle as paddle_backend
import ivy
from ivy import promote_types_of_inputs
-from ivy.func_wrapper import with_unsupported_device_and_dtypes, with_supported_dtypes
+from ivy.func_wrapper import (
+ with_unsupported_device_and_dtypes,
+ with_supported_device_and_dtypes,
+ with_supported_dtypes,
+ with_unsupported_dtypes,
+)
# local
from . import backend_version
def _elementwise_helper(x1, x2):
- x1, x2 = ivy.promote_types_of_inputs(x1, x2)
- x1, x2 = paddle_backend.broadcast_arrays(x1, x2)
+ if (not hasattr(x1, "dtype") or not hasattr(x2, "dtype")) or (x1.dtype != x2.dtype):
+ x1, x2 = ivy.promote_types_of_inputs(x1, x2)
+ # the following was needed in versions <=2.4.2 because most functions didn't
+ # accept 0D inputs along other inputs
+ # if x1.shape != x2.shape:
+ # x1, x2 = paddle_backend.broadcast_arrays(x1, x2)
return x1, x2, x1.dtype
+@with_unsupported_dtypes(
+ {"2.5.1 and below": ("int8", "uint8", "float16", "bool", "bfloat16")},
+ backend_version,
+)
def add(
x1: Union[float, paddle.Tensor],
x2: Union[float, paddle.Tensor],
@@ -27,14 +40,6 @@ def add(
out: Optional[paddle.Tensor] = None,
) -> paddle.Tensor:
x1, x2, ret_dtype = _elementwise_helper(x1, x2)
- if x1.dtype in [
- paddle.int8,
- paddle.uint8,
- paddle.float16,
- paddle.bool,
- paddle.bfloat16,
- ]:
- x1, x2 = x1.astype("float32"), x2.astype("float32")
if alpha not in (1, None):
x2 = paddle_backend.multiply(x2, alpha)
x1, x2 = ivy.promote_types_of_inputs(x1, x2)
@@ -52,10 +57,18 @@ def bitwise_xor(
return paddle.bitwise_xor(x1, x2)
+@with_supported_dtypes(
+ {
+ "2.5.1 and below": (
+ "float16",
+ "float32",
+ "float64",
+ )
+ },
+ backend_version,
+)
def expm1(x: paddle.Tensor, /, *, out: Optional[paddle.Tensor] = None) -> paddle.Tensor:
- if x.dtype in [paddle.float16, paddle.float32, paddle.float64]:
- return paddle.expm1(x)
- return paddle_backend.subtract(paddle_backend.exp(x), 1.0).astype(x.dtype)
+ return paddle.expm1(x)
def bitwise_invert(
@@ -66,7 +79,7 @@ def bitwise_invert(
@with_unsupported_device_and_dtypes(
{
- "2.5.1 and below": {
+ "2.5.2 and below": {
"cpu": (
"int8",
"int16",
@@ -123,6 +136,10 @@ def equal(
)
+@with_unsupported_dtypes(
+ {"2.5.1 and below": ("int8", "int16", "bfloat16", "unsigned", "float16")},
+ backend_version,
+)
def less_equal(
x1: Union[float, paddle.Tensor],
x2: Union[float, paddle.Tensor],
@@ -131,13 +148,11 @@ def less_equal(
out: Optional[paddle.Tensor] = None,
) -> paddle.Tensor:
x1, x2, ret_dtype = _elementwise_helper(x1, x2)
- if x1.dtype in [paddle.int8, paddle.uint8, paddle.complex64, paddle.complex128]:
+ if paddle.is_complex(x1):
if paddle.is_complex(x1):
- if paddle.is_complex(x1):
- real = paddle.less_equal(x1.real(), x2.real())
- imag = paddle.less_equal(x1.imag(), x2.imag())
- return paddle_backend.logical_and(real, imag)
- return paddle.less_equal(x1.astype("float32"), x2.astype("float32"))
+ real = paddle.less_equal(x1.real(), x2.real())
+ imag = paddle.less_equal(x1.imag(), x2.imag())
+ return paddle_backend.logical_and(real, imag)
return paddle.less_equal(x1, x2)
@@ -153,95 +168,57 @@ def bitwise_and(
return paddle.bitwise_and(x1, x2)
+@with_supported_dtypes(
+ {"2.5.1 and below": ("float32", "float64", "complex")},
+ backend_version,
+)
def ceil(x: paddle.Tensor, /, *, out: Optional[paddle.Tensor] = None) -> paddle.Tensor:
- x_dtype = x.dtype
- if x_dtype in [
- paddle.int8,
- paddle.int16,
- paddle.int32,
- paddle.uint8,
- paddle.float16,
- paddle.complex64,
- paddle.complex128,
- paddle.bool,
- ]:
- if paddle.is_complex(x):
- return paddle.complex(paddle.ceil(x.real()), paddle.ceil(x.imag()))
- return paddle.ceil(x.astype("float32")).astype(x_dtype)
- elif x_dtype == paddle.int64:
- return paddle.ceil(x.astype("float64")).astype(x_dtype)
+ if paddle.is_complex(x):
+ return paddle.complex(paddle.ceil(x.real()), paddle.ceil(x.imag()))
return paddle.ceil(x)
+@with_supported_dtypes(
+ {"2.5.1 and below": ("float16", "float32", "float64", "complex")},
+ backend_version,
+)
def floor(x: paddle.Tensor, /, *, out: Optional[paddle.Tensor] = None) -> paddle.Tensor:
- x_dtype = x.dtype
- if x_dtype in [
- paddle.int8,
- paddle.int16,
- paddle.int32,
- paddle.uint8,
- paddle.float16,
- paddle.complex64,
- paddle.complex128,
- paddle.bool,
- ]:
- if paddle.is_complex(x):
- return paddle.complex(paddle.floor(x.real()), paddle.floor(x.imag()))
- return paddle.floor(x.astype("float32")).astype(x_dtype)
- elif x_dtype == paddle.int64:
- return paddle.floor(x.astype("float64")).astype(x_dtype)
+ if paddle.is_complex(x):
+ return paddle.complex(paddle.floor(x.real()), paddle.floor(x.imag()))
return paddle.floor(x)
-@with_unsupported_device_and_dtypes(
- {"2.5.1 and below": {"cpu": ("bool", "bfloat16")}},
+@with_supported_device_and_dtypes(
+ {
+ "2.5.2 and below": {
+ "cpu": (
+ "float32",
+ "float64",
+ )
+ }
+ },
backend_version,
)
def asin(x: paddle.Tensor, /, *, out: Optional[paddle.Tensor] = None) -> paddle.Tensor:
- if x.dtype in [
- paddle.int8,
- paddle.int16,
- paddle.int32,
- paddle.int64,
- paddle.uint8,
- paddle.float16,
- ]:
- ret_dtype = x.dtype
- return paddle.asin(x.astype("float32")).astype(ret_dtype)
- if paddle.is_complex(x):
- asinh_iz = paddle_backend.asinh(paddle.complex(-x.imag(), x.real()))
- return paddle.complex(asinh_iz.imag(), -asinh_iz.real())
return paddle.asin(x)
-@with_unsupported_device_and_dtypes(
- {"2.5.1 and below": {"cpu": ("bool", "bfloat16")}},
+@with_supported_dtypes(
+ {
+ "2.5.2 and below": (
+ "float16",
+ "float32",
+ "float64",
+ )
+ },
backend_version,
)
def asinh(x: paddle.Tensor, /, *, out: Optional[paddle.Tensor] = None) -> paddle.Tensor:
- if x.dtype in [
- paddle.int8,
- paddle.int16,
- paddle.int32,
- paddle.int64,
- paddle.uint8,
- paddle.float16,
- ]:
- ret_dtype = x.dtype
- return paddle.asinh(x.astype("float32")).astype(ret_dtype)
- if paddle.is_complex(x):
- # From https://github.com/python/cpython/blob/39ef93edb9802dccdb6555d4209ac2e60875a011/Modules/cmathmodule.c#L276 # noqa
- s1 = paddle_backend.sqrt(paddle.complex(1 + x.imag(), -x.real()))
- s2 = paddle_backend.sqrt(paddle.complex(1 - x.imag(), x.real()))
- return paddle.complex(
- paddle.asinh(s1.real() * s2.imag() - s2.real() * s1.imag()),
- paddle.atan2(x.imag(), s1.real() * s2.real() - s1.imag() * s2.imag()),
- )
return paddle.asinh(x)
-@with_unsupported_device_and_dtypes(
- {"2.5.1 and below": {"cpu": ("complex64", "complex128")}},
+@with_supported_device_and_dtypes(
+ {"2.5.2 and below": {"cpu": ("float16", "float32", "float64", "complex")}},
backend_version,
)
def sign(
@@ -251,50 +228,12 @@ def sign(
np_variant: Optional[bool] = True,
out: Optional[paddle.Tensor] = None,
) -> paddle.Tensor:
- if x.dtype in [
- paddle.int8,
- paddle.int16,
- paddle.int32,
- paddle.int64,
- paddle.uint8,
- paddle.float16,
- paddle.bfloat16,
- paddle.bool,
- ]:
- return paddle.sgn(x.astype("float32")).astype(x.dtype)
return paddle.sgn(x)
-# TODO: Remove `float16` from the list once paddle add it's supporting kernel to `CPU`.
-def _determine_sqrt_dtype_cast(
- dtype: Type[paddle.Tensor],
-) -> Tuple[Optional[str], Optional[str]]:
- """
- Determine the appropriate casting dtype for sqrt operations.
-
- Returns:
- (intermediate_dtype, output_dtype)
- """
- cast_and_return_float32_dtype = {
- paddle.int8,
- paddle.int16,
- paddle.int32,
- paddle.uint8,
- paddle.bool,
- }
-
- if dtype in cast_and_return_float32_dtype:
- return "float32", "float32"
- elif dtype == paddle.int64:
- return "float64", "float64"
- elif dtype == paddle.float16:
- return "float32", "float16"
- elif dtype == paddle.bfloat16:
- return "float32", "bfloat16"
- else:
- return None, None
-
-
+@with_supported_dtypes(
+ {"2.5.1 and below": ("float32", "float64", "complex")}, backend_version
+)
def sqrt(x: paddle.Tensor, /, *, out: Optional[paddle.Tensor] = None) -> paddle.Tensor:
"""Calculate the square root with type handling."""
if paddle.is_complex(x):
@@ -303,116 +242,85 @@ def sqrt(x: paddle.Tensor, /, *, out: Optional[paddle.Tensor] = None) -> paddle.
paddle.cos(angle / 2), paddle.sin(angle / 2)
) * paddle.sqrt(paddle.abs(x))
- if x.dtype in {paddle.float32, paddle.float64}:
- return paddle.sqrt(x)
+ return paddle.sqrt(x)
- intermediate_dtype, output_dtype = _determine_sqrt_dtype_cast(x.dtype)
- if intermediate_dtype:
- result = paddle.sqrt(x.astype(intermediate_dtype))
- return result.astype(output_dtype)
- raise ValueError(f"Unsupported data type for sqrt: {x.dtype}")
-
-
-@with_unsupported_device_and_dtypes(
- {"2.5.1 and below": {"cpu": ("bool", "bfloat16")}},
+@with_supported_device_and_dtypes(
+ {
+ "2.5.2 and below": {
+ "cpu": (
+ "float32",
+ "float64",
+ )
+ }
+ },
backend_version,
)
def cosh(x: paddle.Tensor, /, *, out: Optional[paddle.Tensor] = None) -> paddle.Tensor:
- if x.dtype in [
- paddle.int8,
- paddle.int16,
- paddle.int32,
- paddle.int64,
- paddle.uint8,
- paddle.float16,
- ]:
- ret_dtype = x.dtype
- return paddle.cosh(x.astype("float32")).astype(ret_dtype)
- if paddle.is_complex(x):
- re = x.real()
- im = x.imag()
- return paddle.complex(
- paddle.cosh(re) * paddle.cos(im), paddle.sinh(re) * paddle.sin(im)
- )
return paddle.cosh(x)
+@with_supported_dtypes(
+ {"2.5.1 and below": ("float32", "float64", "complex")}, backend_version
+)
def log10(x: paddle.Tensor, /, *, out: Optional[paddle.Tensor] = None) -> paddle.Tensor:
- if x.dtype in [
- paddle.int8,
- paddle.int16,
- paddle.int32,
- paddle.int64,
- paddle.uint8,
- paddle.float16,
- paddle.complex64,
- paddle.complex128,
- paddle.bool,
- ]:
- if paddle.is_complex(x):
- base = paddle.to_tensor(10.0).squeeze()
- return paddle_backend.divide(
- paddle_backend.log(x), paddle_backend.log(base)
- ).astype(x.dtype)
- return paddle.log10(x.astype("float32")).astype(x.dtype)
+ if paddle.is_complex(x):
+ base = paddle.to_tensor(10.0).squeeze()
+ return paddle_backend.divide(
+ paddle_backend.log(x), paddle_backend.log(base)
+ ).astype(x.dtype)
return paddle.log10(x)
+@with_supported_dtypes(
+ {"2.5.1 and below": ("float32", "float64", "complex")},
+ backend_version,
+)
def log2(x: paddle.Tensor, /, *, out: Optional[paddle.Tensor] = None) -> paddle.Tensor:
- if x.dtype in [
- paddle.int8,
- paddle.int16,
- paddle.int32,
- paddle.int64,
- paddle.uint8,
- paddle.float16,
- paddle.complex64,
- paddle.complex128,
- paddle.bool,
- ]:
- if paddle.is_complex(x):
- base = paddle.to_tensor(2.0).squeeze()
- return paddle_backend.divide(
- paddle_backend.log(x), paddle_backend.log(base)
- ).astype(x.dtype)
- return paddle.log2(x.astype("float32")).astype(x.dtype)
+ if paddle.is_complex(x):
+ base = paddle.to_tensor(2.0).squeeze()
+ return paddle_backend.divide(
+ paddle_backend.log(x), paddle_backend.log(base)
+ ).astype(x.dtype)
return paddle.log2(x)
+@with_supported_dtypes(
+ {"2.5.1 and below": ("float32", "float64", "complex")},
+ backend_version,
+)
def log1p(x: paddle.Tensor, /, *, out: Optional[paddle.Tensor] = None) -> paddle.Tensor:
- if x.dtype in [
- paddle.int8,
- paddle.int16,
- paddle.int32,
- paddle.int64,
- paddle.uint8,
- paddle.float16,
- paddle.complex64,
- paddle.complex128,
- paddle.bool,
- ]:
- if paddle.is_complex(x):
- return paddle_backend.log(x + 1)
- return paddle.log1p(x.astype("float32")).astype(x.dtype)
+ if paddle.is_complex(x):
+ return paddle.complex(paddle.log1p(paddle.abs(x)), paddle.angle(x + 1))
return paddle.log1p(x)
+@with_supported_dtypes(
+ {
+ "2.5.1 and below": (
+ "float",
+ "int32",
+ "int64",
+ "complex",
+ )
+ },
+ backend_version,
+)
def isnan(x: paddle.Tensor, /, *, out: Optional[paddle.Tensor] = None) -> paddle.Tensor:
- if x.dtype in [
- paddle.int8,
- paddle.int16,
- paddle.uint8,
- paddle.complex64,
- paddle.complex128,
- paddle.bool,
- ]:
- if paddle.is_complex(x):
- return paddle.logical_or(paddle.isnan(x.real()), paddle.isnan(x.imag()))
- return paddle.isnan(x.astype("float32"))
+ if paddle.is_complex(x):
+ return paddle.logical_or(paddle.isnan(x.real()), paddle.isnan(x.imag()))
return paddle.isnan(x)
+@with_unsupported_dtypes(
+ {
+ "2.5.1 and below": (
+ "int8",
+ "uint8",
+ )
+ },
+ backend_version,
+)
def less(
x1: Union[float, paddle.Tensor],
x2: Union[float, paddle.Tensor],
@@ -421,16 +329,25 @@ def less(
out: Optional[paddle.Tensor] = None,
) -> paddle.Tensor:
x1, x2, ret_dtype = _elementwise_helper(x1, x2)
- if x1.dtype in [paddle.int8, paddle.uint8, paddle.complex64, paddle.complex128]:
- if paddle.is_complex(x1):
- real = paddle.less_than(x1.real(), x2.real())
- imag = paddle.less_than(x1.imag(), x2.imag())
- return logical_and(real, imag)
- return paddle.less_than(x1.astype("float32"), x2.astype("float32"))
+ if paddle.is_complex(x1):
+ real = paddle.less_than(x1.real(), x2.real())
+ imag = paddle.less_than(x1.imag(), x2.imag())
+ return logical_and(real, imag)
return paddle.less_than(x1, x2)
+@with_unsupported_dtypes(
+ {
+ "2.5.1 and below": (
+ "int8",
+ "int16",
+ "uint8",
+ "float16",
+ )
+ },
+ backend_version,
+)
def multiply(
x1: Union[float, paddle.Tensor],
x2: Union[float, paddle.Tensor],
@@ -439,48 +356,39 @@ def multiply(
out: Optional[paddle.Tensor] = None,
) -> paddle.Tensor:
x1, x2, ret_dtype = _elementwise_helper(x1, x2)
- if x1.dtype in [paddle.int8, paddle.int16, paddle.uint8, paddle.float16]:
- x1, x2 = x1.astype("float32"), x2.astype("float32")
return paddle.multiply(x1, x2).astype(ret_dtype)
-@with_unsupported_device_and_dtypes(
- {"2.5.1 and below": {"cpu": ("bool", "bfloat16")}},
+@with_supported_device_and_dtypes(
+ {
+ "2.5.2 and below": {
+ "cpu": (
+ "float32",
+ "float64",
+ )
+ }
+ },
backend_version,
)
def cos(x: paddle.Tensor, /, *, out: Optional[paddle.Tensor] = None) -> paddle.Tensor:
- if x.dtype in [
- paddle.int8,
- paddle.int16,
- paddle.int32,
- paddle.int64,
- paddle.uint8,
- paddle.float16,
- ]:
- ret_dtype = x.dtype
- return paddle.cos(x.astype("float32")).astype(ret_dtype)
- if paddle.is_complex(x):
- re = x.real()
- im = x.imag()
- return paddle.complex(
- paddle.cos(re) * paddle.cosh(im),
- -paddle.sin(re) * paddle.sinh(im),
- )
return paddle.cos(x)
+@with_unsupported_dtypes({"2.5.1 and below": ("uint", "float16")}, backend_version)
def logical_not(
x: paddle.Tensor, /, *, out: Optional[paddle.Tensor] = None
) -> paddle.Tensor:
- if x.dtype in [paddle.uint8, paddle.float16, paddle.complex64, paddle.complex128]:
- if paddle.is_complex(x):
- return paddle.logical_and(
- paddle.logical_not(x.real()), paddle.logical_not(x.imag())
- )
- return paddle.logical_not(x.astype("float32"))
+ if paddle.is_complex(x):
+ return paddle.logical_and(
+ paddle.logical_not(x.real()), paddle.logical_not(x.imag())
+ )
return paddle.logical_not(x)
+@with_supported_dtypes(
+ {"2.5.1 and below": ("float32", "float64", "int32", "int64", "complex")},
+ backend_version,
+)
def divide(
x1: Union[float, paddle.Tensor],
x2: Union[float, paddle.Tensor],
@@ -488,16 +396,18 @@ def divide(
*,
out: Optional[paddle.Tensor] = None,
) -> paddle.Tensor:
+ if paddle.is_complex(x1) or paddle.is_complex(x2):
+ angle_value = paddle.angle(x1) - paddle.angle(x2)
+ abs_value = paddle.abs(x1) / paddle.abs(x2)
+ return paddle.complex(
+ abs_value * paddle.cos(angle_value), abs_value * paddle.sin(angle_value)
+ )
x1, x2, ret_dtype = _elementwise_helper(x1, x2)
- if x1.dtype in [paddle.float16, paddle.bfloat16]:
- x1, x2 = x1.astype("float32"), x2.astype("float32")
- if not (ivy.is_float_dtype(ret_dtype) or ivy.is_complex_dtype(ret_dtype)):
- ret_dtype = ivy.default_float_dtype(as_native=True)
return (x1 / x2).astype(ret_dtype)
@with_supported_dtypes(
- {"2.5.1 and below": ("float64", "float32", "int64", "int64")},
+ {"2.5.2 and below": ("float32", "float64", "int32", "int64")},
backend_version,
)
def fmin(
@@ -512,6 +422,27 @@ def fmin(
return paddle.fmin(x1, x2)
+def _apply_for_real_and_imag(fn, x1, x2):
+ return fn(
+ fn(x1.real(), x2.real()),
+ fn(x1.imag(), x2.imag()),
+ )
+
+
+@with_supported_dtypes(
+ {
+ "2.5.1 and below": (
+ "bool",
+ "float32",
+ "float64",
+ "int16",
+ "int32",
+ "int64",
+ "complex",
+ )
+ },
+ backend_version,
+)
def greater(
x1: Union[float, paddle.Tensor],
x2: Union[float, paddle.Tensor],
@@ -520,16 +451,28 @@ def greater(
out: Optional[paddle.Tensor] = None,
) -> paddle.Tensor:
x1, x2, ret_dtype = _elementwise_helper(x1, x2)
- if x1.dtype in [paddle.int8, paddle.uint8, paddle.complex64, paddle.complex128]:
+ if paddle.is_complex(x1):
if paddle.is_complex(x1):
- if paddle.is_complex(x1):
- real = paddle.greater_than(x1.real(), x2.real())
- imag = paddle.greater_than(x1.imag(), x2.imag())
- return paddle.logical_and(real, imag)
- return paddle.greater_than(x1.astype("float32"), x2.astype("float32"))
+ real = paddle.greater_than(x1.real(), x2.real())
+ imag = paddle.greater_than(x1.imag(), x2.imag())
+ return paddle.logical_and(real, imag)
return paddle.greater_than(x1, x2)
+@with_supported_dtypes(
+ {
+ "2.5.1 and below": (
+ "bool",
+ "float32",
+ "float64",
+ "int16",
+ "int32",
+ "int64",
+ "complex",
+ )
+ },
+ backend_version,
+)
def greater_equal(
x1: Union[float, paddle.Tensor],
x2: Union[float, paddle.Tensor],
@@ -538,30 +481,27 @@ def greater_equal(
out: Optional[paddle.Tensor] = None,
) -> paddle.Tensor:
x1, x2, ret_dtype = _elementwise_helper(x1, x2)
- if x1.dtype in [paddle.int8, paddle.uint8, paddle.complex64, paddle.complex128]:
+ if paddle.is_complex(x1):
if paddle.is_complex(x1):
- if paddle.is_complex(x1):
- real = paddle.greater_equal(x1.real(), x2.real())
- imag = paddle.greater_equal(x1.imag(), x2.imag())
- return paddle.logical_and(real, imag)
- return paddle.greater_equal(x1.astype("float32"), x2.astype("float32"))
+ real = paddle.greater_equal(x1.real(), x2.real())
+ imag = paddle.greater_equal(x1.imag(), x2.imag())
+ return paddle.logical_and(real, imag)
return paddle.greater_equal(x1, x2)
-@with_unsupported_device_and_dtypes(
- {"2.5.1 and below": {"cpu": ("bool", "bfloat16")}},
+@with_supported_device_and_dtypes(
+ {
+ "2.5.2 and below": {
+ "cpu": (
+ "float32",
+ "float64",
+ "complex",
+ )
+ }
+ },
backend_version,
)
def acos(x: paddle.Tensor, /, *, out: Optional[paddle.Tensor] = None) -> paddle.Tensor:
- if x.dtype in [
- paddle.int8,
- paddle.int16,
- paddle.int32,
- paddle.int64,
- paddle.uint8,
- paddle.float16,
- ]:
- return paddle.acos(x.astype("float32")).astype(x.dtype)
if paddle.is_complex(x):
# From https://github.com/python/cpython/blob/39ef93edb9802dccdb6555d4209ac2e60875a011/Modules/cmathmodule.c#L178 # noqa
s1 = paddle_backend.sqrt(1 - x)
@@ -573,75 +513,66 @@ def acos(x: paddle.Tensor, /, *, out: Optional[paddle.Tensor] = None) -> paddle.
return paddle.acos(x)
-@with_unsupported_device_and_dtypes(
- {"2.5.1 and below": {"cpu": ("complex64", "complex128")}},
+@with_supported_device_and_dtypes(
+ {
+ "2.5.2 and below": {
+ "cpu": ("bool", "float32", "int32", "float64", "int64", "complex")
+ }
+ },
backend_version,
)
def logical_xor(
x1: paddle.Tensor, x2: paddle.Tensor, /, *, out: Optional[paddle.Tensor] = None
) -> paddle.Tensor:
x1, x2, ret_dtype = _elementwise_helper(x1, x2)
- if ret_dtype in [paddle.uint8, paddle.float16, paddle.complex64, paddle.complex128]:
- # this logic works well when both inputs are complex but when one of them
- # is casted from real to complex, the imaginary part is zero which messes
- # with the XOR logic
- # if paddle.is_complex(x1):
- # return paddle.logical_xor(
- # paddle.logical_xor(x1.real(), x2.real()),
- # paddle.logical_xor(x1.imag(), x2.imag()),
- # )
- return paddle.logical_xor(x1.astype("float32"), x2.astype("float32"))
+ if paddle.is_complex(x1):
+ return _apply_for_real_and_imag(paddle.logical_xor, x1, x2)
return paddle.logical_xor(x1, x2)
-@with_unsupported_device_and_dtypes(
- {"2.5.1 and below": {"cpu": ("complex64", "complex128")}},
+@with_supported_device_and_dtypes(
+ {
+ "2.5.2 and below": {
+ "cpu": ("bool", "float32", "int32", "float64", "int64", "complex")
+ }
+ },
backend_version,
)
def logical_and(
x1: paddle.Tensor, x2: paddle.Tensor, /, *, out: Optional[paddle.Tensor] = None
) -> paddle.Tensor:
x1, x2, ret_dtype = _elementwise_helper(x1, x2)
- if ret_dtype in [paddle.uint8, paddle.float16, paddle.complex64, paddle.complex128]:
- # this logic works well when both inputs are complex but when one of them
- # is casted from real to complex, the imaginary part is zero which messes
- # if paddle.is_complex(x1):
- # return paddle.logical_and(
- # paddle.logical_and(x1.real(), x2.real()),
- # paddle.logical_and(x1.imag(), x2.imag()),
- # )
- return paddle.logical_and(x1.astype("float32"), x2.astype("float32"))
+ if paddle.is_complex(x1):
+ return _apply_for_real_and_imag(paddle.logical_and, x1, x2)
return paddle.logical_and(x1, x2)
+@with_supported_dtypes(
+ {"2.5.1 and below": ("bool", "float32", "int32", "float64", "int64", "complex")},
+ backend_version,
+)
def logical_or(
x1: paddle.Tensor, x2: paddle.Tensor, /, *, out: Optional[paddle.Tensor] = None
) -> paddle.Tensor:
x1, x2, ret_dtype = _elementwise_helper(x1, x2)
- if ret_dtype in [paddle.uint8, paddle.float16, paddle.complex64, paddle.complex128]:
- if paddle.is_complex(x1):
- return paddle.logical_or(
- paddle.logical_or(x1.real(), x2.real()),
- paddle.logical_or(x1.imag(), x2.imag()),
- )
- return paddle.logical_or(x1.astype("float32"), x2.astype("float32"))
+ if paddle.is_complex(x1):
+ return _apply_for_real_and_imag(paddle.logical_or, x1, x2)
return paddle.logical_or(x1, x2)
-@with_unsupported_device_and_dtypes(
- {"2.5.1 and below": {"cpu": ("bool", "bfloat16")}},
+@with_supported_device_and_dtypes(
+ {
+ "2.5.2 and below": {
+ "cpu": (
+ "float32",
+ "float64",
+ "complex",
+ )
+ }
+ },
backend_version,
)
def acosh(x: paddle.Tensor, /, *, out: Optional[paddle.Tensor] = None) -> paddle.Tensor:
- if x.dtype in [
- paddle.int8,
- paddle.int16,
- paddle.int32,
- paddle.int64,
- paddle.uint8,
- paddle.float16,
- ]:
- return paddle.acosh(x.astype("float32")).astype(x.dtype)
if paddle.is_complex(x):
# From https://github.com/python/cpython/blob/39ef93edb9802dccdb6555d4209ac2e60875a011/Modules/cmathmodule.c#L221 # noqa
s1 = paddle_backend.sqrt(paddle.complex(x.real() - 1, x.imag()))
@@ -653,20 +584,11 @@ def acosh(x: paddle.Tensor, /, *, out: Optional[paddle.Tensor] = None) -> paddle
return paddle.acosh(x)
-@with_unsupported_device_and_dtypes(
- {"2.5.1 and below": {"cpu": ("bool", "bfloat16")}},
+@with_supported_device_and_dtypes(
+ {"2.5.2 and below": {"cpu": ("float32", "float64", "complex")}},
backend_version,
)
def sin(x: paddle.Tensor, /, *, out: Optional[paddle.Tensor] = None) -> paddle.Tensor:
- if x.dtype in [
- paddle.int8,
- paddle.int16,
- paddle.int32,
- paddle.int64,
- paddle.uint8,
- paddle.float16,
- ]:
- return paddle.sin(x.astype("float32")).astype(x.dtype)
if paddle.is_complex(x):
re = x.real()
im = x.imag()
@@ -676,15 +598,13 @@ def sin(x: paddle.Tensor, /, *, out: Optional[paddle.Tensor] = None) -> paddle.T
return paddle.sin(x)
+@with_supported_dtypes(
+ {"2.5.1 and below": ("float32", "float64", "int8", "int16", "int32", "int64")},
+ backend_version,
+)
def negative(
x: Union[float, paddle.Tensor], /, *, out: Optional[paddle.Tensor] = None
) -> paddle.Tensor:
- if not isinstance(x, paddle.Tensor):
- x = paddle.to_tensor(
- x, dtype=ivy.default_dtype(item=x, as_native=True)
- ).squeeze()
- if x.dtype == paddle.bool:
- return paddle.logical_not(x)
return paddle.neg(x)
@@ -698,22 +618,11 @@ def not_equal(
return paddle.logical_not(paddle_backend.equal(x1, x2))
-@with_unsupported_device_and_dtypes(
- {"2.5.1 and below": {"cpu": ("bool", "bfloat16")}},
+@with_supported_device_and_dtypes(
+ {"2.5.2 and below": {"cpu": ("bfloat16", "float32", "float64", "complex")}},
backend_version,
)
-def tanh(
- x: paddle.Tensor, /, *, complex_mode="jax", out: Optional[paddle.Tensor] = None
-) -> paddle.Tensor:
- if x.dtype in [
- paddle.int8,
- paddle.int16,
- paddle.int32,
- paddle.int64,
- paddle.uint8,
- paddle.float16,
- ]:
- return paddle.tanh(x.astype("float32")).astype(x.dtype)
+def tanh(x: paddle.Tensor, /, *, out: Optional[paddle.Tensor] = None) -> paddle.Tensor:
if paddle.is_complex(x):
tanh_a = paddle.tanh(x.real())
tan_b = paddle.tan(x.imag())
@@ -727,6 +636,21 @@ def tanh(
return paddle.tanh(x)
+@with_supported_dtypes(
+ {
+ "2.5.1 and below": (
+ "uint8",
+ "int8",
+ "int32",
+ "int64",
+ "float32",
+ "float64",
+ "float16",
+ "bfloat16",
+ )
+ },
+ backend_version,
+)
def floor_divide(
x1: Union[float, paddle.Tensor],
x2: Union[float, paddle.Tensor],
@@ -735,11 +659,13 @@ def floor_divide(
out: Optional[paddle.Tensor] = None,
) -> paddle.Tensor:
x1, x2, ret_dtype = _elementwise_helper(x1, x2)
- if x1.dtype in [paddle.int32, paddle.int64]:
- return paddle.floor_divide(x1, x2)
- return paddle_backend.floor(paddle_backend.divide(x1, x2)).astype(ret_dtype)
+ return paddle.floor_divide(x1, x2)
+@with_supported_dtypes(
+ {"2.5.1 and below": ("bool", "uint8", "int8", "int16", "int32", "int64")},
+ backend_version,
+)
def bitwise_or(
x1: Union[int, bool, paddle.Tensor],
x2: Union[int, bool, paddle.Tensor],
@@ -751,21 +677,10 @@ def bitwise_or(
return paddle.bitwise_or(x1, x2)
-@with_unsupported_device_and_dtypes(
- {"2.5.1 and below": {"cpu": ("bool", "bfloat16")}},
- backend_version,
+@with_supported_dtypes(
+ {"2.5.2 and below": ("float32", "float64", "complex")}, backend_version
)
def sinh(x: paddle.Tensor, /, *, out: Optional[paddle.Tensor] = None) -> paddle.Tensor:
- if x.dtype in [
- paddle.int8,
- paddle.int16,
- paddle.int32,
- paddle.int64,
- paddle.uint8,
- paddle.float16,
- ]:
- ret_dtype = x.dtype
- return paddle.sinh(x.astype("float32")).astype(ret_dtype)
if paddle.is_complex(x):
re = x.real()
im = x.imag()
@@ -785,21 +700,27 @@ def positive(
return x.clone()
+@with_supported_dtypes(
+ {
+ "2.5.1 and below": (
+ "int32",
+ "int64",
+ "float32",
+ "float64",
+ "complex",
+ )
+ },
+ backend_version,
+)
def square(
x: paddle.Tensor, /, *, out: Optional[paddle.Tensor] = None
) -> paddle.Tensor:
- if x.dtype in [paddle.int32, paddle.int64, paddle.float32, paddle.float64]:
- return paddle.square(x)
- if paddle.is_complex(x):
- return paddle.complex(
- paddle.square(paddle.real(x)) - paddle.square(paddle.imag(x)),
- 2.0 * paddle.real(x) * paddle.imag(x),
- )
- return paddle_backend.pow(x, 2).astype(x.dtype)
+ return paddle.square(x)
-@with_unsupported_device_and_dtypes(
- {"2.5.1 and below": {"cpu": ("bfloat16",)}}, backend_version
+@with_supported_device_and_dtypes(
+ {"2.5.2 and below": {"cpu": ("float32", "float64", "int32", "int64", "complex")}},
+ backend_version,
)
def pow(
x1: paddle.Tensor,
@@ -809,14 +730,6 @@ def pow(
out: Optional[paddle.Tensor] = None,
) -> paddle.Tensor:
x1, x2, ret_dtype = _elementwise_helper(x1, x2)
- if x1.dtype in [
- paddle.int8,
- paddle.int16,
- paddle.uint8,
- paddle.float16,
- paddle.bool,
- ]:
- return paddle.pow(x1.astype("float32"), x2.astype("float32")).astype(ret_dtype)
if paddle.is_complex(x1):
# https://math.stackexchange.com/questions/476968/complex-power-of-a-complex-number
r = paddle.abs(x1)
@@ -828,61 +741,60 @@ def pow(
return paddle.pow(x1, x2)
-def round(
- x: paddle.Tensor, /, *, decimals: int = 0, out: Optional[paddle.Tensor] = None
-) -> paddle.Tensor:
- def _np_round(x):
- # this is a logic to mimic np.round behaviour
- # which rounds odd numbers up and even numbers down at limits like 0.5
+# Implementation based on TensorFlow's scalar_round_half_to_even_op logic
+# Reference: https://github.com/tensorflow/tensorflow/blob/7f1050a6976d11bfb0bb37bdfc82350c0a238faa/tensorflow/core/kernels/cwise_ops.h#L510 # noqa: E501
+def _round_half_to_even(x):
+ round_val = paddle_backend.floor(x + 0.5)
+ fraction = round_val - x
- one = paddle.to_tensor(1, dtype="int64")
+ # Identify elements with a fractional part of 0.5
+ mask = paddle_backend.equal(fraction, paddle.to_tensor(0.5, dtype=fraction.dtype))
- # check if the number is even or odd
- is_even = paddle.bitwise_and(paddle_backend.trunc(x).astype("int64"), one) == 0
+ # Round to the nearest even number if the fraction is 0.5
+ even_round_val = 2 * paddle_backend.floor(0.5 * x + 0.5)
- # round the number to the nearest integer
- round_x = paddle.sign(x) * paddle.where(
- is_even, paddle.floor(x.abs()), paddle.ceil(x.abs())
- )
+ # Combine the results
+ return paddle.where(mask, even_round_val, round_val)
- # if the number was rounded up from an even number
- # round the number down to the nearest even number
- return paddle.where(
- paddle.logical_and(
- paddle.bitwise_and(round_x.astype("int64"), one) == 1.0,
- is_even,
- ),
- round_x - 1.0,
- round_x,
- )
- if x.dtype not in [paddle.float32, paddle.float64]:
- if paddle.is_complex(x):
- return paddle.complex(_np_round(x.real()), _np_round(x.imag()))
- return _np_round(x.astype("float32")).astype(x.dtype)
- return _np_round(x).astype(x.dtype)
+# This function aims to mimic the behavior of np.round similar to how tf.experimental.numpy.round does # noqa: E501
+# Reference for tf.experimental.numpy.round:https://github.com/tensorflow/tensorflow/blob/v2.13.0/tensorflow/python/ops/numpy_ops/np_array_ops.py#L724 # noqa: E501
+@with_unsupported_device_and_dtypes(
+ {"2.5.2 and below": {"cpu": ("bfloat16", "float16", "complex")}}, backend_version
+)
+def round(
+ x: paddle.Tensor, /, *, decimals: int = 0, out: Optional[paddle.Tensor] = None
+) -> paddle.Tensor:
+ x = paddle.to_tensor(x, dtype=x.dtype)
+ dtype_ = x.dtype
+ factor = math.pow(10, decimals)
+ factor = paddle.to_tensor(factor)
+
+ # Handle floating point and complex numbers
+ if paddle.is_floating_point(x) or paddle.is_complex(x):
+ factor = paddle.to_tensor(factor)
+ factor = paddle.cast(factor, dtype_)
+ else:
+ float_dtype_ = paddle.float32 # paddle.get_default_dtype()
+ x = x.astype(float_dtype_)
+ factor = paddle.cast(factor, float_dtype_)
+
+ x = paddle.multiply(x, factor)
+ x = _round_half_to_even(x)
+ x = paddle.divide(x, factor)
+ return x.astype(dtype_)
+@with_supported_dtypes(
+ {"2.5.1 and below": ("float32", "float64", "complex")}, backend_version
+)
def trunc(x: paddle.Tensor, /, *, out: Optional[paddle.Tensor] = None) -> paddle.Tensor:
- if x.dtype in [
- paddle.int8,
- paddle.int16,
- paddle.uint8,
- paddle.float16,
- paddle.complex64,
- paddle.complex128,
- paddle.bool,
- ]:
- if paddle.is_complex(x):
- return paddle.complex(paddle.trunc(x.real()), paddle.trunc(x.imag()))
- return paddle.trunc(x.astype("float32")).astype(x.dtype)
+ if paddle.is_complex(x):
+ return paddle.complex(paddle.trunc(x.real()), paddle.trunc(x.imag()))
return paddle.trunc(x)
-@with_supported_dtypes(
- {"2.5.1 and below": ("float64", "float32")},
- backend_version,
-)
+@with_supported_dtypes({"2.5.2 and below": ("float64", "float32")}, backend_version)
def trapz(
y: paddle.Tensor,
/,
@@ -937,20 +849,11 @@ def abs(
) -> paddle.Tensor:
if not isinstance(x, paddle.Tensor):
x = paddle.to_tensor(x, dtype=ivy.default_dtype(item=x)).squeeze()
- if x.dtype in [
- paddle.int8,
- paddle.int16,
- paddle.uint8,
- paddle.float16,
- paddle.bfloat16,
- paddle.bool,
- ]:
- return paddle.abs(x.astype("float32")).astype(x.dtype)
return paddle.abs(x)
@with_unsupported_device_and_dtypes(
- {"2.5.1 and below": {"cpu": ("float16",)}}, backend_version
+ {"2.5.2 and below": {"cpu": ("float16",)}}, backend_version
)
def logaddexp(
x1: paddle.Tensor, x2: paddle.Tensor, /, *, out: Optional[paddle.Tensor] = None
@@ -963,7 +866,7 @@ def logaddexp(
@with_unsupported_device_and_dtypes(
- {"2.5.1 and below": {"cpu": ("float16",)}}, backend_version
+ {"2.5.2 and below": {"cpu": ("float16",)}}, backend_version
)
def logaddexp2(
x1: Union[paddle.Tensor, float, list, tuple],
@@ -978,7 +881,7 @@ def logaddexp2(
@with_unsupported_device_and_dtypes(
{
- "2.5.1 and below": {
+ "2.5.2 and below": {
"cpu": (
"int8",
"int16",
@@ -998,88 +901,69 @@ def real(x: paddle.Tensor, /, *, out: Optional[paddle.Tensor] = None) -> paddle.
return paddle.real(x)
-@with_unsupported_device_and_dtypes(
- {"2.5.1 and below": {"cpu": ("bool", "bfloat16")}},
+@with_supported_device_and_dtypes(
+ {"2.5.2 and below": {"cpu": ("float32", "float64", "complex")}},
backend_version,
)
def tan(x: paddle.Tensor, /, *, out: Optional[paddle.Tensor] = None) -> paddle.Tensor:
- if x.dtype in [
- paddle.int8,
- paddle.int16,
- paddle.int32,
- paddle.int64,
- paddle.uint8,
- paddle.float16,
- ]:
- ret_dtype = x.dtype
- return paddle.tan(x.astype("float32")).astype(ret_dtype)
if paddle.is_complex(x):
tanh_ix = paddle_backend.tanh(paddle.complex(-x.imag(), x.real()))
return paddle.complex(tanh_ix.imag(), -tanh_ix.real())
return paddle.tan(x)
-@with_unsupported_device_and_dtypes(
- {"2.5.1 and below": {"cpu": ("bool", "bfloat16")}},
+@with_supported_device_and_dtypes(
+ {"2.5.2 and below": {"cpu": ("float32", "float64", "complex")}},
backend_version,
)
def atan(x: paddle.Tensor, /, *, out: Optional[paddle.Tensor] = None) -> paddle.Tensor:
- if x.dtype in [
- paddle.int8,
- paddle.int16,
- paddle.int32,
- paddle.int64,
- paddle.uint8,
- paddle.float16,
- ]:
- ret_dtype = x.dtype
- return paddle.atan(x.astype("float32")).astype(ret_dtype)
if x.dtype in [paddle.complex64, paddle.complex128]:
atanh_iz = paddle_backend.atanh(paddle.complex(-x.imag(), x.real()))
return paddle.complex(atanh_iz.imag(), -atanh_iz.real())
return paddle.atan(x)
-@with_unsupported_device_and_dtypes(
- {"2.5.1 and below": {"cpu": ("complex64", "complex128", "bool")}},
+@with_supported_device_and_dtypes(
+ {
+ "2.5.2 and below": {
+ "cpu": (
+ "int32",
+ "int64",
+ "float32",
+ "float64",
+ )
+ }
+ },
backend_version,
)
def atan2(
x1: paddle.Tensor, x2: paddle.Tensor, /, *, out: Optional[paddle.Tensor] = None
) -> paddle.Tensor:
x1, x2, ret_dtype = _elementwise_helper(x1, x2)
- if x1.dtype in [paddle.int8, paddle.int16, paddle.uint8]:
- x1, x2 = x1.astype("float32"), x2.astype("float32")
return paddle.atan2(x1, x2).astype(ret_dtype)
+@with_supported_dtypes(
+ {"2.5.1 and below": ("float32", "float64", "complex")},
+ backend_version,
+)
def log(x: paddle.Tensor, /, *, out: Optional[paddle.Tensor] = None) -> paddle.Tensor:
- if x.dtype in [
- paddle.int8,
- paddle.int16,
- paddle.int32,
- paddle.int64,
- paddle.uint8,
- paddle.float16,
- paddle.complex64,
- paddle.complex128,
- paddle.bool,
- ]:
- if paddle.is_complex(x):
- return paddle.complex(paddle.log(paddle.abs(x)), paddle.angle(x))
- return paddle.log(x.astype("float32")).astype(x.dtype)
+ if paddle.is_complex(x):
+ return paddle.complex(paddle.log(paddle.abs(x)), paddle.angle(x))
return paddle.log(x)
+@with_supported_dtypes(
+ {"2.5.1 and below": ("int32", "int64", "float32", "float64", "complex")},
+ backend_version,
+)
def exp(x: paddle.Tensor, /, *, out: Optional[paddle.Tensor] = None) -> paddle.Tensor:
- if x.dtype in [paddle.int32, paddle.int64, paddle.float32, paddle.float64]:
- return paddle.exp(x)
if paddle.is_complex(x):
return paddle.multiply(
paddle.exp(x.real()),
paddle.complex(paddle.cos(x.imag()), paddle.sin(x.imag())),
)
- return paddle_backend.pow(math.e, x).astype(x.dtype)
+ return paddle.exp(x)
def exp2(
@@ -1092,6 +976,9 @@ def exp2(
return ivy.pow(2, x)
+@with_supported_dtypes(
+ {"2.5.1 and below": ("float32", "float64", "int32", "int64")}, backend_version
+)
def subtract(
x1: Union[float, paddle.Tensor],
x2: Union[float, paddle.Tensor],
@@ -1101,16 +988,14 @@ def subtract(
out: Optional[paddle.Tensor] = None,
) -> paddle.Tensor:
x1, x2, ret_dtype = _elementwise_helper(x1, x2)
- if x1.dtype in [paddle.int8, paddle.uint8, paddle.float16, paddle.bool]:
- x1, x2 = x1.astype("float32"), x2.astype("float32")
if alpha not in (1, None):
x2 = paddle_backend.multiply(x2, alpha)
x1, x2 = ivy.promote_types_of_inputs(x1, x2)
return paddle.subtract(x1, x2).astype(ret_dtype)
-@with_unsupported_device_and_dtypes(
- {"2.5.1 and below": {"cpu": ("complex64", "complex128", "bool")}},
+@with_supported_device_and_dtypes(
+ {"2.5.2 and below": {"cpu": ("float32", "float64", "int32", "int64")}},
backend_version,
)
def remainder(
@@ -1132,26 +1017,14 @@ def remainder(
diff = paddle_backend.subtract(res, res_floored).astype(res.dtype)
return paddle_backend.round(paddle_backend.multiply(diff, x2)).astype(x1.dtype)
- if x1.dtype in [paddle.int8, paddle.int16, paddle.uint8, paddle.float16]:
- x1, x2 = x1.astype("float32"), x2.astype("float32")
return paddle.remainder(x1, x2).astype(ret_dtype)
-@with_unsupported_device_and_dtypes(
- {"2.5.1 and below": {"cpu": ("bool", "bfloat16")}},
+@with_supported_device_and_dtypes(
+ {"2.5.2 and below": {"cpu": ("float32", "float64", "complex")}},
backend_version,
)
def atanh(x: paddle.Tensor, /, *, out: Optional[paddle.Tensor] = None) -> paddle.Tensor:
- if x.dtype in [
- paddle.int8,
- paddle.int16,
- paddle.int32,
- paddle.int64,
- paddle.uint8,
- paddle.float16,
- ]:
- ret_dtype = x.dtype
- return paddle.atanh(x.astype("float32")).astype(ret_dtype)
if paddle.is_complex(x):
return 0.5 * (paddle_backend.log(1 + x) - paddle_backend.log(1 - x))
return paddle.atanh(x)
@@ -1187,17 +1060,15 @@ def bitwise_left_shift(
# ------#
-@with_unsupported_device_and_dtypes(
- {"2.5.1 and below": {"cpu": ("complex64", "complex128", "bool")}},
- backend_version,
-)
+@with_supported_dtypes({"2.5.2 and below": ("float32", "float64")}, backend_version)
def erf(x: paddle.Tensor, /, *, out: Optional[paddle.Tensor] = None) -> paddle.Tensor:
- # TODO: add support for complex x, supported in scipy only atm
- if x.dtype in [paddle.int8, paddle.int16, paddle.int32, paddle.int64, paddle.uint8]:
- return paddle.erf(x.astype("float32")).astype(x.dtype)
return paddle.erf(x)
+@with_supported_dtypes(
+ {"2.5.1 and below": ("float32", "float64", "int32", "int64", "complex")},
+ backend_version,
+)
def minimum(
x1: Union[float, paddle.Tensor],
x2: Union[float, paddle.Tensor],
@@ -1207,19 +1078,8 @@ def minimum(
out: Optional[paddle.Tensor] = None,
) -> paddle.Tensor:
x1, x2, ret_dtype = _elementwise_helper(x1, x2)
- if x1.dtype in [
- paddle.int8,
- paddle.int16,
- paddle.uint8,
- paddle.float16,
- paddle.complex64,
- paddle.complex128,
- paddle.bool,
- ]:
- if paddle.is_complex(x1):
- use_where = True
- else:
- x1, x2 = x1.astype("float32"), x2.astype("float32")
+ if paddle.is_complex(x1):
+ use_where = True
if use_where:
return paddle_backend.where(paddle_backend.less_equal(x1, x2), x1, x2).astype(
@@ -1229,6 +1089,10 @@ def minimum(
return paddle.minimum(x1, x2).astype(ret_dtype)
+@with_supported_dtypes(
+ {"2.5.1 and below": ("float32", "float64", "int32", "int64", "complex")},
+ backend_version,
+)
def maximum(
x1: Union[float, paddle.Tensor],
x2: Union[float, paddle.Tensor],
@@ -1238,19 +1102,8 @@ def maximum(
out: Optional[paddle.Tensor] = None,
) -> paddle.Tensor:
x1, x2, ret_dtype = _elementwise_helper(x1, x2)
- if x1.dtype in [
- paddle.int8,
- paddle.int16,
- paddle.uint8,
- paddle.float16,
- paddle.complex64,
- paddle.complex128,
- paddle.bool,
- ]:
- if paddle.is_complex(x1):
- use_where = True
- else:
- x1, x2 = x1.astype("float32"), x2.astype("float32")
+ if paddle.is_complex(x1):
+ use_where = True
if use_where:
return paddle_backend.where(
paddle_backend.greater_equal(x1, x2), x1, x2
@@ -1258,27 +1111,36 @@ def maximum(
return paddle.maximum(x1, x2).astype(ret_dtype)
+@with_supported_dtypes(
+ {
+ "2.5.1 and below": (
+ "float32",
+ "float64",
+ )
+ },
+ backend_version,
+)
def reciprocal(
x: Union[float, paddle.Tensor], /, *, out: Optional[paddle.Tensor] = None
) -> paddle.Tensor:
- if x.dtype in [paddle.float32, paddle.float64]:
- return paddle.reciprocal(x)
- return paddle_backend.divide(1, x)
+ return paddle.reciprocal(x)
+@with_supported_dtypes(
+ {"2.5.1 and below": ("float32", "float64", "int32", "int64")}, backend_version
+)
def deg2rad(
x: paddle.Tensor, /, *, out: Optional[paddle.Tensor] = None
) -> paddle.Tensor:
- if x.dtype in [paddle.int32, paddle.int64, paddle.bool]:
- return paddle.deg2rad(x.astype("float32")).astype(x.dtype)
return paddle.deg2rad(x)
+@with_supported_dtypes(
+ {"2.5.1 and below": ("float32", "float64", "int32", "int64")}, backend_version
+)
def rad2deg(
x: paddle.Tensor, /, *, out: Optional[paddle.Tensor] = None
) -> paddle.Tensor:
- if x.dtype in [paddle.int32, paddle.int64, paddle.bool]:
- return paddle.rad2deg(x.astype("float32")).astype(x.dtype)
return paddle.rad2deg(x)
@@ -1313,10 +1175,7 @@ def fmod(
return paddle_backend.where(paddle_backend.less(x1, 0), -res, res)
-@with_unsupported_device_and_dtypes(
- {"2.5.1 and below": {"cpu": ("int8", "uint8")}},
- backend_version,
-)
+@with_supported_dtypes({"2.5.2 and below": ("int32", "int64")}, backend_version)
def lcm(
x1: paddle.Tensor,
x2: paddle.Tensor,
@@ -1324,18 +1183,19 @@ def lcm(
*,
out: Optional[paddle.Tensor] = None,
) -> paddle.Tensor:
- x1_dtype = x1.dtype
- x2_dtype = x2.dtype
- if (x1_dtype, x2_dtype) == (paddle.int16, paddle.int16):
- return paddle.cast(
- paddle.lcm(paddle.cast(x1, paddle.int32), paddle.cast(x2, paddle.int32)),
- paddle.int16,
- )
- elif x1_dtype != x2_dtype:
- x1, x2 = ivy.promote_types_of_inputs(x1, x2)
return paddle.lcm(x1, x2)
+@with_supported_dtypes(
+ {
+ "2.5.1 and below": (
+ "float32",
+ "float64",
+ "complex",
+ )
+ },
+ backend_version,
+)
def angle(
input: paddle.Tensor,
/,
@@ -1349,8 +1209,8 @@ def angle(
return result
-@with_unsupported_device_and_dtypes(
- {"2.5.1 and below": {"cpu": ("int8", "int16", "uint8")}}, backend_version
+@with_supported_device_and_dtypes(
+ {"2.5.2 and below": {"cpu": ("int32", "int64")}}, backend_version
)
def gcd(
x1: Union[paddle.Tensor, int, list, tuple],
@@ -1363,24 +1223,7 @@ def gcd(
return paddle.gcd(x1, x2)
-@with_unsupported_device_and_dtypes(
- {
- "2.5.1 and below": {
- "cpu": (
- "int8",
- "int16",
- "int32",
- "int64",
- "uint8",
- "float16",
- "float32",
- "float64",
- "bool",
- )
- }
- },
- backend_version,
-)
+@with_supported_dtypes({"2.5.2 and below": ("complex",)}, backend_version)
def imag(
val: paddle.Tensor,
/,
diff --git a/ivy/functional/backends/paddle/experimental/__init__.py b/ivy/functional/backends/paddle/experimental/__init__.py
index d50ca9bf1c253..b85b671859139 100644
--- a/ivy/functional/backends/paddle/experimental/__init__.py
+++ b/ivy/functional/backends/paddle/experimental/__init__.py
@@ -14,7 +14,6 @@
from .layers import *
from .losses import *
from .linear_algebra import *
-from .losses import *
from .manipulation import *
from .norms import *
from .random import *
diff --git a/ivy/functional/backends/paddle/experimental/activations.py b/ivy/functional/backends/paddle/experimental/activations.py
index 358af07fd6246..8d13b487369da 100644
--- a/ivy/functional/backends/paddle/experimental/activations.py
+++ b/ivy/functional/backends/paddle/experimental/activations.py
@@ -5,12 +5,16 @@
# local
import ivy.functional.backends.paddle as paddle_backend
-from ivy.func_wrapper import with_unsupported_device_and_dtypes
+from ivy.func_wrapper import (
+ with_unsupported_device_and_dtypes,
+ with_supported_dtypes,
+ with_supported_device_and_dtypes,
+)
from . import backend_version
@with_unsupported_device_and_dtypes(
- {"2.5.1 and below": {"cpu": ("float16", "bfloat16")}}, backend_version
+ {"2.5.2 and below": {"cpu": ("float16", "bfloat16")}}, backend_version
)
def logit(
x: paddle.Tensor,
@@ -40,6 +44,7 @@ def logit(
).cast(x.dtype)
+@with_supported_dtypes({"2.5.2 and below": ("float32", "float64")}, backend_version)
def thresholded_relu(
x: paddle.Tensor,
/,
@@ -47,46 +52,39 @@ def thresholded_relu(
threshold: Optional[Union[int, float]] = 0,
out: Optional[paddle.Tensor] = None,
) -> paddle.Tensor:
- if x.dtype in [paddle.float32, paddle.float64]:
- return F.thresholded_relu(x, threshold=threshold)
- return paddle_backend.where(paddle_backend.greater(x, threshold), x, 0).cast(
- x.dtype
- )
+ return F.thresholded_relu(x, threshold=threshold)
-@with_unsupported_device_and_dtypes(
- {"2.5.1 and below": {"cpu": ("bfloat16",)}}, backend_version
+@with_supported_dtypes(
+ {"2.5.2 and below": ("complex", "float32", "float64")}, backend_version
)
def relu6(
x: paddle.Tensor, /, *, complex_mode="jax", out: Optional[paddle.Tensor] = None
) -> paddle.Tensor:
- if x.dtype in [paddle.float32, paddle.float64]:
- return F.relu6(x)
if paddle.is_complex(x):
return paddle.complex(F.relu6(x.real()), F.relu6(x.imag()))
- return F.relu6(x.cast("float32")).cast(x.dtype)
+ return F.relu6(x)
-@with_unsupported_device_and_dtypes(
- {"2.5.1 and below": {"cpu": ("bfloat16",)}}, backend_version
+@with_supported_dtypes(
+ {"2.5.2 and below": ("complex", "float32", "float64")}, backend_version
)
def logsigmoid(
input: paddle.Tensor, /, *, complex_mode="jax", out: Optional[paddle.Tensor] = None
) -> paddle.Tensor:
- if input.dtype in [paddle.float32, paddle.float64]:
- return F.log_sigmoid(input)
if paddle.is_complex(input):
return paddle_backend.log(
paddle_backend.divide(
1.0, (paddle_backend.add(1.0, paddle_backend.exp(-input)))
)
)
- return F.log_sigmoid(input.cast("float32")).cast(input.dtype)
+ return F.log_sigmoid(input)
+@with_supported_dtypes(
+ {"2.5.2 and below": ("complex", "float32", "float64")}, backend_version
+)
def selu(x: paddle.Tensor, /, *, out: Optional[paddle.Tensor] = None) -> paddle.Tensor:
- if x.dtype in [paddle.float32, paddle.float64]:
- return F.selu(x)
if paddle.is_complex(x):
alpha = 1.6732632423543772848170429916717
scale = 1.0507009873554804934193349852946
@@ -99,19 +97,38 @@ def selu(x: paddle.Tensor, /, *, out: Optional[paddle.Tensor] = None) -> paddle.
),
)
return ret
- return F.selu(x.cast("float32")).cast(x.dtype)
+ return F.selu(x)
+@with_supported_dtypes(
+ {"2.5.2 and below": ("complex", "float32", "float64")}, backend_version
+)
def silu(x: paddle.Tensor, /, *, out: Optional[paddle.Tensor] = None) -> paddle.Tensor:
- if x.dtype in [paddle.float32, paddle.float64]:
- return F.silu(x)
if paddle.is_complex(x):
return x * (1.0 / (1.0 + paddle_backend.exp(-x)))
- return F.silu(x.cast("float32")).cast(x.dtype)
+ return F.silu(x)
+
+
+@with_supported_dtypes(
+ {"2.5.2 and below": ("complex", "float32", "float64")}, backend_version
+)
+def elu(
+ x: paddle.Tensor, /, *, alpha: float = 1.0, out: Optional[paddle.Tensor] = None
+) -> paddle.Tensor:
+ if paddle.is_complex(x):
+ ret = (
+ paddle_backend.where(
+ paddle_backend.greater(x, 0),
+ x,
+ paddle_backend.multiply(alpha, paddle_backend.expm1(x)),
+ ),
+ )
+ return ret
+ return F.elu(x, alpha=alpha)
@with_unsupported_device_and_dtypes(
- {"2.5.1 and below": {"cpu": ("bfloat16", "float16")}}, backend_version
+ {"2.5.2 and below": {"cpu": ("bfloat16", "float16")}}, backend_version
)
def hardtanh(
x: paddle.Tensor,
@@ -134,3 +151,104 @@ def hardtanh(
)
return ret
return F.hardtanh(x.cast("float32"), min=min_val, max=max_val).cast(x.dtype)
+
+
+@with_unsupported_device_and_dtypes(
+ {"2.5.2 and below": {"cpu": ("bfloat16", "float16")}}, backend_version
+)
+def tanhshrink(
+ x: paddle.Tensor, /, *, out: Optional[paddle.Tensor] = None
+) -> paddle.Tensor:
+ if x.dtype in [paddle.float32, paddle.float64]:
+ return F.tanhshrink(x)
+ if paddle.is_complex(x):
+ return paddle.complex(F.tanhshrink(x.real()), F.tanhshrink(x.imag()))
+ return F.tanhshrink(x.cast("float32")).cast(x.dtype)
+
+
+@with_unsupported_device_and_dtypes(
+ {"2.5.2 and below": {"cpu": ("bfloat16", "float16")}}, backend_version
+)
+def threshold(
+ x: paddle.Tensor,
+ /,
+ *,
+ threshold: float,
+ value: float,
+ out: Optional[paddle.Tensor] = None,
+) -> paddle.Tensor:
+ if x.dtype in [paddle.float32, paddle.float64]:
+ return paddle_backend.where(paddle_backend.greater(x, threshold), x, value)
+ if paddle.is_complex(x):
+ return paddle_backend.where(paddle_backend.greater(x, threshold), x, value)
+ x = x.cast("float32")
+ return paddle_backend.where(paddle_backend.greater(x, threshold), x, value).cast(
+ x.dtype
+ )
+
+
+@with_unsupported_device_and_dtypes(
+ {"2.5.2 and below": {"cpu": ("bfloat16", "float16")}}, backend_version
+)
+def softshrink(
+ x: paddle.Tensor, /, *, lambd: float = 0.5, out: Optional[paddle.Tensor] = None
+) -> paddle.Tensor:
+ if x.dtype in [paddle.float32, paddle.float64]:
+ return F.softshrink(x, threshold=lambd)
+ if paddle.is_complex(x):
+ return paddle.complex(
+ F.softshrink(x.real(), threshold=lambd),
+ F.softshrink(x.img(), threshold=lambd),
+ )
+ return F.softshrink(x.cast("float32"), threshold=lambd).cast(x.dtype)
+
+
+@with_unsupported_device_and_dtypes(
+ {"2.5.2 and below": {"cpu": ("bfloat16", "float16")}}, backend_version
+)
+def celu(
+ x: paddle.Tensor,
+ /,
+ *,
+ alpha: float = 1.0,
+ complex_mode="jax",
+ out: Optional[paddle.Tensor] = None,
+) -> paddle.Tensor:
+ return F.celu(x, alpha=alpha)
+
+
+@with_supported_device_and_dtypes(
+ {
+ "2.5.2 and below": {
+ "cpu": ("float32", "float64"),
+ "gpu": ("uint16", "float16", "float32", "float64"),
+ }
+ },
+ backend_version,
+)
+def scaled_tanh(
+ x: paddle.Tensor,
+ /,
+ *,
+ alpha: float = 1.7159,
+ beta: float = 0.67,
+ out: Optional[paddle.Tensor] = None,
+) -> paddle.Tensor:
+ return paddle.stanh(x, scale_a=beta, scale_b=alpha)
+
+
+@with_unsupported_device_and_dtypes(
+ {"2.5.2 and below": {"cpu": ("float16", "bfloat16")}},
+ backend_version,
+)
+def hardshrink(
+ x: paddle.Tensor, /, *, lambd: float = 0.5, out: Optional[paddle.Tensor] = None
+) -> paddle.Tensor:
+ if x.dtype in [paddle.float32, paddle.float64]:
+ return F.hardshrink(x, threshold=lambd)
+ if paddle.is_complex(x):
+ return paddle.complex(
+ F.hardshrink(x.real(), threshold=lambd),
+ F.hardshrink(x.img(), threshold=lambd),
+ )
+ return F.hardshrink(x.cast("float32"), threshold=lambd).cast(x.dtype)
diff --git a/ivy/functional/backends/paddle/experimental/creation.py b/ivy/functional/backends/paddle/experimental/creation.py
index 1b86145baea42..4a0da45868140 100644
--- a/ivy/functional/backends/paddle/experimental/creation.py
+++ b/ivy/functional/backends/paddle/experimental/creation.py
@@ -103,7 +103,7 @@ def unsorted_segment_min(
segment_ids: paddle.Tensor,
num_segments: Union[int, paddle.Tensor],
) -> paddle.Tensor:
- ivy.utils.assertions.check_unsorted_segment_min_valid_params(
+ ivy.utils.assertions.check_unsorted_segment_valid_params(
data, segment_ids, num_segments
)
if data.dtype == paddle.float32:
@@ -116,7 +116,7 @@ def unsorted_segment_min(
init_val = 9223372036854775807
else:
raise ValueError("Unsupported data type")
- # Using paddle.full is causing interger overflow for int64
+ # Using paddle.full is causing integer overflow for int64
res = paddle.empty((num_segments,) + tuple(data.shape[1:]), dtype=data.dtype)
res[:] = init_val
for i in range(num_segments):
@@ -156,7 +156,7 @@ def unsorted_segment_sum(
# check should be same
# Might require to change the assertion function name to
# check_unsorted_segment_valid_params
- ivy.utils.assertions.check_unsorted_segment_min_valid_params(
+ ivy.utils.assertions.check_unsorted_segment_valid_params(
data, segment_ids, num_segments
)
@@ -183,7 +183,7 @@ def unsorted_segment_sum(
@with_unsupported_device_and_dtypes(
{
- "2.5.1 and below": {
+ "2.5.2 and below": {
"cpu": (
"int8",
"int16",
@@ -223,3 +223,56 @@ def mel_weight_matrix(
upper_edge_hertz,
)
return paddle.transpose(mel_mat, (1, 0))
+
+
+def unsorted_segment_mean(
+ data: paddle.Tensor,
+ segment_ids: paddle.Tensor,
+ num_segments: Union[int, paddle.Tensor],
+) -> paddle.Tensor:
+ ivy.utils.assertions.check_unsorted_segment_valid_params(
+ data, segment_ids, num_segments
+ )
+
+ # Sum computation in paddle does not support int32, so needs to
+ # be converted to float32
+ needs_conv = False
+ if data.dtype == paddle.int32:
+ data = paddle.cast(data, "float32")
+ needs_conv = True
+
+ res = paddle.zeros((num_segments,) + tuple(data.shape[1:]), dtype=data.dtype)
+
+ count = paddle.bincount(segment_ids)
+ count = paddle.where(count > 0, count, paddle.to_tensor([1], dtype="int32"))
+ res = unsorted_segment_sum(data, segment_ids, num_segments)
+ res = res / paddle.reshape(count, (-1, 1))
+
+ # condition for converting float32 back to int32
+ if needs_conv is True:
+ res = paddle.cast(res, "int32")
+
+ return res
+
+
+@with_unsupported_device_and_dtypes(
+ {
+ "2.5.2 and below": {
+ "cpu": ("float16", "int8", "int16", "uint8", "complex", "bool")
+ }
+ },
+ backend_version,
+)
+def polyval(
+ coeffs: paddle.Tensor,
+ x: paddle.Tensor,
+) -> paddle.Tensor:
+ with ivy.PreciseMode(True):
+ promoted_type = ivy.promote_types(ivy.dtype(coeffs[0]), ivy.dtype(x[0]))
+ coeffs, x = ivy.promote_types_of_inputs(coeffs, x)
+ y = paddle.zeros_like(x)
+ for coeff in coeffs:
+ y = y * x + coeff
+ y = paddle.to_tensor(y)
+ y = y.astype(promoted_type)
+ return y
diff --git a/ivy/functional/backends/paddle/experimental/elementwise.py b/ivy/functional/backends/paddle/experimental/elementwise.py
index d0a598463c5fa..eb49701742a33 100644
--- a/ivy/functional/backends/paddle/experimental/elementwise.py
+++ b/ivy/functional/backends/paddle/experimental/elementwise.py
@@ -1,12 +1,13 @@
# global
import operator
-from typing import Optional, Union, Tuple, List
+from typing import Optional, Union, Tuple, List, Sequence
from numbers import Number
import paddle
from ivy.utils.exceptions import IvyNotImplementedException
from ivy.func_wrapper import (
with_supported_dtypes,
with_unsupported_device_and_dtypes,
+ with_unsupported_dtypes,
)
import ivy.functional.backends.paddle as paddle_backend
import ivy
@@ -18,7 +19,51 @@
@with_supported_dtypes(
- {"2.5.1 and below": ("float32", "float64")},
+ {
+ "2.5.2 and below": (
+ "float32",
+ "float64",
+ "int32",
+ "int64",
+ )
+ },
+ backend_version,
+)
+def amax(
+ x: paddle.Tensor,
+ /,
+ *,
+ axis: Optional[Union[int, Sequence[int]]] = None,
+ keepdims: bool = False,
+ out: Optional[paddle.Tensor] = None,
+) -> paddle.Tensor:
+ return paddle.amax(x, axis=axis, keepdim=keepdims)
+
+
+@with_supported_dtypes(
+ {
+ "2.5.2 and below": (
+ "float32",
+ "float64",
+ "int32",
+ "int64",
+ )
+ },
+ backend_version,
+)
+def amin(
+ x: paddle.Tensor,
+ /,
+ *,
+ axis: Optional[Union[int, Sequence[int]]] = None,
+ keepdims: bool = False,
+ out: Optional[paddle.Tensor] = None,
+) -> paddle.Tensor:
+ return paddle.amin(x, axis=axis, keepdim=keepdims)
+
+
+@with_supported_dtypes(
+ {"2.5.2 and below": ("float32", "float64")},
backend_version,
)
def lgamma(
@@ -28,7 +73,7 @@ def lgamma(
@with_supported_dtypes(
- {"2.5.1 and below": ("float64", "float32", "int32", "int64")},
+ {"2.5.2 and below": ("float64", "float32", "int32", "int64")},
backend_version,
)
def fmax(
@@ -44,7 +89,7 @@ def fmax(
@with_unsupported_device_and_dtypes(
- {"2.5.1 and below": {"cpu": ("float16",)}}, backend_version
+ {"2.5.2 and below": {"cpu": ("float16",)}}, backend_version
)
def sinc(x: paddle.Tensor, /, *, out: Optional[paddle.Tensor] = None) -> paddle.Tensor:
y = ivy.pi * paddle.where(x == 0, paddle.to_tensor(1.0e-20, dtype=x.dtype), x)
@@ -108,7 +153,8 @@ def copysign(
@with_unsupported_device_and_dtypes(
- {"2.5.1 and below": {"cpu": ("uint8", "int8", "int16", "float16")}}, backend_version
+ {"2.5.2 and below": {"cpu": ("uint8", "int8", "int16", "float16")}},
+ backend_version,
)
def nansum(
x: paddle.Tensor,
@@ -126,7 +172,7 @@ def nansum(
@with_unsupported_device_and_dtypes(
- {"2.5.1 and below": {"cpu": ("float16",)}}, backend_version
+ {"2.5.2 and below": {"cpu": ("float16",)}}, backend_version
)
def isclose(
a: paddle.Tensor,
@@ -141,6 +187,9 @@ def isclose(
return paddle.isclose(a, b, rtol=rtol, atol=atol, equal_nan=equal_nan)
+@with_unsupported_dtypes(
+ {"2.5.2 and below": ("float16", "int16", "int8", "uint8")}, backend_version
+)
def diff(
x: Union[paddle.Tensor, list, tuple],
/,
@@ -152,8 +201,6 @@ def diff(
out: Optional[paddle.Tensor] = None,
) -> paddle.Tensor:
ret_dtype = x.dtype
- if x.dtype in [paddle.int8, paddle.int16, paddle.uint8, paddle.float16]:
- x = x.cast("float32")
def _tensor(val):
if val is not None and not isinstance(val, paddle.Tensor):
@@ -190,7 +237,7 @@ def hypot(
@with_unsupported_device_and_dtypes(
{
- "2.5.1 and below": {
+ "2.5.2 and below": {
"cpu": (
"int8",
"int16",
@@ -230,7 +277,7 @@ def fix(
@with_unsupported_device_and_dtypes(
- {"2.5.1 and below": {"cpu": ("float16",)}}, backend_version
+ {"2.5.2 and below": {"cpu": ("float16",)}}, backend_version
)
def nextafter(
x1: paddle.Tensor,
@@ -271,7 +318,7 @@ def nextafter(
@with_unsupported_device_and_dtypes(
{
- "2.5.1 and below": {
+ "2.5.2 and below": {
"cpu": (
"int8",
"int16",
@@ -301,7 +348,7 @@ def zeta(
if q.dtype == paddle.float32
else paddle.to_tensor(8.0, dtype="float64")
)
- assert M <= len(_BERNOULLI_COEFS)
+ assert len(_BERNOULLI_COEFS) >= M
k = paddle.unsqueeze(ivy.arange(N, dtype=q.dtype), tuple(range(q.ndim)))
S = paddle.sum((a_ + k) ** -s_, -1)
Q = ivy.divide((q + N) ** (1 - x), x - 1)
@@ -338,18 +385,18 @@ def _normalize_axis_tuple(axis: Union[int, list, tuple], ndim: int) -> Tuple[int
axis = [operator.index(axis)]
except TypeError:
pass
- axis = tuple([_normalize_axis_index(ax, ndim) for ax in axis])
+ axis = tuple(_normalize_axis_index(ax, ndim) for ax in axis)
if len(set(axis)) != len(axis):
raise ValueError("repeated axis")
return axis
def _np_ndim(x):
- return ivy.array(x).ndim
+ return paddle.to_tensor(x).ndim
@with_supported_dtypes(
- {"2.5.1 and below": ("float64", "float32")},
+ {"2.5.2 and below": ("float32", "float64")},
backend_version,
)
def gradient(
@@ -363,214 +410,215 @@ def gradient(
"""Https://github.com/numpy/numpy/blob/v1.24.3/numpy/lib/
function_base.py#L969-L1312."""
# TODO: Remove % x.shape[axis] once scatter_nd supports negative indices
- with ivy.ArrayMode(False):
- N = x.ndim # number of dimensions
- if axis is None:
- axes = tuple(range(N))
- else:
- axes = _normalize_axis_tuple(axis, N)
-
- len_axes = len(axes)
- n = (
- -1
- if spacing is None
- else (0 if type(spacing) in (int, float) else len(spacing))
- )
- if n == -1:
- # no spacing argument - use 1 in all axes
- dx = [1.0] * len_axes
- elif n == 0:
- dx = [spacing] * len_axes
- elif n == 1 and _np_ndim(spacing[0]) == 0:
- # single scalar for all axes
- dx = spacing * len_axes
- elif n == len_axes:
- # scalar or 1d array for each axis
- dx = list(spacing)
- for i, distances in enumerate(dx):
- distances = paddle.to_tensor(distances)
- if _np_ndim(distances) == 0:
- continue
- elif _np_ndim(distances) != 1:
- raise ValueError("distances must be either scalars or 1d")
- if len(distances) != x.shape[axes[i]]:
- raise ValueError(
- "when 1d, distances must match "
- "the length of the corresponding dimension {} {}".format(
- len(distances), x.shape[axes[i]]
- )
- )
- if ivy.is_int_dtype(distances.dtype):
- # Convert numpy integer types to float64 to avoid modular
- # arithmetic in np.diff(distances).
- distances = distances.astype("float64")
- diffx = ivy.diff(distances)
- # if distances are constant reduce to the scalar case
- # since it brings a consistent speedup
- # cmp = diffx == diffx[0]
- if ivy.all(ivy.equal(diffx, diffx[0])):
- diffx = diffx[0]
- # if tf.reduce_sum(tf.cast(cmp, tf.int32)) == cmp.numel():
- # print(diffx, (diffx == diffx[0]))
- # diffx = diffx[0]
- dx[i] = diffx
- else:
- raise TypeError("invalid number of arguments")
-
- if edge_order > 2:
- raise ValueError("'edge_order' greater than 2 not supported")
-
- # use central differences on interior and one-sided differences on the
- # endpoints. This preserves second order-accuracy over the full domain.
-
- outvals = []
-
- # create slice objects --- initially all are [:, :, ..., :]
- slice1 = [slice(None)] * N
- slice2 = [slice(None)] * N
- slice3 = [slice(None)] * N
- slice4 = [slice(None)] * N
+ N = x.ndim # number of dimensions
+ if axis is None:
+ axes = tuple(range(N))
+ else:
+ axes = _normalize_axis_tuple(axis, N)
- if ivy.is_int_dtype(x.dtype):
- x = x.astype("float64")
- for axis, ax_dx in zip(axes, dx):
- if x.shape[axis] < edge_order + 1:
+ len_axes = len(axes)
+ n = (
+ -1
+ if spacing is None
+ else (0 if type(spacing) in (int, float) else len(spacing))
+ )
+ if n == -1:
+ # no spacing argument - use 1 in all axes
+ dx = [1.0] * len_axes
+ elif n == 0:
+ dx = [spacing] * len_axes
+ elif n == 1 and _np_ndim(spacing[0]) == 0:
+ # single scalar for all axes
+ dx = spacing * len_axes
+ elif n == len_axes:
+ # scalar or 1d array for each axis
+ dx = list(spacing)
+ for i, distances in enumerate(dx):
+ distances = paddle.to_tensor(distances)
+ if _np_ndim(distances) == 0:
+ continue
+ elif _np_ndim(distances) != 1:
+ raise ValueError("distances must be either scalars or 1d")
+ if len(distances) != x.shape[axes[i]]:
raise ValueError(
- "Shape of array too small to calculate a numerical gradient, "
- "at least (edge_order + 1) elements are required."
+ "when 1d, distances must match the length of the corresponding"
+ f" dimension {len(distances)} {x.shape[axes[i]]}"
)
- # result allocation
- out = ivy.empty_like(x) # x.clone()
-
- # spacing for the current axis
- uniform_spacing = _np_ndim(ax_dx) == 0
- # Numerical differentiation: 2nd order interior
- slice1[axis] = slice(1, -1)
- slice2[axis] = slice(None, -2)
- slice3[axis] = slice(1, -1)
- slice4[axis] = slice(2, None)
+ if paddle.is_integer(distances):
+ # Convert numpy integer types to float64 to avoid modular
+ # arithmetic in np.diff(distances).
+ distances = distances.astype("float64")
+ diffx = paddle.diff(distances)
+ # if distances are constant reduce to the scalar case
+ # since it brings a consistent speedup
+ # cmp = diffx == diffx[0]
+ if paddle.all(paddle.equal(diffx, diffx[0])):
+ diffx = diffx[0]
+ # if tf.reduce_sum(tf.cast(cmp, tf.int32)) == cmp.numel():
+ # print(diffx, (diffx == diffx[0]))
+ # diffx = diffx[0]
+ dx[i] = diffx
+ else:
+ raise TypeError("invalid number of arguments")
+
+ if edge_order > 2:
+ raise ValueError("'edge_order' greater than 2 not supported")
+
+ # use central differences on interior and one-sided differences on the
+ # endpoints. This preserves second order-accuracy over the full domain.
+
+ outvals = []
+ dx = paddle.to_tensor(dx)
+ # create slice objects --- initially all are [:, :, ..., :]
+ slice1 = [slice(None)] * N
+ slice2 = [slice(None)] * N
+ slice3 = [slice(None)] * N
+ slice4 = [slice(None)] * N
+
+ if paddle.is_integer(x):
+ x = x.astype("float64")
+ for axis, ax_dx in zip(axes, dx):
+ if x.shape[axis] < edge_order + 1:
+ raise ValueError(
+ "Shape of array too small to calculate a numerical gradient, "
+ "at least (edge_order + 1) elements are required."
+ )
+ # result allocation
+ out = paddle.empty_like(x) # x.clone()
+
+ # spacing for the current axis
+ uniform_spacing = _np_ndim(ax_dx) == 0
+
+ # Numerical differentiation: 2nd order interior
+ slice1[axis] = slice(1, -1)
+ slice2[axis] = slice(None, -2)
+ slice3[axis] = slice(1, -1)
+ slice4[axis] = slice(2, None)
+ if uniform_spacing:
+ x_slice2 = x[tuple(slice2)]
+ x_slice4 = x[tuple(slice4)]
+ # since paddle doesn't support elementwise operations for empty tensors
+ # numpy behaviour needs to be replicated manually
+ if 0 not in x_slice2.shape + x_slice4.shape:
+ out[tuple(slice1)] = x_slice4 - x_slice2 / (2.0 * ax_dx)
+ else:
+ # fix the shape for broadcasting
+ shape = [1] * N
+ shape[axis] = -1
+
+ dx1 = ax_dx[0:-1]
+ dx2 = ax_dx[1:]
+ a = (-(dx2) / (dx1 * (dx1 + dx2))).reshape(shape)
+ b = ((dx2 - dx1) / (dx1 * dx2)).reshape(shape)
+ c = (dx1 / (dx2 * (dx1 + dx2))).reshape(shape)
+
+ x_slice2 = x[tuple(slice2)]
+ x_slice3 = x[tuple(slice3)]
+ x_slice4 = x[tuple(slice4)]
+ # 1D equivalent -- out[1:-1] = a * f[:-2] + b * f[1:-1] + c * f[2:]
+ if (
+ 0
+ not in x_slice2.shape
+ + x_slice3.shape
+ + x_slice4.shape
+ + a.shape
+ + b.shape
+ + c.shape
+ ):
+ out[tuple(slice1)] = a * x_slice2 + b * x_slice3 + c * x_slice4
+
+ # Numerical differentiation: 1st order edges
+ if edge_order == 1:
+ slice1[axis] = 0
+ slice2[axis] = 1
+ slice3[axis] = 0
+ dx_0 = ax_dx if uniform_spacing else ax_dx[0]
+
+ x_slice2 = x[tuple(slice2)]
+ x_slice3 = x[tuple(slice3)]
+ # 1D equivalent -- out[0] = (f[1] - f[0]) / (x[1] - x[0])
+ if 0 not in x_slice2.shape + x_slice3.shape:
+ out[tuple(slice1)] = (x_slice2 - x_slice3) / dx_0
+
+ slice1[axis] = -1
+ slice2[axis] = -1
+ slice3[axis] = -2
+ dx_n = ax_dx if uniform_spacing else ax_dx[-1]
+
+ x_slice2 = x[tuple(slice2)]
+ x_slice3 = x[tuple(slice3)]
+ # 1D equivalent -- out[-1] = (f[-1] - f[-2]) / (x[-1] - x[-2])
+ if 0 not in x_slice2.shape + x_slice3.shape:
+ out[tuple(slice1)] = (x_slice2 - x_slice3) / dx_n
+
+ # Numerical differentiation: 2nd order edges
+ else:
+ slice1[axis] = 0
+ slice2[axis] = 0
+ slice3[axis] = 1
+ slice4[axis] = 2
if uniform_spacing:
- x_slice2 = ivy.get_item(x, tuple(slice2))
- x_slice4 = ivy.get_item(x, tuple(slice4))
- # since paddle doesn't support elementwise operations for empty tensors
- # numpy behaviour needs to be replicated manually
- if 0 not in x_slice2.shape + x_slice4.shape:
- updates = ivy.divide(
- ivy.subtract(x_slice2, x_slice4),
- ivy.multiply(2.0, ax_dx),
- )
- ivy.scatter_nd(tuple(slice1), updates, reduction="replace", out=out)
+ a = -1.5 / ax_dx
+ b = 2.0 / ax_dx
+ c = -0.5 / ax_dx
else:
- dx1 = ax_dx[0:-1]
- dx2 = ax_dx[1:]
- a = -(dx2) / (dx1 * (dx1 + dx2))
- b = (dx2 - dx1) / (dx1 * dx2)
- c = dx1 / (dx2 * (dx1 + dx2))
- ivy.scatter_nd(
- tuple(slice1),
- (
- a * x[tuple(slice2)]
- + b * x[tuple(slice3)]
- + c * x[tuple(slice4)]
- ),
- reduction="replace",
- out=out,
- )
-
- # Numerical differentiation: 1st order edges
- if edge_order == 1:
- slice1[axis] = 0
- slice2[axis] = 1
- slice3[axis] = 0
- dx_0 = ax_dx if uniform_spacing else ax_dx[0]
- # 1D equivalent -- out[0] = (f[1] - f[0]) / (x[1] - x[0])
- x_slice2 = ivy.get_item(x, tuple(slice2))
- x_slice3 = ivy.get_item(x, tuple(slice3))
- updates = ivy.divide(ivy.subtract(x_slice2, x_slice3), dx_0)
- ivy.scatter_nd(
- tuple(slice1),
- updates,
- reduction="replace",
- out=out,
- )
-
- slice1[axis] = -1 % x.shape[axis]
- slice2[axis] = -1 % x.shape[axis]
- slice3[axis] = -2 % x.shape[axis]
- dx_n = ax_dx if uniform_spacing else ax_dx[-1]
- # 1D equivalent -- out[-1] = (f[-1] - f[-2]) / (x[-1] - x[-2])
- x_slice2 = ivy.get_item(x, tuple(slice2))
- x_slice3 = ivy.get_item(x, tuple(slice3))
- updates = ivy.divide(ivy.subtract(x_slice2, x_slice3), dx_n)
- ivy.scatter_nd(
- tuple(slice1),
- updates,
- reduction="replace",
- out=out,
- )
-
- # Numerical differentiation: 2nd order edges
+ dx1 = ax_dx[0]
+ dx2 = ax_dx[1]
+ a = -(2.0 * dx1 + dx2) / (dx1 * (dx1 + dx2))
+ b = (dx1 + dx2) / (dx1 * dx2)
+ c = -dx1 / (dx2 * (dx1 + dx2))
+ # 1D equivalent -- out[0] = a * f[0] + b * f[1] + c * f[2]
+ x_slice2 = x[tuple(slice2)]
+ x_slice3 = x[tuple(slice3)]
+ x_slice4 = x[tuple(slice4)]
+ if (
+ 0
+ not in x_slice2.shape
+ + x_slice3.shape
+ + x_slice4.shape
+ + a.shape
+ + b.shape
+ + c.shape
+ ):
+ out[tuple(slice1)] = a * x_slice2 + b * x_slice3 + c * x_slice4
+
+ slice1[axis] = -1
+ slice2[axis] = -3
+ slice3[axis] = -2
+ slice4[axis] = -1
+ if uniform_spacing:
+ a = 0.5 / ax_dx
+ b = -2.0 / ax_dx
+ c = 1.5 / ax_dx
else:
- slice1[axis] = 0
- slice2[axis] = 0
- slice3[axis] = 1
- slice4[axis] = 2
- if uniform_spacing:
- a = -1.5 / ax_dx
- b = 2.0 / ax_dx
- c = -0.5 / ax_dx
- else:
- dx1 = ax_dx[0]
- dx2 = ax_dx[1]
- a = -(2.0 * dx1 + dx2) / (dx1 * (dx1 + dx2))
- b = (dx1 + dx2) / (dx1 * dx2)
- c = -dx1 / (dx2 * (dx1 + dx2))
- # 1D equivalent -- out[0] = a * f[0] + b * f[1] + c * f[2]
- ivy.scatter_nd(
- tuple(slice1),
- (
- a * x[tuple(slice2)]
- + b * x[tuple(slice3)]
- + c * x[tuple(slice4)]
- ),
- reduction="replace",
- out=out,
- )
-
- slice1[axis] = -1 % x.shape[axis]
- slice2[axis] = -3 % x.shape[axis]
- slice3[axis] = -2 % x.shape[axis]
- slice4[axis] = -1 % x.shape[axis]
- if uniform_spacing:
- a = 0.5 / ax_dx
- b = -2.0 / ax_dx
- c = 1.5 / ax_dx
- else:
- dx1 = ax_dx[-2]
- dx2 = ax_dx[-1]
- a = (dx2) / (dx1 * (dx1 + dx2))
- b = -(dx2 + dx1) / (dx1 * dx2)
- c = (2.0 * dx2 + dx1) / (dx2 * (dx1 + dx2))
- # 1D equivalent -- out[-1] = a * f[-3] + b * f[-2] + c * f[-1]
- ivy.scatter_nd(
- tuple(slice1),
- (
- a * x[tuple(slice2)]
- + b * x[tuple(slice3)]
- + c * x[tuple(slice4)]
- ),
- reduction="replace",
- out=out,
- )
-
- outvals.append(out)
-
- # reset the slice object in this dimension to ":"
- slice1[axis] = slice(None)
- slice2[axis] = slice(None)
- slice3[axis] = slice(None)
- slice4[axis] = slice(None)
+ dx1 = ax_dx[-2]
+ dx2 = ax_dx[-1]
+ a = (dx2) / (dx1 * (dx1 + dx2))
+ b = -(dx2 + dx1) / (dx1 * dx2)
+ c = (2.0 * dx2 + dx1) / (dx2 * (dx1 + dx2))
+ # 1D equivalent -- out[-1] = a * f[-3] + b * f[-2] + c * f[-1]
+ x_slice2 = x[tuple(slice2)]
+ x_slice3 = x[tuple(slice3)]
+ x_slice4 = x[tuple(slice4)]
+ if (
+ 0
+ not in x_slice2.shape
+ + x_slice3.shape
+ + x_slice4.shape
+ + a.shape
+ + b.shape
+ + c.shape
+ ):
+ out[tuple(slice1)] = a * x_slice2 + b * x_slice3 + c * x_slice4
+
+ outvals.append(out)
+
+ # reset the slice object in this dimension to ":"
+ slice1[axis] = slice(None)
+ slice2[axis] = slice(None)
+ slice3[axis] = slice(None)
+ slice4[axis] = slice(None)
if len_axes == 1:
return outvals[0]
@@ -606,7 +654,7 @@ def count_nonzero(
@with_supported_dtypes(
{
- "2.5.1 and below": (
+ "2.5.2 and below": (
"complex64",
"complex128",
"float32",
@@ -723,7 +771,7 @@ def _is_scalar(x):
# TODO: Repalce once native function becomes available.
# Compute an approximation of the error function complement (1 - erf(x)).
@with_supported_dtypes(
- {"2.5.1 and below": ("float64", "float32")},
+ {"2.5.2 and below": ("float64", "float32")},
backend_version,
)
def erfc(x: paddle.Tensor, /, *, out: Optional[paddle.Tensor] = None) -> paddle.Tensor:
diff --git a/ivy/functional/backends/paddle/experimental/gradients.py b/ivy/functional/backends/paddle/experimental/gradients.py
index 94cb64fbb7014..f1e661276d441 100644
--- a/ivy/functional/backends/paddle/experimental/gradients.py
+++ b/ivy/functional/backends/paddle/experimental/gradients.py
@@ -1,7 +1,75 @@
# global
+from typing import Callable
+import paddle
+# local
+import ivy
+from ivy.func_wrapper import inputs_to_native_arrays
+from ivy.functional.ivy.gradients import (
+ _flatten_containers,
+ _rebuild_flattened_containers,
+)
from ivy.utils.exceptions import IvyNotImplementedException
def bind_custom_gradient_function(func, custom_grad_fn):
+ class _CustomModule(paddle.autograd.PyLayer):
+ @staticmethod
+ def forward(ctx, x):
+ ret = ivy.to_native(func(x), nested=True, include_derived=True)
+ ctx.save_for_backward(x, ret)
+ return ret
+
+ @staticmethod
+ def backward(ctx, upstream):
+ grads = custom_grad_fn(
+ *ivy.to_ivy(
+ (ctx.saved_tensor(), upstream), nested=True, include_derived=True
+ )
+ )
+ return ivy.to_native(grads, nested=True, include_derived=True)
+
+ custom_module = _CustomModule.apply
+ return inputs_to_native_arrays(custom_module)
+
+
+def vjp(func: Callable, *primals):
+ flattened_primals, ret_idxs = _flatten_containers(primals)
+
+ def grad_fn(*x_in):
+ return _flatten_containers(
+ ivy.to_native(
+ func(
+ *ivy.to_ivy(
+ _rebuild_flattened_containers(x_in, ret_idxs), nested=True
+ )
+ ),
+ nested=True,
+ include_derived=True,
+ )
+ )[0]
+
+ # primals_out = _rebuild_flattened_containers(
+ # grad_fn(*ivy.to_ivy(flattened_primals, nested=True)), ret_idxs
+ # )
+ primals_out = func(*ivy.to_ivy(primals, nested=True))
+
+ def vjpfun(x_in):
+ _, vjp_result = ivy.to_ivy(
+ paddle.incubate.autograd.vjp(
+ grad_fn,
+ ivy.to_native(flattened_primals, nested=True),
+ ivy.to_native(_flatten_containers(x_in)[0], nested=True),
+ )
+ )
+ return ivy.to_ivy(
+ _rebuild_flattened_containers(vjp_result, ret_idxs),
+ nested=True,
+ include_derived=True,
+ )
+
+ return (ivy.to_ivy(primals_out, nested=True, include_derived=True), vjpfun)
+
+
+def jvp(func: Callable, primals, tangents):
raise IvyNotImplementedException()
diff --git a/ivy/functional/backends/paddle/experimental/layers.py b/ivy/functional/backends/paddle/experimental/layers.py
index 4908c8a0601a9..999e01ef87bf3 100644
--- a/ivy/functional/backends/paddle/experimental/layers.py
+++ b/ivy/functional/backends/paddle/experimental/layers.py
@@ -30,7 +30,7 @@ def _determine_depth_max_pooling(x, kernel, strides, dims, data_format="channel_
@with_supported_device_and_dtypes(
{
- "2.5.1 and below": {
+ "2.5.2 and below": {
"cpu": ("float32", "float64"),
"gpu": ("bfloat16", "float16", "float32", "float64"),
}
@@ -51,7 +51,7 @@ def max_pool1d(
) -> paddle.Tensor:
dims = 1
kernel, strides, padding, dilation = _validate_max_pool_params(
- kernel, strides, padding, dilation, ceil_mode, dims=dims
+ kernel, strides, padding, dilation, ceil_mode, dims, data_format
)
if data_format == "NWC":
@@ -97,7 +97,7 @@ def max_pool1d(
@with_supported_device_and_dtypes(
{
- "2.5.1 and below": {
+ "2.5.2 and below": {
"cpu": ("float32", "float64"),
"gpu": ("bfloat16", "float16", "float32", "float64"),
}
@@ -118,7 +118,7 @@ def max_pool2d(
) -> paddle.Tensor:
dims = 2
kernel, strides, padding, dilation = _validate_max_pool_params(
- kernel, strides, padding, dilation, ceil_mode, dims=dims
+ kernel, strides, padding, dilation, ceil_mode, dims, data_format
)
if data_format == "NHWC":
@@ -168,7 +168,7 @@ def max_pool2d(
@with_supported_device_and_dtypes(
{
- "2.5.1 and below": {
+ "2.5.2 and below": {
"cpu": ("float32", "float64"),
"gpu": ("bfloat16", "float16", "float32", "float64"),
}
@@ -189,7 +189,7 @@ def max_pool3d(
) -> paddle.Tensor:
dims = 3
kernel, strides, padding, dilation = _validate_max_pool_params(
- kernel, strides, padding, dilation, ceil_mode, dims=dims
+ kernel, strides, padding, dilation, ceil_mode, dims, data_format
)
if data_format == "NDHWC":
@@ -243,7 +243,7 @@ def avg_pool1d(
x: paddle.Tensor,
kernel: Union[int, Tuple[int]],
strides: Union[int, Tuple[int]],
- padding: str,
+ padding: Union[str, int, List[Tuple[int, int]]],
/,
*,
data_format: str = "NWC",
@@ -258,7 +258,7 @@ def avg_pool2d(
x: paddle.Tensor,
kernel: Union[int, Tuple[int], Tuple[int, int]],
strides: Union[int, Tuple[int], Tuple[int, int]],
- padding: str,
+ padding: Union[str, int, List[Tuple[int, int]]],
/,
*,
data_format: str = "NHWC",
@@ -274,7 +274,7 @@ def avg_pool3d(
x: paddle.Tensor,
kernel: Union[int, Tuple[int], Tuple[int, int, int]],
strides: Union[int, Tuple[int], Tuple[int, int, int]],
- padding: str,
+ padding: Union[str, int, List[Tuple[int, int]]],
/,
*,
data_format: str = "NDHWC",
@@ -299,6 +299,9 @@ def dct(
raise IvyNotImplementedException()
+@with_unsupported_dtypes(
+ {"2.5.2 and below": ("bfloat16", "bool", "float16")}, backend_version
+)
def fft(
x: paddle.Tensor,
dim: int,
@@ -332,17 +335,16 @@ def fft(
f" {valid_norm_modes}"
)
- if x.dtype in [paddle.int64, paddle.float64, paddle.complex128]:
- x = x.cast(paddle.complex128)
- else:
- x = x.cast(paddle.complex64)
-
- return paddle.fft.fft(x, n, dim, norm=norm)
+ ret = paddle.fft.fft(x, n, dim, norm=norm)
+ # to make it compatible with other backends
+ if x.dtype == paddle.int64:
+ ret = ret.astype("complex128")
+ return ret
@with_supported_device_and_dtypes(
{
- "2.5.1 and below": {
+ "2.5.2 and below": {
"cpu": ("bfloat16", "float32", "float64"),
"gpu": ("bfloat16", "float16", "float32", "float64"),
}
@@ -364,7 +366,7 @@ def dropout1d(
@with_supported_device_and_dtypes(
{
- "2.5.1 and below": {
+ "2.5.2 and below": {
"cpu": ("bfloat16", "float32", "float64"),
"gpu": ("bfloat16", "float16", "float32", "float64"),
}
@@ -386,7 +388,7 @@ def dropout2d(
@with_supported_device_and_dtypes(
{
- "2.5.1 and below": {
+ "2.5.2 and below": {
"cpu": ("bfloat16", "float32", "float64"),
"gpu": ("bfloat16", "float16", "float32", "float64"),
}
@@ -419,7 +421,7 @@ def ifft(
@with_supported_device_and_dtypes(
{
- "2.5.1 and below": {
+ "2.5.2 and below": {
"cpu": ("int8", "float32", "float64"),
"gpu": ("int8", "bfloat16", "float16", "float32", "float64"),
},
@@ -462,7 +464,7 @@ def interpolate(
mode: Optional[Literal["linear", "bilinear", "trilinear"]] = "linear",
scale_factor: Optional[Union[Sequence[int], int]] = None,
recompute_scale_factor: Optional[bool] = None,
- align_corners: Optional[bool] = None,
+ align_corners: bool = False,
antialias: Optional[bool] = False,
out: Optional[paddle.Tensor] = None,
):
@@ -489,8 +491,29 @@ def ifftn(
return paddle.fft.ifftn(x, s, axes, norm)
+def rfft(
+ x: paddle.Tensor,
+ /,
+ *,
+ n: Optional[int] = None,
+ axis: int = -1,
+ norm: Literal["backward", "ortho", "forward"] = "backward",
+ out: Optional[paddle.Tensor] = None,
+) -> paddle.Tensor:
+ if x.dtype in [paddle.complex64, paddle.complex128]:
+ x = x.real()
+ if x.dtype == paddle.float16:
+ x = x.astype(paddle.float32)
+
+ ret = paddle.fft.rfft(x, n=n, axis=axis, norm=norm)
+
+ if ivy.exists(out):
+ return ivy.inplace_update(out, ret)
+ return ret
+
+
@with_unsupported_dtypes(
- {"2.5.1 and below": ("bfloat16", "float16", "complex64", "complex128", "bool")},
+ {"2.5.2 and below": ("bfloat16", "float16", "complex64", "complex128", "bool")},
backend_version,
)
def rfftn(
@@ -507,7 +530,7 @@ def rfftn(
@with_supported_dtypes(
{
- "2.5.1 and below": (
+ "2.5.2 and below": (
"complex64",
"complex128",
)
@@ -529,7 +552,7 @@ def fft2(
# stft
@with_supported_dtypes(
{
- "2.5.1 and below": (
+ "2.5.2 and below": (
"complex64",
"complex128",
)
diff --git a/ivy/functional/backends/paddle/experimental/linear_algebra.py b/ivy/functional/backends/paddle/experimental/linear_algebra.py
index abc29c7dc5b81..aa76b96d31996 100644
--- a/ivy/functional/backends/paddle/experimental/linear_algebra.py
+++ b/ivy/functional/backends/paddle/experimental/linear_algebra.py
@@ -13,7 +13,8 @@
@with_unsupported_device_and_dtypes(
- {"2.5.1 and below": {"cpu": ("int8", "int16", "uint8", "float16")}}, backend_version
+ {"2.5.2 and below": {"cpu": ("int8", "int16", "uint8", "float16", "bfloat16")}},
+ backend_version,
)
def diagflat(
x: paddle.Tensor,
@@ -46,7 +47,7 @@ def diagflat(
@with_unsupported_device_and_dtypes(
- {"2.5.1 and below": {"cpu": ("int8", "uint8", "int16")}}, backend_version
+ {"2.5.2 and below": {"cpu": ("int8", "uint8", "int16")}}, backend_version
)
def kron(
a: paddle.Tensor,
@@ -89,6 +90,27 @@ def adjoint(
return paddle.moveaxis(x, -2, -1).conj()
+@with_unsupported_device_and_dtypes(
+ {"2.5.2 and below": {"cpu": ("int8", "uint8", "int16", "float16")}},
+ backend_version,
+)
+def solve_triangular(
+ x1: paddle.Tensor,
+ x2: paddle.Tensor,
+ /,
+ *,
+ upper: bool = True,
+ adjoint: bool = False,
+ unit_diagonal: bool = False,
+ out: Optional[paddle.Tensor] = None,
+) -> paddle.Tensor:
+ # Paddle does not support complex tensors for this operation (cpu and gpu),
+ # so adjoint always equals transpose.
+ return paddle.linalg.triangular_solve(
+ x1, x2, upper=upper, transpose=adjoint, unitriangular=unit_diagonal
+ )
+
+
def cond(
x: paddle.Tensor,
/,
@@ -111,7 +133,7 @@ def lu_factor(
@with_supported_device_and_dtypes(
{
- "2.5.1 and below": {
+ "2.5.2 and below": {
"cpu": (
"float32",
"float64",
@@ -146,7 +168,7 @@ def dot(
@with_supported_device_and_dtypes(
{
- "2.5.1 and below": {
+ "2.5.2 and below": {
"cpu": (
"float32",
"float64",
diff --git a/ivy/functional/backends/paddle/experimental/losses.py b/ivy/functional/backends/paddle/experimental/losses.py
index d2ec322ea218e..a6a4b4973bf89 100644
--- a/ivy/functional/backends/paddle/experimental/losses.py
+++ b/ivy/functional/backends/paddle/experimental/losses.py
@@ -14,7 +14,7 @@
@with_unsupported_device_and_dtypes(
{
- "2.5.1 and below": {
+ "2.5.2 and below": {
"cpu": (
"float16",
"int8",
@@ -42,7 +42,7 @@ def l1_loss(
@with_unsupported_device_and_dtypes(
{
- "2.5.1 and below": {
+ "2.5.2 and below": {
"cpu": (
"int8",
"int16",
@@ -72,7 +72,7 @@ def smooth_l1_loss(
@with_unsupported_device_and_dtypes(
{
- "2.5.1 and below": {
+ "2.5.2 and below": {
"cpu": (
"float16",
"int8",
@@ -100,7 +100,7 @@ def huber_loss(
@with_unsupported_device_and_dtypes(
{
- "2.5.1 and below": {
+ "2.5.2 and below": {
"cpu": (
"float16",
"int8",
@@ -127,7 +127,7 @@ def soft_margin_loss(
@with_supported_device_and_dtypes(
- {"2.5.1 and below": {"cpu": ("float32", "float64")}},
+ {"2.5.2 and below": {"cpu": ("float32", "float64")}},
backend_version,
)
def kl_div(
@@ -195,7 +195,7 @@ def _validate_poisson_nll_params(
@with_supported_device_and_dtypes(
{
- "2.5.1 and below": {
+ "2.5.2 and below": {
"cpu": ("float32", "float64"),
"gpu": ("bfloat16", "float16", "float32", "float64"),
}
diff --git a/ivy/functional/backends/paddle/experimental/manipulation.py b/ivy/functional/backends/paddle/experimental/manipulation.py
index b20a6e8ca2d89..9fa5e60405ac0 100644
--- a/ivy/functional/backends/paddle/experimental/manipulation.py
+++ b/ivy/functional/backends/paddle/experimental/manipulation.py
@@ -14,11 +14,15 @@
from .. import backend_version
-from ivy.func_wrapper import with_unsupported_device_and_dtypes, with_supported_dtypes
+from ivy.func_wrapper import (
+ with_supported_device_and_dtypes,
+ with_unsupported_device_and_dtypes,
+ with_supported_dtypes,
+ with_unsupported_dtypes,
+)
import paddle
import ivy
import ivy.functional.backends.paddle as paddle_backend
-from ivy.func_wrapper import with_supported_device_and_dtypes
from ivy.functional.ivy.experimental.manipulation import (
_check_paddle_pad,
_to_paddle_padding,
@@ -88,6 +92,17 @@
]
+@with_unsupported_dtypes(
+ {
+ "2.5.2 and below": (
+ "int16",
+ "int8",
+ "uint8",
+ "bfloat16",
+ )
+ },
+ backend_version,
+)
def moveaxis(
a: paddle.Tensor,
source: Union[int, Sequence[int]],
@@ -101,13 +116,11 @@ def moveaxis(
source = list(source)
if isinstance(destination, tuple):
source = list(destination)
- if a.dtype in [paddle.int8, paddle.int16, paddle.uint8]:
- return paddle.moveaxis(a.cast("float32"), source, destination).cast(a.dtype)
return paddle.moveaxis(a, source, destination)
@with_supported_dtypes(
- {"2.5.1 and below": ("float16", "float32", "float64", "int32", "int64")},
+ {"2.5.2 and below": ("float16", "float32", "float64", "int32", "int64")},
backend_version,
)
def pad(
@@ -153,8 +166,11 @@ def pad(
pad.partial_mixed_handler = (
lambda *args, mode="constant", constant_values=0, reflect_type="even", **kwargs: (
- _check_paddle_pad(
- mode, reflect_type, args[1], args[0].shape, constant_values, 3
+ len(args[0].shape) <= 3
+ and (
+ _check_paddle_pad(
+ mode, reflect_type, args[1], args[0].shape, constant_values, 3
+ )
)
)
)
@@ -162,7 +178,7 @@ def pad(
@with_unsupported_device_and_dtypes(
{
- "2.5.1 and below": {
+ "2.5.2 and below": {
"cpu": (
"int8",
"int16",
@@ -186,6 +202,10 @@ def heaviside(
return paddle.heaviside(x1, x2)
+@with_unsupported_dtypes(
+ {"2.5.2 and below": ("bfloat16", "float16", "int16", "int8", "uint8")},
+ backend_version,
+)
def flipud(
m: paddle.Tensor,
/,
@@ -193,8 +213,6 @@ def flipud(
copy: Optional[bool] = None,
out: Optional[paddle.Tensor] = None,
) -> paddle.Tensor:
- if m.dtype in [paddle.int8, paddle.int16, paddle.uint8, paddle.float16]:
- return paddle.flip(m.cast("float32"), axis=0).cast(m.dtype)
return paddle.flip(m, axis=0)
@@ -210,7 +228,7 @@ def vstack(
@with_unsupported_device_and_dtypes(
- {"2.5.1 and below": {"cpu": ("int16", "bfloat16")}},
+ {"2.5.2 and below": {"cpu": ("int16", "bfloat16")}},
backend_version,
)
def hstack(
@@ -226,19 +244,8 @@ def hstack(
return ivy.concat(arrays, axis=0)
-@with_supported_device_and_dtypes(
- {
- "2.5.1 and above": {
- "cpu": (
- "bool",
- "int32",
- "int64",
- "float32",
- "float64",
- ),
- "gpu": ("float16",),
- },
- },
+@with_unsupported_dtypes(
+ {"2.5.2 and below": ("bfloat16", "float16", "int16", "int8", "uint8")},
backend_version,
)
def rot90(
@@ -250,13 +257,11 @@ def rot90(
axes: Optional[Tuple[int, int]] = (0, 1),
out: Optional[paddle.Tensor] = None,
) -> paddle.Tensor:
- if (k % 4) and m.dtype in [paddle.int8, paddle.int16, paddle.uint8, paddle.float16]:
- return paddle.rot90(m.cast("float32"), k=k, axes=axes).cast(m.dtype)
return paddle.rot90(m, k=k, axes=axes)
@with_unsupported_device_and_dtypes(
- {"2.5.1 and below": {"cpu": ("complex64", "complex128")}},
+ {"2.5.2 and below": {"cpu": ("complex64", "complex128")}},
backend_version,
)
def top_k(
@@ -282,6 +287,10 @@ def top_k(
return topk_res(val, indices)
+@with_unsupported_dtypes(
+ {"2.5.2 and below": ("bfloat16", "float16", "int16", "int8", "uint8")},
+ backend_version,
+)
def fliplr(
m: paddle.Tensor,
/,
@@ -289,8 +298,6 @@ def fliplr(
copy: Optional[bool] = None,
out: Optional[paddle.Tensor] = None,
) -> paddle.Tensor:
- if m.dtype in [paddle.int8, paddle.int16, paddle.uint8, paddle.float16]:
- return paddle.flip(m.cast("float32"), axis=1).cast(m.dtype)
return paddle.flip(m, axis=1)
@@ -449,7 +456,7 @@ def atleast_2d(
@with_unsupported_device_and_dtypes(
- {"2.5.1 and below": {"cpu": ("float16",)}},
+ {"2.5.2 and below": {"cpu": ("float16",)}},
backend_version,
)
def atleast_3d(
@@ -473,12 +480,8 @@ def atleast_3d(
return res
-@with_unsupported_device_and_dtypes(
- {"2.5.1 and below": {"cpu": ("int8",)}},
- backend_version,
-)
-@with_supported_device_and_dtypes(
- {"2.5.1 and below": {"cpu": ("int32", "int64", "float32", "float64")}},
+@with_unsupported_dtypes(
+ {"2.5.2 and below": ("bfloat16", "bool", "float16", "int16", "int8", "uint8")},
backend_version,
)
def take_along_axis(
@@ -530,22 +533,10 @@ def take_along_axis(
arr = ivy.concat([arr, fill_arr], axis=axis)
indices = ivy.where(indices < 0, arr.shape[axis] + indices, indices)
- if arr.dtype in [
- paddle.int8,
- paddle.int16,
- paddle.uint8,
- paddle.float16,
- paddle.complex64,
- paddle.complex128,
- paddle.bool,
- ]:
- if paddle.is_complex(arr):
- return paddle.complex(
- paddle.take_along_axis(arr.real(), indices, axis),
- paddle.take_along_axis(arr.imag(), indices, axis),
- )
- return paddle.take_along_axis(arr.cast("float32"), indices, axis).cast(
- arr.dtype
+ if paddle.is_complex(arr):
+ return paddle.complex(
+ paddle.take_along_axis(arr.real(), indices, axis),
+ paddle.take_along_axis(arr.imag(), indices, axis),
)
return paddle.take_along_axis(arr, indices, axis)
@@ -612,7 +603,7 @@ def concat_from_sequence(
@with_unsupported_device_and_dtypes(
- {"2.5.1 and below": {"cpu": ("int8", "int16", "uint8")}}, backend_version
+ {"2.5.2 and below": {"cpu": ("int8", "int16", "uint8")}}, backend_version
)
def unique_consecutive(
x: paddle.Tensor,
@@ -675,7 +666,8 @@ def unique_consecutive(
@with_unsupported_device_and_dtypes(
- {"2.5.1 and below": {"cpu": ("int8", "int16", "uint8", "float16")}}, backend_version
+ {"2.5.2 and below": {"cpu": ("int8", "int16", "uint8", "float16")}},
+ backend_version,
)
def fill_diagonal(
a: paddle.Tensor,
@@ -708,8 +700,176 @@ def fill_diagonal(
return a
+def _take_with_axis(
+ x: paddle.Tensor, indices: paddle.Tensor, /, *, axis: int, mode: str
+) -> paddle.Tensor:
+ # has no checks
+ # default behaviour is 'raise' like ON CPU
+ # additional check is recommended
+
+ x_shape = x.shape[axis]
+ if not ivy.exists(axis):
+ x = x.flatten()
+ x_shape = paddle.prod(paddle.to_tensor(x_shape))
+ else:
+ x_shape = x.shape[axis]
+
+ # wrap
+ if mode == "wrap":
+ indices = ((indices % x_shape) + x_shape) % x_shape
+ # clip
+ else:
+ indices = paddle.clip(indices, 0, x_shape - 1)
+
+ rank = len(x.shape)
+ axis = ((axis % rank) + rank) % rank
+ slicer = ([slice(None)] * axis) + [indices.tolist()]
+ ret = ivy.array(x)[tuple(slicer)]
+ if len(indices.shape) == 0 and ret.shape == [1]:
+ ret = ret[0]
+ return ret
+
+
+@with_supported_device_and_dtypes(
+ {
+ "2.5.2 and below": {
+ "cpu": ("int64", "float64", "int32", "uint8", "float32", "bool")
+ }
+ },
+ backend_version,
+)
+def take(
+ x: Union[int, List, paddle.Tensor],
+ indices: Union[int, List, paddle.Tensor],
+ /,
+ *,
+ axis: Optional[int] = None,
+ mode: str = "clip",
+ fill_value: Optional[Number] = None,
+ out: Optional[paddle.Tensor] = None,
+) -> paddle.Tensor:
+ if mode not in ["raise", "wrap", "clip", "fill"]:
+ raise ValueError("mode must be one of 'clip', 'raise', 'wrap', or 'fill'")
+ if not isinstance(x, paddle.Tensor):
+ x = paddle.to_tensor(x)
+ if len(x.shape) == 0:
+ x = paddle.to_tensor([x])
+ if not isinstance(indices, paddle.Tensor):
+ indices = paddle.to_tensor(indices)
+ if paddle.is_floating_point(indices):
+ indices = indices.astype(paddle.int64)
+
+ # raise
+ if mode == "raise":
+ mode = "clip"
+ if ivy.exists(axis):
+ try:
+ x_shape = x.shape[axis]
+ except Exception:
+ rank = len(x.shape)
+ raise IndexError(
+ "(OutOfRange) Attr(axis) is out of range, "
+ "It's expected to be in range of "
+ f"[-{rank}, {rank-1}]. But received Attr(axis) = {axis}."
+ "[Hint: Expected axis < input_dim.size() && axis >= "
+ "(0 - input_dim.size()) == true, "
+ "but received axis < input_dim.size() && axis >= "
+ "(0 - input_dim.size()):0 != true:1.]"
+ )
+ else:
+ x_shape = paddle.prod(paddle.to_tensor(x.shape))
+
+ bound_check = (indices < -x_shape) | (indices >= x_shape)
+ if paddle.any(bound_check):
+ if len(indices.shape) != 0:
+ indices = indices[bound_check].flatten()[0]
+ raise ValueError(
+ "(InvalidArgument) Variable value (indices) of OP(take) "
+ f"expected >= -{x_shape} and < {x_shape}, but got {indices}. "
+ "Please check input value. "
+ "[Hint: Expected index_data[i] < input_dim[axis], "
+ f"but received index_data[i]:{indices} >= input_dim[axis]:2.]"
+ )
+
+ # clip, wrap
+ if mode != "fill":
+ ret = _take_with_axis(x, indices, axis=axis, mode=mode)
+ if ivy.exists(out):
+ ivy.inplace_update(out, ret)
+ return ret
+
+ # fill
+ x_dtype = x.dtype
+ if fill_value is None:
+ # set according to jax behaviour
+ # https://tinyurl.com/66jn68uj
+ if paddle.is_floating_point(x) or paddle.is_complex(x):
+ # NaN for inexact types
+ fill_value = float("NaN")
+ else:
+ if x_dtype == paddle.bool:
+ # True for booleans
+ fill_value = True
+ elif str(x_dtype).split(".")[-1].startswith("u"):
+ # the largest positive value for unsigned types
+ fill_value = paddle.iinfo(x_dtype).max
+ else:
+ # the largest negative value for signed types
+ fill_value = paddle.iinfo(x_dtype).min
+
+ fill_value = paddle.to_tensor(fill_value, dtype=x_dtype)
+ x_shape = x.shape
+ ret = _take_with_axis(x, indices, axis=axis, mode="wrap")
+
+ if len(ret.shape) == 0:
+ # if scalar (paddle scalar), scalar fill (replace)
+ if paddle.any(indices != 0):
+ ret = fill_value
+ else:
+ if ivy.exists(axis):
+ rank = len(x.shape)
+ axis = ((axis % rank) + rank) % rank
+ x_shape = x_shape[axis]
+ else:
+ axis = 0
+ x_shape = paddle.prod(x_shape)
+
+ bound_check = paddle.to_tensor((indices < -x_shape) | (indices >= x_shape))
+
+ if paddle.any(bound_check):
+ if axis > 0:
+ bound_check = paddle.broadcast_to(
+ bound_check, (*x.shape[:axis], *bound_check.shape)
+ )
+ ret[bound_check] = fill_value
+
+ if ivy.exists(out):
+ ivy.inplace_update(out, ret)
+
+ return ret
+
+
+def trim_zeros(a: paddle.Tensor, /, *, trim: Optional[str] = "bf") -> paddle.Tensor:
+ first = 0
+ trim = trim.upper()
+ if "F" in trim:
+ for i in a:
+ if i != 0.0:
+ break
+ else:
+ first = first + 1
+ last = len(a)
+ if "B" in trim:
+ for i in a[::-1]:
+ if i != 0.0:
+ break
+ else:
+ last = last - 1
+ return a[first:last]
+
+
@with_supported_dtypes(
- {"2.5.1 and below": ("float32", "float64", "int32", "int64")}, backend_version
+ {"2.5.2 and below": ("float32", "float64", "int32", "int64")}, backend_version
)
def put_along_axis(
arr: paddle.Tensor,
diff --git a/ivy/functional/backends/paddle/experimental/norms.py b/ivy/functional/backends/paddle/experimental/norms.py
index f43436db40e9e..2ffebad9ed2eb 100644
--- a/ivy/functional/backends/paddle/experimental/norms.py
+++ b/ivy/functional/backends/paddle/experimental/norms.py
@@ -12,7 +12,7 @@
# use numpy implementation with ivy functions
@with_unsupported_device_and_dtypes(
{
- "2.5.1 and below": {
+ "2.5.2 and below": {
"cpu": (
"int8",
"int16",
@@ -42,9 +42,9 @@ def batch_norm(
out: Optional[Tuple[paddle.Tensor, paddle.Tensor, paddle.Tensor]] = None,
) -> Tuple[paddle.Tensor, paddle.Tensor, paddle.Tensor]:
if x.dtype not in [paddle.float32, paddle.float64]:
- x, mean, variance, scale, offset = (
+ x, mean, variance, scale, offset = [
t.cast("float32") for t in [x, mean, variance, scale, offset]
- )
+ ]
runningmean = mean
runningvariance = variance
data_formats = ["NC", "NCL", "NCHW", "NCDHW", "NLC", "NHWC", "NDHWC"]
@@ -57,8 +57,8 @@ def batch_norm(
)
except IndexError:
raise IndexError(
- "data_format must be one of 'NC', 'NCL', 'NCHW', 'NCDHW', "
- "'NLC', 'NHWC', 'NDHWC' but receive {}".format(data_format)
+ "data_format must be one of 'NC', 'NCL', 'NCHW', 'NCDHW', 'NLC', 'NHWC',"
+ f" 'NDHWC' but receive {data_format}"
)
with ivy.ArrayMode(False):
@@ -105,7 +105,7 @@ def batch_norm(
)
-@with_supported_dtypes({"2.5.1 and below": ("float32", "float64")}, backend_version)
+@with_supported_dtypes({"2.5.2 and below": ("float32", "float64")}, backend_version)
def l1_normalize(
x: paddle.Tensor, /, *, axis: int = None, out: paddle.Tensor = None
) -> paddle.Tensor:
@@ -155,7 +155,11 @@ def instance_norm(
paddle.Tensor,
]
] = None,
-) -> Tuple[paddle.Tensor, paddle.Tensor, paddle.Tensor,]:
+) -> Tuple[
+ paddle.Tensor,
+ paddle.Tensor,
+ paddle.Tensor,
+]:
raise IvyNotImplementedException()
diff --git a/ivy/functional/backends/paddle/experimental/random.py b/ivy/functional/backends/paddle/experimental/random.py
index 2f4fd90678077..9e1d00a6d3262 100644
--- a/ivy/functional/backends/paddle/experimental/random.py
+++ b/ivy/functional/backends/paddle/experimental/random.py
@@ -16,7 +16,7 @@
@with_unsupported_device_and_dtypes(
{
- "2.5.1 and below": {
+ "2.5.2 and below": {
"cpu": (
"int8",
"int16",
diff --git a/ivy/functional/backends/paddle/experimental/sparse_array.py b/ivy/functional/backends/paddle/experimental/sparse_array.py
index c79a7c97ffac3..9a1f7870a323d 100644
--- a/ivy/functional/backends/paddle/experimental/sparse_array.py
+++ b/ivy/functional/backends/paddle/experimental/sparse_array.py
@@ -19,7 +19,7 @@ def is_native_sparse_array(x: paddle.Tensor) -> bool:
@with_unsupported_device_and_dtypes(
- {"2.5.1 and below": {"cpu": ("int8",)}}, backend_version
+ {"2.5.2 and below": {"cpu": ("int8",)}}, backend_version
)
def native_sparse_array(
data=None,
diff --git a/ivy/functional/backends/paddle/experimental/statistical.py b/ivy/functional/backends/paddle/experimental/statistical.py
index 0e967126ac4c4..7572b28f0e8d0 100644
--- a/ivy/functional/backends/paddle/experimental/statistical.py
+++ b/ivy/functional/backends/paddle/experimental/statistical.py
@@ -1,29 +1,20 @@
# global
-from typing import Optional, Union, Tuple, Sequence
+from typing import Optional, Union, Tuple, Sequence, Any
import paddle
import ivy.functional.backends.paddle as paddle_backend
import ivy
from copy import deepcopy
# local
-from ivy.func_wrapper import with_unsupported_device_and_dtypes, with_supported_dtypes
+from ivy.func_wrapper import (
+ with_unsupported_device_and_dtypes,
+ with_supported_dtypes,
+)
from . import backend_version
-@with_unsupported_device_and_dtypes(
- {
- "2.5.1 and below": {
- "cpu": (
- "int8",
- "int16",
- "uint8",
- "float16",
- "complex64",
- "complex128",
- "bool",
- )
- }
- },
+@with_supported_dtypes(
+ {"2.5.2 and below": ("complex", "float32", "float64", "int32", "int64")},
backend_version,
)
def median(
@@ -34,21 +25,16 @@ def median(
keepdims: Optional[bool] = False,
out: Optional[paddle.Tensor] = None,
) -> paddle.Tensor:
- # keepdims is set to True because in versions up to 2.5.1
- # there was a problem when the axis was defined and it was the
- # only axis in the tensor so it needs to be handled manually
-
- ret_dtype = input.dtype
- if input.dtype not in [paddle.int32, paddle.int64, paddle.float32, paddle.float64]:
- if paddle.is_complex(input):
- ret = paddle.complex(
- paddle.median(input.real(), axis=axis, keepdim=True),
- paddle.median(input.imag(), axis=axis, keepdim=True),
- )
- else:
- ret = paddle.median(input.cast("float32"), axis=axis, keepdim=True)
+ if paddle.is_complex(input):
+ ret = paddle.complex(
+ paddle.median(input.real(), axis=axis, keepdim=True),
+ paddle.median(input.imag(), axis=axis, keepdim=True),
+ )
else:
ret = paddle.median(input, axis=axis, keepdim=True)
+ # keepdims is set to True because in versions up to 2.5.2
+ # there was a problem when the axis was defined, and it was the
+ # only axis in the tensor, so it needs to be handled manually
if not keepdims:
ret = paddle_backend.squeeze(ret, axis=axis)
# The following code is to simulate other frameworks
@@ -58,9 +44,12 @@ def median(
axis = None
if (input.ndim == 1 or axis is None) and not keepdims:
ret = ret.squeeze()
- return ret.astype(ret_dtype)
+ return ret.astype(input.dtype)
+@with_supported_dtypes(
+ {"2.5.2 and below": ("complex", "float32", "float64", "int64")}, backend_version
+)
def nanmean(
a: paddle.Tensor,
/,
@@ -71,22 +60,17 @@ def nanmean(
out: Optional[paddle.Tensor] = None,
) -> paddle.Tensor:
ret_dtype = dtype if dtype is not None else a.dtype
- a = a.cast(
- ret_dtype
- ) # this is necessary to match other FWs behaviour which cast before calculation
- if a.dtype not in [paddle.int64, paddle.float32, paddle.float64]:
- if paddle.is_complex(a):
- ret = paddle.complex(
- paddle.nanmean(a.real(), axis=axis, keepdim=keepdims),
- paddle.nanmean(a.imag(), axis=axis, keepdim=keepdims),
- )
- else:
- ret = paddle.nanmean(a.cast("float32"), axis=axis, keepdim=keepdims)
+ a = a.cast(ret_dtype)
+ if paddle.is_complex(a):
+ ret = paddle.complex(
+ paddle.nanmean(a.real(), axis=axis, keepdim=keepdims),
+ paddle.nanmean(a.imag(), axis=axis, keepdim=keepdims),
+ )
else:
ret = paddle.nanmean(a, axis=axis, keepdim=keepdims)
# The following code is to simulate other frameworks
- # output shapes behaviour since min output dim is 1 in paddle
+ # output shapes behavior since min output dim is 1 in paddle
if isinstance(axis, Sequence):
if len(axis) == a.ndim:
axis = None
@@ -110,15 +94,53 @@ def _validate_quantile(q):
if not (0.0 <= q[i] <= 1.0):
return False
else:
- if not (paddle.all(0 <= q) and paddle.all(q <= 1)):
+ if not (paddle.all(q >= 0) and paddle.all(q <= 1)):
return False
return True
-@with_supported_dtypes(
- {"2.5.1 and below": ("float64", "float32")},
+@with_unsupported_device_and_dtypes(
+ {
+ "2.5.2 and below": {
+ "cpu": (
+ "int8",
+ "int16",
+ "uint8",
+ "float16",
+ "bfloat16",
+ "complex64",
+ "complex128",
+ )
+ }
+ },
backend_version,
)
+def nanmin(
+ a: paddle.Tensor,
+ /,
+ *,
+ axis: Optional[Union[int, Tuple[int]]] = None,
+ keepdims: Optional[bool] = False,
+ initial: Optional[Union[int, float, complex]] = None,
+ where: Optional[paddle.Tensor] = None,
+ out: Optional[paddle.Tensor] = None,
+) -> paddle.Tensor:
+ nan_mask = paddle.isnan(a)
+ if where is not None:
+ nan_mask = paddle.logical_or(nan_mask, paddle.logical_not(where))
+ a_copy = a.clone()
+ a_copy = paddle.where(nan_mask, paddle.full_like(a_copy, float("inf")), a_copy)
+ if axis is None:
+ result = paddle.min(a_copy, keepdim=keepdims)
+ else:
+ result = paddle.min(a_copy, axis=axis, keepdim=keepdims)
+ if initial is not None:
+ initial = paddle.to_tensor(initial, dtype=a.dtype)
+ result = paddle.minimum(result, initial)
+ return result
+
+
+@with_supported_dtypes({"2.5.2 and below": ("float32", "float64")}, backend_version)
def nanprod(
a: paddle.Tensor,
/,
@@ -136,12 +158,8 @@ def nanprod(
a = a.cast(dtype)
if initial is None:
initial = 1
- if a.dtype not in [paddle.int32, paddle.int64, paddle.float32, paddle.float64]:
- a = paddle.nan_to_num(a.cast("float64"), nan=1.0)
- ret = paddle.prod(a, axis=axis, keepdim=keepdims) * initial
- else:
- a = paddle.nan_to_num(a, nan=1.0)
- ret = paddle.prod(a, axis=axis, keepdim=keepdims) * initial
+ a = paddle.nan_to_num(a, nan=1.0)
+ ret = paddle.prod(a, axis=axis, keepdim=keepdims) * initial
if isinstance(axis, Sequence):
if len(axis) == a.ndim:
@@ -290,7 +308,7 @@ def _compute_quantile_wrapper(
@with_unsupported_device_and_dtypes(
{
- "2.5.1 and below": {
+ "2.5.2 and below": {
"cpu": (
"int8",
"int16",
@@ -363,6 +381,9 @@ def histogram(
return paddle.histogram(a, bins=bins, min=min_range, max=max_range)
+@with_supported_dtypes(
+ {"2.5.2 and below": ("float32", "float64", "int32", "int64")}, backend_version
+)
def nanmedian(
input: paddle.Tensor,
/,
@@ -373,17 +394,14 @@ def nanmedian(
overwrite_input: Optional[bool] = False,
out: Optional[paddle.Tensor] = None,
) -> paddle.Tensor:
- if input.dtype not in [paddle.int32, paddle.int64, paddle.float32, paddle.float64]:
- if dtype is None:
- dtype = input.dtype
- input = input.cast("float32")
- paddle.nanmedian(x=input, axis=axis, keepdim=keepdims).cast(dtype)
- return paddle.nanmedian(x=input, axis=axis, keepdim=keepdims).cast(dtype)
+ if dtype is None:
+ dtype = input.dtype
+ return paddle.nanmedian(x=input, axis=axis, keepdim=keepdims)
@with_unsupported_device_and_dtypes(
{
- "2.5.1 and below": {
+ "2.5.2 and below": {
"cpu": (
"int8",
"int16",
@@ -401,7 +419,7 @@ def unravel_index(
/,
*,
out: Optional[paddle.Tensor] = None,
-) -> paddle.Tensor:
+) -> Tuple[Any, ...]:
if indices.ndim == 0:
indices = indices.unsqueeze(0)
coord = []
@@ -415,7 +433,7 @@ def unravel_index(
@with_unsupported_device_and_dtypes(
{
- "2.5.1 and below": {
+ "2.5.2 and below": {
"cpu": (
"int8",
"int16",
@@ -537,8 +555,9 @@ def cov(
)
-@with_unsupported_device_and_dtypes(
- {"2.5.1 and below": {"cpu": ("uint16", "bfloat16")}}, backend_version
+@with_supported_dtypes(
+ {"2.5.2 and below": ("complex", "bool", "float32", "float64")},
+ backend_version,
)
def cummax(
x: paddle.Tensor,
@@ -550,12 +569,8 @@ def cummax(
dtype: Optional[paddle.dtype] = None,
out: Optional[paddle.Tensor] = None,
) -> Tuple[paddle.Tensor, paddle.Tensor]:
- if x.dtype in (paddle.bool, paddle.float16):
- x = paddle.cast(x, "float64")
- elif x.dtype in (paddle.int16, paddle.int8, paddle.uint8):
- x = paddle.cast(x, "int64")
- elif x.dtype in (paddle.complex128, paddle.complex64):
- x = paddle.cast(paddle.real(x), "float64")
+ if x.dtype in (paddle.complex128, paddle.complex64):
+ x = x.real()
if not (exclusive or reverse):
return __find_cummax(x, axis=axis)
@@ -592,7 +607,7 @@ def __find_cummax(
if (
isinstance(x.tolist()[0], list)
and len(x[0].shape) >= 1
- and (isinstance(x[0], paddle.Tensor) or isinstance(x[0], ivy.Array))
+ and (isinstance(x[0], (paddle.Tensor, ivy.Array)))
):
if axis >= 1:
if not isinstance(x, list):
@@ -666,7 +681,7 @@ def __get_index(lst, indices=None, prefix=None):
@with_unsupported_device_and_dtypes(
- {"2.5.1 and below": {"cpu": ("uint8", "int8", "int16")}},
+ {"2.5.2 and below": {"cpu": ("uint8", "int8", "int16")}},
backend_version,
)
def cummin(
diff --git a/ivy/functional/backends/paddle/general.py b/ivy/functional/backends/paddle/general.py
index d2c25f8f74876..467d5ffd156f9 100644
--- a/ivy/functional/backends/paddle/general.py
+++ b/ivy/functional/backends/paddle/general.py
@@ -1,4 +1,5 @@
"""Collection of Paddle general functions, wrapped to fit Ivy syntax and signature."""
+
# global
from numbers import Number
from typing import Optional, Union, Sequence, Callable, List, Tuple
@@ -9,7 +10,7 @@
# local
import ivy
import ivy.functional.backends.paddle as paddle_backend
-from ivy.func_wrapper import with_unsupported_dtypes
+from ivy.func_wrapper import with_unsupported_device_and_dtypes
from ivy.functional.ivy.general import _broadcast_to
from ivy.utils.exceptions import _check_inplace_update_support
from . import backend_version
@@ -36,19 +37,60 @@ def current_backend_str() -> str:
def _check_query(query):
- return (
- query.ndim > 1
- if ivy.is_array(query)
- else (
- all(ivy.is_array(query) and i.ndim <= 1 for i in query)
- if isinstance(query, tuple)
- else False if isinstance(query, int) else True
+ if isinstance(query, Sequence):
+ return not any(isinstance(item, (Sequence, paddle.Tensor)) for item in query)
+ else:
+ return True
+
+
+def _squeeze_helper(query, x_ndim):
+ # as of paddle v2.5, paddle returns 1d tensors instead of scalars
+ return_scalar = (
+ (isinstance(query, Number) and x_ndim == 1)
+ or (
+ isinstance(query, tuple)
+ and all(isinstance(index, int) for index in query)
+ and len(query) == x_ndim
+ )
+ or (isinstance(query, paddle.Tensor) and query.ndim == x_ndim)
+ )
+
+ # checks if any slice has step > 1, this keeps all the dimensions
+ # in the paddle array which is not desirable
+ if not isinstance(query, Sequence):
+ query = [query]
+ slice_squeeze = list(
+ map(
+ lambda idx: isinstance(idx, slice)
+ and idx.step is not None
+ and idx.step != 1,
+ query,
)
)
+ if any(slice_squeeze):
+ squeeze_indices = tuple(
+ [
+ idx
+ for idx, val in enumerate(slice_squeeze)
+ if (val is False and query[idx] is not None)
+ ]
+ )
+ elif return_scalar:
+ squeeze_indices = ()
+ else:
+ squeeze_indices = None
+
+ return squeeze_indices
-@with_unsupported_dtypes(
- {"2.5.1 and below": ("float16", "int16", "int8")}, backend_version
+
+@with_unsupported_device_and_dtypes(
+ {
+ "2.5.2 and below": {
+ "cpu": ("int8", "int16", "float16", "complex64", "complex128")
+ }
+ },
+ backend_version,
)
def get_item(
x: paddle.Tensor,
@@ -57,7 +99,31 @@ def get_item(
*,
copy: bool = None,
) -> paddle.Tensor:
- return x.__getitem__(query)
+ if copy:
+ x = paddle.clone(x)
+
+ if (
+ isinstance(query, paddle.Tensor)
+ and query.dtype == paddle.bool
+ and query.ndim == 0
+ ) or isinstance(query, bool):
+ # special case to handle scalar boolean indices
+ if query is True:
+ return x[None]
+ else:
+ return paddle.zeros(shape=[0] + x.shape, dtype=x.dtype)
+
+ if isinstance(query, paddle.Tensor) and query.dtype == paddle.bool:
+ # # masked queries x[bool_1,bool_2,...,bool_i]
+ return paddle.gather_nd(x, paddle.nonzero(query))
+ if isinstance(query, paddle.Tensor):
+ query = query.cast("int64")
+
+ squeeze_indices = _squeeze_helper(query, x.ndim)
+ # regular queries x[idx_1,idx_2,...,idx_i]
+ # array queries idx = Tensor(idx_1,idx_2,...,idx_i), x[idx]
+ ret = x.__getitem__(query)
+ return ret.squeeze(squeeze_indices) if squeeze_indices else ret
get_item.partial_mixed_handler = (
@@ -76,10 +142,13 @@ def to_numpy(
else:
return x
elif paddle.is_tensor(x):
+ dtype = ivy.as_ivy_dtype(x.dtype)
+ if dtype == "bfloat16":
+ x = x.astype("float32")
if copy:
- return np.array(x)
+ return np.array(x).astype(dtype)
else:
- return np.asarray(x)
+ return np.asarray(x).astype(dtype)
elif isinstance(x, list):
return [ivy.to_numpy(u) for u in x]
raise ivy.utils.exceptions.IvyException("Expected a Paddle Tensor.")
@@ -441,8 +510,8 @@ def scatter_nd(
)
if reduction not in ["sum", "replace", "min", "max"]:
raise ivy.utils.exceptions.IvyException(
- "reduction is {}, but it must be one of "
- '"sum", "min", "max" or "replace"'.format(reduction)
+ f'reduction is {reduction}, but it must be one of "sum", "min", "max" or'
+ ' "replace"'
)
if reduction == "min":
updates = ivy.minimum(ivy.gather_nd(target, indices), updates).data
@@ -559,7 +628,7 @@ def _vmap(*args, **kwargs):
# Handling None in in_axes by broadcasting the axis_size
if isinstance(in_axes, (tuple, list)) and None in in_axes:
- none_axis_index = list()
+ none_axis_index = []
for index, axis in enumerate(in_axes):
if axis is None:
none_axis_index.append(index)
diff --git a/ivy/functional/backends/paddle/gradients.py b/ivy/functional/backends/paddle/gradients.py
index 700cc83d65e3a..33016d51a5346 100644
--- a/ivy/functional/backends/paddle/gradients.py
+++ b/ivy/functional/backends/paddle/gradients.py
@@ -21,8 +21,6 @@
def variable(x, /):
- if ivy.is_int_dtype(x.dtype):
- x = x.astype(ivy.default_float_dtype())
if not x.is_leaf:
ret = x.detach()
ret.stop_gradient = False
@@ -104,10 +102,10 @@ def grad_(x):
@with_unsupported_device_and_dtypes(
- {"2.5.1 and below": {"cpu": ("float16",)}}, backend_version
+ {"2.5.2 and below": {"cpu": ("float16",)}}, backend_version
)
def execute_with_gradients(
- func, xs, /, *, retain_grads=False, xs_grad_idxs=[[0]], ret_grad_idxs=[[0]]
+ func, xs, /, *, retain_grads=False, xs_grad_idxs=((0,),), ret_grad_idxs=((0,),)
):
# Conversion of required arrays to float variables and duplicate index chains
xs, xs_grad_idxs, xs1, required_duplicate_index_chains, _ = (
diff --git a/ivy/functional/backends/paddle/layers.py b/ivy/functional/backends/paddle/layers.py
index f70c374bc70de..9a27ab9272cb1 100644
--- a/ivy/functional/backends/paddle/layers.py
+++ b/ivy/functional/backends/paddle/layers.py
@@ -70,7 +70,7 @@ def _pad_before_conv(x, filters, strides, padding, dims, dilations, data_format)
else:
raise ValueError(f"Invalid padding format: {padding}")
- if not all([p >= 0 for p in padding]):
+ if not all(p >= 0 for p in padding):
raise ValueError(
"Invalid padding, all values should be larger than"
f"or equal to 0, but received: {padding}."
@@ -157,7 +157,7 @@ def conv1d(
@with_unsupported_device_and_dtypes(
- {"2.5.1 and below": {"cpu": ("float16", "bfloat16")}},
+ {"2.5.2 and below": {"cpu": ("float16", "bfloat16")}},
backend_version,
)
def conv1d_transpose(
@@ -216,7 +216,7 @@ def conv2d(
@with_unsupported_device_and_dtypes(
- {"2.5.1 and below": {"cpu": ("float16",)}},
+ {"2.5.2 and below": {"cpu": ("float16",)}},
backend_version,
)
def conv2d_transpose(
@@ -275,7 +275,7 @@ def depthwise_conv2d(
@with_unsupported_device_and_dtypes(
- {"2.5.1 and below": {"cpu": ("float16",)}},
+ {"2.5.2 and below": {"cpu": ("float16",)}},
backend_version,
)
def conv3d(
@@ -334,7 +334,7 @@ def conv3d_transpose(
@with_unsupported_device_and_dtypes(
- {"2.5.1 and below": {"cpu": ("float16",)}},
+ {"2.5.2 and below": {"cpu": ("float16",)}},
backend_version,
)
def conv_general_dilated(
diff --git a/ivy/functional/backends/paddle/linear_algebra.py b/ivy/functional/backends/paddle/linear_algebra.py
index 78a553d32c1d2..83b274820b503 100644
--- a/ivy/functional/backends/paddle/linear_algebra.py
+++ b/ivy/functional/backends/paddle/linear_algebra.py
@@ -24,7 +24,7 @@
@with_unsupported_device_and_dtypes(
{
- "2.5.1 and below": {
+ "2.5.2 and below": {
"cpu": (
"int8",
"int16",
@@ -91,7 +91,7 @@ def _cross(x1, x2, axisa, axisb, axisc, axis):
@with_unsupported_device_and_dtypes(
- {"2.5.1 and below": {"cpu": ("complex64", "complex128")}},
+ {"2.5.2 and below": {"cpu": ("complex64", "complex128")}},
backend_version,
)
def det(x: paddle.Tensor, /, *, out: Optional[paddle.Tensor] = None) -> paddle.Tensor:
@@ -183,7 +183,7 @@ def inner(
@with_unsupported_device_and_dtypes(
- {"2.5.1 and below": {"cpu": ("complex64", "complex128")}},
+ {"2.5.2 and below": {"cpu": ("complex64", "complex128")}},
backend_version,
)
def inv(
@@ -252,7 +252,7 @@ def matmul(
@with_unsupported_device_and_dtypes(
- {"2.5.1 and below": {"cpu": ("complex64", "complex128")}},
+ {"2.5.2 and below": {"cpu": ("complex64", "complex128")}},
backend_version,
)
def matrix_norm(
@@ -334,7 +334,7 @@ def eig(
@with_unsupported_device_and_dtypes(
- {"2.5.1 and below": {"cpu": ("complex64", "complex128")}},
+ {"2.5.2 and below": {"cpu": ("complex64", "complex128")}},
backend_version,
)
def matrix_power(
@@ -344,7 +344,7 @@ def matrix_power(
@with_unsupported_device_and_dtypes(
- {"2.5.1 and below": {"cpu": ("complex64", "complex128")}},
+ {"2.5.2 and below": {"cpu": ("complex64", "complex128")}},
backend_version,
)
def matrix_rank(
@@ -441,7 +441,7 @@ def tensorsolve(
@with_unsupported_device_and_dtypes(
- {"2.5.1 and below": {"cpu": ("complex64", "complex128")}},
+ {"2.5.2 and below": {"cpu": ("complex64", "complex128")}},
backend_version,
)
def qr(
@@ -457,7 +457,7 @@ def qr(
@with_unsupported_device_and_dtypes(
- {"2.5.1 and below": {"cpu": ("complex64", "complex128")}},
+ {"2.5.2 and below": {"cpu": ("complex64", "complex128")}},
backend_version,
)
def slogdet(
@@ -476,7 +476,7 @@ def slogdet(
@with_unsupported_device_and_dtypes(
- {"2.5.1 and below": {"cpu": ("complex64", "complex128")}},
+ {"2.5.2 and below": {"cpu": ("complex64", "complex128")}},
backend_version,
)
def solve(
@@ -503,7 +503,7 @@ def solve(
return ret
-@with_supported_dtypes({"2.5.1 and below": ("float32", "float64")}, backend_version)
+@with_supported_dtypes({"2.5.2 and below": ("float32", "float64")}, backend_version)
def svd(
x: paddle.Tensor, /, *, full_matrices: bool = True, compute_uv: bool = True
) -> Union[paddle.Tensor, Tuple[paddle.Tensor, ...]]:
@@ -517,17 +517,22 @@ def svd(
@with_unsupported_device_and_dtypes(
- {"2.5.1 and below": {"cpu": ("complex64", "complex128")}},
+ {"2.5.2 and below": {"cpu": ("complex64", "complex128")}},
backend_version,
)
def svdvals(
- x: paddle.Tensor, /, *, out: Optional[paddle.Tensor] = None
+ x: paddle.Tensor,
+ /,
+ *,
+ driver: Optional[str] = None,
+ out: Optional[paddle.Tensor] = None,
) -> paddle.Tensor:
+ # TODO:handling the driver argument
return paddle_backend.svd(x)[1]
@with_supported_dtypes(
- {"2.5.1 and below": ("complex", "float32", "float64")}, backend_version
+ {"2.5.2 and below": ("complex", "float32", "float64")}, backend_version
)
def tensordot(
x1: paddle.Tensor,
@@ -543,7 +548,7 @@ def tensordot(
@with_unsupported_device_and_dtypes(
{
- "2.5.1 and below": {
+ "2.5.2 and below": {
"cpu": (
"int8",
"int16",
@@ -619,7 +624,7 @@ def vector_norm(
@with_supported_dtypes(
- {"2.5.1 and below": ("float16", "float32", "float64", "int32", "int64")},
+ {"2.5.2 and below": ("float16", "float32", "float64", "int32", "int64")},
backend_version,
)
def diag(
@@ -633,7 +638,7 @@ def diag(
@with_unsupported_device_and_dtypes(
- {"2.5.1 and below": {"cpu": ("uint8", "int8", "int16", "complex64", "complex128")}},
+ {"2.5.2 and below": {"cpu": ("uint8", "int8", "int16", "complex64", "complex128")}},
backend_version,
)
def vander(
@@ -655,7 +660,7 @@ def vander(
@with_unsupported_dtypes(
- {"2.5.1 and below": ("unsigned", "int8", "int16", "float16")},
+ {"2.5.2 and below": ("unsigned", "int8", "int16", "float16")},
backend_version,
)
def vector_to_skew_symmetric_matrix(
diff --git a/ivy/functional/backends/paddle/manipulation.py b/ivy/functional/backends/paddle/manipulation.py
index 727d6b4cee475..0aa0957ed9863 100644
--- a/ivy/functional/backends/paddle/manipulation.py
+++ b/ivy/functional/backends/paddle/manipulation.py
@@ -74,7 +74,7 @@ def expand_dims(
@with_unsupported_dtypes(
- {"2.5.1 and below": ("bfloat16", "float16", "int16", "int8", "uint8")},
+ {"2.5.2 and below": ("bfloat16", "float16", "int16", "int8", "uint8")},
backend_version,
)
def flip(
@@ -91,7 +91,7 @@ def flip(
@with_unsupported_dtypes(
- {"2.5.1 and below": ("int16", "int8", "uint8", "bfloat16")}, backend_version
+ {"2.5.2 and below": ("int16", "int8", "uint8", "bfloat16")}, backend_version
)
def permute_dims(
x: paddle.Tensor,
@@ -159,7 +159,7 @@ def reshape(
@with_supported_dtypes(
- {"2.5.1 and below": ("complex", "float32", "float64", "int32", "int64")},
+ {"2.5.2 and below": ("complex", "float32", "float64", "int32", "int64")},
backend_version,
)
def roll(
@@ -174,7 +174,7 @@ def roll(
@with_unsupported_dtypes(
- {"2.5.1 and below": ("bfloat16", "float16", "int16")}, backend_version
+ {"2.5.2 and below": ("bfloat16", "float16", "int16")}, backend_version
)
def squeeze(
x: paddle.Tensor,
@@ -201,7 +201,7 @@ def squeeze(
@with_unsupported_device_and_dtypes(
- {"2.5.1 and below": {"cpu": ("int16", "uint8", "int8", "float16")}},
+ {"2.5.2 and below": {"cpu": ("int16", "uint8", "int8", "float16")}},
backend_version,
)
def stack(
@@ -220,7 +220,7 @@ def stack(
arrays = list(map(lambda x: x.cast(dtype), arrays))
first_shape = arrays[0].shape
- if not all(arr.shape == first_shape for arr in arrays):
+ if any(arr.shape != first_shape for arr in arrays):
raise Exception("Shapes of all inputs must match")
if 0 in first_shape:
return ivy.empty(
@@ -249,7 +249,7 @@ def stack(
# ------#
-@with_unsupported_dtypes({"2.5.1 and below": ("int16",)}, backend_version)
+@with_unsupported_dtypes({"2.5.2 and below": ("int16",)}, backend_version)
def split(
x: paddle.Tensor,
/,
@@ -262,9 +262,8 @@ def split(
if x.shape == ():
if num_or_size_splits is not None and num_or_size_splits != 1:
raise ivy.utils.exceptions.IvyException(
- "input array had no shape, but num_sections specified was {}".format(
- num_or_size_splits
- )
+ "input array had no shape, but num_sections specified was"
+ f" {num_or_size_splits}"
)
return [x]
if num_or_size_splits is None:
@@ -300,7 +299,7 @@ def split(
@with_supported_dtypes(
- {"2.5.1 and below": ("complex", "float32", "float64", "int32", "int64")},
+ {"2.5.2 and below": ("complex", "float32", "float64", "int32", "int64")},
backend_version,
)
def repeat(
@@ -326,7 +325,7 @@ def repeat(
repeats = repeats.item()
if axis is not None:
- axis = axis % x.ndim
+ axis %= x.ndim
if paddle.is_complex(x):
return paddle.complex(
paddle.repeat_interleave(x.real(), repeats=repeats, axis=axis),
@@ -336,7 +335,7 @@ def repeat(
@with_unsupported_dtypes(
- {"2.5.1 and below": ("bfloat16", "float16", "int16", "int8", "uint8")},
+ {"2.5.2 and below": ("bfloat16", "float16", "int16", "int8", "uint8")},
backend_version,
)
def tile(
@@ -379,7 +378,7 @@ def tile(
@with_unsupported_dtypes(
{
- "2.5.1 and below": (
+ "2.5.2 and below": (
"bfloat16",
"float16",
"int8",
@@ -463,7 +462,7 @@ def clip(
@with_unsupported_dtypes(
- {"2.5.1 and below": ("int16", "int8", "uint8", "bfloat16")}, backend_version
+ {"2.5.2 and below": ("int16", "int8", "uint8", "bfloat16")}, backend_version
)
def unstack(
x: paddle.Tensor,
@@ -476,7 +475,7 @@ def unstack(
if x.ndim == 0:
return [x]
if axis is not None:
- axis = axis % x.ndim
+ axis %= x.ndim
else:
axis = 0
if paddle.is_complex(x):
diff --git a/ivy/functional/backends/paddle/random.py b/ivy/functional/backends/paddle/random.py
index c2a846e3f4b5a..c60fd5e24efea 100644
--- a/ivy/functional/backends/paddle/random.py
+++ b/ivy/functional/backends/paddle/random.py
@@ -25,7 +25,7 @@
@with_unsupported_device_and_dtypes(
- {"2.5.1 and below": {"cpu": ("int8",)}},
+ {"2.5.2 and below": {"cpu": ("int8",)}},
backend_version,
)
def random_uniform(
@@ -56,7 +56,7 @@ def random_uniform(
@with_unsupported_dtypes(
- {"2.5.1 and below": ("float16", "int16", "int8")}, backend_version
+ {"2.5.2 and below": ("float16", "int16", "int8")}, backend_version
)
def random_normal(
*,
@@ -77,7 +77,7 @@ def random_normal(
@with_supported_device_and_dtypes(
{
- "2.5.1 and below": {
+ "2.5.2 and below": {
"cpu": (
"float32",
"float64",
@@ -108,7 +108,7 @@ def multinomial(
@with_unsupported_device_and_dtypes(
- {"2.5.1 and below": {"cpu": ("int8",)}},
+ {"2.5.2 and below": {"cpu": ("int8",)}},
backend_version,
)
def randint(
diff --git a/ivy/functional/backends/paddle/searching.py b/ivy/functional/backends/paddle/searching.py
index 64b68a8a63ba1..d5c6a6ffbb9da 100644
--- a/ivy/functional/backends/paddle/searching.py
+++ b/ivy/functional/backends/paddle/searching.py
@@ -16,7 +16,7 @@
@with_supported_dtypes(
- {"2.5.1 and below": ("float32", "float64", "int16", "int32", "int64", "uint8")},
+ {"2.5.2 and below": ("float32", "float64", "int16", "int32", "int64", "uint8")},
backend_version,
)
def argmax(
@@ -48,7 +48,7 @@ def argmax(
@with_unsupported_dtypes(
- {"2.5.1 and below": ("bfloat16", "bool", "complex", "float16", "int8")},
+ {"2.5.2 and below": ("bfloat16", "bool", "complex", "float16", "int8")},
backend_version,
)
def argmin(
@@ -80,7 +80,7 @@ def argmin(
@with_unsupported_dtypes(
- {"2.5.1 and below": ("float16", "int8", "uint8")}, backend_version
+ {"2.5.2 and below": ("float16", "int8", "uint8")}, backend_version
)
def nonzero(
x: paddle.Tensor,
@@ -161,7 +161,7 @@ def where(
@with_unsupported_dtypes(
- {"2.5.1 and below": ("float16", "int8", "uint8")}, backend_version
+ {"2.5.2 and below": ("float16", "int8", "uint8")}, backend_version
)
def argwhere(
x: paddle.Tensor, /, *, out: Optional[paddle.Tensor] = None
diff --git a/ivy/functional/backends/paddle/set.py b/ivy/functional/backends/paddle/set.py
index a11b455c77694..3825cf5914731 100644
--- a/ivy/functional/backends/paddle/set.py
+++ b/ivy/functional/backends/paddle/set.py
@@ -10,7 +10,7 @@
@with_supported_dtypes(
- {"2.5.1 and below": ("float32", "float64", "int32", "int64")}, backend_version
+ {"2.5.2 and below": ("float32", "float64", "int32", "int64")}, backend_version
)
def unique_all(
x: paddle.Tensor,
@@ -88,7 +88,7 @@ def unique_all(
@with_supported_dtypes(
- {"2.5.1 and below": ("float32", "float64", "int32", "int64")}, backend_version
+ {"2.5.2 and below": ("float32", "float64", "int32", "int64")}, backend_version
)
def unique_counts(x: paddle.Tensor, /) -> Tuple[paddle.Tensor, paddle.Tensor]:
unique, counts = paddle.unique(x, return_counts=True)
@@ -111,10 +111,23 @@ def unique_counts(x: paddle.Tensor, /) -> Tuple[paddle.Tensor, paddle.Tensor]:
@with_supported_dtypes(
- {"2.5.1 and below": ("float32", "float64", "int32", "int64")}, backend_version
+ {"2.5.2 and below": ("float32", "float64", "int32", "int64")}, backend_version
)
-def unique_inverse(x: paddle.Tensor, /) -> Tuple[paddle.Tensor, paddle.Tensor]:
- unique, inverse_val = paddle.unique(x, return_inverse=True)
+def unique_inverse(
+ x: paddle.Tensor,
+ /,
+ *,
+ axis: Optional[int] = None,
+) -> Tuple[paddle.Tensor, paddle.Tensor]:
+ if x.dtype not in [paddle.int32, paddle.int64, paddle.float32, paddle.float64]:
+ x = x.cast("float32")
+
+ if axis is not None:
+ unique, inverse_val = paddle.unique(x, return_inverse=True, axis=axis)
+
+ if axis is None:
+ axis = 0
+
nan_idx = paddle.where(paddle.isnan(x) > 0)
nan_count = paddle.count_nonzero(nan_idx).numpy()[0]
@@ -133,7 +146,7 @@ def unique_inverse(x: paddle.Tensor, /) -> Tuple[paddle.Tensor, paddle.Tensor]:
@with_supported_dtypes(
- {"2.5.1 and below": ("float32", "float64", "int32", "int64")}, backend_version
+ {"2.5.2 and below": ("float32", "float64", "int32", "int64")}, backend_version
)
def unique_values(
x: paddle.Tensor, /, *, out: Optional[paddle.Tensor] = None
diff --git a/ivy/functional/backends/paddle/sorting.py b/ivy/functional/backends/paddle/sorting.py
index 5712826cda9c8..0585c975204f9 100644
--- a/ivy/functional/backends/paddle/sorting.py
+++ b/ivy/functional/backends/paddle/sorting.py
@@ -9,7 +9,7 @@
@with_supported_dtypes(
- {"2.5.1 and below": ("float32", "float64", "int32", "int64")}, backend_version
+ {"2.5.2 and below": ("float32", "float64", "int32", "int64")}, backend_version
)
def argsort(
x: paddle.Tensor,
@@ -24,7 +24,7 @@ def argsort(
@with_supported_dtypes(
- {"2.5.1 and below": ("float32", "float64", "int32", "int64")}, backend_version
+ {"2.5.2 and below": ("float32", "float64", "int32", "int64")}, backend_version
)
def sort(
x: paddle.Tensor,
@@ -39,7 +39,7 @@ def sort(
@with_supported_dtypes(
- {"2.5.1 and below": ("float32", "float64", "int32", "int64")}, backend_version
+ {"2.5.2 and below": ("float32", "float64", "int32", "int64")}, backend_version
)
def searchsorted(
x: paddle.Tensor,
@@ -76,7 +76,7 @@ def searchsorted(
@with_unsupported_device_and_dtypes(
- {"2.5.1 and below": {"cpu": ("int8", "uint8", "int16", "float16", "complex")}},
+ {"2.5.2 and below": {"cpu": ("int8", "uint8", "int16", "float16", "complex")}},
backend_version,
)
def msort(
diff --git a/ivy/functional/backends/paddle/statistical.py b/ivy/functional/backends/paddle/statistical.py
index 791eaefee92cc..9cc49c2733942 100644
--- a/ivy/functional/backends/paddle/statistical.py
+++ b/ivy/functional/backends/paddle/statistical.py
@@ -13,7 +13,6 @@
)
import ivy.functional.backends.paddle as paddle_backend
from ivy.utils.einsum_parser import legalise_einsum_expr
-from ivy.functional.ivy.statistical import _get_promoted_type_of_operands
# local
from . import backend_version
@@ -23,7 +22,7 @@
@with_supported_dtypes(
- {"2.5.1 and below": ("complex", "float32", "float64", "int32", "int64")},
+ {"2.5.2 and below": ("complex", "float32", "float64", "int32", "int64")},
backend_version,
)
def min(
@@ -52,7 +51,7 @@ def min(
@with_supported_dtypes(
- {"2.5.1 and below": ("complex", "float32", "float64", "int32", "int64")},
+ {"2.5.2 and below": ("complex", "float32", "float64", "int32", "int64")},
backend_version,
)
def max(
@@ -90,7 +89,7 @@ def max(
@with_supported_dtypes(
- {"2.5.1 and below": ("bool", "complex", "float32", "float64")}, backend_version
+ {"2.5.2 and below": ("bool", "complex", "float32", "float64")}, backend_version
)
def mean(
x: paddle.Tensor,
@@ -120,7 +119,7 @@ def mean(
@with_supported_dtypes(
- {"2.5.1 and below": ("float32", "float64", "int32", "int64")}, backend_version
+ {"2.5.2 and below": ("float32", "float64", "int32", "int64")}, backend_version
)
def prod(
x: paddle.Tensor,
@@ -168,7 +167,10 @@ def std(
return _std(x, axis, correction, keepdims).cast(x.dtype)
-@with_unsupported_dtypes({"2.5.1 and below": ("int8", "uint8")}, backend_version)
+@with_unsupported_dtypes(
+ {"2.5.2 and below": ("int8", "int16", "uint8")},
+ backend_version,
+)
def sum(
x: paddle.Tensor,
/,
@@ -207,7 +209,7 @@ def var(
# Extra #
# ----- #
@with_supported_dtypes(
- {"2.5.1 and below": ("complex", "float32", "float64", "int32", "int64")},
+ {"2.5.2 and below": ("complex", "float32", "float64", "int32", "int64")},
backend_version,
)
def cumprod(
@@ -257,7 +259,7 @@ def cumprod(
@with_supported_dtypes(
- {"2.5.1 and below": ("float32", "float64", "int32", "int64")}, backend_version
+ {"2.5.2 and below": ("float32", "float64", "int32", "int64")}, backend_version
)
def cumsum(
x: paddle.Tensor,
@@ -306,7 +308,7 @@ def cumsum(
@with_supported_device_and_dtypes(
{
- "2.5.1 and below": {
+ "2.5.2 and below": {
"cpu": ("float32", "float64", "complex64", "complex128"),
"gpu": (
"bfloat16",
@@ -329,6 +331,15 @@ def einsum(
*operands: paddle.Tensor,
out: Optional[paddle.Tensor] = None,
) -> paddle.Tensor:
- dtype = _get_promoted_type_of_operands(operands)
equation = legalise_einsum_expr(*[equation, *operands])
- return paddle.einsum(equation, *operands).astype(dtype)
+
+ dtype_list = set(map(lambda x: x.dtype, operands))
+ dtype = dtype_list.pop()
+ if len(dtype_list) > 0:
+ for d in dtype_list:
+ dtype = ivy.promote_types(dtype, d)
+ operands = list(
+ map(lambda x: x.cast(dtype) if x.dtype != dtype else x, operands)
+ )
+
+ return paddle.einsum(equation, *operands)
diff --git a/ivy/functional/backends/tensorflow/__init__.py b/ivy/functional/backends/tensorflow/__init__.py
index 07f69d6e75068..4d2a7e97c0398 100644
--- a/ivy/functional/backends/tensorflow/__init__.py
+++ b/ivy/functional/backends/tensorflow/__init__.py
@@ -128,7 +128,7 @@ def rep_method(*args, **kwargs):
# update these to add new dtypes
valid_dtypes = {
- "2.13.0 and below": (
+ "2.14.0 and below": (
ivy.int8,
ivy.int16,
ivy.int32,
@@ -147,7 +147,7 @@ def rep_method(*args, **kwargs):
)
}
valid_numeric_dtypes = {
- "2.13.0 and below": (
+ "2.14.0 and below": (
ivy.int8,
ivy.int16,
ivy.int32,
@@ -165,7 +165,7 @@ def rep_method(*args, **kwargs):
)
}
valid_int_dtypes = {
- "2.13.0 and below": (
+ "2.14.0 and below": (
ivy.int8,
ivy.int16,
ivy.int32,
@@ -177,12 +177,12 @@ def rep_method(*args, **kwargs):
)
}
valid_float_dtypes = {
- "2.13.0 and below": (ivy.bfloat16, ivy.float16, ivy.float32, ivy.float64)
+ "2.14.0 and below": (ivy.bfloat16, ivy.float16, ivy.float32, ivy.float64)
}
valid_uint_dtypes = {
- "2.13.0 and below": (ivy.uint8, ivy.uint16, ivy.uint32, ivy.uint64)
+ "2.14.0 and below": (ivy.uint8, ivy.uint16, ivy.uint32, ivy.uint64)
}
-valid_complex_dtypes = {"2.13.0 and below": (ivy.complex64, ivy.complex128)}
+valid_complex_dtypes = {"2.14.0 and below": (ivy.complex64, ivy.complex128)}
# leave these untouched
valid_dtypes = _dtype_from_version(valid_dtypes, backend_version)
@@ -194,12 +194,12 @@ def rep_method(*args, **kwargs):
# invalid data types
# update these to add new dtypes
-invalid_dtypes = {"2.13.0 and below": ()}
-invalid_numeric_dtypes = {"2.13.0 and below": ()}
-invalid_int_dtypes = {"2.13.0 and below": ()}
-invalid_float_dtypes = {"2.13.0 and below": ()}
-invalid_uint_dtypes = {"2.13.0 and below": ()}
-invalid_complex_dtypes = {"2.13.0 and below": ()}
+invalid_dtypes = {"2.14.0 and below": ()}
+invalid_numeric_dtypes = {"2.14.0 and below": ()}
+invalid_int_dtypes = {"2.14.0 and below": ()}
+invalid_float_dtypes = {"2.14.0 and below": ()}
+invalid_uint_dtypes = {"2.14.0 and below": ()}
+invalid_complex_dtypes = {"2.14.0 and below": ()}
# leave these untouched
invalid_dtypes = _dtype_from_version(invalid_dtypes, backend_version)
diff --git a/ivy/functional/backends/tensorflow/activations.py b/ivy/functional/backends/tensorflow/activations.py
index ffd4efbe705b1..0b1a5e85a99cb 100644
--- a/ivy/functional/backends/tensorflow/activations.py
+++ b/ivy/functional/backends/tensorflow/activations.py
@@ -12,7 +12,6 @@
from tensorflow.python.types.core import Tensor
# local
-import ivy
from ivy.func_wrapper import with_unsupported_dtypes, with_supported_dtypes
from . import backend_version
import ivy.functional.backends.tensorflow as tf_backend
@@ -49,9 +48,7 @@ def relu(x: Tensor, /, *, complex_mode="jax", out: Optional[Tensor] = None) -> T
def sigmoid(
x: Tensor, /, *, complex_mode="jax", out: Optional[Tensor] = None
) -> Tensor:
- if not ivy.is_array(x):
- x = float(x)
- return tf.nn.sigmoid(x)
+ return 1 / (1 + tf.exp(-x))
def softmax(
@@ -71,7 +68,7 @@ def softmax(
@with_supported_dtypes(
{
- "2.13.0 and below": (
+ "2.14.0 and below": (
"float16",
"bfloat16",
"float32",
@@ -105,7 +102,7 @@ def softplus(
# Softsign
@with_supported_dtypes(
{
- "2.13.0 and below": (
+ "2.14.0 and below": (
"float16",
"bfloat16",
"float32",
@@ -151,7 +148,7 @@ def mish(
return tf.multiply(x, tf.math.tanh(x_norm))
-@with_unsupported_dtypes({"2.13.0 and below": ("complex",)}, backend_version)
+@with_unsupported_dtypes({"2.14.0 and below": ("complex",)}, backend_version)
def hardswish(
x: Tensor,
/,
diff --git a/ivy/functional/backends/tensorflow/control_flow_ops.py b/ivy/functional/backends/tensorflow/control_flow_ops.py
index ba6cacc5820da..e56beb4e35731 100644
--- a/ivy/functional/backends/tensorflow/control_flow_ops.py
+++ b/ivy/functional/backends/tensorflow/control_flow_ops.py
@@ -65,4 +65,4 @@ def _tuple_to_dict(t):
def _dict_to_tuple(d):
- return tuple([d[k] for k in d])
+ return tuple(d[k] for k in d)
diff --git a/ivy/functional/backends/tensorflow/creation.py b/ivy/functional/backends/tensorflow/creation.py
index 263e7bd943729..7bd7f0b2d6b14 100644
--- a/ivy/functional/backends/tensorflow/creation.py
+++ b/ivy/functional/backends/tensorflow/creation.py
@@ -26,7 +26,7 @@
@with_unsupported_dtypes(
{
- "2.13.0 and below": (
+ "2.14.0 and below": (
"float16",
"bfloat16",
"complex",
@@ -90,14 +90,12 @@ def asarray(
out: Optional[Union[tf.Tensor, tf.Variable]] = None,
) -> Union[tf.Tensor, tf.Variable]:
# convert the input to a tensor using the appropriate function
- try:
- ret = tf.convert_to_tensor(obj, dtype)
- except (TypeError, ValueError):
- obj = (
- obj if isinstance(obj, tf.Tensor) else tf.convert_to_tensor(obj, tf.float64)
- )
- ret = tf.cast(obj, dtype)
- return tf.identity(ret) if copy else ret
+ with tf.device(device):
+ if tf.is_tensor(obj):
+ ret = tf.cast(obj, dtype) if obj.dtype != dtype else obj
+ else:
+ ret = tf.convert_to_tensor(obj, dtype)
+ return tf.identity(ret) if (copy or ret.device != device) else ret
def empty(
@@ -121,7 +119,7 @@ def empty_like(
return tf.experimental.numpy.empty_like(x, dtype=dtype)
-@with_unsupported_dtypes({"2.13.0 and below": ("uint16",)}, backend_version)
+@with_unsupported_dtypes({"2.14.0 and below": ("uint16",)}, backend_version)
def eye(
n_rows: int,
n_cols: Optional[int] = None,
@@ -192,7 +190,11 @@ def from_dlpack(
) -> Union[tf.Tensor, tf.Variable]:
if isinstance(x, tf.Variable):
x = x.read_value()
- return tf.experimental.dlpack.from_dlpack(x)
+ if hasattr(x, "__dlpack__"):
+ capsule = x.__dlpack__()
+ else:
+ capsule = x
+ return tf.experimental.dlpack.from_dlpack(capsule)
def full(
@@ -251,7 +253,7 @@ def linspace(
return tf.cast(ans, dtype)
-@with_unsupported_dtypes({"2.13.0 and below": ("bool",)}, backend_version)
+@with_unsupported_dtypes({"2.14.0 and below": ("bool",)}, backend_version)
def meshgrid(
*arrays: Union[tf.Tensor, tf.Variable],
sparse: bool = False,
@@ -295,7 +297,7 @@ def ones_like(
return tf.ones_like(x, dtype=dtype)
-@with_unsupported_dtypes({"2.13.0 and below": ("bool",)}, backend_version)
+@with_unsupported_dtypes({"2.14.0 and below": ("bool",)}, backend_version)
def tril(
x: Union[tf.Tensor, tf.Variable],
/,
@@ -308,7 +310,7 @@ def tril(
return tf.experimental.numpy.tril(x, k)
-@with_unsupported_dtypes({"2.13.0 and below": ("bool",)}, backend_version)
+@with_unsupported_dtypes({"2.14.0 and below": ("bool",)}, backend_version)
def triu(
x: Union[tf.Tensor, tf.Variable],
/,
@@ -375,7 +377,7 @@ def one_hot(
)
-@with_unsupported_dtypes({"2.13.0 and below": ("uint32", "uint64")}, backend_version)
+@with_unsupported_dtypes({"2.14.0 and below": ("uint32", "uint64")}, backend_version)
def frombuffer(
buffer: bytes,
dtype: Optional[tf.DType] = float,
diff --git a/ivy/functional/backends/tensorflow/data_type.py b/ivy/functional/backends/tensorflow/data_type.py
index 819a8fc381d75..b5fb0f80784fe 100644
--- a/ivy/functional/backends/tensorflow/data_type.py
+++ b/ivy/functional/backends/tensorflow/data_type.py
@@ -86,8 +86,9 @@ def __init__(self):
self.tiny = 1.17549e-38
def __repr__(self):
- return "finfo(resolution={}, min={}, max={}, dtype={})".format(
- self.resolution, self.min, self.max, "bfloat16"
+ return (
+ f"finfo(resolution={self.resolution}, min={self.min}, max={self.max},"
+ " dtype='bfloat16')"
)
@@ -163,7 +164,7 @@ def iinfo(type: Union[DType, str, tf.Tensor, tf.Variable, np.ndarray], /) -> np.
return tf.experimental.numpy.iinfo(ivy.as_ivy_dtype(type))
-@with_unsupported_dtypes({"2.13.0 and below": ("bfloat16",)}, backend_version)
+@with_unsupported_dtypes({"2.14.0 and below": ("bfloat16",)}, backend_version)
def result_type(
*arrays_and_dtypes: Union[tf.Tensor, tf.Variable, tf.DType],
) -> ivy.Dtype:
@@ -238,7 +239,7 @@ def as_native_dtype(
dtype_in = dtype_in.name
if not isinstance(dtype_in, str):
return dtype_in
- if dtype_in in native_dtype_dict.keys():
+ if dtype_in in native_dtype_dict:
return native_dtype_dict[ivy.Dtype(dtype_in)]
else:
raise ivy.utils.exceptions.IvyException(
diff --git a/ivy/functional/backends/tensorflow/device.py b/ivy/functional/backends/tensorflow/device.py
index d5ec902b1db09..09416a79ffc02 100644
--- a/ivy/functional/backends/tensorflow/device.py
+++ b/ivy/functional/backends/tensorflow/device.py
@@ -48,7 +48,7 @@ def to_device(
device = as_native_dev(device)
current_dev = dev(x)
if not _same_device(current_dev, device):
- with tf.device("/" + device.upper()):
+ with tf.device(f"/{device.upper()}"):
return tf.identity(x)
return x
@@ -69,7 +69,7 @@ def as_ivy_dev(device: str, /):
def as_native_dev(device: str, /):
if isinstance(device, str) and "/" in device:
return device
- ret = "/" + ivy.Device(device).upper()
+ ret = f"/{ivy.Device(device).upper()}"
if not ret[-1].isnumeric():
ret += ":0"
return ret
diff --git a/ivy/functional/backends/tensorflow/elementwise.py b/ivy/functional/backends/tensorflow/elementwise.py
index 5ca04f39e04b1..5fd6391aa85b0 100644
--- a/ivy/functional/backends/tensorflow/elementwise.py
+++ b/ivy/functional/backends/tensorflow/elementwise.py
@@ -1,7 +1,7 @@
# global
from typing import Union, Optional
import tensorflow as tf
-import tensorflow_probability as tfp
+
# local
import ivy
@@ -83,7 +83,7 @@ def atan(
return tf.math.atan(x)
-@with_unsupported_dtypes({"2.13.0 and below": ("complex",)}, backend_version)
+@with_unsupported_dtypes({"2.14.0 and below": ("complex",)}, backend_version)
def atan2(
x1: Union[tf.Tensor, tf.Variable],
x2: Union[tf.Tensor, tf.Variable],
@@ -104,7 +104,7 @@ def atanh(
return tf.math.atanh(x)
-@with_unsupported_dtypes({"2.13.0 and below": ("complex",)}, backend_version)
+@with_unsupported_dtypes({"2.14.0 and below": ("complex",)}, backend_version)
def bitwise_and(
x1: Union[int, tf.Tensor, tf.Variable],
x2: Union[int, tf.Tensor, tf.Variable],
@@ -119,7 +119,7 @@ def bitwise_and(
return tf.bitwise.bitwise_and(x1, x2)
-@with_unsupported_dtypes({"2.13.0 and below": ("complex",)}, backend_version)
+@with_unsupported_dtypes({"2.14.0 and below": ("complex",)}, backend_version)
def bitwise_invert(
x: Union[int, tf.Tensor, tf.Variable],
/,
@@ -132,7 +132,7 @@ def bitwise_invert(
return tf.bitwise.invert(x)
-@with_unsupported_dtypes({"2.13.0 and below": ("complex",)}, backend_version)
+@with_unsupported_dtypes({"2.14.0 and below": ("complex",)}, backend_version)
def bitwise_left_shift(
x1: Union[int, tf.Tensor, tf.Variable],
x2: Union[int, tf.Tensor, tf.Variable],
@@ -144,7 +144,7 @@ def bitwise_left_shift(
return tf.bitwise.left_shift(x1, x2)
-@with_unsupported_dtypes({"2.13.0 and below": ("complex",)}, backend_version)
+@with_unsupported_dtypes({"2.14.0 and below": ("complex",)}, backend_version)
def bitwise_or(
x1: Union[int, tf.Tensor, tf.Variable],
x2: Union[int, tf.Tensor, tf.Variable],
@@ -159,7 +159,7 @@ def bitwise_or(
return tf.bitwise.bitwise_or(x1, x2)
-@with_unsupported_dtypes({"2.13.0 and below": ("complex",)}, backend_version)
+@with_unsupported_dtypes({"2.14.0 and below": ("complex",)}, backend_version)
def bitwise_right_shift(
x1: Union[int, tf.Tensor, tf.Variable],
x2: Union[int, tf.Tensor, tf.Variable],
@@ -171,7 +171,7 @@ def bitwise_right_shift(
return tf.bitwise.right_shift(x1, x2)
-@with_unsupported_dtypes({"2.13.0 and below": ("complex",)}, backend_version)
+@with_unsupported_dtypes({"2.14.0 and below": ("complex",)}, backend_version)
def bitwise_xor(
x1: Union[int, tf.Tensor, tf.Variable],
x2: Union[int, tf.Tensor, tf.Variable],
@@ -186,7 +186,7 @@ def bitwise_xor(
return tf.bitwise.bitwise_xor(x1, x2)
-@with_unsupported_dtypes({"2.13.0 and below": ("complex",)}, backend_version)
+@with_unsupported_dtypes({"2.14.0 and below": ("complex",)}, backend_version)
def ceil(
x: Union[tf.Tensor, tf.Variable],
/,
@@ -208,7 +208,7 @@ def cos(
return tf.cos(x)
-@with_unsupported_dtypes({"2.13.0 and below": ("float16",)}, backend_version)
+@with_unsupported_dtypes({"2.14.0 and below": ("float16",)}, backend_version)
def cosh(
x: Union[tf.Tensor, tf.Variable],
/,
@@ -263,7 +263,7 @@ def exp2(
return tf.math.pow(2, x, name=None)
-@with_supported_dtypes({"2.13.0 and below": ("float", "complex")}, backend_version)
+@with_supported_dtypes({"2.14.0 and below": ("float", "complex")}, backend_version)
def expm1(
x: Union[tf.Tensor, tf.Variable],
/,
@@ -273,7 +273,7 @@ def expm1(
return tf.math.expm1(x)
-@with_unsupported_dtypes({"2.13.0 and below": ("complex",)}, backend_version)
+@with_unsupported_dtypes({"2.14.0 and below": ("complex",)}, backend_version)
def floor(
x: Union[tf.Tensor, tf.Variable],
/,
@@ -286,7 +286,7 @@ def floor(
return tf.math.floor(x)
-@with_unsupported_dtypes({"2.13.0 and below": ("complex",)}, backend_version)
+@with_unsupported_dtypes({"2.14.0 and below": ("complex",)}, backend_version)
def floor_divide(
x1: Union[float, tf.Tensor, tf.Variable],
x2: Union[float, tf.Tensor, tf.Variable],
@@ -298,7 +298,7 @@ def floor_divide(
return tf.experimental.numpy.floor_divide(x1, x2)
-@with_supported_dtypes({"2.13.0 and below": ("float",)}, backend_version)
+@with_supported_dtypes({"2.14.0 and below": ("float",)}, backend_version)
def fmin(
x1: Union[tf.Tensor, tf.Variable],
x2: Union[tf.Tensor, tf.Variable],
@@ -313,7 +313,7 @@ def fmin(
return ret
-@with_unsupported_dtypes({"2.13.0 and below": ("complex",)}, backend_version)
+@with_unsupported_dtypes({"2.14.0 and below": ("complex",)}, backend_version)
def greater(
x1: Union[float, tf.Tensor, tf.Variable],
x2: Union[float, tf.Tensor, tf.Variable],
@@ -322,10 +322,10 @@ def greater(
out: Optional[Union[tf.Tensor, tf.Variable]] = None,
) -> Union[tf.Tensor, tf.Variable]:
x1, x2 = ivy.promote_types_of_inputs(x1, x2)
- return tf.math.greater(x1, x2)
+ return tf.experimental.numpy.greater(x1, x2)
-@with_unsupported_dtypes({"2.13.0 and below": ("complex",)}, backend_version)
+@with_unsupported_dtypes({"2.14.0 and below": ("complex",)}, backend_version)
def greater_equal(
x1: Union[float, tf.Tensor, tf.Variable],
x2: Union[float, tf.Tensor, tf.Variable],
@@ -374,7 +374,7 @@ def isinf(
return tf.zeros_like(x, tf.bool)
-@with_unsupported_dtypes({"2.13.0 and below": ("complex", "bool")}, backend_version)
+@with_unsupported_dtypes({"2.14.0 and below": ("complex", "bool")}, backend_version)
def isnan(
x: Union[tf.Tensor, tf.Variable],
/,
@@ -387,7 +387,7 @@ def isnan(
return tf.math.is_nan(x)
-@with_unsupported_dtypes({"2.13.0 and below": ("unsigned",)}, backend_version)
+@with_unsupported_dtypes({"2.14.0 and below": ("unsigned",)}, backend_version)
def lcm(
x1: Union[tf.Tensor, tf.Variable],
x2: Union[tf.Tensor, tf.Variable],
@@ -401,7 +401,7 @@ def lcm(
@with_unsupported_dtypes(
{
- "2.13.0 and below": (
+ "2.14.0 and below": (
"bool",
"complex",
)
@@ -419,7 +419,7 @@ def less(
return tf.math.less(x1, x2)
-@with_unsupported_dtypes({"2.13.0 and below": ("complex",)}, backend_version)
+@with_unsupported_dtypes({"2.14.0 and below": ("complex",)}, backend_version)
def less_equal(
x1: Union[float, tf.Tensor, tf.Variable],
x2: Union[float, tf.Tensor, tf.Variable],
@@ -467,7 +467,7 @@ def log2(
return tf.math.log(x) / tf.math.log(tf.constant(2.0, x.dtype))
-@with_unsupported_dtypes({"2.13.0 and below": ("float16", "bfloat16")}, backend_version)
+@with_unsupported_dtypes({"2.14.0 and below": ("float16", "bfloat16")}, backend_version)
def logaddexp(
x1: Union[tf.Tensor, tf.Variable],
x2: Union[tf.Tensor, tf.Variable],
@@ -479,7 +479,7 @@ def logaddexp(
return tf.experimental.numpy.logaddexp(x1, x2)
-@with_unsupported_dtypes({"2.13.0 and below": ("float16",)}, backend_version)
+@with_unsupported_dtypes({"2.14.0 and below": ("float16",)}, backend_version)
def real(
x: Union[tf.Tensor, tf.Variable],
/,
@@ -491,7 +491,7 @@ def real(
@with_unsupported_dtypes(
{
- "2.13.0 and below": (
+ "2.14.0 and below": (
"uint8",
"uint16",
"uint32",
@@ -563,7 +563,7 @@ def logical_xor(
return tf.math.logical_xor(tf.cast(x1, tf.bool), tf.cast(x2, tf.bool))
-@with_unsupported_dtypes({"2.13.0 and below": ("bool",)}, backend_version)
+@with_unsupported_dtypes({"2.14.0 and below": ("bool",)}, backend_version)
def multiply(
x1: Union[float, tf.Tensor, tf.Variable],
x2: Union[float, tf.Tensor, tf.Variable],
@@ -575,7 +575,7 @@ def multiply(
return tf.math.multiply(x1, x2)
-@with_unsupported_dtypes({"2.13.0 and below": ("bool", "unsigned")}, backend_version)
+@with_unsupported_dtypes({"2.14.0 and below": ("bool", "unsigned")}, backend_version)
def negative(
x: Union[float, tf.Tensor, tf.Variable],
/,
@@ -605,7 +605,7 @@ def positive(
return tf.experimental.numpy.positive(x)
-@with_unsupported_dtypes({"2.13.0 and below": ("bool", "unsigned")}, backend_version)
+@with_unsupported_dtypes({"2.14.0 and below": ("bool", "unsigned")}, backend_version)
def pow(
x1: Union[tf.Tensor, tf.Variable],
x2: Union[int, float, tf.Tensor, tf.Variable],
@@ -630,7 +630,7 @@ def pow(
return tf.experimental.numpy.power(x1, x2)
-@with_unsupported_dtypes({"2.13.0 and below": ("bfloat16", "complex")}, backend_version)
+@with_unsupported_dtypes({"2.14.0 and below": ("bfloat16", "complex")}, backend_version)
def remainder(
x1: Union[float, tf.Tensor, tf.Variable],
x2: Union[float, tf.Tensor, tf.Variable],
@@ -649,7 +649,7 @@ def remainder(
return tf.experimental.numpy.remainder(x1, x2)
-@with_unsupported_dtypes({"2.13.0 and below": ("bfloat16", "complex")}, backend_version)
+@with_unsupported_dtypes({"2.14.0 and below": ("bfloat16", "complex")}, backend_version)
def round(
x: Union[tf.Tensor, tf.Variable],
/,
@@ -670,7 +670,7 @@ def round(
return tf.cast(tf.round(x * factor) / factor_deno, ret_dtype)
-@with_unsupported_dtypes({"2.13.0 and below": ("bool", "unsigned")}, backend_version)
+@with_unsupported_dtypes({"2.14.0 and below": ("bool", "unsigned")}, backend_version)
def sign(
x: Union[tf.Tensor, tf.Variable],
/,
@@ -749,7 +749,7 @@ def tanh(
complex_mode="jax",
out: Optional[Union[tf.Tensor, tf.Variable]] = None,
) -> Union[tf.Tensor, tf.Variable]:
- return tf.tanh(x)
+ return tf.math.tanh(x)
def trapz(
@@ -761,10 +761,11 @@ def trapz(
axis: int = -1,
out: Optional[Union[tf.Tensor, tf.Variable]] = None,
) -> Union[tf.Tensor, tf.Variable]:
- return tfp.math.trapz(y, x=x, dx=dx, axis=axis, name=None)
+ pass
+ # TODO: Implement purely in tensorflow
-@with_unsupported_dtypes({"2.13.0 and below": ("complex",)}, backend_version)
+@with_unsupported_dtypes({"2.14.0 and below": ("complex",)}, backend_version)
def trunc(
x: Union[tf.Tensor, tf.Variable],
/,
@@ -774,8 +775,8 @@ def trunc(
ret = x
if not ivy.is_array(x):
raise ivy.utils.exceptions.IvyException("Input must be array")
- elif not ("int" in str(x.dtype)):
- if not ret.get_shape().ndims == 0:
+ elif "int" not in str(x.dtype):
+ if ret.get_shape().ndims != 0:
ret = tf.tensor_scatter_nd_update(
x, tf.where(tf.greater_equal(x, 0)), tf.math.floor(x[x >= 0])
)
@@ -791,7 +792,7 @@ def trunc(
# ------#
-@with_unsupported_dtypes({"2.13.0 and below": ("complex",)}, backend_version)
+@with_unsupported_dtypes({"2.14.0 and below": ("complex",)}, backend_version)
def erf(
x: Union[tf.Tensor, tf.Variable],
/,
@@ -801,7 +802,7 @@ def erf(
return tf.math.erf(x)
-@with_unsupported_dtypes({"2.13.0 and below": ("complex", "bool")}, backend_version)
+@with_unsupported_dtypes({"2.14.0 and below": ("complex", "bool")}, backend_version)
def maximum(
x1: Union[tf.Tensor, tf.Variable],
x2: Union[tf.Tensor, tf.Variable],
@@ -814,7 +815,7 @@ def maximum(
return tf.math.maximum(x1, x2)
-@with_unsupported_dtypes({"2.13.0 and below": ("complex", "bool")}, backend_version)
+@with_unsupported_dtypes({"2.14.0 and below": ("complex", "bool")}, backend_version)
def minimum(
x1: Union[tf.Tensor, tf.Variable],
x2: Union[tf.Tensor, tf.Variable],
@@ -829,7 +830,7 @@ def minimum(
@with_unsupported_dtypes(
{
- "2.13.0 and below": (
+ "2.14.0 and below": (
"uint8",
"uint16",
"uint32",
@@ -851,7 +852,7 @@ def reciprocal(
return tf.math.reciprocal(x)
-@with_unsupported_dtypes({"2.13.0 and below": ("bfloat16",)}, backend_version)
+@with_unsupported_dtypes({"2.14.0 and below": ("bfloat16",)}, backend_version)
def deg2rad(
x: Union[tf.Tensor, tf.Variable],
/,
@@ -880,7 +881,7 @@ def isreal(
@with_unsupported_dtypes(
- {"2.13.0 and below": ("uint8", "uint16", "uint32", "uint64", "complex", "bool")},
+ {"2.14.0 and below": ("uint8", "uint16", "uint32", "uint64", "complex", "bool")},
backend_version,
)
def fmod(
@@ -897,7 +898,7 @@ def fmod(
@with_unsupported_dtypes(
- {"2.13.0 and below": ("uint8", "uint16", "uint32", "uint64")}, backend_version
+ {"2.14.0 and below": ("uint8", "uint16", "uint32", "uint64")}, backend_version
)
def gcd(
x1: Union[tf.Tensor, tf.Variable, int, list, tuple],
@@ -915,7 +916,7 @@ def gcd(
@with_unsupported_dtypes(
{
- "2.13.0 and below": (
+ "2.14.0 and below": (
"uint8",
"uint16",
"uint32",
@@ -941,7 +942,7 @@ def angle(
@with_unsupported_dtypes(
{
- "2.13.0 and below": (
+ "2.14.0 and below": (
"uint8",
"uint16",
"uint32",
diff --git a/ivy/functional/backends/tensorflow/experimental/activations.py b/ivy/functional/backends/tensorflow/experimental/activations.py
index 16ee974538b56..1da7e9e2e80a7 100644
--- a/ivy/functional/backends/tensorflow/experimental/activations.py
+++ b/ivy/functional/backends/tensorflow/experimental/activations.py
@@ -26,7 +26,7 @@ def logit(
return tf.cast(tf.math.log(x / (1 - x)), x_dtype)
-@with_unsupported_dtypes({"2.13.0 and below": ("complex", "bool")}, backend_version)
+@with_unsupported_dtypes({"2.14.0 and below": ("complex", "bool")}, backend_version)
def thresholded_relu(
x: Tensor,
/,
@@ -42,7 +42,7 @@ def relu6(x: Tensor, /, *, complex_mode="jax", out: Optional[Tensor] = None) ->
return tf.nn.relu6(x)
-@with_supported_dtypes({"2.13.0 and below": ("float",)}, backend_version)
+@with_supported_dtypes({"2.14.0 and below": ("float",)}, backend_version)
def logsigmoid(
input: Tensor, /, *, complex_mode="jax", out: Optional[Tensor] = None
) -> Tensor:
@@ -51,7 +51,7 @@ def logsigmoid(
return tf.math.log_sigmoid(input)
-@with_supported_dtypes({"2.13.0 and below": ("float",)}, backend_version)
+@with_supported_dtypes({"2.14.0 and below": ("float",)}, backend_version)
def selu(x: Tensor, /, *, out: Optional[Tensor] = None) -> Tensor:
ret = tf.nn.selu(x)
if ivy.exists(out):
@@ -59,7 +59,7 @@ def selu(x: Tensor, /, *, out: Optional[Tensor] = None) -> Tensor:
return ivy.astype(ret, x.dtype)
-@with_unsupported_dtypes({"2.13.0 and below": ("complex",)}, backend_version)
+@with_unsupported_dtypes({"2.14.0 and below": ("complex",)}, backend_version)
def silu(
x: Tensor,
/,
@@ -72,7 +72,7 @@ def silu(
return ivy.astype(ret, x.dtype)
-@with_supported_dtypes({"2.13.0 and below": ("float",)}, backend_version)
+@with_supported_dtypes({"2.14.0 and below": ("float",)}, backend_version)
def elu(x: Tensor, /, *, alpha: float = 1.0, out: Optional[Tensor] = None) -> Tensor:
alpha = tf.cast(alpha, x.dtype)
ret = tf.cast(tf.where(x > 0, x, tf.multiply(alpha, tf.math.expm1(x))), x.dtype)
@@ -81,7 +81,7 @@ def elu(x: Tensor, /, *, alpha: float = 1.0, out: Optional[Tensor] = None) -> Te
return ivy.astype(ret, x.dtype)
-@with_supported_dtypes({"2.13.0 and below": ("float",)}, backend_version)
+@with_supported_dtypes({"2.14.0 and below": ("float",)}, backend_version)
def hardtanh(
x: Tensor,
/,
@@ -98,3 +98,91 @@ def hardtanh(
if ivy.exists(out):
return ivy.inplace_update(out, ret).astype(x.dtype)
return ivy.astype(ret, x.dtype)
+
+
+@with_supported_dtypes({"2.14.0 and below": ("float",)}, backend_version)
+def tanhshrink(
+ x: Tensor,
+ /,
+ *,
+ out: Optional[Tensor] = None,
+) -> Tensor:
+ ret = tf.math.subtract(x, tf.math.tanh(x))
+ if ivy.exists(out):
+ return ivy.inplace_update(out, ret).astype(x.dtype)
+ return ivy.astype(ret, x.dtype)
+
+
+@with_supported_dtypes({"2.14.0 and below": ("float",)}, backend_version)
+def threshold(
+ x: Tensor,
+ /,
+ *,
+ threshold: Union[int, float],
+ value: Union[int, float],
+ out: Optional[Tensor] = None,
+) -> Tensor:
+ ret = tf.where(x > threshold, x, value)
+ if ivy.exists(out):
+ return ivy.inplace_update(out, ret).astype(x.dtype)
+ return ivy.astype(ret, x.dtype)
+
+
+@with_supported_dtypes({"2.14.0 and below": ("float",)}, backend_version)
+def softshrink(
+ x: Tensor,
+ /,
+ *,
+ lambd: float = 0.5,
+ out: Optional[Tensor] = None,
+) -> Tensor:
+ ret = tf.where(
+ tf.math.greater(x, lambd),
+ x - lambd,
+ tf.where(tf.math.less(x, -lambd), x + lambd, 0),
+ )
+ if ivy.exists(out):
+ return ivy.inplace_update(out, ret).astype(x.dtype)
+ return ivy.astype(ret, x.dtype)
+
+
+@with_unsupported_dtypes({"2.14.0 and below": ("complex",)}, backend_version)
+def celu(
+ x: Tensor,
+ /,
+ *,
+ alpha: float = 1.0,
+ complex_mode="jax",
+ out: Optional[Tensor] = None,
+) -> Tensor:
+ return tf.math.maximum(0, x) + alpha * tf.math.expm1(tf.math.minimum(0, x) / alpha)
+
+
+@with_unsupported_dtypes({"2.14.0 and below": ("uint16",)}, backend_version)
+def scaled_tanh(
+ x: Tensor,
+ /,
+ *,
+ alpha: float = 1.7159,
+ beta: float = 0.67,
+ out: Optional[Tensor] = None,
+) -> Tensor:
+ return alpha * tf.nn.tanh(beta * x)
+
+
+@with_supported_dtypes({"2.14.0 and below": ("float",)}, backend_version)
+def hardshrink(
+ x: Tensor,
+ /,
+ *,
+ lambd: float = 0.5,
+ out: Optional[Tensor] = None,
+) -> Tensor:
+ ret = tf.where(
+ tf.math.greater(x, lambd),
+ x,
+ tf.where(tf.math.less(x, -lambd), x, 0),
+ )
+ if ivy.exists(out):
+ return ivy.inplace_update(out, ret).astype(x.dtype)
+ return ivy.astype(ret, x.dtype)
diff --git a/ivy/functional/backends/tensorflow/experimental/creation.py b/ivy/functional/backends/tensorflow/experimental/creation.py
index 871cb109f0eb3..86af4561091c2 100644
--- a/ivy/functional/backends/tensorflow/experimental/creation.py
+++ b/ivy/functional/backends/tensorflow/experimental/creation.py
@@ -13,7 +13,7 @@
@with_unsupported_device_and_dtypes(
- {"2.13.0 and below": {"cpu": ("bfloat16",)}},
+ {"2.14.0 and below": {"cpu": ("bfloat16",)}},
backend_version,
)
def kaiser_window(
@@ -107,14 +107,18 @@ def blackman_window(
out: Optional[Union[tf.Tensor, tf.Variable]] = None,
) -> Union[tf.Tensor, tf.Variable]:
if size < 2:
- return tf.ones([size], dtype=tf.result_type(size, 0.0))
+ return tf.cast(
+ tf.ones([size], dtype=tf.experimental.numpy.result_type(size, 0.0)),
+ dtype=dtype,
+ )
if periodic:
- count = tf.arange(size) / size
+ count = tf.experimental.numpy.arange(size) / size
else:
count = tf.linspace(start=0, stop=size, num=size)
-
- return (0.42 - 0.5 * tf.cos(2 * tf.pi * count)) + (
- 0.08 * tf.cos(2 * tf.pi * 2 * count)
+ return tf.cast(
+ (0.42 - 0.5 * tf.cos(2 * tf.experimental.numpy.pi * count))
+ + (0.08 * tf.cos(2 * tf.experimental.numpy.pi * 2 * count)),
+ dtype=dtype,
)
@@ -126,7 +130,7 @@ def unsorted_segment_sum(
return tf.math.unsorted_segment_sum(data, segment_ids, num_segments)
-@with_unsupported_dtypes({"2.13.0 and below": ("bool",)}, backend_version)
+@with_unsupported_dtypes({"2.14.0 and below": ("bool",)}, backend_version)
def trilu(
x: Union[tf.Tensor, tf.Variable],
/,
@@ -154,3 +158,22 @@ def mel_weight_matrix(
lower_edge_hertz=lower_edge_hertz,
upper_edge_hertz=upper_edge_hertz,
)
+
+
+def unsorted_segment_mean(
+ data: tf.Tensor,
+ segment_ids: tf.Tensor,
+ num_segments: Union[int, tf.Tensor],
+) -> tf.Tensor:
+ return tf.math.unsorted_segment_mean(data, segment_ids, num_segments)
+
+
+@with_unsupported_dtypes(
+ {"2.13.0 and below": ("bool", "bfloat16", "float16", "complex")}, backend_version
+)
+def polyval(coeffs: tf.Tensor, x: tf.Tensor):
+ result = tf.experimental.numpy.polyval(
+ coeffs,
+ x,
+ )
+ return result
diff --git a/ivy/functional/backends/tensorflow/experimental/elementwise.py b/ivy/functional/backends/tensorflow/experimental/elementwise.py
index 35790e70e0418..f23d1aa615677 100644
--- a/ivy/functional/backends/tensorflow/experimental/elementwise.py
+++ b/ivy/functional/backends/tensorflow/experimental/elementwise.py
@@ -1,5 +1,5 @@
import operator
-from typing import Union, Optional, Tuple, List
+from typing import Union, Optional, Tuple, List, Sequence
from numbers import Number
import tensorflow as tf
from tensorflow.python.ops.numpy_ops import np_math_ops
@@ -11,8 +11,50 @@
from .. import backend_version
+@with_unsupported_dtypes(
+ {
+ "2.13.0 and below": (
+ "complex64",
+ "complex128",
+ )
+ },
+ backend_version,
+)
+def amax(
+ x: tf.Tensor,
+ /,
+ *,
+ axis: Optional[Union[int, Sequence[int]]] = None,
+ keepdims: bool = False,
+ out: Optional[tf.Tensor] = None,
+) -> tf.Tensor:
+ axis = tuple(axis) if isinstance(axis, list) else axis
+ return tf.experimental.numpy.amax(x, axis=axis, keepdims=keepdims)
+
+
+@with_unsupported_dtypes(
+ {
+ "2.13.0 and below": (
+ "complex64",
+ "complex128",
+ )
+ },
+ backend_version,
+)
+def amin(
+ x: tf.Tensor,
+ /,
+ *,
+ axis: Optional[Union[int, Sequence[int]]] = None,
+ keepdims: bool = False,
+ out: Optional[tf.Tensor] = None,
+) -> tf.Tensor:
+ axis = tuple(axis) if isinstance(axis, list) else axis
+ return tf.experimental.numpy.amin(x, axis=axis, keepdims=keepdims)
+
+
@with_supported_dtypes(
- {"2.13.0 and below": ("float16", "float32", "float64")},
+ {"2.14.0 and below": ("float16", "float32", "float64")},
backend_version,
)
def lgamma(
@@ -35,7 +77,7 @@ def sinc(
@with_supported_dtypes(
- {"2.13.0 and below": ("bfloat16", "float16", "float32", "float64")}, backend_version
+ {"2.14.0 and below": ("bfloat16", "float16", "float32", "float64")}, backend_version
)
def fmax(
x1: Union[tf.Tensor, tf.Variable],
@@ -51,7 +93,7 @@ def fmax(
@with_unsupported_dtypes(
- {"2.13.0 and below": ("uint8", "uint16", "uint32", "uint64")}, backend_version
+ {"2.14.0 and below": ("uint8", "uint16", "uint32", "uint64")}, backend_version
)
def float_power(
x1: Union[tf.Tensor, tf.Variable, float, list, tuple],
@@ -103,7 +145,7 @@ def count_nonzero(
)
-@with_unsupported_dtypes({"2.13.0 and below": ("complex",)}, backend_version)
+@with_unsupported_dtypes({"2.14.0 and below": ("complex",)}, backend_version)
def nansum(
x: Union[tf.Tensor, tf.Variable],
/,
@@ -166,7 +208,7 @@ def allclose(
)
-@with_unsupported_dtypes({"2.13.0 and below": ("bfloat16",)}, backend_version)
+@with_unsupported_dtypes({"2.14.0 and below": ("bfloat16",)}, backend_version)
def fix(
x: Union[tf.Tensor, tf.Variable],
/,
@@ -176,7 +218,7 @@ def fix(
return tf.cast(tf.where(x > 0, tf.math.floor(x), tf.math.ceil(x)), x.dtype)
-@with_unsupported_dtypes({"2.13.0 and below": ("bflaot16", "float16")}, backend_version)
+@with_unsupported_dtypes({"2.14.0 and below": ("bflaot16", "float16")}, backend_version)
def nextafter(
x1: Union[tf.Tensor, tf.Variable],
x2: Union[tf.Tensor, tf.Variable],
@@ -188,7 +230,7 @@ def nextafter(
@with_unsupported_dtypes(
- {"2.13.0 and below": ("uint8", "uint16", "uint32", "uint64")}, backend_version
+ {"2.14.0 and below": ("uint8", "uint16", "uint32", "uint64")}, backend_version
)
def diff(
x: Union[tf.Tensor, tf.Variable, list, tuple],
@@ -211,7 +253,7 @@ def diff(
@with_supported_dtypes(
{
- "2.13.0 and below": (
+ "2.14.0 and below": (
"float32",
"float64",
)
@@ -240,7 +282,7 @@ def _normalize_axis_tuple(axis: Union[int, list, tuple], ndim: int) -> Tuple[int
axis = [operator.index(axis)]
except TypeError:
pass
- axis = tuple([_normalize_axis_index(ax, ndim) for ax in axis])
+ axis = tuple(_normalize_axis_index(ax, ndim) for ax in axis)
if len(set(axis)) != len(axis):
raise ValueError("repeated axis")
return axis
@@ -255,7 +297,6 @@ def gradient(
edge_order: int = 1,
) -> Union[tf.Tensor, List[tf.Tensor]]:
# https://github.com/numpy/numpy/blob/v1.24.3/numpy/lib/function_base.py#L969-L1312
- x.device
x = tf.experimental.numpy.asanyarray(x)
N = x.ndim # number of dimensions
if axis is None:
@@ -288,15 +329,13 @@ def gradient(
raise ValueError("distances must be either scalars or 1d")
if len(distances) != x.shape[axes[i]]:
raise ValueError(
- "when 1d, distances must match "
- "the length of the corresponding dimension {} {}".format(
- len(distances), x.shape[axes[i]]
- )
+ "when 1d, distances must match the length of the corresponding"
+ f" dimension {len(distances)} {x.shape[axes[i]]}"
)
if distances.dtype.is_integer:
# Convert numpy integer types to float64 to avoid modular
# arithmetic in np.diff(distances).
- distances = distances.astype(tf.experimental.numpy.float64)
+ distances = tf.cast(distances, tf.float64)
diffx = tf.experimental.numpy.diff(distances)
# if distances are constant reduce to the scalar case
# since it brings a consistent speedup
@@ -325,7 +364,7 @@ def gradient(
slice4 = [slice(None)] * N
if x.dtype.is_integer:
- x = x.astype(tf.experimental.numpy.float64)
+ x = tf.cast(x, tf.float64)
for axis, ax_dx in zip(axes, dx):
if x.shape[axis] < edge_order + 1:
@@ -430,7 +469,7 @@ def gradient(
@with_supported_dtypes(
{
- "2.13.0 and below": (
+ "2.14.0 and below": (
"float16",
"float32",
"float64",
@@ -460,7 +499,7 @@ def conj(
return tf.math.conj(x)
-@with_unsupported_dtypes({"2.13.0 and below": ("unsigned",)}, backend_version)
+@with_unsupported_dtypes({"2.14.0 and below": ("unsigned",)}, backend_version)
def ldexp(
x1: Union[tf.Tensor, tf.Variable],
x2: Union[tf.Tensor, tf.Variable, int],
@@ -481,7 +520,7 @@ def ldexp(
return tf.cast(ret, out_dtype)
-@with_unsupported_dtypes({"2.13.0 and below": ("unsigned",)}, backend_version)
+@with_unsupported_dtypes({"2.14.0 and below": ("unsigned",)}, backend_version)
def frexp(
x: Union[tf.Tensor, tf.Variable],
/,
diff --git a/ivy/functional/backends/tensorflow/experimental/gradients.py b/ivy/functional/backends/tensorflow/experimental/gradients.py
index 3ff76cd53b7fd..d2f4f45587273 100644
--- a/ivy/functional/backends/tensorflow/experimental/gradients.py
+++ b/ivy/functional/backends/tensorflow/experimental/gradients.py
@@ -1,10 +1,15 @@
# global
import tensorflow as tf
+from typing import Callable
# local
import ivy
from ivy.func_wrapper import inputs_to_native_arrays
from ivy.functional.ivy.gradients import _get_required_float_variables
+from ivy.functional.ivy.gradients import (
+ _flatten_containers,
+ _rebuild_flattened_containers,
+)
def bind_custom_gradient_function(func, custom_grad_fn):
@@ -19,3 +24,76 @@ def grad(upstream):
return ivy.to_native((ret, grad), nested=True, include_derived=True)
return inputs_to_native_arrays(custom_module)
+
+
+def vjp(func: Callable, *primals):
+ flattened_primals, ret_idxs = _flatten_containers(primals)
+ native_flattened_primals = ivy.to_native(flattened_primals, nested=True)
+
+ def grad_fn(*x_in):
+ return _flatten_containers(
+ ivy.to_native(
+ func(
+ *ivy.to_ivy(
+ _rebuild_flattened_containers(x_in, ret_idxs), nested=True
+ )
+ ),
+ nested=True,
+ include_derived=True,
+ )
+ )
+
+ with tf.GradientTape(persistent=True, watch_accessed_variables=False) as tape:
+ tape.watch(native_flattened_primals)
+ flat_primals_out, func_ret_idxs = grad_fn(*native_flattened_primals)
+
+ primals_out = _rebuild_flattened_containers(flat_primals_out, func_ret_idxs)
+
+ def vjpfun(x_in):
+ grads = tape.gradient(
+ flat_primals_out,
+ native_flattened_primals,
+ output_gradients=ivy.to_native(_flatten_containers(x_in)[0], nested=True),
+ )
+ return _rebuild_flattened_containers(
+ ivy.to_ivy(grads, nested=True, include_derived=True), ret_idxs
+ )
+
+ return (ivy.to_ivy(primals_out, nested=True, include_derived=True), vjpfun)
+
+
+def jvp(func: Callable, primals, tangents):
+ flattened_primals, ret_idxs = _flatten_containers(primals)
+ flattened_tangents, _ = _flatten_containers(tangents)
+
+ def grad_fn(*x_in):
+ return _flatten_containers(
+ ivy.to_native(
+ func(
+ *ivy.to_ivy(
+ _rebuild_flattened_containers(x_in, ret_idxs), nested=True
+ )
+ ),
+ nested=True,
+ include_derived=True,
+ )
+ )
+
+ flattened_primals = ivy.to_native(flattened_primals, nested=True)
+ flattened_tangents = ivy.to_native(flattened_tangents, nested=True)
+
+ with tf.autodiff.ForwardAccumulator(
+ flattened_primals,
+ flattened_tangents,
+ ) as acc:
+ flat_primals_out, func_ret_idxs = grad_fn(*flattened_primals)
+ tangents_out = acc.jvp(flat_primals_out)
+
+ return ivy.to_ivy(
+ (
+ _rebuild_flattened_containers(flat_primals_out, func_ret_idxs),
+ _rebuild_flattened_containers(tangents_out, func_ret_idxs),
+ ),
+ nested=True,
+ include_derived=True,
+ )
diff --git a/ivy/functional/backends/tensorflow/experimental/layers.py b/ivy/functional/backends/tensorflow/experimental/layers.py
index 59ccbf983b637..a75fd79321f50 100644
--- a/ivy/functional/backends/tensorflow/experimental/layers.py
+++ b/ivy/functional/backends/tensorflow/experimental/layers.py
@@ -44,7 +44,7 @@ def max_pool1d(
) -> Union[tf.Tensor, tf.Variable]:
dims = 1
kernel, strides, padding, dilation = _validate_max_pool_params(
- kernel, strides, padding, dilation, ceil_mode, dims=dims
+ kernel, strides, padding, dilation, ceil_mode, dims, data_format
)
if data_format == "NCW":
@@ -76,13 +76,12 @@ def max_pool1d(
)
padding = [(0, 0)] + list(padding) + [(0, 0)]
x = tf.pad(x, padding, constant_values=-math.inf)
- else:
- if isinstance(padding, list) and any(
- [item != 0 for sublist in padding for item in sublist]
- ):
- raise NotImplementedError(
- "Nonzero explicit padding is not supported for depthwise max pooling"
- )
+ elif isinstance(padding, list) and any(
+ item != 0 for sublist in padding for item in sublist
+ ):
+ raise NotImplementedError(
+ "Nonzero explicit padding is not supported for depthwise max pooling"
+ )
res = tf.nn.pool(x, kernel, "MAX", strides, "VALID", dilations=dilation)
@@ -109,7 +108,7 @@ def max_pool2d(
) -> Union[tf.Tensor, tf.Variable]:
dims = 2
kernel, strides, padding, dilation = _validate_max_pool_params(
- kernel, strides, padding, dilation, ceil_mode, dims=dims
+ kernel, strides, padding, dilation, ceil_mode, dims, data_format
)
if data_format == "NCHW":
@@ -145,22 +144,21 @@ def max_pool2d(
(pad_w // 2, pad_w - pad_w // 2),
]
- x_shape = x.shape[1:-1]
-
if ceil_mode:
+ x_shape = x.shape[1:-1]
+
for i in range(dims):
padding[i] = _padding_ceil_mode(
x_shape[i], new_kernel[i], padding[i], strides[i]
)
padding = [(0, 0)] + list(padding) + [(0, 0)]
x = tf.pad(x, padding, constant_values=-math.inf)
- else:
- if isinstance(padding, list) and any(
- [item != 0 for sublist in padding for item in sublist]
- ):
- raise NotImplementedError(
- "Nonzero explicit padding is not supported for depthwise max pooling"
- )
+ elif isinstance(padding, list) and any(
+ item != 0 for sublist in padding for item in sublist
+ ):
+ raise NotImplementedError(
+ "Nonzero explicit padding is not supported for depthwise max pooling"
+ )
res = tf.nn.pool(x, kernel, "MAX", strides, "VALID", dilations=dilation)
@@ -174,7 +172,7 @@ def max_pool2d(
@with_unsupported_dtypes(
- {"2.13.0 and below": ("bfloat16", "float64", "float16")}, backend_version
+ {"2.14.0 and below": ("bfloat16", "float64", "float16")}, backend_version
)
def max_pool3d(
x: Union[tf.Tensor, tf.Variable],
@@ -190,7 +188,7 @@ def max_pool3d(
) -> Union[tf.Tensor, tf.Variable]:
dims = 3
kernel, strides, padding, dilation = _validate_max_pool_params(
- kernel, strides, padding, dilation, ceil_mode, dims=dims
+ kernel, strides, padding, dilation, ceil_mode, dims, data_format
)
if data_format == "NCDHW":
@@ -217,8 +215,8 @@ def max_pool3d(
)
if not depth_pooling:
- x_shape = x.shape[1:-1]
new_kernel = [dilation[i] * (kernel[i] - 1) + 1 for i in range(dims)]
+ x_shape = x.shape[1:-1]
if isinstance(padding, str):
pad_d = _handle_padding(x_shape[0], strides[0], new_kernel[0], padding)
pad_h = _handle_padding(x_shape[1], strides[1], new_kernel[1], padding)
@@ -236,13 +234,12 @@ def max_pool3d(
)
padding = [(0, 0)] + list(padding) + [(0, 0)]
x = tf.pad(x, padding, constant_values=-math.inf)
- else:
- if isinstance(padding, list) and any(
- [item != 0 for sublist in padding for item in sublist]
- ):
- raise NotImplementedError(
- "Nonzero explicit padding is not supported for depthwise max pooling"
- )
+ elif isinstance(padding, list) and any(
+ item != 0 for sublist in padding for item in sublist
+ ):
+ raise NotImplementedError(
+ "Nonzero explicit padding is not supported for depthwise max pooling"
+ )
res = tf.nn.pool(x, kernel, "MAX", strides, "VALID", dilations=dilation)
@@ -280,12 +277,12 @@ def _handle_manual_pad_avg_pool(x, kernel, strides, padding, ceil_mode, dims):
return padding, pad_specific, c
-@with_unsupported_dtypes({"2.13.0 and below": ("bfloat16", "float64")}, backend_version)
+@with_unsupported_dtypes({"2.14.0 and below": ("bfloat16", "float64")}, backend_version)
def avg_pool1d(
x: Union[tf.Tensor, tf.Variable],
kernel: Union[int, Tuple[int]],
strides: Union[int, Tuple[int]],
- padding: str,
+ padding: Union[str, int, List[Tuple[int, int]]],
/,
*,
data_format: str = "NWC",
@@ -351,13 +348,13 @@ def avg_pool1d(
@with_unsupported_dtypes(
- {"2.13.0 and below": ("bfloat16", "float64", "float16")}, backend_version
+ {"2.14.0 and below": ("bfloat16", "float64", "float16")}, backend_version
)
def avg_pool2d(
x: Union[tf.Tensor, tf.Variable],
kernel: Union[int, Tuple[int], Tuple[int, int]],
strides: Union[int, Tuple[int], Tuple[int, int]],
- padding: str,
+ padding: Union[str, int, List[Tuple[int, int]]],
/,
*,
data_format: str = "NHWC",
@@ -443,13 +440,13 @@ def avg_pool2d(
@with_unsupported_dtypes(
- {"2.13.0 and below": ("bfloat16", "float64", "float16")}, backend_version
+ {"2.14.0 and below": ("bfloat16", "float64", "float16")}, backend_version
)
def avg_pool3d(
x: Union[tf.Tensor, tf.Variable],
kernel: Union[int, Tuple[int], Tuple[int, int, int]],
strides: Union[int, Tuple[int], Tuple[int, int, int]],
- padding: str,
+ padding: Union[str, int, List[Tuple[int, int]]],
/,
*,
data_format: str = "NDHWC",
@@ -548,7 +545,7 @@ def avg_pool3d(
@with_unsupported_dtypes(
- {"2.13.0 and below": ("bfloat16", "float64", "float16")}, backend_version
+ {"2.14.0 and below": ("bfloat16", "float64", "float16")}, backend_version
)
def pool(
x: Union[tf.Tensor, tf.Variable],
@@ -574,7 +571,7 @@ def pool(
)
-@with_supported_dtypes({"2.13.0 and below": ("float32", "float64")}, backend_version)
+@with_supported_dtypes({"2.14.0 and below": ("float32", "float64")}, backend_version)
def dct(
x: Union[tf.Tensor, tf.Variable],
/,
@@ -649,7 +646,7 @@ def _ifft_norm(
@with_supported_dtypes(
- {"2.13.0 and below": ("complex", "float32", "float64")}, backend_version
+ {"2.14.0 and below": ("complex", "float32", "float64")}, backend_version
)
def fft(
x: Union[tf.Tensor, tf.Variable],
@@ -684,7 +681,7 @@ def fft(
raise ivy.utils.exceptions.IvyError(
f"Invalid data points {n}, expecting more than 1"
)
- if norm != "backward" and norm != "ortho" and norm != "forward":
+ if norm not in ["backward", "ortho", "forward"]:
raise ivy.utils.exceptions.IvyError(f"Unrecognized normalization mode {norm}")
if x.shape[dim] != n:
s = list(x.shape)
@@ -712,7 +709,7 @@ def fft(
return ret
-@with_unsupported_dtypes({"2.13.0 and below": ("complex",)}, backend_version)
+@with_unsupported_dtypes({"2.14.0 and below": ("complex",)}, backend_version)
def dropout(
x: Union[tf.Tensor, tf.Variable],
prob: float,
@@ -727,7 +724,7 @@ def dropout(
) -> Union[tf.Tensor, tf.Variable]:
x = ivy.astype(x, dtype) if dtype else x
res = tf.nn.dropout(x, prob, noise_shape=noise_shape, seed=seed) if training else x
- res = tf.multiply(res, (1.0 - prob)) if not scale else res
+ res = res if scale else tf.multiply(res, (1.0 - prob))
return res
@@ -826,7 +823,7 @@ def ifft(
raise ivy.utils.exceptions.IvyError(
f"Invalid data points {n}, expecting more than 1"
)
- if norm != "backward" and norm != "ortho" and norm != "forward":
+ if norm not in ["backward", "ortho", "forward"]:
raise ivy.utils.exceptions.IvyError(f"Unrecognized normalization mode {norm}")
if x.shape[dim] != n:
s = list(x.shape)
@@ -854,7 +851,7 @@ def ifft(
return ret
-@with_unsupported_dtypes({"2.13.0 and below": ("complex",)}, backend_version)
+@with_unsupported_dtypes({"2.14.0 and below": ("complex",)}, backend_version)
def embedding(
weights: Union[tf.Tensor, tf.Variable],
indices: Union[tf.Tensor, tf.Variable],
@@ -878,12 +875,13 @@ def interpolate(
"linear",
"bilinear",
"trilinear",
+ "nd",
"nearest",
"area",
- "nearest-exact",
+ "nearest_exact",
"tf_area",
+ "tf_bicubic",
"bicubic",
- "bicubic_tensorflow",
"mitchellcubic",
"lanczos3",
"lanczos5",
@@ -891,45 +889,56 @@ def interpolate(
] = "linear",
scale_factor: Optional[Union[Sequence[int], int]] = None,
recompute_scale_factor: Optional[bool] = None,
- align_corners: Optional[bool] = None,
+ align_corners: bool = False,
antialias: bool = False,
out: Optional[Union[tf.Tensor, tf.Variable]] = None,
):
- dims = len(x.shape) - 2
- size = _get_size(scale_factor, size, dims, x.shape)
- remove_dim = False
- if mode in ["linear", "tf_area", "lanczos3", "lanczos5", "nearest-exact"]:
- if dims == 1:
- size = (1,) + tuple(size)
- x = tf.expand_dims(x, axis=-2)
- dims = 2
- remove_dim = True
- mode = (
- "bilinear"
- if mode == "linear"
- else (
- "area"
- if mode == "tf_area"
- else "nearest" if mode == "nearest-exact" else mode
+ input_size = ivy.shape(x)[2:]
+ dims = len(input_size)
+ size, _ = _get_size(scale_factor, size, dims, input_size)
+ if all(a == b for a, b in zip(size, input_size)):
+ ret = x
+ else:
+ remove_dim = False
+ if mode in ["linear", "tf_area", "lanczos3", "lanczos5", "nearest-exact"]:
+ if dims == 1:
+ size = (1,) + tuple(size)
+ x = tf.expand_dims(x, axis=-2)
+ dims = 2
+ remove_dim = True
+ mode = (
+ "bilinear"
+ if mode == "linear"
+ else (
+ "area"
+ if mode == "tf_area"
+ else "nearest" if mode == "nearest-exact" else mode
+ )
)
+ if mode == "tf_bicubic":
+ mode = "bicubic"
+ x = tf.transpose(x, (0, *range(2, dims + 2), 1))
+ ret = tf.transpose(
+ tf.cast(
+ tf.image.resize(x, size=size, method=mode, antialias=antialias), x.dtype
+ ),
+ (0, dims + 1, *range(1, dims + 1)),
)
- if mode == "bicubic_tensorflow":
- mode = "bicubic"
- x = tf.transpose(x, (0, *range(2, dims + 2), 1))
- ret = tf.transpose(
- tf.cast(
- tf.image.resize(x, size=size, method=mode, antialias=antialias), x.dtype
- ),
- (0, dims + 1, *range(1, dims + 1)),
- )
- if remove_dim:
- ret = tf.squeeze(ret, axis=-2)
+ if remove_dim:
+ ret = tf.squeeze(ret, axis=-2)
+ if ivy.exists(out):
+ return ivy.inplace_update(out, ret)
return ret
-interpolate.partial_mixed_handler = lambda x, *args, mode="linear", scale_factor=None, recompute_scale_factor=None, align_corners=None, **kwargs: ( # noqa: E501
- (not align_corners and (len(x.shape) - 2) < 2)
+interpolate.partial_mixed_handler = (
+ lambda x, *args, mode="linear", recompute_scale_factor=None, align_corners=None, **kwargs: len( # noqa: E501
+ x.shape
+ )
+ < 4
and mode not in ["nearest", "area", "bicubic", "nd"]
+ and not align_corners
+ and recompute_scale_factor
)
@@ -956,10 +965,10 @@ def trans_x_to_s(
dim: Sequence[int] = (-2, -1),
) -> Union[tf.Tensor, tf.Variable]:
"""Change the shape of the input array x to the desired output shape s."""
- if x.dtype != tf.complex128 and x.dtype != tf.complex64:
+ if x.dtype not in [tf.complex128, tf.complex64]:
x = tf.cast(x, tf.float32)
x_shape = x.shape
- if dim == (-1, -2) or dim == (1, 0):
+ if dim in [(-1, -2), (1, 0)]:
s = (s[1], s[0])
if s[0] >= x_shape[0] and s[1] >= x_shape[1]:
paddings = tf.constant([[0, s[0] - x_shape[0]], [0, s[1] - x_shape[1]]])
@@ -1040,7 +1049,7 @@ def _fft2_helper(x, shape, axes):
return x
-@with_supported_dtypes({"2.13.0 and below": ("complex",)}, backend_version)
+@with_supported_dtypes({"2.14.0 and below": ("complex",)}, backend_version)
def fft2(
x: Union[tf.Tensor, tf.Variable],
*,
@@ -1081,9 +1090,9 @@ def shape_and_axes_validation(shape, axes, input_rank_tensor):
tf.size(shape),
input_rank_tensor,
message=(
- "Argument `shape` cannot have length greater than the rank of `x`. "
- "Received: {}"
- ).format(shape),
+ "Argument `shape` cannot have length greater than the rank of `x`."
+ f" Received: {shape}"
+ ),
)
]
with tf.control_dependencies(checks_shape):
@@ -1096,9 +1105,9 @@ def shape_and_axes_validation(shape, axes, input_rank_tensor):
tf.size(axes),
input_rank_tensor,
message=(
- "Argument `axes` cannot have length greater than the rank of `x`. "
- "Received: {}"
- ).format(axes),
+ "Argument `axes` cannot have length greater than the rank of `x`."
+ f" Received: {axes}"
+ ),
),
tf.debugging.assert_less(
axes,
@@ -1120,9 +1129,9 @@ def shape_and_axes_validation(shape, axes, input_rank_tensor):
tf.size(shape),
tf.size(axes),
message=(
- "Arguments `shape` and `axes` must have equal length. "
- "Received: {}, {}"
- ).format(shape, axes),
+ "Arguments `shape` and `axes` must have equal length. Received:"
+ f" {shape}, {axes}"
+ ),
)
]
with tf.control_dependencies(checks_shape_axes):
@@ -1177,7 +1186,7 @@ def rank_initialization(axes):
def norm_initialization(norm, shape, x):
if norm == "backward":
norm_factor = tf.constant(1, x.dtype)
- elif norm == "forward" or norm == "ortho":
+ elif norm in ["forward", "ortho"]:
norm_factor = tf.cast(tf.math.reduce_prod(shape), x.dtype)
if norm == "ortho":
norm_factor = tf.math.sqrt(norm_factor)
@@ -1398,6 +1407,89 @@ def _rfftn_helper(x, shape, axes, norm):
return x
+def rfft(
+ x: Union[tf.Tensor, tf.Variable],
+ /,
+ *,
+ n: Optional[int] = None,
+ axis: int = -1,
+ norm: Literal["backward", "ortho", "forward"] = "backward",
+ out: Optional[Union[tf.Tensor, tf.Variable]] = None,
+) -> Union[tf.Tensor, tf.Variable]:
+ # type cast
+ if x.dtype in [tf.complex64, tf.complex128]:
+ x = tf.math.real(x)
+ if x.dtype not in [tf.float32, tf.float64]:
+ x = tf.cast(x, tf.float32)
+
+ # axis check
+ if not isinstance(axis, int):
+ raise ivy.utils.exceptions.IvyError(
+ f"Expecting instead of {type(axis)}"
+ )
+
+ # axis normalization
+ naxis = axis
+ if axis < 0:
+ naxis = x.ndim + axis
+ if naxis < 0 or naxis >= x.ndim:
+ raise ivy.utils.exceptions.IvyError(
+ f"Axis {axis} is out of bounds for array of dimension {x.ndim}"
+ )
+ axis = naxis
+
+ # n checks
+ if n is None:
+ n = x.shape[axis]
+ if not isinstance(n, int):
+ raise ivy.utils.exceptions.IvyError(
+ f"Expecting instead of {type(n)}"
+ )
+ if n < 1:
+ raise ivy.utils.exceptions.IvyError(
+ f"Invalid number of FFT data points ({n}) specified."
+ )
+
+ # norm check & value
+ if norm == "backward":
+ inv_norm = tf.constant(1, dtype=x.dtype)
+ elif norm in ["forward", "ortho"]:
+ inv_norm = tf.cast(tf.math.reduce_prod(n), dtype=x.dtype)
+ if norm == "ortho":
+ inv_norm = tf.math.sqrt(inv_norm)
+ else:
+ raise ivy.utils.exceptions.IvyError(
+ f'Invalid norm value {norm}; should be "backward", "ortho" or "forward".'
+ )
+ fct = 1 / inv_norm
+
+ if x.shape[axis] != n:
+ s = list(x.shape)
+ if s[axis] > n:
+ index = [slice(None)] * len(s)
+ index[axis] = slice(0, n)
+ x = x[tuple(index)]
+ else:
+ s[axis] = n - s[axis]
+ z = tf.zeros(s, x.dtype)
+ x = tf.concat([x, z], axis=axis)
+
+ if axis == x.ndim - 1:
+ ret = tf.signal.rfft(x, fft_length=None, name=None)
+ else:
+ x = tf.experimental.numpy.swapaxes(x, axis, -1)
+ ret = tf.signal.rfft(x, fft_length=None, name=None)
+ ret = tf.experimental.numpy.swapaxes(ret, axis, -1)
+
+ ret *= tf.cast(fct, dtype=ret.dtype)
+
+ if x.dtype != tf.float64:
+ ret = tf.cast(ret, dtype=tf.complex64)
+ if ivy.exists(out):
+ return ivy.inplace_update(out, ret)
+ return ret
+
+
@with_supported_device_and_dtypes(
{
"2.5.0 and above": {
@@ -1430,7 +1522,7 @@ def rfftn(
# stft
-@with_supported_dtypes({"2.13.0 and below": ("complex",)}, backend_version)
+@with_supported_dtypes({"2.14.0 and below": ("complex",)}, backend_version)
def stft(
signals: Union[tf.Tensor, tf.Variable],
frame_length: int,
@@ -1538,15 +1630,14 @@ def sliding_window(
if isinstance(padding, str) and padding.upper() in ["VALID", "SAME"]:
padding = padding
+ elif padding[0] == padding[1] == 0:
+ padding = "VALID"
+ elif padding[0] == padding[1] != 0:
+ padding = "SAME"
else:
- if padding[0] == padding[1] == 0:
- padding = "VALID"
- elif padding[0] == padding[1] != 0:
- padding = "SAME"
- else:
- raise ivy.utils.exceptions.IvyError(
- f"Cannot convert padding sequence {padding} to TensorFlow padding mode"
- )
+ raise ivy.utils.exceptions.IvyError(
+ f"Cannot convert padding sequence {padding} to TensorFlow padding mode"
+ )
return tf.image.extract_patches(
images=input, sizes=kernel_size, strides=stride, rates=dilation, padding=padding
diff --git a/ivy/functional/backends/tensorflow/experimental/linear_algebra.py b/ivy/functional/backends/tensorflow/experimental/linear_algebra.py
index e4116a5111ba0..826360c73a34c 100644
--- a/ivy/functional/backends/tensorflow/experimental/linear_algebra.py
+++ b/ivy/functional/backends/tensorflow/experimental/linear_algebra.py
@@ -12,7 +12,7 @@
@with_unsupported_dtypes(
- {"2.13.0 and below": ("int", "float16", "bfloat16")}, backend_version
+ {"2.14.0 and below": ("int", "float16", "bfloat16")}, backend_version
)
def eigh_tridiagonal(
alpha: Union[tf.Tensor, tf.Variable],
@@ -96,7 +96,7 @@ def matrix_exp(
@with_supported_dtypes(
{
- "2.13.0 and below": (
+ "2.14.0 and below": (
"complex",
"float32",
"float64",
@@ -115,7 +115,7 @@ def eig(
@with_supported_dtypes(
{
- "2.13.0 and below": (
+ "2.14.0 and below": (
"complex",
"float32",
"float64",
@@ -140,9 +140,29 @@ def adjoint(
return tf.linalg.adjoint(x)
+@with_unsupported_dtypes(
+ {"2.13.0 and below": ("int", "float16", "bfloat16", "float64")}, backend_version
+)
+def solve_triangular(
+ x1: Union[tf.Tensor, tf.Variable],
+ x2: Union[tf.Tensor, tf.Variable],
+ /,
+ *,
+ upper: bool = True,
+ adjoint: bool = False,
+ unit_diagonal: bool = False,
+ out: Optional[Union[tf.Tensor, tf.Variable]] = None,
+) -> Union[tf.Tensor, tf.Variable]:
+ # Multiplying with a mask matrix can stop gradients on the diagonal.
+ if unit_diagonal:
+ w = tf.constant(tf.eye(x1.shape[-2], batch_shape=x1.shape[:-2], dtype=x1.dtype))
+ x1 = w + (1 - w) * x1
+ return tf.linalg.triangular_solve(x1, x2, lower=not upper, adjoint=adjoint)
+
+
@with_supported_dtypes(
{
- "2.13.0 and below": (
+ "2.14.0 and below": (
"bfloat16",
"float16",
"float32",
@@ -221,7 +241,7 @@ def lu_factor(
@with_supported_dtypes(
{
- "2.13.0 and below": (
+ "2.14.0 and below": (
"bfloat16",
"float16",
"float32",
diff --git a/ivy/functional/backends/tensorflow/experimental/losses.py b/ivy/functional/backends/tensorflow/experimental/losses.py
index 7af54de6bc2d4..e9140d6ee382e 100644
--- a/ivy/functional/backends/tensorflow/experimental/losses.py
+++ b/ivy/functional/backends/tensorflow/experimental/losses.py
@@ -8,7 +8,7 @@
from . import backend_version
-@with_unsupported_dtypes({"2.13.0 and below": "bool"}, backend_version)
+@with_unsupported_dtypes({"2.14.0 and below": "bool"}, backend_version)
def huber_loss(
input: tf.Tensor,
target: tf.Tensor,
@@ -30,7 +30,7 @@ def huber_loss(
return loss
-@with_unsupported_dtypes({"2.13.0 and below": "bool"}, backend_version)
+@with_unsupported_dtypes({"2.14.0 and below": "bool"}, backend_version)
def smooth_l1_loss(
input: tf.Tensor,
target: tf.Tensor,
@@ -50,7 +50,7 @@ def smooth_l1_loss(
return loss
-@with_unsupported_dtypes({"2.13.0 and below": "bool"}, backend_version)
+@with_unsupported_dtypes({"2.14.0 and below": "bool"}, backend_version)
def soft_margin_loss(
input: tf.Tensor,
target: tf.Tensor,
@@ -68,11 +68,11 @@ def soft_margin_loss(
return loss
-def _apply_loss_reduction(loss: tf.Tensor, reduction: str) -> tf.Tensor:
+def _apply_loss_reduction(loss: tf.Tensor, reduction: str, axis) -> tf.Tensor:
if reduction == "sum":
- return tf.math.reduce_sum(loss)
+ return tf.math.reduce_sum(loss, axis=axis)
elif reduction == "mean":
- return tf.reduce_mean(loss)
+ return tf.reduce_mean(loss, axis=axis)
else: # reduction == "none"
return loss
@@ -118,7 +118,7 @@ def _validate_poisson_nll_params(
@with_supported_device_and_dtypes(
{
- "2.13.0 and below": {
+ "2.14.0 and below": {
"cpu": ("float32", "float64"),
"gpu": ("float32", "float64"),
}
diff --git a/ivy/functional/backends/tensorflow/experimental/manipulation.py b/ivy/functional/backends/tensorflow/experimental/manipulation.py
index 109c23a8354ba..17fff8ff50f42 100644
--- a/ivy/functional/backends/tensorflow/experimental/manipulation.py
+++ b/ivy/functional/backends/tensorflow/experimental/manipulation.py
@@ -33,7 +33,7 @@ def moveaxis(
return tf.experimental.numpy.moveaxis(a, source, destination)
-@with_unsupported_dtypes({"2.13.0 and below": ("bfloat16",)}, backend_version)
+@with_unsupported_dtypes({"2.14.0 and below": ("bfloat16",)}, backend_version)
def heaviside(
x1: Union[tf.Tensor, tf.Variable],
x2: Union[tf.Tensor, tf.Variable],
@@ -84,7 +84,7 @@ def rot90(
return tf.experimental.numpy.rot90(m, k, axes)
-@with_unsupported_dtypes({"2.13.0 and below": ("unsigned", "complex")}, backend_version)
+@with_unsupported_dtypes({"2.14.0 and below": ("unsigned", "complex")}, backend_version)
def top_k(
x: tf.Tensor,
k: int,
@@ -126,7 +126,7 @@ def fliplr(
return tf.experimental.numpy.fliplr(m)
-@with_unsupported_dtypes({"2.13.0 and below": ("bfloat16",)}, backend_version)
+@with_unsupported_dtypes({"2.14.0 and below": ("bfloat16",)}, backend_version)
def i0(
x: Union[tf.Tensor, tf.Variable],
/,
@@ -339,9 +339,7 @@ def expand(
shape = list(shape)
for i, dim in enumerate(shape):
if dim < 0:
- shape[i] = x.shape.num_elements() / tf.reduce_prod(
- [s for s in shape if s > 0]
- )
+ shape[i] = x.shape[i]
return tf.broadcast_to(x, shape)
@@ -419,3 +417,137 @@ def unique_consecutive(
tf.cast(inverse_indices, tf.int64),
tf.cast(counts, tf.int64),
)
+
+
+def take(
+ x: Union[int, List, tf.Tensor, tf.Variable],
+ indices: Union[int, List, tf.Tensor, tf.Variable],
+ /,
+ *,
+ axis: Optional[int] = None,
+ mode: str = "clip",
+ fill_value: Optional[Number] = None,
+ out: Optional[Union[tf.Tensor, tf.Variable]] = None,
+) -> Union[tf.Tensor, tf.Variable]:
+ if mode not in ["raise", "wrap", "clip", "fill"]:
+ raise ValueError("mode must be one of 'clip', 'raise', 'wrap', or 'fill'")
+ if not isinstance(x, (tf.Tensor, tf.Variable)):
+ x = tf.constant(x)
+ if len(x.shape) == 0:
+ x = tf.constant([x])
+ if not isinstance(indices, (tf.Tensor, tf.Variable)):
+ indices = tf.constant(indices)
+ if indices.dtype.is_floating:
+ indices = tf.cast(indices, tf.int64)
+
+ # raise
+ if mode == "raise":
+ mode = "clip"
+ if ivy.exists(axis):
+ if axis >= len(x.shape):
+ raise tf.errors.InvalidArgumentError(
+ None,
+ None,
+ f"Shape must be at least rank {axis+1} but is rank {len(x.shape)}",
+ )
+ x_shape = x.shape[axis]
+ else:
+ x_shape = tf.reduce_prod(x.shape)
+
+ bound_check = (indices < -x_shape) | (indices >= x_shape)
+ if tf.reduce_any(bound_check):
+ if len(indices.shape) == 0:
+ raise tf.errors.InvalidArgumentError(
+ None, None, f"index {indices} is not in [-{x_shape}, {x_shape})"
+ )
+ else:
+ first_non_zero = tuple(
+ map(
+ lambda n: n[0].numpy(),
+ tf.experimental.numpy.nonzero(bound_check),
+ )
+ )
+ raise tf.errors.InvalidArgumentError(
+ None,
+ None,
+ f"indices{list(first_non_zero)} = {indices[first_non_zero]} "
+ f"is not in [-{x_shape}, {x_shape})",
+ )
+
+ # clip, wrap
+ if mode != "fill":
+ ret = tf.experimental.numpy.take(x, indices, axis=axis, mode=mode)
+ if ivy.exists(out):
+ ivy.inplace_update(out, ret)
+ return ret
+
+ # fill
+ x_dtype = x.dtype
+ if fill_value is None:
+ # set according to jax behaviour
+ # https://tinyurl.com/66jn68uj
+ if x_dtype.is_floating or x_dtype.is_complex:
+ # NaN for inexact types
+ fill_value = float("NaN")
+ else:
+ if x_dtype == tf.bool:
+ # True for booleans
+ fill_value = True
+ elif x_dtype.is_unsigned:
+ # the largest positive value for unsigned types
+ fill_value = x_dtype.max
+ else:
+ # the largest negative value for signed types
+ fill_value = x_dtype.min
+
+ fill_value = tf.constant(fill_value, dtype=x_dtype)
+ x_shape = x.shape
+ ret = tf.experimental.numpy.take(x, indices, axis=axis, mode="wrap")
+
+ if len(ret.shape) == 0:
+ # if scalar, scalar fill (replace)
+ if tf.reduce_any(indices != 0):
+ ret = fill_value
+ else:
+ rank = len(x.shape)
+ if ivy.exists(axis):
+ axis = ((axis % rank) + rank) % rank
+ x_shape = x_shape[axis]
+ else:
+ axis = 0
+ x_shape = tf.reduce_prod(x_shape)
+
+ bound_check = tf.constant((indices < -x_shape) | (indices >= x_shape))
+
+ if tf.reduce_any(bound_check):
+ if axis > 0:
+ bound_check = tf.broadcast_to(
+ bound_check, (*x.shape[:axis], *bound_check.shape)
+ )
+ end_dim = x.shape[-((rank - axis) - 1) :]
+ else:
+ end_dim = x.shape[-(rank - 1) :]
+
+ if bound_check.shape != ret.shape:
+ slicer = list([Ellipsis] + ([None] * len(end_dim)))
+ bound_check = tf.broadcast_to(bound_check[slicer], ret.shape)
+
+ ret = tf.where(bound_check, fill_value[None], ret)
+
+ if ivy.exists(out):
+ ivy.inplace_update(out, ret)
+ return ret
+
+
+def trim_zeros(a: tf.Tensor, /, *, trim: Optional[str] = "bf") -> tf.Tensor:
+ nonzero_indices = tf.where(a != 0)
+ first = tf.reduce_min(nonzero_indices)
+ last = tf.reduce_max(nonzero_indices) + 1
+
+ trim = trim.upper()
+ if "F" in trim:
+ first = tf.maximum(first, 0)
+ if "B" in trim:
+ last = tf.minimum(last, tf.cast(tf.shape(a)[0], tf.int64))
+
+ return a[first:last]
diff --git a/ivy/functional/backends/tensorflow/experimental/norms.py b/ivy/functional/backends/tensorflow/experimental/norms.py
index bd3a1abb624fa..d589909759f28 100644
--- a/ivy/functional/backends/tensorflow/experimental/norms.py
+++ b/ivy/functional/backends/tensorflow/experimental/norms.py
@@ -4,7 +4,7 @@
from . import backend_version
-@with_unsupported_dtypes({"2.13.0 and below": "uint8"}, backend_version)
+@with_unsupported_dtypes({"2.14.0 and below": "uint8"}, backend_version)
def l1_normalize(
x: Union[tf.Tensor, tf.Variable],
/,
@@ -29,7 +29,7 @@ def l2_normalize(
return tf.math.divide(x, denorm)
-@with_unsupported_dtypes({"2.13.0 and below": ("float16", "bfloat16")}, backend_version)
+@with_unsupported_dtypes({"2.14.0 and below": ("float16", "bfloat16")}, backend_version)
def batch_norm(
x: Union[tf.Tensor, tf.Variable],
mean: Union[tf.Tensor, tf.Variable],
diff --git a/ivy/functional/backends/tensorflow/experimental/random.py b/ivy/functional/backends/tensorflow/experimental/random.py
index 2b8e63d81efe1..d07cd3504420b 100644
--- a/ivy/functional/backends/tensorflow/experimental/random.py
+++ b/ivy/functional/backends/tensorflow/experimental/random.py
@@ -1,8 +1,6 @@
# global
from typing import Union, Optional, Sequence
import tensorflow as tf
-import tensorflow_probability as tfp
-from tensorflow_probability import distributions as tfd
from tensorflow.python.framework.dtypes import DType
# local
@@ -10,7 +8,6 @@
from .. import backend_version
from ivy.func_wrapper import with_unsupported_dtypes
from ivy.functional.ivy.random import (
- _check_bounds_and_get_shape,
_check_shapes_broadcastable,
)
@@ -18,7 +15,7 @@
# dirichlet
@with_unsupported_dtypes(
{
- "2.13.0 and below": (
+ "2.14.0 and below": (
"blfoat16",
"float16",
)
@@ -34,24 +31,8 @@ def dirichlet(
seed: Optional[int] = None,
dtype: Optional[tf.Tensor] = None,
) -> Union[tf.Tensor, tf.Variable]:
- size = size if size is not None else len(alpha)
-
- if dtype is None:
- dtype = tf.float64
- else:
- dtype = dtype
- if seed is not None:
- tf.random.set_seed(seed)
- return tf.cast(
- tfd.Dirichlet(
- concentration=alpha,
- validate_args=False,
- allow_nan_stats=True,
- force_probs_to_zero_outside_support=False,
- name="Dirichlet",
- ).sample(size),
- dtype=dtype,
- )
+ pass
+ # TODO: Implement purely in tensorflow
def beta(
@@ -65,13 +46,8 @@ def beta(
seed: Optional[int] = None,
out: Optional[Union[tf.Tensor, tf.Variable]] = None,
) -> Union[tf.Tensor, tf.Variable]:
- if not dtype:
- dtype = ivy.default_float_dtype()
- dtype = ivy.as_native_dtype(dtype)
- shape = _check_bounds_and_get_shape(alpha, beta, shape).shape
- alpha = tf.cast(alpha, dtype)
- beta = tf.cast(beta, dtype)
- return tfp.distributions.Beta(alpha, beta).sample(shape, seed=seed)
+ pass
+ # TODO: Implement purely in tensorflow
def gamma(
@@ -85,16 +61,11 @@ def gamma(
seed: Optional[int] = None,
out: Optional[Union[tf.Tensor, tf.Variable]] = None,
) -> Union[tf.Tensor, tf.Variable]:
- if not dtype:
- dtype = ivy.default_float_dtype()
- dtype = ivy.as_native_dtype(dtype)
- shape = _check_bounds_and_get_shape(alpha, beta, shape).shape
- alpha = tf.cast(alpha, dtype)
- beta = tf.cast(beta, dtype)
- return tfp.distributions.Gamma(alpha, beta).sample(shape, seed=seed)
+ pass
+ # TODO: Implement purely in tensorflow
-@with_unsupported_dtypes({"2.13.0 and below": ("bfloat16",)}, backend_version)
+@with_unsupported_dtypes({"2.14.0 and below": ("bfloat16",)}, backend_version)
def poisson(
lam: Union[float, tf.Tensor, tf.Variable],
*,
@@ -129,16 +100,5 @@ def bernoulli(
seed: Optional[int] = None,
out: Optional[Union[tf.Tensor, tf.Variable]] = None,
) -> Union[tf.Tensor, tf.Variable]:
- if seed is not None:
- tf.random.set_seed(seed)
- if logits is not None:
- logits = tf.cast(logits, dtype)
- if not _check_shapes_broadcastable(shape, logits.shape):
- shape = logits.shape
- elif probs is not None:
- probs = tf.cast(probs, dtype)
- if not _check_shapes_broadcastable(shape, probs.shape):
- shape = probs.shape
- return tfp.distributions.Bernoulli(
- logits=logits, probs=probs, dtype=dtype, allow_nan_stats=True
- ).sample(shape, seed)
+ pass
+ # TODO: Implement purely in tensorflow
diff --git a/ivy/functional/backends/tensorflow/experimental/searching.py b/ivy/functional/backends/tensorflow/experimental/searching.py
index a03488aa304c7..7fe85411390f1 100644
--- a/ivy/functional/backends/tensorflow/experimental/searching.py
+++ b/ivy/functional/backends/tensorflow/experimental/searching.py
@@ -9,7 +9,7 @@
@with_supported_dtypes(
{
- "2.13.0 and below": (
+ "2.14.0 and below": (
"int32",
"int64",
)
diff --git a/ivy/functional/backends/tensorflow/experimental/statistical.py b/ivy/functional/backends/tensorflow/experimental/statistical.py
index 77dfecb960c07..8bc2357b31b39 100644
--- a/ivy/functional/backends/tensorflow/experimental/statistical.py
+++ b/ivy/functional/backends/tensorflow/experimental/statistical.py
@@ -1,6 +1,6 @@
from typing import Union, Optional, Tuple, Sequence
import tensorflow as tf
-import tensorflow_probability as tfp
+
from tensorflow.python.ops.numpy_ops import np_math_ops
import ivy
from ivy import (
@@ -28,57 +28,13 @@ def histogram(
density: Optional[bool] = False,
out: Optional[Union[tf.Tensor, tf.Variable]] = None,
) -> Tuple[tf.Tensor]:
- min_a = tf.reduce_min(a)
- max_a = tf.reduce_max(a)
- if isinstance(bins, tf.Tensor) and range:
- raise ivy.exceptions.IvyException(
- "Must choose between specifying bins and range or bin edges directly"
- )
- if range:
- if isinstance(bins, int):
- bins = tf.cast(
- tf.linspace(start=range[0], stop=range[1], num=bins + 1), dtype=a.dtype
- )
- elif isinstance(bins, int):
- range = (min_a, max_a)
- bins = tf.cast(
- tf.linspace(start=range[0], stop=range[1], num=bins + 1), dtype=a.dtype
- )
- if tf.shape(bins)[0] < 2:
- raise ivy.exceptions.IvyException("bins must have at least 1 bin (size > 1)")
- if min_a < bins[0] and not extend_lower_interval:
- raise ivy.exceptions.IvyException(
- "Values of x outside of the intervals cause errors in tensorflow backend. "
- "Consider using extend_lower_interval to deal with this."
- )
- if max_a > bins[-1] and not extend_upper_interval:
- raise ivy.exceptions.IvyException(
- "Values of x outside of the intervals cause errors in tensorflow backend. "
- "Consider using extend_upper_interval to deal with this."
- )
- ret = tfp.stats.histogram(
- x=a,
- edges=bins,
- axis=axis,
- weights=weights,
- extend_lower_interval=extend_lower_interval,
- extend_upper_interval=extend_upper_interval,
- dtype=dtype,
- name="histogram",
- )
- if density:
- pass
- # TODO: Tensorflow native dtype argument is not working
- if dtype:
- ret = tf.cast(ret, dtype)
- bins = tf.cast(bins, dtype)
- # TODO: weird error when returning bins: return ret, bins
- return ret
+ # TODO: Implement in pure tensorflow
+ pass
@with_supported_dtypes(
{
- "2.13.0 and below": (
+ "2.14.0 and below": (
"float",
"complex",
)
@@ -93,13 +49,8 @@ def median(
keepdims: bool = False,
out: Optional[Union[tf.Tensor, tf.Variable]] = None,
) -> Union[tf.Tensor, tf.Variable]:
- return tfp.stats.percentile(
- input,
- 50.0,
- axis=axis,
- interpolation="midpoint",
- keepdims=keepdims,
- )
+ pass
+ # TODO: Implement in pure tensorflow
def nanmean(
@@ -115,6 +66,32 @@ def nanmean(
return tf.experimental.numpy.nanmean(a, axis=axis, keepdims=keepdims, dtype=dtype)
+def nanmin(
+ a: Union[tf.Tensor, tf.Variable],
+ /,
+ *,
+ axis: Optional[Union[int, Tuple[int]]] = None,
+ keepdims: Optional[bool] = False,
+ initial: Optional[Union[int, float, complex]] = None,
+ where: Optional[Union[tf.Tensor, tf.Variable]] = None,
+ out: Optional[Union[tf.Tensor, tf.Variable]] = None,
+) -> Union[tf.Tensor, tf.Variable]:
+ axis = tuple(axis) if isinstance(axis, list) else axis
+ nan_mask = tf.math.is_nan(a)
+ if where is not None:
+ nan_mask = tf.math.logical_or(nan_mask, tf.math.logical_not(where))
+
+ masked_tensor = tf.where(nan_mask, tf.constant(float("inf"), dtype=a.dtype), a)
+
+ if axis is None:
+ result = tf.math.reduce_min(masked_tensor, keepdims=keepdims)
+ else:
+ result = tf.math.reduce_min(masked_tensor, axis=axis, keepdims=keepdims)
+ if initial is not None:
+ result = tf.minimum(result, initial)
+ return result
+
+
def _infer_dtype(dtype: tf.DType):
default_dtype = ivy.infer_default_dtype(dtype)
if ivy.dtype_bits(dtype) < ivy.dtype_bits(default_dtype):
@@ -152,7 +129,7 @@ def _validate_quantile(q):
if not (0.0 <= q[i] <= 1.0):
return False
else:
- if not (tf.math.reduce_all(0 <= q) and tf.math.reduce_all(q <= 1)):
+ if not (tf.math.reduce_all(q >= 0) and tf.math.reduce_all(q <= 1)):
return False
return True
@@ -243,41 +220,6 @@ def _quantile(a, q, axis=None):
return tf.cast(out, ret_dtype)
-def _compute_quantile_wrapper(
- x,
- q,
- axis=None,
- keepdims=False,
- interpolation="linear",
-):
- if not _validate_quantile(q):
- raise ValueError("Quantiles must be in the range [0, 1]")
- if interpolation in [
- "linear",
- "lower",
- "higher",
- "midpoint",
- "nearest",
- "nearest_jax",
- ]:
- if interpolation == "nearest_jax":
- return _handle_axis(x, q, _quantile, keepdims=keepdims, axis=axis)
- else:
- axis = tuple(axis) if isinstance(axis, list) else axis
-
- return tfp.stats.percentile(
- x,
- tf.math.multiply(q, 100),
- axis=axis,
- interpolation=interpolation,
- keepdims=keepdims,
- )
- else:
- raise ValueError(
- "Interpolation must be 'linear', 'lower', 'higher', 'midpoint' or 'nearest'"
- )
-
-
def quantile(
a: Union[tf.Tensor, tf.Variable],
q: Union[tf.Tensor, float],
@@ -288,14 +230,8 @@ def quantile(
keepdims: bool = False,
out: Optional[Union[tf.Tensor, tf.Variable]] = None,
) -> Union[tf.Tensor, tf.Variable]:
- # added the nearest_jax mode to enable jax-like calculations for method="nearest"
- return _compute_quantile_wrapper(
- a,
- q,
- axis=axis,
- keepdims=keepdims,
- interpolation=interpolation,
- )
+ pass
+ # TODO: Implement in pure tensorflow
def corrcoef(
@@ -324,165 +260,6 @@ def corrcoef(
return cor
-def _nanmedian_helper(input, axis=None, keepdims=False):
- """
- The approach to Handle Nans in single dimensional plus multi-dimensional inputs are
- composed on two-parts.
-
- PART 1: In this part, you have axis=None, it means we have to work on
- flattened data, we don't need to work on different axis.there are two cases here
-
- Case 1: which is if our input data does contain all the Nans or not,
- if our input have just Nans (means no numbers) then we'll not use
- temp[~tf.math.is_nan(temp)] function with our input because it will remove all Nans
- and we get empty tensor and this raise an error when it sent to percentile function,
- in this case we need to keep this input but just we flatten the input and percentile
- function returns nan if it find nan in median and here all the input is nan then we
- get our result.
-
- Case 2: if we have a number (0.4, 0.3, 0. ,1., 2., .....) with nans then we use this
- function temp[~tf.math.is_nan(temp)], it will return a tensor by extracting the nans
- and just keeping the values, but remember the returned tensor will be flattened and
- axis=None work on flattene inputs, so in this case we are also on same page :)
-
- for example: [[12.0 ,4.0 ,ivy.nan], [ivy.nan, ivy.nan,2.2]] => returned:
- [12.0 ,4.0, 2.2] now this will be our new input in percentile function.
-
- PART 2: In this case you have to do more work because we now don't allow to work
- directly on flattened data, Here are two cases also.
-
- CASE 1: we need to consider axis parameter here, but percentile axis does work
- differently and we don't have median function in tensorflow yet, so we need to make
- our input data compatible to the axis, then we compute nanmedian along that specific
- axis. we transpose the input data according to our axis, axis can be (0,), (1,),
- (0,1), (0,1,2) and input can be multi-dimensional, so we need to take care of edge
- cases before making it compatible.
-
- CASE 2: Here the main Nan handling part comes, you can only use 1D inputs here so we
- have to flatten the input then we have jump parameter which is use to say how many
- iterations we want to make because we have to calculate the row-wise median along
- axis=None now, so we slice out some data from the flattened input and then we use
- that 1D Input to remove the nans and use it in our percentile.
-
- For example: input = [[ivy.nan, 3, ivy.nan, 7],[4, ivy.nan,6, 9]], axis=1
-
- flatten data -> [[nan 3. nan 7. 4. nan 6. 9.]]
- num_jumps -> 2 because we have to slice out this in (1, 4) and (1,4),
- then it works same as PART 1 CASE 1 AND CASE 2.
- now for first slice we get -> 5.0 and for second we get -> 6.0, these calculated
- along axis=1 now we append the data into result, so to make the shape of result
- compatible with the numpy output, we reshaped it.
-
- the result which we get from our _nanmedian_helper = [5., 6.]
- """
- dtype = input.dtype
- temp = tf.cast(input, tf.float64)
- num_dim = tf.rank(temp)
- keepdim_shape = tf.shape(temp)
- q = 50.0
-
- # PART 1
- if axis is None:
- # PART 1 CASE 1
- if tf.reduce_all(tf.math.is_nan(temp)):
- temp = tf.reshape(temp, shape=(1, -1))
- else:
- # PART 1 CASE 2
- temp = temp[~tf.math.is_nan(temp)]
-
- ret = tfp.stats.percentile(
- temp,
- q,
- axis=axis,
- interpolation="midpoint",
- keepdims=keepdims,
- )
- if dtype in [tf.int32, tf.int64, tf.float64]:
- ret = tf.cast(ret, dtype=tf.float64)
- elif dtype in [tf.float16, tf.bfloat16]:
- ret = tf.cast(ret, dtype=tf.float16)
- else:
- ret = tf.cast(ret, dtype=tf.float32)
- return ret
-
- axis = [axis] if isinstance(axis, int) else list(axis)
- # PART 2 CASE 1
- for i in axis:
- keepdim_shape = tf.tensor_scatter_nd_update(keepdim_shape, [[i]], [1])
- axis = [num_dim + x if x < 0 else x for x in axis]
- axis.sort()
- dimension = tf.size(temp.shape)
- while tf.size(axis) > 0:
- axis1 = axis[0]
- for axis2 in range(axis1 + 1, dimension):
- temp = tf.transpose(
- temp,
- perm=tf.tensor_scatter_nd_update(
- tf.range(tf.rank(temp)), [[axis1], [axis2]], [axis2, axis1]
- ),
- )
- axis1 = axis2
- axis = [x - 1 for x in axis]
- axis.pop(0)
- dimension = dimension - 1
- temp = tf.reshape(
- temp, shape=tf.concat([tf.shape(temp)[: (dimension - len(axis))], [-1]], axis=0)
- )
-
- tensor = tf.reshape(temp, shape=(1, -1))
- shape = temp.shape
- dim = temp.ndim
- slice_size = shape[len(shape) - 1]
- num_jumps = 1
- result = []
-
- if slice_size == 1:
- if dim == 2 and input.shape[0] == 1:
- return tensor
- if dim > 2 and input.shape[0] == 1:
- return tf.reshape(tensor, shape=input.shape)
-
- tensor = tf.reshape(tensor, shape=shape[:-1])
- return tensor
- # PART 2 CASE 2
- i = dim
- while i > 1:
- num_jumps *= shape[len(shape) - i]
- i -= 1
-
- for i in range(num_jumps):
- start = i * slice_size
- end = (i + 1) * slice_size
- arr = tensor[:, start:end]
- if tf.reduce_all(tf.math.is_nan(arr)):
- arr = tf.reshape(arr, shape=(1, -1))
- else:
- arr = arr[~tf.math.is_nan(arr)]
-
- ret = tfp.stats.percentile(
- arr, q, axis=None, interpolation="midpoint", keepdims=keepdims
- )
- if keepdims:
- ret = tf.squeeze(ret)
-
- result.append(ret)
-
- result = tf.reshape(result, shape=shape[:-1])
-
- if keepdims:
- keepdim_shape = tuple(keepdim_shape)
- result = tf.reshape(result, shape=keepdim_shape)
-
- if dtype in [tf.int32, tf.int64, tf.float64]:
- result = tf.cast(result, dtype=tf.float64)
- elif dtype in [tf.float16, tf.bfloat16]:
- result = tf.cast(result, dtype=tf.float16)
- else:
- result = tf.cast(result, dtype=tf.float32)
-
- return result
-
-
def nanmedian(
input: Union[tf.Tensor, tf.Variable],
/,
@@ -492,18 +269,13 @@ def nanmedian(
overwrite_input: bool = False,
out: Optional[Union[tf.Tensor, tf.Variable]] = None,
) -> Union[tf.Tensor, tf.Variable]:
- if overwrite_input:
- copied_input = tf.identity(input)
- return _nanmedian_helper(copied_input, axis, keepdims)
-
- else:
- result = _nanmedian_helper(input, axis, keepdims)
- return result
+ pass
+ # TODO: Implement in pure tensorflow
@with_supported_device_and_dtypes(
{
- "2.13.0 and below": {
+ "2.14.0 and below": {
"cpu": (
"int64",
"int32",
@@ -538,7 +310,7 @@ def bincount(
@with_supported_device_and_dtypes(
{
- "2.13.0 and below": {
+ "2.14.0 and below": {
"cpu": ("float32", "float64"),
"gpu": ("bfloat16", "float16", "float32", "float64"),
}
@@ -551,7 +323,7 @@ def igamma(
return tf.math.igamma(a, x)
-@with_unsupported_dtypes({"2.13.0 and below": ("float16", "bfloat16")}, backend_version)
+@with_unsupported_dtypes({"2.14.0 and below": ("float16", "bfloat16")}, backend_version)
def cov(
x1: tf.Tensor,
x2: tf.Tensor = None,
@@ -654,7 +426,7 @@ def cov(
@with_unsupported_dtypes(
- {"2.13.0 and below": ("bool",)},
+ {"2.14.0 and below": ("bool",)},
backend_version,
)
def cummax(
@@ -783,7 +555,7 @@ def __get_index(lst, indices=None, prefix=None):
@with_unsupported_dtypes(
- {"2.13.0 and below": ("bfloat16", "complex")},
+ {"2.14.0 and below": ("bfloat16", "complex")},
backend_version,
)
def cummin(
diff --git a/ivy/functional/backends/tensorflow/general.py b/ivy/functional/backends/tensorflow/general.py
index 7c484d9a7d414..bc70f80919cac 100644
--- a/ivy/functional/backends/tensorflow/general.py
+++ b/ivy/functional/backends/tensorflow/general.py
@@ -50,7 +50,7 @@ def current_backend_str() -> str:
def _check_query(query):
return not isinstance(query, list) and (
- not (ivy.is_array(query) and ivy.is_bool_dtype(query) ^ bool(query.ndim > 0))
+ not (ivy.is_array(query) and ivy.is_bool_dtype(query) and bool(query.ndim > 0))
)
@@ -66,6 +66,7 @@ def get_item(
get_item.partial_mixed_handler = lambda x, query, **kwargs: (
all(_check_query(i) for i in query)
+ and len({i.shape for i in query if ivy.is_array(i)}) == 1
if isinstance(query, tuple)
else _check_query(query)
)
@@ -109,8 +110,8 @@ def gather(
batch_dims: int = 0,
out: Optional[Union[tf.Tensor, tf.Variable]] = None,
) -> Union[tf.Tensor, tf.Variable]:
- axis = axis % len(params.shape)
- batch_dims = batch_dims % len(params.shape)
+ axis %= len(params.shape)
+ batch_dims %= len(params.shape)
ivy.utils.assertions.check_gather_input_valid(params, indices, axis, batch_dims)
return tf.gather(params, indices, axis=axis, batch_dims=batch_dims)
@@ -159,7 +160,7 @@ def gather_nd(
try:
return tf.gather_nd(params, indices, batch_dims=batch_dims)
except Exception: # fall back to compositional implementation
- batch_dims = batch_dims % len(params.shape)
+ batch_dims %= len(params.shape)
result = []
if batch_dims == 0:
result = gather_nd_helper(params, indices)
@@ -206,11 +207,10 @@ def inplace_decrement(
x.data = x_native
else:
x = ivy.Array(x_native)
+ elif ivy.is_ivy_array(x):
+ x.data -= val_native
else:
- if ivy.is_ivy_array(x):
- x.data -= val_native
- else:
- x = ivy.Array(val_native)
+ x = ivy.Array(val_native)
return x
@@ -326,31 +326,26 @@ def scatter_flat(
if not target_given:
target = tf.zeros([size], dtype=updates.dtype)
res = tf.tensor_scatter_nd_update(target, tf.expand_dims(indices, -1), updates)
+ elif reduction == "max":
+ res = tf.tensor_scatter_nd_max(target, tf.expand_dims(indices, -1), updates)
+ elif reduction == "min":
+ res = tf.tensor_scatter_nd_min(target, tf.expand_dims(indices, -1), updates)
+ elif reduction == "replace":
+ res = tf.tensor_scatter_nd_update(target, tf.expand_dims(indices, -1), updates)
+ elif reduction == "sum":
+ res = tf.tensor_scatter_nd_add(target, tf.expand_dims(indices, -1), updates)
else:
- if reduction == "sum":
- res = tf.tensor_scatter_nd_add(target, tf.expand_dims(indices, -1), updates)
- elif reduction == "min":
- res = tf.tensor_scatter_nd_min(target, tf.expand_dims(indices, -1), updates)
- elif reduction == "max":
- res = tf.tensor_scatter_nd_max(target, tf.expand_dims(indices, -1), updates)
- elif reduction == "replace":
- res = tf.tensor_scatter_nd_update(
- target, tf.expand_dims(indices, -1), updates
- )
- else:
- raise ivy.utils.exceptions.IvyException(
- "reduction is {}, but it must be one of "
- '"sum", "min", "max" or "replace"'.format(reduction)
- )
- if ivy.exists(out):
- return ivy.inplace_update(out, res)
- return res
+ raise ivy.utils.exceptions.IvyException(
+ f'reduction is {reduction}, but it must be one of "sum", "min", "max" or'
+ ' "replace"'
+ )
+ return ivy.inplace_update(out, res) if ivy.exists(out) else res
scatter_flat.support_native_out = True
-@with_unsupported_dtypes({"2.13.0 and below": ("bfloat16", "complex")}, backend_version)
+@with_unsupported_dtypes({"2.14.0 and below": ("bfloat16", "complex")}, backend_version)
def scatter_nd(
indices: Union[tf.Tensor, tf.Variable],
updates: Union[tf.Tensor, tf.Variable],
@@ -401,8 +396,8 @@ def scatter_nd(
res = tf.tensor_scatter_nd_update(target, indices, updates)
else:
raise ivy.utils.exceptions.IvyException(
- "reduction is {}, but it must be one of "
- '"sum", "min", "max", "mul" or "replace"'.format(reduction)
+ f'reduction is {reduction}, but it must be one of "sum", "min", "max",'
+ ' "mul" or "replace"'
)
if ivy.exists(out):
return ivy.inplace_update(out, res)
@@ -476,7 +471,7 @@ def _vmap(*args, **kwargs):
# Handling None in in_axes by broadcasting the axis_size
if isinstance(in_axes, (tuple, list)) and None in in_axes:
- none_axis_index = list()
+ none_axis_index = []
for index, axis in enumerate(in_axes):
if axis is None:
none_axis_index.append(index)
@@ -510,7 +505,7 @@ def _vmap(*args, **kwargs):
return _vmap
-@with_unsupported_dtypes({"2.13.0 and below": ("bfloat16", "complex")}, backend_version)
+@with_unsupported_dtypes({"2.14.0 and below": ("bfloat16", "complex")}, backend_version)
def isin(
elements: tf.Tensor,
test_elements: tf.Tensor,
diff --git a/ivy/functional/backends/tensorflow/gradients.py b/ivy/functional/backends/tensorflow/gradients.py
index 0c198817af2c5..d548e21bc7738 100644
--- a/ivy/functional/backends/tensorflow/gradients.py
+++ b/ivy/functional/backends/tensorflow/gradients.py
@@ -69,8 +69,8 @@ def execute_with_gradients(
/,
*,
retain_grads: bool = False,
- xs_grad_idxs: Optional[Sequence[Sequence[Union[str, int]]]] = [[0]],
- ret_grad_idxs: Optional[Sequence[Sequence[Union[str, int]]]] = [[0]],
+ xs_grad_idxs: Sequence[Sequence[Union[str, int]]] = ((0,),),
+ ret_grad_idxs: Sequence[Sequence[Union[str, int]]] = ((0,),),
):
# Conversion of required arrays to float variables and duplicate index chains
xs, xs_grad_idxs, xs_required, required_duplicate_index_chains, _ = (
diff --git a/ivy/functional/backends/tensorflow/layers.py b/ivy/functional/backends/tensorflow/layers.py
index e0af9420d0761..cc37b00bade0a 100644
--- a/ivy/functional/backends/tensorflow/layers.py
+++ b/ivy/functional/backends/tensorflow/layers.py
@@ -82,7 +82,7 @@ def _output_shape(
return output_shape
-@with_unsupported_dtypes({"2.13.0 and below": ("bfloat16", "complex")}, backend_version)
+@with_unsupported_dtypes({"2.14.0 and below": ("bfloat16", "complex")}, backend_version)
def conv1d(
x: Union[tf.Tensor, tf.Variable],
filters: Union[tf.Tensor, tf.Variable],
@@ -108,7 +108,7 @@ def conv1d(
return res
-@with_unsupported_dtypes({"2.13.0 and below": ("bfloat16", "complex")}, backend_version)
+@with_unsupported_dtypes({"2.14.0 and below": ("bfloat16", "complex")}, backend_version)
def conv1d_transpose(
x: Union[tf.Tensor, tf.Variable],
filters: Union[tf.Tensor, tf.Variable],
@@ -144,7 +144,7 @@ def conv1d_transpose(
return res
-@with_unsupported_dtypes({"2.13.0 and below": ("bfloat16", "complex")}, backend_version)
+@with_unsupported_dtypes({"2.14.0 and below": ("bfloat16", "complex")}, backend_version)
def conv2d(
x: Union[tf.Tensor, tf.Variable],
filters: Union[tf.Tensor, tf.Variable],
@@ -170,7 +170,7 @@ def conv2d(
return res
-@with_unsupported_dtypes({"2.13.0 and below": ("bfloat16", "complex")}, backend_version)
+@with_unsupported_dtypes({"2.14.0 and below": ("bfloat16", "complex")}, backend_version)
def conv2d_transpose(
x: Union[tf.Tensor, tf.Variable],
filters: Union[tf.Tensor, tf.Variable],
@@ -205,7 +205,7 @@ def conv2d_transpose(
return res
-@with_unsupported_dtypes({"2.13.0 and below": ("bfloat16", "complex")}, backend_version)
+@with_unsupported_dtypes({"2.14.0 and below": ("bfloat16", "complex")}, backend_version)
def depthwise_conv2d(
x: Union[tf.Tensor, tf.Variable],
filters: Union[tf.Tensor, tf.Variable],
@@ -231,7 +231,7 @@ def depthwise_conv2d(
return res
-@with_unsupported_dtypes({"2.13.0 and below": ("bfloat16", "complex")}, backend_version)
+@with_unsupported_dtypes({"2.14.0 and below": ("bfloat16", "complex")}, backend_version)
def conv3d(
x: Union[tf.Tensor, tf.Variable],
filters: Union[tf.Tensor, tf.Variable],
@@ -261,7 +261,7 @@ def conv3d(
return res
-@with_unsupported_dtypes({"2.13.0 and below": ("bfloat16", "complex")}, backend_version)
+@with_unsupported_dtypes({"2.14.0 and below": ("bfloat16", "complex")}, backend_version)
def conv3d_transpose(
x: Tensor,
filters: Tensor,
@@ -300,7 +300,7 @@ def conv3d_transpose(
return res
-@with_unsupported_dtypes({"2.13.0 and below": ("bfloat16", "complex")}, backend_version)
+@with_unsupported_dtypes({"2.14.0 and below": ("bfloat16", "complex")}, backend_version)
def conv_general_dilated(
x: Union[tf.Tensor, tf.Variable],
filters: Union[tf.Tensor, tf.Variable],
@@ -415,7 +415,7 @@ def conv_general_dilated(
return res
-@with_unsupported_dtypes({"2.13.0 and below": ("bfloat16", "complex")}, backend_version)
+@with_unsupported_dtypes({"2.14.0 and below": ("bfloat16", "complex")}, backend_version)
def conv_general_transpose(
x: Union[tf.Tensor, tf.Variable],
filters: Union[tf.Tensor, tf.Variable],
diff --git a/ivy/functional/backends/tensorflow/linear_algebra.py b/ivy/functional/backends/tensorflow/linear_algebra.py
index 2f0918588f289..10bf64c766fba 100644
--- a/ivy/functional/backends/tensorflow/linear_algebra.py
+++ b/ivy/functional/backends/tensorflow/linear_algebra.py
@@ -17,7 +17,7 @@
# -------------------#
-@with_unsupported_dtypes({"2.13.0 and below": ("float16", "bfloat16")}, backend_version)
+@with_unsupported_dtypes({"2.14.0 and below": ("float16", "bfloat16")}, backend_version)
def cholesky(
x: Union[tf.Tensor, tf.Variable],
/,
@@ -35,7 +35,7 @@ def cholesky(
@with_unsupported_dtypes(
{
- "2.13.0 and below": (
+ "2.14.0 and below": (
"complex",
"float16",
)
@@ -61,7 +61,7 @@ def cross(
@with_unsupported_dtypes(
{
- "2.13.0 and below": (
+ "2.14.0 and below": (
"float16",
"bfloat16",
)
@@ -77,7 +77,7 @@ def det(
return tf.linalg.det(x)
-@with_unsupported_dtypes({"2.13.0 and below": ("complex",)}, backend_version)
+@with_unsupported_dtypes({"2.14.0 and below": ("complex",)}, backend_version)
def diagonal(
x: Union[tf.Tensor, tf.Variable],
/,
@@ -90,7 +90,7 @@ def diagonal(
return tf.experimental.numpy.diagonal(x, offset, axis1=axis1, axis2=axis2)
-@with_unsupported_dtypes({"2.13.0 and below": ("float16", "bfloat16")}, backend_version)
+@with_unsupported_dtypes({"2.14.0 and below": ("float16", "bfloat16")}, backend_version)
def eig(
x: Union[tf.Tensor, tf.Variable],
/,
@@ -108,7 +108,7 @@ def eig(
return result_tuple(eigenvalues, eigenvectors)
-@with_unsupported_dtypes({"2.13.0 and below": ("float16", "bfloat16")}, backend_version)
+@with_unsupported_dtypes({"2.14.0 and below": ("float16", "bfloat16")}, backend_version)
def eigh(
x: Union[tf.Tensor, tf.Variable],
/,
@@ -135,7 +135,7 @@ def eigh(
return result_tuple(eigenvalues, eigenvectors)
-@with_unsupported_dtypes({"2.13.0 and below": ("float16", "bfloat16")}, backend_version)
+@with_unsupported_dtypes({"2.14.0 and below": ("float16", "bfloat16")}, backend_version)
def eigvalsh(
x: Union[tf.Tensor, tf.Variable],
/,
@@ -156,7 +156,7 @@ def eigvalsh(
@with_unsupported_dtypes(
{
- "2.13.0 and below": (
+ "2.14.0 and below": (
"int8",
"uint8",
"int16",
@@ -182,7 +182,7 @@ def inner(
@with_unsupported_dtypes(
{
- "2.13.0 and below": (
+ "2.14.0 and below": (
"float16",
"bfloat16",
)
@@ -200,7 +200,7 @@ def inv(
@with_unsupported_dtypes(
- {"2.13.0 and below": ("float16", "bfloat16", "bool")}, backend_version
+ {"2.14.0 and below": ("float16", "bfloat16", "bool")}, backend_version
)
def matmul(
x1: Union[tf.Tensor, tf.Variable],
@@ -279,7 +279,7 @@ def matmul(
@with_supported_dtypes(
- {"2.13.0 and below": ("float32", "float64", "complex")}, backend_version
+ {"2.14.0 and below": ("float32", "float64", "complex")}, backend_version
)
def matrix_norm(
x: Union[tf.Tensor, tf.Variable],
@@ -323,7 +323,7 @@ def matrix_norm(
return ret
-@with_unsupported_dtypes({"2.13.0 and below": ("float16", "bfloat16")}, backend_version)
+@with_unsupported_dtypes({"2.14.0 and below": ("float16", "bfloat16")}, backend_version)
def matrix_power(
x: Union[tf.Tensor, tf.Variable],
n: int,
@@ -356,7 +356,7 @@ def matrix_power(
@with_unsupported_dtypes(
- {"2.13.0 and below": ("bfloat16", "float16", "complex")},
+ {"2.14.0 and below": ("bfloat16", "float16", "complex")},
backend_version,
)
# noinspection PyPep8Naming
@@ -394,7 +394,7 @@ def matrix_rank(
@with_unsupported_dtypes(
{
- "2.13.0 and below": (
+ "2.14.0 and below": (
"float16",
"int8",
"int16",
@@ -421,7 +421,7 @@ def matrix_transpose(
# noinspection PyUnusedLocal,PyShadowingBuiltins
-@with_unsupported_dtypes({"2.13.0 and below": ("complex",)}, backend_version)
+@with_unsupported_dtypes({"2.14.0 and below": ("complex",)}, backend_version)
def outer(
x1: Union[tf.Tensor, tf.Variable],
x2: Union[tf.Tensor, tf.Variable],
@@ -434,7 +434,7 @@ def outer(
@with_unsupported_dtypes(
- {"2.13.0 and below": ("bfloat16", "float16", "complex")},
+ {"2.14.0 and below": ("bfloat16", "float16", "complex")},
backend_version,
)
def pinv(
@@ -452,7 +452,7 @@ def pinv(
return ret
-@with_unsupported_dtypes({"2.13.0 and below": ("float16", "bfloat16")}, backend_version)
+@with_unsupported_dtypes({"2.14.0 and below": ("float16", "bfloat16")}, backend_version)
def qr(
x: Union[tf.Tensor, tf.Variable],
/,
@@ -461,7 +461,7 @@ def qr(
out: Optional[
Tuple[Union[tf.Tensor, tf.Variable], Union[tf.Tensor, tf.Variable]]
] = None,
-) -> NamedTuple:
+) -> Tuple[Union[tf.Tensor, tf.Variable], Union[tf.Tensor, tf.Variable]]:
res = namedtuple("qr", ["Q", "R"])
if mode == "reduced":
q, r = tf.linalg.qr(x, full_matrices=False)
@@ -477,7 +477,7 @@ def qr(
return ret
-@with_unsupported_dtypes({"2.13.0 and below": ("float16", "bfloat16")}, backend_version)
+@with_unsupported_dtypes({"2.14.0 and below": ("float16", "bfloat16")}, backend_version)
def slogdet(
x: Union[tf.Tensor, tf.Variable],
/,
@@ -488,7 +488,7 @@ def slogdet(
@with_unsupported_dtypes(
- {"2.13.0 and below": ("bfloat16", "float16", "complex")},
+ {"2.14.0 and below": ("bfloat16", "float16", "complex")},
backend_version,
)
def solve(
@@ -531,7 +531,7 @@ def solve(
@with_unsupported_dtypes(
- {"2.13.0 and below": ("bfloat16", "float16", "complex")},
+ {"2.14.0 and below": ("bfloat16", "float16", "complex")},
backend_version,
)
def svd(
@@ -559,18 +559,20 @@ def svd(
return results(D)
-@with_unsupported_dtypes({"2.13.0 and below": ("float16", "bfloat16")}, backend_version)
+@with_unsupported_dtypes({"2.14.0 and below": ("float16", "bfloat16")}, backend_version)
def svdvals(
x: Union[tf.Tensor, tf.Variable],
/,
*,
+ driver: Optional[str] = None,
out: Optional[Union[tf.Tensor, tf.Variable]] = None,
) -> Union[tf.Tensor, tf.Variable]:
+ # TODO: handling the driver argument
ret = tf.linalg.svd(x, compute_uv=False)
return ret
-@with_supported_dtypes({"2.13.0 and below": ("float32",)}, backend_version)
+@with_supported_dtypes({"2.14.0 and below": ("float32",)}, backend_version)
def tensordot(
x1: Union[tf.Tensor, tf.Variable],
x2: Union[tf.Tensor, tf.Variable],
@@ -585,7 +587,7 @@ def tensordot(
@with_unsupported_dtypes(
- {"2.13.0 and below": ("bfloat16", "float16", "complex")},
+ {"2.14.0 and below": ("bfloat16", "float16", "complex")},
backend_version,
)
def trace(
@@ -604,7 +606,7 @@ def trace(
@with_unsupported_dtypes(
- {"2.13.0 and below": ("int16", "int8", "bool", "unsigned")}, backend_version
+ {"2.14.0 and below": ("int16", "int8", "bool", "unsigned")}, backend_version
)
def vecdot(
x1: Union[tf.Tensor, tf.Variable],
@@ -620,7 +622,7 @@ def vecdot(
@with_unsupported_dtypes(
{
- "2.13.0 and below": (
+ "2.14.0 and below": (
"float16",
"bfloat16",
"integer",
@@ -655,7 +657,7 @@ def vector_norm(
# ----- #
-@with_unsupported_dtypes({"2.13.0 and below": ("complex",)}, backend_version)
+@with_unsupported_dtypes({"2.14.0 and below": ("complex",)}, backend_version)
def diag(
x: Union[tf.Tensor, tf.Variable],
/,
@@ -667,7 +669,7 @@ def diag(
@with_unsupported_dtypes(
- {"2.13.0 and below": ("bfloat16", "float16", "complex", "unsigned")},
+ {"2.14.0 and below": ("bfloat16", "float16", "complex", "unsigned")},
backend_version,
)
def vander(
@@ -683,7 +685,7 @@ def vander(
@with_unsupported_dtypes(
{
- "2.13.0 and below": (
+ "2.14.0 and below": (
"int8",
"int16",
"int32",
diff --git a/ivy/functional/backends/tensorflow/manipulation.py b/ivy/functional/backends/tensorflow/manipulation.py
index d55c169b46e9b..2350017b1931e 100644
--- a/ivy/functional/backends/tensorflow/manipulation.py
+++ b/ivy/functional/backends/tensorflow/manipulation.py
@@ -10,7 +10,7 @@
import ivy
# noinspection PyProtectedMember
-from ivy.func_wrapper import with_supported_dtypes, with_unsupported_dtypes
+from ivy.func_wrapper import with_unsupported_dtypes
from ivy.functional.ivy.manipulation import _calculate_out_shape
from . import backend_version
@@ -106,7 +106,7 @@ def permute_dims(
return tf.transpose(x, perm=axes)
-@with_unsupported_dtypes({"2.13.0 and below": ("bool",)}, backend_version)
+@with_unsupported_dtypes({"2.14.0 and below": ("bool",)}, backend_version)
def reshape(
x: Union[tf.Tensor, tf.Variable],
/,
@@ -159,7 +159,7 @@ def squeeze(
) -> Union[tf.Tensor, tf.Variable]:
if isinstance(axis, int):
if ivy.any(x.shape[axis] > 1):
- raise ValueError(f"{x.shape[axis]} must be lesser than or equal to {1}")
+ raise ValueError(f"{x.shape[axis]} must be lesser than or equal to 1")
ret = tf.squeeze(x, axis)
elif axis is None:
ret = tf.squeeze(x)
@@ -177,9 +177,8 @@ def squeeze(
for i in axis_updated_after_squeeze:
if x.shape[i] > 1:
raise ValueError(
- "Expected dimension of size 1, but found dimension size {}".format(
- x.shape[i]
- )
+ "Expected dimension of size 1, but found dimension size"
+ f" {x.shape[i]}"
)
else:
x = tf.squeeze(x, i)
@@ -187,7 +186,7 @@ def squeeze(
return ret
-@with_unsupported_dtypes({"2.13.0 and below": ("bfloat16",)}, backend_version)
+@with_unsupported_dtypes({"2.14.0 and below": ("bfloat16",)}, backend_version)
def stack(
arrays: Union[Tuple[tf.Tensor], List[tf.Tensor]],
/,
@@ -219,9 +218,8 @@ def split(
if x.shape == ():
if num_or_size_splits is not None and num_or_size_splits != 1:
raise ivy.utils.exceptions.IvyException(
- "input array had no shape, but num_sections specified was {}".format(
- num_or_size_splits
- )
+ "input array had no shape, but num_sections specified was"
+ f" {num_or_size_splits}"
)
return [x]
if num_or_size_splits is None:
@@ -242,7 +240,6 @@ def split(
return tf.split(x, num_or_size_splits, axis)
-@with_supported_dtypes({"2.13.0 and below": ("int32", "int64")}, backend_version)
def repeat(
x: Union[tf.Tensor, tf.Variable],
/,
@@ -256,7 +253,7 @@ def repeat(
@with_unsupported_dtypes(
{
- "2.13.0 and below": (
+ "2.14.0 and below": (
"uint8",
"uint16",
"uint32",
@@ -327,7 +324,7 @@ def swapaxes(
return tf.transpose(x, config)
-@with_unsupported_dtypes({"2.13.0 and below": ("complex",)}, backend_version)
+@with_unsupported_dtypes({"2.14.0 and below": ("complex",)}, backend_version)
def clip(
x: Union[tf.Tensor, tf.Variable],
/,
diff --git a/ivy/functional/backends/tensorflow/random.py b/ivy/functional/backends/tensorflow/random.py
index a67fd37d46d6a..6508a5ac2603b 100644
--- a/ivy/functional/backends/tensorflow/random.py
+++ b/ivy/functional/backends/tensorflow/random.py
@@ -27,7 +27,7 @@
@with_supported_dtypes(
- {"2.13.0 and below": ("float", "int32", "int64")}, backend_version
+ {"2.14.0 and below": ("float", "int32", "int64")}, backend_version
)
def random_uniform(
*,
@@ -66,7 +66,7 @@ def random_normal(
return tf.random.normal(shape, mean, std, dtype=dtype, seed=seed)
-@with_unsupported_dtypes({"2.13.0 and below": ("bfloat16",)}, backend_version)
+@with_unsupported_dtypes({"2.14.0 and below": ("bfloat16",)}, backend_version)
def multinomial(
population_size: int,
num_samples: int,
diff --git a/ivy/functional/backends/tensorflow/searching.py b/ivy/functional/backends/tensorflow/searching.py
index 047441cff9b08..b9abffbee4076 100644
--- a/ivy/functional/backends/tensorflow/searching.py
+++ b/ivy/functional/backends/tensorflow/searching.py
@@ -12,7 +12,7 @@
# ------------------ #
-@with_unsupported_dtypes({"2.13.0 and below": ("complex",)}, backend_version)
+@with_unsupported_dtypes({"2.14.0 and below": ("complex",)}, backend_version)
def argmax(
x: Union[tf.Tensor, tf.Variable],
/,
diff --git a/ivy/functional/backends/tensorflow/set.py b/ivy/functional/backends/tensorflow/set.py
index dbc834e90c6a8..abb0c8d90e8f6 100644
--- a/ivy/functional/backends/tensorflow/set.py
+++ b/ivy/functional/backends/tensorflow/set.py
@@ -6,7 +6,7 @@
from . import backend_version
-@with_unsupported_dtypes({"2.13.0 and below": ("complex",)}, backend_version)
+@with_unsupported_dtypes({"2.14.0 and below": ("complex",)}, backend_version)
def unique_all(
x: Union[tf.Tensor, tf.Variable],
/,
@@ -78,7 +78,7 @@ def unique_all(
)
-@with_unsupported_dtypes({"2.13.0 and below": ("complex",)}, backend_version)
+@with_unsupported_dtypes({"2.14.0 and below": ("complex",)}, backend_version)
def unique_counts(
x: Union[tf.Tensor, tf.Variable],
/,
@@ -90,12 +90,18 @@ def unique_counts(
return Results(v, c)
-@with_unsupported_dtypes({"2.13.0 and below": ("complex",)}, backend_version)
+@with_unsupported_dtypes({"2.14.0 and below": ("complex",)}, backend_version)
def unique_inverse(
x: Union[tf.Tensor, tf.Variable],
/,
+ *,
+ axis: Optional[int] = None,
) -> Tuple[Union[tf.Tensor, tf.Variable], Union[tf.Tensor, tf.Variable]]:
Results = namedtuple("Results", ["values", "inverse_indices"])
+ if axis is None:
+ x = tf.reshape(x, shape=(-1,))
+ axis = 0
+
flat_tensor = tf.reshape(x, -1)
values = tf.unique(tf.sort(flat_tensor))[0]
values = tf.cast(values, dtype=x.dtype)
@@ -107,7 +113,7 @@ def unique_inverse(
return Results(values, inverse_indices)
-@with_unsupported_dtypes({"2.13.0 and below": ("complex",)}, backend_version)
+@with_unsupported_dtypes({"2.14.0 and below": ("complex",)}, backend_version)
def unique_values(
x: Union[tf.Tensor, tf.Variable],
/,
diff --git a/ivy/functional/backends/tensorflow/sorting.py b/ivy/functional/backends/tensorflow/sorting.py
index d7ca0ba90cdcf..efaf951cdc61c 100644
--- a/ivy/functional/backends/tensorflow/sorting.py
+++ b/ivy/functional/backends/tensorflow/sorting.py
@@ -8,7 +8,7 @@
from . import backend_version
-@with_unsupported_dtypes({"2.13.0 and below": ("complex", "bool")}, backend_version)
+@with_unsupported_dtypes({"2.14.0 and below": ("complex", "bool")}, backend_version)
def argsort(
x: Union[tf.Tensor, tf.Variable],
/,
@@ -24,7 +24,7 @@ def argsort(
return tf.cast(ret, dtype=tf.int64)
-@with_unsupported_dtypes({"2.13.0 and below": ("complex", "bool")}, backend_version)
+@with_unsupported_dtypes({"2.14.0 and below": ("complex", "bool")}, backend_version)
def sort(
x: Union[tf.Tensor, tf.Variable],
/,
@@ -43,7 +43,7 @@ def sort(
# msort
-@with_unsupported_dtypes({"2.13.0 and below": ("complex", "bool")}, backend_version)
+@with_unsupported_dtypes({"2.14.0 and below": ("complex", "bool")}, backend_version)
def msort(
a: Union[tf.Tensor, tf.Variable, list, tuple],
/,
@@ -53,7 +53,7 @@ def msort(
return tf.sort(a, axis=0)
-@with_unsupported_dtypes({"2.13.0 and below": ("complex",)}, backend_version)
+@with_unsupported_dtypes({"2.14.0 and below": ("complex",)}, backend_version)
def searchsorted(
x: Union[tf.Tensor, tf.Variable],
v: Union[tf.Tensor, tf.Variable],
diff --git a/ivy/functional/backends/tensorflow/statistical.py b/ivy/functional/backends/tensorflow/statistical.py
index 5a3a61afabad7..938f21c1834be 100644
--- a/ivy/functional/backends/tensorflow/statistical.py
+++ b/ivy/functional/backends/tensorflow/statistical.py
@@ -4,7 +4,6 @@
# local
import ivy
-from ivy.functional.ivy.statistical import _get_promoted_type_of_operands
from ivy.func_wrapper import with_unsupported_dtypes
from . import backend_version
from ivy.utils.einsum_parser import legalise_einsum_expr
@@ -13,7 +12,7 @@
# -------------------#
-@with_unsupported_dtypes({"2.13.0 and below": ("complex",)}, backend_version)
+@with_unsupported_dtypes({"2.14.0 and below": ("complex",)}, backend_version)
def min(
x: Union[tf.Tensor, tf.Variable],
/,
@@ -52,7 +51,7 @@ def max(
return tf.math.reduce_max(x, axis=axis, keepdims=keepdims)
-@with_unsupported_dtypes({"2.13.0 and below": ("bool",)}, backend_version)
+@with_unsupported_dtypes({"2.14.0 and below": ("bool",)}, backend_version)
def mean(
x: Union[tf.Tensor, tf.Variable],
/,
@@ -164,7 +163,7 @@ def var(
# ------#
-@with_unsupported_dtypes({"2.13.0 and below": "bfloat16"}, backend_version)
+@with_unsupported_dtypes({"2.14.0 and below": "bfloat16"}, backend_version)
def cumprod(
x: Union[tf.Tensor, tf.Variable],
/,
@@ -209,7 +208,7 @@ def cumsum(
@with_unsupported_dtypes(
- {"2.13.0 and below": ("unsigned", "int8", "int16")},
+ {"2.14.0 and below": ("unsigned", "int8", "int16")},
backend_version,
)
def einsum(
@@ -217,6 +216,15 @@ def einsum(
*operands: Union[tf.Tensor, tf.Variable],
out: Optional[Union[tf.Tensor, tf.Variable]] = None,
) -> Union[tf.Tensor, tf.Variable]:
- dtype = _get_promoted_type_of_operands(operands)
equation = legalise_einsum_expr(*[equation, *operands])
- return tf.cast(tf.einsum(equation, *operands), dtype)
+ dtype_list = set(map(lambda x: x.dtype, operands))
+ dtype = dtype_list.pop()
+ if len(dtype_list) > 0:
+ for d in dtype_list:
+ dtype = ivy.promote_types(dtype, d)
+ dtype = ivy.as_native_dtype(dtype)
+ operands = list(
+ map(lambda x: tf.cast(x, dtype) if x.dtype != dtype else x, operands)
+ )
+
+ return tf.einsum(equation, *operands)
diff --git a/ivy/functional/backends/tensorflow/sub_backends/tf_probability/__init__.py b/ivy/functional/backends/tensorflow/sub_backends/tf_probability/__init__.py
new file mode 100644
index 0000000000000..0eb7a6e446d71
--- /dev/null
+++ b/ivy/functional/backends/tensorflow/sub_backends/tf_probability/__init__.py
@@ -0,0 +1,10 @@
+from .experimental import random, statistical
+from . import elementwise
+from .elementwise import *
+from .experimental.random import *
+from .experimental.statistical import *
+
+
+name = "tf_probability"
+
+incompatible_sub_backends = ()
diff --git a/ivy/functional/backends/tensorflow/sub_backends/tf_probability/elementwise.py b/ivy/functional/backends/tensorflow/sub_backends/tf_probability/elementwise.py
new file mode 100644
index 0000000000000..5af27f2aa7741
--- /dev/null
+++ b/ivy/functional/backends/tensorflow/sub_backends/tf_probability/elementwise.py
@@ -0,0 +1,15 @@
+from typing import Optional, Union
+import tensorflow_probability as tfp
+import tensorflow as tf
+
+
+def trapz(
+ y: Union[tf.Tensor, tf.Variable],
+ /,
+ *,
+ x: Optional[Union[tf.Tensor, tf.Variable]] = None,
+ dx: float = 1.0,
+ axis: int = -1,
+ out: Optional[Union[tf.Tensor, tf.Variable]] = None,
+) -> Union[tf.Tensor, tf.Variable]:
+ return tfp.math.trapz(y, x=x, dx=dx, axis=axis, name=None)
diff --git a/ivy/functional/backends/tensorflow/sub_backends/tf_probability/experimental/random.py b/ivy/functional/backends/tensorflow/sub_backends/tf_probability/experimental/random.py
new file mode 100644
index 0000000000000..75f4bd49eabd7
--- /dev/null
+++ b/ivy/functional/backends/tensorflow/sub_backends/tf_probability/experimental/random.py
@@ -0,0 +1,117 @@
+from ivy.func_wrapper import with_unsupported_dtypes
+from ivy.functional.ivy.random import (
+ _check_bounds_and_get_shape,
+ _check_shapes_broadcastable,
+)
+import tensorflow_probability as tfp
+from tensorflow_probability import distributions as tfd
+import tensorflow as tf
+from tensorflow.python.framework.dtypes import DType
+
+from typing import Optional, Sequence, Union
+from .... import backend_version
+import ivy
+
+
+def beta(
+ alpha: Union[float, tf.Tensor, tf.Variable],
+ beta: Union[float, tf.Tensor, tf.Variable],
+ /,
+ *,
+ shape: Optional[Union[ivy.NativeShape, Sequence[int]]] = None,
+ device: Optional[str] = None,
+ dtype: Optional[Union[ivy.Dtype]] = None,
+ seed: Optional[int] = None,
+ out: Optional[Union[tf.Tensor, tf.Variable]] = None,
+) -> Union[tf.Tensor, tf.Variable]:
+ if not dtype:
+ dtype = ivy.default_float_dtype()
+ dtype = ivy.as_native_dtype(dtype)
+ shape = _check_bounds_and_get_shape(alpha, beta, shape).shape
+ alpha = tf.cast(alpha, dtype)
+ beta = tf.cast(beta, dtype)
+ return tfp.distributions.Beta(alpha, beta).sample(shape, seed=seed)
+
+
+def gamma(
+ alpha: Union[float, tf.Tensor, tf.Variable],
+ beta: Union[float, tf.Tensor, tf.Variable],
+ /,
+ *,
+ shape: Optional[Union[ivy.NativeShape, Sequence[int]]] = None,
+ device: Optional[str] = None,
+ dtype: Optional[Union[DType, ivy.Dtype]] = None,
+ seed: Optional[int] = None,
+ out: Optional[Union[tf.Tensor, tf.Variable]] = None,
+) -> Union[tf.Tensor, tf.Variable]:
+ if not dtype:
+ dtype = ivy.default_float_dtype()
+ dtype = ivy.as_native_dtype(dtype)
+ shape = _check_bounds_and_get_shape(alpha, beta, shape).shape
+ alpha = tf.cast(alpha, dtype)
+ beta = tf.cast(beta, dtype)
+ return tfp.distributions.Gamma(alpha, beta).sample(shape, seed=seed)
+
+
+def bernoulli(
+ probs: Union[float, tf.Tensor, tf.Variable],
+ *,
+ logits: Union[float, tf.Tensor, tf.Variable] = None,
+ shape: Optional[Union[ivy.NativeShape, Sequence[int]]] = None,
+ device: str = None,
+ dtype: DType,
+ seed: Optional[int] = None,
+ out: Optional[Union[tf.Tensor, tf.Variable]] = None,
+) -> Union[tf.Tensor, tf.Variable]:
+ if seed is not None:
+ tf.random.set_seed(seed)
+ if logits is not None:
+ logits = tf.cast(logits, dtype)
+ if not _check_shapes_broadcastable(shape, logits.shape):
+ shape = logits.shape
+ elif probs is not None:
+ probs = tf.cast(probs, dtype)
+ if not _check_shapes_broadcastable(shape, probs.shape):
+ shape = probs.shape
+ return tfp.distributions.Bernoulli(
+ logits=logits, probs=probs, dtype=dtype, allow_nan_stats=True
+ ).sample(shape, seed)
+
+
+# dirichlet
+@with_unsupported_dtypes(
+ {
+ "2.14.0 and below": (
+ "blfoat16",
+ "float16",
+ )
+ },
+ backend_version,
+)
+def dirichlet(
+ alpha: Union[tf.Tensor, tf.Variable, float, Sequence[float]],
+ /,
+ *,
+ size: Optional[Union[ivy.NativeShape, Sequence[int]]] = None,
+ out: Optional[Union[tf.Tensor, tf.Variable]] = None,
+ seed: Optional[int] = None,
+ dtype: Optional[tf.Tensor] = None,
+) -> Union[tf.Tensor, tf.Variable]:
+ size = size if size is not None else len(alpha)
+
+ if dtype is None:
+ dtype = tf.float64
+ else:
+ dtype = dtype
+ if seed is not None:
+ tf.random.set_seed(seed)
+ return tf.cast(
+ tfd.Dirichlet(
+ concentration=alpha,
+ validate_args=False,
+ allow_nan_stats=True,
+ force_probs_to_zero_outside_support=False,
+ name="Dirichlet",
+ ).sample(size),
+ dtype=dtype,
+ )
diff --git a/ivy/functional/backends/tensorflow/sub_backends/tf_probability/experimental/statistical.py b/ivy/functional/backends/tensorflow/sub_backends/tf_probability/experimental/statistical.py
new file mode 100644
index 0000000000000..4b73e332dc85b
--- /dev/null
+++ b/ivy/functional/backends/tensorflow/sub_backends/tf_probability/experimental/statistical.py
@@ -0,0 +1,331 @@
+from typing import Optional, Sequence, Tuple, Union
+import ivy
+from ivy.func_wrapper import with_supported_dtypes
+from ivy.functional.backends.numpy.experimental.statistical import (
+ _handle_axis,
+ _quantile,
+ _validate_quantile,
+)
+import tensorflow_probability as tfp
+import tensorflow as tf
+from .... import backend_version
+
+
+def histogram(
+ a: tf.Tensor,
+ /,
+ *,
+ bins: Optional[Union[int, tf.Tensor]] = None,
+ axis: Optional[int] = None,
+ extend_lower_interval: Optional[bool] = False,
+ extend_upper_interval: Optional[bool] = False,
+ dtype: Optional[tf.DType] = None,
+ range: Optional[Tuple[float]] = None,
+ weights: Optional[tf.Tensor] = None,
+ density: Optional[bool] = False,
+ out: Optional[Union[tf.Tensor, tf.Variable]] = None,
+) -> Tuple[tf.Tensor]:
+ min_a = tf.reduce_min(a)
+ max_a = tf.reduce_max(a)
+ if isinstance(bins, tf.Tensor) and range:
+ raise ivy.exceptions.IvyException(
+ "Must choose between specifying bins and range or bin edges directly"
+ )
+ if range:
+ if isinstance(bins, int):
+ bins = tf.cast(
+ tf.linspace(start=range[0], stop=range[1], num=bins + 1), dtype=a.dtype
+ )
+ elif isinstance(bins, int):
+ range = (min_a, max_a)
+ bins = tf.cast(
+ tf.linspace(start=range[0], stop=range[1], num=bins + 1), dtype=a.dtype
+ )
+ if tf.shape(bins)[0] < 2:
+ raise ivy.exceptions.IvyException("bins must have at least 1 bin (size > 1)")
+ if min_a < bins[0] and not extend_lower_interval:
+ raise ivy.exceptions.IvyException(
+ "Values of x outside of the intervals cause errors in tensorflow backend. "
+ "Consider using extend_lower_interval to deal with this."
+ )
+ if max_a > bins[-1] and not extend_upper_interval:
+ raise ivy.exceptions.IvyException(
+ "Values of x outside of the intervals cause errors in tensorflow backend. "
+ "Consider using extend_upper_interval to deal with this."
+ )
+ ret = tfp.stats.histogram(
+ x=a,
+ edges=bins,
+ axis=axis,
+ weights=weights,
+ extend_lower_interval=extend_lower_interval,
+ extend_upper_interval=extend_upper_interval,
+ dtype=dtype,
+ name="histogram",
+ )
+ if density:
+ pass
+ # TODO: Tensorflow native dtype argument is not working
+ if dtype:
+ ret = tf.cast(ret, dtype)
+ bins = tf.cast(bins, dtype)
+ # TODO: weird error when returning bins: return ret, bins
+ return ret
+
+
+@with_supported_dtypes(
+ {
+ "2.14.0 and below": (
+ "float",
+ "complex",
+ )
+ },
+ backend_version,
+)
+def median(
+ input: Union[tf.Tensor, tf.Variable],
+ /,
+ *,
+ axis: Optional[Union[Tuple[int], int]] = None,
+ keepdims: bool = False,
+ out: Optional[Union[tf.Tensor, tf.Variable]] = None,
+) -> Union[tf.Tensor, tf.Variable]:
+ return tfp.stats.percentile(
+ input,
+ 50.0,
+ axis=axis,
+ interpolation="midpoint",
+ keepdims=keepdims,
+ )
+
+
+def nanmedian(
+ input: Union[tf.Tensor, tf.Variable],
+ /,
+ *,
+ axis: Optional[Union[Tuple[int], int]] = None,
+ keepdims: bool = False,
+ overwrite_input: bool = False,
+ out: Optional[Union[tf.Tensor, tf.Variable]] = None,
+) -> Union[tf.Tensor, tf.Variable]:
+ if overwrite_input:
+ copied_input = tf.identity(input)
+ return _nanmedian_helper(copied_input, axis, keepdims)
+
+ else:
+ result = _nanmedian_helper(input, axis, keepdims)
+ return result
+
+
+def _nanmedian_helper(input, axis=None, keepdims=False):
+ """
+ The approach to Handle Nans in single dimensional plus multi-dimensional inputs are
+ composed on two-parts.
+
+ PART 1: In this part, you have axis=None, it means we have to work on
+ flattened data, we don't need to work on different axis.there are two cases here
+
+ Case 1: which is if our input data does contain all the Nans or not,
+ if our input have just Nans (means no numbers) then we'll not use
+ temp[~tf.math.is_nan(temp)] function with our input because it will remove all Nans
+ and we get empty tensor and this raise an error when it sent to percentile function,
+ in this case we need to keep this input but just we flatten the input and percentile
+ function returns nan if it find nan in median and here all the input is nan then we
+ get our result.
+
+ Case 2: if we have a number (0.4, 0.3, 0. ,1., 2., .....) with nans then we use this
+ function temp[~tf.math.is_nan(temp)], it will return a tensor by extracting the nans
+ and just keeping the values, but remember the returned tensor will be flattened and
+ axis=None work on flattene inputs, so in this case we are also on same page :)
+
+ for example: [[12.0 ,4.0 ,ivy.nan], [ivy.nan, ivy.nan,2.2]] => returned:
+ [12.0 ,4.0, 2.2] now this will be our new input in percentile function.
+
+ PART 2: In this case you have to do more work because we now don't allow to work
+ directly on flattened data, Here are two cases also.
+
+ CASE 1: we need to consider axis parameter here, but percentile axis does work
+ differently and we don't have median function in tensorflow yet, so we need to make
+ our input data compatible to the axis, then we compute nanmedian along that specific
+ axis. we transpose the input data according to our axis, axis can be (0,), (1,),
+ (0,1), (0,1,2) and input can be multi-dimensional, so we need to take care of edge
+ cases before making it compatible.
+
+ CASE 2: Here the main Nan handling part comes, you can only use 1D inputs here so we
+ have to flatten the input then we have jump parameter which is use to say how many
+ iterations we want to make because we have to calculate the row-wise median along
+ axis=None now, so we slice out some data from the flattened input and then we use
+ that 1D Input to remove the nans and use it in our percentile.
+
+ For example: input = [[ivy.nan, 3, ivy.nan, 7],[4, ivy.nan,6, 9]], axis=1
+
+ flatten data -> [[nan 3. nan 7. 4. nan 6. 9.]]
+ num_jumps -> 2 because we have to slice out this in (1, 4) and (1,4),
+ then it works same as PART 1 CASE 1 AND CASE 2.
+ now for first slice we get -> 5.0 and for second we get -> 6.0, these calculated
+ along axis=1 now we append the data into result, so to make the shape of result
+ compatible with the numpy output, we reshaped it.
+
+ the result which we get from our _nanmedian_helper = [5., 6.]
+ """
+ dtype = input.dtype
+ temp = tf.cast(input, tf.float64)
+ num_dim = tf.rank(temp)
+ keepdim_shape = tf.shape(temp)
+ q = 50.0
+
+ # PART 1
+ if axis is None:
+ # PART 1 CASE 1
+ if tf.reduce_all(tf.math.is_nan(temp)):
+ temp = tf.reshape(temp, shape=(1, -1))
+ else:
+ # PART 1 CASE 2
+ temp = temp[~tf.math.is_nan(temp)]
+
+ ret = tfp.stats.percentile(
+ temp,
+ q,
+ axis=axis,
+ interpolation="midpoint",
+ keepdims=keepdims,
+ )
+ if dtype in [tf.int32, tf.int64, tf.float64]:
+ ret = tf.cast(ret, dtype=tf.float64)
+ elif dtype in [tf.float16, tf.bfloat16]:
+ ret = tf.cast(ret, dtype=tf.float16)
+ else:
+ ret = tf.cast(ret, dtype=tf.float32)
+ return ret
+
+ axis = [axis] if isinstance(axis, int) else list(axis)
+ # PART 2 CASE 1
+ for i in axis:
+ keepdim_shape = tf.tensor_scatter_nd_update(keepdim_shape, [[i]], [1])
+ axis = [num_dim + x if x < 0 else x for x in axis]
+ axis.sort()
+ dimension = tf.size(temp.shape)
+ while tf.size(axis) > 0:
+ axis1 = axis[0]
+ for axis2 in range(axis1 + 1, dimension):
+ temp = tf.transpose(
+ temp,
+ perm=tf.tensor_scatter_nd_update(
+ tf.range(tf.rank(temp)), [[axis1], [axis2]], [axis2, axis1]
+ ),
+ )
+ axis1 = axis2
+ axis = [x - 1 for x in axis]
+ axis.pop(0)
+ dimension = dimension - 1
+ temp = tf.reshape(
+ temp, shape=tf.concat([tf.shape(temp)[: (dimension - len(axis))], [-1]], axis=0)
+ )
+
+ tensor = tf.reshape(temp, shape=(1, -1))
+ shape = temp.shape
+ dim = temp.ndim
+ slice_size = shape[len(shape) - 1]
+ num_jumps = 1
+ result = []
+
+ if slice_size == 1:
+ if dim == 2 and input.shape[0] == 1:
+ return tensor
+ if dim > 2 and input.shape[0] == 1:
+ return tf.reshape(tensor, shape=input.shape)
+
+ tensor = tf.reshape(tensor, shape=shape[:-1])
+ return tensor
+ # PART 2 CASE 2
+ i = dim
+ while i > 1:
+ num_jumps *= shape[len(shape) - i]
+ i -= 1
+
+ for i in range(num_jumps):
+ start = i * slice_size
+ end = (i + 1) * slice_size
+ arr = tensor[:, start:end]
+ if tf.reduce_all(tf.math.is_nan(arr)):
+ arr = tf.reshape(arr, shape=(1, -1))
+ else:
+ arr = arr[~tf.math.is_nan(arr)]
+
+ ret = tfp.stats.percentile(
+ arr, q, axis=None, interpolation="midpoint", keepdims=keepdims
+ )
+ if keepdims:
+ ret = tf.squeeze(ret)
+
+ result.append(ret)
+
+ result = tf.reshape(result, shape=shape[:-1])
+
+ if keepdims:
+ keepdim_shape = tuple(keepdim_shape)
+ result = tf.reshape(result, shape=keepdim_shape)
+
+ if dtype in [tf.int32, tf.int64, tf.float64]:
+ result = tf.cast(result, dtype=tf.float64)
+ elif dtype in [tf.float16, tf.bfloat16]:
+ result = tf.cast(result, dtype=tf.float16)
+ else:
+ result = tf.cast(result, dtype=tf.float32)
+
+ return result
+
+
+def _compute_quantile_wrapper(
+ x,
+ q,
+ axis=None,
+ keepdims=False,
+ interpolation="linear",
+):
+ if not _validate_quantile(q):
+ raise ValueError("Quantiles must be in the range [0, 1]")
+ if interpolation in [
+ "linear",
+ "lower",
+ "higher",
+ "midpoint",
+ "nearest",
+ "nearest_jax",
+ ]:
+ if interpolation == "nearest_jax":
+ return _handle_axis(x, q, _quantile, keepdims=keepdims, axis=axis)
+ else:
+ axis = tuple(axis) if isinstance(axis, list) else axis
+
+ return tfp.stats.percentile(
+ x,
+ tf.math.multiply(q, 100),
+ axis=axis,
+ interpolation=interpolation,
+ keepdims=keepdims,
+ )
+ else:
+ raise ValueError(
+ "Interpolation must be 'linear', 'lower', 'higher', 'midpoint' or 'nearest'"
+ )
+
+
+def quantile(
+ a: Union[tf.Tensor, tf.Variable],
+ q: Union[tf.Tensor, float],
+ /,
+ *,
+ axis: Optional[Union[int, Sequence[int]]] = None,
+ interpolation: str = "linear",
+ keepdims: bool = False,
+ out: Optional[Union[tf.Tensor, tf.Variable]] = None,
+) -> Union[tf.Tensor, tf.Variable]:
+ # added the nearest_jax mode to enable jax-like calculations for method="nearest"
+ return _compute_quantile_wrapper(
+ a,
+ q,
+ axis=axis,
+ keepdims=keepdims,
+ interpolation=interpolation,
+ )
diff --git a/ivy/functional/backends/torch/__init__.py b/ivy/functional/backends/torch/__init__.py
index 279b5364a1384..986b1eace46f7 100644
--- a/ivy/functional/backends/torch/__init__.py
+++ b/ivy/functional/backends/torch/__init__.py
@@ -129,7 +129,7 @@ def rep_method(*args, **kwargs):
# update these to add new dtypes
valid_dtypes = {
- "2.0.1 and below": (
+ "2.1.0 and below": (
ivy.int8,
ivy.int16,
ivy.int32,
@@ -147,7 +147,7 @@ def rep_method(*args, **kwargs):
valid_numeric_dtypes = {
- "2.0.1 and below": (
+ "2.1.0 and below": (
ivy.int8,
ivy.int16,
ivy.int32,
@@ -163,13 +163,13 @@ def rep_method(*args, **kwargs):
}
valid_int_dtypes = {
- "2.0.1 and below": (ivy.int8, ivy.int16, ivy.int32, ivy.int64, ivy.uint8)
+ "2.1.0 and below": (ivy.int8, ivy.int16, ivy.int32, ivy.int64, ivy.uint8)
}
valid_float_dtypes = {
- "2.0.1 and below": (ivy.bfloat16, ivy.float16, ivy.float32, ivy.float64)
+ "2.1.0 and below": (ivy.bfloat16, ivy.float16, ivy.float32, ivy.float64)
}
-valid_uint_dtypes = {"2.0.1 and below": (ivy.uint8,)}
-valid_complex_dtypes = {"2.0.1 and below": (ivy.complex64, ivy.complex128)}
+valid_uint_dtypes = {"2.1.0 and below": (ivy.uint8,)}
+valid_complex_dtypes = {"2.1.0 and below": (ivy.complex64, ivy.complex128)}
# leave these untouched
valid_dtypes = _dtype_from_version(valid_dtypes, backend_version)
@@ -182,17 +182,17 @@ def rep_method(*args, **kwargs):
# invalid data types
# update these to add new dtypes
invalid_dtypes = {
- "2.0.1 and below": (
+ "2.1.0 and below": (
ivy.uint16,
ivy.uint32,
ivy.uint64,
)
}
-invalid_numeric_dtypes = {"2.0.1 and below": (ivy.uint16, ivy.uint32, ivy.uint64)}
-invalid_int_dtypes = {"2.0.1 and below": (ivy.uint16, ivy.uint32, ivy.uint64)}
-invalid_float_dtypes = {"2.0.1 and below": ()}
-invalid_uint_dtypes = {"2.0.1 and below": (ivy.uint16, ivy.uint32, ivy.uint64)}
-invalid_complex_dtypes = {"2.0.1 and below": ()}
+invalid_numeric_dtypes = {"2.1.0 and below": (ivy.uint16, ivy.uint32, ivy.uint64)}
+invalid_int_dtypes = {"2.1.0 and below": (ivy.uint16, ivy.uint32, ivy.uint64)}
+invalid_float_dtypes = {"2.1.0 and below": ()}
+invalid_uint_dtypes = {"2.1.0 and below": (ivy.uint16, ivy.uint32, ivy.uint64)}
+invalid_complex_dtypes = {"2.1.0 and below": ()}
invalid_dtypes = _dtype_from_version(invalid_dtypes, backend_version)
# leave these untouched
diff --git a/ivy/functional/backends/torch/activations.py b/ivy/functional/backends/torch/activations.py
index b863be601dfa2..dc3fe5e33a5b4 100644
--- a/ivy/functional/backends/torch/activations.py
+++ b/ivy/functional/backends/torch/activations.py
@@ -4,6 +4,7 @@
Collection of PyTorch activation functions, wrapped to fit Ivy syntax
and signature.
"""
+
from typing import Optional, Union, Literal
# global
@@ -18,14 +19,14 @@
import ivy.functional.backends.torch as torch_backend
-@with_unsupported_dtypes({"2.0.1 and below": ("float16",)}, backend_version)
+@with_unsupported_dtypes({"2.1.0 and below": ("float16",)}, backend_version)
def relu(
x: torch.Tensor, /, *, complex_mode="jax", out: Optional[torch.Tensor] = None
) -> torch.Tensor:
return torch.relu(x)
-@with_unsupported_dtypes({"2.0.1 and below": ("float16",)}, backend_version)
+@with_unsupported_dtypes({"2.1.0 and below": ("float16",)}, backend_version)
def leaky_relu(
x: torch.Tensor,
/,
@@ -37,7 +38,7 @@ def leaky_relu(
return torch.nn.functional.leaky_relu(x, alpha)
-@with_unsupported_dtypes({"2.0.1 and below": ("float16",)}, backend_version)
+@with_unsupported_dtypes({"2.1.0 and below": ("float16",)}, backend_version)
def gelu(
x: torch.Tensor,
/,
@@ -47,13 +48,11 @@ def gelu(
out: Optional[torch.Tensor] = None,
) -> torch.Tensor:
if approximate:
- return (
- 0.5 * x * (1 + torch.tanh(((2 / np.pi) ** 0.5) * (x + 0.044715 * x**3)))
- )
+ return 0.5 * x * (1 + torch.tanh(((2 / np.pi) ** 0.5) * (x + 0.044715 * x**3)))
return torch.nn.functional.gelu(x)
-@with_unsupported_dtypes({"2.0.1 and below": ("float16",)}, backend_version)
+@with_unsupported_dtypes({"2.1.0 and below": ("float16",)}, backend_version)
def sigmoid(
x: torch.Tensor, /, *, complex_mode="jax", out: Optional[torch.Tensor] = None
) -> torch.Tensor:
@@ -65,7 +64,7 @@ def sigmoid(
sigmoid.support_native_out = True
-@with_unsupported_dtypes({"2.0.1 and below": ("bfloat16", "float16")}, backend_version)
+@with_unsupported_dtypes({"2.1.0 and below": ("bfloat16", "float16")}, backend_version)
def softmax(
x: torch.Tensor,
/,
@@ -82,7 +81,7 @@ def softmax(
return torch.nn.functional.softmax(x, axis)
-@with_unsupported_dtypes({"2.0.1 and below": ("float16", "bfloat16")}, backend_version)
+@with_unsupported_dtypes({"2.1.0 and below": ("float16", "bfloat16")}, backend_version)
def softplus(
x: torch.Tensor,
/,
@@ -99,7 +98,7 @@ def softplus(
# Softsign
-@with_unsupported_dtypes({"2.0.1 and below": ("float16", "bfloat16")}, backend_version)
+@with_unsupported_dtypes({"2.1.0 and below": ("float16", "bfloat16")}, backend_version)
def softsign(x: torch.Tensor, /, out: Optional[torch.Tensor] = None) -> torch.Tensor:
# return x / (1 + torch.abs(x))
return torch.nn.functional.softsign(x)
@@ -109,7 +108,7 @@ def softsign(x: torch.Tensor, /, out: Optional[torch.Tensor] = None) -> torch.Te
@with_unsupported_dtypes(
- {"2.0.1 and below": ("float16",)},
+ {"2.1.0 and below": ("float16",)},
backend_version,
)
def log_softmax(
@@ -130,7 +129,7 @@ def log_softmax(
@with_unsupported_dtypes(
- {"2.0.1 and below": ("float16",)},
+ {"2.1.0 and below": ("float16",)},
backend_version,
)
def mish(
@@ -148,7 +147,7 @@ def mish(
@with_unsupported_dtypes(
{
- "2.0.1 and below": (
+ "2.1.0 and below": (
"complex",
"float16",
)
diff --git a/ivy/functional/backends/torch/creation.py b/ivy/functional/backends/torch/creation.py
index 9ded44d60defe..ea34478abeaa8 100644
--- a/ivy/functional/backends/torch/creation.py
+++ b/ivy/functional/backends/torch/creation.py
@@ -47,7 +47,7 @@ def _differentiable_linspace(start, stop, num, *, device, dtype=None):
return res
-@with_unsupported_dtypes({"2.0.1 and below": ("complex",)}, backend_version)
+@with_unsupported_dtypes({"2.1.0 and below": ("complex",)}, backend_version)
def arange(
start: float,
/,
@@ -95,7 +95,7 @@ def _stack_tensors(x, dtype):
return x
-@with_unsupported_dtypes({"2.0.1 and below": ("bfloat16",)}, backend_version)
+@with_unsupported_dtypes({"2.1.0 and below": ("bfloat16",)}, backend_version)
@_asarray_to_native_arrays_and_back
@_asarray_infer_device
@_asarray_handle_nestable
@@ -166,7 +166,7 @@ def empty_like(
return torch.empty_like(x, dtype=dtype, device=device)
-@with_unsupported_dtypes({"2.0.1 and below": ("bfloat16",)}, backend_version)
+@with_unsupported_dtypes({"2.1.0 and below": ("bfloat16",)}, backend_version)
def eye(
n_rows: int,
n_cols: Optional[int] = None,
@@ -276,7 +276,7 @@ def _slice_at_axis(sl, axis):
@with_unsupported_device_and_dtypes(
- {"2.0.1 and below": {"cpu": ("float16",)}}, backend_version
+ {"2.1.0 and below": {"cpu": ("float16",)}}, backend_version
)
def linspace(
start: Union[torch.Tensor, float],
@@ -477,7 +477,7 @@ def ones_like_v_0p1p12_to_0p2p0(
x[i] = 1
return x
for i in range(x.shape[0]):
- x[i, :] = ones_like_v_0p1p12_to_0p2p0(x[i, :])
+ x[i, :] = ones_like_v_0p1p12_to_0p2p0(x[i, :], dtype=dtype)
return x
@@ -607,3 +607,15 @@ def triu_indices(
row=n_rows, col=n_cols, offset=k, dtype=torch.int64, device=device
)
)
+
+
+def pad(tensor, sizes_of_pad, mode="constant", value=0):
+ if len(sizes_of_pad) == tensor.dim():
+ pad_pairs = []
+ for size in sizes_of_pad:
+ if size >= 0:
+ pad_pairs.append((size // 2, size - size // 2))
+ pad_pairs = pad_pairs[::-1]
+ pad_list = [item for pair in pad_pairs for item in pair]
+
+ return torch.nn.functional.pad(tensor, pad_list, mode, value)
diff --git a/ivy/functional/backends/torch/data_type.py b/ivy/functional/backends/torch/data_type.py
index 05b4ccb37a4c4..31265bff3577e 100644
--- a/ivy/functional/backends/torch/data_type.py
+++ b/ivy/functional/backends/torch/data_type.py
@@ -180,7 +180,7 @@ def as_ivy_dtype(
)
-@with_unsupported_dtypes({"2.0.1 and below": ("uint16",)}, backend_version)
+@with_unsupported_dtypes({"2.1.0 and below": ("uint16",)}, backend_version)
def as_native_dtype(
dtype_in: Union[torch.dtype, str, bool, int, float, np.dtype]
) -> torch.dtype:
@@ -196,7 +196,7 @@ def as_native_dtype(
dtype_in = dtype_in.name
if not isinstance(dtype_in, str):
return dtype_in
- if dtype_in in native_dtype_dict.keys():
+ if dtype_in in native_dtype_dict:
return native_dtype_dict[ivy.Dtype(dtype_in)]
else:
raise ivy.utils.exceptions.IvyException(
diff --git a/ivy/functional/backends/torch/device.py b/ivy/functional/backends/torch/device.py
index 4cf418af1b4fc..59d35e007d109 100644
--- a/ivy/functional/backends/torch/device.py
+++ b/ivy/functional/backends/torch/device.py
@@ -1,4 +1,5 @@
"""Collection of PyTorch general functions, wrapped to fit Ivy syntax and signature."""
+
import inspect
# global
diff --git a/ivy/functional/backends/torch/elementwise.py b/ivy/functional/backends/torch/elementwise.py
index 998fda4946cab..9fba80a8d5c8a 100644
--- a/ivy/functional/backends/torch/elementwise.py
+++ b/ivy/functional/backends/torch/elementwise.py
@@ -7,6 +7,7 @@
import ivy
from ivy.func_wrapper import (
with_unsupported_dtypes,
+ with_supported_dtypes,
handle_numpy_arrays_in_specific_backend,
)
from ivy import promote_types_of_inputs
@@ -37,7 +38,7 @@ def add(
add.support_native_out = True
-@with_unsupported_dtypes({"2.0.1 and below": ("complex",)}, backend_version)
+@with_unsupported_dtypes({"2.1.0 and below": ("complex",)}, backend_version)
@handle_numpy_arrays_in_specific_backend
def bitwise_xor(
x1: Union[int, bool, torch.Tensor],
@@ -53,21 +54,20 @@ def bitwise_xor(
bitwise_xor.support_native_out = True
+@with_supported_dtypes({"2.1.0 and below": ("complex",)}, backend_version)
def imag(
val: torch.Tensor,
/,
*,
out: Optional[torch.Tensor] = None,
) -> torch.Tensor:
- if val.dtype not in (torch.complex64, torch.complex128):
- return torch.zeros_like(val, dtype=val.dtype)
return torch.imag(val)
imag.support_native_out = False
-@with_unsupported_dtypes({"2.0.1 and below": ("float16", "complex")}, backend_version)
+@with_unsupported_dtypes({"2.1.0 and below": ("float16", "complex")}, backend_version)
@handle_numpy_arrays_in_specific_backend
def expm1(x: torch.Tensor, /, *, out: Optional[torch.Tensor] = None) -> torch.Tensor:
x = _cast_for_unary_op(x)
@@ -77,7 +77,7 @@ def expm1(x: torch.Tensor, /, *, out: Optional[torch.Tensor] = None) -> torch.Te
expm1.support_native_out = True
-@with_unsupported_dtypes({"2.0.1 and below": ("complex",)}, backend_version)
+@with_unsupported_dtypes({"2.1.0 and below": ("complex",)}, backend_version)
@handle_numpy_arrays_in_specific_backend
def bitwise_invert(
x: Union[int, bool, torch.Tensor], /, *, out: Optional[torch.Tensor] = None
@@ -129,7 +129,7 @@ def equal(
equal.support_native_out = True
-@with_unsupported_dtypes({"2.0.1 and below": ("complex",)}, backend_version)
+@with_unsupported_dtypes({"2.1.0 and below": ("complex",)}, backend_version)
@handle_numpy_arrays_in_specific_backend
def less_equal(
x1: Union[float, torch.Tensor],
@@ -145,7 +145,7 @@ def less_equal(
less_equal.support_native_out = True
-@with_unsupported_dtypes({"2.0.1 and below": ("complex",)}, backend_version)
+@with_unsupported_dtypes({"2.1.0 and below": ("complex",)}, backend_version)
@handle_numpy_arrays_in_specific_backend
def bitwise_and(
x1: Union[int, bool, torch.Tensor],
@@ -161,7 +161,7 @@ def bitwise_and(
bitwise_and.support_native_out = True
-@with_unsupported_dtypes({"2.0.1 and below": ("float16", "complex")}, backend_version)
+@with_unsupported_dtypes({"2.1.0 and below": ("float16", "complex")}, backend_version)
@handle_numpy_arrays_in_specific_backend
def ceil(x: torch.Tensor, /, *, out: Optional[torch.Tensor] = None) -> torch.Tensor:
x = _cast_for_unary_op(x)
@@ -175,7 +175,7 @@ def ceil(x: torch.Tensor, /, *, out: Optional[torch.Tensor] = None) -> torch.Ten
ceil.support_native_out = True
-@with_unsupported_dtypes({"2.0.1 and below": ("float16", "complex")}, backend_version)
+@with_unsupported_dtypes({"2.1.0 and below": ("float16", "complex")}, backend_version)
@handle_numpy_arrays_in_specific_backend
def floor(x: torch.Tensor, /, *, out: Optional[torch.Tensor] = None) -> torch.Tensor:
x = _cast_for_unary_op(x)
@@ -189,7 +189,7 @@ def floor(x: torch.Tensor, /, *, out: Optional[torch.Tensor] = None) -> torch.Te
floor.support_native_out = True
-@with_unsupported_dtypes({"2.0.1 and below": ("complex",)}, backend_version)
+@with_unsupported_dtypes({"2.1.0 and below": ("complex",)}, backend_version)
def fmin(
x1: torch.Tensor,
x2: torch.Tensor,
@@ -203,7 +203,7 @@ def fmin(
fmin.support_native_out = True
-@with_unsupported_dtypes({"2.0.1 and below": ("float16",)}, backend_version)
+@with_unsupported_dtypes({"2.1.0 and below": ("float16",)}, backend_version)
@handle_numpy_arrays_in_specific_backend
def asin(x: torch.Tensor, /, *, out: Optional[torch.Tensor] = None) -> torch.Tensor:
x = _cast_for_unary_op(x)
@@ -213,7 +213,7 @@ def asin(x: torch.Tensor, /, *, out: Optional[torch.Tensor] = None) -> torch.Ten
asin.support_native_out = True
-@with_unsupported_dtypes({"2.0.1 and below": ("float16",)}, backend_version)
+@with_unsupported_dtypes({"2.1.0 and below": ("float16",)}, backend_version)
@handle_numpy_arrays_in_specific_backend
def asinh(x: torch.Tensor, /, *, out: Optional[torch.Tensor] = None) -> torch.Tensor:
x = _cast_for_unary_op(x)
@@ -223,7 +223,7 @@ def asinh(x: torch.Tensor, /, *, out: Optional[torch.Tensor] = None) -> torch.Te
asinh.support_native_out = True
-@with_unsupported_dtypes({"2.0.1 and below": ("float16",)}, backend_version)
+@with_unsupported_dtypes({"2.1.0 and below": ("float16",)}, backend_version)
@handle_numpy_arrays_in_specific_backend
def sign(
x: torch.Tensor,
@@ -245,7 +245,7 @@ def sign(
sign.support_native_out = True
-@with_unsupported_dtypes({"2.0.1 and below": ("float16",)}, backend_version)
+@with_unsupported_dtypes({"2.1.0 and below": ("float16",)}, backend_version)
@handle_numpy_arrays_in_specific_backend
def sqrt(x: torch.Tensor, /, *, out: Optional[torch.Tensor] = None) -> torch.Tensor:
x = _cast_for_unary_op(x)
@@ -255,7 +255,7 @@ def sqrt(x: torch.Tensor, /, *, out: Optional[torch.Tensor] = None) -> torch.Ten
sqrt.support_native_out = True
-@with_unsupported_dtypes({"2.0.1 and below": ("float16",)}, backend_version)
+@with_unsupported_dtypes({"2.1.0 and below": ("float16",)}, backend_version)
@handle_numpy_arrays_in_specific_backend
def cosh(x: torch.Tensor, /, *, out: Optional[torch.Tensor] = None) -> torch.Tensor:
x = _cast_for_unary_op(x)
@@ -265,7 +265,7 @@ def cosh(x: torch.Tensor, /, *, out: Optional[torch.Tensor] = None) -> torch.Ten
cosh.support_native_out = True
-@with_unsupported_dtypes({"2.0.1 and below": ("float16",)}, backend_version)
+@with_unsupported_dtypes({"2.1.0 and below": ("float16",)}, backend_version)
@handle_numpy_arrays_in_specific_backend
def log10(x: torch.Tensor, /, *, out: Optional[torch.Tensor] = None) -> torch.Tensor:
x = _cast_for_unary_op(x)
@@ -275,14 +275,14 @@ def log10(x: torch.Tensor, /, *, out: Optional[torch.Tensor] = None) -> torch.Te
log10.support_native_out = True
-@with_unsupported_dtypes({"2.0.1 and below": ("float16",)}, backend_version)
+@with_unsupported_dtypes({"2.1.0 and below": ("float16",)}, backend_version)
@handle_numpy_arrays_in_specific_backend
def log2(x: torch.Tensor, /, *, out: Optional[torch.Tensor] = None) -> torch.Tensor:
x = _cast_for_unary_op(x)
return torch.log2(x, out=out)
-@with_unsupported_dtypes({"2.0.1 and below": ("float16", "complex")}, backend_version)
+@with_unsupported_dtypes({"2.1.0 and below": ("float16", "complex")}, backend_version)
@handle_numpy_arrays_in_specific_backend
def log1p(x: torch.Tensor, /, *, out: Optional[torch.Tensor] = None) -> torch.Tensor:
x = _cast_for_unary_op(x)
@@ -298,7 +298,7 @@ def isnan(x: torch.Tensor, /, *, out: Optional[torch.Tensor] = None) -> torch.Te
return torch.isnan(x)
-@with_unsupported_dtypes({"2.0.1 and below": ("complex",)}, backend_version)
+@with_unsupported_dtypes({"2.1.0 and below": ("complex",)}, backend_version)
@handle_numpy_arrays_in_specific_backend
def less(
x1: Union[float, torch.Tensor],
@@ -329,7 +329,7 @@ def multiply(
multiply.support_native_out = True
-@with_unsupported_dtypes({"2.0.1 and below": ("float16",)}, backend_version)
+@with_unsupported_dtypes({"2.1.0 and below": ("float16",)}, backend_version)
@handle_numpy_arrays_in_specific_backend
def cos(x: torch.Tensor, /, *, out: Optional[torch.Tensor] = None) -> torch.Tensor:
x = _cast_for_unary_op(x)
@@ -370,7 +370,7 @@ def divide(
divide.support_native_out = True
-@with_unsupported_dtypes({"2.0.1 and below": ("complex",)}, backend_version)
+@with_unsupported_dtypes({"2.1.0 and below": ("complex",)}, backend_version)
@handle_numpy_arrays_in_specific_backend
def greater(
x1: Union[float, torch.Tensor],
@@ -386,7 +386,7 @@ def greater(
greater.support_native_out = True
-@with_unsupported_dtypes({"2.0.1 and below": ("complex",)}, backend_version)
+@with_unsupported_dtypes({"2.1.0 and below": ("complex",)}, backend_version)
@handle_numpy_arrays_in_specific_backend
def greater_equal(
x1: Union[float, torch.Tensor],
@@ -402,7 +402,7 @@ def greater_equal(
greater_equal.support_native_out = True
-@with_unsupported_dtypes({"2.0.1 and below": ("float16",)}, backend_version)
+@with_unsupported_dtypes({"2.1.0 and below": ("float16",)}, backend_version)
@handle_numpy_arrays_in_specific_backend
def acos(x: torch.Tensor, /, *, out: Optional[torch.Tensor] = None) -> torch.Tensor:
x = _cast_for_unary_op(x)
@@ -412,7 +412,7 @@ def acos(x: torch.Tensor, /, *, out: Optional[torch.Tensor] = None) -> torch.Ten
acos.support_native_out = True
-@with_unsupported_dtypes({"2.0.1 and below": ("float",)}, backend_version)
+@with_unsupported_dtypes({"2.1.0 and below": ("float",)}, backend_version)
@handle_numpy_arrays_in_specific_backend
def lcm(
x1: torch.Tensor,
@@ -458,7 +458,7 @@ def logical_or(
logical_or.support_native_out = True
-@with_unsupported_dtypes({"2.0.1 and below": ("float16",)}, backend_version)
+@with_unsupported_dtypes({"2.1.0 and below": ("float16",)}, backend_version)
@handle_numpy_arrays_in_specific_backend
def acosh(x: torch.Tensor, /, *, out: Optional[torch.Tensor] = None) -> torch.Tensor:
x = _cast_for_unary_op(x)
@@ -468,7 +468,7 @@ def acosh(x: torch.Tensor, /, *, out: Optional[torch.Tensor] = None) -> torch.Te
acosh.support_native_out = True
-@with_unsupported_dtypes({"2.0.1 and below": ("float16",)}, backend_version)
+@with_unsupported_dtypes({"2.1.0 and below": ("float16",)}, backend_version)
@handle_numpy_arrays_in_specific_backend
def sin(x: torch.Tensor, /, *, out: Optional[torch.Tensor] = None) -> torch.Tensor:
x = _cast_for_unary_op(x)
@@ -504,7 +504,7 @@ def not_equal(
not_equal.support_native_out = True
-@with_unsupported_dtypes({"2.0.1 and below": ("float16",)}, backend_version)
+@with_unsupported_dtypes({"2.1.0 and below": ("float16",)}, backend_version)
@handle_numpy_arrays_in_specific_backend
def tanh(
x: torch.Tensor, /, *, complex_mode="jax", out: Optional[torch.Tensor] = None
@@ -516,7 +516,7 @@ def tanh(
tanh.support_native_out = True
-@with_unsupported_dtypes({"2.0.1 and below": ("float16", "complex")}, backend_version)
+@with_unsupported_dtypes({"2.1.0 and below": ("float16", "complex")}, backend_version)
@handle_numpy_arrays_in_specific_backend
def floor_divide(
x1: Union[float, torch.Tensor],
@@ -537,7 +537,7 @@ def floor_divide(
floor_divide.support_native_out = True
-@with_unsupported_dtypes({"2.0.1 and below": ("complex",)}, backend_version)
+@with_unsupported_dtypes({"2.1.0 and below": ("complex",)}, backend_version)
@handle_numpy_arrays_in_specific_backend
def bitwise_or(
x1: Union[int, bool, torch.Tensor],
@@ -553,7 +553,7 @@ def bitwise_or(
bitwise_or.support_native_out = True
-@with_unsupported_dtypes({"2.0.1 and below": ("float16",)}, backend_version)
+@with_unsupported_dtypes({"2.1.0 and below": ("float16",)}, backend_version)
@handle_numpy_arrays_in_specific_backend
def sinh(x: torch.Tensor, /, *, out: Optional[torch.Tensor] = None) -> torch.Tensor:
x = _cast_for_unary_op(x)
@@ -618,7 +618,7 @@ def pow(
pow.support_native_out = True
-@with_unsupported_dtypes({"2.0.1 and below": ("float16", "complex")}, backend_version)
+@with_unsupported_dtypes({"2.1.0 and below": ("float16", "complex")}, backend_version)
@handle_numpy_arrays_in_specific_backend
def round(
x: torch.Tensor, /, *, decimals: int = 0, out: Optional[torch.Tensor] = None
@@ -659,7 +659,7 @@ def trapz(
trapz.support_native_out = False
-@with_unsupported_dtypes({"2.0.1 and below": ("float16", "complex")}, backend_version)
+@with_unsupported_dtypes({"2.1.0 and below": ("float16", "complex")}, backend_version)
@handle_numpy_arrays_in_specific_backend
def trunc(x: torch.Tensor, /, *, out: Optional[torch.Tensor] = None) -> torch.Tensor:
x = _cast_for_unary_op(x)
@@ -692,7 +692,7 @@ def abs(
abs.support_native_out = True
-@with_unsupported_dtypes({"2.0.1 and below": ("float16", "complex")}, backend_version)
+@with_unsupported_dtypes({"2.1.0 and below": ("float16", "complex")}, backend_version)
@handle_numpy_arrays_in_specific_backend
def logaddexp(
x1: torch.Tensor, x2: torch.Tensor, /, *, out: Optional[torch.Tensor] = None
@@ -704,7 +704,7 @@ def logaddexp(
logaddexp.support_native_out = True
-@with_unsupported_dtypes({"2.0.1 and below": ("float16",)}, backend_version)
+@with_unsupported_dtypes({"2.1.0 and below": ("float16",)}, backend_version)
@handle_numpy_arrays_in_specific_backend
def logaddexp2(
x1: Union[torch.Tensor, float, list, tuple],
@@ -723,7 +723,7 @@ def logaddexp2(
logaddexp2.support_native_out = True
-@with_unsupported_dtypes({"2.0.1 and below": ("float16",)}, backend_version)
+@with_unsupported_dtypes({"2.1.0 and below": ("float16",)}, backend_version)
@handle_numpy_arrays_in_specific_backend
def tan(x: torch.Tensor, /, *, out: Optional[torch.Tensor] = None) -> torch.Tensor:
x = _cast_for_unary_op(x)
@@ -733,7 +733,7 @@ def tan(x: torch.Tensor, /, *, out: Optional[torch.Tensor] = None) -> torch.Tens
tan.support_native_out = True
-@with_unsupported_dtypes({"2.0.1 and below": ("float16",)}, backend_version)
+@with_unsupported_dtypes({"2.1.0 and below": ("float16",)}, backend_version)
@handle_numpy_arrays_in_specific_backend
def atan(x: torch.Tensor, /, *, out: Optional[torch.Tensor] = None) -> torch.Tensor:
x = _cast_for_unary_op(x)
@@ -744,7 +744,7 @@ def atan(x: torch.Tensor, /, *, out: Optional[torch.Tensor] = None) -> torch.Ten
@with_unsupported_dtypes(
- {"2.0.1 and below": ("float16", "bfloat16", "complex")}, backend_version
+ {"2.1.0 and below": ("float16", "bfloat16", "complex")}, backend_version
) # TODO Fixed in PyTorch 1.12.1 (this note excludes complex)
@handle_numpy_arrays_in_specific_backend
def atan2(
@@ -757,7 +757,7 @@ def atan2(
atan2.support_native_out = True
-@with_unsupported_dtypes({"2.0.1 and below": ("float16",)}, backend_version)
+@with_unsupported_dtypes({"2.1.0 and below": ("float16",)}, backend_version)
@handle_numpy_arrays_in_specific_backend
def log(x: torch.Tensor, /, *, out: Optional[torch.Tensor] = None) -> torch.Tensor:
x = _cast_for_unary_op(x)
@@ -767,7 +767,7 @@ def log(x: torch.Tensor, /, *, out: Optional[torch.Tensor] = None) -> torch.Tens
log.support_native_out = True
-@with_unsupported_dtypes({"2.0.1 and below": ("float16",)}, backend_version)
+@with_unsupported_dtypes({"2.1.0 and below": ("float16",)}, backend_version)
@handle_numpy_arrays_in_specific_backend
def exp(x: torch.Tensor, /, *, out: Optional[torch.Tensor] = None) -> torch.Tensor:
x = _cast_for_unary_op(x)
@@ -808,7 +808,7 @@ def subtract(
subtract.support_native_out = True
-@with_unsupported_dtypes({"2.0.1 and below": ("float16", "complex")}, backend_version)
+@with_unsupported_dtypes({"2.1.0 and below": ("float16", "complex")}, backend_version)
@handle_numpy_arrays_in_specific_backend
def remainder(
x1: Union[float, torch.Tensor],
@@ -836,7 +836,7 @@ def remainder(
remainder.support_native_out = True
-@with_unsupported_dtypes({"2.0.1 and below": ("float16",)}, backend_version)
+@with_unsupported_dtypes({"2.1.0 and below": ("float16",)}, backend_version)
@handle_numpy_arrays_in_specific_backend
def atanh(x: torch.Tensor, /, *, out: Optional[torch.Tensor] = None) -> torch.Tensor:
x = _cast_for_unary_op(x)
@@ -846,7 +846,7 @@ def atanh(x: torch.Tensor, /, *, out: Optional[torch.Tensor] = None) -> torch.Te
atanh.support_native_out = True
-@with_unsupported_dtypes({"2.0.1 and below": ("complex",)}, backend_version)
+@with_unsupported_dtypes({"2.1.0 and below": ("complex",)}, backend_version)
@handle_numpy_arrays_in_specific_backend
def bitwise_right_shift(
x1: Union[int, bool, torch.Tensor],
@@ -863,7 +863,7 @@ def bitwise_right_shift(
bitwise_right_shift.support_native_out = True
-@with_unsupported_dtypes({"2.0.1 and below": ("complex",)}, backend_version)
+@with_unsupported_dtypes({"2.1.0 and below": ("complex",)}, backend_version)
@handle_numpy_arrays_in_specific_backend
def bitwise_left_shift(
x1: Union[int, bool, torch.Tensor],
@@ -883,7 +883,7 @@ def bitwise_left_shift(
# ------#
-@with_unsupported_dtypes({"2.0.1 and below": ("float16", "complex")}, backend_version)
+@with_unsupported_dtypes({"2.1.0 and below": ("float16", "complex")}, backend_version)
@handle_numpy_arrays_in_specific_backend
def erf(x: torch.Tensor, /, *, out: Optional[torch.Tensor] = None) -> torch.Tensor:
x = _cast_for_unary_op(x)
@@ -893,7 +893,7 @@ def erf(x: torch.Tensor, /, *, out: Optional[torch.Tensor] = None) -> torch.Tens
erf.support_native_out = True
-@with_unsupported_dtypes({"2.0.1 and below": ("complex",)}, backend_version)
+@with_unsupported_dtypes({"2.1.0 and below": ("complex",)}, backend_version)
@handle_numpy_arrays_in_specific_backend
def minimum(
x1: Union[float, torch.Tensor],
@@ -912,7 +912,7 @@ def minimum(
minimum.support_native_out = True
-@with_unsupported_dtypes({"2.0.1 and below": ("complex",)}, backend_version)
+@with_unsupported_dtypes({"2.1.0 and below": ("complex",)}, backend_version)
@handle_numpy_arrays_in_specific_backend
def maximum(
x1: Union[float, torch.Tensor],
@@ -931,7 +931,7 @@ def maximum(
maximum.support_native_out = True
-@with_unsupported_dtypes({"2.0.1 and below": ("float16",)}, backend_version)
+@with_unsupported_dtypes({"2.1.0 and below": ("float16",)}, backend_version)
@handle_numpy_arrays_in_specific_backend
def reciprocal(
x: Union[float, torch.Tensor], /, *, out: Optional[torch.Tensor] = None
@@ -944,7 +944,7 @@ def reciprocal(
@with_unsupported_dtypes(
- {"2.0.1 and below": ("complex64", "complex128")}, backend_version
+ {"2.1.0 and below": ("complex64", "complex128")}, backend_version
)
@handle_numpy_arrays_in_specific_backend
def deg2rad(x: torch.Tensor, /, *, out: Optional[torch.Tensor] = None) -> torch.Tensor:
@@ -955,7 +955,7 @@ def deg2rad(x: torch.Tensor, /, *, out: Optional[torch.Tensor] = None) -> torch.
@with_unsupported_dtypes(
- {"2.0.1 and below": ("complex64", "complex128")}, backend_version
+ {"2.1.0 and below": ("complex64", "complex128")}, backend_version
)
@handle_numpy_arrays_in_specific_backend
def rad2deg(x: torch.Tensor, /, *, out: Optional[torch.Tensor] = None) -> torch.Tensor:
@@ -965,7 +965,7 @@ def rad2deg(x: torch.Tensor, /, *, out: Optional[torch.Tensor] = None) -> torch.
rad2deg.support_native_out = True
-@with_unsupported_dtypes({"2.0.1 and below": ("complex",)}, backend_version)
+@with_unsupported_dtypes({"2.1.0 and below": ("complex",)}, backend_version)
@handle_numpy_arrays_in_specific_backend
def trunc_divide(
x1: Union[float, torch.Tensor],
@@ -989,7 +989,7 @@ def isreal(x: torch.Tensor, /, *, out: Optional[torch.Tensor] = None) -> torch.T
@with_unsupported_dtypes(
- {"2.0.1 and below": ("bfloat16", "complex")},
+ {"2.1.0 and below": ("bfloat16", "complex")},
backend_version,
)
@handle_numpy_arrays_in_specific_backend
diff --git a/ivy/functional/backends/torch/experimental/activations.py b/ivy/functional/backends/torch/experimental/activations.py
index 734938f1fb279..a883a4f1fbbb7 100644
--- a/ivy/functional/backends/torch/experimental/activations.py
+++ b/ivy/functional/backends/torch/experimental/activations.py
@@ -10,7 +10,7 @@
from . import backend_version
-@with_unsupported_dtypes({"2.0.1 and below": ("float16",)}, backend_version)
+@with_unsupported_dtypes({"2.1.0 and below": ("float16",)}, backend_version)
def logit(
x: torch.Tensor,
/,
@@ -22,7 +22,7 @@ def logit(
return torch.logit(x, eps=eps, out=out)
-@with_unsupported_dtypes({"2.0.1 and below": ("complex", "float16")}, backend_version)
+@with_unsupported_dtypes({"2.1.0 and below": ("complex", "float16")}, backend_version)
def thresholded_relu(
x: torch.Tensor,
/,
@@ -33,14 +33,14 @@ def thresholded_relu(
return torch.threshold(x, threshold=threshold, value=0)
-@with_unsupported_dtypes({"2.0.1 and below": ("float16",)}, backend_version)
+@with_unsupported_dtypes({"2.1.0 and below": ("float16",)}, backend_version)
def relu6(
x: torch.Tensor, /, *, complex_mode="jax", out: Optional[torch.Tensor] = None
) -> torch.Tensor:
return torch.nn.functional.relu6(x)
-@with_unsupported_dtypes({"2.0.1 and below": ("float16",)}, backend_version)
+@with_unsupported_dtypes({"2.1.0 and below": ("float16",)}, backend_version)
def logsigmoid(
input: torch.Tensor, /, *, complex_mode="jax", out: Optional[torch.Tensor] = None
) -> torch.Tensor:
@@ -49,7 +49,7 @@ def logsigmoid(
return torch.nn.functional.logsigmoid(input)
-@with_unsupported_dtypes({"2.0.1 and below": ("float16",)}, backend_version)
+@with_unsupported_dtypes({"2.1.0 and below": ("float16",)}, backend_version)
def selu(x: torch.Tensor, /, *, out: Optional[torch.Tensor] = None) -> torch.Tensor:
ret = torch.nn.functional.selu(x)
if ivy.exists(out):
@@ -57,12 +57,12 @@ def selu(x: torch.Tensor, /, *, out: Optional[torch.Tensor] = None) -> torch.Ten
return ivy.astype(ret, x.dtype)
-@with_unsupported_dtypes({"2.0.1 and below": ("float16",)}, backend_version)
+@with_unsupported_dtypes({"2.1.0 and below": ("float16",)}, backend_version)
def silu(x: torch.Tensor, /, *, out: Optional[torch.Tensor] = None) -> torch.Tensor:
return torch.nn.functional.silu(x)
-@with_unsupported_dtypes({"2.0.1 and below": ("float16",)}, backend_version)
+@with_unsupported_dtypes({"2.1.0 and below": ("float16",)}, backend_version)
def elu(
x: torch.Tensor, /, *, alpha: float = 1.0, out: Optional[torch.Tensor] = None
) -> torch.Tensor:
@@ -72,7 +72,28 @@ def elu(
return ivy.astype(ret, x.dtype)
-@with_unsupported_dtypes({"2.0.1 and below": ("float16", "bfloat16")}, backend_version)
+@with_unsupported_dtypes(
+ {
+ "2.1.0 and below": (
+ "complex",
+ "float16",
+ "bfloat16",
+ )
+ },
+ backend_version,
+)
+def celu(
+ x: torch.Tensor,
+ /,
+ *,
+ alpha: float = 1.0,
+ complex_mode="jax",
+ out: Optional[torch.Tensor] = None,
+) -> torch.Tensor:
+ return torch.celu(x, alpha=alpha)
+
+
+@with_unsupported_dtypes({"2.1.0 and below": ("float16", "bfloat16")}, backend_version)
def hardtanh(
x: torch.Tensor,
/,
@@ -85,3 +106,59 @@ def hardtanh(
if ivy.exists(out):
return ivy.inplace_update(out, ret).astype(x.dtype)
return ivy.astype(ret, x.dtype)
+
+
+@with_unsupported_dtypes({"2.1.0 and below": ("float16",)}, backend_version)
+def tanhshrink(
+ x: torch.Tensor, /, *, out: Optional[torch.Tensor] = None
+) -> torch.Tensor:
+ ret = torch.nn.functional.tanhshrink(x)
+ if ivy.exists(out):
+ return ivy.inplace_update(out, ret).astype(x.dtype)
+ return ivy.astype(ret, x.dtype)
+
+
+@with_unsupported_dtypes({"2.1.0 and below": ("float16", "bfloat16")}, backend_version)
+def threshold(
+ x: torch.Tensor,
+ /,
+ *,
+ threshold: float,
+ value: float,
+ out: Optional[torch.Tensor] = None,
+) -> torch.Tensor:
+ ret = torch.nn.functional.threshold(threshold=threshold, value=value, input=x)
+ if ivy.exists(out):
+ return ivy.inplace_update(out, ret).astype(x.dtype)
+ return ivy.astype(ret, x.dtype)
+
+
+@with_unsupported_dtypes({"2.1.0 and below": ("float16", "bfloat16")}, backend_version)
+def softshrink(
+ x: torch.Tensor, /, *, lambd: float = 0.5, out: Optional[torch.Tensor] = None
+) -> torch.Tensor:
+ ret = torch.nn.functional.softshrink(x, lambd=lambd)
+ if ivy.exists(out):
+ return ivy.inplace_update(out, ret).astype(x.dtype)
+ return ivy.astype(ret, x.dtype)
+
+
+def scaled_tanh(
+ x: torch.Tensor,
+ /,
+ *,
+ alpha: float = 1.7159,
+ beta: float = 0.67,
+ out: Optional[torch.Tensor] = None,
+) -> torch.Tensor:
+ return alpha * torch.nn.functional.tanh(beta * x)
+
+
+@with_unsupported_dtypes({"2.1.0 and below": ("float16", "bfloat16")}, backend_version)
+def hardshrink(
+ x: torch.Tensor, /, *, lambd: float = 0.5, out: Optional[torch.Tensor] = None
+) -> torch.Tensor:
+ ret = torch.nn.functional.hardshrink(x, lambd=lambd)
+ if ivy.exists(out):
+ return ivy.inplace_update(out, ret).astype(x.dtype)
+ return ivy.astype(ret, x.dtype)
diff --git a/ivy/functional/backends/torch/experimental/creation.py b/ivy/functional/backends/torch/experimental/creation.py
index 17c619e449429..953970525fd66 100644
--- a/ivy/functional/backends/torch/experimental/creation.py
+++ b/ivy/functional/backends/torch/experimental/creation.py
@@ -20,7 +20,7 @@
@with_unsupported_device_and_dtypes(
- {"2.0.1 and below": {"cpu": ("float16",)}},
+ {"2.1.0 and below": {"cpu": ("float16",)}},
backend_version,
)
def kaiser_window(
@@ -87,7 +87,7 @@ def vorbis_window(
vorbis_window.support_native_out = False
-@with_unsupported_dtypes({"2.0.1 and below": ("float16",)}, backend_version)
+@with_unsupported_dtypes({"2.1.0 and below": ("float16",)}, backend_version)
def hann_window(
size: int,
/,
@@ -131,7 +131,7 @@ def unsorted_segment_min(
segment_ids: torch.Tensor,
num_segments: Union[int, torch.Tensor],
) -> torch.Tensor:
- ivy.utils.assertions.check_unsorted_segment_min_valid_params(
+ ivy.utils.assertions.check_unsorted_segment_valid_params(
data, segment_ids, num_segments
)
if data.dtype in [torch.float32, torch.float64, torch.float16, torch.bfloat16]:
@@ -152,7 +152,7 @@ def unsorted_segment_min(
return res
-@with_unsupported_dtypes({"2.0.1 and below": ("float16",)}, backend_version)
+@with_unsupported_dtypes({"2.1.0 and below": ("float16",)}, backend_version)
def blackman_window(
size: int,
/,
@@ -180,7 +180,7 @@ def unsorted_segment_sum(
# check should be same
# Might require to change the assertion function name to
# check_unsorted_segment_valid_params
- ivy.utils.assertions.check_unsorted_segment_min_valid_params(
+ ivy.utils.assertions.check_unsorted_segment_valid_params(
data, segment_ids, num_segments
)
@@ -238,10 +238,53 @@ def hz_to_mel(f):
)
# create overlapping frames of size 3
mel_edges = mel_edges.unfold(0, size=3, step=1)
- lower_edge_mel, center_mel, upper_edge_mel = (
+ lower_edge_mel, center_mel, upper_edge_mel = [
t.reshape((1, num_mel_bins)) for t in mel_edges.split(1, dim=1)
- )
+ ]
lower_slopes = (spec_bin_mels - lower_edge_mel) / (center_mel - lower_edge_mel)
upper_slopes = (upper_edge_mel - spec_bin_mels) / (upper_edge_mel - center_mel)
mel_weights = torch.maximum(zero, torch.minimum(lower_slopes, upper_slopes))
return torch.nn.functional.pad(mel_weights, (0, 0, 1, 0))
+
+
+def unsorted_segment_mean(
+ data: torch.Tensor,
+ segment_ids: torch.Tensor,
+ num_segments: Union[int, torch.Tensor],
+) -> torch.Tensor:
+ ivy.utils.assertions.check_unsorted_segment_valid_params(
+ data, segment_ids, num_segments
+ )
+
+ # Initialize an array to store the sum of elements for each segment
+ segment_sum = torch.zeros(
+ (num_segments,) + data.shape[1:], dtype=data.dtype, device=data.device
+ )
+
+ # Initialize an array to keep track of the number of elements in each segment
+ counts = torch.zeros(num_segments, dtype=torch.int64, device=data.device)
+
+ for i in range(len(segment_ids)):
+ seg_id = segment_ids[i]
+ segment_sum[seg_id] += data[i]
+ counts[seg_id] += 1
+
+ return segment_sum / counts[:, None]
+
+
+@with_unsupported_dtypes({"2.0.1 and below": "float16"}, backend_version)
+def polyval(
+ coeffs: torch.Tensor,
+ x: torch.Tensor,
+) -> torch.Tensor:
+ with ivy.PreciseMode(True):
+ promoted_type = ivy.promote_types(ivy.dtype(coeffs[0]), ivy.dtype(x[0]))
+ coeffs, x = ivy.promote_types_of_inputs(coeffs, x)
+ y = torch.zeros_like(x)
+ for coeff in coeffs:
+ y = y * x + coeff
+ if y.shape == (1,):
+ y = torch.unsqueeze(y, 0)
+ promoted_type = getattr(torch, promoted_type)
+ y = torch.tensor(y).to(dtype=promoted_type)
+ return y
diff --git a/ivy/functional/backends/torch/experimental/elementwise.py b/ivy/functional/backends/torch/experimental/elementwise.py
index e0b79c353113c..2c6fb41c8b1a5 100644
--- a/ivy/functional/backends/torch/experimental/elementwise.py
+++ b/ivy/functional/backends/torch/experimental/elementwise.py
@@ -1,5 +1,5 @@
# global
-from typing import Optional, Union, Tuple, List
+from typing import Optional, Union, Tuple, List, Sequence
from numbers import Number
import torch
@@ -14,12 +14,60 @@
from .. import backend_version
-@with_supported_dtypes({"2.0.1 and below": ("float32", "float64")}, backend_version)
+@with_unsupported_dtypes(
+ {
+ "2.1.0 and below": (
+ "complex64",
+ "complex128",
+ )
+ },
+ backend_version,
+)
+def amax(
+ x: torch.Tensor,
+ /,
+ *,
+ axis: Optional[Union[int, Sequence[int]]] = None,
+ keepdims: bool = False,
+ out: Optional[torch.Tensor] = None,
+) -> torch.Tensor:
+ axis = tuple(axis) if isinstance(axis, list) else axis
+ return torch.amax(x, dim=axis, keepdim=keepdims)
+
+
+amax.support_native_out = True
+
+
+@with_unsupported_dtypes(
+ {
+ "2.1.0 and below": (
+ "complex64",
+ "complex128",
+ )
+ },
+ backend_version,
+)
+def amin(
+ x: torch.Tensor,
+ /,
+ *,
+ axis: Optional[Union[int, Sequence[int]]] = None,
+ keepdims: bool = False,
+ out: Optional[torch.Tensor] = None,
+) -> torch.Tensor:
+ axis = tuple(axis) if isinstance(axis, list) else axis
+ return torch.amin(x, dim=axis, keepdim=keepdims)
+
+
+amin.support_native_out = True
+
+
+@with_supported_dtypes({"2.1.0 and below": ("float32", "float64")}, backend_version)
def lgamma(x: torch.Tensor, /, *, out: Optional[torch.Tensor] = None) -> torch.Tensor:
return torch.lgamma(x, out=out)
-@with_unsupported_dtypes({"2.0.1 and below": ("complex",)}, backend_version)
+@with_unsupported_dtypes({"2.1.0 and below": ("complex",)}, backend_version)
def fmax(
x1: torch.Tensor,
x2: torch.Tensor,
@@ -34,7 +82,7 @@ def fmax(
fmax.support_native_out = True
-@with_unsupported_dtypes({"2.0.1 and below": ("float16",)}, backend_version)
+@with_unsupported_dtypes({"2.1.0 and below": ("float16",)}, backend_version)
def sinc(x: torch.Tensor, /, *, out: Optional[torch.Tensor] = None) -> torch.Tensor:
x = _cast_for_unary_op(x)
return torch.sinc(x, out=out)
@@ -95,14 +143,14 @@ def count_nonzero(
return x
if isinstance(axis, int):
if axis == -1:
- temp = x.dim() - 2
+ temp = x.dim() - 1
if temp < -1:
temp = 0
return x.unsqueeze(temp)
- return x.unsqueeze(axis - 1)
+ return x.unsqueeze(axis)
elif axis is not None:
for d in sorted(axis):
- x = x.unsqueeze(d - 1)
+ x = x.unsqueeze(d)
return x
return x
@@ -110,7 +158,7 @@ def count_nonzero(
count_nonzero.support_native_out = False
-@with_unsupported_dtypes({"2.0.1 and below": ("complex",)}, backend_version)
+@with_unsupported_dtypes({"2.1.0 and below": ("complex",)}, backend_version)
def nansum(
x: torch.Tensor,
/,
@@ -179,7 +227,7 @@ def signbit(
signbit.support_native_out = True
-@with_unsupported_dtypes({"2.0.1 and below": ("float16",)}, backend_version)
+@with_unsupported_dtypes({"2.1.0 and below": ("float16",)}, backend_version)
def hypot(
x1: torch.Tensor,
x2: torch.Tensor,
@@ -204,7 +252,7 @@ def allclose(
return torch.tensor(ret)
-@with_unsupported_dtypes({"2.0.1 and below": ("float16",)}, backend_version)
+@with_unsupported_dtypes({"2.1.0 and below": ("float16",)}, backend_version)
def fix(
x: torch.Tensor,
/,
@@ -217,7 +265,7 @@ def fix(
fix.support_native_out = True
-@with_unsupported_dtypes({"2.0.1 and below": ("float16",)}, backend_version)
+@with_unsupported_dtypes({"2.1.0 and below": ("float16",)}, backend_version)
def nextafter(
x1: torch.Tensor,
x2: torch.Tensor,
@@ -271,7 +319,7 @@ def gradient(
@with_supported_dtypes(
- {"2.0.1 and below": ("float16", "float32", "float64")},
+ {"2.1.0 and below": ("float16", "float32", "float64")},
backend_version,
)
def xlogy(
@@ -334,7 +382,7 @@ def _are_suitable_types_for_torch_lerp(input, end, weight):
return True
-@with_unsupported_dtypes({"2.0.1 and below": ("float16", "bfloat16")}, backend_version)
+@with_unsupported_dtypes({"2.1.0 and below": ("float16", "bfloat16")}, backend_version)
def lerp(
input: torch.Tensor,
end: torch.Tensor,
@@ -372,7 +420,7 @@ def modf(
return torch.resolve_modf(input=modf_x)
-@with_unsupported_dtypes({"2.0.1 and below": ("float16",)}, backend_version)
+@with_unsupported_dtypes({"2.1.0 and below": ("float16",)}, backend_version)
def digamma(
x: torch.Tensor,
/,
@@ -385,7 +433,7 @@ def digamma(
digamma.support_native_out = True
-@with_unsupported_dtypes({"2.0.1 and below": ("float16",)}, backend_version)
+@with_unsupported_dtypes({"2.1.0 and below": ("float16",)}, backend_version)
def erfc(
x: torch.Tensor,
/,
diff --git a/ivy/functional/backends/torch/experimental/gradients.py b/ivy/functional/backends/torch/experimental/gradients.py
index 50bbe71ba0487..b6c0a7cedf6e4 100644
--- a/ivy/functional/backends/torch/experimental/gradients.py
+++ b/ivy/functional/backends/torch/experimental/gradients.py
@@ -1,9 +1,14 @@
# global
import torch
+from typing import Callable
# local
import ivy
from ivy.func_wrapper import inputs_to_native_arrays
+from ivy.functional.ivy.gradients import (
+ _flatten_containers,
+ _rebuild_flattened_containers,
+)
def bind_custom_gradient_function(func, custom_grad_fn):
@@ -25,3 +30,120 @@ def backward(ctx, upstream):
custom_module = _CustomModule.apply
return inputs_to_native_arrays(custom_module)
+
+
+def vjp(func: Callable, *primals):
+ flattened_primals, ret_idxs = _flatten_containers(primals)
+ unique_keys = list(
+ {
+ ivy.index_nest(ret_idxs, i)
+ for i in ivy.nested_argwhere(ret_idxs, lambda x: isinstance(x, str))
+ }
+ )
+
+ def grad_fn(*x_in):
+ ret, idxs = _flatten_containers(
+ ivy.to_native(
+ func(
+ *ivy.to_ivy(
+ _rebuild_flattened_containers(x_in, ret_idxs), nested=True
+ )
+ ),
+ nested=True,
+ include_derived=True,
+ )
+ )
+
+ # replave the idxs with the unique keys
+ func_ret_idxs = torch.tensor(
+ ivy.nested_map(
+ lambda x: (
+ unique_keys.index(x)
+ if isinstance(x, str)
+ else -1 if x is None else x
+ ),
+ idxs,
+ )
+ )
+
+ return (ret, func_ret_idxs)
+
+ primals_out, _vjpfun, func_ret_idxs = ivy.outputs_to_ivy_arrays(torch.func.vjp)(
+ grad_fn, *ivy.to_native(flattened_primals, nested=True), has_aux=True
+ )
+
+ func_ret_idxs = ivy.nested_map(
+ lambda x: unique_keys[x] if x >= 0 and x < len(unique_keys) else None,
+ func_ret_idxs.tolist(),
+ )
+ primals_out = _rebuild_flattened_containers(primals_out, func_ret_idxs)
+
+ def vjpfun(*x_in):
+ ivy.assertions.check_isinstance(x_in, tuple)
+ return _rebuild_flattened_containers(
+ ivy.to_ivy(
+ _vjpfun(ivy.to_native(_flatten_containers(x_in)[0], nested=True)),
+ nested=True,
+ include_derived=True,
+ ),
+ ret_idxs,
+ )
+
+ return (primals_out, vjpfun)
+
+
+def jvp(func: Callable, primals, tangents):
+ flattened_primals, ret_idxs = _flatten_containers(primals)
+ flattened_tangents, _ = _flatten_containers(tangents)
+ unique_keys = list(
+ {
+ ivy.index_nest(ret_idxs, i)
+ for i in ivy.nested_argwhere(ret_idxs, lambda x: isinstance(x, str))
+ }
+ )
+
+ def grad_fn(*x_in):
+ ret, idxs = _flatten_containers(
+ ivy.to_native(
+ func(
+ *ivy.to_ivy(
+ _rebuild_flattened_containers(x_in, ret_idxs), nested=True
+ )
+ ),
+ nested=True,
+ include_derived=True,
+ )
+ )
+
+ # replave the idxs with the unique keys
+ func_ret_idxs = torch.tensor(
+ ivy.nested_map(
+ lambda x: (
+ unique_keys.index(x)
+ if isinstance(x, str)
+ else -1 if x is None else x
+ ),
+ idxs,
+ )
+ )
+
+ return (ret, func_ret_idxs)
+
+ primals_out, tangents_out, func_ret_idxs = ivy.outputs_to_ivy_arrays(
+ torch.func.jvp
+ )(
+ grad_fn,
+ ivy.to_native(flattened_primals, nested=True),
+ ivy.to_native(flattened_tangents, nested=True),
+ has_aux=True,
+ )
+
+ func_ret_idxs = ivy.nested_map(
+ lambda x: unique_keys[x] if x >= 0 and x < len(unique_keys) else None,
+ func_ret_idxs.tolist(),
+ )
+
+ primals_out = _rebuild_flattened_containers(primals_out, func_ret_idxs)
+ tangents_out = _rebuild_flattened_containers(tangents_out, func_ret_idxs)
+
+ return (primals_out, tangents_out)
diff --git a/ivy/functional/backends/torch/experimental/layers.py b/ivy/functional/backends/torch/experimental/layers.py
index cc387c4d8896a..6fd6b8e7aac07 100644
--- a/ivy/functional/backends/torch/experimental/layers.py
+++ b/ivy/functional/backends/torch/experimental/layers.py
@@ -29,10 +29,10 @@ def _determine_depth_max_pooling(x, kernel, strides, dims, data_format="channel_
def _broadcast_pooling_helper(x, pool_dims: str = "2d", name: str = "padding"):
dims = {"1d": 1, "2d": 2, "3d": 3}
if isinstance(x, int):
- return tuple([x for _ in range(dims[pool_dims])])
+ return tuple(x for _ in range(dims[pool_dims]))
if len(x) == 1:
- return tuple([x[0] for _ in range(dims[pool_dims])])
+ return tuple(x[0] for _ in range(dims[pool_dims]))
elif len(x) == dims[pool_dims]:
return tuple(x)
@@ -44,7 +44,7 @@ def _broadcast_pooling_helper(x, pool_dims: str = "2d", name: str = "padding"):
)
-@with_unsupported_dtypes({"2.0.1 and below": ("bfloat16", "float16")}, backend_version)
+@with_unsupported_dtypes({"2.1.0 and below": ("bfloat16", "float16")}, backend_version)
def max_pool1d(
x: torch.Tensor,
kernel: Union[int, Tuple[int, ...]],
@@ -59,7 +59,7 @@ def max_pool1d(
) -> torch.Tensor:
dims = 1
kernel, strides, padding, dilation = _validate_max_pool_params(
- kernel, strides, padding, dilation, ceil_mode, dims=dims
+ kernel, strides, padding, dilation, ceil_mode, dims, data_format
)
if data_format == "NWC":
@@ -95,7 +95,7 @@ def max_pool1d(
)
else:
if isinstance(padding, list) and any(
- [item != 0 for sublist in padding for item in sublist]
+ item != 0 for sublist in padding for item in sublist
):
raise NotImplementedError(
"Nonzero explicit padding is not supported for depthwise max pooling"
@@ -112,7 +112,7 @@ def max_pool1d(
@with_unsupported_dtypes(
{
- "2.0.1 and below": (
+ "2.1.0 and below": (
"float16",
"bfloat16",
)
@@ -133,7 +133,7 @@ def max_pool2d(
) -> torch.Tensor:
dims = 2
kernel, strides, padding, dilation = _validate_max_pool_params(
- kernel, strides, padding, dilation, ceil_mode, dims=dims
+ kernel, strides, padding, dilation, ceil_mode, dims, data_format
)
if data_format == "NHWC":
@@ -177,7 +177,7 @@ def max_pool2d(
)
else:
if isinstance(padding, list) and any(
- [item != 0 for sublist in padding for item in sublist]
+ item != 0 for sublist in padding for item in sublist
):
raise NotImplementedError(
"Nonzero explicit padding is not supported for depthwise max pooling"
@@ -193,7 +193,7 @@ def max_pool2d(
@with_unsupported_dtypes(
{
- "2.0.1 and below": (
+ "2.1.0 and below": (
"float16",
"bfloat16",
)
@@ -214,7 +214,7 @@ def max_pool3d(
) -> torch.Tensor:
dims = 3
kernel, strides, padding, dilation = _validate_max_pool_params(
- kernel, strides, padding, dilation, ceil_mode, dims=dims
+ kernel, strides, padding, dilation, ceil_mode, dims, data_format
)
if data_format == "NDHWC":
@@ -268,7 +268,7 @@ def max_pool3d(
)
else:
if isinstance(padding, list) and any(
- [item != 0 for sublist in padding for item in sublist]
+ item != 0 for sublist in padding for item in sublist
):
raise NotImplementedError(
"Nonzero explicit padding is not supported for depthwise max pooling"
@@ -311,12 +311,12 @@ def _get_specific_pad(x_shape, kernel, strides, padding, dims):
return padding, pad_specific
-@with_unsupported_dtypes({"2.0.1 and below": ("bfloat16", "float16")}, backend_version)
+@with_unsupported_dtypes({"2.1.0 and below": ("bfloat16", "float16")}, backend_version)
def avg_pool1d(
x: torch.Tensor,
kernel: Union[int, Tuple[int]],
strides: Union[int, Tuple[int]],
- padding: str,
+ padding: Union[str, int, List[Tuple[int, int]]],
/,
*,
data_format: str = "NWC",
@@ -395,7 +395,7 @@ def _adjust_num_padded_values_to_ceil(
@with_unsupported_dtypes(
{
- "2.0.1 and below": (
+ "2.1.0 and below": (
"float16",
"bfloat16",
)
@@ -406,7 +406,7 @@ def avg_pool2d(
x: torch.Tensor,
kernel: Union[int, Tuple[int], Tuple[int, int]],
strides: Union[int, Tuple[int], Tuple[int, int]],
- padding: str,
+ padding: Union[str, int, List[Tuple[int, int]]],
/,
*,
data_format: str = "NHWC",
@@ -482,7 +482,7 @@ def avg_pool2d(
@with_unsupported_dtypes(
{
- "2.0.1 and below": (
+ "2.1.0 and below": (
"float16",
"bfloat16",
)
@@ -493,7 +493,7 @@ def avg_pool3d(
x: torch.Tensor,
kernel: Union[int, Tuple[int], Tuple[int, int, int]],
strides: Union[int, Tuple[int], Tuple[int, int, int]],
- padding: str,
+ padding: Union[str, int, List[Tuple[int, int]]],
/,
*,
data_format: str = "NDHWC",
@@ -568,7 +568,7 @@ def avg_pool3d(
return res
-@with_supported_dtypes({"2.0.1 and below": ("float32", "float64")}, backend_version)
+@with_supported_dtypes({"2.1.0 and below": ("float32", "float64")}, backend_version)
def dct(
x: torch.Tensor,
/,
@@ -681,7 +681,7 @@ def idct(
@with_unsupported_dtypes(
{
- "2.0.1 and below": (
+ "2.1.0 and below": (
"float16",
"bfloat16",
)
@@ -716,7 +716,7 @@ def fft(
raise ivy.utils.exceptions.IvyError(
f"Invalid data points {n}, expecting more than 1"
)
- if norm != "backward" and norm != "ortho" and norm != "forward":
+ if norm not in {"backward", "ortho", "forward"}:
raise ivy.utils.exceptions.IvyError(f"Unrecognized normalization mode {norm}")
if x.dtype in [torch.int64, torch.float64, torch.complex128]:
out_dtype = torch.complex128
@@ -727,7 +727,7 @@ def fft(
@with_unsupported_dtypes(
{
- "2.0.1 and below": (
+ "2.1.0 and below": (
"float16",
"bfloat16",
"complex",
@@ -749,7 +749,7 @@ def dropout(
) -> torch.Tensor:
x = ivy.astype(x, dtype) if dtype else x
res = torch.nn.functional.dropout(x, prob, training=training)
- res = torch.multiply(res, (1.0 - prob)) if not scale else res
+ res = res if scale else torch.multiply(res, (1.0 - prob))
return res
@@ -759,7 +759,7 @@ def dropout(
@with_unsupported_dtypes(
- {"2.0.1 and below": ("float16",)},
+ {"2.1.0 and below": ("float16",)},
backend_version,
)
def dropout1d(
@@ -782,7 +782,7 @@ def dropout1d(
@with_unsupported_dtypes(
- {"2.0.1 and below": ("float16",)},
+ {"2.1.0 and below": ("float16",)},
backend_version,
)
def dropout2d(
@@ -807,7 +807,7 @@ def dropout2d(
@with_unsupported_dtypes(
{
- "2.0.1 and below": (
+ "2.1.0 and below": (
"float16",
"bfloat16",
)
@@ -861,12 +861,12 @@ def ifft(
raise ivy.utils.exceptions.IvyError(
f"Invalid data points {n}, expecting more than 1"
)
- if norm != "backward" and norm != "ortho" and norm != "forward":
+ if norm not in {"backward", "ortho", "forward"}:
raise ivy.utils.exceptions.IvyError(f"Unrecognized normalization mode {norm}")
return torch.fft.ifft(x, n, dim, norm, out=out).resolve_conj()
-@with_unsupported_dtypes({"2.0.1 and below": ("complex",)}, backend_version)
+@with_unsupported_dtypes({"2.1.0 and below": ("complex",)}, backend_version)
def embedding(
weights: torch.Tensor,
indices: torch.Tensor,
@@ -893,10 +893,12 @@ def interpolate(
"linear",
"bilinear",
"trilinear",
+ "nd",
"nearest",
"area",
"nearest_exact",
"tf_area",
+ "tf_bicubic",
"bicubic",
"mitchellcubic",
"lanczos3",
@@ -905,10 +907,12 @@ def interpolate(
] = "linear",
scale_factor: Optional[Union[Sequence[int], int]] = None,
recompute_scale_factor: Optional[bool] = None,
- align_corners: Optional[bool] = None,
+ align_corners: bool = False,
antialias: bool = False,
out: Optional[torch.Tensor] = None,
):
+ if mode not in ["linear", "bilinear", "bicubic", "trilinear"]:
+ align_corners = None
return torch.nn.functional.interpolate(
x,
size=size,
@@ -920,35 +924,39 @@ def interpolate(
)
-interpolate.partial_mixed_handler = lambda *args, mode="linear", **kwargs: mode not in [
- "tf_area",
- "nd",
- "bicubic_tensorflow",
- "mitchellcubic",
- "lanczos3",
- "lanczos5",
- "gaussian",
-]
+interpolate.partial_mixed_handler = (
+ lambda *args, mode="linear", align_corners=False, **kwargs: mode
+ not in [
+ "tf_area",
+ "nd",
+ "tf_bicubic",
+ "mitchellcubic",
+ "lanczos3",
+ "lanczos5",
+ "gaussian",
+ ]
+ and (mode in ["linear", "bilinear", "bicubic", "trilinear"] or not align_corners)
+)
-@with_unsupported_dtypes({"2.0.1 and below": ("bfloat16", "float16")}, backend_version)
+@with_unsupported_dtypes({"2.1.0 and below": ("bfloat16", "float16")}, backend_version)
def adaptive_max_pool2d(
input: torch.Tensor, output_size: Union[Sequence[int], int]
) -> torch.Tensor:
return torch.nn.functional.adaptive_max_pool2d(input, output_size)
-@with_unsupported_dtypes({"2.0.1 and below": ("bfloat16", "float16")}, backend_version)
+@with_unsupported_dtypes({"2.1.0 and below": ("bfloat16", "float16")}, backend_version)
def adaptive_avg_pool1d(input, output_size):
return torch.nn.functional.adaptive_avg_pool1d(input, output_size)
-@with_unsupported_dtypes({"2.0.1 and below": ("bfloat16", "float16")}, backend_version)
+@with_unsupported_dtypes({"2.1.0 and below": ("bfloat16", "float16")}, backend_version)
def adaptive_avg_pool2d(input, output_size):
return torch.nn.functional.adaptive_avg_pool2d(input, output_size)
-@with_unsupported_dtypes({"2.0.1 and below": ("bfloat16", "float16")}, backend_version)
+@with_unsupported_dtypes({"2.1.0 and below": ("bfloat16", "float16")}, backend_version)
def fft2(
x: torch.Tensor,
*,
@@ -976,7 +984,7 @@ def fft2(
raise ivy.utils.exceptions.IvyError(
f"Invalid data points {s}, expecting s points larger than 1"
)
- if norm != "backward" and norm != "ortho" and norm != "forward":
+ if norm not in {"backward", "ortho", "forward"}:
raise ivy.utils.exceptions.IvyError(f"Unrecognized normalization mode {norm}")
return torch.tensor(
torch.fft.fft2(x, s, dim, norm, out=out), dtype=torch.complex128
@@ -994,7 +1002,27 @@ def ifftn(
return torch.fft.ifftn(x, s=s, dim=axes, norm=norm, out=out)
-@with_unsupported_dtypes({"2.0.1 and below": ("bfloat16", "float16")}, backend_version)
+def rfft(
+ x: torch.Tensor,
+ /,
+ *,
+ n: Optional[int] = None,
+ axis: int = -1,
+ norm: Literal["backward", "ortho", "forward"] = "backward",
+ out: Optional[torch.Tensor] = None,
+) -> torch.Tensor:
+ x = x.real
+ if x.dtype == torch.float16:
+ x = x.to(torch.float32)
+
+ ret = torch.fft.rfft(x, n=n, dim=axis, norm=norm)
+
+ if ivy.exists(out):
+ return ivy.inplace_update(out, ret)
+ return ret
+
+
+@with_unsupported_dtypes({"2.1.0 and below": ("bfloat16", "float16")}, backend_version)
def rfftn(
x: torch.Tensor,
s: Sequence[int] = None,
@@ -1022,7 +1050,7 @@ def rfftn(
raise ivy.utils.exceptions.IvyError(
f"Invalid data points {s}, expecting s points larger than 1"
)
- if norm != "backward" and norm != "ortho" and norm != "forward":
+ if norm not in {"backward", "ortho", "forward"}:
raise ivy.utils.exceptions.IvyError(f"Unrecognized normalization mode {norm}")
return torch.tensor(
torch.fft.rfftn(x, s, axes, norm=norm, out=out), dtype=torch.complex128
@@ -1032,7 +1060,7 @@ def rfftn(
# stft
@with_unsupported_dtypes(
{
- "2.0.1 and below": (
+ "2.1.0 and below": (
"float16",
"bfloat16",
)
diff --git a/ivy/functional/backends/torch/experimental/linear_algebra.py b/ivy/functional/backends/torch/experimental/linear_algebra.py
index 0d204e26676be..9ae68a74b6af5 100644
--- a/ivy/functional/backends/torch/experimental/linear_algebra.py
+++ b/ivy/functional/backends/torch/experimental/linear_algebra.py
@@ -12,7 +12,7 @@
from ivy.functional.ivy.experimental.linear_algebra import _check_valid_dimension_size
-@with_unsupported_dtypes({"2.0.1 and below": ("float16",)}, backend_version)
+@with_unsupported_dtypes({"2.1.0 and below": ("float16",)}, backend_version)
def diagflat(
x: torch.Tensor,
/,
@@ -155,7 +155,28 @@ def adjoint(
return torch.adjoint(x).resolve_conj()
-@with_unsupported_dtypes({"2.0.1 and below": ("float16",)}, backend_version)
+def solve_triangular(
+ x1: torch.Tensor,
+ x2: torch.Tensor,
+ /,
+ *,
+ upper: bool = True,
+ adjoint: bool = False,
+ unit_diagonal: bool = False,
+ out: Optional[torch.Tensor] = None,
+) -> torch.Tensor:
+ if adjoint:
+ x1 = torch.adjoint(x1)
+ upper = not upper
+ return torch.linalg.solve_triangular(
+ x1, x2, upper=upper, unitriangular=unit_diagonal, out=out
+ )
+
+
+solve_triangular.support_native_out = True
+
+
+@with_unsupported_dtypes({"2.1.0 and below": ("float16",)}, backend_version)
def multi_dot(
x: Sequence[torch.Tensor],
/,
diff --git a/ivy/functional/backends/torch/experimental/losses.py b/ivy/functional/backends/torch/experimental/losses.py
index 88efa250fdbf0..adb6d6e76510b 100644
--- a/ivy/functional/backends/torch/experimental/losses.py
+++ b/ivy/functional/backends/torch/experimental/losses.py
@@ -11,7 +11,7 @@
@with_unsupported_dtypes(
- {"2.0.1 and below": ("unit8", "int8", "int16", "int32", "int64", "bool")},
+ {"2.1.0 and below": ("unit8", "int8", "int16", "int32", "int64", "bool")},
backend_version,
)
def l1_loss(
@@ -30,7 +30,7 @@ def l1_loss(
@with_unsupported_dtypes(
{
- "2.0.1 and below": (
+ "2.1.0 and below": (
"complex",
"uint8",
"int8",
@@ -59,7 +59,7 @@ def smooth_l1_loss(
@with_unsupported_dtypes(
- {"2.0.1 and below": ("uint8", "int8", "int16", "int32", "int64", "bool")},
+ {"2.1.0 and below": ("uint8", "int8", "int16", "int32", "int64", "bool")},
backend_version,
)
def huber_loss(
@@ -77,7 +77,7 @@ def huber_loss(
@with_unsupported_dtypes(
{
- "2.0.1 and below": (
+ "2.1.0 and below": (
"float16",
"uint8",
"int8",
@@ -104,7 +104,7 @@ def soft_margin_loss(
@with_supported_dtypes(
- {"2.0.1 and below": ("float",)},
+ {"2.1.0 and below": ("float",)},
backend_version,
)
def kl_div(
@@ -124,7 +124,7 @@ def kl_div(
@with_supported_device_and_dtypes(
{
- "2.13.0 and below": {
+ "2.14.0 and below": {
"cpu": (
"float32",
"float64",
diff --git a/ivy/functional/backends/torch/experimental/manipulation.py b/ivy/functional/backends/torch/experimental/manipulation.py
index 68cc236c25009..589441629ccbd 100644
--- a/ivy/functional/backends/torch/experimental/manipulation.py
+++ b/ivy/functional/backends/torch/experimental/manipulation.py
@@ -14,6 +14,7 @@
from collections import namedtuple
import torch
+
# local
from ivy.func_wrapper import with_unsupported_dtypes, with_supported_dtypes
from .. import backend_version
@@ -58,7 +59,7 @@ def heaviside(
@with_supported_dtypes(
- {"2.0.1 and below": ("float32", "float64", "complex64", "complex128")},
+ {"2.1.0 and below": ("float32", "float64", "complex64", "complex128")},
backend_version,
)
def pad(
@@ -122,6 +123,13 @@ def pad(
def _check_torch_pad(mode, reflect_type, pad_width, input_shape, constant_values):
pad_width = _to_tf_padding(pad_width, len(input_shape))
+ if mode != "constant" and (
+ len(input_shape) > 4
+ or (len(input_shape) == 4 and len(pad_width) > 3)
+ or (len(input_shape) == 3 and len(pad_width) > 2)
+ or (len(input_shape) == 2 and len(pad_width) > 1)
+ ):
+ return False
return _check_paddle_pad(
mode, reflect_type, pad_width, input_shape, constant_values, 4
) and (
@@ -219,7 +227,7 @@ def fliplr(
fliplr.support_native_out = False
-@with_unsupported_dtypes({"2.0.1 and below": ("float16",)}, backend_version)
+@with_unsupported_dtypes({"2.1.0 and below": ("float16",)}, backend_version)
def i0(
x: torch.Tensor,
/,
@@ -312,7 +320,7 @@ def atleast_3d(
return transformed
-@with_unsupported_dtypes({"2.0.1 and below": ("float16", "bfloat16")}, backend_version)
+@with_unsupported_dtypes({"2.1.0 and below": ("float16", "bfloat16")}, backend_version)
def take_along_axis(
arr: torch.Tensor,
indices: torch.Tensor,
@@ -338,7 +346,7 @@ def take_along_axis(
if mode == "clip":
max_index = arr.shape[axis] - 1
indices = torch.clamp(indices, 0, max_index)
- elif mode == "fill" or mode == "drop":
+ elif mode in {"fill", "drop"}:
if "float" in str(arr.dtype) or "complex" in str(arr.dtype):
fill_value = float("nan")
elif "uint" in str(arr.dtype):
@@ -390,26 +398,7 @@ def expand(
expand.support_native_out = False
-def concat_from_sequence(
- input_sequence: Union[Tuple[torch.Tensor], List[torch.Tensor]],
- /,
- *,
- new_axis: int = 0,
- axis: int = 0,
- out: Optional[torch.Tensor] = None,
-) -> torch.Tensor:
- is_tuple = type(input_sequence) is tuple
- if is_tuple:
- input_sequence = list(input_sequence)
- if new_axis == 0:
- ret = torch.cat(input_sequence, dim=axis)
- return ret
- elif new_axis == 1:
- ret = torch.stack(input_sequence, dim=axis)
- return ret
-
-
-@with_unsupported_dtypes({"2.0.1 and below": ("complex", "float16")}, backend_version)
+@with_unsupported_dtypes({"2.1.0 and below": ("complex", "float16")}, backend_version)
def unique_consecutive(
x: torch.Tensor,
/,
@@ -439,7 +428,7 @@ def column_stack(
return torch.column_stack(arrays)
-@with_supported_dtypes({"2.0.1 and below": ("float32", "float64")}, backend_version)
+@with_supported_dtypes({"2.1.0 and below": ("float32", "float64")}, backend_version)
def put_along_axis(
arr: torch.Tensor,
indices: torch.Tensor,
@@ -473,3 +462,169 @@ def put_along_axis(
"max",
"min",
]
+
+
+def concat_from_sequence(
+ input_sequence: Union[Tuple[torch.Tensor], List[torch.Tensor]],
+ /,
+ *,
+ new_axis: int = 0,
+ axis: int = 0,
+ out: Optional[torch.Tensor] = None,
+) -> torch.Tensor:
+ is_tuple = type(input_sequence) is tuple
+ if is_tuple:
+ input_sequence = list(input_sequence)
+ if new_axis == 0:
+ ret = torch.cat(input_sequence, dim=axis)
+ return ret
+ elif new_axis == 1:
+ ret = torch.stack(input_sequence, dim=axis)
+ return ret
+
+
+def _take_with_axis(
+ x: torch.Tensor, indices: torch.Tensor, /, *, axis: int, mode: str
+) -> torch.Tensor:
+ # has no checks
+ # default behaviour is 'raise' like ON CPU
+ # additional check is recommended
+
+ x_shape = x.shape[axis]
+ if not ivy.exists(axis):
+ x = x.flatten()
+ x_shape = torch.prod(torch.tensor(x_shape))
+ else:
+ x_shape = x.shape[axis]
+
+ # wrap
+ if mode == "wrap":
+ indices = ((indices % x_shape) + x_shape) % x_shape
+ # clip
+ else:
+ indices = torch.clip(indices, 0, x_shape - 1)
+
+ rank = len(x.shape)
+ axis = ((axis % rank) + rank) % rank
+ slicer = ([slice(None)] * axis) + [indices]
+ slicer = tuple(slicer)
+
+ return x[slicer]
+
+
+def take(
+ x: Union[int, List, torch.Tensor],
+ indices: Union[int, List, torch.Tensor],
+ /,
+ *,
+ axis: Optional[int] = None,
+ mode: str = "clip",
+ fill_value: Optional[Number] = None,
+ out: Optional[torch.Tensor] = None,
+) -> torch.Tensor:
+ if mode not in ["raise", "wrap", "clip", "fill"]:
+ raise ValueError("mode must be one of 'clip', 'raise', 'wrap', or 'fill'")
+ if not isinstance(x, torch.Tensor):
+ x = torch.tensor(x)
+ if len(x.shape) == 0:
+ x = torch.tensor([x])
+ if not isinstance(indices, torch.Tensor):
+ indices = torch.tensor(indices)
+ if indices.dtype.is_floating_point:
+ indices = indices.to(torch.int64)
+
+ # raise
+ if mode == "raise":
+ mode = "clip"
+ if ivy.exists(axis):
+ try:
+ x_shape = x.shape[axis]
+ except Exception:
+ rank = len(x.shape)
+ raise IndexError(
+ "IndexError: Dimension out of range"
+ f"(expected to be in range of[-{rank}, {rank-1}]"
+ f", but got {axis})"
+ )
+ else:
+ x_shape = torch.prod(torch.tensor(x.shape))
+
+ bound_check = (indices < -x_shape) | (indices >= x_shape)
+ if torch.any(torch.tensor(bound_check)):
+ raise IndexError("index out of range in self")
+
+ # clip, wrap
+ if mode != "fill":
+ ret = _take_with_axis(x, indices, axis=axis, mode=mode)
+ if ivy.exists(out):
+ ivy.inplace_update(out, ret)
+ return ret
+
+ # fill
+ x_dtype = x.dtype
+ if fill_value is None:
+ # set according to jax behaviour
+ # https://tinyurl.com/66jn68uj
+ if x_dtype.is_floating_point or x_dtype.is_complex:
+ # NaN for inexact types
+ fill_value = float("NaN")
+ else:
+ if x_dtype == torch.bool:
+ # True for booleans
+ fill_value = True
+ elif str(x_dtype).split(".")[-1].startswith("u"):
+ # the largest positive value for unsigned types
+ fill_value = torch.iinfo(x_dtype).max
+ else:
+ # the largest negative value for signed types
+ fill_value = torch.iinfo(x_dtype).min
+
+ fill_value = torch.tensor(fill_value, dtype=x_dtype)
+ x_shape = x.shape
+ ret = _take_with_axis(x, indices, axis=axis, mode="wrap")
+
+ if len(ret.shape) == 0:
+ # if scalar (paddle scalar), scalar fill (replace)
+ if torch.any(torch.tensor(indices != 0)):
+ ret = fill_value
+ else:
+ if ivy.exists(axis):
+ rank = len(x.shape)
+ axis = ((axis % rank) + rank) % rank
+ x_shape = x_shape[axis]
+ else:
+ axis = 0
+ x_shape = torch.prod(x_shape)
+
+ bound_check = torch.tensor((indices < -x_shape) | (indices >= x_shape))
+
+ if torch.any(bound_check):
+ if axis > 0:
+ bound_check = torch.broadcast_to(
+ bound_check, (*x.shape[:axis], *bound_check.shape)
+ )
+ ret[bound_check] = fill_value
+
+ if ivy.exists(out):
+ ivy.inplace_update(out, ret)
+
+ return ret
+
+
+def trim_zeros(a: torch.Tensor, /, *, trim: Optional[str] = "bf") -> torch.Tensor:
+ first = 0
+ trim = trim.upper()
+ if "F" in trim:
+ for i in a:
+ if i != 0.0:
+ break
+ else:
+ first = first + 1
+ last = len(a)
+ if "B" in trim:
+ for i in torch.flip(a, [0]):
+ if i != 0.0:
+ break
+ else:
+ last = last - 1
+ return a[first:last]
diff --git a/ivy/functional/backends/torch/experimental/norms.py b/ivy/functional/backends/torch/experimental/norms.py
index 87de6064a2caf..97ec9ae1d02ab 100644
--- a/ivy/functional/backends/torch/experimental/norms.py
+++ b/ivy/functional/backends/torch/experimental/norms.py
@@ -18,7 +18,7 @@ def l1_normalize(
l1_normalize.support_native_out = True
-@with_unsupported_dtypes({"2.0.1 and below": ("float16",)}, backend_version)
+@with_unsupported_dtypes({"2.1.0 and below": ("float16",)}, backend_version)
def l2_normalize(
x: torch.Tensor,
/,
@@ -32,7 +32,7 @@ def l2_normalize(
l2_normalize.support_native_out = True
-@with_unsupported_dtypes({"2.0.1 and below": ("bfloat16", "float16")}, backend_version)
+@with_unsupported_dtypes({"2.1.0 and below": ("bfloat16", "float16")}, backend_version)
def batch_norm(
x: torch.Tensor,
mean: torch.Tensor,
@@ -50,8 +50,8 @@ def batch_norm(
xdims = x.ndim
if data_format == "NSC":
x = torch.permute(x, dims=(0, xdims - 1, *range(1, xdims - 1)))
- runningmean = mean.clone()
- runningvariance = variance.clone()
+ runningmean = mean.detach().clone()
+ runningvariance = variance.detach().clone()
xnormalized = torch.nn.functional.batch_norm(
x,
runningmean,
@@ -78,7 +78,7 @@ def batch_norm(
)
-@with_unsupported_dtypes({"2.0.1 and below": ("float16", "bfloat16")}, backend_version)
+@with_unsupported_dtypes({"2.1.0 and below": ("float16", "bfloat16")}, backend_version)
def instance_norm(
x: torch.Tensor,
mean: torch.Tensor,
@@ -126,7 +126,7 @@ def instance_norm(
)
-@with_unsupported_dtypes({"2.0.1 and below": ("float16", "bfloat16")}, backend_version)
+@with_unsupported_dtypes({"2.1.0 and below": ("float16", "bfloat16")}, backend_version)
def group_norm(
x: torch.Tensor,
num_groups: int = 1,
@@ -151,7 +151,7 @@ def group_norm(
return xnormalized
-@with_unsupported_dtypes({"2.0.1 and below": ("float16",)}, backend_version)
+@with_unsupported_dtypes({"2.1.0 and below": ("float16",)}, backend_version)
def lp_normalize(
x: torch.Tensor,
/,
diff --git a/ivy/functional/backends/torch/experimental/random.py b/ivy/functional/backends/torch/experimental/random.py
index 22b33f52001ca..7dc5f4b295913 100644
--- a/ivy/functional/backends/torch/experimental/random.py
+++ b/ivy/functional/backends/torch/experimental/random.py
@@ -13,7 +13,7 @@
# dirichlet
-@with_unsupported_dtypes({"2.0.1 and below": ("float16",)}, backend_version)
+@with_unsupported_dtypes({"2.1.0 and below": ("float16",)}, backend_version)
def dirichlet(
alpha: Union[torch.tensor, float, Sequence[float]],
/,
@@ -32,7 +32,7 @@ def dirichlet(
)
-@with_unsupported_dtypes({"2.0.1 and below": ("bfloat16",)}, backend_version)
+@with_unsupported_dtypes({"2.1.0 and below": ("bfloat16",)}, backend_version)
def beta(
alpha: Union[float, torch.Tensor],
beta: Union[float, torch.Tensor],
@@ -53,7 +53,7 @@ def beta(
return ret
-@with_unsupported_dtypes({"2.0.1 and below": ("bfloat16",)}, backend_version)
+@with_unsupported_dtypes({"2.1.0 and below": ("bfloat16",)}, backend_version)
def gamma(
alpha: Union[float, torch.Tensor],
beta: Union[float, torch.Tensor],
diff --git a/ivy/functional/backends/torch/experimental/sorting.py b/ivy/functional/backends/torch/experimental/sorting.py
index c6b7ee0f5bb06..b7af8ee599db1 100644
--- a/ivy/functional/backends/torch/experimental/sorting.py
+++ b/ivy/functional/backends/torch/experimental/sorting.py
@@ -33,7 +33,7 @@ def lexsort(
return torch.tensor([0])
_, result = torch.sort(keys[0], dim=axis, stable=True)
# result = torch.argsort(keys[0], dim=axis, stable=True)
- # only valid for torch > 2.0.1
+ # only valid for torch > 2.1.0
if shape[0] == 1:
return result
for i in range(1, shape[0]):
@@ -41,7 +41,7 @@ def lexsort(
ind = key[result]
_, temp = torch.sort(ind, dim=axis, stable=True)
# temp = torch.argsort(ind, dim=axis, stable=True)
- # only valid for torch > 2.0.1
+ # only valid for torch > 2.1.0
result = result[temp]
return result
diff --git a/ivy/functional/backends/torch/experimental/sparse_array.py b/ivy/functional/backends/torch/experimental/sparse_array.py
index 809ac1cd5f997..809913ac67828 100644
--- a/ivy/functional/backends/torch/experimental/sparse_array.py
+++ b/ivy/functional/backends/torch/experimental/sparse_array.py
@@ -102,13 +102,13 @@ def native_sparse_array_to_indices_values_and_shape(x):
if x.layout == torch.sparse_coo:
x = x.coalesce()
return {"coo_indices": x.indices()}, x.values(), x.size()
- elif x.layout == torch.sparse_csr or x.layout == torch.sparse_bsr:
+ elif x.layout in [torch.sparse_csr, torch.sparse_bsr]:
return (
{"crow_indices": x.crow_indices(), "col_indices": x.col_indices()},
x.values(),
x.size(),
)
- elif x.layout == torch.sparse_bsc or x.layout == torch.sparse_csc:
+ elif x.layout in [torch.sparse_bsc, torch.sparse_csc]:
return (
{"ccol_indices": x.crow_indices(), "row_indices": x.col_indices()},
x.values(),
diff --git a/ivy/functional/backends/torch/experimental/statistical.py b/ivy/functional/backends/torch/experimental/statistical.py
index cd390469f3a74..d9757720b8e53 100644
--- a/ivy/functional/backends/torch/experimental/statistical.py
+++ b/ivy/functional/backends/torch/experimental/statistical.py
@@ -12,7 +12,7 @@
@with_unsupported_dtypes(
{
- "2.0.1 and below": (
+ "2.1.0 and below": (
"uint8",
"int8",
"int16",
@@ -139,7 +139,7 @@ def histogram(
histogram.support_native_out = True
-@with_unsupported_dtypes({"2.0.1 and below": ("float16", "bool")}, backend_version)
+@with_unsupported_dtypes({"2.1.0 and below": ("float16", "bool")}, backend_version)
def median(
input: torch.Tensor,
/,
@@ -185,6 +185,31 @@ def nanmean(
nanmean.support_native_out = True
+def nanmin(
+ a: torch.Tensor,
+ /,
+ *,
+ axis: Optional[Union[int, Tuple[int]]] = None,
+ keepdims: Optional[bool] = False,
+ initial: Optional[Union[int, float, complex, ivy.Container]] = None,
+ where: Optional[torch.Tensor] = None,
+ out: Optional[torch.Tensor] = None,
+) -> torch.Tensor:
+ nan_mask = torch.isnan(a)
+ if where is not None:
+ nan_mask = torch.logical_or(nan_mask, torch.logical_not(where))
+ a_copy = a.clone()
+ a_copy[nan_mask] = float("inf")
+ if axis is None:
+ result, _ = a_copy.min(), None
+ else:
+ result, _ = a_copy.min(dim=axis, keepdim=keepdims)
+ if initial is not None:
+ initial = torch.tensor(initial)
+ result = torch.minimum(result, initial)
+ return result
+
+
def nanprod(
a: torch.Tensor,
/,
@@ -209,7 +234,7 @@ def nanprod(
return a.type(dtype)
if axis is None:
return torch.prod(input=a, out=out).type(dtype) * initial
- if isinstance(axis, tuple) or isinstance(axis, list):
+ if isinstance(axis, (tuple, list)):
for i in axis:
a = torch.prod(a, dim=i, keepdim=keepdims, out=out).type(dtype)
if a.dtype == torch.float16:
@@ -229,7 +254,7 @@ def _validate_quantile(q):
if not (0.0 <= q[i] <= 1.0):
return False
else:
- if not (torch.all(0 <= q) and torch.all(q <= 1)):
+ if not (torch.all(q >= 0) and torch.all(q <= 1)):
return False
return True
@@ -341,7 +366,7 @@ def _compute_quantile_wrapper(
)
-@with_unsupported_dtypes({"2.0.1 and below": ("bfloat16", "float16")}, backend_version)
+@with_unsupported_dtypes({"2.1.0 and below": ("bfloat16", "float16")}, backend_version)
def quantile(
a: torch.Tensor,
q: Union[torch.Tensor, float],
@@ -422,7 +447,7 @@ def _nanmedian(input, axis, keepdims):
return ret
-@with_unsupported_dtypes({"2.0.1 and below": ("bfloat16", "float16")}, backend_version)
+@with_unsupported_dtypes({"2.1.0 and below": ("bfloat16", "float16")}, backend_version)
def nanmedian(
input: torch.Tensor,
/,
@@ -510,7 +535,7 @@ def igamma(
igamma.support_native_out = True
-@with_unsupported_dtypes({"2.0.1 and below": ("float16", "bfloat16")}, backend_version)
+@with_unsupported_dtypes({"2.1.0 and below": ("float16", "bfloat16")}, backend_version)
def cov(
x1: torch.Tensor,
x2: torch.Tensor = None,
@@ -567,7 +592,7 @@ def cov(
@with_unsupported_dtypes(
- {"2.0.1 and below": ("float16",)},
+ {"2.1.0 and below": ("float16", "complex")},
backend_version,
)
def cummax(
@@ -580,9 +605,6 @@ def cummax(
dtype: Optional[torch.dtype] = None,
out: Optional[torch.Tensor] = None,
) -> Tuple[torch.Tensor, torch.Tensor]:
- if x.dtype in (torch.complex64, torch.complex128):
- x = x.real.to(dtype=torch.float64)
-
if exclusive or reverse:
if exclusive and reverse:
x1, x2 = torch.cummax(torch.flip(x, dims=(axis,)), axis)
@@ -607,7 +629,7 @@ def cummax(
@with_unsupported_dtypes(
{
- "2.0.1 and below": ("uint8", "float16", "bfloat16"),
+ "2.1.0 and below": ("uint8", "float16", "bfloat16"),
"1.12.1 and above": ("uint8", "float16"),
},
backend_version,
diff --git a/ivy/functional/backends/torch/general.py b/ivy/functional/backends/torch/general.py
index 7c4f729821123..41e1a3deb2b26 100644
--- a/ivy/functional/backends/torch/general.py
+++ b/ivy/functional/backends/torch/general.py
@@ -1,4 +1,5 @@
"""Collection of PyTorch general functions, wrapped to fit Ivy syntax and signature."""
+
# global
from functools import reduce as _reduce
from numbers import Number
@@ -22,14 +23,14 @@
def _parse_index(indices, ndims):
- ind = list()
+ ind = []
for so in indices:
- pre = list()
+ pre = []
for s in so:
if s == -1:
break
pre.append(s.item())
- post = list()
+ post = []
for s in reversed(so):
if s == -1:
break
@@ -52,7 +53,7 @@ def is_native_array(x, /, *, exclusive=False):
return False
-@with_unsupported_dtypes({"2.0.1 and below": ("complex", "bfloat16")}, backend_version)
+@with_unsupported_dtypes({"2.1.0 and below": ("complex", "bfloat16")}, backend_version)
def array_equal(x0: torch.Tensor, x1: torch.Tensor, /) -> bool:
x0, x1 = ivy.promote_types_of_inputs(x0, x1)
return torch.equal(x0, x1)
@@ -137,6 +138,9 @@ def to_numpy(
# ml_dtypes
# TODO: use torch's numpy() method once this feature is accepted
# https://github.com/pytorch/pytorch/issues/109873
+ if 0 in x.shape:
+ # this is necessary because tolist converts all empty shapes to (0,)
+ return np.empty(x.shape, dtype=ivy.as_ivy_dtype(x.dtype))
return np.array(x.tolist(), dtype=ivy.as_ivy_dtype(x.dtype))
else:
raise ivy.utils.exceptions.IvyException(
@@ -178,8 +182,8 @@ def gather(
batch_dims: int = 0,
out: Optional[torch.Tensor] = None,
) -> torch.Tensor:
- axis = axis % len(params.shape)
- batch_dims = batch_dims % len(params.shape)
+ axis %= len(params.shape)
+ batch_dims %= len(params.shape)
ivy.utils.assertions.check_gather_input_valid(params, indices, axis, batch_dims)
result = []
if batch_dims == 0:
@@ -244,7 +248,7 @@ def gather_nd(
out: Optional[torch.Tensor] = None,
) -> torch.Tensor:
ivy.utils.assertions.check_gather_nd_input_valid(params, indices, batch_dims)
- batch_dims = batch_dims % len(params.shape)
+ batch_dims %= len(params.shape)
result = []
if batch_dims == 0:
result = gather_nd_helper(params, indices)
@@ -347,7 +351,7 @@ def multiprocessing(context: Optional[str] = None):
@with_unsupported_dtypes(
{
- "2.0.1 and below": ("bfloat16",),
+ "2.1.0 and below": ("bfloat16",),
},
backend_version,
)
@@ -368,8 +372,8 @@ def scatter_flat(
dtype = updates.dtype
if reduction not in ["sum", "replace", "min", "max"]:
raise ivy.utils.exceptions.IvyException(
- "reduction is {}, but it must be one of "
- '"sum", "min", "max" or "replace"'.format(reduction)
+ f'reduction is {reduction}, but it must be one of "sum", "min", "max" or'
+ ' "replace"'
)
if target_given:
output = out
@@ -399,7 +403,7 @@ def scatter_flat(
@with_unsupported_dtypes(
{
- "2.0.1 and below": (
+ "2.1.0 and below": (
"float16",
"bfloat16",
)
@@ -449,8 +453,8 @@ def scatter_nd(
flat_result_size = _reduce(mul, shape, 1)
if reduction not in ["sum", "replace", "min", "max"]:
raise ivy.utils.exceptions.IvyException(
- "reduction is {}, but it must be one of "
- '"sum", "min", "max" or "replace"'.format(reduction)
+ f'reduction is {reduction}, but it must be one of "sum", "min", "max" or'
+ ' "replace"'
)
if target_given:
flat_output = torch.reshape(out, (flat_result_size,)).detach()
@@ -506,7 +510,7 @@ def shape(
return ivy.Shape(x.shape)
-@with_unsupported_dtypes({"2.0.1 and below": ("bfloat16",)}, backend_version)
+@with_unsupported_dtypes({"2.1.0 and below": ("bfloat16",)}, backend_version)
def vmap(
func: Callable,
in_axes: Union[int, Sequence[int], Sequence[None]] = 0,
@@ -525,7 +529,7 @@ def new_fun(*args):
@with_unsupported_dtypes(
- {"2.0.1 and below": ("bfloat16", "float16", "complex", "bool")}, backend_version
+ {"2.1.0 and below": ("bfloat16", "float16", "complex", "bool")}, backend_version
)
def isin(
elements: torch.tensor,
diff --git a/ivy/functional/backends/torch/gradients.py b/ivy/functional/backends/torch/gradients.py
index ee5c8441fc9c8..3434409fa0509 100644
--- a/ivy/functional/backends/torch/gradients.py
+++ b/ivy/functional/backends/torch/gradients.py
@@ -98,8 +98,8 @@ def execute_with_gradients(
/,
*,
retain_grads: bool = False,
- xs_grad_idxs: Optional[Sequence[Sequence[Union[str, int]]]] = [[0]],
- ret_grad_idxs: Optional[Sequence[Sequence[Union[str, int]]]] = [[0]],
+ xs_grad_idxs: Sequence[Sequence[Union[str, int]]] = ((0,),),
+ ret_grad_idxs: Sequence[Sequence[Union[str, int]]] = ((0,),),
):
# Conversion of required arrays to float variables and duplicate index chains
xs, xs_grad_idxs, xs1, required_duplicate_index_chains, _ = (
@@ -239,7 +239,7 @@ def _inner(*args, **kwargs):
# Avoid zero gradients setting requires_grads as False
if isinstance(y, tuple):
- y_ones = tuple([torch.ones_like(y_) for y_ in y])
+ y_ones = tuple(torch.ones_like(y_) for y_ in y)
[y_.requires_grad_() for y_ in y if y_.requires_grad is False]
elif y.requires_grad is False:
y.requires_grad_()
diff --git a/ivy/functional/backends/torch/layers.py b/ivy/functional/backends/torch/layers.py
index cf1c88d87a7c1..bb69a79915748 100644
--- a/ivy/functional/backends/torch/layers.py
+++ b/ivy/functional/backends/torch/layers.py
@@ -1,4 +1,5 @@
"""Collection of PyTorch network layers, wrapped to fit Ivy syntax and signature."""
+
from typing import Optional, Tuple, Union, Sequence
# global
@@ -8,11 +9,11 @@
import ivy
from ivy.func_wrapper import with_unsupported_dtypes, with_supported_dtypes
from . import backend_version
-from ivy.functional.ivy.layers import _handle_padding, _deconv_length
+from ivy.functional.ivy.layers import _get_embed_dim, _handle_padding, _deconv_length
@with_supported_dtypes(
- {"2.0.1 and below": ("float32", "float64", "complex")},
+ {"2.1.0 and below": ("float32", "float64", "complex")},
backend_version,
)
def multi_head_attention(
@@ -56,7 +57,7 @@ def multi_head_attention(
)[1]
num_dims = query.ndim
if num_dims == 3 and batch_first:
- query, key, value = (torch.swapaxes(x, 0, 1) for x in [query, key, value])
+ query, key, value = [torch.swapaxes(x, 0, 1) for x in [query, key, value]]
ret = torch.nn.functional.multi_head_attention_forward(
query,
key,
@@ -93,7 +94,7 @@ def multi_head_attention(
multi_head_attention.partial_mixed_handler = (
- lambda *args, scale=None, out_proj_weights=None, is_causal=False, attention_mask=None, return_attention_weights=False, in_proj_weights=None, q_proj_weights=None, k_proj_weights=None, v_proj_weights=None, **kwargs: not ivy.exists(
+ lambda *args, scale=None, out_proj_weights=None, is_causal=False, attention_mask=None, return_attention_weights=False, in_proj_weights=None, q_proj_weights=None, k_proj_weights=None, v_proj_weights=None, **kwargs: not ivy.exists( # noqa: E501
scale
)
and ivy.exists(out_proj_weights)
@@ -101,9 +102,7 @@ def multi_head_attention(
and (not is_causal or not return_attention_weights)
and (
ivy.exists(in_proj_weights)
- or all(
- [ivy.exists(x) for x in [q_proj_weights, k_proj_weights, v_proj_weights]]
- )
+ or all(ivy.exists(x) for x in [q_proj_weights, k_proj_weights, v_proj_weights])
)
and len(
set(
@@ -116,21 +115,8 @@ def multi_head_attention(
)
-def _get_embed_dim(
- in_proj_weights, q_proj_weights, k_proj_weights, v_proj_weights, query
-):
- pre_embed_dim = query.shape[-1]
- if ivy.exists(in_proj_weights):
- embed_dim = in_proj_weights.shape[0] / 3
- elif all([ivy.exists(x) for x in [q_proj_weights, k_proj_weights, v_proj_weights]]):
- embed_dim = q_proj_weights.shape[0]
- else:
- embed_dim = None
- return pre_embed_dim, embed_dim
-
-
@with_unsupported_dtypes(
- {"2.0.1 and below": ("float16", "bfloat16", "complex")},
+ {"2.1.0 and below": ("float16", "bfloat16", "complex")},
backend_version,
)
def linear(
@@ -245,7 +231,7 @@ def _pad_before_conv_tranpose(
@with_unsupported_dtypes(
- {"2.0.1 and below": ("float16", "bfloat16", "complex")},
+ {"2.1.0 and below": ("float16", "bfloat16", "complex")},
backend_version,
)
# noinspection PyUnresolvedReferences
@@ -277,7 +263,7 @@ def conv1d(
@with_unsupported_dtypes(
{
- "2.0.1 and below": (
+ "2.1.0 and below": (
"float16",
"bfloat16",
"complex",
@@ -324,7 +310,7 @@ def conv1d_transpose(
@with_unsupported_dtypes(
- {"2.0.1 and below": ("float16", "bfloat16", "complex")},
+ {"2.1.0 and below": ("float16", "bfloat16", "complex")},
backend_version,
)
# noinspection PyUnresolvedReferences
@@ -356,7 +342,7 @@ def conv2d(
@with_unsupported_dtypes(
{
- "2.0.1 and below": (
+ "2.1.0 and below": (
"float16",
"bfloat16",
"complex",
@@ -408,7 +394,7 @@ def conv2d_transpose(
@with_unsupported_dtypes(
{
- "2.0.1 and below": (
+ "2.1.0 and below": (
"float16",
"bfloat16",
"complex",
@@ -449,7 +435,7 @@ def depthwise_conv2d(
@with_unsupported_dtypes(
- {"2.0.1 and below": ("float16", "bfloat16", "complex")}, backend_version
+ {"2.1.0 and below": ("float16", "bfloat16", "complex")}, backend_version
)
# noinspection PyUnresolvedReferences
def conv3d(
@@ -479,7 +465,7 @@ def conv3d(
@with_unsupported_dtypes(
- {"2.0.1 and below": ("float16", "bfloat16", "complex")},
+ {"2.1.0 and below": ("float16", "bfloat16", "complex")},
backend_version,
)
# noinspection PyUnresolvedReferences
@@ -525,7 +511,7 @@ def conv3d_transpose(
@with_unsupported_dtypes(
- {"2.0.1 and below": ("float16", "bfloat16", "complex")},
+ {"2.1.0 and below": ("float16", "bfloat16", "complex")},
backend_version,
)
def conv_general_dilated(
@@ -588,7 +574,7 @@ def conv_general_dilated(
@with_unsupported_dtypes(
- {"2.0.1 and below": ("float16", "bfloat16", "complex")},
+ {"2.1.0 and below": ("float16", "bfloat16", "complex")},
backend_version,
)
def conv_general_transpose(
diff --git a/ivy/functional/backends/torch/linear_algebra.py b/ivy/functional/backends/torch/linear_algebra.py
index e7d5491add162..d01aad788a9a8 100644
--- a/ivy/functional/backends/torch/linear_algebra.py
+++ b/ivy/functional/backends/torch/linear_algebra.py
@@ -18,7 +18,7 @@
@with_unsupported_dtypes(
- {"2.0.1 and below": ("bfloat16", "float16", "complex")},
+ {"2.1.0 and below": ("bfloat16", "float16", "complex")},
backend_version,
)
def cholesky(
@@ -42,7 +42,7 @@ def cholesky(
cholesky.support_native_out = True
-@with_unsupported_dtypes({"2.0.1 and below": ("float16", "complex")}, backend_version)
+@with_unsupported_dtypes({"2.1.0 and below": ("float16", "complex")}, backend_version)
def cross(
x1: torch.Tensor,
x2: torch.Tensor,
@@ -70,7 +70,7 @@ def cross(
cross.support_native_out = True
-@with_unsupported_dtypes({"2.0.1 and below": ("float16", "bfloat16")}, backend_version)
+@with_unsupported_dtypes({"2.1.0 and below": ("float16", "bfloat16")}, backend_version)
def det(x: torch.Tensor, /, *, out: Optional[torch.Tensor] = None) -> torch.Tensor:
return torch.linalg.det(x, out=out)
@@ -90,7 +90,7 @@ def diagonal(
return torch.diagonal(x, offset=offset, dim1=axis1, dim2=axis2)
-@with_unsupported_dtypes({"2.0.1 and below": ("float16", "bfloat16")}, backend_version)
+@with_unsupported_dtypes({"2.1.0 and below": ("float16", "bfloat16")}, backend_version)
def eigh(
x: torch.Tensor, /, *, UPLO: str = "L", out: Optional[torch.Tensor] = None
) -> Tuple[torch.Tensor]:
@@ -104,7 +104,7 @@ def eigh(
eigh.support_native_out = True
-@with_unsupported_dtypes({"2.0.1 and below": ("float16", "bfloat16")}, backend_version)
+@with_unsupported_dtypes({"2.1.0 and below": ("float16", "bfloat16")}, backend_version)
def eigvalsh(
x: torch.Tensor, /, *, UPLO: str = "L", out: Optional[torch.Tensor] = None
) -> torch.Tensor:
@@ -114,7 +114,7 @@ def eigvalsh(
eigvalsh.support_native_out = True
-@with_unsupported_dtypes({"2.0.1 and below": ("float16",)}, backend_version)
+@with_unsupported_dtypes({"2.1.0 and below": ("float16",)}, backend_version)
def inner(
x1: torch.Tensor, x2: torch.Tensor, /, *, out: Optional[torch.Tensor] = None
) -> torch.Tensor:
@@ -134,7 +134,7 @@ def inner(
inner.support_native_out = True
-@with_unsupported_dtypes({"2.0.1 and below": ("float16", "bfloat16")}, backend_version)
+@with_unsupported_dtypes({"2.1.0 and below": ("float16", "bfloat16")}, backend_version)
def inv(
x: torch.Tensor,
/,
@@ -160,7 +160,7 @@ def inv(
@with_unsupported_dtypes(
- {"2.0.1 and below": ("float16", "bfloat16", "bool")}, backend_version
+ {"2.1.0 and below": ("float16", "bfloat16", "bool")}, backend_version
)
def matmul(
x1: torch.Tensor,
@@ -193,7 +193,7 @@ def matmul(
matmul.support_native_out = True
-@with_supported_dtypes({"2.0.1 and below": ("float", "complex")}, backend_version)
+@with_supported_dtypes({"2.1.0 and below": ("float", "complex")}, backend_version)
def matrix_norm(
x: torch.Tensor,
/,
@@ -209,7 +209,7 @@ def matrix_norm(
matrix_norm.support_native_out = True
-@with_unsupported_dtypes({"2.0.1 and below": ("float16", "bfloat16")}, backend_version)
+@with_unsupported_dtypes({"2.1.0 and below": ("float16", "bfloat16")}, backend_version)
def eig(
x: torch.Tensor, /, *, out: Optional[torch.Tensor] = None
) -> Tuple[torch.Tensor]:
@@ -223,7 +223,7 @@ def eig(
eig.support_native_out = True
-@with_unsupported_dtypes({"2.0.1 and below": ("float16", "bfloat16")}, backend_version)
+@with_unsupported_dtypes({"2.1.0 and below": ("float16", "bfloat16")}, backend_version)
def matrix_power(
x: torch.Tensor, n: int, /, *, out: Optional[torch.Tensor] = None
) -> torch.Tensor:
@@ -233,7 +233,7 @@ def matrix_power(
matrix_power.support_native_out = True
-@with_unsupported_dtypes({"2.0.1 and below": ("float16", "bfloat16")}, backend_version)
+@with_unsupported_dtypes({"2.1.0 and below": ("float16", "bfloat16")}, backend_version)
def matrix_rank(
x: torch.Tensor,
/,
@@ -281,7 +281,7 @@ def matrix_transpose(
return torch.swapaxes(x, -1, -2)
-@with_unsupported_dtypes({"2.0.1 and below": ("float16", "bfloat16")}, backend_version)
+@with_unsupported_dtypes({"2.1.0 and below": ("float16", "bfloat16")}, backend_version)
def outer(
x1: torch.Tensor,
x2: torch.Tensor,
@@ -296,7 +296,7 @@ def outer(
outer.support_native_out = True
-@with_unsupported_dtypes({"2.0.1 and below": ("float16", "bfloat16")}, backend_version)
+@with_unsupported_dtypes({"2.1.0 and below": ("float16", "bfloat16")}, backend_version)
def pinv(
x: torch.Tensor,
/,
@@ -312,7 +312,7 @@ def pinv(
pinv.support_native_out = True
-@with_unsupported_dtypes({"2.0.1 and below": ("float16", "bfloat16")}, backend_version)
+@with_unsupported_dtypes({"2.1.0 and below": ("float16", "bfloat16")}, backend_version)
def tensorsolve(
x1: torch.Tensor,
x2: torch.Tensor,
@@ -324,7 +324,7 @@ def tensorsolve(
return torch.linalg.tensorsolve(x1, x2, dims=axes)
-@with_unsupported_dtypes({"2.0.1 and below": ("float16", "bfloat16")}, backend_version)
+@with_unsupported_dtypes({"2.1.0 and below": ("float16", "bfloat16")}, backend_version)
def qr(
x: torch.Tensor,
/,
@@ -346,7 +346,7 @@ def qr(
return ret
-@with_unsupported_dtypes({"2.0.1 and below": ("float16", "bfloat16")}, backend_version)
+@with_unsupported_dtypes({"2.1.0 and below": ("float16", "bfloat16")}, backend_version)
def slogdet(
x: torch.Tensor,
/,
@@ -361,7 +361,7 @@ def slogdet(
slogdet.support_native_out = True
-@with_unsupported_dtypes({"2.0.1 and below": ("float16", "bfloat16")}, backend_version)
+@with_unsupported_dtypes({"2.1.0 and below": ("float16", "bfloat16")}, backend_version)
def solve(
x1: torch.Tensor,
x2: torch.Tensor,
@@ -397,7 +397,7 @@ def solve(
return ret
-@with_unsupported_dtypes({"2.0.1 and below": ("float16", "bfloat16")}, backend_version)
+@with_unsupported_dtypes({"2.1.0 and below": ("float16", "bfloat16")}, backend_version)
def svd(
x: torch.Tensor, /, *, full_matrices: bool = True, compute_uv: bool = True
) -> Union[torch.Tensor, Tuple[torch.Tensor, ...]]:
@@ -415,8 +415,14 @@ def svd(
@with_unsupported_dtypes({"2.0.1 and below": ("float16", "bfloat16")}, backend_version)
-def svdvals(x: torch.Tensor, /, *, out: Optional[torch.Tensor] = None) -> torch.Tensor:
- return torch.linalg.svdvals(x, out=out)
+def svdvals(
+ x: torch.Tensor,
+ /,
+ *,
+ driver: Optional[str] = None,
+ out: Optional[torch.Tensor] = None,
+) -> torch.Tensor:
+ return torch.linalg.svdvals(x, driver=driver, out=out)
svdvals.support_native_out = True
@@ -424,7 +430,7 @@ def svdvals(x: torch.Tensor, /, *, out: Optional[torch.Tensor] = None) -> torch.
# ToDo: re-add int32 support once
# (https://github.com/pytorch/pytorch/issues/84530) is fixed
-@with_supported_dtypes({"2.0.1 and below": ("float32",)}, backend_version)
+@with_supported_dtypes({"2.1.0 and below": ("float32",)}, backend_version)
def tensordot(
x1: torch.Tensor,
x2: torch.Tensor,
@@ -443,7 +449,7 @@ def tensordot(
return ret
-@with_unsupported_dtypes({"2.0.1 and below": ("float16", "bfloat16")}, backend_version)
+@with_unsupported_dtypes({"2.1.0 and below": ("float16", "bfloat16")}, backend_version)
def trace(
x: torch.Tensor,
/,
@@ -483,7 +489,7 @@ def vecdot(
vecdot.support_native_out = True
-@with_unsupported_dtypes({"2.0.1 and below": ("integer",)}, backend_version)
+@with_unsupported_dtypes({"2.1.0 and below": ("integer",)}, backend_version)
def vector_norm(
x: torch.Tensor,
/,
@@ -509,7 +515,7 @@ def vector_norm(
# ----- #
-@with_unsupported_dtypes({"2.0.1 and below": ("float16", "bfloat16")}, backend_version)
+@with_unsupported_dtypes({"2.1.0 and below": ("float16", "bfloat16")}, backend_version)
def diag(
x: torch.Tensor,
/,
@@ -520,7 +526,7 @@ def diag(
return torch.diag(x, diagonal=k)
-@with_unsupported_dtypes({"2.0.1 and below": ("float16", "bfloat16")}, backend_version)
+@with_unsupported_dtypes({"2.1.0 and below": ("float16", "bfloat16")}, backend_version)
def vander(
x: torch.tensor,
/,
@@ -546,7 +552,7 @@ def vander(
@with_unsupported_dtypes(
{
- "2.0.1 and below": (
+ "2.1.0 and below": (
"complex",
"unsigned",
)
diff --git a/ivy/functional/backends/torch/manipulation.py b/ivy/functional/backends/torch/manipulation.py
index 21e166e9def85..b1676faacc2f5 100644
--- a/ivy/functional/backends/torch/manipulation.py
+++ b/ivy/functional/backends/torch/manipulation.py
@@ -67,6 +67,8 @@ def flip(
axis: Optional[Union[int, Sequence[int]]] = None,
out: Optional[torch.Tensor] = None,
) -> torch.Tensor:
+ if copy:
+ x = x.clone().detach()
num_dims = len(x.shape)
if not num_dims:
return x
@@ -143,8 +145,8 @@ def squeeze(
if isinstance(axis, int):
if x.size(dim=axis) > 1:
raise ValueError(
- "Expected dimension of size [{}, {}], but found "
- "dimension size {}".format(-x.dim(), x.dim(), axis)
+ f"Expected dimension of size [{-x.dim()}, {x.dim()}], but found"
+ f" dimension size {axis}"
)
if x.shape[axis] != 1:
raise ivy.utils.exceptions.IvyException(
@@ -169,8 +171,8 @@ def squeeze(
shape = x.shape[i]
if shape > 1 and (shape < -dim or dim <= shape):
raise ValueError(
- "Expected dimension of size [{}, {}], "
- "but found dimension size {}".format(-dim, dim, shape)
+ f"Expected dimension of size [{-dim}, {dim}], but found dimension size"
+ f" {shape}"
)
else:
if copy:
@@ -211,9 +213,8 @@ def split(
if x.shape == ():
if num_or_size_splits is not None and num_or_size_splits != 1:
raise ivy.utils.exceptions.IvyException(
- "input array had no shape, but num_sections specified was {}".format(
- num_or_size_splits
- )
+ "input array had no shape, but num_sections specified was"
+ f" {num_or_size_splits}"
)
return [x]
dim_size: int = x.shape[axis]
@@ -245,7 +246,7 @@ def split(
@with_unsupported_dtypes(
- {"2.0.1 and below": ("int8", "int16", "uint8")}, backend_version
+ {"2.1.0 and below": ("int8", "int16", "uint8")}, backend_version
)
def repeat(
x: torch.Tensor,
@@ -284,7 +285,7 @@ def constant_pad(
x = x.unsqueeze(0)
if isinstance(pad_width, torch.Tensor):
pad_width = pad_width.detach().cpu().numpy().tolist()
- pad_width_flat: List[int] = list()
+ pad_width_flat: List[int] = []
for pad_width_sec in reversed(pad_width):
for item in pad_width_sec:
pad_width_flat.append(item)
@@ -314,7 +315,7 @@ def swapaxes(
@with_unsupported_dtypes(
- {"2.0.1 and below": ("bool", "float16", "complex")}, backend_version
+ {"2.1.0 and below": ("bool", "float16", "complex")}, backend_version
)
def clip(
x: torch.Tensor,
diff --git a/ivy/functional/backends/torch/norms.py b/ivy/functional/backends/torch/norms.py
index 2b1c24bd87a2b..704a2a4d506c0 100644
--- a/ivy/functional/backends/torch/norms.py
+++ b/ivy/functional/backends/torch/norms.py
@@ -5,7 +5,7 @@
from . import backend_version
-@with_unsupported_dtypes({"2.0.1 and below": ("bfloat16", "float16")}, backend_version)
+@with_unsupported_dtypes({"2.1.0 and below": ("bfloat16", "float16")}, backend_version)
def layer_norm(
x: torch.Tensor,
normalized_idxs: List[int],
diff --git a/ivy/functional/backends/torch/random.py b/ivy/functional/backends/torch/random.py
index e9c5267b54ef9..2fac63ffdd2af 100644
--- a/ivy/functional/backends/torch/random.py
+++ b/ivy/functional/backends/torch/random.py
@@ -62,7 +62,7 @@ def random_normal(
random_normal.support_native_out = True
-@with_unsupported_dtypes({"2.0.1 and below": ("bfloat16",)}, backend_version)
+@with_unsupported_dtypes({"2.1.0 and below": ("bfloat16",)}, backend_version)
def multinomial(
population_size: int,
num_samples: int,
diff --git a/ivy/functional/backends/torch/searching.py b/ivy/functional/backends/torch/searching.py
index b86ca5941b6db..05951657703f7 100644
--- a/ivy/functional/backends/torch/searching.py
+++ b/ivy/functional/backends/torch/searching.py
@@ -13,7 +13,7 @@
# ------------------ #
-@with_unsupported_dtypes({"2.0.1 and below": ("complex",)}, backend_version)
+@with_unsupported_dtypes({"2.1.0 and below": ("complex",)}, backend_version)
def argmax(
x: torch.Tensor,
/,
@@ -41,7 +41,7 @@ def argmax(
return ret
-@with_unsupported_dtypes({"2.0.1 and below": ("complex",)}, backend_version)
+@with_unsupported_dtypes({"2.1.0 and below": ("complex",)}, backend_version)
def argmin(
x: torch.Tensor,
/,
diff --git a/ivy/functional/backends/torch/set.py b/ivy/functional/backends/torch/set.py
index 76119bb23a939..5eb412bab77be 100644
--- a/ivy/functional/backends/torch/set.py
+++ b/ivy/functional/backends/torch/set.py
@@ -11,7 +11,7 @@
@with_unsupported_dtypes(
{
- "2.0.1 and below": ("complex", "float16"),
+ "2.1.0 and below": ("complex", "float16"),
},
backend_version,
)
@@ -84,7 +84,7 @@ def unique_all(
@with_unsupported_dtypes(
{
- "2.0.1 and below": ("float16",),
+ "2.1.0 and below": ("float16",),
},
backend_version,
)
@@ -98,13 +98,23 @@ def unique_counts(x: torch.Tensor, /) -> Tuple[torch.Tensor, torch.Tensor]:
@with_unsupported_dtypes(
{
- "2.0.1 and below": ("float16",),
+ "2.1.0 and below": ("float16",),
},
backend_version,
)
-def unique_inverse(x: torch.Tensor, /) -> Tuple[torch.Tensor, torch.Tensor]:
+def unique_inverse(
+ x: torch.Tensor,
+ /,
+ *,
+ axis: Optional[int] = None,
+) -> Tuple[torch.Tensor, torch.Tensor]:
Results = namedtuple("Results", ["values", "inverse_indices"])
- values, inverse_indices = torch.unique(x, return_inverse=True)
+
+ if axis is None:
+ x = torch.flatten(x)
+ axis = 0
+
+ values, inverse_indices = torch.unique(x, return_inverse=True, axis=axis)
nan_idx = torch.isnan(x)
if nan_idx.any():
inverse_indices[nan_idx] = torch.where(torch.isnan(values))[0][0]
@@ -114,7 +124,7 @@ def unique_inverse(x: torch.Tensor, /) -> Tuple[torch.Tensor, torch.Tensor]:
@with_unsupported_dtypes(
{
- "2.0.1 and below": ("float16", "complex"),
+ "2.1.0 and below": ("float16", "complex"),
},
backend_version,
)
diff --git a/ivy/functional/backends/torch/sorting.py b/ivy/functional/backends/torch/sorting.py
index 6fea3ca9794c3..fa9e1c85254ca 100644
--- a/ivy/functional/backends/torch/sorting.py
+++ b/ivy/functional/backends/torch/sorting.py
@@ -8,7 +8,7 @@
from . import backend_version
-@with_unsupported_dtypes({"2.0.1 and below": ("complex",)}, backend_version)
+@with_unsupported_dtypes({"2.1.0 and below": ("complex",)}, backend_version)
def argsort(
x: torch.Tensor,
/,
@@ -29,7 +29,7 @@ def argsort(
argsort.support_native_out = True
-@with_unsupported_dtypes({"2.0.1 and below": ("complex",)}, backend_version)
+@with_unsupported_dtypes({"2.1.0 and below": ("complex",)}, backend_version)
def sort(
x: torch.Tensor,
/,
@@ -51,7 +51,7 @@ def sort(
# msort
-@with_unsupported_dtypes({"2.0.1 and below": ("complex",)}, backend_version)
+@with_unsupported_dtypes({"2.1.0 and below": ("complex",)}, backend_version)
def msort(
a: Union[torch.Tensor, list, tuple], /, *, out: Optional[torch.Tensor] = None
) -> torch.Tensor:
@@ -61,7 +61,7 @@ def msort(
msort.support_native_out = True
-@with_unsupported_dtypes({"2.0.1 and below": ("complex",)}, backend_version)
+@with_unsupported_dtypes({"2.1.0 and below": ("complex",)}, backend_version)
def searchsorted(
x: torch.Tensor,
v: torch.Tensor,
diff --git a/ivy/functional/backends/torch/statistical.py b/ivy/functional/backends/torch/statistical.py
index 59c1be8505bb9..dc28d9a86e2e6 100644
--- a/ivy/functional/backends/torch/statistical.py
+++ b/ivy/functional/backends/torch/statistical.py
@@ -14,7 +14,7 @@
# -------------------#
-@with_unsupported_dtypes({"2.0.1 and below": ("complex",)}, backend_version)
+@with_unsupported_dtypes({"2.1.0 and below": ("complex",)}, backend_version)
def min(
x: torch.Tensor,
/,
@@ -66,7 +66,7 @@ def max(
max.support_native_out = True
-@with_supported_dtypes({"2.0.1 and below": ("float", "complex")}, backend_version)
+@with_supported_dtypes({"2.1.0 and below": ("float", "complex")}, backend_version)
def mean(
x: torch.Tensor,
/,
@@ -78,7 +78,7 @@ def mean(
if axis is None:
num_dims = len(x.shape)
axis = list(range(num_dims))
- if axis == () or axis == []:
+ if axis in [(), []]:
if ivy.exists(out):
return ivy.inplace_update(out, x)
else:
@@ -101,7 +101,7 @@ def _infer_dtype(dtype: torch.dtype) -> torch.dtype:
# the function to break the upcasting rule defined in the Array API Standard
@with_unsupported_dtypes(
{
- "2.0.1 and below": ("uint8", "float16", "bfloat16"),
+ "2.1.0 and below": ("uint8", "float16", "bfloat16"),
},
backend_version,
)
@@ -121,7 +121,7 @@ def prod(
return x.type(dtype)
if axis is None:
return torch.prod(input=x, dtype=dtype)
- if isinstance(axis, tuple) or isinstance(axis, list):
+ if isinstance(axis, (tuple, list)):
for i in axis:
x = torch.prod(x, i, keepdim=keepdims, dtype=dtype)
return x
@@ -129,7 +129,7 @@ def prod(
@with_unsupported_dtypes(
- {"2.0.1 and below": ("int8", "int16", "int32", "int64", "float16")},
+ {"2.1.0 and below": ("int8", "int16", "int32", "int64", "float16")},
backend_version,
)
def std(
@@ -166,7 +166,7 @@ def std(
# Function does support uint8, but allowing support for unsigned will cause
# the function to break the upcasting rule defined in the Array API Standard
-@with_unsupported_dtypes({"2.0.1 and below": ("uint8",)}, backend_version)
+@with_unsupported_dtypes({"2.1.0 and below": ("uint8",)}, backend_version)
def sum(
x: torch.Tensor,
/,
@@ -228,7 +228,7 @@ def var(
# TODO: bfloat16 support is added in PyTorch 1.12.1
@with_unsupported_dtypes(
{
- "2.0.1 and below": ("uint8", "float16", "bfloat16"),
+ "2.1.0 and below": ("uint8", "float16", "bfloat16"),
},
backend_version,
)
@@ -319,7 +319,7 @@ def cumsum(
@with_unsupported_dtypes(
- {"2.0.1 and below": ("float16",)},
+ {"2.1.0 and below": ("float16",)},
backend_version,
)
def einsum(
diff --git a/ivy/functional/backends/torch/sub_backends/torchvision/layers.py b/ivy/functional/backends/torch/sub_backends/torchvision/layers.py
index ed5369a7cf6bf..f4af53baf2e05 100644
--- a/ivy/functional/backends/torch/sub_backends/torchvision/layers.py
+++ b/ivy/functional/backends/torch/sub_backends/torchvision/layers.py
@@ -1,5 +1,5 @@
import torch
-from torchvision.ops import roi_align as torch_roi_align, nms as torch_nms
+import torchvision
from ivy.func_wrapper import to_native_arrays_and_back
@@ -7,7 +7,7 @@
def roi_align(
input, boxes, output_size, spatial_scale=1.0, sampling_ratio=-1, aligned=False
):
- ret = torch_roi_align(
+ ret = torchvision.ops.roi_align(
input, boxes, output_size, spatial_scale, sampling_ratio, aligned
)
return ret
@@ -40,7 +40,7 @@ def nms(
else:
ret = torch.tensor([], dtype=torch.int64)
else:
- ret = torch_nms(boxes, scores, iou_threshold)
+ ret = torchvision.ops.nms(boxes, scores, iou_threshold)
if change_id and len(ret) > 0:
ret = torch.tensor(nonzero[ret], dtype=torch.int64).flatten()
diff --git a/ivy/functional/frontends/__init__.py b/ivy/functional/frontends/__init__.py
index 920a7c4f804d8..923e5029fa248 100644
--- a/ivy/functional/frontends/__init__.py
+++ b/ivy/functional/frontends/__init__.py
@@ -2,14 +2,15 @@
versions = {
- "torch": "2.0.1",
- "tensorflow": "2.13.0",
+ "torch": "2.1.0",
+ "tensorflow": "2.14.0",
"numpy": "1.25.2",
"jax": "0.4.14",
"scipy": "1.10.1",
- "paddle": "2.5.1",
+ "paddle": "2.5.2",
"sklearn": "1.3.0",
"xgboost": "1.7.6",
+ "torchvision": "0.15.2.",
}
@@ -26,6 +27,7 @@ def fn_name_from_version_specific_fn_name(name, version):
the version is inferred by importing the framework in the case of frontend
version support and defaults to the highest available version in case of import
failure
+
Returns
-------
the name of the original function which will then point to the version specific
diff --git a/ivy/functional/frontends/jax/array.py b/ivy/functional/frontends/jax/array.py
index e2d579396a8b5..7e5aa872f4c04 100644
--- a/ivy/functional/frontends/jax/array.py
+++ b/ivy/functional/frontends/jax/array.py
@@ -74,7 +74,7 @@ def astype(self, dtype):
f"Dtype {self.dtype} is not castable to {dtype}"
)
- @with_unsupported_dtypes({"2.5.1 and below": ("float16", "bfloat16")}, "paddle")
+ @with_unsupported_dtypes({"2.5.2 and below": ("float16", "bfloat16")}, "paddle")
def argmax(
self,
/,
@@ -90,7 +90,7 @@ def argmax(
keepdims=keepdims,
)
- @with_unsupported_dtypes({"2.5.1 and below": ("float16", "bfloat16")}, "paddle")
+ @with_unsupported_dtypes({"2.5.2 and below": ("float16", "bfloat16")}, "paddle")
def argmin(
self,
/,
@@ -384,6 +384,19 @@ def min(
self, axis=axis, out=out, keepdims=keepdims, where=where
)
+ def std(
+ self, axis=None, dtype=None, out=None, ddof=0, keepdims=False, *, where=None
+ ):
+ return jax_frontend.numpy.std(
+ self,
+ axis=axis,
+ dtype=dtype,
+ out=out,
+ ddof=ddof,
+ keepdims=keepdims,
+ where=where,
+ )
+
def var(
self, *, axis=None, dtype=None, out=None, ddof=False, keepdims=False, where=None
):
@@ -397,6 +410,9 @@ def var(
where=where,
)
+ def swapaxes(self, axis1, axis2):
+ return jax_frontend.numpy.swapaxes(self, axis1=axis1, axis2=axis2)
+
# Jax supports DeviceArray from 0.4.13 and below
# Hence aliasing it here
diff --git a/ivy/functional/frontends/jax/lax/linalg.py b/ivy/functional/frontends/jax/lax/linalg.py
index e5e2042f9a817..a48964c859054 100644
--- a/ivy/functional/frontends/jax/lax/linalg.py
+++ b/ivy/functional/frontends/jax/lax/linalg.py
@@ -1,5 +1,6 @@
import ivy
from ivy.functional.frontends.jax.func_wrapper import to_ivy_arrays_and_back
+from ivy.func_wrapper import with_unsupported_dtypes
@to_ivy_arrays_and_back
@@ -33,6 +34,15 @@ def symmetrize(x):
return ivy.eigh(x, UPLO=UPLO)
+@to_ivy_arrays_and_back
+@with_unsupported_dtypes({"0.4.14 and below": ("bfloat16",)}, "jax")
+def qr(x, /, *, full_matrices=False):
+ mode = "reduced"
+ if full_matrices is True:
+ mode = "complete"
+ return ivy.qr(x, mode=mode)
+
+
@to_ivy_arrays_and_back
def svd(x, /, *, full_matrices=True, compute_uv=True):
if not compute_uv:
diff --git a/ivy/functional/frontends/jax/lax/operators.py b/ivy/functional/frontends/jax/lax/operators.py
index 15276991bc985..fb1285179cfe5 100644
--- a/ivy/functional/frontends/jax/lax/operators.py
+++ b/ivy/functional/frontends/jax/lax/operators.py
@@ -18,7 +18,7 @@
def _argsort_tuple(the_tuple):
- return tuple([i for i, _ in sorted(enumerate(the_tuple), key=lambda x: x[1])])
+ return tuple(i for i, _ in sorted(enumerate(the_tuple), key=lambda x: x[1]))
def _conv_transpose_padding(k, s, padding):
@@ -157,7 +157,7 @@ def broadcast(operand, sizes):
@with_supported_dtypes(
{
- "0.4.16 and below": (
+ "0.4.19 and below": (
"float16",
"float32",
"float64",
@@ -180,6 +180,11 @@ def clamp(min, x, max):
return ivy.clip(x, min, max)
+@to_ivy_arrays_and_back
+def complex(x, y):
+ return ivy.complex(x, y)
+
+
@to_ivy_arrays_and_back
def concatenate(operands, dimension):
return ivy.concat(operands, axis=dimension)
@@ -229,7 +234,7 @@ def conv_general_dilated(
rhs = ivy.astype(rhs, preferred_element_type)
dims = len(lhs.shape) - 2
dim_nums = _dimension_numbers(dimension_numbers, dims + 2)
- rhs_spec = tuple([dim_nums[1][i] for i in (*range(2, dims + 2), 1, 0)])
+ rhs_spec = tuple(dim_nums[1][i] for i in (*range(2, dims + 2), 1, 0))
return ivy.permute_dims(
ivy.conv_general_dilated(
ivy.permute_dims(lhs, axes=dim_nums[0]),
@@ -264,7 +269,7 @@ def conv_transpose(
rhs = ivy.astype(rhs, preferred_element_type)
dims = len(lhs.shape) - 2
dim_nums = _dimension_numbers(dimension_numbers, dims + 2, transp=True)
- rhs_spec = tuple([dim_nums[1][i] for i in (*range(2, dims + 2), 1, 0)])
+ rhs_spec = tuple(dim_nums[1][i] for i in (*range(2, dims + 2), 1, 0))
rhs_dilation = 1 if rhs_dilation is None else rhs_dilation
if isinstance(padding, str):
k_sdims = [rhs.shape[i] for i in rhs_spec[:-2]]
@@ -304,7 +309,7 @@ def cosh(x):
@with_unsupported_dtypes(
- {"0.4.16 and below": ("bfloat16", "float16", "bool", "complex64", "complex128")},
+ {"0.4.19 and below": ("bfloat16", "float16", "bool", "complex64", "complex128")},
"jax",
)
@to_ivy_arrays_and_back
@@ -395,7 +400,7 @@ def erf(x):
@with_supported_dtypes(
{
- "0.4.16 and below": (
+ "0.4.19 and below": (
"float16",
"float32",
"float64",
@@ -449,13 +454,18 @@ def gt(x, y):
return ivy.greater(x, y)
+@to_ivy_arrays_and_back
+def igamma(a, x):
+ return ivy.igamma(a, x=x)
+
+
@to_ivy_arrays_and_back
def imag(x):
return ivy.imag(x)
@with_unsupported_dtypes(
- {"0.4.16 and below": ("bool", "bfloat16")},
+ {"0.4.19 and below": ("bool", "bfloat16")},
"jax",
)
@to_ivy_arrays_and_back
diff --git a/ivy/functional/frontends/jax/nn/non_linear_activations.py b/ivy/functional/frontends/jax/nn/non_linear_activations.py
index 6d36c7440a717..7d1cf70ce0660 100644
--- a/ivy/functional/frontends/jax/nn/non_linear_activations.py
+++ b/ivy/functional/frontends/jax/nn/non_linear_activations.py
@@ -120,9 +120,7 @@ def _type_conversion_64(x):
@to_ivy_arrays_and_back
def celu(x, alpha=1.0):
- ret = ivy.where(x > 0, x, alpha * ivy.expm1(x / alpha))
- dtype = _batch_promotion(x, alpha, default_dtype="float64")
- return ivy.asarray(ret, dtype=dtype)
+ return ivy.celu(x, alpha=alpha)
@to_ivy_arrays_and_back
@@ -291,7 +289,7 @@ def sigmoid(x):
@with_supported_dtypes(
- {"0.4.16 and below": ("complex", "float")},
+ {"0.4.19 and below": ("complex", "float")},
"jax",
)
@to_ivy_arrays_and_back
diff --git a/ivy/functional/frontends/jax/numpy/__init__.py b/ivy/functional/frontends/jax/numpy/__init__.py
index ec899befa786d..6972b2d115109 100644
--- a/ivy/functional/frontends/jax/numpy/__init__.py
+++ b/ivy/functional/frontends/jax/numpy/__init__.py
@@ -399,6 +399,7 @@ def promote_types_jax(
the first of the two types to promote
type2
the second of the two types to promote
+
Returns
-------
ret
diff --git a/ivy/functional/frontends/jax/numpy/creation.py b/ivy/functional/frontends/jax/numpy/creation.py
index fece64da21d92..d82c05b0b3569 100644
--- a/ivy/functional/frontends/jax/numpy/creation.py
+++ b/ivy/functional/frontends/jax/numpy/creation.py
@@ -10,11 +10,28 @@
)
from ivy.func_wrapper import handle_out_argument
-
+from ivy import with_unsupported_device_and_dtypes
ndarray = Array
+@with_unsupported_device_and_dtypes(
+ {
+ "0.4.19 and below": {
+ "cpu": (
+ "float16",
+ "bflooat16",
+ "complex64",
+ "complex128",
+ ),
+ "gpu": (
+ "complex64",
+ "complex128",
+ ),
+ }
+ },
+ "jax",
+)
@handle_jax_dtype
@outputs_to_frontend_arrays
def arange(start, stop=None, step=1, dtype=None):
@@ -179,7 +196,7 @@ def iterable(y):
@to_ivy_arrays_and_back
@with_unsupported_dtypes(
{
- "0.4.16 and below": (
+ "0.4.19 and below": (
"float16",
"bfloat16",
)
@@ -200,7 +217,7 @@ def linspace(start, stop, num=50, endpoint=True, retstep=False, dtype=None, axis
@to_ivy_arrays_and_back
@with_unsupported_dtypes(
{
- "0.4.16 and below": (
+ "0.4.19 and below": (
"float16",
"bfloat16",
)
diff --git a/ivy/functional/frontends/jax/numpy/dtype.py b/ivy/functional/frontends/jax/numpy/dtype.py
index 769e791fafa78..8a7a1d39e5f46 100644
--- a/ivy/functional/frontends/jax/numpy/dtype.py
+++ b/ivy/functional/frontends/jax/numpy/dtype.py
@@ -37,7 +37,7 @@ def can_cast(from_, to, casting="safe"):
"to must be one of dtype, or dtype specifier"
)
- if casting == "no" or casting == "equiv":
+ if casting in ["no", "equiv"]:
return from_ == to
if casting == "safe":
@@ -73,7 +73,7 @@ def can_cast(from_, to, casting="safe"):
@with_supported_dtypes(
- {"2.13.0 and below": ("float16", "float32", "float64")},
+ {"2.14.0 and below": ("float16", "float32", "float64")},
"jax",
)
@to_ivy_arrays_and_back
@@ -82,7 +82,7 @@ def finfo(dtype):
@with_supported_dtypes(
- {"2.13.0 and below": ("integer",)},
+ {"2.14.0 and below": ("integer",)},
"jax",
)
@to_ivy_arrays_and_back
diff --git a/ivy/functional/frontends/jax/numpy/fft.py b/ivy/functional/frontends/jax/numpy/fft.py
index 4291615222f8e..69b9415b6176b 100644
--- a/ivy/functional/frontends/jax/numpy/fft.py
+++ b/ivy/functional/frontends/jax/numpy/fft.py
@@ -19,7 +19,7 @@ def fft2(a, s=None, axes=(-2, -1), norm=None):
@to_ivy_arrays_and_back
-@with_unsupported_dtypes({"2.5.1 and below": ("float16", "bfloat16")}, "paddle")
+@with_unsupported_dtypes({"2.5.2 and below": ("float16", "bfloat16")}, "paddle")
def fftfreq(n, d=1.0, *, dtype=None):
if not isinstance(
n, (int, type(ivy.int8), type(ivy.int16), type(ivy.int32), type(ivy.int64))
diff --git a/ivy/functional/frontends/jax/numpy/linalg.py b/ivy/functional/frontends/jax/numpy/linalg.py
index 7bbf0aa09d62e..6700c92f33cef 100644
--- a/ivy/functional/frontends/jax/numpy/linalg.py
+++ b/ivy/functional/frontends/jax/numpy/linalg.py
@@ -88,7 +88,7 @@ def multi_dot(arrays, *, precision=None):
@to_ivy_arrays_and_back
@with_supported_dtypes(
- {"0.4.16 and below": ("float32", "float64")},
+ {"0.4.19 and below": ("float32", "float64")},
"jax",
)
def norm(x, ord=None, axis=None, keepdims=False):
@@ -127,7 +127,7 @@ def svd(a, /, *, full_matrices=True, compute_uv=True, hermitian=None):
@to_ivy_arrays_and_back
-@with_unsupported_dtypes({"0.4.16 and below": ("float16", "bfloat16")}, "jax")
+@with_unsupported_dtypes({"0.4.19 and below": ("float16", "bfloat16")}, "jax")
def tensorinv(a, ind=2):
old_shape = ivy.shape(a)
prod = 1
diff --git a/ivy/functional/frontends/jax/numpy/logic.py b/ivy/functional/frontends/jax/numpy/logic.py
index 8511db5da28b5..171f864c1dad0 100644
--- a/ivy/functional/frontends/jax/numpy/logic.py
+++ b/ivy/functional/frontends/jax/numpy/logic.py
@@ -101,7 +101,7 @@ def equal(x1, x2, /):
@to_ivy_arrays_and_back
-@with_unsupported_dtypes({"0.4.16 and below": ("bfloat16",)}, "jax")
+@with_unsupported_dtypes({"0.4.19 and below": ("bfloat16",)}, "jax")
def fromfunction(function, shape, *, dtype=float, **kwargs):
def canonicalize_shape(shape, context="shape argument"):
if isinstance(shape, int):
@@ -111,8 +111,8 @@ def canonicalize_shape(shape, context="shape argument"):
elif isinstance(shape, tuple):
return shape
else:
- msg = "{} must be an int, list, or tuple, but got {}."
- raise TypeError(msg.format(context, type(shape)))
+ msg = f"{context} must be an int, list, or tuple, but got {type(shape)}."
+ raise TypeError(msg)
arr = ivy.zeros(shape, dtype=dtype)
shape = canonicalize_shape(shape)
@@ -285,7 +285,7 @@ def right_shift(x1, x2, /):
@to_ivy_arrays_and_back
-@with_unsupported_dtypes({"0.4.16 and below": ("bfloat16", "bool")}, "jax")
+@with_unsupported_dtypes({"0.4.19 and below": ("bfloat16", "bool")}, "jax")
def setxor1d(ar1, ar2, assume_unique=False):
common_dtype = ivy.promote_types(ivy.dtype(ar1), ivy.dtype(ar2))
ar1 = ivy.asarray(ar1, dtype=common_dtype)
diff --git a/ivy/functional/frontends/jax/numpy/manipulations.py b/ivy/functional/frontends/jax/numpy/manipulations.py
index badba2a055349..f3f3c1721c546 100644
--- a/ivy/functional/frontends/jax/numpy/manipulations.py
+++ b/ivy/functional/frontends/jax/numpy/manipulations.py
@@ -37,6 +37,21 @@ def atleast_3d(*arys):
return ivy.atleast_3d(*arys)
+@to_ivy_arrays_and_back
+def bartlett(M):
+ if M < 1:
+ return ivy.array([])
+ if M == 1:
+ return ivy.ones(M, dtype=ivy.float64)
+ res = ivy.arange(0, M)
+ res = ivy.where(
+ ivy.less_equal(res, (M - 1) / 2.0),
+ 2.0 * res / (M - 1),
+ 2.0 - 2.0 * res / (M - 1),
+ )
+ return res
+
+
@to_ivy_arrays_and_back
def blackman(M):
if M < 1:
@@ -116,6 +131,14 @@ def concatenate(arrays, axis=0, dtype=None):
return ret
+@to_ivy_arrays_and_back
+def diagflat(v, k=0):
+ ret = ivy.diagflat(v, offset=k)
+ while len(ivy.shape(ret)) < 2:
+ ret = ret.expand_dims(axis=0)
+ return ret
+
+
@to_ivy_arrays_and_back
def dsplit(ary, indices_or_sections):
if isinstance(indices_or_sections, (list, tuple, ivy.Array)):
diff --git a/ivy/functional/frontends/jax/numpy/mathematical_functions.py b/ivy/functional/frontends/jax/numpy/mathematical_functions.py
index 44c06932e4251..c8a429121b7c7 100644
--- a/ivy/functional/frontends/jax/numpy/mathematical_functions.py
+++ b/ivy/functional/frontends/jax/numpy/mathematical_functions.py
@@ -6,6 +6,15 @@
from ivy.func_wrapper import with_unsupported_dtypes
from ivy.functional.frontends.jax.numpy import promote_types_of_jax_inputs
from ivy.functional.frontends.numpy.manipulation_routines import trim_zeros
+from ivy.utils.einsum_path_helpers import (
+ parse_einsum_input,
+ compute_size_by_dict,
+ flop_count,
+ greedy_path,
+ optimal_path,
+ find_contraction,
+ can_dot,
+)
@to_ivy_arrays_and_back
@@ -67,7 +76,7 @@ def around(a, decimals=0, out=None):
@with_unsupported_dtypes(
- {"0.4.16 and below": ("bfloat16",)},
+ {"0.4.19 and below": ("bfloat16",)},
"jax",
)
@to_ivy_arrays_and_back
@@ -81,7 +90,7 @@ def ceil(x, /):
return ivy.ceil(x)
-@with_unsupported_dtypes({"2.5.1 and below": ("float16", "bfloat16")}, "paddle")
+@with_unsupported_dtypes({"2.5.2 and below": ("float16", "bfloat16")}, "paddle")
@to_ivy_arrays_and_back
def clip(a, a_min=None, a_max=None, out=None):
return ivy.array(ivy.clip(a, a_min, a_max), dtype=a.dtype)
@@ -198,6 +207,197 @@ def ediff1d(ary, to_end=None, to_begin=None):
return diffs
+@to_ivy_arrays_and_back
+def einsum_path(subscripts, *operands, optimize="greedy"):
+ # Figure out what the path really is
+ path_type = optimize
+ if path_type is True:
+ path_type = "greedy"
+ if path_type is None:
+ path_type = False
+
+ explicit_einsum_path = False
+ memory_limit = None
+
+ # No optimization or a named path algorithm
+ if (path_type is False) or isinstance(path_type, str):
+ pass
+
+ # Given an explicit path
+ elif len(path_type) and (path_type[0] == "einsum_path"):
+ explicit_einsum_path = True
+
+ # Path tuple with memory limit
+ elif (
+ (len(path_type) == 2)
+ and isinstance(path_type[0], str)
+ and isinstance(path_type[1], (int, float))
+ ):
+ memory_limit = int(path_type[1])
+ path_type = path_type[0]
+
+ else:
+ raise TypeError("Did not understand the path: %s" % str(path_type))
+
+ # Python side parsing
+ if subscripts:
+ input_subscripts, output_subscript, operands = parse_einsum_input(
+ operands, subscripts=subscripts
+ )
+ else:
+ input_subscripts, output_subscript, operands = parse_einsum_input(operands)
+
+ # Build a few useful list and sets
+ input_list = input_subscripts.split(",")
+ input_sets = [set(x) for x in input_list]
+ output_set = set(output_subscript)
+ indices = set(input_subscripts.replace(",", ""))
+
+ # Get length of each unique dimension and ensure all dimensions are correct
+ dimension_dict = {}
+ broadcast_indices = [[] for x in range(len(input_list))]
+ for tnum, term in enumerate(input_list):
+ sh = operands[tnum].shape
+ if len(sh) != len(term):
+ raise ValueError(
+ "Einstein sum subscript %s does not contain the "
+ "correct number of indices for operand %d."
+ % (input_subscripts[tnum], tnum)
+ )
+ for cnum, char in enumerate(term):
+ dim = sh[cnum]
+
+ # Build out broadcast indices
+ if dim == 1:
+ broadcast_indices[tnum].append(char)
+
+ if char in dimension_dict.keys():
+ # For broadcasting cases we always want the largest dim size
+ if dimension_dict[char] == 1:
+ dimension_dict[char] = dim
+ elif dim not in (1, dimension_dict[char]):
+ raise ValueError(
+ "Size of label '%s' for operand %d (%d) "
+ "does not match previous terms (%d)."
+ % (char, tnum, dimension_dict[char], dim)
+ )
+ else:
+ dimension_dict[char] = dim
+
+ # Convert broadcast inds to sets
+ broadcast_indices = [set(x) for x in broadcast_indices]
+
+ # Compute size of each input array plus the output array
+ size_list = [
+ compute_size_by_dict(term, dimension_dict)
+ for term in input_list + [output_subscript]
+ ]
+ max_size = max(size_list)
+
+ if memory_limit is None:
+ memory_arg = max_size
+ else:
+ memory_arg = memory_limit
+
+ # Compute naive cost
+ # This isn't quite right, need to look into exactly how einsum does this
+ inner_product = (sum(len(x) for x in input_sets) - len(indices)) > 0
+ naive_cost = flop_count(indices, inner_product, len(input_list), dimension_dict)
+
+ # Compute the path
+ if explicit_einsum_path:
+ path = path_type[1:]
+ elif (path_type is False) or (len(input_list) in [1, 2]) or (indices == output_set):
+ # Nothing to be optimized, leave it to einsum
+ path = [tuple(range(len(input_list)))]
+ elif path_type == "greedy":
+ path = greedy_path(input_sets, output_set, dimension_dict, memory_arg)
+ elif path_type == "optimal":
+ path = optimal_path(input_sets, output_set, dimension_dict, memory_arg)
+ else:
+ raise KeyError("Path name %s not found", path_type)
+
+ cost_list, scale_list, size_list, contraction_list = [], [], [], []
+
+ # Build contraction tuple (positions, gemm, einsum_str, remaining)
+ for cnum, contract_inds in enumerate(path):
+ # Make sure we remove inds from right to left
+ contract_inds = tuple(sorted(list(contract_inds), reverse=True))
+
+ contract = find_contraction(contract_inds, input_sets, output_set)
+ out_inds, input_sets, idx_removed, idx_contract = contract
+
+ cost = flop_count(idx_contract, idx_removed, len(contract_inds), dimension_dict)
+ cost_list.append(cost)
+ scale_list.append(len(idx_contract))
+ size_list.append(compute_size_by_dict(out_inds, dimension_dict))
+
+ bcast = set()
+ tmp_inputs = []
+ for x in contract_inds:
+ tmp_inputs.append(input_list.pop(x))
+ bcast |= broadcast_indices.pop(x)
+
+ new_bcast_inds = bcast - idx_removed
+
+ # If we're broadcasting, nix blas
+ if not len(idx_removed & bcast):
+ do_blas = can_dot(tmp_inputs, out_inds, idx_removed)
+ else:
+ do_blas = False
+
+ # Last contraction
+ if (cnum - len(path)) == -1:
+ idx_result = output_subscript
+ else:
+ sort_result = [(dimension_dict[ind], ind) for ind in out_inds]
+ idx_result = "".join([x[1] for x in sorted(sort_result)])
+
+ input_list.append(idx_result)
+ broadcast_indices.append(new_bcast_inds)
+ einsum_str = ",".join(tmp_inputs) + "->" + idx_result
+
+ contraction = (contract_inds, idx_removed, einsum_str, input_list[:], do_blas)
+ contraction_list.append(contraction)
+
+ opt_cost = sum(cost_list) + 1
+
+ if len(input_list) != 1:
+ # Explicit "einsum_path" is usually trusted, but we detect this kind of
+ # mistake in order to prevent from returning an intermediate value.
+ raise RuntimeError(
+ "Invalid einsum_path is specified: {} more operands has to be "
+ "contracted.".format(len(input_list) - 1)
+ )
+
+ # Return the path along with a nice string representation
+ overall_contraction = input_subscripts + "->" + output_subscript
+ header = ("scaling", "current", "remaining")
+
+ speedup = naive_cost / opt_cost
+ max_i = max(size_list)
+
+ path_print = " Complete contraction: %s\n" % overall_contraction
+ path_print += " Naive scaling: %d\n" % len(indices)
+ path_print += " Optimized scaling: %d\n" % max(scale_list)
+ path_print += " Naive FLOP count: %.3e\n" % naive_cost
+ path_print += " Optimized FLOP count: %.3e\n" % opt_cost
+ path_print += " Theoretical speedup: %3.3f\n" % speedup
+ path_print += " Largest intermediate: %.3e elements\n" % max_i
+ path_print += "-" * 74 + "\n"
+ path_print += "%6s %24s %40s\n" % header
+ path_print += "-" * 74
+
+ for n, contraction in enumerate(contraction_list):
+ inds, idx_rm, einsum_str, remaining, blas = contraction
+ remaining_str = ",".join(remaining) + "->" + output_subscript
+ path_run = (scale_list[n], einsum_str, remaining_str)
+ path_print += "\n%4d %24s %40s" % path_run
+
+ ret = (path, path_print)
+ return ret
+
+
@to_ivy_arrays_and_back
def exp(
x,
@@ -220,7 +420,7 @@ def expm1(
@with_unsupported_dtypes(
- {"0.4.16 and below": ("uint16",)},
+ {"0.4.19 and below": ("uint16",)},
"jax",
)
@to_ivy_arrays_and_back
@@ -322,6 +522,11 @@ def inner(a, b):
return ivy.inner(a, b)
+@to_ivy_arrays_and_back
+def interp(x, xp, fp, left=None, right=None, period=None):
+ return ivy.interp(x, xp, fp, left=left, right=right, period=period)
+
+
@to_ivy_arrays_and_back
def kron(a, b):
a, b = promote_types_of_jax_inputs(a, b)
@@ -390,7 +595,7 @@ def minimum(x1, x2, /):
@to_ivy_arrays_and_back
-@with_unsupported_dtypes({"0.4.16 and below": ("complex",)}, "jax")
+@with_unsupported_dtypes({"0.4.19 and below": ("complex",)}, "jax")
def mod(x1, x2, /):
x1, x2 = promote_types_of_jax_inputs(x1, x2)
return ivy.remainder(x1, x2)
@@ -432,7 +637,7 @@ def negative(
@with_unsupported_dtypes(
{
- "0.4.16 and below": (
+ "0.4.19 and below": (
"bfloat16",
"float16",
)
@@ -477,7 +682,7 @@ def polyadd(a1, a2):
@with_unsupported_dtypes(
- {"0.4.16 and below": ("float16",)},
+ {"0.4.19 and below": ("float16",)},
"jax",
)
@to_ivy_arrays_and_back
@@ -519,7 +724,7 @@ def polydiv(u, v, *, trim_leading_zeros=False):
@with_unsupported_dtypes(
- {"0.4.16 and below": ("float16",)},
+ {"0.4.19 and below": ("float16",)},
"jax",
)
@to_ivy_arrays_and_back
diff --git a/ivy/functional/frontends/jax/numpy/searching_sorting.py b/ivy/functional/frontends/jax/numpy/searching_sorting.py
index 8a7a4b891449e..cf538122e1fc5 100644
--- a/ivy/functional/frontends/jax/numpy/searching_sorting.py
+++ b/ivy/functional/frontends/jax/numpy/searching_sorting.py
@@ -15,7 +15,7 @@
@to_ivy_arrays_and_back
@with_unsupported_dtypes(
{
- "0.4.16 and below": (
+ "0.4.19 and below": (
"float16",
"bfloat16",
)
@@ -56,6 +56,21 @@ def argwhere(a, /, *, size=None, fill_value=None):
return result.reshape(result.shape[0], num_of_dimensions)
+@with_unsupported_dtypes(
+ {
+ "0.4.19 and below": (
+ "uint8",
+ "int8",
+ "bool",
+ )
+ },
+ "jax",
+)
+@to_ivy_arrays_and_back
+def count_nonzero(a, axis=None, keepdims=False):
+ return ivy.astype(ivy.count_nonzero(a, axis=axis, keepdims=keepdims), "int64")
+
+
@to_ivy_arrays_and_back
def extract(condition, arr):
if condition.dtype is not bool:
diff --git a/ivy/functional/frontends/jax/numpy/statistical.py b/ivy/functional/frontends/jax/numpy/statistical.py
index 3fa1d35ed66ec..96a10c56c0053 100644
--- a/ivy/functional/frontends/jax/numpy/statistical.py
+++ b/ivy/functional/frontends/jax/numpy/statistical.py
@@ -7,6 +7,7 @@
handle_jax_dtype,
)
from ivy.functional.frontends.jax.numpy import promote_types_of_jax_inputs
+from ivy.functional.backends.jax.experimental.elementwise import _normalize_axis_tuple
@to_ivy_arrays_and_back
@@ -102,7 +103,7 @@ def corrcoef(x, y=None, rowvar=True):
@to_ivy_arrays_and_back
-@with_unsupported_dtypes({"0.4.16 and below": ("float16", "bfloat16")}, "jax")
+@with_unsupported_dtypes({"0.4.19 and below": ("float16", "bfloat16")}, "jax")
def correlate(a, v, mode="valid", precision=None):
if ivy.get_num_dims(a) != 1 or ivy.get_num_dims(v) != 1:
raise ValueError("correlate() only support 1-dimensional inputs.")
@@ -359,6 +360,168 @@ def nanmin(
return res.astype(ivy.dtype(a))
+@to_ivy_arrays_and_back
+@with_unsupported_dtypes(
+ {"0.4.14 and below": ("complex64", "complex128", "bfloat16", "bool", "float16")},
+ "jax",
+)
+def nanpercentile(
+ a, q, axis=None, out=None, overwrite_input=False, method="linear", keepdims=None
+):
+ def _remove_nan_1d(arr1d, overwrite_input=False):
+ if arr1d.dtype == object:
+ c = ivy.not_equal(arr1d, arr1d)
+ else:
+ c = ivy.isnan(arr1d)
+ s = ivy.nonzero(c)[0]
+ if s.size == arr1d.size:
+ return arr1d[:0], True
+ elif s.size == 0:
+ return arr1d, overwrite_input
+ else:
+ if not overwrite_input:
+ arr1d = arr1d.copy()
+
+ enonan = arr1d[-s.size :][~c[-s.size :]]
+ arr1d[s[: enonan.size]] = enonan
+
+ return arr1d[: -s.size], True
+
+ def _nanquantile_1d(arr1d, q, overwrite_input=False, method="linear"):
+ arr1d, overwrite_input = _remove_nan_1d(arr1d, overwrite_input=overwrite_input)
+ if arr1d.size == 0:
+ return ivy.full(q.shape, ivy.nan)
+ return ivy.quantile(arr1d, q, interpolation=method)
+
+ def apply_along_axis(func1d, axis, arr, *args, **kwargs):
+ ndim = ivy.get_num_dims(arr)
+ if axis is None:
+ raise ValueError("Axis must be an integer.")
+ if not -ndim <= axis < ndim:
+ raise ValueError(
+ f"axis {axis} is out of bounds for array of dimension {ndim}"
+ )
+ if axis < 0:
+ axis = axis + ndim
+
+ func = lambda elem: func1d(elem, *args, **kwargs)
+ for i in range(1, ndim - axis):
+ func = ivy.vmap(func, in_axes=i, out_axes=-1)
+ for i in range(axis):
+ func = ivy.vmap(func, in_axes=0, out_axes=0)
+
+ return ivy.asarray(func(arr))
+
+ def _nanquantile_ureduce_func(
+ a, q, axis=None, out=None, overwrite_input=False, method="linear"
+ ):
+ if axis is None or a.ndim == 1:
+ part = a.ravel()
+ result = _nanquantile_1d(
+ part, q, overwrite_input=overwrite_input, method=method
+ )
+ else:
+ result = apply_along_axis(
+ _nanquantile_1d, axis, a, q, overwrite_input, method
+ )
+
+ if q.ndim != 0:
+ result = ivy.moveaxis(result, axis, 0)
+
+ if out is not None:
+ out[...] = result
+
+ return result
+
+ def _ureduce(a, func, keepdims=False, **kwargs):
+ axis = kwargs.get("axis", None)
+ out = kwargs.get("out", None)
+
+ if keepdims is None:
+ keepdims = False
+
+ nd = a.ndim
+ if axis is not None:
+ axis = _normalize_axis_tuple(axis, nd)
+
+ if keepdims:
+ if out is not None:
+ index_out = tuple(
+ 0 if i in axis else slice(None) for i in range(nd)
+ )
+ kwargs["out"] = out[(Ellipsis,) + index_out]
+
+ if len(axis) == 1:
+ kwargs["axis"] = axis[0]
+ else:
+ keep = set(range(nd)) - set(axis)
+ nkeep = len(keep)
+ # swap axis that should not be reduced to front
+ for i, s in enumerate(sorted(keep)):
+ a = a.swapaxes(i, s)
+ # merge reduced axis
+ a = a.reshape(a.shape[:nkeep] + (-1,))
+ kwargs["axis"] = -1
+ else:
+ if keepdims:
+ if out is not None:
+ index_out = (0,) * nd
+ kwargs["out"] = out[(Ellipsis,) + index_out]
+
+ r = func(a, **kwargs)
+
+ if out is not None:
+ return out
+
+ if keepdims:
+ if axis is None:
+ index_r = (ivy.newaxis,) * nd
+ else:
+ index_r = tuple(
+ ivy.newaxis if i in axis else slice(None) for i in range(nd)
+ )
+ r = r[(Ellipsis,) + index_r]
+
+ return r
+
+ def _nanquantile_unchecked(
+ a,
+ q,
+ axis=None,
+ out=None,
+ overwrite_input=False,
+ method="linear",
+ keepdims=None,
+ ):
+ """Assumes that q is in [0, 1], and is an ndarray."""
+ if a.size == 0:
+ return ivy.nanmean(a, axis, out=out, keepdims=keepdims)
+ return _ureduce(
+ a,
+ func=_nanquantile_ureduce_func,
+ q=q,
+ keepdims=keepdims,
+ axis=axis,
+ out=out,
+ overwrite_input=overwrite_input,
+ method=method,
+ )
+
+ a = ivy.array(a)
+ q = ivy.divide(q, 100.0)
+ q = ivy.array(q)
+ if q.ndim == 1 and q.size < 10:
+ for i in range(q.size):
+ if not (0.0 <= q[i] <= 1.0):
+ ivy.logging.warning("percentile s must be in the range [0, 100]")
+ return []
+ else:
+ if not (ivy.all(q >= 0) and ivy.all(q <= 1)):
+ ivy.logging.warning("percentile s must be in the range [0, 100]")
+ return []
+ return _nanquantile_unchecked(a, q, axis, out, overwrite_input, method, keepdims)
+
+
@handle_jax_dtype
@to_ivy_arrays_and_back
def nanstd(
@@ -409,7 +572,7 @@ def ptp(a, axis=None, out=None, keepdims=False):
@to_ivy_arrays_and_back
@with_unsupported_dtypes(
- {"0.4.16 and below": ("complex64", "complex128", "bfloat16", "bool", "float16")},
+ {"0.4.19 and below": ("complex64", "complex128", "bfloat16", "bool", "float16")},
"jax",
)
def quantile(
@@ -434,7 +597,7 @@ def quantile(
@handle_jax_dtype
-@with_unsupported_dtypes({"0.4.16 and below": ("bfloat16",)}, "jax")
+@with_unsupported_dtypes({"0.4.19 and below": ("bfloat16",)}, "jax")
@to_ivy_arrays_and_back
def std(a, axis=None, dtype=None, out=None, ddof=0, keepdims=False, *, where=None):
axis = tuple(axis) if isinstance(axis, list) else axis
diff --git a/ivy/functional/frontends/jax/random.py b/ivy/functional/frontends/jax/random.py
index 393e3af95e933..8a67fe804d687 100644
--- a/ivy/functional/frontends/jax/random.py
+++ b/ivy/functional/frontends/jax/random.py
@@ -15,6 +15,8 @@
def _get_seed(key):
+ if "PRNGKeyArray" in repr(key):
+ key = key._base_array
key1, key2 = int(key[0]), int(key[1])
return ivy.to_scalar(int("".join(map(str, [key1, key2]))))
@@ -36,7 +38,7 @@ def PRNGKey(seed):
@to_ivy_arrays_and_back
@with_supported_dtypes(
{
- "0.4.16 and below": (
+ "0.4.19 and below": (
"float32",
"float64",
)
@@ -68,7 +70,7 @@ def bernoulli(key, p=0.5, shape=None):
@to_ivy_arrays_and_back
@with_unsupported_dtypes(
{
- "0.4.16 and below": (
+ "0.4.19 and below": (
"float16",
"bfloat16",
)
@@ -83,7 +85,7 @@ def beta(key, a, b, shape=None, dtype=None):
@to_ivy_arrays_and_back
@with_unsupported_dtypes(
{
- "0.4.16 and below": (
+ "0.4.19 and below": (
"float16",
"bfloat16",
)
@@ -91,7 +93,6 @@ def beta(key, a, b, shape=None, dtype=None):
"jax",
)
def categorical(key, logits, axis, shape=None):
- _get_seed(key)
logits_arr = ivy.asarray(logits)
if axis >= 0:
@@ -132,7 +133,7 @@ def cauchy(key, shape=(), dtype="float64"):
@to_ivy_arrays_and_back
@with_unsupported_dtypes(
{
- "0.4.16 and below": (
+ "0.4.19 and below": (
"float16",
"bfloat16",
)
@@ -148,7 +149,7 @@ def dirichlet(key, alpha, shape=None, dtype="float32"):
@handle_jax_dtype
@to_ivy_arrays_and_back
@with_unsupported_dtypes(
- {"0.4.16 and below": "uint32"},
+ {"0.4.19 and below": "uint32"},
"jax",
)
def double_sided_maxwell(key, loc, scale, shape=(), dtype="float64"):
@@ -167,7 +168,7 @@ def double_sided_maxwell(key, loc, scale, shape=(), dtype="float64"):
@to_ivy_arrays_and_back
@with_unsupported_dtypes(
{
- "0.4.16 and below": (
+ "0.4.19 and below": (
"float16",
"bfloat16",
)
@@ -183,6 +184,8 @@ def exponential(key, shape=(), dtype="float64"):
@to_ivy_arrays_and_back
def fold_in(key, data):
+ if "PRNGKeyArray" in repr(key):
+ key = key._base_array
s = ivy.bitwise_left_shift(
ivy.asarray(data, dtype=ivy.uint32), ivy.array(32, dtype=ivy.uint32)
)
@@ -193,7 +196,7 @@ def fold_in(key, data):
@to_ivy_arrays_and_back
@with_unsupported_dtypes(
{
- "0.4.16 and below": (
+ "0.4.19 and below": (
"float16",
"bfloat16",
)
@@ -209,7 +212,7 @@ def gamma(key, a, shape=None, dtype="float64"):
@to_ivy_arrays_and_back
@with_unsupported_dtypes(
{
- "0.4.16 and below": (
+ "0.4.19 and below": (
"float16",
"bfloat16",
)
@@ -228,7 +231,7 @@ def generalized_normal(key, p, shape=(), dtype="float64"):
@to_ivy_arrays_and_back
@with_unsupported_dtypes(
{
- "0.4.16 and below": (
+ "0.4.19 and below": (
"float16",
"bfloat16",
)
@@ -252,7 +255,7 @@ def gumbel(key, shape=(), dtype="float64"):
@to_ivy_arrays_and_back
@with_unsupported_dtypes(
{
- "0.4.16 and below": (
+ "0.4.19 and below": (
"float16",
"bfloat16",
)
@@ -267,7 +270,7 @@ def loggamma(key, a, shape=None, dtype="float64"):
@handle_jax_dtype
@to_ivy_arrays_and_back
@with_unsupported_dtypes(
- {"0.4.16 and below": ("float16", "bfloat16")},
+ {"0.4.19 and below": ("float16", "bfloat16")},
"jax",
)
def logistic(key, shape=(), dtype="float64"):
@@ -298,7 +301,7 @@ def maxwell(key, shape, dtype="float64"):
@to_ivy_arrays_and_back
@with_unsupported_dtypes(
{
- "0.4.16 and below": (
+ "0.4.19 and below": (
"float16",
"bfloat16",
)
@@ -326,18 +329,20 @@ def multivariate_normal(key, mean, cov, shape=None, dtype="float64", method="cho
@handle_jax_dtype
@to_ivy_arrays_and_back
def normal(key, shape=(), dtype=None):
- return ivy.random_normal(shape=shape, dtype=dtype, seed=ivy.to_scalar(key[1]))
+ seed = _get_seed(key)
+ return ivy.random_normal(shape=shape, dtype=dtype, seed=seed)
@handle_jax_dtype
@to_ivy_arrays_and_back
def orthogonal(key, n, shape=(), dtype=None):
+ seed = _get_seed(key)
flat_shape = (n, n)
if shape:
flat_shape = shape + flat_shape
# Generate a random matrix with the given shape and dtype
- random_matrix = ivy.random_uniform(key, shape=flat_shape, dtype=dtype)
+ random_matrix = ivy.random_uniform(seed=seed, shape=flat_shape, dtype=dtype)
# Compute the QR decomposition of the random matrix
q, _ = ivy.linalg.qr(random_matrix)
@@ -353,7 +358,7 @@ def orthogonal(key, n, shape=(), dtype=None):
@to_ivy_arrays_and_back
@with_unsupported_dtypes(
{
- "0.4.16 and below": (
+ "0.4.19 and below": (
"float16",
"bfloat16",
)
@@ -388,7 +393,7 @@ def permutation(key, x, axis=0, independent=False):
@handle_jax_dtype
@to_ivy_arrays_and_back
@with_unsupported_dtypes(
- {"0.4.16 and below": ("unsigned", "int8", "int16")},
+ {"0.4.19 and below": ("unsigned", "int8", "int16")},
"jax",
)
def poisson(key, lam, shape=None, dtype=None):
@@ -399,7 +404,7 @@ def poisson(key, lam, shape=None, dtype=None):
@handle_jax_dtype
@to_ivy_arrays_and_back
@with_unsupported_dtypes(
- {"0.4.16 and below": ("unsigned", "int8", "int16")},
+ {"0.4.19 and below": ("unsigned", "int8", "int16")},
"jax",
)
def rademacher(key, shape, dtype="int64"):
@@ -413,7 +418,7 @@ def rademacher(key, shape, dtype="int64"):
@handle_jax_dtype
@to_ivy_arrays_and_back
@with_unsupported_dtypes(
- {"0.4.16 and below": ("unsigned", "int8", "int16")},
+ {"0.4.19 and below": ("unsigned", "int8", "int16")},
"jax",
)
def randint(key, shape, minval, maxval, dtype="int64"):
@@ -441,8 +446,9 @@ def t(key, df, shape=(), dtype="float64"):
@handle_jax_dtype
@to_ivy_arrays_and_back
def uniform(key, shape=(), dtype=None, minval=0.0, maxval=1.0):
+ seed = _get_seed(key)
return ivy.random_uniform(
- low=minval, high=maxval, shape=shape, dtype=dtype, seed=ivy.to_scalar(key[1])
+ low=minval, high=maxval, shape=shape, dtype=dtype, seed=seed
)
diff --git a/ivy/functional/frontends/mindspore/ops/function/nn_func.py b/ivy/functional/frontends/mindspore/ops/function/nn_func.py
index 329c1808e1fa3..18cff733e9baf 100644
--- a/ivy/functional/frontends/mindspore/ops/function/nn_func.py
+++ b/ivy/functional/frontends/mindspore/ops/function/nn_func.py
@@ -12,13 +12,13 @@ def _broadcast_pooling_helper(x, pool_dims: str = "2d", name: str = "padding"):
dims = {"1d": 1, "2d": 2, "3d": 3}
if isinstance(x, int):
- return tuple([x for _ in range(dims[pool_dims])])
+ return tuple(x for _ in range(dims[pool_dims]))
if len(x) == 1:
- return tuple([x[0] for _ in range(dims[pool_dims])])
+ return tuple(x[0] for _ in range(dims[pool_dims]))
elif len(x) == dims[pool_dims]:
return tuple(x)
- elif len(x) != dims[pool_dims]:
+ else:
raise ValueError(
f"`{name}` must either be a single int, "
f"or a tuple of {dims[pool_dims]} ints. "
@@ -147,14 +147,14 @@ def avg_pool2d(
kernel_pads = list(zip(kernel_size, padding))
# Padding should be less than or equal to half of kernel size
- if not all([pad <= kernel / 2 for kernel, pad in kernel_pads]):
+ if not all(pad <= kernel / 2 for kernel, pad in kernel_pads):
raise ValueError(
"pad should be smaller than or equal to half of kernel size, "
f"but got padding={padding}, kernel_size={kernel_size}. "
)
# Figure out padding string
- if all([pad == ivy.ceil((kernel - 1) / 2) for kernel, pad in kernel_pads]):
+ if all(pad == ivy.ceil((kernel - 1) / 2) for kernel, pad in kernel_pads):
padding_str = "SAME"
else:
padding_str = "VALID"
@@ -183,7 +183,7 @@ def conv1d(
dilation=1,
groups=1,
):
- if pad_mode == "valid" or pad_mode == "same":
+ if pad_mode in ["valid", "same"]:
padding = pad_mode
elif pad_mode == "pad":
padding = padding
@@ -204,7 +204,7 @@ def conv2d(
dilation=1,
groups=1,
):
- if pad_mode == "valid" or pad_mode == "same":
+ if pad_mode in ["valid", "same"]:
padding = pad_mode
elif pad_mode == "pad":
padding = padding
@@ -225,7 +225,7 @@ def conv3d(
dilation=1,
groups=1,
):
- if pad_mode == "valid" or pad_mode == "same":
+ if pad_mode in ["valid", "same"]:
padding = pad_mode
elif pad_mode == "pad":
padding = padding
diff --git a/ivy/functional/frontends/numpy/__init__.py b/ivy/functional/frontends/numpy/__init__.py
index 6f17b4b6998b4..8d2eac7018fd3 100644
--- a/ivy/functional/frontends/numpy/__init__.py
+++ b/ivy/functional/frontends/numpy/__init__.py
@@ -454,11 +454,11 @@ def promote_types_of_numpy_inputs(
type1 = ivy.default_dtype(item=x1).strip("u123456789")
type2 = ivy.default_dtype(item=x2).strip("u123456789")
# Ignore type of 0-dim arrays or scalars to mimic numpy
- if not x1.shape == () and x2.shape == () and type1 == type2:
+ if x1.shape != () and x2.shape == () and type1 == type2:
x2 = ivy.asarray(
x2, dtype=x1.dtype, device=ivy.default_device(item=x1, as_native=False)
)
- elif x1.shape == () and not x2.shape == () and type1 == type2:
+ elif x1.shape == () and x2.shape != () and type1 == type2:
x1 = ivy.asarray(
x1, dtype=x2.dtype, device=ivy.default_device(item=x2, as_native=False)
)
@@ -495,7 +495,6 @@ def promote_types_of_numpy_inputs(
from . import ma
from . import fft
-from . import random
from .ufunc import ufunc
from . import linalg
@@ -551,7 +550,6 @@ def promote_types_of_numpy_inputs(
_reciprocal,
_subtract,
_divmod,
- _remainder,
)
from ivy.functional.frontends.numpy.mathematical_functions.trigonometric_functions import ( # noqa
@@ -638,6 +636,7 @@ def promote_types_of_numpy_inputs(
from ivy.functional.frontends.numpy.mathematical_functions.floating_point_routines import ( # noqa
_nextafter,
+ _signbit,
_spacing,
)
@@ -721,6 +720,7 @@ def promote_types_of_numpy_inputs(
conj = ufunc("_conj")
rint = ufunc("_rint")
nextafter = ufunc("_nextafter")
+signbit = ufunc("_signbit")
conjugate = ufunc("_conj")
lcm = ufunc("_lcm")
gcd = ufunc("_gcd")
diff --git a/ivy/functional/frontends/numpy/data_type_routines/general.py b/ivy/functional/frontends/numpy/data_type_routines/general.py
index 8cd3433e82a5d..73695f02f35b9 100644
--- a/ivy/functional/frontends/numpy/data_type_routines/general.py
+++ b/ivy/functional/frontends/numpy/data_type_routines/general.py
@@ -39,7 +39,7 @@ def can_cast(from_, to, casting="safe"):
else:
raise ivy.utils.exceptions.IvyException("to must be dtype or dtype specifier")
- if casting == "no" or casting == "equiv":
+ if casting in ["no", "equiv"]:
return from_ == to
if casting == "safe" and to in np_frontend.numpy_casting_rules[from_]:
diff --git a/ivy/functional/frontends/numpy/fft/discrete_fourier_transform.py b/ivy/functional/frontends/numpy/fft/discrete_fourier_transform.py
index 51ac7b49b2443..67755d6fe3419 100644
--- a/ivy/functional/frontends/numpy/fft/discrete_fourier_transform.py
+++ b/ivy/functional/frontends/numpy/fft/discrete_fourier_transform.py
@@ -25,7 +25,7 @@ def fft(a, n=None, axis=-1, norm=None):
return ivy.fft(ivy.astype(a, ivy.complex128), axis, norm=norm, n=n)
-@with_unsupported_dtypes({"1.26.0 and below": ("int",)}, "numpy")
+@with_unsupported_dtypes({"1.26.1 and below": ("int",)}, "numpy")
@to_ivy_arrays_and_back
def fftfreq(n, d=1.0):
if not isinstance(
@@ -46,7 +46,7 @@ def fftfreq(n, d=1.0):
@to_ivy_arrays_and_back
-@with_unsupported_dtypes({"1.26.0 and below": ("float16",)}, "numpy")
+@with_unsupported_dtypes({"1.26.1 and below": ("float16",)}, "numpy")
def fftshift(x, axes=None):
x = ivy.asarray(x)
@@ -74,6 +74,14 @@ def ifft(a, n=None, axis=-1, norm=None):
return ivy.ifft(a, axis, norm=norm, n=n)
+@with_unsupported_dtypes({"1.24.3 and below": ("float16",)}, "numpy")
+@to_ivy_arrays_and_back
+def ifft2(a, s=None, axes=(-2, -1), norm=None):
+ a = ivy.asarray(a, dtype=ivy.complex128)
+ a = ivy.ifftn(a, s=s, axes=axes, norm=norm)
+ return a
+
+
@with_unsupported_dtypes({"1.24.3 and below": ("float16",)}, "numpy")
@to_ivy_arrays_and_back
def ifftn(a, s=None, axes=None, norm=None):
@@ -83,7 +91,7 @@ def ifftn(a, s=None, axes=None, norm=None):
@to_ivy_arrays_and_back
-@with_unsupported_dtypes({"1.26.0 and below": ("float16",)}, "numpy")
+@with_unsupported_dtypes({"1.26.1 and below": ("float16",)}, "numpy")
def ifftshift(x, axes=None):
x = ivy.asarray(x)
@@ -103,7 +111,7 @@ def ifftshift(x, axes=None):
return roll
-@with_unsupported_dtypes({"1.26.0 and below": ("float16",)}, "numpy")
+@with_unsupported_dtypes({"1.26.1 and below": ("float16",)}, "numpy")
@to_ivy_arrays_and_back
def ihfft(a, n=None, axis=-1, norm=None):
if n is None:
@@ -113,7 +121,7 @@ def ihfft(a, n=None, axis=-1, norm=None):
return output
-@with_unsupported_dtypes({"1.26.0 and below": ("float16",)}, "numpy")
+@with_unsupported_dtypes({"1.26.1 and below": ("float16",)}, "numpy")
@to_ivy_arrays_and_back
def rfft(a, n=None, axis=-1, norm=None):
if norm is None:
diff --git a/ivy/functional/frontends/numpy/func_wrapper.py b/ivy/functional/frontends/numpy/func_wrapper.py
index 54f3a4262b32c..0c2d35f5801f8 100644
--- a/ivy/functional/frontends/numpy/func_wrapper.py
+++ b/ivy/functional/frontends/numpy/func_wrapper.py
@@ -30,10 +30,7 @@ def _assert_array(args, dtype, scalar_check=False, casting="safe"):
if ivy.is_bool_dtype(dtype):
assert_fn = ivy.is_bool_dtype
if ivy.is_int_dtype(dtype):
-
- def assert_fn(x):
- return not ivy.is_float_dtype(x)
-
+ assert_fn = lambda x: not ivy.is_float_dtype(x)
if assert_fn:
ivy.utils.assertions.check_all_or_any_fn(
*args,
@@ -54,19 +51,13 @@ def _assert_no_array(args, dtype, scalar_check=False, none=False):
if args:
first_arg = args[0]
fn_func = ivy.as_ivy_dtype(dtype) if ivy.exists(dtype) else ivy.dtype(first_arg)
-
- def assert_fn(x):
- return ivy.dtype(x) == fn_func
-
+ assert_fn = lambda x: ivy.dtype(x) == fn_func
if scalar_check:
-
- def assert_fn(x):
- return (
- ivy.dtype(x) == fn_func
- if ivy.shape(x) != ()
- else _casting_no_special_case(ivy.dtype(x), fn_func, none)
- )
-
+ assert_fn = lambda x: (
+ ivy.dtype(x) == fn_func
+ if ivy.shape(x) != ()
+ else _casting_no_special_case(ivy.dtype(x), fn_func, none)
+ )
ivy.utils.assertions.check_all_or_any_fn(
*args,
fn=assert_fn,
@@ -82,7 +73,7 @@ def _assert_no_scalar(args, dtype, none=False):
*args,
fn=lambda x: type(x) == type(first_arg), # noqa: E721
type="all",
- message=f"type of input is incompatible with dtype {dtype}",
+ message=f"type of input is incompatible with dtype: {dtype}",
)
if dtype:
if ivy.is_int_dtype(dtype):
@@ -94,7 +85,7 @@ def _assert_no_scalar(args, dtype, none=False):
ivy.utils.assertions.check_equal(
type(args[0]),
check_dtype,
- message=f"type of input is incompatible with dtype {dtype}",
+ message=f"type of input is incompatible with dtype: {dtype}",
as_array=False,
)
if ivy.as_ivy_dtype(dtype) not in ["float64", "int8", "int64", "uint8"]:
@@ -114,15 +105,9 @@ def _assert_scalar(args, dtype):
if args and dtype:
assert_fn = None
if ivy.is_int_dtype(dtype):
-
- def assert_fn(x):
- return not isinstance(x, float)
-
+ assert_fn = lambda x: not isinstance(x, float)
elif ivy.is_bool_dtype(dtype):
-
- def assert_fn(x):
- return isinstance(x, bool)
-
+ assert_fn = lambda x: isinstance(x, bool)
if assert_fn:
ivy.utils.assertions.check_all_or_any_fn(
*args,
@@ -249,7 +234,7 @@ def _from_zero_dim_arrays_to_scalar(*args, **kwargs):
if ("out" in kwargs and kwargs["out"] is None) or "out" not in kwargs:
if isinstance(ret, tuple):
# converting every scalar element of the tuple to float
- data = tuple([ivy.native_array(i) for i in ret])
+ data = tuple(ivy.native_array(i) for i in ret)
data = ivy.copy_nest(data, to_mutable=True)
ret_idx = ivy.nested_argwhere(data, lambda x: x.shape == ())
try:
@@ -258,10 +243,10 @@ def _from_zero_dim_arrays_to_scalar(*args, **kwargs):
ret_idx,
lambda x: np_frontend.numpy_dtype_to_scalar[ivy.dtype(x)](x),
)
- except KeyError:
+ except KeyError as e:
raise ivy.utils.exceptions.IvyException(
"Casting to specified type is unsupported"
- )
+ ) from e
return tuple(data)
else:
# converting the scalar to float
@@ -269,10 +254,10 @@ def _from_zero_dim_arrays_to_scalar(*args, **kwargs):
if data.shape == ():
try:
return np_frontend.numpy_dtype_to_scalar[ivy.dtype(data)](data)
- except KeyError:
+ except KeyError as e:
raise ivy.utils.exceptions.IvyException(
f"Casting to {ivy.dtype(data)} is unsupported"
- )
+ ) from e
return ret
_from_zero_dim_arrays_to_scalar.from_zero_dim_arrays_to_scalar = True
@@ -476,7 +461,7 @@ def _outputs_to_frontend_arrays(*args, order="K", **kwargs):
# once frontend specific backend setting is added
set_default_dtype = False
if not ("dtype" in kwargs and ivy.exists(kwargs["dtype"])) and any(
- [not (ivy.is_array(i) or hasattr(i, "ivy_array")) for i in args]
+ not (ivy.is_array(i) or hasattr(i, "ivy_array")) for i in args
):
if ivy.current_backend_str() == "jax":
import jax
diff --git a/ivy/functional/frontends/numpy/indexing_routines/generating_index_arrays.py b/ivy/functional/frontends/numpy/indexing_routines/generating_index_arrays.py
index 40cb057e7d4b2..71ada70954e77 100644
--- a/ivy/functional/frontends/numpy/indexing_routines/generating_index_arrays.py
+++ b/ivy/functional/frontends/numpy/indexing_routines/generating_index_arrays.py
@@ -1,3 +1,5 @@
+import inspect
+
import ivy
from ivy.functional.frontends.numpy.func_wrapper import (
to_ivy_arrays_and_back,
@@ -17,6 +19,21 @@ def indices(dimensions, dtype=int, sparse=False):
return ivy.indices(dimensions, dtype=dtype, sparse=sparse)
+@to_ivy_arrays_and_back
+def mask_indices(n, mask_func, k=0):
+ mask_func_obj = inspect.unwrap(mask_func)
+ mask_func_name = mask_func_obj.__name__
+ try:
+ ivy_mask_func_obj = getattr(ivy.functional.frontends.numpy, mask_func_name)
+ a = ivy.ones((n, n))
+ mask = ivy_mask_func_obj(a, k=k)
+ indices = ivy.argwhere(mask.ivy_array)
+ ret = indices[:, 0], indices[:, 1]
+ return tuple(ret)
+ except AttributeError as e:
+ print(f"Attribute error: {e}")
+
+
@to_ivy_arrays_and_back
def tril_indices(n, k=0, m=None):
return ivy.tril_indices(n, m, k)
diff --git a/ivy/functional/frontends/numpy/indexing_routines/indexing_like_operations.py b/ivy/functional/frontends/numpy/indexing_routines/indexing_like_operations.py
index 1fbbe1cc5a6bb..4d56c0f208c1d 100644
--- a/ivy/functional/frontends/numpy/indexing_routines/indexing_like_operations.py
+++ b/ivy/functional/frontends/numpy/indexing_routines/indexing_like_operations.py
@@ -66,7 +66,7 @@ def indices(dimensions, dtype=int, sparse=False):
N = len(dimensions)
shape = (1,) * N
if sparse:
- res = tuple()
+ res = ()
else:
res = ivy.empty((N,) + dimensions, dtype=dtype)
for i, dim in enumerate(dimensions):
diff --git a/ivy/functional/frontends/numpy/indexing_routines/inserting_data_into_arrays.py b/ivy/functional/frontends/numpy/indexing_routines/inserting_data_into_arrays.py
index 1aea91f0c99c6..19d474e01f35a 100644
--- a/ivy/functional/frontends/numpy/indexing_routines/inserting_data_into_arrays.py
+++ b/ivy/functional/frontends/numpy/indexing_routines/inserting_data_into_arrays.py
@@ -61,7 +61,7 @@ def __getitem__(self, key):
if "," in item:
vec = item.split(",")
try:
- axis, ndmin = (int(x) for x in vec[:2])
+ axis, ndmin = [int(x) for x in vec[:2]]
if len(vec) == 3:
trans1d = int(vec[2])
continue
diff --git a/ivy/functional/frontends/numpy/linalg/norms_and_other_numbers.py b/ivy/functional/frontends/numpy/linalg/norms_and_other_numbers.py
index c7817eca77720..9f80975cbfcdd 100644
--- a/ivy/functional/frontends/numpy/linalg/norms_and_other_numbers.py
+++ b/ivy/functional/frontends/numpy/linalg/norms_and_other_numbers.py
@@ -23,7 +23,7 @@ def matrix_rank(A, tol=None, hermitian=False):
# solve
-@with_unsupported_dtypes({"1.26.0 and below": ("float16",)}, "numpy")
+@with_unsupported_dtypes({"1.26.1 and below": ("float16",)}, "numpy")
@to_ivy_arrays_and_back
@from_zero_dim_arrays_to_scalar
def norm(x, ord=None, axis=None, keepdims=False):
@@ -46,7 +46,7 @@ def norm(x, ord=None, axis=None, keepdims=False):
# slogdet
-@with_unsupported_dtypes({"1.26.0 and below": ("float16",)}, "numpy")
+@with_unsupported_dtypes({"1.26.1 and below": ("float16",)}, "numpy")
@to_ivy_arrays_and_back
@from_zero_dim_arrays_to_scalar
def slogdet(a):
diff --git a/ivy/functional/frontends/numpy/linalg/solving_equations_and_inverting_matrices.py b/ivy/functional/frontends/numpy/linalg/solving_equations_and_inverting_matrices.py
index 60263d5e5da8f..e97015cde5f8b 100644
--- a/ivy/functional/frontends/numpy/linalg/solving_equations_and_inverting_matrices.py
+++ b/ivy/functional/frontends/numpy/linalg/solving_equations_and_inverting_matrices.py
@@ -10,7 +10,7 @@
# inv
-@with_unsupported_dtypes({"1.26.0 and below": ("float16",)}, "numpy")
+@with_unsupported_dtypes({"1.26.1 and below": ("float16",)}, "numpy")
@to_ivy_arrays_and_back
def inv(a):
return ivy.inv(a)
@@ -19,7 +19,7 @@ def inv(a):
# TODO: replace this with function from API
# As the compositon provides unstable results
@to_ivy_arrays_and_back
-@with_unsupported_dtypes({"1.26.0 and below": ("float16",)}, "numpy")
+@with_unsupported_dtypes({"1.26.1 and below": ("float16",)}, "numpy")
def lstsq(a, b, rcond="warn"):
solution = ivy.matmul(
ivy.pinv(a, rtol=1e-15).astype(ivy.float64), b.astype(ivy.float64)
@@ -32,14 +32,14 @@ def lstsq(a, b, rcond="warn"):
# pinv
# TODO: add hermitian functionality
-@with_unsupported_dtypes({"1.26.0 and below": ("float16",)}, "numpy")
+@with_unsupported_dtypes({"1.26.1 and below": ("float16",)}, "numpy")
@to_ivy_arrays_and_back
def pinv(a, rcond=1e-15, hermitian=False):
return ivy.pinv(a, rtol=rcond)
# solve
-@with_unsupported_dtypes({"1.26.0 and below": ("float16",)}, "numpy")
+@with_unsupported_dtypes({"1.26.1 and below": ("float16",)}, "numpy")
@to_ivy_arrays_and_back
def solve(a, b):
a, b = promote_types_of_numpy_inputs(a, b)
@@ -47,7 +47,7 @@ def solve(a, b):
@to_ivy_arrays_and_back
-@with_unsupported_dtypes({"1.26.0 and below": ("float16", "blfloat16")}, "numpy")
+@with_unsupported_dtypes({"1.26.1 and below": ("float16", "blfloat16")}, "numpy")
def tensorinv(a, ind=2):
old_shape = ivy.shape(a)
prod = 1
diff --git a/ivy/functional/frontends/numpy/logic/truth_value_testing.py b/ivy/functional/frontends/numpy/logic/truth_value_testing.py
index 6af6804ff72fb..0421def6ee57e 100644
--- a/ivy/functional/frontends/numpy/logic/truth_value_testing.py
+++ b/ivy/functional/frontends/numpy/logic/truth_value_testing.py
@@ -78,8 +78,17 @@ def isrealobj(x: any):
@to_ivy_arrays_and_back
def isscalar(element):
- return (
- isinstance(element, (int, float, complex, bool, bytes, str, memoryview))
- or isinstance(element, numbers.Number)
- or isinstance(element, np_frontend.generic)
+ return isinstance(
+ element,
+ (
+ int,
+ float,
+ complex,
+ bool,
+ bytes,
+ str,
+ memoryview,
+ numbers.Number,
+ np_frontend.generic,
+ ),
)
diff --git a/ivy/functional/frontends/numpy/mathematical_functions/floating_point_routines.py b/ivy/functional/frontends/numpy/mathematical_functions/floating_point_routines.py
index a05be440fb7fe..44411b84554ac 100644
--- a/ivy/functional/frontends/numpy/mathematical_functions/floating_point_routines.py
+++ b/ivy/functional/frontends/numpy/mathematical_functions/floating_point_routines.py
@@ -35,6 +35,26 @@ def _nextafter(
return ivy.nextafter(x1, x2, out=out)
+@handle_numpy_out
+@handle_numpy_dtype
+@to_ivy_arrays_and_back
+@handle_numpy_casting
+@from_zero_dim_arrays_to_scalar
+def _signbit(
+ x,
+ /,
+ out=None,
+ *,
+ where=True,
+ casting="safe",
+ order="K",
+ dtype=None,
+ subok=True,
+):
+ x = ivy.astype(x, ivy.float64)
+ return ivy.logical_or(ivy.less(x, 0), ivy.atan2(0.0, x) == ivy.pi, out=out)
+
+
@handle_numpy_out
@handle_numpy_dtype
@to_ivy_arrays_and_back
diff --git a/ivy/functional/frontends/numpy/mathematical_functions/miscellaneous.py b/ivy/functional/frontends/numpy/mathematical_functions/miscellaneous.py
index 5a393d264818d..15e7a81552e49 100644
--- a/ivy/functional/frontends/numpy/mathematical_functions/miscellaneous.py
+++ b/ivy/functional/frontends/numpy/mathematical_functions/miscellaneous.py
@@ -147,7 +147,7 @@ def _fabs(
@to_ivy_arrays_and_back
@from_zero_dim_arrays_to_scalar
@with_supported_dtypes(
- {"1.26.0 and below": ("int8", "int16", "int32", "int64")}, "numpy"
+ {"1.26.1 and below": ("int8", "int16", "int32", "int64")}, "numpy"
) # Add
def _gcd(
x1,
diff --git a/ivy/functional/frontends/numpy/matrix/methods.py b/ivy/functional/frontends/numpy/matrix/methods.py
index 6b49256111058..864bf94b9708b 100644
--- a/ivy/functional/frontends/numpy/matrix/methods.py
+++ b/ivy/functional/frontends/numpy/matrix/methods.py
@@ -32,7 +32,7 @@ def _init_data(self, data, dtype, copy):
if self._data.ndim < 2:
self._data = self._data.reshape((1, -1))
elif self._data.ndim > 2:
- newshape = tuple([x for x in self._data.shape if x > 1])
+ newshape = tuple(x for x in self._data.shape if x > 1)
ndim = len(newshape)
if ndim == 2:
self._data = self._data.reshape(newshape)
diff --git a/ivy/functional/frontends/numpy/ndarray/ndarray.py b/ivy/functional/frontends/numpy/ndarray/ndarray.py
index 8ac0ccb0458c5..c34a2d8b0d314 100644
--- a/ivy/functional/frontends/numpy/ndarray/ndarray.py
+++ b/ivy/functional/frontends/numpy/ndarray/ndarray.py
@@ -17,7 +17,7 @@ def __init__(self, shape, dtype="float32", order=None, _init_overload=False):
if isinstance(dtype, np_frontend.dtype):
dtype = dtype.ivy_dtype
- # in thise case shape is actually the desired array
+ # in this case shape is actually the desired array
if _init_overload:
self._ivy_array = (
ivy.array(shape) if not isinstance(shape, ivy.Array) else shape
@@ -618,9 +618,28 @@ def __rshift__(self, value, /):
def __lshift__(self, value, /):
return ivy.bitwise_left_shift(self.ivy_array, value)
+ def __ilshift__(self, value, /):
+ return ivy.bitwise_left_shift(self.ivy_array, value, out=self)
+
def round(self, decimals=0, out=None):
return np_frontend.round(self, decimals=decimals, out=out)
+ def var(
+ self, axis=None, dtype=None, out=None, ddof=0, keepdims=False, *, where=True
+ ):
+ return np_frontend.var(
+ self,
+ axis=axis,
+ dtype=dtype,
+ out=out,
+ ddof=ddof,
+ keepdims=keepdims,
+ where=where,
+ )
+
+ def __irshift__(self, value, /):
+ return ivy.bitwise_right_shift(self.ivy_array, value, out=self)
+
# --- Helpers --- #
# --------------- #
diff --git a/ivy/functional/frontends/numpy/random/functions.py b/ivy/functional/frontends/numpy/random/functions.py
index 478b50d700128..b6d0e7a6c668a 100644
--- a/ivy/functional/frontends/numpy/random/functions.py
+++ b/ivy/functional/frontends/numpy/random/functions.py
@@ -265,6 +265,15 @@ def standard_cauchy(size=None):
return ivy.tan(ivy.pi * (u - 0.5))
+@to_ivy_arrays_and_back
+@from_zero_dim_arrays_to_scalar
+def standard_exponential(size=None):
+ if size is None:
+ size = 1
+ U = ivy.random_uniform(low=0.0, high=1.0, shape=size, dtype="float64")
+ return -ivy.log(U)
+
+
@to_ivy_arrays_and_back
@from_zero_dim_arrays_to_scalar
def standard_gamma(shape, size=None):
@@ -346,7 +355,7 @@ def wald(mean, scale, size=None):
Y = mean * ivy.square(Y)
X = mean + mu_2l * (Y - ivy.sqrt(((4 * scale) * Y) + ivy.square(Y)))
- condition = U <= mean / (mean + X)
+ condition = mean / (mean + X) >= U
value1 = X
value2 = mean * mean / X
diff --git a/ivy/functional/frontends/numpy/statistics/histograms.py b/ivy/functional/frontends/numpy/statistics/histograms.py
index a8d24fe054241..57d3ae685cb2f 100644
--- a/ivy/functional/frontends/numpy/statistics/histograms.py
+++ b/ivy/functional/frontends/numpy/statistics/histograms.py
@@ -3,7 +3,7 @@
from ivy.func_wrapper import with_supported_dtypes
-@with_supported_dtypes({"1.26.0 and below": ("int64",)}, "numpy")
+@with_supported_dtypes({"1.26.1 and below": ("int64",)}, "numpy")
@to_ivy_arrays_and_back
def bincount(x, /, weights=None, minlength=0):
return ivy.bincount(x, weights=weights, minlength=minlength)
diff --git a/ivy/functional/frontends/numpy/statistics/order_statistics.py b/ivy/functional/frontends/numpy/statistics/order_statistics.py
index 9139b4b3b422f..1484182c4f62a 100644
--- a/ivy/functional/frontends/numpy/statistics/order_statistics.py
+++ b/ivy/functional/frontends/numpy/statistics/order_statistics.py
@@ -38,7 +38,7 @@ def _quantile_is_valid(q):
if not (0.0 <= q[i] <= 1.0):
return False
else:
- if not (ivy.all(0 <= q) and ivy.all(q <= 1)):
+ if not (ivy.all(q >= 0) and ivy.all(q <= 1)):
return False
return True
diff --git a/ivy/functional/frontends/paddle/creation.py b/ivy/functional/frontends/paddle/creation.py
index 5a110fb73d326..24d90399e4f8a 100644
--- a/ivy/functional/frontends/paddle/creation.py
+++ b/ivy/functional/frontends/paddle/creation.py
@@ -7,14 +7,14 @@
)
-@with_unsupported_dtypes({"2.5.1 and below": ("float16", "bfloat16")}, "paddle")
+@with_unsupported_dtypes({"2.5.2 and below": ("float16", "bfloat16")}, "paddle")
@to_ivy_arrays_and_back
def arange(start, end=None, step=1, dtype=None, name=None):
return ivy.arange(start, end, step=step, dtype=dtype)
@with_supported_dtypes(
- {"2.5.1 and below": ("float16", "float32", "float64", "int32", "int64", "bool")},
+ {"2.5.2 and below": ("float16", "float32", "float64", "int32", "int64", "bool")},
"paddle",
)
@to_ivy_arrays_and_back
@@ -30,7 +30,7 @@ def assign(x, output=None):
@with_unsupported_dtypes(
- {"2.5.1 and below": ("bfloat16", "uint16", "uint32", "uint64")}, "paddle"
+ {"2.5.2 and below": ("bfloat16", "uint16", "uint32", "uint64")}, "paddle"
)
@to_ivy_arrays_and_back
def clone(x):
@@ -38,7 +38,7 @@ def clone(x):
@with_supported_dtypes(
- {"2.5.1 and below": ("float32", "float64")},
+ {"2.5.2 and below": ("float32", "float64")},
"paddle",
)
@to_ivy_arrays_and_back
@@ -54,7 +54,7 @@ def complex(real, imag, name=None):
@with_supported_dtypes(
- {"2.5.1 and below": ("float32", "float64", "int32", "int64")}, "paddle"
+ {"2.5.2 and below": ("float32", "float64", "int32", "int64")}, "paddle"
)
@to_ivy_arrays_and_back
def diag(x, offset=0, padding_value=0, name=None):
@@ -69,7 +69,7 @@ def diag(x, offset=0, padding_value=0, name=None):
@with_supported_dtypes(
- {"2.5.1 and below": ("float32", "float64", "int32", "int64")}, "paddle"
+ {"2.5.2 and below": ("float32", "float64", "int32", "int64")}, "paddle"
)
@to_ivy_arrays_and_back
def diagflat(x, offset=0, name=None):
@@ -105,7 +105,7 @@ def full_like(x, fill_value, /, *, dtype=None, name=None):
@with_supported_dtypes(
- {"2.5.1 and below": ("float32", "float64", "int32", "int64")}, "paddle"
+ {"2.5.2 and below": ("float32", "float64", "int32", "int64")}, "paddle"
)
@to_ivy_arrays_and_back
def linspace(start, stop, num, dtype=None, name=None):
@@ -113,7 +113,7 @@ def linspace(start, stop, num, dtype=None, name=None):
@with_supported_dtypes(
- {"2.5.1 and below": ("float32", "float64", "int32", "int64")}, "paddle"
+ {"2.5.2 and below": ("float32", "float64", "int32", "int64")}, "paddle"
)
@to_ivy_arrays_and_back
def logspace(start, stop, num, base=10.0, dtype=None, name=None):
@@ -121,14 +121,14 @@ def logspace(start, stop, num, base=10.0, dtype=None, name=None):
@with_supported_dtypes(
- {"2.5.1 and below": ("float32", "float64", "int32", "int64")}, "paddle"
+ {"2.5.2 and below": ("float32", "float64", "int32", "int64")}, "paddle"
)
@to_ivy_arrays_and_back
def meshgrid(*args, **kwargs):
return ivy.meshgrid(*args, indexing="ij")
-@with_unsupported_dtypes({"2.5.1 and below": "int8"}, "paddle")
+@with_unsupported_dtypes({"2.5.2 and below": "int8"}, "paddle")
@to_ivy_arrays_and_back
def ones(shape, /, *, dtype=None, name=None):
dtype = "float32" if dtype is None else dtype
@@ -136,7 +136,7 @@ def ones(shape, /, *, dtype=None, name=None):
@with_unsupported_dtypes(
- {"2.5.1 and below": ("uint8", "int8", "complex64", "complex128")}, "paddle"
+ {"2.5.2 and below": ("uint8", "int8", "complex64", "complex128")}, "paddle"
)
@to_ivy_arrays_and_back
def ones_like(x, /, *, dtype=None, name=None):
@@ -152,7 +152,7 @@ def to_tensor(data, /, *, dtype=None, place=None, stop_gradient=True):
@with_unsupported_dtypes(
{
- "2.5.1 and below": (
+ "2.5.2 and below": (
"uint8",
"int8",
"int16",
@@ -169,7 +169,7 @@ def tril(x, diagonal=0, name=None):
return ivy.tril(x, k=diagonal)
-@with_supported_dtypes({"2.5.1 and below": ("int32", "int64")}, "paddle")
+@with_supported_dtypes({"2.5.2 and below": ("int32", "int64")}, "paddle")
@to_ivy_arrays_and_back
def tril_indices(row, col, offset=0, dtype="int64"):
arr = ivy.tril_indices(row, col, offset)
@@ -179,7 +179,7 @@ def tril_indices(row, col, offset=0, dtype="int64"):
@with_unsupported_dtypes(
{
- "2.5.1 and below": (
+ "2.5.2 and below": (
"uint8",
"int8",
"int16",
@@ -196,7 +196,7 @@ def triu(x, diagonal=0, name=None):
return ivy.triu(x, k=diagonal)
-@with_supported_dtypes({"2.5.1 and below": ("int32", "int64")}, "paddle")
+@with_supported_dtypes({"2.5.2 and below": ("int32", "int64")}, "paddle")
@to_ivy_arrays_and_back
def triu_indices(row, col=None, offset=0, dtype="int64"):
arr = ivy.triu_indices(row, col, offset)
@@ -206,7 +206,7 @@ def triu_indices(row, col=None, offset=0, dtype="int64"):
return arr
-@with_unsupported_dtypes({"2.5.1 and below": "int8"}, "paddle")
+@with_unsupported_dtypes({"2.5.2 and below": "int8"}, "paddle")
@to_ivy_arrays_and_back
def zeros(shape, /, *, dtype=None, name=None):
dtype = "float32" if dtype is None else dtype
@@ -214,7 +214,7 @@ def zeros(shape, /, *, dtype=None, name=None):
@with_unsupported_dtypes(
- {"2.5.1 and below": ("uint8", "int8", "complex64", "complex128")}, "paddle"
+ {"2.5.2 and below": ("uint8", "int8", "complex64", "complex128")}, "paddle"
)
@to_ivy_arrays_and_back
def zeros_like(x, /, *, dtype=None, name=None):
diff --git a/ivy/functional/frontends/paddle/fft.py b/ivy/functional/frontends/paddle/fft.py
index ae868ddf3e6f5..6687cadf7cd0d 100644
--- a/ivy/functional/frontends/paddle/fft.py
+++ b/ivy/functional/frontends/paddle/fft.py
@@ -7,7 +7,7 @@
@with_supported_dtypes(
- {"2.5.1 and below": ("complex64", "complex128")},
+ {"2.5.2 and below": ("complex64", "complex128")},
"paddle",
)
@to_ivy_arrays_and_back
@@ -18,7 +18,7 @@ def fft(x, n=None, axis=-1.0, norm="backward", name=None):
@with_supported_dtypes(
{
- "2.5.1 and below": (
+ "2.5.2 and below": (
"int32",
"int64",
"float32",
@@ -44,7 +44,7 @@ def fftfreq(n, d=1.0, dtype=None, name=None):
@with_supported_dtypes(
{
- "2.5.1 and below": (
+ "2.5.2 and below": (
"int32",
"int64",
"float32",
@@ -73,7 +73,7 @@ def fftshift(x, axes=None, name=None):
@with_supported_dtypes(
- {"2.5.1 and below": ("complex64", "complex128")},
+ {"2.5.2 and below": ("complex64", "complex128")},
"paddle",
)
@to_ivy_arrays_and_back
@@ -95,7 +95,7 @@ def hfft(x, n=None, axes=-1, norm="backward", name=None):
@with_supported_dtypes(
- {"2.5.1 and below": "complex64"},
+ {"2.5.2 and below": "complex64"},
"paddle",
)
@to_ivy_arrays_and_back
@@ -116,7 +116,7 @@ def hfft2(x, s=None, axis=(-2, -1), norm="backward"):
@with_supported_dtypes(
- {"2.5.1 and below": ("complex64", "complex128")},
+ {"2.5.2 and below": ("complex64", "complex128")},
"paddle",
)
@to_ivy_arrays_and_back
@@ -125,9 +125,19 @@ def ifft(x, n=None, axis=-1.0, norm="backward", name=None):
return ivy.astype(ret, x.dtype)
+@with_supported_dtypes(
+ {"2.5.2 and below": ("complex64", "complex128")},
+ "paddle",
+)
+@to_ivy_arrays_and_back
+def ifftn(x, s=None, axes=None, norm="backward", name=None):
+ ret = ivy.ifftn(ivy.astype(x, "complex128"), s=s, axes=axes, norm=norm)
+ return ivy.astype(ret, x.dtype)
+
+
@with_supported_dtypes(
{
- "2.5.1 and below": (
+ "2.5.2 and below": (
"int32",
"int64",
"float32",
@@ -154,7 +164,42 @@ def ifftshift(x, axes=None, name=None):
@with_supported_dtypes(
- {"2.5.1 and below": ("complex64", "complex128")},
+ {
+ "2.5.2 and below": (
+ "int32",
+ "int64",
+ "float32",
+ "float64",
+ )
+ },
+ "paddle",
+)
+@to_ivy_arrays_and_back
+def ihfft2(x, s=None, axes=(-2, -1), norm="backward", name=None):
+ # check if the input array is two-dimensional and real
+ if len(ivy.array(x).shape) != 2 or ivy.is_complex_dtype(x):
+ raise ValueError("input must be a two-dimensional real array")
+
+ # cast the input to the same float64 type so that there are no backend issues
+ x_ = ivy.astype(x, ivy.float64)
+
+ ihfft2_result = 0
+ # Compute the complex conjugate of the 2-dimensional discrete Fourier Transform
+ if norm == "backward":
+ ihfft2_result = ivy.conj(ivy.rfftn(x_, s=s, axes=axes, norm="forward"))
+ if norm == "forward":
+ ihfft2_result = ivy.conj(ivy.rfftn(x_, s=s, axes=axes, norm="backward"))
+ if norm == "ortho":
+ ihfft2_result = ivy.conj(ivy.rfftn(x_, s=s, axes=axes, norm="ortho"))
+
+ if x.dtype in [ivy.float32, ivy.int32, ivy.int64]:
+ return ivy.astype(ihfft2_result, ivy.complex64)
+ if x.dtype == ivy.float64:
+ return ivy.astype(ihfft2_result, ivy.complex128)
+
+
+@with_supported_dtypes(
+ {"2.5.2 and below": ("complex64", "complex128")},
"paddle",
)
@to_ivy_arrays_and_back
@@ -173,7 +218,7 @@ def irfft(x, n=None, axis=-1.0, norm="backward", name=None):
@with_supported_dtypes(
{
- "2.5.1 and below": (
+ "2.5.2 and below": (
"int32",
"int64",
"float16",
@@ -209,7 +254,7 @@ def irfft2(x, s=None, axes=(-2, -1), norm="backward"):
@with_supported_dtypes(
- {"2.5.1 and below": ("complex64", "complex128")},
+ {"2.5.2 and below": ("complex64", "complex128")},
"paddle",
)
@to_ivy_arrays_and_back
@@ -260,7 +305,7 @@ def irfftn(x, s=None, axes=None, norm="backward", name=None):
return result_t
-@with_supported_dtypes({"2.5.1 and below": ("float32", "float64")}, "paddle")
+@with_supported_dtypes({"2.5.2 and below": ("float32", "float64")}, "paddle")
@to_ivy_arrays_and_back
def rfft(x, n=None, axis=-1, norm="backward", name=None):
return ivy.dft(x, axis=axis, inverse=False, onesided=True, dft_length=n, norm=norm)
diff --git a/ivy/functional/frontends/paddle/linalg.py b/ivy/functional/frontends/paddle/linalg.py
index bf48e8434a4e6..23d7e51f918de 100644
--- a/ivy/functional/frontends/paddle/linalg.py
+++ b/ivy/functional/frontends/paddle/linalg.py
@@ -14,7 +14,7 @@ def bincount(x, weights=None, minlength=0, name=None):
# bmm
-@with_unsupported_dtypes({"2.5.1 and below": ("float16", "bfloat16")}, "paddle")
+@with_unsupported_dtypes({"2.5.2 and below": ("float16", "bfloat16")}, "paddle")
@to_ivy_arrays_and_back
def bmm(x, y, transpose_x=False, transpose_y=False, name=None):
if len(ivy.shape(x)) != 3 or len(ivy.shape(y)) != 3:
@@ -24,14 +24,14 @@ def bmm(x, y, transpose_x=False, transpose_y=False, name=None):
# cholesky
-@with_supported_dtypes({"2.5.1 and below": ("float32", "float64")}, "paddle")
+@with_supported_dtypes({"2.5.2 and below": ("float32", "float64")}, "paddle")
@to_ivy_arrays_and_back
def cholesky(x, /, *, upper=False, name=None):
return ivy.cholesky(x, upper=upper)
# cholesky_solve
-@with_supported_dtypes({"2.5.1 and below": ("float32", "float64")}, "paddle")
+@with_supported_dtypes({"2.5.2 and below": ("float32", "float64")}, "paddle")
@to_ivy_arrays_and_back
def cholesky_solve(x, y, /, *, upper=False, name=None):
if upper:
@@ -41,7 +41,7 @@ def cholesky_solve(x, y, /, *, upper=False, name=None):
# cond
-@with_supported_dtypes({"2.5.1 and below": ("float32", "float64")}, "paddle")
+@with_supported_dtypes({"2.5.2 and below": ("float32", "float64")}, "paddle")
@to_ivy_arrays_and_back
def cond(x, p=None, name=None):
ret = ivy.cond(x, p=p, out=name)
@@ -51,7 +51,7 @@ def cond(x, p=None, name=None):
@with_supported_dtypes(
- {"2.5.1 and below": ("float32", "float64", "int32", "int64")}, "paddle"
+ {"2.5.2 and below": ("float32", "float64", "int32", "int64")}, "paddle"
)
@to_ivy_arrays_and_back
def cross(x, y, /, *, axis=9, name=None):
@@ -59,6 +59,26 @@ def cross(x, y, /, *, axis=9, name=None):
return ivy.cross(x, y, axis=axis)
+# diagonal
+@with_supported_dtypes(
+ {
+ "2.5.2 and below": (
+ "int32",
+ "int64",
+ "float64",
+ "complex128",
+ "float32",
+ "complex64",
+ "bool",
+ )
+ },
+ "paddle",
+)
+@to_ivy_arrays_and_back
+def diagonal(x, offset=0, axis1=0, axis2=1, name=None):
+ return ivy.diagonal(x, offset=offset, axis1=axis1, axis2=axis2)
+
+
@with_supported_dtypes({"2.4.1 and above": ("float64", "float32")}, "paddle")
@to_ivy_arrays_and_back
def dist(x, y, p=2):
@@ -67,7 +87,7 @@ def dist(x, y, p=2):
# dot
-@with_supported_dtypes({"2.5.1 and below": ("float32", "float64")}, "paddle")
+@with_supported_dtypes({"2.5.2 and below": ("float32", "float64")}, "paddle")
@to_ivy_arrays_and_back
def dot(x, y, name=None):
x, y = promote_types_of_paddle_inputs(x, y)
@@ -99,8 +119,39 @@ def eigvalsh(x, UPLO="L", name=None):
return ivy.eigvalsh(x, UPLO=UPLO)
+@to_ivy_arrays_and_back
+def lu_unpack(lu_data, lu_pivots, unpack_datas=True, unpack_pivots=True, *, out=None):
+ A = lu_data
+ n = A.shape
+ m = len(lu_pivots)
+ pivot_matrix = ivy.eye(m)
+ L = ivy.tril(A)
+ L.fill_diagonal(1.000)
+ U = ivy.triu(A)
+ for i in range(m):
+ if i != lu_pivots[i] - 1:
+ pivot_matrix[[i, lu_pivots[i] - 1]] = pivot_matrix[[lu_pivots[i] - 1, i]]
+ P = pivot_matrix
+ if not unpack_datas:
+ L = ivy.zeros(n)
+ U = ivy.zeros(n)
+ if not unpack_pivots:
+ P = ivy.zeros(n)
+ else:
+ P = pivot_matrix
+ result = f"P={P}\n" + f"L={L}\n" + f"U={U}"
+ return result
+ elif not unpack_pivots:
+ P = ivy.zeros(n)
+ result = f"P={P}\n" + f"L={L}\n" + f"U={U}"
+ return result
+ else:
+ result = f"P={P}\n" + f"L={L}\n" + f"U={U}"
+ return result
+
+
# matmul
-@with_unsupported_dtypes({"2.5.1 and below": ("float16", "bfloat16")}, "paddle")
+@with_unsupported_dtypes({"2.5.2 and below": ("float16", "bfloat16")}, "paddle")
@to_ivy_arrays_and_back
def matmul(x, y, transpose_x=False, transpose_y=False, name=None):
x, y = promote_types_of_paddle_inputs(x, y)
@@ -108,21 +159,21 @@ def matmul(x, y, transpose_x=False, transpose_y=False, name=None):
# matrix_power
-@with_unsupported_dtypes({"2.5.1 and below": ("float16", "bfloat16")}, "paddle")
+@with_unsupported_dtypes({"2.5.2 and below": ("float16", "bfloat16")}, "paddle")
@to_ivy_arrays_and_back
def matrix_power(x, n, name=None):
return ivy.matrix_power(x, n)
# mv
-@with_supported_dtypes({"2.5.1 and below": ("float32", "float64")}, "paddle")
+@with_supported_dtypes({"2.5.2 and below": ("float32", "float64")}, "paddle")
@to_ivy_arrays_and_back
def mv(x, vec, name=None):
return ivy.dot(x, vec)
# norm
-@with_supported_dtypes({"2.5.1 and below": ("float32", "float64")}, "paddle")
+@with_supported_dtypes({"2.5.2 and below": ("float32", "float64")}, "paddle")
@to_ivy_arrays_and_back
def norm(x, p="fro", axis=None, keepdim=False, name=None):
if axis is None and p is not None:
@@ -154,7 +205,7 @@ def norm(x, p="fro", axis=None, keepdim=False, name=None):
raise ValueError
elif p == 1:
ret = ivy.sum(ivy.abs(x), axis=axis, keepdims=keepdim)
- elif p == 2 or p == "fro":
+ elif p in [2, "fro"]:
ret = ivy.matrix_norm(x, ord="fro", axis=axis, keepdims=keepdim)
elif p == ivy.inf:
ret = ivy.max(ivy.abs(x), axis=axis, keepdims=keepdim)
@@ -175,7 +226,7 @@ def norm(x, p="fro", axis=None, keepdim=False, name=None):
# pinv
-@with_unsupported_dtypes({"2.5.1 and below": ("float16", "bfloat16")}, "paddle")
+@with_unsupported_dtypes({"2.5.2 and below": ("float16", "bfloat16")}, "paddle")
@to_ivy_arrays_and_back
def pinv(x, rcond=1e-15, hermitian=False, name=None):
# TODO: Add hermitian functionality
@@ -183,21 +234,21 @@ def pinv(x, rcond=1e-15, hermitian=False, name=None):
# qr
-@with_supported_dtypes({"2.5.1 and below": ("float32", "float64")}, "paddle")
+@with_supported_dtypes({"2.5.2 and below": ("float32", "float64")}, "paddle")
@to_ivy_arrays_and_back
def qr(x, mode="reduced", name=None):
return ivy.qr(x, mode=mode)
# solve
-@with_supported_dtypes({"2.5.1 and below": ("float32", "float64")}, "paddle")
+@with_supported_dtypes({"2.5.2 and below": ("float32", "float64")}, "paddle")
@to_ivy_arrays_and_back
def solve(x, y, name=None):
return ivy.solve(x, y)
# transpose
-@with_unsupported_dtypes({"2.5.1 and below": ("uint8", "int8", "int16")}, "paddle")
+@with_unsupported_dtypes({"2.5.2 and below": ("uint8", "int8", "int16")}, "paddle")
@to_ivy_arrays_and_back
def transpose(x, perm, name=None):
return ivy.permute_dims(x, axes=perm)
diff --git a/ivy/functional/frontends/paddle/logic.py b/ivy/functional/frontends/paddle/logic.py
index e1b4c3d502058..220d26ded9aaf 100644
--- a/ivy/functional/frontends/paddle/logic.py
+++ b/ivy/functional/frontends/paddle/logic.py
@@ -13,7 +13,7 @@
@with_supported_dtypes(
{
- "2.5.1 and below": (
+ "2.5.2 and below": (
"float32",
"float64",
"bool",
@@ -35,7 +35,7 @@ def allclose(x, y, rtol=1e-05, atol=1e-08, equal_nan=False, name=None):
@with_supported_dtypes(
{
- "2.5.1 and below": (
+ "2.5.2 and below": (
"bool",
"uint8",
"int8",
@@ -54,7 +54,7 @@ def bitwise_and(x, y, /, *, name=None, out=None):
@with_supported_dtypes(
{
- "2.5.1 and below": (
+ "2.5.2 and below": (
"bool",
"uint8",
"int8",
@@ -73,7 +73,7 @@ def bitwise_not(x, out=None, name=None):
@with_supported_dtypes(
{
- "2.5.1 and below": (
+ "2.5.2 and below": (
"bool",
"uint8",
"int8",
@@ -92,7 +92,7 @@ def bitwise_or(x, y, name=None, out=None):
@with_supported_dtypes(
{
- "2.5.1 and below": (
+ "2.5.2 and below": (
"bool",
"uint8",
"int8",
@@ -110,7 +110,8 @@ def bitwise_xor(x, y, /, *, name=None, out=None):
@with_unsupported_dtypes(
- {"2.5.1 and below": ("uint8", "int8", "int16", "complex64", "complex128")}, "paddle"
+ {"2.5.2 and below": ("uint8", "int8", "int16", "complex64", "complex128")},
+ "paddle",
)
@to_ivy_arrays_and_back
def equal(x, y, /, *, name=None):
@@ -119,7 +120,7 @@ def equal(x, y, /, *, name=None):
@with_unsupported_dtypes(
{
- "2.5.1 and below": (
+ "2.5.2 and below": (
"uint8",
"int8",
"int16",
@@ -136,7 +137,7 @@ def equal_all(x, y, /, *, name=None):
@with_unsupported_dtypes(
- {"2.5.1 and below": ("bool", "uint8", "int8", "int16", "complex64", "complex128")},
+ {"2.5.2 and below": ("bool", "uint8", "int8", "int16", "complex64", "complex128")},
"paddle",
)
@to_ivy_arrays_and_back
@@ -145,7 +146,7 @@ def greater_equal(x, y, /, *, name=None):
@with_unsupported_dtypes(
- {"2.5.1 and below": ("bool", "uint8", "int8", "int16", "complex64", "complex128")},
+ {"2.5.2 and below": ("bool", "uint8", "int8", "int16", "complex64", "complex128")},
"paddle",
)
@to_ivy_arrays_and_back
@@ -154,7 +155,8 @@ def greater_than(x, y, /, *, name=None):
@with_unsupported_dtypes(
- {"2.5.1 and below": ("uint8", "int8", "int16", "complex64", "complex128")}, "paddle"
+ {"2.5.2 and below": ("uint8", "int8", "int16", "complex64", "complex128")},
+ "paddle",
)
@to_ivy_arrays_and_back
def is_empty(x, name=None):
@@ -168,7 +170,7 @@ def is_tensor(x):
@with_supported_dtypes(
{
- "2.5.1 and below": (
+ "2.5.2 and below": (
"float32",
"float64",
)
@@ -181,7 +183,7 @@ def isclose(x, y, rtol=1e-05, atol=1e-08, equal_nan=False, name=None):
@with_unsupported_dtypes(
- {"2.5.1 and below": ("bool", "uint8", "int8", "int16", "complex64", "complex128")},
+ {"2.5.2 and below": ("bool", "uint8", "int8", "int16", "complex64", "complex128")},
"paddle",
)
@to_ivy_arrays_and_back
@@ -190,7 +192,7 @@ def less_equal(x, y, /, *, name=None):
@with_supported_dtypes(
- {"2.5.1 and below": ("bool", "float16", "float32", "float64", "int32", "int64")},
+ {"2.5.2 and below": ("bool", "float16", "float32", "float64", "int32", "int64")},
"paddle",
)
@to_ivy_arrays_and_back
@@ -200,7 +202,7 @@ def less_than(x, y, /, *, name=None):
@with_supported_dtypes(
{
- "2.5.1 and below": (
+ "2.5.2 and below": (
"bool",
"int8",
"int16",
@@ -220,7 +222,7 @@ def logical_and(x, y, /, *, name=None, out=None):
@with_supported_dtypes(
{
- "2.5.1 and below": (
+ "2.5.2 and below": (
"bool",
"int8",
"int16",
@@ -240,7 +242,7 @@ def logical_not(x, /, *, name=None, out=None):
@with_supported_dtypes(
{
- "2.5.1 and below": (
+ "2.5.2 and below": (
"bool",
"int8",
"int16",
@@ -260,7 +262,7 @@ def logical_or(x, y, /, *, name=None, out=None):
@with_supported_dtypes(
{
- "2.5.1 and below": (
+ "2.5.2 and below": (
"bool",
"int8",
"int16",
@@ -279,8 +281,13 @@ def logical_xor(x, y, /, *, name=None, out=None):
@with_unsupported_dtypes(
- {"2.5.1 and below": ("uint8", "int8", "int16", "complex64", "complex128")}, "paddle"
+ {"2.5.2 and below": ("uint8", "int8", "int16", "complex64", "complex128")},
+ "paddle",
)
@to_ivy_arrays_and_back
def not_equal(x, y, /, *, name=None):
+ if ivy.is_float_dtype(x):
+ diff = ivy.abs(ivy.subtract(x, y))
+ res = ivy.not_equal(x, y)
+ return ivy.where(diff < 1e-8, False, res)
return ivy.not_equal(x, y)
diff --git a/ivy/functional/frontends/paddle/manipulation.py b/ivy/functional/frontends/paddle/manipulation.py
index b6a3ffc88127c..69e9f6c22dbb3 100644
--- a/ivy/functional/frontends/paddle/manipulation.py
+++ b/ivy/functional/frontends/paddle/manipulation.py
@@ -10,14 +10,14 @@
)
-@with_unsupported_dtypes({"2.5.1 and below": ("float16", "bfloat16")}, "paddle")
+@with_unsupported_dtypes({"2.5.2 and below": ("float16", "bfloat16")}, "paddle")
@to_ivy_arrays_and_back
def abs(x, name=None):
return ivy.abs(x)
@with_supported_dtypes(
- {"2.5.1 and below": ("bool", "float32", "float64", "int32", "int64")},
+ {"2.5.2 and below": ("bool", "float32", "float64", "int32", "int64")},
"paddle",
)
@to_ivy_arrays_and_back
@@ -27,7 +27,7 @@ def broadcast_to(x, shape, name=None):
@with_supported_dtypes(
{
- "2.5.1 and below": (
+ "2.5.2 and below": (
"bool",
"float16",
"float32",
@@ -44,14 +44,14 @@ def cast(x, dtype):
return ivy.astype(x, dtype)
-@with_unsupported_dtypes({"2.5.1 and below": ("int8", "int16")}, "paddle")
+@with_unsupported_dtypes({"2.5.2 and below": ("int8", "int16")}, "paddle")
@to_ivy_arrays_and_back
def concat(x, axis, name=None):
return ivy.concat(x, axis=axis)
@with_supported_dtypes(
- {"2.5.1 and below": ("bool", "float32", "float64", "int32", "int64")},
+ {"2.5.2 and below": ("bool", "float32", "float64", "int32", "int64")},
"paddle",
)
@to_ivy_arrays_and_back
@@ -60,7 +60,7 @@ def expand(x, shape, name=None):
@with_unsupported_dtypes(
- {"2.5.1 and below": ("int8", "uint8", "int16", "float16")},
+ {"2.5.2 and below": ("int8", "uint8", "int16", "float16")},
"paddle",
)
@to_ivy_arrays_and_back
@@ -69,7 +69,7 @@ def flip(x, axis, name=None):
@with_supported_dtypes(
- {"2.5.1 and below": ("bool", "float32", "float64", "int32", "int64")},
+ {"2.5.2 and below": ("bool", "float32", "float64", "int32", "int64")},
"paddle",
)
@to_ivy_arrays_and_back
@@ -77,6 +77,15 @@ def gather(params, indices, axis=-1, batch_dims=0, name=None):
return ivy.gather(params, indices, axis=axis, batch_dims=batch_dims)
+@with_unsupported_dtypes(
+ {"2.5.2 and below": ("int8", "uint8", "int16", "uint16", "float16", "bfloat16")},
+ "paddle",
+)
+@to_ivy_arrays_and_back
+def gather_nd(x, index, name=None):
+ return ivy.gather_nd(x, index)
+
+
@to_ivy_arrays_and_back
def put_along_axis(arr, indices, values, axis, reduce="assign"):
result = ivy.put_along_axis(arr, indices, values, axis)
@@ -84,7 +93,7 @@ def put_along_axis(arr, indices, values, axis, reduce="assign"):
@with_supported_dtypes(
- {"2.5.1 and below": ("int32", "int64", "float32", "float64")},
+ {"2.5.2 and below": ("int32", "int64", "float32", "float64")},
"paddle",
)
@to_ivy_arrays_and_back
@@ -93,7 +102,7 @@ def repeat_interleave(x, repeats, axis=None, name=None):
@to_ivy_arrays_and_back
-def reshape(x, shape):
+def reshape(x, shape, name=None):
return ivy.reshape(x, shape)
@@ -117,7 +126,7 @@ def roll(x, shifts, axis=None, name=None):
@with_supported_device_and_dtypes(
{
- "2.5.1 and above": {
+ "2.5.2 and above": {
"cpu": (
"bool",
"int32",
@@ -136,7 +145,7 @@ def rot90(x, k=1, axes=(0, 1), name=None):
@with_unsupported_dtypes(
- {"2.5.1 and below": ("int16", "complex64", "complex128")},
+ {"2.5.2 and below": ("int16", "complex64", "complex128")},
"paddle",
)
@to_ivy_arrays_and_back
@@ -145,7 +154,7 @@ def split(x, num_or_sections, axis=0, name=None):
@with_unsupported_dtypes(
- {"2.5.1 and below": ("float16", "bfloat16", "int8", "int16")},
+ {"2.5.2 and below": ("float16", "bfloat16", "int8", "int16")},
"paddle",
)
@to_ivy_arrays_and_back
@@ -163,7 +172,7 @@ def take_along_axis(arr, indices, axis):
@with_unsupported_dtypes(
- {"2.5.1 and below": ("int8", "uint8", "int16", "float16")},
+ {"2.5.2 and below": ("int8", "uint8", "int16", "float16")},
"paddle",
)
@to_ivy_arrays_and_back
@@ -171,8 +180,13 @@ def tile(x, repeat_times, name=None):
return ivy.tile(x, repeats=repeat_times)
+@to_ivy_arrays_and_back
+def tolist(x):
+ return ivy.to_list(x)
+
+
@with_supported_dtypes(
- {"2.5.1 and below": ("bool", "int32", "int64", "float16", "float32", "float64")},
+ {"2.5.2 and below": ("bool", "int32", "int64", "float16", "float32", "float64")},
"paddle",
)
@to_ivy_arrays_and_back
@@ -180,12 +194,21 @@ def unbind(input, axis=0):
shape = list(input.shape)
num_splits = shape[axis]
shape.pop(axis)
- return tuple([x.reshape(tuple(shape)) for x in split(input, num_splits, axis=axis)])
+ return tuple(x.reshape(tuple(shape)) for x in split(input, num_splits, axis=axis))
+
+
+@with_supported_dtypes(
+ {"2.5.2 and below": ("bool", "int32", "int64", "float16", "float32", "float64")},
+ "paddle",
+)
+@to_ivy_arrays_and_back
+def unique_consecutive(x, axis=0):
+ return ivy.unique_consecutive(x, axis=axis)
@with_supported_dtypes(
{
- "2.5.1 and below": (
+ "2.5.2 and below": (
"float32",
"float64",
"int32",
diff --git a/ivy/functional/frontends/paddle/math.py b/ivy/functional/frontends/paddle/math.py
index d0d297af952f6..f8cd603766781 100644
--- a/ivy/functional/frontends/paddle/math.py
+++ b/ivy/functional/frontends/paddle/math.py
@@ -8,26 +8,26 @@
from ivy.functional.frontends.paddle.func_wrapper import to_ivy_arrays_and_back
-@with_unsupported_dtypes({"2.5.1 and below": ("float16", "bfloat16")}, "paddle")
+@with_unsupported_dtypes({"2.5.2 and below": ("float16", "bfloat16")}, "paddle")
@to_ivy_arrays_and_back
def abs(x, name=None):
return ivy.abs(x)
-@with_unsupported_dtypes({"2.5.1 and below": ("float16", "bfloat16")}, "paddle")
+@with_unsupported_dtypes({"2.5.2 and below": ("float16", "bfloat16")}, "paddle")
@to_ivy_arrays_and_back
def acos(x, name=None):
return ivy.acos(x)
-@with_unsupported_dtypes({"2.5.1 and below": ("float16", "bfloat16")}, "paddle")
+@with_unsupported_dtypes({"2.5.2 and below": ("float16", "bfloat16")}, "paddle")
@to_ivy_arrays_and_back
def acosh(x, name=None):
return ivy.acosh(x)
@with_unsupported_dtypes(
- {"2.5.1 and below": ("bool", "unsigned", "int8", "float16", "bfloat16")}, "paddle"
+ {"2.5.2 and below": ("bool", "unsigned", "int8", "float16", "bfloat16")}, "paddle"
)
@to_ivy_arrays_and_back
def add(x, y, name=None):
@@ -35,7 +35,7 @@ def add(x, y, name=None):
@with_unsupported_dtypes(
- {"2.5.1 and below": ("bool", "unsigned", "int8", "float16", "bfloat16")}, "paddle"
+ {"2.5.2 and below": ("bool", "unsigned", "int8", "float16", "bfloat16")}, "paddle"
)
@to_ivy_arrays_and_back
def add_(x, y, name=None):
@@ -43,7 +43,7 @@ def add_(x, y, name=None):
@with_supported_dtypes(
- {"2.5.1 and below": ("float32", "float64", "int32", "int64")}, "paddle"
+ {"2.5.2 and below": ("float32", "float64", "int32", "int64")}, "paddle"
)
@to_ivy_arrays_and_back
def addmm(input, x, y, beta=1.0, alpha=1.0, name=None):
@@ -51,8 +51,14 @@ def addmm(input, x, y, beta=1.0, alpha=1.0, name=None):
return value
+@with_supported_dtypes({"2.5.0 and below": "bool"}, "paddle")
+@to_ivy_arrays_and_back
+def all(x, axis, keepdim=False, name=None):
+ return ivy.all(x, axis=axis, keepdims=keepdim)
+
+
@with_supported_dtypes(
- {"2.5.1 and below": ("float32", "float64", "int32", "int64")}, "paddle"
+ {"2.5.2 and below": ("float32", "float64", "int32", "int64")}, "paddle"
)
@to_ivy_arrays_and_back
def amax(x, axis=None, keepdims=False):
@@ -65,12 +71,12 @@ def amax(x, axis=None, keepdims=False):
axis[i] += x.ndim
for i in axis:
if i < 0 or i >= x.ndim:
- raise ValueError(f"axis {i} is out of range [-{0}:{x.ndim}]")
+ raise ValueError(f"axis {i} is out of range [-0:{x.ndim}]")
return ivy.max(x, axis=axis, keepdims=keepdims)
@with_supported_dtypes(
- {"2.5.1 and below": ("float32", "float64", "int32", "int64")}, "paddle"
+ {"2.5.2 and below": ("float32", "float64", "int32", "int64")}, "paddle"
)
@to_ivy_arrays_and_back
def amin(x, axis=None, keepdim=False, name=None):
@@ -78,7 +84,7 @@ def amin(x, axis=None, keepdim=False, name=None):
@with_supported_dtypes(
- {"2.5.1 and below": ("complex64", "complex128", "float32", "float64")},
+ {"2.5.2 and below": ("complex64", "complex128", "float32", "float64")},
"paddle",
)
@to_ivy_arrays_and_back
@@ -92,19 +98,19 @@ def any(x, axis=None, keepdim=False, name=None):
return ivy.any(x, axis=axis, keepdims=keepdim)
-@with_supported_dtypes({"2.5.1 and below": ("float32", "float64")}, "paddle")
+@with_supported_dtypes({"2.5.2 and below": ("float32", "float64")}, "paddle")
@to_ivy_arrays_and_back
def asin(x, name=None):
return ivy.asin(x)
-@with_unsupported_dtypes({"2.5.1 and below": ("float16", "bfloat16")}, "paddle")
+@with_unsupported_dtypes({"2.5.2 and below": ("float16", "bfloat16")}, "paddle")
@to_ivy_arrays_and_back
def asinh(x, name=None):
return ivy.asinh(x)
-@with_unsupported_dtypes({"2.5.1 and below": ("float16", "bfloat16")}, "paddle")
+@with_unsupported_dtypes({"2.5.2 and below": ("float16", "bfloat16")}, "paddle")
@to_ivy_arrays_and_back
def atan(x, name=None):
return ivy.atan(x)
@@ -116,13 +122,19 @@ def atan2(x, y, name=None):
return ivy.atan2(x, y)
-@with_unsupported_dtypes({"2.5.1 and below": ("float16", "bfloat16")}, "paddle")
+@with_unsupported_dtypes({"2.5.2 and below": ("float16", "bfloat16")}, "paddle")
@to_ivy_arrays_and_back
def atanh(x, name=None):
return ivy.atanh(x)
-@with_unsupported_dtypes({"2.5.1 and below": ("float16", "bfloat16")}, "paddle")
+@with_supported_dtypes({"2.5.2 and below": ("int32", "int64")}, "paddle")
+@to_ivy_arrays_and_back
+def broadcast_shape(x_shape, y_shape):
+ return ivy.broadcast_shapes(x_shape, y_shape)
+
+
+@with_unsupported_dtypes({"2.5.2 and below": ("float16", "bfloat16")}, "paddle")
@to_ivy_arrays_and_back
def ceil(x, name=None):
return ivy.ceil(x)
@@ -134,20 +146,20 @@ def conj(x, name=None):
return ivy.conj(x)
-@with_unsupported_dtypes({"2.5.1 and below": ("float16", "bfloat16")}, "paddle")
+@with_unsupported_dtypes({"2.5.2 and below": ("float16", "bfloat16")}, "paddle")
@to_ivy_arrays_and_back
def cos(x, name=None):
return ivy.cos(x)
-@with_unsupported_dtypes({"2.5.1 and below": ("float16", "bfloat16")}, "paddle")
+@with_unsupported_dtypes({"2.5.2 and below": ("float16", "bfloat16")}, "paddle")
@to_ivy_arrays_and_back
def cosh(x, name=None):
return ivy.cosh(x)
@with_supported_dtypes(
- {"2.5.1 and below": ("int32", "int64", "float16", "float32", "float64", "bool")},
+ {"2.5.2 and below": ("int32", "int64", "float16", "float32", "float64", "bool")},
"paddle",
)
@to_ivy_arrays_and_back
@@ -157,7 +169,7 @@ def count_nonzero(x, axis=None, keepdim=False, name=None):
@with_supported_dtypes(
{
- "2.5.1 and below": (
+ "2.5.2 and below": (
"int32",
"int64",
"float32",
@@ -173,60 +185,87 @@ def cumprod(x, dim=None, dtype=None, name=None):
return ivy.cumprod(x, axis=dim, dtype=dtype)
-@with_unsupported_dtypes({"2.5.1 and below": ("float16", "bfloat16")}, "paddle")
+@with_supported_dtypes(
+ {"2.5.2 and below": ("float32", "float64", "int32", "int64")}, "paddle"
+)
+@to_ivy_arrays_and_back
+def cumsum(x, axis=None, dtype=None, name=None):
+ return ivy.cumsum(x, axis=axis, dtype=dtype)
+
+
+@with_unsupported_dtypes({"2.5.2 and below": ("float16", "bfloat16")}, "paddle")
@to_ivy_arrays_and_back
def deg2rad(x, name=None):
return ivy.deg2rad(x)
@with_supported_dtypes(
- {"2.5.1 and below": ("float32", "float64", "int32", "int64")}, "paddle"
+ {
+ "2.5.2 and below": (
+ "int32",
+ "int64",
+ "float64",
+ "complex128",
+ "float32",
+ "complex64",
+ "bool",
+ )
+ },
+ "paddle",
+)
+@to_ivy_arrays_and_back
+def diagonal(x, offset=0, axis1=0, axis2=1, name=None):
+ return ivy.diagonal(x, offset=offset, axis1=axis1, axis2=axis2)
+
+
+@with_supported_dtypes(
+ {"2.5.2 and below": ("float32", "float64", "int32", "int64")}, "paddle"
)
@to_ivy_arrays_and_back
def diff(x, n=1, axis=-1, prepend=None, append=None, name=None):
return ivy.diff(x, n=n, axis=axis, prepend=prepend, append=append)
-@with_supported_dtypes({"2.5.1 and below": ("float32", "float64")}, "paddle")
+@with_supported_dtypes({"2.5.2 and below": ("float32", "float64")}, "paddle")
@to_ivy_arrays_and_back
def digamma(x, name=None):
digamma_fun = ivy.digamma
return ivy.array(digamma_fun(x), dtype=x.dtype)
-@with_unsupported_dtypes({"2.5.1 and below": ("float16", "bfloat16")}, "paddle")
+@with_unsupported_dtypes({"2.5.2 and below": ("float16", "bfloat16")}, "paddle")
@to_ivy_arrays_and_back
def divide(x, y, name=None):
return ivy.divide(x, y)
-@with_supported_dtypes({"2.5.1 and below": ("float32", "float64")}, "paddle")
+@with_supported_dtypes({"2.5.2 and below": ("float32", "float64")}, "paddle")
@to_ivy_arrays_and_back
def erf(x, name=None):
return ivy.erf(x)
-@with_supported_dtypes({"2.5.1 and below": ("float32", "float64")}, "paddle")
+@with_supported_dtypes({"2.5.2 and below": ("float32", "float64")}, "paddle")
@to_ivy_arrays_and_back
def exp(x, name=None):
return ivy.exp(x)
-@with_supported_dtypes({"2.5.1 and below": ("float16", "float32", "float64")}, "paddle")
+@with_supported_dtypes({"2.5.2 and below": ("float16", "float32", "float64")}, "paddle")
@to_ivy_arrays_and_back
def expm1(x, name=None):
return ivy.expm1(x)
@with_supported_dtypes(
- {"2.5.1 and below": ("bfloat16", "float32", "float64")}, "paddle"
+ {"2.5.2 and below": ("bfloat16", "float32", "float64")}, "paddle"
)
@to_ivy_arrays_and_back
def floor(x, name=None):
return ivy.floor(x)
-@with_supported_dtypes({"2.5.1 and below": ("float32", "float64")}, "paddle")
+@with_supported_dtypes({"2.5.2 and below": ("float32", "float64")}, "paddle")
@to_ivy_arrays_and_back
def floor_divide(x, y, name=None):
return ivy.floor_divide(x, y)
@@ -234,7 +273,7 @@ def floor_divide(x, y, name=None):
@with_supported_device_and_dtypes(
{
- "2.5.1 and below": {
+ "2.5.2 and below": {
"cpu": ("float32", "float64", "int32", "int64"),
"gpu": ("float16", "float32", "float64", "int32", "int64"),
}
@@ -246,20 +285,20 @@ def floor_mod(x, y, name=None):
return ivy.remainder(x, y)
-@with_unsupported_dtypes({"2.5.1 and below": "bfloat16"}, "paddle")
+@with_unsupported_dtypes({"2.5.2 and below": "bfloat16"}, "paddle")
@to_ivy_arrays_and_back
def fmax(x, y, name=None):
return ivy.fmax(x, y)
-@with_unsupported_dtypes({"2.5.1 and below": "bfloat16"}, "paddle")
+@with_unsupported_dtypes({"2.5.2 and below": "bfloat16"}, "paddle")
@to_ivy_arrays_and_back
def fmin(x, y, name=None):
return ivy.fmin(x, y)
@with_supported_dtypes(
- {"2.5.1 and below": ("float32", "float64", "int32", "int64")}, "paddle"
+ {"2.5.2 and below": ("float32", "float64", "int32", "int64")}, "paddle"
)
@to_ivy_arrays_and_back
def frac(x, name=None):
@@ -267,21 +306,21 @@ def frac(x, name=None):
return ivy.subtract(x, y)
-@with_supported_dtypes({"2.5.1 and below": ("int32", "int64")}, "paddle")
+@with_supported_dtypes({"2.5.2 and below": ("int32", "int64")}, "paddle")
@to_ivy_arrays_and_back
def gcd(x, y, name=None):
return ivy.gcd(x, y)
@with_supported_dtypes(
- {"2.5.1 and below": ("float16", "float32", "float64", "int32", "int64")}, "paddle"
+ {"2.5.2 and below": ("float16", "float32", "float64", "int32", "int64")}, "paddle"
)
@to_ivy_arrays_and_back
def heaviside(x, y, name=None):
return ivy.heaviside(x, y)
-@with_supported_dtypes({"2.5.1 and below": ("float32", "float64")}, "paddle")
+@with_supported_dtypes({"2.5.2 and below": ("float32", "float64")}, "paddle")
@to_ivy_arrays_and_back
def inner(x, y, name=None):
result = ivy.inner(x, y)
@@ -292,8 +331,14 @@ def inner(x, y, name=None):
return result
+@with_supported_dtypes({"2.5.2 and below": ("float32", "float64")}, "paddle")
+@to_ivy_arrays_and_back
+def inverse(x, name=None):
+ return ivy.inv(x)
+
+
@with_supported_dtypes(
- {"2.5.1 and below": ("float16", "float32", "float64", "int32", "int64")}, "paddle"
+ {"2.5.2 and below": ("float16", "float32", "float64", "int32", "int64")}, "paddle"
)
@to_ivy_arrays_and_back
def isfinite(x, name=None):
@@ -301,7 +346,7 @@ def isfinite(x, name=None):
@with_supported_dtypes(
- {"2.5.1 and below": ("float16", "float32", "float64", "int32", "int64")}, "paddle"
+ {"2.5.2 and below": ("float16", "float32", "float64", "int32", "int64")}, "paddle"
)
@to_ivy_arrays_and_back
def isinf(x, name=None):
@@ -309,7 +354,7 @@ def isinf(x, name=None):
@with_supported_dtypes(
- {"2.5.1 and below": ("float16", "float32", "float64", "int32", "int64")}, "paddle"
+ {"2.5.2 and below": ("float16", "float32", "float64", "int32", "int64")}, "paddle"
)
@to_ivy_arrays_and_back
def isnan(x, name=None):
@@ -317,63 +362,63 @@ def isnan(x, name=None):
@with_supported_dtypes(
- {"2.5.1 and below": ("float16", "float32", "float64", "int32", "int64")}, "paddle"
+ {"2.5.2 and below": ("float16", "float32", "float64", "int32", "int64")}, "paddle"
)
@to_ivy_arrays_and_back
def kron(x, y, name=None):
return ivy.kron(x, y)
-@with_supported_dtypes({"2.5.1 and below": ("int32", "int64")}, "paddle")
+@with_supported_dtypes({"2.5.2 and below": ("int32", "int64")}, "paddle")
@to_ivy_arrays_and_back
def lcm(x, y, name=None):
return ivy.lcm(x, y)
-@with_supported_dtypes({"2.5.1 and below": ("float32", "float64")}, "paddle")
+@with_supported_dtypes({"2.5.2 and below": ("float32", "float64")}, "paddle")
@to_ivy_arrays_and_back
def lerp(x, y, weight, name=None):
return ivy.lerp(x, y, weight)
-@with_supported_dtypes({"2.5.1 and below": ("float32", "float64")}, "paddle")
+@with_supported_dtypes({"2.5.2 and below": ("float32", "float64")}, "paddle")
@to_ivy_arrays_and_back
def lgamma(x, name=None):
return ivy.lgamma(x)
-@with_unsupported_dtypes({"2.5.1 and below": ("float16", "bfloat16")}, "paddle")
+@with_unsupported_dtypes({"2.5.2 and below": ("float16", "bfloat16")}, "paddle")
@to_ivy_arrays_and_back
def log(x, name=None):
return ivy.log(x)
-@with_supported_dtypes({"2.5.1 and below": ("float32", "float64")}, "paddle")
+@with_supported_dtypes({"2.5.2 and below": ("float32", "float64")}, "paddle")
@to_ivy_arrays_and_back
def log10(x, name=None):
return ivy.log10(x)
-@with_supported_dtypes({"2.5.1 and below": ("float32", "float64")}, "paddle")
+@with_supported_dtypes({"2.5.2 and below": ("float32", "float64")}, "paddle")
@to_ivy_arrays_and_back
def log1p(x, name=None):
return ivy.log1p(x)
-@with_supported_dtypes({"2.5.1 and below": ("float32", "float64")}, "paddle")
+@with_supported_dtypes({"2.5.2 and below": ("float32", "float64")}, "paddle")
@to_ivy_arrays_and_back
def log2(x, name=None):
return ivy.log2(x)
-@with_unsupported_dtypes({"2.5.1 and below": ("float16", "bfloat16")}, "paddle")
+@with_unsupported_dtypes({"2.5.2 and below": ("float16", "bfloat16")}, "paddle")
@to_ivy_arrays_and_back
def logit(x, eps=None, name=None):
return ivy.logit(x, eps=eps)
@with_supported_dtypes(
- {"2.5.1 and below": ("float32", "float64", "int32", "int64")}, "paddle"
+ {"2.5.2 and below": ("float32", "float64", "int32", "int64")}, "paddle"
)
@to_ivy_arrays_and_back
def max(x, axis=None, keepdim=False, name=None):
@@ -381,14 +426,14 @@ def max(x, axis=None, keepdim=False, name=None):
# maximum
-@with_unsupported_dtypes({"2.5.1 and below": ("float16", "bfloat16")}, "paddle")
+@with_unsupported_dtypes({"2.5.2 and below": ("float16", "bfloat16")}, "paddle")
@to_ivy_arrays_and_back
def maximum(x, y, name=None):
return ivy.maximum(x, y)
@with_supported_dtypes(
- {"2.5.1 and below": ("float32", "float64", "int32", "int64")}, "paddle"
+ {"2.5.2 and below": ("float32", "float64", "int32", "int64")}, "paddle"
)
@to_ivy_arrays_and_back
def min(x, axis=None, keepdim=False, name=None):
@@ -396,7 +441,7 @@ def min(x, axis=None, keepdim=False, name=None):
@with_supported_dtypes(
- {"2.5.1 and below": ("float32", "float64", "int32", "int64")}, "paddle"
+ {"2.5.2 and below": ("float32", "float64", "int32", "int64")}, "paddle"
)
@to_ivy_arrays_and_back
def minimum(x, y, name=None):
@@ -404,27 +449,27 @@ def minimum(x, y, name=None):
@with_supported_dtypes(
- {"2.5.1 and below": ("float32", "float64", "int32", "int64")}, "paddle"
+ {"2.5.2 and below": ("float32", "float64", "int32", "int64")}, "paddle"
)
@to_ivy_arrays_and_back
def mm(input, mat2, name=None):
return ivy.matmul(input, mat2)
-@with_unsupported_dtypes({"2.5.1 and below": ("float16", "bfloat16")}, "paddle")
+@with_unsupported_dtypes({"2.5.2 and below": ("float16", "bfloat16")}, "paddle")
@to_ivy_arrays_and_back
def multiply(x, y, name=None):
return ivy.multiply(x, y)
-@with_supported_dtypes({"2.5.1 and below": ("float32", "float64")}, "paddle")
+@with_supported_dtypes({"2.5.2 and below": ("float32", "float64")}, "paddle")
@to_ivy_arrays_and_back
def nanmean(x, axis=None, keepdims=False):
return ivy.nanmean(x, axis=axis, keepdims=keepdims)
@with_supported_dtypes(
- {"2.5.1 and below": ("float32", "float64", "int32", "int64")}, "paddle"
+ {"2.5.2 and below": ("float32", "float64", "int32", "int64")}, "paddle"
)
@to_ivy_arrays_and_back
def nansum(x, axis=None, dtype=None, name=None):
@@ -432,7 +477,7 @@ def nansum(x, axis=None, dtype=None, name=None):
@with_supported_dtypes(
- {"2.5.1 and below": ("float32", "float64", "int8", "int16", "int32", "int64")},
+ {"2.5.2 and below": ("float32", "float64", "int8", "int16", "int32", "int64")},
"paddle",
)
@to_ivy_arrays_and_back
@@ -440,101 +485,109 @@ def neg(x, name=None):
return ivy.negative(x)
-@with_supported_dtypes({"2.5.1 and below": ("float32", "float64")}, "paddle")
+@with_supported_dtypes({"2.5.2 and below": ("float32", "float64")}, "paddle")
@to_ivy_arrays_and_back
def outer(x, y, name=None):
return ivy.outer(x, y)
-@with_unsupported_dtypes({"2.5.1 and below": ("float16", "bfloat16")}, "paddle")
+@with_unsupported_dtypes({"2.5.2 and below": ("float16", "bfloat16")}, "paddle")
@to_ivy_arrays_and_back
def pow(x, y, name=None):
return ivy.pow(x, y)
@with_supported_dtypes(
- {"2.5.1 and below": ("float32", "float64", "int32", "int64")}, "paddle"
+ {"2.5.2 and below": ("float32", "float64", "int32", "int64")}, "paddle"
)
@to_ivy_arrays_and_back
def prod(x, axis=None, keepdim=False, dtype=None, name=None):
return ivy.prod(x, axis=axis, keepdims=keepdim, dtype=dtype)
-@with_unsupported_dtypes({"2.5.1 and below": ("float16", "bfloat16")}, "paddle")
+@with_unsupported_dtypes({"2.5.2 and below": ("float16", "bfloat16")}, "paddle")
@to_ivy_arrays_and_back
def rad2deg(x, name=None):
return ivy.rad2deg(x)
-@with_unsupported_dtypes({"2.5.1 and below": ("float16", "bfloat16")}, "paddle")
+@with_unsupported_dtypes({"2.5.2 and below": ("float16", "bfloat16")}, "paddle")
@to_ivy_arrays_and_back
def reciprocal(x, name=None):
return ivy.reciprocal(x)
-@with_supported_dtypes(
- {"2.5.1 and below": ("float32", "float64", "int32", "int64")}, "paddle"
-)
+@with_unsupported_dtypes({"2.5.2 and below": ("float16", "bfloat16")}, "paddle")
@to_ivy_arrays_and_back
def remainder(x, y, name=None):
return ivy.remainder(x, y)
-@with_unsupported_dtypes({"2.5.1 and below": ("float16", "bfloat16")}, "paddle")
+@with_supported_device_and_dtypes(
+ {
+ "2.5.2 and below": {
+ "cpu": ("float32", "float64"),
+ "gpu": ("float16", "float32", "float64"),
+ }
+ },
+ "paddle",
+)
@to_ivy_arrays_and_back
def remainder_(x, y, name=None):
return ivy.inplace_update(x, remainder(x, y))
-@with_unsupported_dtypes({"2.5.1 and below": ("float16", "bfloat16")}, "paddle")
+@with_unsupported_dtypes({"2.5.2 and below": ("float16", "bfloat16")}, "paddle")
@to_ivy_arrays_and_back
def round(x, name=None):
- return ivy.round(x)
+ sign = ivy.sign(x)
+ x = sign * ivy.floor(ivy.abs(x) + 0.5)
+ return x
-@with_supported_dtypes({"2.5.1 and below": ("float32", "float64")}, "paddle")
+@with_supported_dtypes({"2.5.2 and below": ("float32", "float64")}, "paddle")
@to_ivy_arrays_and_back
def rsqrt(x, name=None):
return 1 / ivy.sqrt(x)
-@with_unsupported_dtypes({"2.5.1 and below": ("float16", "bfloat16")}, "paddle")
+@with_unsupported_dtypes({"2.5.2 and below": ("float16", "bfloat16")}, "paddle")
@to_ivy_arrays_and_back
def sgn(x, name=None):
return ivy.sign(x, np_variant=True)
-@with_unsupported_dtypes({"2.5.1 and below": ("float16", "bfloat16")}, "paddle")
+@with_unsupported_dtypes({"2.5.2 and below": ("float16", "bfloat16")}, "paddle")
@to_ivy_arrays_and_back
def sign(x, name=None):
return ivy.sign(x, np_variant=False)
-@with_unsupported_dtypes({"2.5.1 and below": ("float16", "bfloat16")}, "paddle")
+@with_unsupported_dtypes({"2.5.2 and below": ("float16", "bfloat16")}, "paddle")
@to_ivy_arrays_and_back
def sin(x, name=None):
return ivy.sin(x)
-@with_supported_dtypes({"2.5.1 and below": ("float32", "float64")}, "paddle")
+@with_supported_dtypes({"2.5.2 and below": ("float32", "float64")}, "paddle")
@to_ivy_arrays_and_back
def sinh(x, name=None):
return ivy.sinh(x)
-@with_supported_dtypes({"2.5.1 and below": ("float32", "float64")}, "paddle")
+@with_supported_dtypes({"2.5.2 and below": ("float32", "float64")}, "paddle")
@to_ivy_arrays_and_back
def sqrt(x, name=None):
return ivy.sqrt(x)
-@with_supported_dtypes({"2.5.1 and below": ("float32", "float64")}, "paddle")
+@with_supported_dtypes({"2.5.2 and below": ("float32", "float64")}, "paddle")
@to_ivy_arrays_and_back
def square(x, name=None):
return ivy.square(x)
-@with_supported_dtypes({"2.5.1 and below": ("float32", "float64")}, "paddle")
+@with_supported_dtypes({"2.5.2 and below": ("float32", "float64")}, "paddle")
@to_ivy_arrays_and_back
def stanh(x, scale_a=0.67, scale_b=1.7159, name=None):
# TODO this function will be simplified as soon as the ivy.stanh(x,a,b) is added
@@ -546,7 +599,7 @@ def stanh(x, scale_a=0.67, scale_b=1.7159, name=None):
return ret
-@with_unsupported_dtypes({"2.5.1 and below": ("float16", "bfloat16")}, "paddle")
+@with_unsupported_dtypes({"2.5.2 and below": ("float16", "bfloat16")}, "paddle")
@to_ivy_arrays_and_back
def subtract(x, y, name=None):
return ivy.subtract(x, y)
@@ -554,7 +607,7 @@ def subtract(x, y, name=None):
@with_supported_dtypes(
{
- "2.5.1 and below": (
+ "2.5.2 and below": (
"float64",
"int64",
)
@@ -572,7 +625,7 @@ def sum(x, axis=None, dtype=None, keepdim=False, name=None):
@with_supported_dtypes(
- {"2.5.1 and below": ("float32", "float64", "int32", "int6")}, "paddle"
+ {"2.5.2 and below": ("float32", "float64", "int32", "int6")}, "paddle"
)
@to_ivy_arrays_and_back
def take(
@@ -594,20 +647,20 @@ def take(
return ivy.gather(x, index, axis=0)
-@with_unsupported_dtypes({"2.5.1 and below": ("float16", "bfloat16")}, "paddle")
+@with_unsupported_dtypes({"2.5.2 and below": ("float16", "bfloat16")}, "paddle")
@to_ivy_arrays_and_back
def tan(x, name=None):
return ivy.tan(x)
-@with_supported_dtypes({"2.5.1 and below": ("float32", "float64")}, "paddle")
+@with_supported_dtypes({"2.5.2 and below": ("float32", "float64")}, "paddle")
@to_ivy_arrays_and_back
def tanh(x, name=None):
return ivy.tanh(x)
@with_supported_dtypes(
- {"2.5.1 and below": ("int32", "int64", "float32", "float64")}, "paddle"
+ {"2.5.2 and below": ("int32", "int64", "float32", "float64")}, "paddle"
)
@to_ivy_arrays_and_back
def trace(x, offset=0, axis1=0, axis2=1, name=None):
diff --git a/ivy/functional/frontends/paddle/nn/functional/activation.py b/ivy/functional/frontends/paddle/nn/functional/activation.py
index 761b070309f67..f8621b7f3f998 100644
--- a/ivy/functional/frontends/paddle/nn/functional/activation.py
+++ b/ivy/functional/frontends/paddle/nn/functional/activation.py
@@ -8,7 +8,7 @@
tanh = paddle_tanh
-@with_supported_dtypes({"2.5.1 and below": ("float32", "float64")}, "paddle")
+@with_supported_dtypes({"2.5.2 and below": ("float32", "float64")}, "paddle")
@to_ivy_arrays_and_back
def celu(
x,
@@ -17,12 +17,10 @@ def celu(
alpha=1.0,
name=None,
):
- prod = alpha * (ivy.exp(x / alpha) - 1)
- ret = ivy.maximum(0, x) + ivy.minimum(0, prod)
- return ret
+ return ivy.celu(x, alpha=alpha)
-@with_supported_dtypes({"2.5.1 and below": ("float32", "float64")}, "paddle")
+@with_supported_dtypes({"2.5.2 and below": ("float32", "float64")}, "paddle")
@to_ivy_arrays_and_back
def elu(
x,
@@ -34,13 +32,13 @@ def elu(
return ivy.elu(x, alpha=alpha)
-@with_supported_dtypes({"2.5.1 and below": ("float32", "float64")}, "paddle")
+@with_supported_dtypes({"2.5.2 and below": ("float32", "float64")}, "paddle")
@to_ivy_arrays_and_back
def gelu(x, approximate=False, name=None):
return ivy.gelu(x, approximate=approximate)
-@with_supported_dtypes({"2.5.1 and below": ("float32", "float64")}, "paddle")
+@with_supported_dtypes({"2.5.2 and below": ("float32", "float64")}, "paddle")
@to_ivy_arrays_and_back
def glu(x, axis=-1, name=None):
size = x.shape[axis]
@@ -65,21 +63,21 @@ def gumbel_softmax(x, temperature=1.0, hard=False, axis=-1, name=None):
return y_soft
-@with_supported_dtypes({"2.5.1 and below": ("float32", "float64")}, "paddle")
+@with_supported_dtypes({"2.5.2 and below": ("float32", "float64")}, "paddle")
@to_ivy_arrays_and_back
def hardshrink(x, threshold=0.5, name=None):
mask = ivy.logical_or(ivy.greater(x, threshold), ivy.less(x, -threshold))
return ivy.where(mask, x, 0.0)
-@with_supported_dtypes({"2.5.1 and below": ("float32", "float64")}, "paddle")
+@with_supported_dtypes({"2.5.2 and below": ("float32", "float64")}, "paddle")
@to_ivy_arrays_and_back
def hardsigmoid(x, slope=0.1666667, offset=0.5, name=None):
ret = ivy.minimum(ivy.maximum(ivy.add(ivy.multiply(x, slope), offset), 0), 1)
return ret
-@with_supported_dtypes({"2.5.1 and below": ("float32", "float64")}, "paddle")
+@with_supported_dtypes({"2.5.2 and below": ("float32", "float64")}, "paddle")
@to_ivy_arrays_and_back
def hardswish(x, name=None):
relu6_val = ivy.relu6(ivy.add(x, 3))
@@ -87,7 +85,7 @@ def hardswish(x, name=None):
return ret
-@with_supported_dtypes({"2.5.1 and below": ("float32", "float64")}, "paddle")
+@with_supported_dtypes({"2.5.2 and below": ("float32", "float64")}, "paddle")
@to_ivy_arrays_and_back
def hardtanh(
x,
@@ -108,13 +106,13 @@ def leaky_relu(x, negative_slope=0.01, name=None):
return ivy.leaky_relu(x)
-@with_supported_dtypes({"2.5.1 and below": ("float32", "float64")}, "paddle")
+@with_supported_dtypes({"2.5.2 and below": ("float32", "float64")}, "paddle")
@to_ivy_arrays_and_back
def log_sigmoid(x, name=None):
return -ivy.softplus(-x)
-@with_supported_dtypes({"2.5.1 and below": ("float32", "float64")}, "paddle")
+@with_supported_dtypes({"2.5.2 and below": ("float32", "float64")}, "paddle")
@to_ivy_arrays_and_back
def log_softmax(x, axis=-1, dtype=None, name=None):
x = ivy.astype(x, dtype) if dtype else x
@@ -123,31 +121,31 @@ def log_softmax(x, axis=-1, dtype=None, name=None):
return ret
-@with_supported_dtypes({"2.5.1 and below": ("float32", "float64")}, "paddle")
+@with_supported_dtypes({"2.5.2 and below": ("float32", "float64")}, "paddle")
@to_ivy_arrays_and_back
def mish(x, name=None):
return ivy.mish(x)
-@with_supported_dtypes({"2.5.1 and below": ("float32", "float64")}, "paddle")
+@with_supported_dtypes({"2.5.2 and below": ("float32", "float64")}, "paddle")
@to_ivy_arrays_and_back
def prelu(x, weight, data_format="NCHW", name=None):
return ivy.add(ivy.maximum(0, x), ivy.multiply(weight, ivy.minimum(0, x)))
-@with_supported_dtypes({"2.5.1 and below": ("float32", "float64")}, "paddle")
+@with_supported_dtypes({"2.5.2 and below": ("float32", "float64")}, "paddle")
@to_ivy_arrays_and_back
def relu(x, name=None):
return ivy.relu(x)
-@with_supported_dtypes({"2.5.1 and below": ("float32", "float64")}, "paddle")
+@with_supported_dtypes({"2.5.2 and below": ("float32", "float64")}, "paddle")
@to_ivy_arrays_and_back
def relu6(x, name=None):
return ivy.relu6(x)
-@with_supported_dtypes({"2.5.1 and below": ("float32", "float64")}, "paddle")
+@with_supported_dtypes({"2.5.2 and below": ("float32", "float64")}, "paddle")
@to_ivy_arrays_and_back
def relu_(x, name=None):
ret = ivy.relu(x)
@@ -155,7 +153,7 @@ def relu_(x, name=None):
return x
-@with_supported_dtypes({"2.5.1 and below": ("float32", "float64")}, "paddle")
+@with_supported_dtypes({"2.5.2 and below": ("float32", "float64")}, "paddle")
@to_ivy_arrays_and_back
def rrelu(
x,
@@ -191,7 +189,7 @@ def rrelu(
return out.astype(x.dtype)
-@with_supported_dtypes({"2.5.1 and below": ("float32", "float64")}, "paddle")
+@with_supported_dtypes({"2.5.2 and below": ("float32", "float64")}, "paddle")
@to_ivy_arrays_and_back
def selu(
x,
@@ -224,13 +222,13 @@ def softmax_(x, axis=-1, dtype=None, name=None):
return x
-@with_supported_dtypes({"2.5.1 and below": ("float32", "float64")}, "paddle")
+@with_supported_dtypes({"2.5.2 and below": ("float32", "float64")}, "paddle")
@to_ivy_arrays_and_back
def softplus(x, beta=1, threshold=20, name=None):
return ivy.softplus(x, beta=beta, threshold=threshold)
-@with_supported_dtypes({"2.5.1 and below": ("float32", "float64")}, "paddle")
+@with_supported_dtypes({"2.5.2 and below": ("float32", "float64")}, "paddle")
@to_ivy_arrays_and_back
def softshrink(
x,
@@ -245,7 +243,7 @@ def softshrink(
return ivy.astype(add, x.dtype)
-@with_supported_dtypes({"2.5.1 and below": ("float32", "float64")}, "paddle")
+@with_supported_dtypes({"2.5.2 and below": ("float32", "float64")}, "paddle")
@to_ivy_arrays_and_back
def softsign(
x,
@@ -256,7 +254,7 @@ def softsign(
return ivy.divide(x, ivy.add(1, ivy.abs(x)))
-@with_supported_dtypes({"2.5.1 and below": ("float32", "float64")}, "paddle")
+@with_supported_dtypes({"2.5.2 and below": ("float32", "float64")}, "paddle")
@to_ivy_arrays_and_back
def swish(x, name=None):
return ivy.multiply(x, ivy.sigmoid(x))
@@ -275,7 +273,7 @@ def tanh_(x, name=None):
# return ret.astype(x.dtype)
-@with_supported_dtypes({"2.5.1 and below": ("float32", "float64")}, "paddle")
+@with_supported_dtypes({"2.5.2 and below": ("float32", "float64")}, "paddle")
@to_ivy_arrays_and_back
def tanhshrink(
x,
diff --git a/ivy/functional/frontends/paddle/nn/functional/common.py b/ivy/functional/frontends/paddle/nn/functional/common.py
index 160351f666177..d43f6a42a93bc 100644
--- a/ivy/functional/frontends/paddle/nn/functional/common.py
+++ b/ivy/functional/frontends/paddle/nn/functional/common.py
@@ -5,7 +5,7 @@
@to_ivy_arrays_and_back
-@with_supported_dtypes({"2.5.1 and below": ("float32", "float64")}, "paddle")
+@with_supported_dtypes({"2.5.2 and below": ("float32", "float64")}, "paddle")
def cosine_similarity(x1, x2, *, axis=1, eps=1e-08):
if len(x1.shape) == len(x2.shape) and len(x2.shape) >= 2:
numerator = ivy.sum(x1 * x2, axis=axis)
@@ -26,9 +26,9 @@ def cosine_similarity(x1, x2, *, axis=1, eps=1e-08):
@to_ivy_arrays_and_back
-@with_supported_dtypes({"2.5.1 and below": ("float32", "float64")}, "paddle")
+@with_supported_dtypes({"2.5.2 and below": ("float32", "float64")}, "paddle")
def dropout(x, p=0.5, axis=None, training=True, mode="upscale_in_train", name=None):
- if axis > 1:
+ if axis is not None and axis > 1:
raise ValueError("Axis value can only be 0 or 1 or None.")
elif axis is None or (isinstance(axis, list) and len(axis) == 2):
mask = get_mask(shape=x.shape, device=ivy.dev(x), prob=p, seed=None)
@@ -53,13 +53,13 @@ def dropout(x, p=0.5, axis=None, training=True, mode="upscale_in_train", name=No
@to_ivy_arrays_and_back
-@with_supported_dtypes({"2.5.1 and below": ("float32", "float64")}, "paddle")
+@with_supported_dtypes({"2.5.2 and below": ("float32", "float64")}, "paddle")
def dropout2d(x, *, p=0.5, training=True, data_format="NCHW", name=None):
return ivy.dropout2d(x, p=p, training=training, data_format=data_format)
@to_ivy_arrays_and_back
-@with_supported_dtypes({"2.5.1 and below": ("float32", "float64")}, "paddle")
+@with_supported_dtypes({"2.5.2 and below": ("float32", "float64")}, "paddle")
def dropout3d(x, p=0.5, training=True, data_format="NCDHW", name=None):
return ivy.dropout3d(x, p, training=training, data_format=data_format)
@@ -74,7 +74,7 @@ def get_mask(shape, device, prob, seed=None):
@to_ivy_arrays_and_back
-@with_supported_dtypes({"2.5.1 and below": ("float32", "float64")}, "paddle")
+@with_supported_dtypes({"2.5.2 and below": ("float32", "float64")}, "paddle")
def interpolate(
x,
size=None,
@@ -91,19 +91,19 @@ def interpolate(
@to_ivy_arrays_and_back
-@with_supported_dtypes({"2.5.1 and below": ("float32", "float64")}, "paddle")
+@with_supported_dtypes({"2.5.2 and below": ("float32", "float64")}, "paddle")
def linear(x, weight, bias=None, name=None):
weight = ivy.swapaxes(weight, -1, -2)
return ivy.linear(x, weight, bias=bias)
@to_ivy_arrays_and_back
-@with_supported_dtypes({"2.5.1 and below": ("float32", "float64")}, "paddle")
+@with_supported_dtypes({"2.5.2 and below": ("float32", "float64")}, "paddle")
def unfold(x, kernel_sizes, strides=1, paddings=0, dilations=1, name=None):
# Input checking
if isinstance(kernel_sizes, int):
kernel_sizes = [kernel_sizes, kernel_sizes]
- elif not (isinstance(kernel_sizes, list) or isinstance(kernel_sizes, tuple)):
+ elif not (isinstance(kernel_sizes, (list, tuple))):
raise ivy.exceptions.IvyError(
"Expected kernel size input as type int, tuple or list but got"
f" {type(kernel_sizes)}"
@@ -111,14 +111,14 @@ def unfold(x, kernel_sizes, strides=1, paddings=0, dilations=1, name=None):
if isinstance(strides, int):
strides = [strides, strides]
- elif not (isinstance(strides, list) or isinstance(strides, tuple)):
+ elif not (isinstance(strides, (list, tuple))):
raise ivy.exceptions.IvyError(
f"Expected strides input as type int, tuple or list but got {type(strides)}"
)
if isinstance(dilations, int):
dilations = [dilations, dilations]
- elif not (isinstance(dilations, list) or isinstance(dilations, tuple)):
+ elif not (isinstance(dilations, (list, tuple))):
raise ivy.exceptions.IvyError(
"Expected dilations input as type int, tuple or list but got"
f" {type(dilations)}"
@@ -126,7 +126,7 @@ def unfold(x, kernel_sizes, strides=1, paddings=0, dilations=1, name=None):
if isinstance(paddings, int):
paddings = [paddings, paddings]
- elif not (isinstance(paddings, list) or isinstance(paddings, tuple)):
+ elif not (isinstance(paddings, (list, tuple))):
raise ivy.exceptions.IvyError(
"Expected paddings, input as type int, tuple or list but got"
f" {type(paddings)}"
@@ -178,7 +178,7 @@ def unfold(x, kernel_sizes, strides=1, paddings=0, dilations=1, name=None):
@to_ivy_arrays_and_back
-@with_supported_dtypes({"2.5.1 and below": ("float32", "float64")}, "paddle")
+@with_supported_dtypes({"2.5.2 and below": ("float32", "float64")}, "paddle")
def zeropad2d(x, padding, data_format="NCHW", name=None):
if ivy.is_array(padding):
padding = padding.to_list()
diff --git a/ivy/functional/frontends/paddle/nn/functional/conv.py b/ivy/functional/frontends/paddle/nn/functional/conv.py
index b9a0f4a37335f..296ba22e4237c 100644
--- a/ivy/functional/frontends/paddle/nn/functional/conv.py
+++ b/ivy/functional/frontends/paddle/nn/functional/conv.py
@@ -79,7 +79,7 @@ def _conv_transpose(
# ------------ #
-@with_supported_dtypes({"2.5.1 and below": ("float32", "float64")}, "paddle")
+@with_supported_dtypes({"2.5.2 and below": ("float32", "float64")}, "paddle")
@to_ivy_arrays_and_back
def conv1d(
x,
@@ -95,7 +95,7 @@ def conv1d(
return _conv(x, weight, bias, stride, padding, dilation, groups, data_format)
-@with_supported_dtypes({"2.5.1 and below": ("float32", "float64")}, "paddle")
+@with_supported_dtypes({"2.5.2 and below": ("float32", "float64")}, "paddle")
@to_ivy_arrays_and_back
def conv1d_transpose(
x,
@@ -115,7 +115,7 @@ def conv1d_transpose(
)
-@with_supported_dtypes({"2.5.1 and below": ("float32", "float64")}, "paddle")
+@with_supported_dtypes({"2.5.2 and below": ("float32", "float64")}, "paddle")
@to_ivy_arrays_and_back
def conv2d(
x,
@@ -131,7 +131,7 @@ def conv2d(
return _conv(x, weight, bias, stride, padding, dilation, groups, data_format)
-@with_supported_dtypes({"2.5.1 and below": ("float32", "float64")}, "paddle")
+@with_supported_dtypes({"2.5.2 and below": ("float32", "float64")}, "paddle")
@to_ivy_arrays_and_back
def conv2d_transpose(
x,
@@ -151,7 +151,7 @@ def conv2d_transpose(
)
-@with_supported_dtypes({"2.5.1 and below": ("float32", "float64")}, "paddle")
+@with_supported_dtypes({"2.5.2 and below": ("float32", "float64")}, "paddle")
@to_ivy_arrays_and_back
def conv3d(
x,
@@ -167,7 +167,7 @@ def conv3d(
return _conv(x, weight, bias, stride, padding, dilation, groups, data_format)
-@with_supported_dtypes({"2.5.1 and below": ("float32", "float64")}, "paddle")
+@with_supported_dtypes({"2.5.2 and below": ("float32", "float64")}, "paddle")
@to_ivy_arrays_and_back
def conv3d_transpose(
x,
diff --git a/ivy/functional/frontends/paddle/nn/functional/loss.py b/ivy/functional/frontends/paddle/nn/functional/loss.py
index 181383b7bfeef..52d07db32110b 100644
--- a/ivy/functional/frontends/paddle/nn/functional/loss.py
+++ b/ivy/functional/frontends/paddle/nn/functional/loss.py
@@ -47,11 +47,11 @@ def _pairwise_distance(x1, x2, *, p=2.0, eps=1e-06, keepdim=False):
# ------------ #
-@with_supported_dtypes({"2.5.1 and below": ("float32", "float64")}, "paddle")
+@with_supported_dtypes({"2.5.2 and below": ("float32", "float64")}, "paddle")
@to_ivy_arrays_and_back
def binary_cross_entropy(input, label, weight=None, reduction="mean", name=None):
reduction = _get_reduction_func(reduction)
- result = ivy.binary_cross_entropy(label, input, epsilon=0.0)
+ result = ivy.binary_cross_entropy(label, input, epsilon=0.0, reduction="none")
if weight is not None:
result = ivy.multiply(weight, result)
result = reduction(result)
@@ -59,7 +59,7 @@ def binary_cross_entropy(input, label, weight=None, reduction="mean", name=None)
@with_supported_dtypes(
- {"2.5.1 and below": ("float32",)},
+ {"2.5.2 and below": ("float32",)},
"paddle",
)
@inputs_to_ivy_arrays
@@ -83,7 +83,7 @@ def binary_cross_entropy_with_logits(
@handle_exceptions
@to_ivy_arrays_and_back
-@with_supported_dtypes({"2.5.1 and below": ("float32", "float64")}, "paddle")
+@with_supported_dtypes({"2.5.2 and below": ("float32", "float64")}, "paddle")
def cosine_embedding_loss(
input1, input2, label, margin=0.0, reduction="mean", name=None
):
@@ -124,7 +124,7 @@ def cosine_embedding_loss(
return out
-@with_supported_dtypes({"2.5.1 and below": ("float32", "float64")}, "paddle")
+@with_supported_dtypes({"2.5.2 and below": ("float32", "float64")}, "paddle")
@to_ivy_arrays_and_back
def dice_loss(input, label, epsilon=0.00001, name=None):
ivy.assertions.check_true(
@@ -164,15 +164,15 @@ def dice_loss(input, label, epsilon=0.00001, name=None):
@with_supported_dtypes(
- {"2.5.1 and below": ("float32",)},
+ {"2.5.2 and below": ("float32",)},
"paddle",
)
@to_ivy_arrays_and_back
def hinge_embedding_loss(input, label, margin=1.0, reduction="mean"):
if reduction not in ["sum", "mean", "none"]:
raise ValueError(
- "'reduction' in 'hinge_embedding_loss' should be 'sum', 'mean' or 'none', "
- "but received {}.".format(reduction)
+ "'reduction' in 'hinge_embedding_loss' should be 'sum', 'mean' or 'none',"
+ f" but received {reduction}."
)
zero_ = ivy.zeros([1], dtype=input.dtype)
@@ -188,7 +188,7 @@ def hinge_embedding_loss(input, label, margin=1.0, reduction="mean"):
return loss
-@with_supported_dtypes({"2.5.1 and below": ("float32", "float64")}, "paddle")
+@with_supported_dtypes({"2.5.2 and below": ("float32", "float64")}, "paddle")
@to_ivy_arrays_and_back
def kl_div(
input,
@@ -235,7 +235,7 @@ def l1_loss(
@with_supported_dtypes(
- {"2.5.1 and below": ("float32",)},
+ {"2.5.2 and below": ("float32",)},
"paddle",
)
@to_ivy_arrays_and_back
@@ -246,7 +246,7 @@ def log_loss(input, label, epsilon=0.0001, name=None):
return out
-@with_supported_dtypes({"2.5.1 and below": ("float32", "float64")}, "paddle")
+@with_supported_dtypes({"2.5.2 and below": ("float32", "float64")}, "paddle")
@to_ivy_arrays_and_back
def margin_ranking_loss(input, other, label, margin=0.0, reduction="mean", name=None):
reduction = _get_reduction_func(reduction)
@@ -266,7 +266,7 @@ def margin_ranking_loss(input, other, label, margin=0.0, reduction="mean", name=
return out
-@with_supported_dtypes({"2.5.1 and below": ("float32", "float64")}, "paddle")
+@with_supported_dtypes({"2.5.2 and below": ("float32", "float64")}, "paddle")
@inputs_to_ivy_arrays
def mse_loss(input, label, reduction="mean", name=None):
reduction = _get_reduction_func(reduction)
@@ -276,7 +276,7 @@ def mse_loss(input, label, reduction="mean", name=None):
return paddle.to_tensor(ret)
-@with_supported_dtypes({"2.5.1 and below": ("float32", "float64")}, "paddle")
+@with_supported_dtypes({"2.5.2 and below": ("float32", "float64")}, "paddle")
@to_ivy_arrays_and_back
def multi_label_soft_margin_loss(
input, label, weight=None, reduction="mean", name=None
@@ -294,7 +294,7 @@ def multi_label_soft_margin_loss(
return ret
-@with_supported_dtypes({"2.5.1 and below": ("float32", "float64")}, "paddle")
+@with_supported_dtypes({"2.5.2 and below": ("float32", "float64")}, "paddle")
@to_ivy_arrays_and_back
def nll_loss(
input,
@@ -327,7 +327,7 @@ def nll_loss(
return output
-@with_supported_dtypes({"2.5.1 and below": ("float32", "float64")}, "paddle")
+@with_supported_dtypes({"2.5.2 and below": ("float32", "float64")}, "paddle")
@to_ivy_arrays_and_back
def sigmoid_focal_loss(
logit,
@@ -373,7 +373,7 @@ def sigmoid_focal_loss(
return loss
-@with_supported_dtypes({"2.5.1 and below": ("float32", "float64")}, "paddle")
+@with_supported_dtypes({"2.5.2 and below": ("float32", "float64")}, "paddle")
@to_ivy_arrays_and_back
def smooth_l1_loss(
input,
@@ -400,7 +400,7 @@ def smooth_l1_loss(
@with_supported_dtypes(
- {"2.5.1 and below": ("float32", "float64")},
+ {"2.5.2 and below": ("float32", "float64")},
"paddle",
)
@inputs_to_ivy_arrays
@@ -415,12 +415,12 @@ def softmax_with_cross_entropy(
):
input_dims = len(list(logits.shape))
if input_dims == 0:
- raise ValueError("The dimention of input should be larger than zero!")
+ raise ValueError("The dimension of input should be larger than zero!")
label_dims = len(list(label.shape))
if input_dims - 1 != label_dims and input_dims != label_dims:
raise ValueError(
- "Expected nput_dims - 1 = label_dims or input_dims == label_dims "
- " (got nput_dims{}, label_dims{})".format(input_dims, label_dims)
+ "Expected nput_dims - 1 = label_dims or input_dims == label_dims "
+ f" (got nput_dims{input_dims}, label_dims{label_dims})"
)
logits = ivy.array(logits)
label = ivy.array(label)
@@ -460,13 +460,13 @@ def softmax_with_cross_entropy(
return paddle.to_tensor(loss)
-@with_supported_dtypes({"2.5.1 and below": ("float32",)}, "paddle")
+@with_supported_dtypes({"2.5.2 and below": ("float32",)}, "paddle")
@to_ivy_arrays_and_back
def square_error_cost(input, label):
return ivy.square(ivy.subtract(input, label))
-@with_supported_dtypes({"2.5.1 and below": ("float32", "float64")}, "paddle")
+@with_supported_dtypes({"2.5.2 and below": ("float32", "float64")}, "paddle")
@to_ivy_arrays_and_back
def triplet_margin_loss(
input,
diff --git a/ivy/functional/frontends/paddle/nn/functional/norm.py b/ivy/functional/frontends/paddle/nn/functional/norm.py
index 76bc210cabbb6..17e2aca071c79 100644
--- a/ivy/functional/frontends/paddle/nn/functional/norm.py
+++ b/ivy/functional/frontends/paddle/nn/functional/norm.py
@@ -5,13 +5,13 @@
@to_ivy_arrays_and_back
-@with_supported_dtypes({"2.5.1 and below": ("float32", "float64")}, "paddle")
+@with_supported_dtypes({"2.5.2 and below": ("float32", "float64")}, "paddle")
def layer_norm(x, normalized_shape, weight=None, bias=None, epsilon=1e-05, name=None):
return ivy.layer_norm(x, normalized_shape, weight, bias, epsilon)
@to_ivy_arrays_and_back
-@with_supported_dtypes({"2.5.1 and below": ("float32", "float64")}, "paddle")
+@with_supported_dtypes({"2.5.2 and below": ("float32", "float64")}, "paddle")
def normalize(x, p=2, axis=1, epsilon=1e-12, name=None):
if axis < 0:
axis = ivy.get_num_dims(x) + axis
diff --git a/ivy/functional/frontends/paddle/nn/functional/pooling.py b/ivy/functional/frontends/paddle/nn/functional/pooling.py
index b40b468ed7441..9d8e02c90b86e 100644
--- a/ivy/functional/frontends/paddle/nn/functional/pooling.py
+++ b/ivy/functional/frontends/paddle/nn/functional/pooling.py
@@ -9,13 +9,13 @@
@to_ivy_arrays_and_back
-@with_supported_dtypes({"2.5.1 and below": ("float32", "float64")}, "paddle")
+@with_supported_dtypes({"2.5.2 and below": ("float32", "float64")}, "paddle")
def adaptive_avg_pool1d(x, output_size, name=None):
return ivy.adaptive_avg_pool1d(x, output_size)
@to_ivy_arrays_and_back
-@with_supported_dtypes({"2.5.1 and below": ("float32", "float64")}, "paddle")
+@with_supported_dtypes({"2.5.2 and below": ("float32", "float64")}, "paddle")
def adaptive_avg_pool2d(x, output_size, data_format="NCHW", name=None):
return ivy.adaptive_avg_pool2d(x, output_size)
@@ -27,13 +27,13 @@ def adaptive_avg_pool3d(x, output_size, data_format="NCHW", name=None):
@to_ivy_arrays_and_back
-@with_supported_dtypes({"2.5.1 and below": ("float32", "float64")}, "paddle")
+@with_supported_dtypes({"2.5.2 and below": ("float32", "float64")}, "paddle")
def adaptive_max_pool2d(x, output_size, return_mask=None, name=None):
return ivy.adaptive_max_pool2d(x, output_size)
@to_ivy_arrays_and_back
-@with_unsupported_dtypes({"2.5.1 and below": ("float16", "bfloat16")}, "paddle")
+@with_unsupported_dtypes({"2.5.2 and below": ("float16", "bfloat16")}, "paddle")
def avg_pool1d(
x, kernel_size, stride=None, padding=0, exclusive=True, ceil_mode=False, name=None
):
@@ -45,7 +45,7 @@ def avg_pool1d(
padding = _broadcast_pooling_helper(padding, "1d", name="padding")
# Figure out padding string
if all(
- [pad == ivy.ceil((kernel - 1) / 2) for kernel, pad in zip(kernel_size, padding)]
+ pad == ivy.ceil((kernel - 1) / 2) for kernel, pad in zip(kernel_size, padding)
):
padding = "SAME"
else:
@@ -63,7 +63,7 @@ def avg_pool1d(
@to_ivy_arrays_and_back
-@with_supported_dtypes({"2.5.1 and below": ("float32", "float64")}, "paddle")
+@with_supported_dtypes({"2.5.2 and below": ("float32", "float64")}, "paddle")
def avg_pool2d(
x,
kernel_size,
@@ -81,7 +81,7 @@ def avg_pool2d(
padding = _broadcast_pooling_helper(padding, "2d", name="padding")
# Figure out padding string
if all(
- [pad == ivy.ceil((kernel - 1) / 2) for kernel, pad in zip(kernel_size, padding)]
+ pad == ivy.ceil((kernel - 1) / 2) for kernel, pad in zip(kernel_size, padding)
):
padding = "SAME"
else:
@@ -102,6 +102,41 @@ def avg_pool2d(
@to_ivy_arrays_and_back
@with_supported_dtypes({"2.5.1 and below": ("float32", "float64")}, "paddle")
+def max_pool2d(
+ x,
+ kernel_size,
+ stride=None,
+ padding=0,
+ return_mask=False,
+ ceil_mode=False,
+ data_format="NCHW",
+ name=None,
+):
+ if stride is None:
+ stride = kernel_size
+ kernel_size = _broadcast_pooling_helper(kernel_size, "2d", name="kernel_size")
+ padding = _broadcast_pooling_helper(padding, "2d", name="padding")
+
+ if data_format not in ["NCHW", "NHWC"]:
+ raise ValueError(
+ "Attr(data_format) should be 'NCHW' or 'NHWC'. Received "
+ "Attr(data_format): %s."
+ % str(data_format)
+ )
+
+ if data_format == "NHWC" and return_mask:
+ raise ValueError(
+ "When setting return_mask to true, data_format must be set to NCHW in"
+ " API:max_pool2d"
+ )
+
+ return ivy.max_pool2d(
+ x, kernel_size, stride, padding, data_format=data_format, ceil_mode=ceil_mode
+ )
+
+
+@to_ivy_arrays_and_back
+@with_supported_dtypes({"2.5.2 and below": ("float32", "float64")}, "paddle")
def max_unpool1d(
x,
indices,
@@ -112,4 +147,11 @@ def max_unpool1d(
output_size=None,
name=None,
):
- return ivy.max_unpool1d(x, indices, kernel_size, stride, padding, data_format)
+ return ivy.max_unpool1d(
+ x,
+ indices,
+ kernel_size,
+ strides=stride,
+ padding=padding,
+ data_format=data_format,
+ )
diff --git a/ivy/functional/frontends/paddle/nn/functional/vision.py b/ivy/functional/frontends/paddle/nn/functional/vision.py
index 015dd20535645..cf0ad4893580a 100644
--- a/ivy/functional/frontends/paddle/nn/functional/vision.py
+++ b/ivy/functional/frontends/paddle/nn/functional/vision.py
@@ -9,7 +9,7 @@
@to_ivy_arrays_and_back
-@with_unsupported_dtypes({"2.5.1 and below": ("float16", "bfloat16")}, "paddle")
+@with_unsupported_dtypes({"2.5.2 and below": ("float16", "bfloat16")}, "paddle")
def affine_grid(theta, out_shape, align_corners=True):
if len(out_shape) == 4:
N, C, H, W = out_shape
@@ -52,12 +52,6 @@ def affine_grid(theta, out_shape, align_corners=True):
ivy.expand_dims(ivy.linspace(-1, 1, D), axis=-1), axis=-1
)
width_values = ivy.linspace(-1, 1, D)
- base_grid[:, :, :, :, 2] = ivy.array(
- [[ivy.array([[width_values[i]] * W] * H) for i in range(D)]]
- )
- base_grid[:, :, :, :, 3] = ivy.full((D, H, W), 1)
- grid = ivy.matmul(base_grid.view((N, D * H * W, 4)), theta.swapaxes(1, 2))
- return grid.view((N, D, H, W, 3))
else:
base_grid[:, :, :, :, 0] = ivy.linspace(-1, 1, W) * (W - 1) / W
base_grid[:, :, :, :, 1] = ivy.expand_dims(
@@ -71,22 +65,22 @@ def affine_grid(theta, out_shape, align_corners=True):
ivy.expand_dims(ivy.linspace(-1, 1, D) * (D - 1) / D, axis=-1), axis=-1
)
width_values = ivy.linspace(-1, 1, D) * (D - 1) / D
- base_grid[:, :, :, :, 2] = ivy.array(
- [[ivy.array([[width_values[i]] * W] * H) for i in range(D)]]
- )
- base_grid[:, :, :, :, 3] = ivy.full((D, H, W), 1)
- grid = ivy.matmul(base_grid.view((N, D * H * W, 4)), theta.swapaxes(1, 2))
- return grid.view((N, D, H, W, 3))
+
+ base_grid[:, :, :, :, 2] = ivy.array(
+ [[ivy.array([[width_values[i]] * W] * H) for i in range(D)]]
+ )
+ base_grid[:, :, :, :, 3] = ivy.full((D, H, W), 1)
+ grid = ivy.matmul(base_grid.view((N, D * H * W, 4)), theta.swapaxes(1, 2))
+ return grid.view((N, D, H, W, 3))
@to_ivy_arrays_and_back
-@with_supported_dtypes({"2.5.1 and below": ("float32", "float64")}, "paddle")
+@with_supported_dtypes({"2.5.2 and below": ("float32", "float64")}, "paddle")
def channel_shuffle(x, groups, data_format="NCHW", name=None):
if len(ivy.shape(x)) != 4:
raise ValueError(
- "Input x should be 4D tensor, but received x with the shape of {}".format(
- ivy.shape(x)
- )
+ "Input x should be 4D tensor, but received x with the shape of"
+ f" {ivy.shape(x)}"
)
if not isinstance(groups, int):
@@ -97,8 +91,8 @@ def channel_shuffle(x, groups, data_format="NCHW", name=None):
if data_format not in ["NCHW", "NHWC"]:
raise ValueError(
- "Attr(data_format) should be 'NCHW' or 'NHWC'."
- "But recevie Attr(data_format): {} ".format(data_format)
+ "Attr(data_format) should be 'NCHW' or 'NHWC'.But receive"
+ f" Attr(data_format): {data_format} "
)
if data_format == "NCHW":
@@ -128,8 +122,8 @@ def pixel_shuffle(x, upscale_factor, data_format="NCHW"):
if data_format not in ["NCHW", "NHWC"]:
raise ValueError(
- "Attr(data_format) should be 'NCHW' or 'NHWC'."
- "But recevie Attr(data_format): {} ".format(data_format)
+ "Attr(data_format) should be 'NCHW' or 'NHWC'.But receive"
+ f" Attr(data_format): {data_format} "
)
b = input_shape[0]
@@ -144,10 +138,9 @@ def pixel_shuffle(x, upscale_factor, data_format="NCHW"):
0,
message=(
"pixel shuffle expects input channel to be divisible by square of upscale"
- " factor, but got input with sizes {}, upscale factor={}, and"
- " self.size(1)={}, is not divisible by {}".format(
- input_shape, upscale_factor, c, upscale_factor_squared
- )
+ f" factor, but got input with sizes {input_shape}, upscale"
+ f" factor={upscale_factor}, and self.size(1)={c}, is not divisible by"
+ f" {upscale_factor_squared}"
),
as_array=False,
)
@@ -174,9 +167,8 @@ def pixel_shuffle(x, upscale_factor, data_format="NCHW"):
def pixel_unshuffle(x, downscale_factor, data_format="NCHW"):
if len(ivy.shape(x)) != 4:
raise ValueError(
- "Input x should be 4D tensor, but received x with the shape of {}".format(
- ivy.shape(x)
- )
+ "Input x should be 4D tensor, but received x with the shape of"
+ f" {ivy.shape(x)}"
)
if not isinstance(downscale_factor, int):
@@ -187,8 +179,8 @@ def pixel_unshuffle(x, downscale_factor, data_format="NCHW"):
if data_format not in ["NCHW", "NHWC"]:
raise ValueError(
- "Attr(data_format) should be 'NCHW' or 'NHWC'."
- "But recevie Attr(data_format): {} ".format(data_format)
+ "Attr(data_format) should be 'NCHW' or 'NHWC'.But receive"
+ f" Attr(data_format): {data_format} "
)
if data_format == "NCHW":
diff --git a/ivy/functional/frontends/paddle/random.py b/ivy/functional/frontends/paddle/random.py
index f5e84b9c43c65..2a436935b9933 100644
--- a/ivy/functional/frontends/paddle/random.py
+++ b/ivy/functional/frontends/paddle/random.py
@@ -8,7 +8,17 @@
@with_supported_dtypes(
- {"2.5.1 and below": ("float32", "float64")},
+ {"2.5.2 and below": ("float32", "float64")},
+ "paddle",
+)
+@to_ivy_arrays_and_back
+def multinomial(x, num_samples=1, replacement=False, name=None):
+ n = num_samples + 1
+ return ivy.multinomial(n, num_samples, probs=x, replace=replacement)
+
+
+@with_supported_dtypes(
+ {"2.5.2 and below": ("float32", "float64")},
"paddle",
)
@to_ivy_arrays_and_back
@@ -17,7 +27,7 @@ def normal(mean=0.0, std=1.0, shape=None, name=None):
@with_supported_dtypes(
- {"2.5.1 and below": ("float32", "float64")},
+ {"2.5.2 and below": ("float32", "float64")},
"paddle",
)
@to_ivy_arrays_and_back
@@ -27,7 +37,7 @@ def poisson(x, name=None):
@with_supported_device_and_dtypes(
{
- "2.5.1 and above": {
+ "2.5.2 and above": {
"cpu": (
"bfloat16",
"float32",
@@ -65,7 +75,7 @@ def randint(low=0, high=None, shape=[1], dtype=None, name=None):
@with_unsupported_dtypes(
- {"2.5.1 and below": ("int16", "float16", "bfloat16", "uint8")},
+ {"2.5.2 and below": ("int16", "float16", "bfloat16", "uint8")},
"paddle",
)
@to_ivy_arrays_and_back
@@ -89,7 +99,7 @@ def randn(shape, dtype=None, name=None):
@with_supported_dtypes(
- {"2.5.1 and below": ("float32", "float64")},
+ {"2.5.2 and below": ("float32", "float64")},
"paddle",
)
@to_ivy_arrays_and_back
@@ -98,7 +108,7 @@ def standard_normal(shape, dtype=None, name=None):
@with_supported_dtypes(
- {"2.5.1 and below": ("float32", "float64")},
+ {"2.5.2 and below": ("float32", "float64")},
"paddle",
)
@to_ivy_arrays_and_back
diff --git a/ivy/functional/frontends/paddle/search.py b/ivy/functional/frontends/paddle/search.py
index 306ffe1db6099..c26923d08ae2e 100644
--- a/ivy/functional/frontends/paddle/search.py
+++ b/ivy/functional/frontends/paddle/search.py
@@ -7,7 +7,7 @@
@with_supported_dtypes(
- {"2.5.1 and below": ("float32", "float64", "int16", "int32", "int64", "uint8")},
+ {"2.5.2 and below": ("float32", "float64", "int16", "int32", "int64", "uint8")},
"paddle",
)
@to_ivy_arrays_and_back
@@ -16,7 +16,7 @@ def argmax(x, /, *, axis=None, keepdim=False, dtype="int64", name=None):
@with_supported_dtypes(
- {"2.5.1 and below": ("float32", "float64", "int16", "int32", "int64", "uint8")},
+ {"2.5.2 and below": ("float32", "float64", "int16", "int32", "int64", "uint8")},
"paddle",
)
@to_ivy_arrays_and_back
@@ -33,9 +33,18 @@ def argsort(x, /, *, axis=-1, descending=False, name=None):
return ivy.argsort(x, axis=axis, descending=descending)
+@with_supported_dtypes(
+ {"2.5.2 and below": ("int32", "int64", "float32", "float64")},
+ "paddle",
+)
+@to_ivy_arrays_and_back
+def index_sample(x, index):
+ return x[ivy.arange(x.shape[0])[:, None], index]
+
+
# kthvalue
@with_supported_dtypes(
- {"2.5.1 and below": ("float32", "float64", "int32", "int64")}, "paddle"
+ {"2.5.2 and below": ("float32", "float64", "int32", "int64")}, "paddle"
)
@to_ivy_arrays_and_back
def kthvalue(x, k, axis=None, keepdim=False, name=None):
@@ -56,7 +65,7 @@ def kthvalue(x, k, axis=None, keepdim=False, name=None):
@with_supported_dtypes(
- {"2.5.1 and below": ("float32", "float64", "int32", "int64")},
+ {"2.5.2 and below": ("float32", "float64", "int32", "int64")},
"paddle",
)
@to_ivy_arrays_and_back
@@ -77,7 +86,7 @@ def nonzero(input, /, *, as_tuple=False):
@with_supported_dtypes(
- {"2.5.1 and below": ("float32", "float64", "int32", "int64")},
+ {"2.5.2 and below": ("float32", "float64", "int32", "int64")},
"paddle",
)
@to_ivy_arrays_and_back
@@ -93,7 +102,7 @@ def searchsorted(sorted_sequence, values, out_int32=False, right=False, name=Non
@with_supported_dtypes(
- {"2.5.1 and below": ("float32", "float64", "int32", "int64")},
+ {"2.5.2 and below": ("float32", "float64", "int32", "int64")},
"paddle",
)
@to_ivy_arrays_and_back
@@ -102,7 +111,7 @@ def sort(x, /, *, axis=-1, descending=False, name=None):
@with_supported_dtypes(
- {"2.5.1 and below": ("float32", "float64", "int32", "int64")},
+ {"2.5.2 and below": ("float32", "float64", "int32", "int64")},
"paddle",
)
@to_ivy_arrays_and_back
@@ -112,7 +121,7 @@ def topk(x, k, axis=None, largest=True, sorted=True, name=None):
# where
@with_supported_dtypes(
- {"2.5.1 and below": ("float32", "float64", "int32", "int64")},
+ {"2.5.2 and below": ("float32", "float64", "int32", "int64")},
"paddle",
)
@to_ivy_arrays_and_back
diff --git a/ivy/functional/frontends/paddle/stat.py b/ivy/functional/frontends/paddle/stat.py
index fc76cdad7b72c..1ee6edd5ce54d 100644
--- a/ivy/functional/frontends/paddle/stat.py
+++ b/ivy/functional/frontends/paddle/stat.py
@@ -6,7 +6,7 @@
)
-@with_unsupported_dtypes({"2.5.1 and below": ("float16", "bfloat16")}, "paddle")
+@with_unsupported_dtypes({"2.5.2 and below": ("float16", "bfloat16")}, "paddle")
@to_ivy_arrays_and_back
def mean(input, axis=None, keepdim=False, out=None):
ret = ivy.mean(input, axis=axis, keepdims=keepdim, out=out)
@@ -14,7 +14,7 @@ def mean(input, axis=None, keepdim=False, out=None):
@with_supported_dtypes(
- {"2.5.1 and below": ("float32", "float64", "int32", "int64")},
+ {"2.5.2 and below": ("float32", "float64", "int32", "int64")},
"paddle",
)
@to_ivy_arrays_and_back
@@ -37,7 +37,7 @@ def nanmedian(x, axis=None, keepdim=True, name=None):
@with_supported_dtypes(
- {"2.5.1 and below": ("bool", "float16", "float32", "float64", "int32", "int64")},
+ {"2.5.2 and below": ("bool", "float16", "float32", "float64", "int32", "int64")},
"paddle",
)
@to_ivy_arrays_and_back
@@ -51,7 +51,7 @@ def numel(x, name=None):
@with_supported_dtypes(
- {"2.5.1 and below": ("float32", "float64", "uint16")},
+ {"2.5.2 and below": ("float32", "float64", "uint16")},
"paddle",
)
@to_ivy_arrays_and_back
@@ -64,7 +64,7 @@ def std(x, axis=None, unbiased=True, keepdim=False, name=None):
return ivy.std(x, axis=axis, correction=int(unbiased), keepdims=keepdim)
-@with_supported_dtypes({"2.5.1 and below": ("float32", "float64")}, "paddle")
+@with_supported_dtypes({"2.5.2 and below": ("float32", "float64")}, "paddle")
@to_ivy_arrays_and_back
def var(x, axis=None, unbiased=True, keepdim=False, name=None):
if unbiased:
diff --git a/ivy/functional/frontends/paddle/tensor/manipulation.py b/ivy/functional/frontends/paddle/tensor/manipulation.py
index 35ce51915f140..5300c5ba213d9 100644
--- a/ivy/functional/frontends/paddle/tensor/manipulation.py
+++ b/ivy/functional/frontends/paddle/tensor/manipulation.py
@@ -12,7 +12,7 @@
@with_unsupported_dtypes(
- {"2.5.1 and below": ("int8", "uint8", "int16", "uint16", "float16", "bfloat16")},
+ {"2.5.2 and below": ("int8", "uint8", "int16", "uint16", "float16", "bfloat16")},
"paddle",
)
@to_ivy_arrays_and_back
diff --git a/ivy/functional/frontends/paddle/tensor/math.py b/ivy/functional/frontends/paddle/tensor/math.py
index 7f9d56ea6452a..ceb36b11d7310 100644
--- a/ivy/functional/frontends/paddle/tensor/math.py
+++ b/ivy/functional/frontends/paddle/tensor/math.py
@@ -9,14 +9,14 @@
# Please add non-inplace counterparts to `/frontends/paddle/math.py`.
-@with_unsupported_dtypes({"2.5.1 and below": ("float16", "bfloat16")}, "paddle")
+@with_unsupported_dtypes({"2.5.2 and below": ("float16", "bfloat16")}, "paddle")
@to_ivy_arrays_and_back
def ceil_(x, name=None):
return ivy.ceil(x, out=x)
@with_supported_dtypes(
- {"2.5.1 and below": ("float32", "float64", "int32", "int64")}, "paddle"
+ {"2.5.2 and below": ("float32", "float64", "int32", "int64")}, "paddle"
)
@to_ivy_arrays_and_back
def clip_(x, min=None, max=None, name=None):
@@ -38,54 +38,54 @@ def clip_(x, min=None, max=None, name=None):
return res
-@with_supported_dtypes({"2.5.1 and below": ("float32", "float64")}, "paddle")
+@with_supported_dtypes({"2.5.2 and below": ("float32", "float64")}, "paddle")
@to_ivy_arrays_and_back
def exp_(x, name=None):
return ivy.inplace_update(x, exp(x))
-@with_supported_dtypes({"2.5.1 and below": ("float32", "float64")}, "paddle")
+@with_supported_dtypes({"2.5.2 and below": ("float32", "float64")}, "paddle")
@to_ivy_arrays_and_back
def floor_(x, name=None):
return ivy.inplace_update(x, floor(x))
-@with_supported_dtypes({"2.5.1 and below": ("float32", "float64")}, "paddle")
+@with_supported_dtypes({"2.5.2 and below": ("float32", "float64")}, "paddle")
@to_ivy_arrays_and_back
def lerp_(x, y, weight, name=None):
return ivy.inplace_update(x, lerp(x, y, weight))
-@with_unsupported_dtypes({"2.5.1 and below": ("float16", "bfloat16")}, "paddle")
+@with_unsupported_dtypes({"2.5.2 and below": ("float16", "bfloat16")}, "paddle")
@to_ivy_arrays_and_back
def reciprocal_(x, name=None):
return ivy.inplace_update(x, reciprocal(x))
-@with_unsupported_dtypes({"2.5.1 and below": ("float16", "bfloat16")}, "paddle")
+@with_unsupported_dtypes({"2.5.2 and below": ("float16", "bfloat16")}, "paddle")
@to_ivy_arrays_and_back
def round_(x, name=None):
return ivy.inplace_update(x, round(x))
-@with_supported_dtypes({"2.5.1 and below": ("float32", "float64")}, "paddle")
+@with_supported_dtypes({"2.5.2 and below": ("float32", "float64")}, "paddle")
def rsqrt_(x, name=None):
return ivy.inplace_update(x, reciprocal(sqrt(x)))
-@with_supported_dtypes({"2.5.1 and below": ("float32", "float64")}, "paddle")
+@with_supported_dtypes({"2.5.2 and below": ("float32", "float64")}, "paddle")
@to_ivy_arrays_and_back
def sqrt_(x, name=None):
return ivy.inplace_update(x, sqrt(x))
-@with_supported_dtypes({"2.5.1 and below": ("float32", "float64")}, "paddle")
+@with_supported_dtypes({"2.5.2 and below": ("float32", "float64")}, "paddle")
@to_ivy_arrays_and_back
def subtract_(x, y, name=None):
return ivy.inplace_update(x, subtract(x, y))
-@with_supported_dtypes({"2.5.1 and below": ("float32", "float64")}, "paddle")
+@with_supported_dtypes({"2.5.2 and below": ("float32", "float64")}, "paddle")
@to_ivy_arrays_and_back
def tanh_(x, name=None):
return ivy.inplace_update(x, tanh(x))
diff --git a/ivy/functional/frontends/paddle/tensor/random.py b/ivy/functional/frontends/paddle/tensor/random.py
index ea6bd38157195..dd6be26c2f4f2 100644
--- a/ivy/functional/frontends/paddle/tensor/random.py
+++ b/ivy/functional/frontends/paddle/tensor/random.py
@@ -12,7 +12,7 @@
@with_supported_dtypes(
- {"2.5.1 and below": ("float32", "float64")},
+ {"2.5.2 and below": ("float32", "float64")},
"paddle",
)
@to_ivy_arrays_and_back
@@ -21,7 +21,7 @@ def exponential_(x, lam=1.0, name=None):
@with_supported_dtypes(
- {"2.5.1 and below": ("float32", "float64")},
+ {"2.5.2 and below": ("float32", "float64")},
"paddle",
)
@to_ivy_arrays_and_back
diff --git a/ivy/functional/frontends/paddle/tensor/tensor.py b/ivy/functional/frontends/paddle/tensor/tensor.py
index 3f906531b6073..870269f06a9f3 100644
--- a/ivy/functional/frontends/paddle/tensor/tensor.py
+++ b/ivy/functional/frontends/paddle/tensor/tensor.py
@@ -4,6 +4,7 @@
from ivy.func_wrapper import (
with_supported_dtypes,
with_unsupported_dtypes,
+ with_supported_device_and_dtypes,
)
from ivy.functional.frontends.paddle.func_wrapper import _to_ivy_array
@@ -61,7 +62,7 @@ def ivy_array(self, array):
# -------------------#
@with_unsupported_dtypes(
- {"2.5.1 and below": ("bool", "unsigned", "int8", "float16", "bfloat16")},
+ {"2.5.2 and below": ("bool", "unsigned", "int8", "float16", "bfloat16")},
"paddle",
)
def __add__(self, y, /, name=None):
@@ -102,134 +103,156 @@ def reshape(self, *args, shape=None):
def dim(self):
return self.ivy_array.ndim
- @with_unsupported_dtypes({"2.5.1 and below": ("float16", "bfloat16")}, "paddle")
+ @with_unsupported_dtypes({"2.5.2 and below": ("float16", "bfloat16")}, "paddle")
def abs(self):
return paddle_frontend.abs(self)
- @with_unsupported_dtypes({"2.5.1 and below": ("float16", "bfloat16")}, "paddle")
+ @with_unsupported_dtypes({"2.5.2 and below": ("float16", "bfloat16")}, "paddle")
def acosh(self, name=None):
return paddle_frontend.acosh(self)
- @with_unsupported_dtypes({"2.5.1 and below": ("float16", "bfloat16")}, "paddle")
+ @with_unsupported_dtypes({"2.5.2 and below": ("float16", "bfloat16")}, "paddle")
+ def add_n(self, inputs, name=None):
+ inputs = ivy.array(inputs)
+ return ivy.sum(inputs, dtype=inputs.dtype, axis=0)
+
+ @with_unsupported_dtypes({"2.5.2 and below": ("float16", "bfloat16")}, "paddle")
def ceil(self):
return paddle_frontend.ceil(self)
- @with_supported_dtypes({"2.5.1 and below": ("float32", "float64")}, "paddle")
+ @with_supported_dtypes({"2.5.2 and below": ("float32", "float64")}, "paddle")
def ceil_(self):
self.ivy_array = self.ceil().ivy_array
return self
- @with_unsupported_dtypes({"2.5.1 and below": ("complex", "int8")}, "paddle")
+ @with_unsupported_dtypes({"2.5.2 and below": ("complex", "int8")}, "paddle")
def numel(self):
return paddle_frontend.numel(self)
- @with_unsupported_dtypes({"2.5.1 and below": ("float16",)}, "paddle")
+ @with_unsupported_dtypes({"2.5.2 and below": ("float16",)}, "paddle")
def asinh(self, name=None):
return paddle_frontend.asinh(self)
- @with_supported_dtypes({"2.5.1 and below": ("float32", "float64")}, "paddle")
+ @with_supported_dtypes({"2.5.2 and below": ("float32", "float64")}, "paddle")
def asin(self, name=None):
return paddle_frontend.asin(self)
- @with_supported_dtypes({"2.5.1 and below": ("float32", "float64")}, "paddle")
+ @with_supported_dtypes({"2.5.2 and below": ("float32", "float64")}, "paddle")
def cosh(self, name=None):
return paddle_frontend.cosh(self)
- @with_supported_dtypes({"2.5.1 and below": ("float32", "float64")}, "paddle")
+ @with_supported_dtypes(
+ {
+ "2.5.2 and below": (
+ "int32",
+ "int64",
+ "float64",
+ "complex128",
+ "float32",
+ "complex64",
+ "bool",
+ )
+ },
+ "paddle",
+ )
+ def diagonal(self, offset, axis1=0, axis2=1, name=None):
+ return paddle_frontend.diagonal(self, offset=offset, axis1=axis1, axis2=axis2)
+
+ @with_supported_dtypes({"2.5.2 and below": ("float32", "float64")}, "paddle")
def log(self, name=None):
return paddle_frontend.log(self)
- @with_supported_dtypes({"2.5.1 and below": ("float32", "float64")}, "paddle")
+ @with_supported_dtypes({"2.5.2 and below": ("float32", "float64")}, "paddle")
def sin(self, name=None):
return paddle_frontend.sin(self)
- @with_supported_dtypes({"2.5.1 and below": ("float32", "float64")}, "paddle")
+ @with_supported_dtypes({"2.5.2 and below": ("float32", "float64")}, "paddle")
def sinh(self, name=None):
return paddle_frontend.sinh(self)
- @with_supported_dtypes({"2.5.1 and below": ("float32", "float64")}, "paddle")
+ @with_supported_dtypes({"2.5.2 and below": ("float32", "float64")}, "paddle")
def lerp(self, y, weight, name=None):
return paddle_frontend.lerp(self, y, weight)
- @with_supported_dtypes({"2.5.1 and below": ("float32", "float64")}, "paddle")
+ @with_supported_dtypes({"2.5.2 and below": ("float32", "float64")}, "paddle")
def lerp_(self, y, weight, name=None):
self.ivy_array = paddle_frontend.lerp(self, y, weight).ivy_array
return self
- @with_unsupported_dtypes({"2.5.1 and below": ("float16", "bfloat16")}, "paddle")
+ @with_unsupported_dtypes({"2.5.2 and below": ("float16", "bfloat16")}, "paddle")
def argmax(self, axis=None, keepdim=False, dtype=None, name=None):
return paddle_frontend.argmax(self, axis=axis, keepdim=keepdim, dtype=dtype)
- @with_unsupported_dtypes({"2.5.1 and below": ("float16", "uint16")}, "paddle")
+ @with_unsupported_dtypes({"2.5.2 and below": ("float16", "uint16")}, "paddle")
def unsqueeze(self, axis=None, name=None):
return paddle_frontend.Tensor(ivy.expand_dims(self._ivy_array, axis=axis))
- @with_supported_dtypes({"2.5.1 and below": ("float32", "float64")}, "paddle")
+ @with_supported_dtypes({"2.5.2 and below": ("float32", "float64")}, "paddle")
def sqrt(self, name=None):
return paddle_frontend.sqrt(self)
- @with_supported_dtypes({"2.5.1 and below": ("float32", "float64")}, "paddle")
+ @with_supported_dtypes({"2.5.2 and below": ("float32", "float64")}, "paddle")
def sqrt_(self, name=None):
self.ivy_array = self.sqrt().ivy_array
return self
- @with_unsupported_dtypes({"2.5.1 and below": ("bfloat16", "uint16")}, "paddle")
+ @with_unsupported_dtypes({"2.5.2 and below": ("bfloat16", "uint16")}, "paddle")
def zero_(self):
self.ivy_array = paddle_frontend.zeros_like(self).ivy_array
return self
- @with_supported_dtypes({"2.5.1 and below": ("float32", "float64")}, "paddle")
+ @with_supported_dtypes({"2.5.2 and below": ("float32", "float64")}, "paddle")
def cos(self, name=None):
return paddle_frontend.cos(self)
- @with_unsupported_dtypes({"2.5.1 and below": ("float16", "bfloat16")}, "paddle")
+ @with_unsupported_dtypes({"2.5.2 and below": ("float16", "bfloat16")}, "paddle")
def exp(self, name=None):
return paddle_frontend.exp(self)
- @with_unsupported_dtypes({"2.5.1 and below": ("float16", "bfloat16")}, "paddle")
+ @with_unsupported_dtypes({"2.5.2 and below": ("float16", "bfloat16")}, "paddle")
def exp_(self, name=None):
self.ivy_array = self.exp().ivy_array
return self
- @with_supported_dtypes({"2.5.1 and below": ("float32", "float64")}, "paddle")
+ @with_supported_dtypes({"2.5.2 and below": ("float32", "float64")}, "paddle")
def erf(self, name=None):
return paddle_frontend.erf(self)
- @with_unsupported_dtypes({"2.5.1 and below": ("float16", "bfloat16")}, "paddle")
+ @with_unsupported_dtypes({"2.5.2 and below": ("float16", "bfloat16")}, "paddle")
def subtract(self, y, name=None):
return paddle_frontend.subtract(self, y)
@with_unsupported_dtypes(
- {"2.5.1 and below": ("float16", "uint8", "int8", "bool")}, "paddle"
+ {"2.5.2 and below": ("float16", "uint8", "int8", "bool")}, "paddle"
)
def subtract_(self, y, name=None):
self.ivy_array = self.subtract(y).ivy_array
return self
- @with_unsupported_dtypes({"2.5.1 and below": ("float16", "bfloat16")}, "paddle")
+ @with_unsupported_dtypes({"2.5.2 and below": ("float16", "bfloat16")}, "paddle")
def log10(self, name=None):
return paddle_frontend.Tensor(ivy.log10(self._ivy_array))
- @with_unsupported_dtypes({"2.5.1 and below": ("float16", "bfloat16")}, "paddle")
+ @with_unsupported_dtypes({"2.5.2 and below": ("float16", "bfloat16")}, "paddle")
def argsort(self, axis=-1, descending=False, name=None):
return paddle_frontend.argsort(self, axis=axis, descending=descending)
- @with_unsupported_dtypes({"2.5.1 and below": ("float16", "bfloat16")}, "paddle")
+ @with_unsupported_dtypes({"2.5.2 and below": ("float16", "bfloat16")}, "paddle")
def floor(self, name=None):
return paddle_frontend.floor(self)
- @with_unsupported_dtypes({"2.5.1 and below": ("float16", "bfloat16")}, "paddle")
+ @with_unsupported_dtypes({"2.5.2 and below": ("float16", "bfloat16")}, "paddle")
def floor_(self):
self.ivy_array = self.floor().ivy_array
return self
- @with_supported_dtypes({"2.5.1 and below": ("float32", "float64")}, "paddle")
+ @with_supported_dtypes({"2.5.2 and below": ("float32", "float64")}, "paddle")
def round_(self, name=None):
self.ivy_array = paddle_frontend.round(self).ivy_array
return self
@with_supported_dtypes(
- {"2.5.1 and below": ("float32", "float64", "int32", "int64")}, "paddle"
+ {"2.5.2 and below": ("float32", "float64", "int32", "int64")}, "paddle"
)
def clip(self, min=None, max=None, name=None):
ivy.utils.assertions.check_all_or_any_fn(
@@ -249,59 +272,63 @@ def clip(self, min=None, max=None, name=None):
return paddle_frontend.Tensor(ret)
@with_supported_dtypes(
- {"2.5.1 and below": ("float32", "float64", "int32", "int64")}, "paddle"
+ {"2.5.2 and below": ("float32", "float64", "int32", "int64")}, "paddle"
)
def clip_(self, min=None, max=None, name=None):
self._ivy_array = self.clip(min, max).ivy_array
return self
- @with_supported_dtypes({"2.5.1 and below": ("float32", "float64")}, "paddle")
+ @with_supported_dtypes({"2.5.2 and below": ("float32", "float64")}, "paddle")
def tanh(self, name=None):
return paddle_frontend.tanh(self)
- @with_supported_dtypes({"2.5.1 and below": ("float32", "float64")}, "paddle")
+ @with_supported_dtypes({"2.5.2 and below": ("float32", "float64")}, "paddle")
def add_(self, y, name=None):
self.ivy_array = paddle_frontend.add(self, y).ivy_array
return self
+ @with_supported_dtypes({"2.5.2 and below": ("float32", "float64")}, "paddle")
+ def addmm(self, x, y, beta=1.0, alpha=1.0, name=None):
+ return paddle_frontend.addmm(self, x, y, beta, alpha)
+
@with_supported_dtypes(
- {"2.5.1 and below": ("float16", "float32", "float64", "int32", "int64")},
+ {"2.5.2 and below": ("float16", "float32", "float64", "int32", "int64")},
"paddle",
)
def isinf(self, name=None):
return paddle_frontend.isinf(self)
- @with_unsupported_dtypes({"2.5.1 and below": ("float16", "uint16")}, "paddle")
+ @with_unsupported_dtypes({"2.5.2 and below": ("float16", "uint16")}, "paddle")
def unsqueeze_(self, axis=None, name=None):
self.ivy_array = self.unsqueeze(axis=axis).ivy_array
return self
- @with_supported_dtypes({"2.5.1 and below": ("float32", "float64")}, "paddle")
+ @with_supported_dtypes({"2.5.2 and below": ("float32", "float64")}, "paddle")
def square(self, name=None):
return paddle_frontend.square(self)
- @with_unsupported_dtypes({"2.5.1 and below": ("float16", "bfloat16")}, "paddle")
+ @with_unsupported_dtypes({"2.5.2 and below": ("float16", "bfloat16")}, "paddle")
def remainder_(self, y, name=None):
self.ivy_array = paddle_frontend.remainder(self, y).ivy_array
return self
- @with_supported_dtypes({"2.5.1 and below": ("float32", "float64")}, "paddle")
+ @with_supported_dtypes({"2.5.2 and below": ("float32", "float64")}, "paddle")
def cholesky(self, upper=False, name=None):
return paddle_frontend.cholesky(self, upper=upper)
@with_unsupported_dtypes(
- {"2.5.1 and below": ("float16", "uint16", "int16")}, "paddle"
+ {"2.5.2 and below": ("float16", "uint16", "int16")}, "paddle"
)
def squeeze_(self, axis=None, name=None):
self.ivy_array = paddle_frontend.squeeze(self, axis=axis).ivy_array
return self
- @with_unsupported_dtypes({"2.5.1 and below": ("float16", "bfloat16")}, "paddle")
+ @with_unsupported_dtypes({"2.5.2 and below": ("float16", "bfloat16")}, "paddle")
def multiply(self, y, name=None):
return paddle_frontend.multiply(self, y)
@with_supported_dtypes(
- {"2.5.1 and below": ("float16", "float32", "float64", "int32", "int64")},
+ {"2.5.2 and below": ("float16", "float32", "float64", "int32", "int64")},
"paddle",
)
def isfinite(self, name=None):
@@ -313,17 +340,17 @@ def all(self, axis=None, keepdim=False, dtype=None, name=None):
ivy.all(self.ivy_array, axis=axis, keepdims=keepdim, dtype=dtype)
)
- @with_supported_dtypes({"2.5.1 and below": ("float16", "bfloat16")}, "paddle")
+ @with_supported_dtypes({"2.5.2 and below": ("float16", "bfloat16")}, "paddle")
def allclose(self, other, rtol=1e-05, atol=1e-08, equal_nan=False, name=None):
return paddle_frontend.allclose(
self, other, rtol=rtol, atol=atol, equal_nan=equal_nan
)
- @with_unsupported_dtypes({"2.5.1 and below": ("float16", "bfloat16")}, "paddle")
+ @with_unsupported_dtypes({"2.5.2 and below": ("float16", "bfloat16")}, "paddle")
def sort(self, axis=-1, descending=False, name=None):
return paddle_frontend.sort(self, axis=axis, descending=descending)
- @with_unsupported_dtypes({"2.5.1 and below": ("float16", "bfloat16")}, "paddle")
+ @with_unsupported_dtypes({"2.5.2 and below": ("float16", "bfloat16")}, "paddle")
def log1p(self, name=None):
return paddle_frontend.log1p(self)
@@ -345,7 +372,7 @@ def bitwise_and(self, y, out=None, name=None):
@with_supported_dtypes(
{
- "2.5.1 and below": (
+ "2.5.2 and below": (
"bool",
"int8",
"int16",
@@ -361,22 +388,22 @@ def logical_or(self, y, out=None, name=None):
return paddle_frontend.logical_or(self, y, out=out)
@with_supported_dtypes(
- {"2.5.1 and below": ("bool", "uint8", "int8", "int16", "int32", "int64")},
+ {"2.5.2 and below": ("bool", "uint8", "int8", "int16", "int32", "int64")},
"paddle",
)
def bitwise_xor(self, y, out=None, name=None):
return paddle_frontend.bitwise_xor(self, y)
- @with_supported_dtypes({"2.5.1 and below": ("float16", "bfloat16")}, "paddle")
+ @with_supported_dtypes({"2.5.2 and below": ("float16", "bfloat16")}, "paddle")
def any(self, axis=None, keepdim=False, name=None):
return paddle_frontend.any(self, axis=axis, keepdim=keepdim)
- @with_unsupported_dtypes({"2.5.1 and below": "bfloat16"}, "paddle")
+ @with_unsupported_dtypes({"2.5.2 and below": "bfloat16"}, "paddle")
def astype(self, dtype):
return paddle_frontend.Tensor(ivy.astype(self._ivy_array, dtype))
@with_supported_dtypes(
- {"2.5.1 and below": ("bool", "uint8", "int8", "int16", "int32", "int64")},
+ {"2.5.2 and below": ("bool", "uint8", "int8", "int16", "int32", "int64")},
"paddle",
)
def bitwise_not(self, out=None, name=None):
@@ -384,7 +411,7 @@ def bitwise_not(self, out=None, name=None):
@with_supported_dtypes(
{
- "2.5.1 and below": (
+ "2.5.2 and below": (
"bool",
"int8",
"int16",
@@ -399,7 +426,7 @@ def bitwise_or(self, y, out=None, name=None):
@with_supported_dtypes(
{
- "2.5.1 and below": (
+ "2.5.2 and below": (
"bool",
"int8",
"int16",
@@ -415,7 +442,7 @@ def logical_xor(self, y, out=None, name=None):
return paddle_frontend.logical_xor(self, y, out=out)
@with_supported_dtypes(
- {"2.5.1 and below": ("float16", "float32", "float64", "int32", "int64")},
+ {"2.5.2 and below": ("float16", "float32", "float64", "int32", "int64")},
"paddle",
)
def isnan(self, name=None):
@@ -423,7 +450,7 @@ def isnan(self, name=None):
@with_unsupported_dtypes(
{
- "2.5.1 and below": (
+ "2.5.2 and below": (
"bool",
"uint8",
"int8",
@@ -437,22 +464,22 @@ def isnan(self, name=None):
def greater_than(self, y, name=None):
return paddle_frontend.greater_than(self, y)
- @with_supported_dtypes({"2.5.1 and below": ("float32", "float64")}, "paddle")
+ @with_supported_dtypes({"2.5.2 and below": ("float32", "float64")}, "paddle")
def rsqrt(self, name=None):
return paddle_frontend.rsqrt(self)
- @with_supported_dtypes({"2.5.1 and below": ("float32", "float64")}, "paddle")
+ @with_supported_dtypes({"2.5.2 and below": ("float32", "float64")}, "paddle")
def rsqrt_(self, name=None):
self.ivy_array = self.rsqrt().ivy_array
return self
- @with_supported_dtypes({"2.5.1 and below": ("float32", "float64")}, "paddle")
+ @with_supported_dtypes({"2.5.2 and below": ("float32", "float64")}, "paddle")
def reciprocal(self, name=None):
return paddle_frontend.reciprocal(self)
@with_supported_dtypes(
{
- "2.5.1 and below": (
+ "2.5.2 and below": (
"bool",
"int8",
"int16",
@@ -467,19 +494,20 @@ def reciprocal(self, name=None):
def logical_and(self, y, out=None, name=None):
return paddle_frontend.logical_and(self, y, out=out)
- @with_supported_dtypes({"2.5.1 and below": ("float32", "float64")}, "paddle")
+ @with_supported_dtypes({"2.5.2 and below": ("float32", "float64")}, "paddle")
def divide(self, y, name=None):
return paddle_frontend.divide(self, y)
@with_supported_dtypes(
- {"2.5.1 and below": ("float32", "float64", "complex64", "complex128")}, "paddle"
+ {"2.5.2 and below": ("float32", "float64", "complex64", "complex128")},
+ "paddle",
)
def eigvals(self, name=None):
return paddle_frontend.eigvals(self)
@with_unsupported_dtypes(
{
- "2.5.1 and below": (
+ "2.5.2 and below": (
"bool",
"uint8",
"int8",
@@ -493,18 +521,18 @@ def eigvals(self, name=None):
def less_than(self, y, name=None):
return paddle_frontend.less_than(self, y)
- @with_unsupported_dtypes({"2.5.1 and below": ("float16", "bfloat16")}, "paddle")
+ @with_unsupported_dtypes({"2.5.2 and below": ("float16", "bfloat16")}, "paddle")
def cumprod(self, dim=None, dtype=None, name=None):
return paddle_frontend.cumprod(self, dim=dim, dtype=dtype)
- @with_unsupported_dtypes({"2.5.1 and below": ("float16", "bfloat16")}, "paddle")
+ @with_unsupported_dtypes({"2.5.2 and below": ("float16", "bfloat16")}, "paddle")
def cumsum(self, axis=None, dtype=None, name=None):
return paddle_frontend.Tensor(
ivy.cumsum(self._ivy_array, axis=axis, dtype=dtype)
)
@with_supported_dtypes(
- {"2.5.1 and below": ("complex64", "complex128", "float32", "float64")},
+ {"2.5.2 and below": ("complex64", "complex128", "float32", "float64")},
"paddle",
)
def angle(self, name=None):
@@ -512,7 +540,7 @@ def angle(self, name=None):
@with_unsupported_dtypes(
{
- "2.5.1 and below": (
+ "2.5.2 and below": (
"uint8",
"int8",
"int16",
@@ -525,13 +553,13 @@ def angle(self, name=None):
def equal(self, y, name=None):
return paddle_frontend.equal(self, y)
- @with_unsupported_dtypes({"2.5.1 and below": ("float16", "bfloat16")}, "paddle")
+ @with_unsupported_dtypes({"2.5.2 and below": ("float16", "bfloat16")}, "paddle")
def rad2deg(self, name=None):
return paddle_frontend.rad2deg(self)
@with_unsupported_dtypes(
{
- "2.5.1 and below": (
+ "2.5.2 and below": (
"uint8",
"int8",
"int16",
@@ -545,46 +573,46 @@ def rad2deg(self, name=None):
def equal_all(self, y, name=None):
return paddle_frontend.equal_all(self, y)
- @with_supported_dtypes({"2.5.1 and below": ("float32", "float64")}, "paddle")
+ @with_supported_dtypes({"2.5.2 and below": ("float32", "float64")}, "paddle")
def maximum(self, other, name=None):
return paddle_frontend.maximum(self, other)
- @with_unsupported_dtypes({"2.5.1 and below": "bfloat16"}, "paddle")
+ @with_unsupported_dtypes({"2.5.2 and below": "bfloat16"}, "paddle")
def fmax(self, y, name=None):
return paddle_frontend.fmax(self, y)
- @with_unsupported_dtypes({"2.5.1 and below": "bfloat16"}, "paddle")
+ @with_unsupported_dtypes({"2.5.2 and below": "bfloat16"}, "paddle")
def fmin(self, y, name=None):
return paddle_frontend.fmin(self, y)
@with_supported_dtypes(
- {"2.5.1 and below": ("float32", "float64", "int32", "int64")}, "paddle"
+ {"2.5.2 and below": ("float32", "float64", "int32", "int64")}, "paddle"
)
def minimum(self, y, name=None):
return paddle_frontend.minimum(self, y)
@with_supported_dtypes(
- {"2.5.1 and below": ("float32", "float64", "int32", "int64")}, "paddle"
+ {"2.5.2 and below": ("float32", "float64", "int32", "int64")}, "paddle"
)
def max(self, axis=None, keepdim=False, name=None):
return paddle_frontend.max(self, axis=axis, keepdim=keepdim)
- @with_unsupported_dtypes({"2.5.1 and below": ("float16", "bfloat16")}, "paddle")
+ @with_unsupported_dtypes({"2.5.2 and below": ("float16", "bfloat16")}, "paddle")
def deg2rad(self, name=None):
return paddle_frontend.deg2rad(self)
- @with_supported_dtypes({"2.5.1 and below": ("float32", "float64")}, "paddle")
+ @with_supported_dtypes({"2.5.2 and below": ("float32", "float64")}, "paddle")
def digamma(self, name=None):
return paddle_frontend.digamma(self)
@with_supported_dtypes(
- {"2.5.1 and below": ("float32", "float64", "int32", "int64", "bool")}, "paddle"
+ {"2.5.2 and below": ("float32", "float64", "int32", "int64", "bool")}, "paddle"
)
def rot90(self, k=1, axes=(0, 1), name=None):
return paddle_frontend.rot90(self, k=k, axes=axes)
@with_supported_dtypes(
- {"2.5.1 and below": ("complex64", "complex128")},
+ {"2.5.2 and below": ("complex64", "complex128")},
"paddle",
)
def imag(self, name=None):
@@ -595,7 +623,7 @@ def is_tensor(self):
@with_supported_dtypes(
{
- "2.5.1 and below": (
+ "2.5.2 and below": (
"float32",
"float64",
)
@@ -607,12 +635,16 @@ def isclose(self, y, rtol=1e-05, atol=1e-08, equal_nan=False, name=None):
self, y, rtol=rtol, atol=atol, equal_nan=equal_nan
)
- @with_supported_dtypes({"2.5.1 and below": ("int32", "int64")}, "paddle")
+ @with_supported_dtypes({"2.5.2 and below": ("int32", "int64")}, "paddle")
def floor_divide(self, y, name=None):
return paddle_frontend.floor_divide(self, y)
+ @with_supported_dtypes({"2.5.2 and below": ("int32", "int64")}, "paddle")
+ def mod(self, y, name=None):
+ return paddle_frontend.Tensor(ivy.fmod(self._ivy_array, _to_ivy_array(y)))
+
# cond
- @with_supported_dtypes({"2.5.1 and below": ("float32", "float64")}, "paddle")
+ @with_supported_dtypes({"2.5.2 and below": ("float32", "float64")}, "paddle")
def cond(self, p=None, name=None):
return paddle_frontend.cond(self, p=p, name=name)
@@ -620,7 +652,7 @@ def cond(self, p=None, name=None):
def conj(self, name=None):
return paddle_frontend.conj(self)
- @with_supported_dtypes({"2.5.1 and below": ("float32", "float64")}, "paddle")
+ @with_supported_dtypes({"2.5.2 and below": ("float32", "float64")}, "paddle")
def log2(self, name=None):
return paddle_frontend.log2(self)
@@ -632,7 +664,7 @@ def neg(self, name=None):
@with_supported_dtypes(
{
- "2.5.1 and below": (
+ "2.5.2 and below": (
"bool",
"int8",
"int16",
@@ -647,15 +679,15 @@ def neg(self, name=None):
def logical_not(self, out=None, name=None):
return paddle_frontend.logical_not(self)
- @with_unsupported_dtypes({"2.5.1 and below": ("float16", "bfloat16")}, "paddle")
+ @with_unsupported_dtypes({"2.5.2 and below": ("float16", "bfloat16")}, "paddle")
def sign(self, name=None):
return paddle_frontend.sign(self)
- @with_supported_dtypes({"2.5.1 and below": ("float32", "float64")}, "paddle")
+ @with_supported_dtypes({"2.5.2 and below": ("float32", "float64")}, "paddle")
def var(self, axis=None, unbiased=True, keepdim=False, name=None):
return paddle_frontend.var(self, axis=axis, unbiased=unbiased, keepdim=keepdim)
- @with_unsupported_dtypes({"2.5.1 and below": ("float16", "bfloat16")}, "paddle")
+ @with_unsupported_dtypes({"2.5.2 and below": ("float16", "bfloat16")}, "paddle")
def sgn(self, name=None):
return paddle_frontend.sgn(self)
@@ -663,45 +695,45 @@ def tolist(self):
return paddle_frontend.Tensor(ivy.to_list(self._ivy_array))
@with_supported_dtypes(
- {"2.5.1 and below": ("float32", "float64", "int32", "int64")},
+ {"2.5.2 and below": ("float32", "float64", "int32", "int64")},
"paddle",
)
def min(self, axis=None, keepdim=False, name=None):
return paddle_frontend.min(self, axis=axis, keepdim=keepdim)
@with_supported_dtypes(
- {"2.5.1 and below": ("int32", "int64", "float32", "float64")}, "paddle"
+ {"2.5.2 and below": ("int32", "int64", "float32", "float64")}, "paddle"
)
def pow(self, y, name=None):
return paddle_frontend.pow(self, y)
@with_supported_dtypes(
- {"2.5.1 and below": ("float32", "float64", "int32", "int64")}, "paddle"
+ {"2.5.2 and below": ("float32", "float64", "int32", "int64")}, "paddle"
)
def prod(self, axis=None, keepdim=False, dtype=None, name=None):
return paddle_frontend.Tensor(
ivy.prod(self._ivy_array, axis=axis, keepdims=keepdim, dtype=dtype)
)
- @with_supported_dtypes({"2.5.1 and below": ("float32", "float64")}, "paddle")
+ @with_supported_dtypes({"2.5.2 and below": ("float32", "float64")}, "paddle")
def atan(self, name=None):
return paddle_frontend.atan(self)
- @with_supported_dtypes({"2.5.1 and below": ("float32", "float64")}, "paddle")
+ @with_supported_dtypes({"2.5.2 and below": ("float32", "float64")}, "paddle")
def atanh(self, name=None):
return paddle_frontend.atanh(self)
- @with_supported_dtypes({"2.5.1 and below": ("float32", "float64")}, "paddle")
+ @with_supported_dtypes({"2.5.2 and below": ("float32", "float64")}, "paddle")
def std(self, axis=None, unbiased=True, keepdim=False, name=None):
return paddle_frontend.std(self, axis=axis, unbiased=unbiased, keepdim=keepdim)
@with_supported_dtypes(
- {"2.5.1 and below": ("int32", "int64", "float32", "float64")}, "paddle"
+ {"2.5.2 and below": ("int32", "int64", "float32", "float64")}, "paddle"
)
def trunc(self, name=None):
return paddle_frontend.trunc(self)
- @with_supported_dtypes({"2.5.1 and below": ("complex64", "complex128")}, "paddle")
+ @with_supported_dtypes({"2.5.2 and below": ("complex64", "complex128")}, "paddle")
def as_real(self, name=None):
if not ivy.is_complex_dtype(self._ivy_array):
raise ivy.exceptions.IvyError(
@@ -711,12 +743,12 @@ def as_real(self, name=None):
im_part = ivy.imag(self._ivy_array)
return paddle_frontend.Tensor(ivy.stack((re_part, im_part), axis=-1))
- @with_supported_dtypes({"2.5.1 and below": ("float32", "float64")}, "paddle")
+ @with_supported_dtypes({"2.5.2 and below": ("float32", "float64")}, "paddle")
def stanh(self, scale_a=0.67, scale_b=1.7159, name=None):
return paddle_frontend.stanh(self, scale_a=scale_a, scale_b=scale_b)
@with_supported_dtypes(
- {"2.5.1 and below": ("int32", "int64", "float32", "float64")}, "paddle"
+ {"2.5.2 and below": ("int32", "int64", "float32", "float64")}, "paddle"
)
def trace(self, offset=0, axis1=0, axis2=1, name=None):
return paddle_frontend.Tensor(
@@ -724,55 +756,64 @@ def trace(self, offset=0, axis1=0, axis2=1, name=None):
)
@with_supported_dtypes(
- {"2.5.1 and below": ("float32", "float64", "int16", "int32", "int64", "uint8")},
+ {
+ "2.5.2 and below": (
+ "float32",
+ "float64",
+ "int16",
+ "int32",
+ "int64",
+ "uint8",
+ )
+ },
"paddle",
)
def argmin(self, axis=None, keepdim=False, dtype=None, name=None):
return paddle_frontend.argmin(self, axis=axis, keepdim=keepdim, dtype=dtype)
@with_supported_dtypes(
- {"2.5.1 and below": ("float32", "float64", "int32", "int64")},
+ {"2.5.2 and below": ("float32", "float64", "int32", "int64")},
"paddle",
)
def topk(self, k, axis=None, largest=True, sorted=True, name=None):
return paddle_frontend.topk(self, k, axis=axis, largest=largest, sorted=sorted)
- @with_unsupported_dtypes({"2.5.1 and below": ("float16", "bfloat16")}, "paddle")
+ @with_unsupported_dtypes({"2.5.2 and below": ("float16", "bfloat16")}, "paddle")
def remainder(self, y, name=None):
return paddle_frontend.remainder(self, y)
def is_floating_point(self):
return paddle_frontend.is_floating_point(self)
- @with_supported_dtypes({"2.5.1 and below": ("float32", "float64")}, "paddle")
+ @with_supported_dtypes({"2.5.2 and below": ("float32", "float64")}, "paddle")
def tanh_(self, name=None):
y = self.tanh(self)
return ivy.inplace_update(self, y)
- @with_supported_dtypes({"2.5.1 and below": ("float32", "float64")}, "paddle")
+ @with_supported_dtypes({"2.5.2 and below": ("float32", "float64")}, "paddle")
def reciprocal_(self, name=None):
y = self.reciprocal(self)
return ivy.inplace_update(self, y)
@with_unsupported_dtypes(
- {"2.5.1 and below": ("complex", "uint8", "uint16")}, "paddle"
+ {"2.5.2 and below": ("complex", "uint8", "uint16")}, "paddle"
)
def numpy(self):
return self.ivy_array.to_numpy()
- @with_unsupported_dtypes({"2.5.1 and below": ("float16", "bfloat16")}, "paddle")
+ @with_unsupported_dtypes({"2.5.2 and below": ("float16", "bfloat16")}, "paddle")
def nonzero(self):
return paddle_frontend.nonzero(self)
- @with_supported_dtypes({"2.5.1 and below": ("float32", "float64")}, "paddle")
+ @with_supported_dtypes({"2.5.2 and below": ("float32", "float64")}, "paddle")
def inner(self, y, name=None):
return paddle_frontend.inner(self, y, name)
- @with_supported_dtypes({"2.5.1 and below": ("float32", "float64")}, "paddle")
+ @with_supported_dtypes({"2.5.2 and below": ("float32", "float64")}, "paddle")
def mean(self, axis=None, keepdim=False, name=None):
return paddle_frontend.mean(self, axis=axis, keepdim=keepdim)
- @with_supported_dtypes({"2.5.1 and below": ("float32", "float64")}, "paddle")
+ @with_supported_dtypes({"2.5.2 and below": ("float32", "float64")}, "paddle")
def as_complex(self, name=None):
if self.ivy_array.shape[-1] != 2:
raise ivy.exceptions.IvyError(
@@ -787,18 +828,29 @@ def as_complex(self, name=None):
return value
@with_supported_dtypes(
- {"2.5.1 and below": ("float32", "float64", "int32", "int64")}, "paddle"
+ {"2.5.2 and below": ("int32", "int64", "float32", "float64", "bool")}, "paddle"
+ )
+ def not_equal(self, y, name=None):
+ return paddle_frontend.not_equal(self._ivy_array, y)
+
+ @with_supported_dtypes(
+ {"2.5.2 and below": ("float32", "float64", "int32", "int64")}, "paddle"
)
def less_equal(self, y, name=None):
return paddle_frontend.less_equal(self._ivy_array, y)
- @with_supported_dtypes({"2.5.1 and below": ("complex64", "complex128")}, "paddle")
+ @with_supported_dtypes({"2.5.2 and below": ("complex64", "complex128")}, "paddle")
def real(self, name=None):
return paddle_frontend.real(self._ivy_array)
+ @with_unsupported_dtypes({"2.5.2 and below": ("float16", "bfloat16")}, "paddle")
+ def t(self, name=None):
+ axes = list(range(len(self.ivy_array.shape)))[::-1]
+ return ivy.permute_dims(self.ivy_array, axes=axes)
+
@with_supported_dtypes(
{
- "2.5.1 and below": (
+ "2.5.2 and below": (
"bool",
"float16",
"float32",
@@ -813,9 +865,21 @@ def real(self, name=None):
def cast(self, dtype):
return paddle_frontend.cast(self, dtype)
+ @with_unsupported_dtypes({"2.5.2 and below": ("float16", "bfloat16")}, "paddle")
+ def bmm(self, y, transpose_x=False, transpose_y=False, name=None):
+ return paddle_frontend.bmm(self, y, transpose_x, transpose_y)
+
+ @with_supported_dtypes(
+ {"2.5.2 and below": ("float16", "float32", "float64", "int32", "int64")},
+ "paddle",
+ )
+ def fill_(self, value):
+ filled_tensor = paddle_frontend.full_like(self, value)
+ return ivy.inplace_update(self, filled_tensor)
+
@with_supported_dtypes(
{
- "2.5.1 and below": (
+ "2.5.2 and below": (
"bool",
"int32",
"int64",
@@ -828,3 +892,78 @@ def cast(self, dtype):
)
def unbind(self, axis=0):
return paddle_frontend.unbind(self._ivy_array, axis=axis)
+
+ @with_supported_dtypes(
+ {
+ "2.5.2 and below": (
+ "bool",
+ "int32",
+ "int64",
+ "float16",
+ "float32",
+ "float64",
+ )
+ },
+ "paddle",
+ )
+ def unique_consecutive(self, axis=0):
+ return paddle_frontend.unique_consecutive(self._ivy_array, axis=axis)
+
+ def cpu(self):
+ self.ivy_array = ivy.to_device(self.ivy_array, ivy.as_ivy_dev("cpu"))
+ return self
+
+ @with_unsupported_dtypes(
+ {"2.5.2 and below": ("int16", "complex64", "complex128")},
+ "paddle",
+ )
+ def split(self, num_or_sections, axis=0, name=None):
+ return paddle_frontend.split(self._ivy_array, num_or_sections, axis, name)
+
+ @with_supported_dtypes(
+ {"2.5.2 and below": ("float32", "float64", "int32", "int64")}, "paddle"
+ )
+ def frac(self, name=None):
+ return paddle_frontend.frac(self._ivy_array)
+
+ @with_unsupported_dtypes({"2.5.2 and below": ("float16", "bfloat16")}, "paddle")
+ def gather(self, y, name=None):
+ return paddle_frontend.gather(self, y)
+
+ @with_unsupported_dtypes(
+ {"2.5.2 and below": ("float16", "uint8", "int8", "bool")}, "paddle"
+ )
+ def gather_(self, y, name=None):
+ res = self.gather(self, y)
+ return ivy.inplace_update(self, res)
+
+ @with_supported_dtypes(
+ {"2.5.2 and below": ("float32", "float64", "int32", "int64")}, "paddle"
+ )
+ def heaviside(self, y, name=None):
+ return paddle_frontend.heaviside(self, y)
+
+ @with_supported_dtypes(
+ {"2.5.2 and below": ("bool", "int32", "int64", "float32", "float64")}, "paddle"
+ )
+ def expand(self, shape, name=None):
+ return paddle_frontend.expand(self._ivy_array, shape)
+
+ @with_supported_device_and_dtypes(
+ {
+ "2.5.2 and below": {
+ "cpu": (
+ "bool",
+ "int32",
+ "int64",
+ "float32",
+ "float64",
+ "complex64",
+ "complex128",
+ )
+ }
+ },
+ "paddle",
+ )
+ def tile(self, repeat_times):
+ return paddle_frontend.Tensor(ivy.tile(self._ivy_array, repeats=repeat_times))
diff --git a/ivy/functional/frontends/paddle/vision/transforms.py b/ivy/functional/frontends/paddle/vision/transforms.py
index 7c27fa7088e6c..dac89b602ea19 100644
--- a/ivy/functional/frontends/paddle/vision/transforms.py
+++ b/ivy/functional/frontends/paddle/vision/transforms.py
@@ -104,7 +104,7 @@ def _rgb_to_hsv(img):
# ------------ #
-@with_supported_dtypes({"2.5.1 and below": ("float32", "float64")}, "paddle")
+@with_supported_dtypes({"2.5.2 and below": ("float32", "float64")}, "paddle")
@to_ivy_arrays_and_back
def adjust_brightness(img, brightness_factor):
assert brightness_factor >= 0, "brightness_factor should be non-negative."
@@ -117,7 +117,7 @@ def adjust_brightness(img, brightness_factor):
return _blend_images(img, extreme_target, brightness_factor)
-@with_supported_dtypes({"2.5.1 and below": ("float32", "float64", "uint8")}, "paddle")
+@with_supported_dtypes({"2.5.2 and below": ("float32", "float64", "uint8")}, "paddle")
@to_ivy_arrays_and_back
def adjust_hue(img, hue_factor):
assert -0.5 <= hue_factor <= 0.5, "hue_factor should be in range [-0.5, 0.5]"
@@ -145,7 +145,7 @@ def adjust_hue(img, hue_factor):
@with_supported_dtypes(
- {"2.5.1 and below": ("float32", "float64", "int32", "int64")}, "paddle"
+ {"2.5.2 and below": ("float32", "float64", "int32", "int64")}, "paddle"
)
@to_ivy_arrays_and_back
def hflip(img):
@@ -154,7 +154,7 @@ def hflip(img):
@with_supported_dtypes(
- {"2.5.1 and below": ("float32", "float64", "int32", "int64")}, "paddle"
+ {"2.5.2 and below": ("float32", "float64", "int32", "int64")}, "paddle"
)
def normalize(img, mean, std, data_format="CHW", to_rgb=False):
if ivy.is_array(img):
@@ -171,7 +171,7 @@ def normalize(img, mean, std, data_format="CHW", to_rgb=False):
@with_supported_dtypes(
- {"2.5.1 and below": ("float32", "float64", "int32", "int64")}, "paddle"
+ {"2.5.2 and below": ("float32", "float64", "int32", "int64")}, "paddle"
)
@to_ivy_arrays_and_back
def pad(img, padding, fill=0, padding_mode="constant"):
@@ -192,16 +192,16 @@ def pad(img, padding, fill=0, padding_mode="constant"):
elif dim_size == 3:
trans_padding = ((0, 0), (padding[1], padding[3]), (padding[0], padding[2]))
else:
- raise "padding can only be 1D with size 1, 2, 4 only"
+ raise ValueError("padding can only be 1D with size 1, 2, 4 only")
if padding_mode in ["constant", "edge", "reflect", "symmetric"]:
return ivy.pad(img, trans_padding, mode=padding_mode, constant_values=fill)
else:
- raise "Unsupported padding_mode"
+ raise ValueError("Unsupported padding_mode")
@with_supported_dtypes(
- {"2.5.1 and below": ("float32", "float64", "int32", "int64")}, "paddle"
+ {"2.5.2 and below": ("float32", "float64", "int32", "int64")}, "paddle"
)
@to_ivy_arrays_and_back
def to_tensor(pic, data_format="CHW"):
@@ -211,7 +211,7 @@ def to_tensor(pic, data_format="CHW"):
@with_unsupported_device_and_dtypes(
{
- "2.5.1 and below": {
+ "2.5.2 and below": {
"cpu": ("int8", "uint8", "int16", "float16", "bfloat16", "bool")
}
},
diff --git a/ivy/functional/frontends/pandas/func_wrapper.py b/ivy/functional/frontends/pandas/func_wrapper.py
index 2036f26eef1c9..7d5f66c4a0966 100644
--- a/ivy/functional/frontends/pandas/func_wrapper.py
+++ b/ivy/functional/frontends/pandas/func_wrapper.py
@@ -1,4 +1,4 @@
-# function wrappers for pandas frontend to handle commmon operations
+# function wrappers for pandas frontend to handle common operations
from functools import wraps
diff --git a/ivy/functional/frontends/pandas/index.py b/ivy/functional/frontends/pandas/index.py
index f8cfef5e30010..44b4cefdf3def 100644
--- a/ivy/functional/frontends/pandas/index.py
+++ b/ivy/functional/frontends/pandas/index.py
@@ -30,7 +30,7 @@ def __init__(self, data, dtype=None, copy=False, name=None, tupleize_cols=True):
@staticmethod
def _tokenize_1d(x: Iterable):
- return ivy.array(list(v for v, _ in enumerate(x)))
+ return ivy.array([v for v, _ in enumerate(x)])
def __repr__(self):
if self.tokens_exist:
diff --git a/ivy/functional/frontends/scipy/linalg/linalg.py b/ivy/functional/frontends/scipy/linalg/linalg.py
index f81f214043312..ec65b318d268c 100644
--- a/ivy/functional/frontends/scipy/linalg/linalg.py
+++ b/ivy/functional/frontends/scipy/linalg/linalg.py
@@ -75,7 +75,7 @@ def norm(a, /, *, ord=None, axis=None, keepdims=False, check_finite=True):
if check_finite:
_check_finite(a)
- if axis is None and not (ord is None):
+ if axis is None and ord is not None:
if a.ndim not in (1, 2):
raise ValueError("Improper number of dimensions to norm.")
else:
diff --git a/ivy/functional/frontends/sklearn/datasets/_samples_generator.py b/ivy/functional/frontends/sklearn/datasets/_samples_generator.py
index cf7bd48bf54b8..41e214700ea3f 100644
--- a/ivy/functional/frontends/sklearn/datasets/_samples_generator.py
+++ b/ivy/functional/frontends/sklearn/datasets/_samples_generator.py
@@ -1,7 +1,9 @@
import ivy
import numbers
+from ivy.functional.frontends.numpy.func_wrapper import outputs_to_frontend_arrays
+@outputs_to_frontend_arrays
def make_circles(
n_samples=100, *, shuffle=True, noise=None, random_state=None, factor=0.8
):
@@ -41,6 +43,7 @@ def make_circles(
return X, y
+@outputs_to_frontend_arrays
def make_moons(n_samples=100, *, shuffle=True, noise=None, random_state=None):
if isinstance(n_samples, numbers.Integral):
n_samples_out = n_samples // 2
diff --git a/ivy/functional/frontends/sklearn/model_selection/_split.py b/ivy/functional/frontends/sklearn/model_selection/_split.py
index 25b51c3451fe6..58f68bcc63bf6 100644
--- a/ivy/functional/frontends/sklearn/model_selection/_split.py
+++ b/ivy/functional/frontends/sklearn/model_selection/_split.py
@@ -74,7 +74,7 @@ def __init__(
)
def _iter_test_indices(self, X=None, y=None, groups=None):
- ivy.seed(self.random_state)
+ ivy.seed(seed_value=self.random_state)
y = ivy.array(y)
y = column_or_1d(y)
_, y_idx, y_inv, _ = ivy.unique_all(y, return_index=True, return_inverse=True)
@@ -139,7 +139,7 @@ def train_test_split(
indices = ivy.arange(0, n_train + n_test)
if shuffle:
if random_state is not None:
- ivy.seed(random_state)
+ ivy.seed(seed_value=random_state)
indices = ivy.shuffle(indices)
train_indices = indices[:n_train]
test_indices = indices[n_train:]
diff --git a/ivy/functional/frontends/sklearn/utils/multiclass.py b/ivy/functional/frontends/sklearn/utils/multiclass.py
index 16e83ba2f8459..3ce04943a7598 100644
--- a/ivy/functional/frontends/sklearn/utils/multiclass.py
+++ b/ivy/functional/frontends/sklearn/utils/multiclass.py
@@ -1,7 +1,7 @@
import ivy
-# reapeated utility function
+# repeated utility function
def type_of_target(y, input_name="y"):
# purely utility function
unique_vals = len(ivy.unique_values(y))
diff --git a/ivy/functional/frontends/tensorflow/__init__.py b/ivy/functional/frontends/tensorflow/__init__.py
index 722c41ff9bb77..8f41d31818161 100644
--- a/ivy/functional/frontends/tensorflow/__init__.py
+++ b/ivy/functional/frontends/tensorflow/__init__.py
@@ -84,11 +84,12 @@ def check_tensorflow_casting(x1, x2):
from . import dtypes
-from .dtypes import DType, as_dtype, cast
+from .dtypes import as_dtype, cast
from . import ragged
from .ragged import *
from . import tensor
from .tensor import EagerTensor, Tensor
+from .tensorarray import TensorArray
from . import variable
from .variable import Variable, IndexedSlices
from . import keras
diff --git a/ivy/functional/frontends/tensorflow/compat/v1/nn.py b/ivy/functional/frontends/tensorflow/compat/v1/nn.py
index c1f4546011222..d1cfcd3c5d3d9 100644
--- a/ivy/functional/frontends/tensorflow/compat/v1/nn.py
+++ b/ivy/functional/frontends/tensorflow/compat/v1/nn.py
@@ -5,7 +5,7 @@
import ivy.functional.frontends.tensorflow.nn as tf_nn
-@with_unsupported_dtypes({"2.13.0 and below": ("float16",)}, "tensorflow")
+@with_unsupported_dtypes({"2.14.0 and below": ("float16",)}, "tensorflow")
def depthwise_conv2d(
input,
filter,
@@ -30,7 +30,7 @@ def depthwise_conv2d(
# should have float16 as well but sqrt doesn't support it
@to_ivy_arrays_and_back
-@with_supported_dtypes({"2.13.0 and below": ("float32",)}, "tensorflow")
+@with_supported_dtypes({"2.14.0 and below": ("float32",)}, "tensorflow")
def fused_batch_norm(
x,
scale,
@@ -105,7 +105,7 @@ def fused_batch_norm(
@to_ivy_arrays_and_back
@with_unsupported_dtypes(
- {"2.13.0 and below": ("float16",)},
+ {"2.14.0 and below": ("float16",)},
"tensorflow",
)
def max_pool(value, ksize, strides, padding, data_format="NHWC", name=None, input=None):
@@ -124,7 +124,7 @@ def max_pool(value, ksize, strides, padding, data_format="NHWC", name=None, inpu
@with_unsupported_dtypes(
{
- "2.13.0 and below": (
+ "2.14.0 and below": (
"float16",
"bfloat16",
)
diff --git a/ivy/functional/frontends/tensorflow/general_functions.py b/ivy/functional/frontends/tensorflow/general_functions.py
index fbfbce38fff63..4464816ce98f1 100644
--- a/ivy/functional/frontends/tensorflow/general_functions.py
+++ b/ivy/functional/frontends/tensorflow/general_functions.py
@@ -62,7 +62,7 @@ def boolean_mask(tensor, mask, axis=None, name=None):
return ivy.get_item(tensor, mask)
-@with_supported_dtypes({"2.13.0 and below": ("float32",)}, "tensorflow")
+@with_supported_dtypes({"2.14.0 and below": ("float32",)}, "tensorflow")
@to_ivy_arrays_and_back
def clip_by_global_norm(t_list, clip_norm, use_norm=None):
if use_norm is not None:
@@ -76,7 +76,7 @@ def clip_by_global_norm(t_list, clip_norm, use_norm=None):
], global_norm
-@with_supported_dtypes({"2.13.0 and below": ("float", "complex")}, "tensorflow")
+@with_supported_dtypes({"2.14.0 and below": ("float", "complex")}, "tensorflow")
@to_ivy_arrays_and_back
def clip_by_norm(t, clip_norm, axes=None):
t, clip_norm = check_tensorflow_casting(t, clip_norm)
@@ -86,9 +86,7 @@ def clip_by_norm(t, clip_norm, axes=None):
l2sum_safe = ivy.where(pred, l2sum, ivy.ones_like(l2sum))
l2norm = ivy.where(pred, ivy.sqrt(l2sum_safe), l2sum)
intermediate = t * clip_norm
- assert (
- t.shape == intermediate.shape
- ), "Dimensions {} and {} are not compatible".format(
+ assert t.shape == intermediate.shape, "Dimensions %s and %s are not compatible" % (
t.shape,
intermediate.shape,
)
@@ -97,7 +95,7 @@ def clip_by_norm(t, clip_norm, axes=None):
@to_ivy_arrays_and_back
-@with_unsupported_dtypes({"2.13.0 and below": ("float16",)}, "tensorflow")
+@with_unsupported_dtypes({"2.14.0 and below": ("float16",)}, "tensorflow")
def clip_by_value(t, clip_value_min, clip_value_max):
ivy.utils.assertions.check_all_or_any_fn(
clip_value_min,
@@ -172,7 +170,7 @@ def expand_dims(input, axis, name=None):
return ivy.expand_dims(input, axis=axis)
-@with_unsupported_dtypes({"2.13.0 and below": ("float16", "bfloat16")}, "tensorflow")
+@with_unsupported_dtypes({"2.14.0 and below": ("float16", "bfloat16")}, "tensorflow")
@handle_tf_dtype
@to_ivy_arrays_and_back
def eye(num_rows, num_columns=None, batch_shape=None, dtype=ivy.float32, name=None):
@@ -257,8 +255,7 @@ def gather(params, indices, validate_indices=None, axis=None, batch_dims=0, name
axis = batch_dims
else:
axis = axis % len(params.shape)
- if axis < batch_dims:
- axis = batch_dims
+ axis = max(axis, batch_dims)
return ivy.gather(params, indices, axis=axis, batch_dims=batch_dims)
@@ -302,7 +299,7 @@ def no_op(name=None):
return
-@with_supported_dtypes({"2.13.0 and below": ("float32", "float64")}, "tensorflow")
+@with_supported_dtypes({"2.14.0 and below": ("float32", "float64")}, "tensorflow")
@to_ivy_arrays_and_back
def norm(tensor, ord="euclidean", axis=None, keepdims=None, name=None):
return tf_frontend.linalg.norm(
@@ -324,7 +321,7 @@ def one_hot(
return ivy.one_hot(indices, depth)
-@with_unsupported_dtypes({"2.13.0 and below": ("float16", "bfloat16")}, "tensorflow")
+@with_unsupported_dtypes({"2.14.0 and below": ("float16", "bfloat16")}, "tensorflow")
@handle_tf_dtype
@to_ivy_arrays_and_back
def ones(shape, dtype=ivy.float32, name=None):
@@ -343,7 +340,7 @@ def pad(tensor, paddings, mode="CONSTANT", constant_values=0, name=None):
return ivy.pad(tensor, paddings, mode=mode.lower(), constant_values=constant_values)
-@with_unsupported_dtypes({"2.13.0 and below": ("float16", "bfloat16")}, "tensorflow")
+@with_unsupported_dtypes({"2.14.0 and below": ("float16", "bfloat16")}, "tensorflow")
@handle_tf_dtype
@to_ivy_arrays_and_back
def range(start, limit=None, delta=1, dtype=None, name=None):
@@ -355,7 +352,7 @@ def rank(input, **kwargs):
return ivy.astype(ivy.array(input.ndim), ivy.int32)
-@with_unsupported_dtypes({"2.13.0 and below": ("unsigned", "integer")}, "tensorflow")
+@with_unsupported_dtypes({"2.14.0 and below": ("unsigned", "integer")}, "tensorflow")
@to_ivy_arrays_and_back
def realdiv(x, y, name=None):
x, y = check_tensorflow_casting(x, y)
@@ -458,7 +455,7 @@ def sort(values, axis=-1, direction="ASCENDING", name=None):
@with_unsupported_dtypes(
- {"2.13.0 and below": ("uint8", "uint16", "uint32", "uint64", "int16")}, "tensorflow"
+ {"2.14.0 and below": ("uint8", "uint16", "uint32", "uint64", "int16")}, "tensorflow"
)
@to_ivy_arrays_and_back
def split(value, num_or_size_splits, axis=0, num=None, name=None):
@@ -565,8 +562,8 @@ def strided_slice(
if new_axis_mask[i]:
full_slice += (ivy.newaxis,)
else:
- b = begin[i] if not begin_mask[i] else None
- e = end[i] if not end_mask[i] else None
+ b = None if begin_mask[i] else begin[i]
+ e = None if end_mask[i] else end[i]
s = strides[i]
if b is None and e is None:
s = 1 if ellipsis_mask[i] else s
@@ -588,7 +585,14 @@ def strided_slice(
return ret
-@with_unsupported_dtypes({"2.13.0 and below": ("uint16",)}, "tensorflow")
+@to_ivy_arrays_and_back
+def tensor_scatter_nd_add(tensor, indices, updates, name=None):
+ zero_tensor = ivy.zeros_like(tensor)
+ scatter_tensor = ivy.scatter_nd(indices, updates, zero_tensor.shape)
+ return ivy.add(tensor, scatter_tensor)
+
+
+@with_unsupported_dtypes({"2.14.0 and below": ("uint16",)}, "tensorflow")
@to_ivy_arrays_and_back
def tile(input, multiples, name=None):
return ivy.tile(input, multiples)
@@ -604,14 +608,14 @@ def transpose(a, perm=None, conjugate=False, name="transpose"):
return ivy.permute_dims(a, axes=perm)
-@with_unsupported_dtypes({"2.13.0 and below": ("float16", "bfloat16")}, "tensorflow")
+@with_unsupported_dtypes({"2.14.0 and below": ("float16", "bfloat16")}, "tensorflow")
@to_ivy_arrays_and_back
def truncatediv(x, y, name=None):
return x.trunc_divide(y)
@with_unsupported_dtypes(
- {"2.13.0 and below": ("int16", "int8", "uint8", " uint16")}, "tensorflow"
+ {"2.14.0 and below": ("int16", "int8", "uint8", " uint16")}, "tensorflow"
)
@to_ivy_arrays_and_back
def truncatemod(x, y):
@@ -672,7 +676,7 @@ def unravel_index(indices, dims, out=None, name=None):
return ivy.unravel_index(indices, dims, out=out)
-@with_unsupported_dtypes({"2.13.0 and below": ("float16", "bfloat16")}, "tensorflow")
+@with_unsupported_dtypes({"2.14.0 and below": ("float16", "bfloat16")}, "tensorflow")
@to_ivy_arrays_and_back
def unstack(value: ivy.Array, axis=0, num=None, name=None):
return ivy.unstack(value, axis=axis)
@@ -707,7 +711,7 @@ def zeros(shape, dtype=ivy.float32, name=None):
return ivy.zeros(shape=shape, dtype=dtype)
-@with_unsupported_dtypes({"2.13.0 and below": ("float16", "bfloat16")}, "tensorflow")
+@with_unsupported_dtypes({"2.14.0 and below": ("float16", "bfloat16")}, "tensorflow")
@to_ivy_arrays_and_back
def zeros_initializer(shape, dtype=None, name=None):
# todo internal: fix behaviour
diff --git a/ivy/functional/frontends/tensorflow/image/cropping.py b/ivy/functional/frontends/tensorflow/image/cropping.py
index b823ec4e93146..f7d71422bfc45 100644
--- a/ivy/functional/frontends/tensorflow/image/cropping.py
+++ b/ivy/functional/frontends/tensorflow/image/cropping.py
@@ -7,7 +7,7 @@
from ivy.func_wrapper import with_supported_dtypes
-@with_supported_dtypes({"2.13.0 and below": ("float",)}, "tensorflow")
+@with_supported_dtypes({"2.14.0 and below": ("float",)}, "tensorflow")
@to_ivy_arrays_and_back
def extract_patches(images, sizes, strides, rates, padding):
depth = images.shape[-1]
@@ -46,7 +46,7 @@ def resize(
else:
new_height, new_width = size
if method == "bicubic":
- method = "bicubic_tensorflow"
+ method = "tf_bicubic"
elif method == "area":
method = "tf_area"
image = ivy.interpolate(
diff --git a/ivy/functional/frontends/tensorflow/keras/activations.py b/ivy/functional/frontends/tensorflow/keras/activations.py
index 9b5e1cf4605b5..5ee4693e3977f 100644
--- a/ivy/functional/frontends/tensorflow/keras/activations.py
+++ b/ivy/functional/frontends/tensorflow/keras/activations.py
@@ -17,7 +17,7 @@
@with_supported_dtypes(
- {"2.13.0 and below": ("float16", "float32", "float64")},
+ {"2.14.0 and below": ("float16", "float32", "float64")},
"tensorflow",
)
def deserialize(name, custom_objects=None):
@@ -47,7 +47,7 @@ def deserialize(name, custom_objects=None):
@with_supported_dtypes(
- {"2.13.0 and below": ("bfloat16", "float16", "float32", "float64")},
+ {"2.14.0 and below": ("bfloat16", "float16", "float32", "float64")},
"tensorflow",
)
@to_ivy_arrays_and_back
@@ -103,7 +103,7 @@ def relu(x, alpha=0.0, max_value=None, threshold=0.0):
@with_supported_dtypes(
- {"2.13.0 and below": ("float16", "float32", "float64")},
+ {"2.14.0 and below": ("float16", "float32", "float64")},
"tensorflow",
)
@to_ivy_arrays_and_back
@@ -112,7 +112,7 @@ def selu(x):
@with_supported_dtypes(
- {"2.13.0 and below": ("float16", "float32", "float64")},
+ {"2.14.0 and below": ("float16", "float32", "float64")},
"tensorflow",
)
def serialize(activation, use_legacy_format=False, custom_objects=None):
diff --git a/ivy/functional/frontends/tensorflow/keras/metrics.py b/ivy/functional/frontends/tensorflow/keras/metrics.py
index d025122e73f65..d32ef36cf1ebc 100644
--- a/ivy/functional/frontends/tensorflow/keras/metrics.py
+++ b/ivy/functional/frontends/tensorflow/keras/metrics.py
@@ -72,9 +72,8 @@ def _in_top_k(targets, predictions, topk):
targets_batch,
pred_batch,
message=(
- "first dim of predictions: {} must match targets length: {}".format(
- pred_batch, targets_batch
- )
+ f"first dim of predictions: {pred_batch} must match targets length:"
+ f" {targets_batch}"
),
as_array=False,
)
diff --git a/ivy/functional/frontends/tensorflow/linalg.py b/ivy/functional/frontends/tensorflow/linalg.py
index a329ecdabcd15..fdbe33455fb1c 100644
--- a/ivy/functional/frontends/tensorflow/linalg.py
+++ b/ivy/functional/frontends/tensorflow/linalg.py
@@ -38,7 +38,7 @@ def symmetrize(input):
@to_ivy_arrays_and_back
-@with_unsupported_dtypes({"2.13.0 and below": ("float16", "bfloat16")}, "tensorflow")
+@with_unsupported_dtypes({"2.14.0 and below": ("float16", "bfloat16")}, "tensorflow")
def cholesky_solve(chol, rhs, name=None):
chol, rhs = check_tensorflow_casting(chol, rhs)
y = ivy.solve(chol, rhs)
@@ -107,7 +107,7 @@ def eigh(tensor, name=None):
@to_ivy_arrays_and_back
@with_supported_dtypes(
- {"2.13.0 and below": ("float32", "float64", "complex64", "complex128")},
+ {"2.14.0 and below": ("float32", "float64", "complex64", "complex128")},
"tensorflow",
)
def eigvals(tensor, name=None):
@@ -130,12 +130,12 @@ def expm(input, name=None):
@handle_tf_dtype
@to_ivy_arrays_and_back
-@with_unsupported_dtypes({"2.13.0 and below": ("float16", "bfloat16")}, "tensorflow")
+@with_unsupported_dtypes({"2.14.0 and below": ("float16", "bfloat16")}, "tensorflow")
def eye(num_rows, num_columns=None, batch_shape=None, dtype=ivy.float32, name=None):
return ivy.eye(num_rows, num_columns, batch_shape=batch_shape, dtype=dtype)
-@with_supported_dtypes({"2.13.0 and below": ("float32", "float64")}, "tensorflow")
+@with_supported_dtypes({"2.14.0 and below": ("float32", "float64")}, "tensorflow")
@to_ivy_arrays_and_back
def global_norm(t_list, name=None):
l2_norms = [ivy.sqrt(ivy.sum(ivy.square(t))) ** 2 for t in t_list if t is not None]
@@ -145,7 +145,7 @@ def global_norm(t_list, name=None):
@to_ivy_arrays_and_back
@with_supported_dtypes(
{
- "2.13.0 and below": (
+ "2.14.0 and below": (
"float32",
"float64",
"complex64",
@@ -159,7 +159,7 @@ def inv(input, adjoint=False, name=None):
@to_ivy_arrays_and_back
-@with_supported_dtypes({"2.13.0 and below": ("float32", "float64")}, "tensorflow")
+@with_supported_dtypes({"2.14.0 and below": ("float32", "float64")}, "tensorflow")
def l2_normalize(x, axis=None, epsilon=1e-12, name=None):
square_sum = ivy.sum(ivy.square(x), axis=axis, keepdims=True)
x_inv_norm = ivy.reciprocal(ivy.sqrt(ivy.maximum(square_sum, epsilon)))
@@ -168,7 +168,7 @@ def l2_normalize(x, axis=None, epsilon=1e-12, name=None):
@to_ivy_arrays_and_back
@with_supported_dtypes(
- {"2.13.0 and below": ("float16", "float32", "float64", "complex64", "complex128")},
+ {"2.14.0 and below": ("float16", "float32", "float64", "complex64", "complex128")},
"tensorflow",
)
def logdet(matrix, name=None):
@@ -185,7 +185,7 @@ def lu_matrix_inverse(lower_upper, perm, validate_args=False, name=None):
@to_ivy_arrays_and_back
@with_supported_dtypes(
{
- "2.13.0 and below": (
+ "2.14.0 and below": (
"float16",
"float32",
"float64",
@@ -244,7 +244,7 @@ def matrix_transpose(a, name="matrix_transpose", conjugate=False):
return ivy.matrix_transpose(a)
-@with_supported_dtypes({"2.13.0 and below": ("float32", "float64")}, "tensorflow")
+@with_supported_dtypes({"2.14.0 and below": ("float32", "float64")}, "tensorflow")
@to_ivy_arrays_and_back
def norm(tensor, ord="euclidean", axis=None, keepdims=None, name=None):
keepdims = keepdims or False
@@ -257,7 +257,7 @@ def norm(tensor, ord="euclidean", axis=None, keepdims=None, name=None):
@to_ivy_arrays_and_back
-@with_supported_dtypes({"2.13.0 and below": ("float32", "float64")}, "tensorflow")
+@with_supported_dtypes({"2.14.0 and below": ("float32", "float64")}, "tensorflow")
def normalize(tensor, ord="euclidean", axis=None, name=None):
tensor = tf_frontend.convert_to_tensor(
tensor, dtype=ivy.dtype(tensor), dtype_hint="Any"
@@ -280,7 +280,7 @@ def qr(input, /, *, full_matrices=False, name=None):
@to_ivy_arrays_and_back
@with_supported_dtypes(
{
- "2.13.0 and below": (
+ "2.14.0 and below": (
"bfloat16",
"half",
"float32",
@@ -350,7 +350,7 @@ def slogdet(input, name=None):
@to_ivy_arrays_and_back
-@with_unsupported_dtypes({"2.13.0 and below": ("float16", "bfloat16")}, "tensorflow")
+@with_unsupported_dtypes({"2.14.0 and below": ("float16", "bfloat16")}, "tensorflow")
def solve(matrix, rhs, /, *, adjoint=False, name=None):
matrix, rhs = check_tensorflow_casting(matrix, rhs)
return ivy.solve(matrix, rhs, adjoint=adjoint)
@@ -364,7 +364,7 @@ def svd(a, /, *, full_matrices=False, compute_uv=True, name=None):
@to_ivy_arrays_and_back
@with_supported_dtypes(
{
- "2.13.0 and below": (
+ "2.14.0 and below": (
"bfloat16",
"half",
"float32",
@@ -388,7 +388,7 @@ def tensor_diag(diagonal, /, *, name=None):
@to_ivy_arrays_and_back
@with_supported_dtypes(
{
- "2.13.0 and below": (
+ "2.14.0 and below": (
"float32",
"float64",
"int32",
@@ -424,7 +424,7 @@ def tensor_diag_part(input, name=None):
@to_ivy_arrays_and_back
@with_supported_dtypes(
- {"2.13.0 and below": ("float32", "float64", "int32")}, "tensorflow"
+ {"2.14.0 and below": ("float32", "float64", "int32")}, "tensorflow"
)
def tensordot(a, b, axes, name=None):
a, b = check_tensorflow_casting(a, b)
@@ -436,7 +436,7 @@ def tensordot(a, b, axes, name=None):
@to_ivy_arrays_and_back
@with_unsupported_dtypes(
{
- "2.13.0 and below": (
+ "2.14.0 and below": (
"float16",
"bfloat16",
"int8",
diff --git a/ivy/functional/frontends/tensorflow/math.py b/ivy/functional/frontends/tensorflow/math.py
index 03db2298fb2e2..9dd3a44045d53 100644
--- a/ivy/functional/frontends/tensorflow/math.py
+++ b/ivy/functional/frontends/tensorflow/math.py
@@ -17,7 +17,7 @@
{
"1.2.0": ("float16", "complex64", "complex128"),
"1.8.0 and below": ("float16",),
- "2.13.0 and below": ("int8", "int16", "uint8", "uint16", "uint32", "uint64"),
+ "2.14.0 and below": ("int8", "int16", "uint8", "uint16", "uint32", "uint64"),
},
"tensorflow",
)
@@ -105,7 +105,7 @@ def atanh(x, name="atanh"):
@with_supported_dtypes(
- {"2.13.0 and below": ("int32",)},
+ {"2.14.0 and below": ("int32",)},
"tensorflow",
)
@to_ivy_arrays_and_back
@@ -297,7 +297,7 @@ def greater_equal(x, y, name=None):
@with_supported_device_and_dtypes(
{
- "2.13.0 and below": {
+ "2.14.0 and below": {
"cpu": ("float32", "float64"),
"gpu": ("bfloat16", "float16", "float32", "float64"),
}
@@ -310,7 +310,7 @@ def igamma(a, x, name=None):
@with_supported_dtypes(
- {"2.13.0 and below": ("float16", "float32", "float64", "complex64", "complex128")},
+ {"2.14.0 and below": ("float16", "float32", "float64", "complex64", "complex128")},
"tensorflow",
)
@to_ivy_arrays_and_back
@@ -326,7 +326,7 @@ def in_top_k(target, pred, k, name=None):
@with_supported_dtypes(
{
- "2.13.0 and below": ("int32", "int64"),
+ "2.14.0 and below": ("int32", "int64"),
},
"tensorflow",
)
@@ -337,7 +337,7 @@ def invert_permutation(x, name=None):
@with_supported_dtypes(
{
- "2.11.0 and below": ("bfloat16", "half", "float32", "float64"),
+ "2.14.0 and below": ("bfloat16", "half", "float32", "float64"),
},
"tensorflow",
)
@@ -375,7 +375,7 @@ def is_strictly_increasing(x, name="is_strictly_increasing"):
@to_ivy_arrays_and_back
-@with_supported_dtypes({"2.13.0 and below": ("float32", "float64")}, "tensorflow")
+@with_supported_dtypes({"2.14.0 and below": ("float32", "float64")}, "tensorflow")
def l2_normalize(x, axis=None, epsilon=1e-12, name=None):
square_sum = ivy.sum(ivy.square(x), axis=axis, keepdims=True)
x_inv_norm = ivy.reciprocal(ivy.sqrt(ivy.maximum(square_sum, epsilon)))
@@ -394,6 +394,13 @@ def less_equal(x, y, name="LessEqual"):
return ivy.less_equal(x, y)
+# lgamma
+@to_ivy_arrays_and_back
+@with_supported_dtypes({"2.14.0 and below": ("float32", "float64")}, "tensorflow")
+def lgamma(x, name=None):
+ return ivy.lgamma(x)
+
+
@to_ivy_arrays_and_back
def log(x, name=None):
return ivy.log(x)
@@ -447,7 +454,7 @@ def minimum(x, y, name=None):
@to_ivy_arrays_and_back
-@with_unsupported_dtypes({"2.5.1 and below": ("bfloat16",)}, "paddle")
+@with_unsupported_dtypes({"2.5.2 and below": ("bfloat16",)}, "paddle")
def mod(x, y, name=None):
x, y = check_tensorflow_casting(x, y)
return ivy.remainder(x, y)
@@ -602,7 +609,7 @@ def reduce_variance(input_tensor, axis=None, keepdims=False, name="reduce_varian
@with_supported_device_and_dtypes(
{
- "2.13.0 and below": {
+ "2.14.0 and below": {
"cpu": ("float32", "float64"),
"gpu": ("bfloat16", "float16", "float32", "float64"),
}
@@ -637,7 +644,7 @@ def sigmoid(x, name=None):
@with_supported_dtypes(
{
- "2.13.0 and below": (
+ "2.14.0 and below": (
"bfloat16",
"float16",
"float32",
@@ -669,7 +676,7 @@ def softplus(features, name=None):
@with_supported_dtypes(
- {"2.13.0 and below": ("bfloat32", "float32", "float64")}, "tensorflow"
+ {"2.14.0 and below": ("bfloat32", "float32", "float64")}, "tensorflow"
)
@to_ivy_arrays_and_back
def softsign(features, name=None):
@@ -688,7 +695,7 @@ def square(x, name=None):
@with_supported_dtypes(
{
- "2.13.0 and below": (
+ "2.14.0 and below": (
"bfloat16",
"float16",
"float32",
@@ -723,7 +730,7 @@ def tan(x, name=None):
@with_supported_dtypes(
- {"2.13.0 and below": ("float16", "float32", "float64", "complex64", "complex128")},
+ {"2.14.0 and below": ("float16", "float32", "float64", "complex64", "complex128")},
"tensorflow",
)
@to_ivy_arrays_and_back
@@ -764,6 +771,22 @@ def unsorted_segment_mean(
return x
+@to_ivy_arrays_and_back
+def unsorted_segment_min(data, segment_ids, num_segments, name="unsorted_segment_min"):
+ data = ivy.array(data)
+ segment_ids = ivy.array(segment_ids)
+
+ ivy.utils.assertions.check_equal(
+ list(segment_ids.shape), [list(data.shape)[0]], as_array=False
+ )
+ min_array = ivy.zeros(
+ tuple([num_segments.item()] + (list(data.shape))[1:]), dtype=ivy.int32
+ )
+ for i in range((segment_ids).shape[0]):
+ min_array[segment_ids[i]] = ivy.minimum(min_array[segment_ids[i]], data[i])
+ return min_array
+
+
@to_ivy_arrays_and_back
def unsorted_segment_sqrt_n(
data, segment_ids, num_segments, name="unsorted_segement_sqrt_n"
@@ -797,7 +820,7 @@ def unsorted_segment_sum(data, segment_ids, num_segments, name="unsorted_segment
@with_supported_dtypes(
- {"2.13.0 and below": ("float32", "float64", "complex64", "complex128")},
+ {"2.14.0 and below": ("float32", "float64", "complex64", "complex128")},
"tensorflow",
)
@to_ivy_arrays_and_back
@@ -809,7 +832,7 @@ def xdivy(x, y, name=None):
@to_ivy_arrays_and_back
-@with_supported_dtypes({"2.13.0 and below": ("float32", "float64")}, "tensorflow")
+@with_supported_dtypes({"2.14.0 and below": ("float32", "float64")}, "tensorflow")
def xlog1py(x, y, name=None):
x, y = check_tensorflow_casting(x, y)
return x * ivy.log1p(y)
@@ -832,7 +855,7 @@ def zero_fraction(value, name="zero_fraction"):
@to_ivy_arrays_and_back
@with_supported_dtypes(
{
- "2.11.0 and below": ("float32", "float64"),
+ "2.14.0 and below": ("float32", "float64"),
},
"tensorflow",
)
diff --git a/ivy/functional/frontends/tensorflow/nn.py b/ivy/functional/frontends/tensorflow/nn.py
index 410dfe0d2e65b..1402aa0ec7f21 100644
--- a/ivy/functional/frontends/tensorflow/nn.py
+++ b/ivy/functional/frontends/tensorflow/nn.py
@@ -1,7 +1,7 @@
# global
import ivy
from ivy.functional.frontends.tensorflow.func_wrapper import to_ivy_arrays_and_back
-from ivy.func_wrapper import with_unsupported_dtypes
+from ivy.func_wrapper import with_unsupported_dtypes, with_supported_dtypes
from ivy.functional.frontends.tensorflow import check_tensorflow_casting
@@ -210,7 +210,7 @@ def conv3d(
)
-@with_unsupported_dtypes({"2.13.0 and below": ("bfloat16",)}, "tensorflow")
+@with_unsupported_dtypes({"2.14.0 and below": ("bfloat16",)}, "tensorflow")
@to_ivy_arrays_and_back
def conv3d_transpose(
input,
@@ -292,7 +292,7 @@ def ctc_unique_labels(labels, name=None):
return unique_pad, ctc_labels[2]
-@with_unsupported_dtypes({"2.13.0 and below": ("bfloat16",)}, "tensorflow")
+@with_unsupported_dtypes({"2.14.0 and below": ("bfloat16",)}, "tensorflow")
@to_ivy_arrays_and_back
def depthwise_conv2d(
input,
@@ -336,13 +336,13 @@ def gelu(features, approximate=False, name=None):
return ivy.gelu(features, approximate=approximate)
-@with_unsupported_dtypes({"2.13.0 and below": "float16"}, "tensorflow")
+@with_unsupported_dtypes({"2.14.0 and below": "float16"}, "tensorflow")
@to_ivy_arrays_and_back
def leaky_relu(features, alpha=0.2, name=None):
return ivy.leaky_relu(features, alpha=alpha)
-@with_unsupported_dtypes({"2.13.0 and below": ("bfloat16",)}, "tensorflow")
+@with_unsupported_dtypes({"2.14.0 and below": ("bfloat16",)}, "tensorflow")
@to_ivy_arrays_and_back
def local_response_normalization(
input, depth_radius=5, bias=1.0, alpha=1.0, beta=0.5, name=None
@@ -382,6 +382,12 @@ def max_pool2d(input, ksize, strides, padding, data_format="NHWC", name=None):
return ivy.max_pool2d(input, ksize, strides, padding, data_format=data_format)
+@with_supported_dtypes({"2.14.0 and below": ("float32",)}, "tensorflow")
+@to_ivy_arrays_and_back
+def max_pool3d(input, ksize, strides, padding, data_format="NDHWC", name=None):
+ return ivy.max_pool3d(input, ksize, strides, padding, data_format=data_format)
+
+
@to_ivy_arrays_and_back
def moments(x, axes, shift=None, keepdims=False, name=None):
return ivy.mean(x, axis=ivy.to_list(axes), keepdims=keepdims), ivy.var(
@@ -391,7 +397,7 @@ def moments(x, axes, shift=None, keepdims=False, name=None):
@with_unsupported_dtypes(
{
- "2.13.0 and below": (
+ "2.14.0 and below": (
"int8",
"int16",
"int32",
@@ -440,19 +446,19 @@ def pool(
)
-@with_unsupported_dtypes({"2.13.0 and below": ("complex",)}, "tensorflow")
+@with_unsupported_dtypes({"2.14.0 and below": ("complex",)}, "tensorflow")
@to_ivy_arrays_and_back
def relu(features, name=None):
return ivy.relu(features)
-@with_unsupported_dtypes({"2.13.0 and below": ("complex",)}, "tensorflow")
+@with_unsupported_dtypes({"2.14.0 and below": ("complex",)}, "tensorflow")
@to_ivy_arrays_and_back
def relu6(features, name=None):
return ivy.relu6(features)
-@with_unsupported_dtypes({"2.13.0 and below": ("bfloat16",)}, "tensorflow")
+@with_unsupported_dtypes({"2.14.0 and below": ("bfloat16",)}, "tensorflow")
@to_ivy_arrays_and_back
def separable_conv2d(
input,
@@ -479,7 +485,7 @@ def separable_conv2d(
@with_unsupported_dtypes(
{
- "2.13.0 and below": (
+ "2.14.0 and below": (
"int8",
"int16",
"int32",
@@ -506,7 +512,7 @@ def silu(features, beta: float = 1.0):
return ivy.multiply(features, ivy.sigmoid(ivy.multiply(beta, features)))
-@with_unsupported_dtypes({"2.13.0 and below": ("float16",)}, "tensorflow")
+@with_unsupported_dtypes({"2.14.0 and below": ("float16",)}, "tensorflow")
@to_ivy_arrays_and_back
def softmax(logits, axis=None, name=None):
return ivy.softmax(logits, axis=axis)
@@ -515,7 +521,7 @@ def softmax(logits, axis=None, name=None):
# Softsign
@with_unsupported_dtypes(
{
- "2.13.0 and below": (
+ "2.14.0 and below": (
"int8",
"int16",
"int32",
@@ -566,7 +572,7 @@ def sufficient_statistics(x, axes, shift=None, keepdims=False, name=None):
@with_unsupported_dtypes(
{
- "2.13.0 and below": (
+ "2.14.0 and below": (
"int8",
"int16",
"int32",
diff --git a/ivy/functional/frontends/tensorflow/random.py b/ivy/functional/frontends/tensorflow/random.py
index d881bd6d392bf..85b0dc873ec53 100644
--- a/ivy/functional/frontends/tensorflow/random.py
+++ b/ivy/functional/frontends/tensorflow/random.py
@@ -12,7 +12,7 @@ def gamma(shape, alpha, beta=None, dtype=ivy.float32, seed=None, name=None):
@with_unsupported_dtypes(
- {"2.13.0 and below": ("int8", "int16", "int32", "int64", "unsigned")}, "tensorflow"
+ {"2.14.0 and below": ("int8", "int16", "int32", "int64", "unsigned")}, "tensorflow"
)
@to_ivy_arrays_and_back
def normal(shape, mean=0.0, stddev=1.0, dtype=ivy.float32, seed=None, name=None):
@@ -20,7 +20,7 @@ def normal(shape, mean=0.0, stddev=1.0, dtype=ivy.float32, seed=None, name=None)
@with_unsupported_dtypes(
- {"2.13.0 and below": ("int8", "int16", "unsigned")}, "tensorflow"
+ {"2.14.0 and below": ("int8", "int16", "unsigned")}, "tensorflow"
)
@to_ivy_arrays_and_back
@handle_tf_dtype
@@ -34,7 +34,7 @@ def poisson(shape, lam, dtype=ivy.float32, seed=None, name=None):
# implement random shuffle
@with_unsupported_dtypes(
- {"2.13.0 and below": ("int8", "int16", "in32", "int64", "unsigned")}, "tensorflow"
+ {"2.14.0 and below": ("int8", "int16", "in32", "int64", "unsigned")}, "tensorflow"
)
@to_ivy_arrays_and_back
def shuffle(value, seed=None, name=None):
@@ -42,7 +42,7 @@ def shuffle(value, seed=None, name=None):
@with_unsupported_dtypes(
- {"2.13.0 and below": ("int8", "int16", "unsigned")}, "tensorflow"
+ {"2.14.0 and below": ("int8", "int16", "unsigned")}, "tensorflow"
)
@to_ivy_arrays_and_back
def stateless_normal(
@@ -54,7 +54,7 @@ def stateless_normal(
@with_unsupported_dtypes(
- {"2.13.0 and below": ("int8", "int16", "unsigned")}, "tensorflow"
+ {"2.14.0 and below": ("int8", "int16", "unsigned")}, "tensorflow"
)
@to_ivy_arrays_and_back
def stateless_poisson(shape, seed, lam, dtype=ivy.int32, name=None):
@@ -71,7 +71,7 @@ def stateless_uniform(
@with_unsupported_dtypes(
- {"2.13.0 and below": ("int8", "int16", "unsigned")}, "tensorflow"
+ {"2.14.0 and below": ("int8", "int16", "unsigned")}, "tensorflow"
)
@to_ivy_arrays_and_back
def uniform(shape, minval=0, maxval=None, dtype=ivy.float32, seed=None, name=None):
diff --git a/ivy/functional/frontends/tensorflow/raw_ops.py b/ivy/functional/frontends/tensorflow/raw_ops.py
index 354afa54bdb71..311fafa4c203a 100644
--- a/ivy/functional/frontends/tensorflow/raw_ops.py
+++ b/ivy/functional/frontends/tensorflow/raw_ops.py
@@ -18,7 +18,7 @@
AddV2 = to_ivy_arrays_and_back(map_raw_ops_alias(tf_frontend.math.add))
ArgMax = to_ivy_arrays_and_back(
with_unsupported_dtypes(
- {"2.13.0 and below": ("complex",)},
+ {"2.14.0 and below": ("complex",)},
"tensorflow",
)(
map_raw_ops_alias(
@@ -28,7 +28,7 @@
)
ArgMin = to_ivy_arrays_and_back(
with_unsupported_dtypes(
- {"2.13.0 and below": ("complex",)},
+ {"2.14.0 and below": ("complex",)},
"tensorflow",
)(
map_raw_ops_alias(
@@ -40,11 +40,26 @@
Atan = to_ivy_arrays_and_back(map_raw_ops_alias(tf_frontend.math.atan))
Atan2 = to_ivy_arrays_and_back(
with_unsupported_dtypes(
- {"2.13.0 and below": "float16"},
+ {"2.14.0 and below": "float16"},
"tensorflow",
)(map_raw_ops_alias(tf_frontend.math.atan2))
)
ConcatV2 = to_ivy_arrays_and_back(map_raw_ops_alias(tf_frontend.concat))
+Conj = to_ivy_arrays_and_back(
+ with_supported_dtypes(
+ {
+ "2.13.0 and below": ("complex64", "complex128", "variant"),
+ },
+ "tensorflow",
+ )(
+ map_raw_ops_alias(
+ tf_frontend.math.conj,
+ kwargs_to_update={
+ "input": "x",
+ },
+ )
+ )
+)
Cos = to_ivy_arrays_and_back(map_raw_ops_alias(tf_frontend.math.cos))
Cosh = to_ivy_arrays_and_back(map_raw_ops_alias(tf_frontend.math.cosh))
Cumprod = to_ivy_arrays_and_back(map_raw_ops_alias(tf_frontend.math.cumprod))
@@ -54,7 +69,7 @@
Einsum = to_ivy_arrays_and_back(
with_supported_dtypes(
{
- "2.13.0 and below": (
+ "2.14.0 and below": (
"bfloat16",
"complex128 ",
"complex64",
@@ -77,7 +92,7 @@
Igamma = to_ivy_arrays_and_back(
with_supported_dtypes(
{
- "2.13.0 and below": (
+ "2.14.0 and below": (
"float64",
"float32",
"half",
@@ -89,7 +104,7 @@
LeakyRelu = to_ivy_arrays_and_back(
with_supported_dtypes(
{
- "2.13.0 and below": ("bfloat16", "float16", "float32", "float64"),
+ "2.14.0 and below": ("bfloat16", "float16", "float32", "float64"),
},
"tensorflow",
)(
@@ -101,7 +116,7 @@
LessEqual = to_ivy_arrays_and_back(
with_unsupported_dtypes(
{
- "2.13.0 and below": ("complex",),
+ "2.14.0 and below": ("complex",),
},
"tensorflow",
)(map_raw_ops_alias(tf_frontend.math.less_equal))
@@ -110,7 +125,7 @@
LogSoftmax = to_ivy_arrays_and_back(
with_supported_dtypes(
{
- "2.13.0 and below": (
+ "2.14.0 and below": (
"bfloat16",
"float32",
"float64",
@@ -124,7 +139,7 @@
Max = to_ivy_arrays_and_back(
with_unsupported_dtypes(
{
- "2.13.0 and below": ("complex",),
+ "2.14.0 and below": ("complex",),
},
"tensorflow",
)(
@@ -137,10 +152,22 @@
)
)
)
+MaxPool3D = to_ivy_arrays_and_back(
+ with_supported_dtypes(
+ {
+ "2.14.0 and below": ("float32",),
+ },
+ "tensorflow",
+ )(
+ map_raw_ops_alias(
+ tf_frontend.nn.max_pool3d,
+ )
+ )
+)
Maximum = to_ivy_arrays_and_back(
with_unsupported_dtypes(
{
- "2.13.0 and below": ("complex",),
+ "2.14.0 and below": ("complex",),
},
"tensorflow",
)(map_raw_ops_alias(tf_frontend.math.maximum))
@@ -157,7 +184,7 @@
Min = to_ivy_arrays_and_back(
with_unsupported_dtypes(
{
- "2.13.0 and below": ("complex",),
+ "2.14.0 and below": ("complex",),
},
"tensorflow",
)(
@@ -170,13 +197,14 @@
)
)
)
+Mod = to_ivy_arrays_and_back(map_raw_ops_alias(tf_frontend.math.mod))
Mul = to_ivy_arrays_and_back(map_raw_ops_alias(tf_frontend.math.multiply))
Neg = to_ivy_arrays_and_back(map_raw_ops_alias(tf_frontend.math.negative))
Pow = to_ivy_arrays_and_back(map_raw_ops_alias(tf_frontend.math.pow))
RealDiv = to_ivy_arrays_and_back(
with_supported_dtypes(
{
- "2.13.0 and below": (
+ "2.14.0 and below": (
"complex",
"bfloat16",
"float16",
@@ -191,7 +219,7 @@
Relu = to_ivy_arrays_and_back(
with_unsupported_dtypes(
{
- "2.13.0 and below": ("complex", "float16"),
+ "2.14.0 and below": ("complex", "float16"),
},
"tensorflow",
)(map_raw_ops_alias(tf_frontend.nn.relu))
@@ -199,7 +227,7 @@
Relu6 = to_ivy_arrays_and_back(
with_unsupported_dtypes(
{
- "2.13.0 and below": ("complex", "float16"),
+ "2.14.0 and below": ("complex", "float16"),
},
"tensorflow",
)(
@@ -223,7 +251,7 @@
Softmax = to_ivy_arrays_and_back(
with_unsupported_dtypes(
{
- "2.13.0 and below": ("float16",),
+ "2.14.0 and below": ("float16",),
},
"tensorflow",
)(map_raw_ops_alias(tf_frontend.nn.softmax))
@@ -236,7 +264,7 @@
SquaredDifference = to_ivy_arrays_and_back(
with_supported_dtypes(
{
- "2.13.0 and below": (
+ "2.14.0 and below": (
"complex",
"bfloat16",
"float16",
@@ -259,7 +287,7 @@
Zeta = to_ivy_arrays_and_back(
with_supported_dtypes(
{
- "2.13.0 and below": ("float32", "float64"),
+ "2.14.0 and below": ("float32", "float64"),
},
"tensorflow",
)(map_raw_ops_alias(tf_frontend.math.zeta))
@@ -314,7 +342,7 @@ def Angle(
@with_unsupported_dtypes(
{
- "2.13.0 and below": (
+ "2.14.0 and below": (
"float16",
"bool",
"bfloat16",
@@ -498,7 +526,7 @@ def Diag(*, diagonal, name="Diag"):
@with_supported_dtypes(
- {"2.13.0 and below": ("bfloat16", "float16", "float32", "float64")},
+ {"2.14.0 and below": ("bfloat16", "float16", "float32", "float64")},
"tensorflow",
)
@to_ivy_arrays_and_back
@@ -553,6 +581,14 @@ def FFT2D(*, input, name="FFT2D"):
return ivy.astype(ivy.fft2(input, dim=(-2, -1)), input.dtype)
+@to_ivy_arrays_and_back
+def FFT3D(*, input, name="FFT3D"):
+ fft_result = ivy.fft(input, -1)
+ fft_result = ivy.fft(fft_result, -2)
+ fft_result = ivy.fft(fft_result, -3)
+ return ivy.astype(fft_result, input.dtype)
+
+
@to_ivy_arrays_and_back
def Fill(*, dims, value, name="Full"):
return ivy.full(dims, value)
@@ -739,7 +775,7 @@ def Shape(*, input, output_type=ivy.int32, name="Shape"):
@with_unsupported_dtypes(
- {"2.13.0 and below": ("unsigned",)},
+ {"2.14.0 and below": ("unsigned",)},
"tensorflow",
)
@to_ivy_arrays_and_back
@@ -784,7 +820,7 @@ def Sum(*, input, axis, keep_dims=False, name="Sum"):
@with_supported_dtypes(
- {"2.13.0 and below": ("float64", "float128", "halfcomplex64", "complex128")},
+ {"2.14.0 and below": ("float64", "float128", "halfcomplex64", "complex128")},
"tensorflow",
)
@to_ivy_arrays_and_back
@@ -808,7 +844,7 @@ def TruncateDiv(*, x, y, name="TruncateDiv"):
return ivy.astype(ivy.trunc_divide(x, y), x.dtype)
-@with_unsupported_dtypes({"2.13.0 and below": ("float16", "bfloat16")}, "tensorflow")
+@with_unsupported_dtypes({"2.14.0 and below": ("float16", "bfloat16")}, "tensorflow")
@to_ivy_arrays_and_back
def Unpack(*, value, num, axis=0, name="Unpack"):
return ivy.unstack(value, axis=axis)[:num]
@@ -821,7 +857,7 @@ def Xdivy(*, x, y, name="Xdivy"):
return ivy.divide(x, y)
-@with_unsupported_dtypes({"2.13.0 and below": ("bfloat16",)}, "tensorflow")
+@with_unsupported_dtypes({"2.14.0 and below": ("bfloat16",)}, "tensorflow")
@to_ivy_arrays_and_back
def Xlog1py(*, x, y, name="Xlog1py"):
if (x == 0).all():
diff --git a/ivy/functional/frontends/tensorflow/signal.py b/ivy/functional/frontends/tensorflow/signal.py
index 137e08e8133f5..0daf1db8a70c3 100644
--- a/ivy/functional/frontends/tensorflow/signal.py
+++ b/ivy/functional/frontends/tensorflow/signal.py
@@ -29,7 +29,7 @@ def kaiser_bessel_derived_window(
@with_supported_dtypes(
- {"2.13.0 and below": ("float32", "float64", "float16", "bfloat16")},
+ {"2.14.0 and below": ("float32", "float64", "float16", "bfloat16")},
"tensorflow",
)
@handle_tf_dtype
@@ -62,7 +62,7 @@ def stft(
@with_supported_dtypes(
- {"2.13.0 and below": ("float16", "float32", "float64", "bfloat16")},
+ {"2.14.0 and below": ("float16", "float32", "float64", "bfloat16")},
"tensorflow",
)
@to_ivy_arrays_and_back
diff --git a/ivy/functional/frontends/tensorflow/tensor.py b/ivy/functional/frontends/tensorflow/tensor.py
index 55a9aa8aee95a..517d842fbe2f5 100644
--- a/ivy/functional/frontends/tensorflow/tensor.py
+++ b/ivy/functional/frontends/tensorflow/tensor.py
@@ -45,7 +45,7 @@ def dtype(self):
@property
def shape(self):
- return tuple(self.ivy_array.shape.shape)
+ return TensorShape(self.ivy_array.shape.shape)
# Instance Methods #
# ---------------- #
@@ -108,7 +108,7 @@ def __floordiv__(self, y, name="floordiv"):
return tf_frontend.raw_ops.FloorDiv(x=self, y=y, name=name)
@with_unsupported_dtypes(
- {"2.13.0 and below": ("complex",)},
+ {"2.14.0 and below": ("complex",)},
"tensorflow",
)
def __ge__(self, y, name="ge"):
@@ -120,7 +120,7 @@ def __getitem__(self, slice_spec, var=None, name="getitem"):
return EagerTensor(ret)
@with_unsupported_dtypes(
- {"2.13.0 and below": ("complex",)},
+ {"2.14.0 and below": ("complex",)},
"tensorflow",
)
def __gt__(self, y, name="gt"):
@@ -130,14 +130,14 @@ def __invert__(self, name="invert"):
return tf_frontend.raw_ops.Invert(x=self, name=name)
@with_unsupported_dtypes(
- {"2.13.0 and below": ("complex",)},
+ {"2.14.0 and below": ("complex",)},
"tensorflow",
)
def __le__(self, y, name="le"):
return tf_frontend.raw_ops.LessEqual(x=self, y=y, name=name)
@with_unsupported_dtypes(
- {"2.13.0 and below": ("complex",)},
+ {"2.14.0 and below": ("complex",)},
"tensorflow",
)
def __lt__(self, y, name="lt"):
@@ -150,7 +150,7 @@ def __mul__(self, y, name="mul"):
return tf_frontend.math.multiply(self, y, name=name)
@with_unsupported_dtypes(
- {"2.13.0 and below": ("complex",)},
+ {"2.14.0 and below": ("complex",)},
"tensorflow",
)
def __mod__(self, y, name="mod"):
@@ -228,6 +228,114 @@ def __iter__(self):
yield self[i]
+class TensorShape:
+ # TODO: there are still some methods that may need implementing
+
+ def __init__(self, dims):
+ self._dims = tuple(dims)
+
+ def __repr__(self):
+ if self._dims is not None:
+ return f"TensorShape({list(self._dims)})"
+ else:
+ return "TensorShape(None)"
+
+ def __str__(self):
+ if self.rank is None:
+ return ""
+ elif self.rank == 1:
+ return "(%s,)" % self._dims[0]
+ else:
+ return "(%s)" % ", ".join(str(d) for d in self._dims)
+
+ # Properties #
+ # ---------- #
+
+ @property
+ def dims(self):
+ return self._dims
+
+ @property
+ def ivy_shape(self):
+ return ivy.Shape(self._dims)
+
+ @property
+ def ndims(self):
+ return self.__len__()
+
+ @property
+ def rank(self):
+ return self.__len__()
+
+ # Instance Methods #
+ # ---------------- #
+
+ def __add__(self, other):
+ return self.concatenate(other)
+
+ def __bool__(self):
+ return self._dims is not None
+
+ def __concat__(self, other):
+ return self.concatenate(other)
+
+ def __eq__(self, other):
+ return self._dims == other.dims
+
+ def __getitem__(self, key):
+ if isinstance(key, slice):
+ return TensorShape(self._dims[key])
+ else:
+ return self._dims[key]
+
+ def __iter__(self):
+ return iter(d for d in self._dims)
+
+ def __len__(self):
+ return len(self._dims)
+
+ def __nonzero__(self):
+ return self.__bool__()
+
+ def __radd__(self, other):
+ return other.concatenate(self)
+
+ def as_list(self):
+ return list(self._dims)
+
+ def concatenate(self, other):
+ other = as_shape(other)
+ if self.dims is None or other.dims is None:
+ return unknown_shape()
+ else:
+ return TensorShape(self.dims + other.dims)
+
+ def num_elements(self):
+ return ivy.to_scalar(ivy.prod(self._dims))
+
+
# Dummy Tensor class to help with compilation, don't add methods here
class Tensor(EagerTensor):
pass
+
+
+# Helpers
+
+
+def as_shape(shape):
+ """Converts the given object to a TensorShape."""
+ if isinstance(shape, TensorShape):
+ return shape
+ else:
+ return TensorShape(shape)
+
+
+def unknown_shape(rank=None, **kwargs):
+ if rank is None and "ndims" in kwargs:
+ rank = kwargs.pop("ndims")
+ if kwargs:
+ raise TypeError("Unknown argument: %s" % kwargs)
+ if rank is None:
+ return TensorShape(None)
+ else:
+ return TensorShape([None] * rank)
diff --git a/ivy/functional/frontends/tensorflow/tensorarray.py b/ivy/functional/frontends/tensorflow/tensorarray.py
new file mode 100644
index 0000000000000..bc9290406aad5
--- /dev/null
+++ b/ivy/functional/frontends/tensorflow/tensorarray.py
@@ -0,0 +1,219 @@
+# global
+import weakref
+
+# local
+import ivy
+import ivy.functional.frontends.tensorflow as tf_frontend
+from ivy.functional.frontends.tensorflow import EagerTensor
+
+
+class TensorArray:
+ def __init__(
+ self,
+ dtype,
+ size=None,
+ dynamic_size=None,
+ clear_after_read=None,
+ tensor_array_name=None,
+ handle=None,
+ flow=None,
+ infer_shape=True,
+ element_shape=None,
+ colocate_with_first_write_call=True,
+ name=None,
+ ):
+ del (flow, tensor_array_name, name)
+ self._handle = None
+ self._flow = tf_frontend.constant(0, dtype=tf_frontend.int32)
+ self._infer_shape = infer_shape
+ self._element_shape = (
+ ivy.Shape(element_shape) if element_shape is not None else element_shape
+ )
+ self._colocate_with_first_write_call = colocate_with_first_write_call
+ self._dtype = tf_frontend.as_dtype(dtype)
+ self._dynamic_size = dynamic_size or False
+ self._clear_after_read = True if clear_after_read is None else clear_after_read
+ self._previously_read_indices = []
+
+ if isinstance(size, EagerTensor):
+ size = size.ivy_array
+ self._tensor_array = [None for _ in range(size)]
+ self._parent = weakref.ref(self)
+
+ @property
+ def flow(self):
+ return self._flow
+
+ @property
+ def dtype(self):
+ return self._dtype
+
+ @property
+ def handle(self):
+ return self._handle
+
+ @property
+ def element_shape(self):
+ return self._element_shape
+
+ def identity(self):
+ return self._parent()
+
+ def grad(self, source, flow=None, name=None):
+ raise NotImplementedError(
+ "TensorArray.grad is not supported when executing eagerly; eager's "
+ "gradient implementation does not use/need this function to compute "
+ "gradients of operations that use TensorArrays."
+ )
+
+ @property
+ def dynamic_size(self):
+ return self._dynamic_size
+
+ @property
+ def infer_shape(self):
+ return self._infer_shape
+
+ def read(self, index, name=None):
+ if isinstance(index, EagerTensor):
+ index = ivy.to_scalar(index.ivy_array)
+
+ if index < 0:
+ raise IndexError(f"Reading from negative indices {index} is not allowed.")
+
+ if index >= len(self._tensor_array):
+ raise IndexError(
+ f"Tried to read from index {index} but array size is:"
+ f" {len(self._tensor_array)} "
+ )
+
+ tensor = self._tensor_array[index]
+ if tensor is None:
+ if index in self._previously_read_indices:
+ raise ValueError(
+ f"Could not read index {index} twice because it was cleared after a"
+ " previous read (perhaps try setting clear_after_read = false?)"
+ )
+ else:
+ tensor = self._tensor_array[index] = tf_frontend.zeros(
+ shape=self._element_shape, dtype=self._dtype
+ )
+
+ if self._clear_after_read:
+ self._tensor_array[index] = None
+ self._previously_read_indices.append(index)
+ return tensor
+
+ def _write(self, index, value, name=None):
+ if isinstance(index, EagerTensor):
+ index = ivy.to_scalar(index.ivy_array)
+
+ if index < 0:
+ raise IndexError(f"Reading from negative indices {index} is not allowed.")
+
+ size = len(self._tensor_array)
+ if index >= size:
+ if not self._dynamic_size:
+ raise IndexError(
+ "Tried to write to index {index} but array is not resizeable and"
+ " size is: {size}"
+ )
+ self._tensor_array.extend(None for _ in range(index - size + 1))
+
+ if not isinstance(value, EagerTensor):
+ value = tf_frontend.cast(value, self.dtype)
+
+ if self._dtype != value.dtype:
+ raise ValueError(
+ f"TensorArray dtype is {self._dtype} but Op is trying to write dtype"
+ f" {value.dtype} "
+ )
+
+ if self._infer_shape:
+ self._element_shape = self._merge_shape(value)
+
+ self._tensor_array[index] = value
+
+ def _merge_shape(self, value):
+ if self._element_shape is None:
+ return value.shape
+ if len(self._element_shape) != len(value.shape):
+ raise ValueError("Shapes not compatible")
+ shape = []
+ for a, b in zip(self._element_shape, value.shape):
+ if a == b or a is None:
+ shape.append(b)
+ else:
+ raise ValueError("Shapes not compatible")
+ return tuple(shape)
+
+ def write(self, index, value, name=None):
+ self._write(index, value)
+ return self._parent()
+
+ def stack(self, name=None):
+ if self._tensor_array:
+ for ix in range(len(self._tensor_array)):
+ if self._tensor_array[ix] is None:
+ self._tensor_array[ix] = tf_frontend.zeros(
+ shape=self._element_shape, dtype=self._dtype
+ )
+ if not self._tensor_array and self._element_shape.is_fully_defined():
+ return tf_frontend.constant(
+ [0] + list(self.element_shape), dtype=self._dtype
+ )
+ else:
+ return tf_frontend.stack(self._tensor_array)
+
+ def _maybe_zero(self, ix):
+ val = self._tensor_array[ix]
+ if val is None:
+ val = self._tensor_array[ix] = tf_frontend.zeros(
+ shape=self._element_shape, dtype=self._dtype
+ )
+ return val
+
+ def gather(self, indices, name=None):
+ if isinstance(indices, EagerTensor):
+ indices = indices.ivy_array
+ return tf_frontend.stack([self._maybe_zero(i) for i in indices])
+
+ def concat(self, name=None):
+ return tf_frontend.concat(
+ [self._maybe_zero(ix) for ix in range(len(self._tensor_array))],
+ 0,
+ name=name,
+ )
+
+ def unstack(self, value, name=None):
+ tensors = tf_frontend.unstack(value, name=name)
+ if len(tensors) > len(self._tensor_array) and not self._dynamic_size:
+ raise ValueError(
+ f"Cannot unstack {len(tensors)} tensors into a TensorArray of static"
+ f" size {len(self._tensor_array)} "
+ )
+ self._tensor_array = tensors
+ return self._parent()
+
+ def scatter(self, indices, value, name=None):
+ if isinstance(indices, EagerTensor):
+ indices = indices.ivy_array
+ for index, val in zip(indices, tf_frontend.unstack(value)):
+ self._write(index, val)
+ return self._parent()
+
+ def size(self, name=None):
+ return tf_frontend.constant(len(self._tensor_array))
+
+ def close(self, name=None):
+ del self._tensor_array[:]
+
+ def split(self, value, lengths, name=None):
+ value = tf_frontend.cast(value, self.dtype)
+ lengths = (
+ tf_frontend.constant(lengths)
+ if not isinstance(lengths, EagerTensor)
+ else lengths
+ )
+ self._tensor_array = tf_frontend.split(value, lengths, name=name)
+ return self._parent()
diff --git a/ivy/functional/frontends/tensorflow/variable.py b/ivy/functional/frontends/tensorflow/variable.py
index cd572c254ed75..7c75a71815528 100644
--- a/ivy/functional/frontends/tensorflow/variable.py
+++ b/ivy/functional/frontends/tensorflow/variable.py
@@ -291,11 +291,11 @@ def dtype(self):
return self.values.dtype
def __repr__(self):
- return "IndexedSlices(\nindices={},\nvalues={}{}\n)".format(
+ return "IndexedSlices(\nindices=%s,\nvalues=%s%s\n)" % (
self._indices,
self._values,
(
- f", dense_shape={self._dense_shape}"
+ ", dense_shape=%s" % (self._dense_shape,)
if self._dense_shape is not None
else ""
),
diff --git a/ivy/functional/frontends/torch/__init__.py b/ivy/functional/frontends/torch/__init__.py
index e218e422ca368..e871198f0a823 100644
--- a/ivy/functional/frontends/torch/__init__.py
+++ b/ivy/functional/frontends/torch/__init__.py
@@ -207,7 +207,9 @@ def promote_types_torch(
The type that both input types promote to
"""
try:
- ret = torch_promotion_table[(ivy.as_ivy_dtype(type1), ivy.as_ivy_dtype(type2))]
+ ret = torch_frontend.torch_promotion_table[
+ (ivy.as_ivy_dtype(type1), ivy.as_ivy_dtype(type2))
+ ]
except KeyError:
raise ivy.utils.exceptions.IvyException("these dtypes are not type promotable")
return ret
@@ -229,28 +231,35 @@ def promote_types_of_torch_inputs(
used as inputs only for those functions that expect an array-like or
tensor-like objects, otherwise it might give unexpected results.
"""
- # Ignore type of 0-dim arrays to mimic torch
- x1 = ivy.asarray(x1)
- x2 = ivy.asarray(x2)
+ if ivy.isscalar(x1) and ivy.is_int_dtype(x1):
+ x1 = ivy.asarray(x1, dtype="int64")
+ elif ivy.isscalar(x1):
+ x1 = ivy.asarray(x1)
+ if ivy.isscalar(x2) and ivy.is_int_dtype(x2):
+ x2 = ivy.asarray(x2, dtype="int64")
+ elif ivy.isscalar(x2):
+ x2 = ivy.asarray(x2)
type1 = ivy.default_dtype(item=x1).strip("u123456789")
type2 = ivy.default_dtype(item=x2).strip("u123456789")
- if not x1.shape == () and x2.shape == () and type1 == type2:
+ if x1.shape != () and x2.shape == () and type1 == type2:
x2 = ivy.asarray(
x2, dtype=x1.dtype, device=ivy.default_device(item=x1, as_native=False)
)
- elif x1.shape == () and not x2.shape == () and type1 == type2:
+ elif x1.shape == () and x2.shape != () and type1 == type2:
x1 = ivy.asarray(
x1, dtype=x2.dtype, device=ivy.default_device(item=x2, as_native=False)
)
elif x1.dtype != x2.dtype:
promoted = promote_types_torch(x1.dtype, x2.dtype)
- x1 = ivy.asarray(x1, dtype=promoted)
- x2 = ivy.asarray(x2, dtype=promoted)
+ if x1.dtype != promoted:
+ x1 = x1.astype(promoted)
+ if x2.dtype != promoted:
+ x2 = x2.astype(promoted)
return x1, x2
from . import nn
-from .nn.functional import softmax, relu
+from .nn.functional import softmax, relu, lstm
from . import tensor
from .tensor import *
from . import blas_and_lapack_ops
diff --git a/ivy/functional/frontends/torch/blas_and_lapack_ops.py b/ivy/functional/frontends/torch/blas_and_lapack_ops.py
index 172b3e41272a7..d25125a87de83 100644
--- a/ivy/functional/frontends/torch/blas_and_lapack_ops.py
+++ b/ivy/functional/frontends/torch/blas_and_lapack_ops.py
@@ -201,7 +201,7 @@ def svd(input, some=True, compute_uv=True, *, out=None):
return ret
-@with_unsupported_dtypes({"2.0.1 and below": ("float16", "bfloat16")}, "torch")
+@with_unsupported_dtypes({"2.1.0 and below": ("float16", "bfloat16")}, "torch")
@to_ivy_arrays_and_back
def trapezoid(y, x=None, *, dx=None, dim=-1):
if x is not None:
diff --git a/ivy/functional/frontends/torch/comparison_ops.py b/ivy/functional/frontends/torch/comparison_ops.py
index 0481f00ddca57..1f9241ab37948 100644
--- a/ivy/functional/frontends/torch/comparison_ops.py
+++ b/ivy/functional/frontends/torch/comparison_ops.py
@@ -98,12 +98,14 @@ def fmin(input, other, *, out=None):
)
+@with_unsupported_dtypes({"2.1.0 and below": ("complex64", "complex128")}, "torch")
@to_ivy_arrays_and_back
def greater(input, other, *, out=None):
input, other = torch_frontend.promote_types_of_torch_inputs(input, other)
return ivy.greater(input, other, out=out)
+@with_unsupported_dtypes({"2.1.0 and below": ("complex64", "complex128")}, "torch")
@to_ivy_arrays_and_back
def greater_equal(input, other, *, out=None):
input, other = torch_frontend.promote_types_of_torch_inputs(input, other)
@@ -140,7 +142,7 @@ def isfinite(input):
return ivy.isfinite(input)
-@with_unsupported_dtypes({"2.0.1 and below": ("float16", "bfloat16")}, "torch")
+@with_unsupported_dtypes({"2.1.0 and below": ("float16", "bfloat16")}, "torch")
@to_ivy_arrays_and_back
def isin(elements, test_elements, *, assume_unique=False, invert=False):
input_elements_copy = ivy.reshape(ivy.to_ivy(elements), (-1,))
@@ -208,14 +210,14 @@ def isposinf(input, *, out=None):
return ivy.logical_and(is_inf, pos_sign_bit, out=out)
-@with_unsupported_dtypes({"2.0.1 and below": ("bfloat16",)}, "torch")
+@with_unsupported_dtypes({"2.1.0 and below": ("bfloat16",)}, "torch")
@to_ivy_arrays_and_back
def isreal(input):
return ivy.isreal(input)
@with_unsupported_dtypes(
- {"2.0.1 and below": ("bfloat16", "float16", "bool", "complex")}, "torch"
+ {"2.1.0 and below": ("bfloat16", "float16", "bool", "complex")}, "torch"
)
@to_ivy_arrays_and_back
def kthvalue(input, k, dim=-1, keepdim=False, *, out=None):
@@ -239,12 +241,14 @@ def kthvalue(input, k, dim=-1, keepdim=False, *, out=None):
return ret
+@with_unsupported_dtypes({"2.1.0 and below": ("complex64", "complex128")}, "torch")
@to_ivy_arrays_and_back
def less(input, other, *, out=None):
input, other = torch_frontend.promote_types_of_torch_inputs(input, other)
return ivy.less(input, other, out=out)
+@with_unsupported_dtypes({"2.1.0 and below": ("complex64", "complex128")}, "torch")
@to_ivy_arrays_and_back
def less_equal(input, other, *, out=None):
input, other = torch_frontend.promote_types_of_torch_inputs(input, other)
@@ -268,7 +272,7 @@ def msort(input, *, out=None):
return ivy.sort(input, axis=0, out=out)
-@with_unsupported_dtypes({"2.0.1 and below": ("float16", "bfloat16")}, "torch")
+@with_unsupported_dtypes({"2.1.0 and below": ("float16", "bfloat16")}, "torch")
@to_ivy_arrays_and_back
def not_equal(input, other, *, out=None):
input, other = torch_frontend.promote_types_of_torch_inputs(input, other)
@@ -283,7 +287,7 @@ def sort(input, *, dim=-1, descending=False, stable=False, out=None):
return namedtuple("sort", ["values", "indices"])(values, indices)
-@with_unsupported_dtypes({"2.0.1 and below": ("float16", "complex")}, "torch")
+@with_unsupported_dtypes({"2.1.0 and below": ("float16", "complex")}, "torch")
@to_ivy_arrays_and_back
def topk(input, k, dim=None, largest=True, sorted=True, *, out=None):
if dim is None:
diff --git a/ivy/functional/frontends/torch/creation_ops.py b/ivy/functional/frontends/torch/creation_ops.py
index 43cd8a389402f..30132ec31bad8 100644
--- a/ivy/functional/frontends/torch/creation_ops.py
+++ b/ivy/functional/frontends/torch/creation_ops.py
@@ -12,7 +12,7 @@
@to_ivy_arrays_and_back
-@with_unsupported_dtypes({"2.0.1 and below": ("float16",)}, "torch")
+@with_unsupported_dtypes({"2.1.0 and below": ("float16",)}, "torch")
def arange(
start=0,
end=None,
@@ -74,7 +74,7 @@ def asarray(
return ivy.asarray(obj, copy=copy, dtype=dtype, device=device)
-@with_supported_dtypes({"2.0.1 and below": ("float32", "float64")}, "torch")
+@with_supported_dtypes({"2.1.0 and below": ("float32", "float64")}, "torch")
@to_ivy_arrays_and_back
def complex(
real,
@@ -208,7 +208,7 @@ def heaviside(input, values, *, out=None):
@to_ivy_arrays_and_back
-@with_unsupported_dtypes({"2.0.1 and below": ("float16",)}, "torch")
+@with_unsupported_dtypes({"2.1.0 and below": ("float16",)}, "torch")
def linspace(
start,
end,
@@ -225,7 +225,7 @@ def linspace(
@to_ivy_arrays_and_back
-@with_unsupported_dtypes({"2.0.1 and below": ("float16",)}, "torch")
+@with_unsupported_dtypes({"2.1.0 and below": ("float16",)}, "torch")
def logspace(
start,
end,
@@ -273,7 +273,7 @@ def ones_like_v_0p4p0_and_above(
return ret
-@with_supported_dtypes({"2.0.1 and below": ("float32", "float64")}, "torch")
+@with_supported_dtypes({"2.1.0 and below": ("float32", "float64")}, "torch")
@to_ivy_arrays_and_back
def polar(
abs,
@@ -285,7 +285,7 @@ def polar(
@to_ivy_arrays_and_back
-@with_unsupported_dtypes({"2.0.1 and below": ("float16",)}, "torch")
+@with_unsupported_dtypes({"2.1.0 and below": ("float16",)}, "torch")
def range(
*args,
dtype=None,
diff --git a/ivy/functional/frontends/torch/func_wrapper.py b/ivy/functional/frontends/torch/func_wrapper.py
index 6730a80af8a0d..8f97234a60295 100644
--- a/ivy/functional/frontends/torch/func_wrapper.py
+++ b/ivy/functional/frontends/torch/func_wrapper.py
@@ -126,7 +126,6 @@ def _to_ivy_array(x):
# else if x is a frontend torch Tensor (or any frontend "Tensor" actually) return the wrapped ivy array # noqa: E501
elif hasattr(x, "ivy_array"):
return x.ivy_array
-
# else just return x
return x
@@ -187,7 +186,7 @@ def outputs_to_frontend_arrays_torch(*args, **kwargs):
# once frontend specific backend setting is added
set_default_dtype = False
if not ("dtype" in kwargs and ivy.exists(kwargs["dtype"])) and all(
- [not (ivy.is_array(i) or hasattr(i, "ivy_array")) for i in args]
+ not (ivy.is_array(i) or hasattr(i, "ivy_array")) for i in args
):
if ivy.current_backend_str() == "jax":
import jax
@@ -210,10 +209,8 @@ def outputs_to_frontend_arrays_torch(*args, **kwargs):
requires_grad=kwargs.get(
"requires_grad",
any(
- [
- isinstance(i, torch_frontend.Tensor) and i.requires_grad
- for i in args
- ]
+ isinstance(i, torch_frontend.Tensor) and i.requires_grad
+ for i in args
),
),
)
@@ -225,32 +222,30 @@ def array_fn(x):
first_array = ivy.func_wrapper._get_first_array(
*args, array_fn=array_fn, **kwargs
)
- # ivy.inplace_update with ensure_in_backend=True fails in jax and tf
- # so update .data directly
- if ivy.is_array(first_array):
- first_array._data = ret.ivy_array.data
+ native_ret_data = ret.ivy_array.data
+ if ivy.is_ivy_array(first_array):
+ first_array.data = native_ret_data
+ elif ivy.is_native_array(first_array):
+ ivy.inplace_update(first_array, native_ret_data)
+ ret = torch_frontend.Tensor(first_array, _init_overload=True)
else:
- first_array.ivy_array._data = ret.ivy_array.data
- ret = first_array
+ first_array.ivy_array.data = native_ret_data
+ ret = first_array
# logic for setting is_leaf
if ret is not None and isinstance(ret, torch_frontend.Tensor):
if fn.__name__ in dir(torch_frontend.creation_ops):
ret.is_leaf = True
elif all(
- [
- not isinstance(i, torch_frontend.Tensor)
- or (not i.requires_grad and not i.grad_fn)
- for i in args
- ]
+ not isinstance(i, torch_frontend.Tensor)
+ or (not i.requires_grad and not i.grad_fn)
+ for i in args
):
ret.is_leaf = True
else:
ret.is_leaf = False
# set grad_fn
- if any(
- [isinstance(i, torch_frontend.Tensor) and i.requires_grad for i in args]
- ):
+ if any(isinstance(i, torch_frontend.Tensor) and i.requires_grad for i in args):
# ToDo: Implement for unbind
grad_fn = GradFn(fn, args)
grad_fn.__self__ = ret
diff --git a/ivy/functional/frontends/torch/indexing_slicing_joining_mutating_ops.py b/ivy/functional/frontends/torch/indexing_slicing_joining_mutating_ops.py
index 37935c7860eb6..1682e5e4f1961 100644
--- a/ivy/functional/frontends/torch/indexing_slicing_joining_mutating_ops.py
+++ b/ivy/functional/frontends/torch/indexing_slicing_joining_mutating_ops.py
@@ -6,6 +6,7 @@
numpy_to_torch_style_args,
to_ivy_shape,
)
+import ivy.functional.frontends.torch as torch_frontend
@to_ivy_arrays_and_back
@@ -70,6 +71,35 @@ def conj(input):
return ivy.conj(input)
+# diagonal_scatter
+@with_unsupported_dtypes(
+ {
+ "2.1.0 and below": (
+ "bfloat16",
+ "float16",
+ )
+ },
+ "torch",
+)
+@to_ivy_arrays_and_back
+def diagonal_scatter(input, src, offset=0, dim1=0, dim2=1):
+ input = ivy.copy_array(input)
+ input_shape = input.shape
+ indices = ivy.arange(0, input.size)
+ diagonal_indices = ivy.diagonal(
+ indices.reshape(input.shape), offset=offset, axis1=dim1, axis2=dim2
+ )
+ if not (src.shape == diagonal_indices.shape):
+ raise ivy.utils.exceptions.IvyException(
+ "src must have shape equal to specified diagonal of input. src size ="
+ f" {src.shape}, diagonal size = {diagonal_indices.shape}"
+ )
+ input = input.reshape((-1,))
+ input[diagonal_indices.reshape((-1,))] = src.reshape((-1,))
+ input = input.reshape(input_shape)
+ return input
+
+
@to_ivy_arrays_and_back
def dsplit(input, indices_or_sections, /):
if isinstance(indices_or_sections, (list, tuple, ivy.Array)):
@@ -144,7 +174,7 @@ def index_add(input, dim, index, source, *, alpha=1, out=None):
while len(_to_adds) < _curr_idx:
_to_adds.append(ivy.zeros_like(source[0]))
_to_add_cum = ivy.get_item(source, index[0][1])
- while (1 < len(index)) and (index[0][0] == index[1][0]):
+ while (len(index) > 1) and (index[0][0] == index[1][0]):
_to_add_cum = _to_add_cum + ivy.get_item(source, index.pop(1)[1])
index.pop(0)
_to_adds.append(_to_add_cum)
@@ -170,7 +200,7 @@ def index_copy(input, dim, index, source, *, out=None):
_curr_idx = index[0][0]
for i in range(len(res), _curr_idx):
res.append(ivy.get_item(input, i))
- while (1 < len(index)) and (index[0][0] == index[1][0]):
+ while (len(index) > 1) and (index[0][0] == index[1][0]):
index.pop(0)
res.append(ivy.get_item(source, index[0][1]))
index.pop(0)
@@ -185,7 +215,7 @@ def index_copy(input, dim, index, source, *, out=None):
@with_unsupported_dtypes(
{
- "2.0.1 and below": (
+ "2.1.0 and below": (
"uint16",
"uint32",
"uint64",
@@ -479,4 +509,5 @@ def vstack(tensors, *, out=None):
def where(condition, input=None, other=None):
if not ivy.exists(input) and not ivy.exists(other):
return nonzero(condition, as_tuple=True)
+ input, other = torch_frontend.promote_types_of_torch_inputs(input, other)
return ivy.where(condition, input, other)
diff --git a/ivy/functional/frontends/torch/linalg.py b/ivy/functional/frontends/torch/linalg.py
index 3902226ada2e3..57cbfaae05107 100644
--- a/ivy/functional/frontends/torch/linalg.py
+++ b/ivy/functional/frontends/torch/linalg.py
@@ -9,7 +9,7 @@
@to_ivy_arrays_and_back
@with_supported_dtypes(
- {"2.0.1 and below": ("float32", "float64", "complex32", "complex64")}, "torch"
+ {"2.1.0 and below": ("float32", "float64", "complex32", "complex64")}, "torch"
)
def cholesky(input, *, upper=False, out=None):
return ivy.cholesky(input, upper=upper, out=out)
@@ -31,14 +31,14 @@ def cholesky_ex(input, *, upper=False, check_errors=False, out=None):
@to_ivy_arrays_and_back
-@with_supported_dtypes({"2.0.1 and below": ("float32", "float64", "complex")}, "torch")
+@with_supported_dtypes({"2.1.0 and below": ("float32", "float64", "complex")}, "torch")
def cond(input, p=None, *, out=None):
return ivy.cond(input, p=p, out=out)
@to_ivy_arrays_and_back
@with_supported_dtypes(
- {"2.0.1 and below": ("float32", "float64", "complex32", "complex64")}, "torch"
+ {"2.1.0 and below": ("float32", "float64", "complex32", "complex64")}, "torch"
)
def cross(input, other, *, dim=None, out=None):
return torch_frontend.miscellaneous_ops.cross(input, other, dim=dim, out=out)
@@ -46,7 +46,7 @@ def cross(input, other, *, dim=None, out=None):
@to_ivy_arrays_and_back
@with_supported_dtypes(
- {"2.0.1 and below": ("float32", "float64", "complex32", "complex64")}, "torch"
+ {"2.1.0 and below": ("float32", "float64", "complex32", "complex64")}, "torch"
)
def det(A, *, out=None):
return ivy.det(A, out=out)
@@ -63,13 +63,13 @@ def divide(input, other, *, rounding_mode=None, out=None):
@to_ivy_arrays_and_back
-@with_unsupported_dtypes({"2.0.1 and below": ("bfloat16", "float16")}, "torch")
+@with_unsupported_dtypes({"2.1.0 and below": ("bfloat16", "float16")}, "torch")
def eig(input, *, out=None):
return ivy.eig(input, out=out)
@with_supported_dtypes(
- {"2.0.1 and below": ("float32", "float64", "complex32", "complex64", "complex128")},
+ {"2.1.0 and below": ("float32", "float64", "complex32", "complex64", "complex128")},
"torch",
)
def eigh(A, UPLO="L", *, out=None):
@@ -78,7 +78,7 @@ def eigh(A, UPLO="L", *, out=None):
@to_ivy_arrays_and_back
@with_supported_dtypes(
- {"2.0.1 and below": ("float32", "float64", "complex32", "complex64")}, "torch"
+ {"2.1.0 and below": ("float32", "float64", "complex32", "complex64")}, "torch"
)
def eigvals(input, *, out=None):
ret = ivy.eigvals(input)
@@ -89,7 +89,7 @@ def eigvals(input, *, out=None):
@to_ivy_arrays_and_back
@with_supported_dtypes(
- {"2.0.1 and below": ("float32", "float64", "complex32", "complex64")}, "torch"
+ {"2.1.0 and below": ("float32", "float64", "complex32", "complex64")}, "torch"
)
def eigvalsh(input, UPLO="L", *, out=None):
ret = ivy.eigvalsh(input, UPLO=UPLO, out=out)
@@ -102,7 +102,7 @@ def eigvalsh(input, UPLO="L", *, out=None):
@to_ivy_arrays_and_back
@with_supported_dtypes(
- {"2.0.1 and below": ("float32", "float64", "complex32", "complex64")}, "torch"
+ {"2.1.0 and below": ("float32", "float64", "complex32", "complex64")}, "torch"
)
def inv(A, *, out=None):
return ivy.inv(A, out=out)
@@ -110,7 +110,7 @@ def inv(A, *, out=None):
@to_ivy_arrays_and_back
@with_supported_dtypes(
- {"2.0.1 and below": ("float32", "float64", "complex32", "complex64")}, "torch"
+ {"2.1.0 and below": ("float32", "float64", "complex32", "complex64")}, "torch"
)
def inv_ex(A, *, check_errors=False, out=None):
if ivy.any(ivy.det(A) == 0):
@@ -129,7 +129,7 @@ def inv_ex(A, *, check_errors=False, out=None):
@to_ivy_arrays_and_back
@with_supported_dtypes(
- {"2.0.1 and below": ("float32", "float64", "complex32", "complex64")}, "torch"
+ {"2.1.0 and below": ("float32", "float64", "complex32", "complex64")}, "torch"
)
def lu_factor(A, *, pivot=True, out=None):
return ivy.lu_factor(A, pivot=pivot, out=out)
@@ -137,21 +137,21 @@ def lu_factor(A, *, pivot=True, out=None):
@to_ivy_arrays_and_back
@with_supported_dtypes(
- {"2.0.1 and below": ("float32", "float64", "complex32", "complex64")}, "torch"
+ {"2.1.0 and below": ("float32", "float64", "complex32", "complex64")}, "torch"
)
def matmul(input, other, *, out=None):
return ivy.matmul(input, other, out=out)
@to_ivy_arrays_and_back
-@with_supported_dtypes({"2.0.1 and below": ("float32", "float64", "complex")}, "torch")
+@with_supported_dtypes({"2.1.0 and below": ("float32", "float64", "complex")}, "torch")
def matrix_exp(A):
return ivy.matrix_exp(A)
@to_ivy_arrays_and_back
@with_supported_dtypes(
- {"2.0.1 and below": ("float32", "float64", "complex32", "complex64")}, "torch"
+ {"2.1.0 and below": ("float32", "float64", "complex32", "complex64")}, "torch"
)
def matrix_norm(input, ord="fro", dim=(-2, -1), keepdim=False, *, dtype=None, out=None):
if "complex" in ivy.as_ivy_dtype(input.dtype):
@@ -163,7 +163,7 @@ def matrix_norm(input, ord="fro", dim=(-2, -1), keepdim=False, *, dtype=None, ou
@to_ivy_arrays_and_back
@with_supported_dtypes(
- {"2.0.1 and below": ("float32", "float64", "complex32", "complex64")}, "torch"
+ {"2.1.0 and below": ("float32", "float64", "complex32", "complex64")}, "torch"
)
def matrix_power(A, n, *, out=None):
return ivy.matrix_power(A, n, out=out)
@@ -171,7 +171,7 @@ def matrix_power(A, n, *, out=None):
@to_ivy_arrays_and_back
@with_supported_dtypes(
- {"2.0.1 and below": ("float32", "float64", "complex32", "complex64")}, "torch"
+ {"2.1.0 and below": ("float32", "float64", "complex32", "complex64")}, "torch"
)
def matrix_rank(A, *, atol=None, rtol=None, hermitian=False, out=None):
return ivy.matrix_rank(A, atol=atol, rtol=rtol, hermitian=hermitian, out=out)
@@ -179,7 +179,7 @@ def matrix_rank(A, *, atol=None, rtol=None, hermitian=False, out=None):
@to_ivy_arrays_and_back
@with_supported_dtypes(
- {"2.0.1 and below": ("float32", "float64", "complex32", "complex64")}, "torch"
+ {"2.1.0 and below": ("float32", "float64", "complex32", "complex64")}, "torch"
)
def multi_dot(tensors, *, out=None):
return ivy.multi_dot(tensors, out=out)
@@ -187,7 +187,7 @@ def multi_dot(tensors, *, out=None):
@to_ivy_arrays_and_back
@with_supported_dtypes(
- {"2.0.1 and below": ("float32", "float64", "complex64", "complex128")}, "torch"
+ {"2.1.0 and below": ("float32", "float64", "complex64", "complex128")}, "torch"
)
def norm(input, ord=None, dim=None, keepdim=False, *, dtype=None, out=None):
if dim is None and (ord is not None):
@@ -207,7 +207,7 @@ def norm(input, ord=None, dim=None, keepdim=False, *, dtype=None, out=None):
@to_ivy_arrays_and_back
@with_supported_dtypes(
- {"2.0.1 and below": ("float32", "float64", "complex32", "complex64")}, "torch"
+ {"2.1.0 and below": ("float32", "float64", "complex32", "complex64")}, "torch"
)
def pinv(input, *, atol=None, rtol=None, hermitian=False, out=None):
# TODO: add handling for hermitian
@@ -226,7 +226,7 @@ def pinv(input, *, atol=None, rtol=None, hermitian=False, out=None):
@to_ivy_arrays_and_back
@with_supported_dtypes(
- {"2.0.1 and below": ("float32", "float64", "complex32", "complex64")}, "torch"
+ {"2.1.0 and below": ("float32", "float64", "complex32", "complex64")}, "torch"
)
def qr(A, mode="reduced", *, out=None):
if mode == "reduced":
@@ -244,7 +244,7 @@ def qr(A, mode="reduced", *, out=None):
@to_ivy_arrays_and_back
@with_supported_dtypes(
- {"2.0.1 and below": ("float32", "float64", "complex32", "complex64")}, "torch"
+ {"2.1.0 and below": ("float32", "float64", "complex32", "complex64")}, "torch"
)
def slogdet(A, *, out=None):
sign, logabsdet = ivy.slogdet(A)
@@ -260,7 +260,7 @@ def slogdet(A, *, out=None):
@to_ivy_arrays_and_back
@with_supported_dtypes(
- {"2.0.1 and below": ("float32", "float64", "complex32", "complex64")}, "torch"
+ {"2.1.0 and below": ("float32", "float64", "complex32", "complex64")}, "torch"
)
def solve(A, B, *, left=True, out=None):
if left:
@@ -274,7 +274,7 @@ def solve(A, B, *, left=True, out=None):
@to_ivy_arrays_and_back
@with_supported_dtypes(
- {"2.0.1 and below": ("float32", "float64", "complex32", "complex64")}, "torch"
+ {"2.1.0 and below": ("float32", "float64", "complex32", "complex64")}, "torch"
)
def solve_ex(A, B, *, left=True, check_errors=False, out=None):
try:
@@ -302,7 +302,7 @@ def solve_ex(A, B, *, left=True, check_errors=False, out=None):
@to_ivy_arrays_and_back
@with_supported_dtypes(
- {"2.0.1 and below": ("float32", "float64", "complex32", "complex64")}, "torch"
+ {"2.1.0 and below": ("float32", "float64", "complex32", "complex64")}, "torch"
)
def svd(A, /, *, full_matrices=True, driver=None, out=None):
# TODO: add handling for driver and out
@@ -311,16 +311,18 @@ def svd(A, /, *, full_matrices=True, driver=None, out=None):
@to_ivy_arrays_and_back
@with_supported_dtypes(
- {"2.0.1 and below": ("float32", "float64", "complex32", "complex64")}, "torch"
+ {"2.1.0 and below": ("float32", "float64", "complex32", "complex64")}, "torch"
)
def svdvals(A, *, driver=None, out=None):
- # TODO: add handling for driver
- return ivy.svdvals(A, out=out)
+ if driver in ["gesvd", "gesvdj", "gesvda", None]:
+ return ivy.svdvals(A, driver=driver, out=out)
+ else:
+ raise ValueError("Unsupported SVD driver")
@to_ivy_arrays_and_back
@with_supported_dtypes(
- {"2.0.1 and below": ("float32", "float64", "complex32", "complex64")}, "torch"
+ {"2.1.0 and below": ("float32", "float64", "complex32", "complex64")}, "torch"
)
def tensorinv(input, ind=2, *, out=None):
not_invertible = "Reshaped tensor is not invertible"
@@ -347,14 +349,14 @@ def tensorinv(input, ind=2, *, out=None):
@to_ivy_arrays_and_back
@with_supported_dtypes(
- {"2.0.1 and below": ("float32", "float64", "complex32", "complex64")}, "torch"
+ {"2.1.0 and below": ("float32", "float64", "complex32", "complex64")}, "torch"
)
def tensorsolve(A, B, dims=None, *, out=None):
return ivy.tensorsolve(A, B, axes=dims, out=out)
@to_ivy_arrays_and_back
-@with_supported_dtypes({"2.0.1 and below": ("integer", "float", "complex")}, "torch")
+@with_supported_dtypes({"2.1.0 and below": ("integer", "float", "complex")}, "torch")
def vander(x, N=None):
if len(x.shape) < 1:
raise RuntimeError("Input dim must be greater than or equal to 1.")
@@ -387,7 +389,7 @@ def vander(x, N=None):
@to_ivy_arrays_and_back
@with_supported_dtypes(
- {"2.0.1 and below": ("float32", "float64", "complex32", "complex64")}, "torch"
+ {"2.1.0 and below": ("float32", "float64", "complex32", "complex64")}, "torch"
)
def vecdot(x, y, *, dim=-1, out=None):
if "complex" in ivy.as_ivy_dtype(x.dtype):
@@ -397,7 +399,7 @@ def vecdot(x, y, *, dim=-1, out=None):
@to_ivy_arrays_and_back
@with_supported_dtypes(
- {"2.0.1 and below": ("float32", "float64", "complex32", "complex64")}, "torch"
+ {"2.1.0 and below": ("float32", "float64", "complex32", "complex64")}, "torch"
)
def vector_norm(input, ord=2, dim=None, keepdim=False, *, dtype=None, out=None):
return ivy.vector_norm(
diff --git a/ivy/functional/frontends/torch/miscellaneous_ops.py b/ivy/functional/frontends/torch/miscellaneous_ops.py
index 0f1d694e32e50..7e1f401b5ed59 100644
--- a/ivy/functional/frontends/torch/miscellaneous_ops.py
+++ b/ivy/functional/frontends/torch/miscellaneous_ops.py
@@ -74,7 +74,7 @@ def broadcast_shapes(*shapes):
return ivy.broadcast_shapes(*shapes)
-@with_unsupported_dtypes({"2.0.1 and below": ("bfloat16",)}, "torch")
+@with_unsupported_dtypes({"2.1.0 and below": ("bfloat16",)}, "torch")
@to_ivy_arrays_and_back
def broadcast_to(tensor, shape):
return ivy.broadcast_to(tensor, shape)
@@ -93,7 +93,7 @@ def cartesian_prod(*tensors):
@to_ivy_arrays_and_back
-def clone(input):
+def clone(input, *, memory_format=None):
return ivy.copy_array(input)
@@ -102,7 +102,7 @@ def corrcoef(input):
if len(ivy.shape(input)) > 2:
raise ivy.exceptions.IvyError(
"corrcoef(): expected input to have two or fewer dimensions but got an"
- f" input with {ivy.shape(input)} dimansions"
+ f" input with {ivy.shape(input)} dimensions"
)
return ivy.corrcoef(input, y=None, rowvar=True)
@@ -113,7 +113,7 @@ def cov(input, /, *, correction=1, fweights=None, aweights=None):
@to_ivy_arrays_and_back
-@with_unsupported_dtypes({"2.0.1 and below": ("float16",)}, "torch")
+@with_unsupported_dtypes({"2.1.0 and below": ("float16",)}, "torch")
def cross(input, other, dim=None, *, out=None):
if dim is None:
dim = -1
@@ -124,7 +124,7 @@ def cross(input, other, dim=None, *, out=None):
@to_ivy_arrays_and_back
@with_unsupported_dtypes(
{
- "2.0.1 and below": (
+ "2.1.0 and below": (
"uint16",
"uint32",
"uint64",
@@ -152,7 +152,7 @@ def cumprod(input, dim, *, dtype=None, out=None):
@to_ivy_arrays_and_back
@with_unsupported_dtypes(
- {"2.0.1 and below": ("uint8", "bfloat16", "float16"), "1.12.1": ()},
+ {"2.1.0 and below": ("uint8", "bfloat16", "float16"), "1.12.1": ()},
"torch",
)
def cumsum(input, dim, *, dtype=None, out=None):
@@ -167,7 +167,7 @@ def diag(input, diagonal=0, *, out=None):
@with_supported_dtypes(
- {"2.0.1 and below": ("float32", "float64", "int32", "int64")}, "torch"
+ {"2.1.0 and below": ("float32", "float64", "int32", "int64")}, "torch"
)
@to_ivy_arrays_and_back
def diagflat(x, offset=0, name=None):
@@ -175,7 +175,7 @@ def diagflat(x, offset=0, name=None):
return arr
-@with_unsupported_dtypes({"2.0.1 and below": ("float16", "bfloat16")}, "torch")
+@with_unsupported_dtypes({"2.1.0 and below": ("float16", "bfloat16")}, "torch")
@to_ivy_arrays_and_back
def diagonal(input, offset=0, dim1=0, dim2=1):
return ivy.diagonal(input, offset=offset, axis1=dim1, axis2=dim2)
@@ -183,14 +183,14 @@ def diagonal(input, offset=0, dim1=0, dim2=1):
@to_ivy_arrays_and_back
@with_unsupported_dtypes(
- {"2.0.1 and below": ("int8", "float16", "bfloat16", "bool")}, "torch"
+ {"2.1.0 and below": ("int8", "float16", "bfloat16", "bool")}, "torch"
)
def diff(input, n=1, dim=-1, prepend=None, append=None):
return ivy.diff(input, n=n, axis=dim, prepend=prepend, append=append, out=None)
@to_ivy_arrays_and_back
-@with_unsupported_dtypes({"2.0.1 and below": ("float16",)}, "torch")
+@with_unsupported_dtypes({"2.1.0 and below": ("float16",)}, "torch")
def einsum(equation, *operands):
if len(operands) == 1 and isinstance(operands[0], (list, tuple)):
operands = operands[0]
@@ -242,14 +242,14 @@ def kron(input, other, *, out=None):
@to_ivy_arrays_and_back
-@with_unsupported_dtypes({"2.0.1 and below": ("int8",)}, "torch")
+@with_unsupported_dtypes({"2.1.0 and below": ("int8",)}, "torch")
def lcm(input, other, *, out=None):
return ivy.lcm(input, other, out=out)
@with_unsupported_dtypes(
{
- "2.0.1 and below": (
+ "2.1.0 and below": (
"float16",
"bfloat16",
"integer",
@@ -287,7 +287,7 @@ def ravel(input):
return ivy.reshape(input, (-1,))
-@with_unsupported_dtypes({"2.0.1 and below": ("float16",)}, "torch")
+@with_unsupported_dtypes({"2.1.0 and below": ("float16",)}, "torch")
@to_ivy_arrays_and_back
def renorm(input, p, dim, maxnorm, *, out=None):
# Torch hardcodes this magic number
@@ -328,7 +328,7 @@ def renorm(input, p, dim, maxnorm, *, out=None):
@with_supported_dtypes(
{
- "2.0.1 and below": (
+ "2.1.0 and below": (
"int32",
"int64",
)
@@ -458,7 +458,7 @@ def searchsorted(
return ret
-@with_unsupported_dtypes({"2.0.1 and below": ("float16", "bfloat16")}, "torch")
+@with_unsupported_dtypes({"2.1.0 and below": ("float16", "bfloat16")}, "torch")
@to_ivy_arrays_and_back
def tensordot(a, b, dims=2, out=None):
a, b = promote_types_of_torch_inputs(a, b)
@@ -466,7 +466,7 @@ def tensordot(a, b, dims=2, out=None):
@to_ivy_arrays_and_back
-@with_unsupported_dtypes({"2.0.1 and below": ("float16", "bfloat16")}, "torch")
+@with_unsupported_dtypes({"2.1.0 and below": ("float16", "bfloat16")}, "torch")
def trace(input):
if "int" in input.dtype:
input = input.astype("int64")
@@ -480,7 +480,7 @@ def tril(input, diagonal=0, *, out=None):
return ivy.tril(input, k=diagonal, out=out)
-@with_unsupported_dtypes({"2.0.1 and below": ("int8", "uint8", "int16")}, "torch")
+@with_unsupported_dtypes({"2.1.0 and below": ("int8", "uint8", "int16")}, "torch")
@to_ivy_arrays_and_back
def tril_indices(row, col, offset=0, *, dtype=ivy.int64, device="cpu", layout=None):
sample_matrix = ivy.tril(ivy.ones((row, col), device=device), k=offset)
@@ -511,7 +511,7 @@ def vander(x, N=None, increasing=False):
@to_ivy_arrays_and_back
-@with_supported_dtypes({"2.0.1 and below": ("float32", "float64")}, "torch")
+@with_supported_dtypes({"2.1.0 and below": ("float32", "float64")}, "torch")
def view_as_complex(input):
if ivy.shape(input)[-1] != 2:
raise ivy.exceptions.IvyError("The last dimension must have a size of 2")
@@ -529,7 +529,7 @@ def view_as_complex(input):
@with_supported_dtypes(
- {"2.0.1 and below": ("complex64", "complex128")},
+ {"2.1.0 and below": ("complex64", "complex128")},
"torch",
)
@to_ivy_arrays_and_back
diff --git a/ivy/functional/frontends/torch/nn/functional/__init__.py b/ivy/functional/frontends/torch/nn/functional/__init__.py
index 6ceb055aeb03b..7adda04645ffe 100644
--- a/ivy/functional/frontends/torch/nn/functional/__init__.py
+++ b/ivy/functional/frontends/torch/nn/functional/__init__.py
@@ -4,6 +4,8 @@
from .distance_functions import *
from . import dropout_functions
from .dropout_functions import *
+from . import layer_functions
+from .layer_functions import *
from . import linear_functions
from .linear_functions import *
from . import loss_functions
diff --git a/ivy/functional/frontends/torch/nn/functional/convolution_functions.py b/ivy/functional/frontends/torch/nn/functional/convolution_functions.py
index 3ff490ace86be..d118282135f0b 100644
--- a/ivy/functional/frontends/torch/nn/functional/convolution_functions.py
+++ b/ivy/functional/frontends/torch/nn/functional/convolution_functions.py
@@ -142,7 +142,7 @@ def _valid_shapes(input, weight, bias, stride, padding, groups, transpose=False)
# ------------ #
-@with_unsupported_dtypes({"2.0.1 and below": ("float16", "bfloat16")}, "torch")
+@with_unsupported_dtypes({"2.1.0 and below": ("float16", "bfloat16")}, "torch")
@to_ivy_arrays_and_back
def conv1d(input, weight, bias=None, stride=1, padding=0, dilation=1, groups=1):
return _conv(
@@ -156,7 +156,7 @@ def conv1d(input, weight, bias=None, stride=1, padding=0, dilation=1, groups=1):
)
-@with_unsupported_dtypes({"2.0.1 and below": ("float16", "bfloat16")}, "torch")
+@with_unsupported_dtypes({"2.1.0 and below": ("float16", "bfloat16")}, "torch")
@to_ivy_arrays_and_back
def conv2d(input, weight, bias=None, stride=1, padding=0, dilation=1, groups=1):
return _conv(
@@ -170,7 +170,7 @@ def conv2d(input, weight, bias=None, stride=1, padding=0, dilation=1, groups=1):
)
-@with_unsupported_dtypes({"2.0.1 and below": ("float16", "bfloat16")}, "torch")
+@with_unsupported_dtypes({"2.1.0 and below": ("float16", "bfloat16")}, "torch")
@to_ivy_arrays_and_back
def conv3d(input, weight, bias=None, stride=1, padding=0, dilation=1, groups=1):
return _conv(
@@ -184,7 +184,7 @@ def conv3d(input, weight, bias=None, stride=1, padding=0, dilation=1, groups=1):
)
-@with_unsupported_dtypes({"2.0.1 and below": ("float16", "bfloat16")}, "torch")
+@with_unsupported_dtypes({"2.1.0 and below": ("float16", "bfloat16")}, "torch")
@to_ivy_arrays_and_back
def conv_transpose1d(
input,
@@ -208,7 +208,7 @@ def conv_transpose1d(
)
-@with_unsupported_dtypes({"2.0.1 and below": ("float16", "bfloat16")}, "torch")
+@with_unsupported_dtypes({"2.1.0 and below": ("float16", "bfloat16")}, "torch")
@to_ivy_arrays_and_back
def conv_transpose2d(
input,
@@ -232,7 +232,7 @@ def conv_transpose2d(
)
-@with_unsupported_dtypes({"2.0.1 and below": ("float16", "bfloat16")}, "torch")
+@with_unsupported_dtypes({"2.1.0 and below": ("float16", "bfloat16")}, "torch")
@to_ivy_arrays_and_back
def conv_transpose3d(
input,
diff --git a/ivy/functional/frontends/torch/nn/functional/distance_functions.py b/ivy/functional/frontends/torch/nn/functional/distance_functions.py
index 393d91e8d717d..92be14eeeb922 100644
--- a/ivy/functional/frontends/torch/nn/functional/distance_functions.py
+++ b/ivy/functional/frontends/torch/nn/functional/distance_functions.py
@@ -4,7 +4,7 @@
from ivy.func_wrapper import with_unsupported_dtypes
-@with_unsupported_dtypes({"2.0.1 and below": ("float16", "bfloat16")}, "torch")
+@with_unsupported_dtypes({"2.1.0 and below": ("float16", "bfloat16")}, "torch")
@to_ivy_arrays_and_back
def cosine_similarity(x1, x2, *, dim=1, eps=1e-08):
x1, x2 = torch_frontend.promote_types_of_torch_inputs(x1, x2)
@@ -28,7 +28,7 @@ def cosine_similarity(x1, x2, *, dim=1, eps=1e-08):
return cosine
-@with_unsupported_dtypes({"2.0.1 and below": ("float16", "bfloat16")}, "torch")
+@with_unsupported_dtypes({"2.1.0 and below": ("float16", "bfloat16")}, "torch")
@to_ivy_arrays_and_back
def pairwise_distance(x1, x2, *, p=2.0, eps=1e-06, keepdim=False):
x1, x2 = torch_frontend.promote_types_of_torch_inputs(x1, x2)
@@ -42,7 +42,7 @@ def pairwise_distance(x1, x2, *, p=2.0, eps=1e-06, keepdim=False):
return ivy.vector_norm(x1 - x2 + eps, ord=p, axis=output_dim - 1, keepdims=keepdim)
-@with_unsupported_dtypes({"2.0.1 and below": ("float16", "bfloat16")}, "torch")
+@with_unsupported_dtypes({"2.1.0 and below": ("float16", "bfloat16")}, "torch")
@to_ivy_arrays_and_back
def pdist(input, p=2):
x = ivy.array(
diff --git a/ivy/functional/frontends/torch/nn/functional/dropout_functions.py b/ivy/functional/frontends/torch/nn/functional/dropout_functions.py
index 92c99d1b0f3e0..5faa28c1e4a7c 100644
--- a/ivy/functional/frontends/torch/nn/functional/dropout_functions.py
+++ b/ivy/functional/frontends/torch/nn/functional/dropout_functions.py
@@ -7,7 +7,8 @@
# ToDo: this function will be simplified once ivy.alpha_dropout is implemented
@to_ivy_arrays_and_back
-@with_unsupported_dtypes({"2.0.1 and below": ("float16",)}, "torch")
+@with_unsupported_dtypes({"2.1.0 and below": ("float16",)}, "torch")
+@with_unsupported_dtypes({"2.5.2 and below": ("float16", "bfloat16")}, "paddle")
def alpha_dropout(input, p=0.5, training=False, inplace=False):
if p == 0.0 or not training or input.shape == () or input.shape == (0,):
return input
@@ -27,13 +28,13 @@ def alpha_dropout(input, p=0.5, training=False, inplace=False):
@to_ivy_arrays_and_back
-@with_unsupported_dtypes({"2.0.1 and below": ("float16",)}, "torch")
+@with_unsupported_dtypes({"2.1.0 and below": ("float16",)}, "torch")
def dropout(input, p=0.5, training=True, inplace=False):
return ivy.dropout(input, p, training=training)
@to_ivy_arrays_and_back
-@with_unsupported_dtypes({"2.0.1 and below": ("float16",)}, "torch")
+@with_unsupported_dtypes({"2.1.0 and below": ("float16",)}, "torch")
def dropout1d(input, p=0.5, training=True, inplace=False):
if inplace:
return ivy.dropout1d(input, p, training=training, data_format="NCW", out=input)
@@ -41,7 +42,7 @@ def dropout1d(input, p=0.5, training=True, inplace=False):
@to_ivy_arrays_and_back
-@with_unsupported_dtypes({"2.0.1 and below": ("float16",)}, "torch")
+@with_unsupported_dtypes({"2.1.0 and below": ("float16",)}, "torch")
def dropout2d(input, p=0.5, training=True, inplace=False):
if input.ndim < 2:
raise ValueError("Feature dropout requires at least 2 dimensions in the input")
@@ -54,7 +55,7 @@ def dropout2d(input, p=0.5, training=True, inplace=False):
@to_ivy_arrays_and_back
-@with_unsupported_dtypes({"2.0.1 and below": ("float16", "bfloat16")}, "torch")
+@with_unsupported_dtypes({"2.1.0 and below": ("float16", "bfloat16")}, "torch")
def dropout3d(input, p=0.5, training=True, inplace=False):
if inplace:
return ivy.dropout3d(
diff --git a/ivy/functional/frontends/torch/nn/functional/layer_functions.py b/ivy/functional/frontends/torch/nn/functional/layer_functions.py
new file mode 100644
index 0000000000000..12f36e34df414
--- /dev/null
+++ b/ivy/functional/frontends/torch/nn/functional/layer_functions.py
@@ -0,0 +1,381 @@
+import ivy
+from ivy.func_wrapper import with_supported_device_and_dtypes, with_supported_dtypes
+from ivy.functional.frontends.torch.func_wrapper import to_ivy_arrays_and_back
+from ivy.functional.ivy.experimental.manipulation import _slice_along_axis
+from ivy.utils.exceptions import IvyNotImplementedException
+
+
+# --- Helpers --- #
+# --------------- #
+
+
+def _extract_states(states, batch_sizes):
+ h = []
+ for i in range(states.shape[1]):
+ h.append(states[int(batch_sizes[i] - 1), i])
+ h = ivy.expand_dims(ivy.stack(h, axis=0), axis=0)
+ return h
+
+
+def _generic_lstm(
+ input,
+ initial_states,
+ all_weights,
+ has_biases,
+ num_layers,
+ dropout,
+ train,
+ bidirectional,
+ batch_first=False,
+ batch_sizes=None,
+):
+ weights_per_layer = 4 if has_biases else 2
+
+ assert len(all_weights) == num_layers * weights_per_layer * (1 + bidirectional)
+ layer_weights = [
+ all_weights[i : i + weights_per_layer]
+ for i in range(0, len(all_weights), weights_per_layer)
+ ]
+
+ if batch_sizes is not None:
+ input, batch_sizes = _pad_packed_sequence(input, batch_sizes)
+
+ if batch_first:
+ input = ivy.swapaxes(input, 0, 1)
+
+ if dropout and train:
+ raise IvyNotImplementedException()
+
+ unidirectional = not bidirectional
+
+ h0, c0 = initial_states
+ h_outs, c_outs = [], []
+
+ output = input
+ for i in range(num_layers):
+ if unidirectional:
+ if weights_per_layer == 4:
+ weight_ih, weight_hh, (bias_i, bias_h) = _transform_weights(
+ layer_weights, i
+ )
+ else:
+ weight_ih, weight_hh = _transform_weights_no_bias(layer_weights, i)
+ bias_i = bias_h = None
+
+ state_indices = i, i + 1
+ else:
+ if weights_per_layer == 4:
+ weight_ih_f, weight_hh_f, (bias_i_f, bias_h_f) = _transform_weights(
+ layer_weights, 2 * i
+ )
+ weight_ih_b, weight_hh_b, (bias_i_b, bias_h_b) = _transform_weights(
+ layer_weights, 2 * i + 1
+ )
+ else:
+ weight_ih_f, weight_hh_f = _transform_weights_no_bias(
+ layer_weights, 2 * i
+ )
+ weight_ih_b, weight_hh_b = _transform_weights_no_bias(
+ layer_weights, 2 * i + 1
+ )
+ bias_i_f = bias_h_f = bias_i_b = bias_h_b = None
+
+ weight_ih = weight_ih_f, weight_ih_b
+ weight_hh = weight_hh_f, weight_hh_b
+ bias_i = bias_i_f, bias_i_b
+ bias_h = bias_h_f, bias_h_b
+
+ state_indices = 2 * i, 2 * i + 2
+
+ output, (h_out, c_out) = _lstm_layer(
+ output,
+ (
+ _retrieve_state(h0, *state_indices, num_layers),
+ _retrieve_state(c0, *state_indices, num_layers),
+ ),
+ (weight_ih, weight_hh),
+ (bias_i, bias_h),
+ bidirectional,
+ batch_sizes=batch_sizes,
+ )
+ h_outs.append(h_out)
+ c_outs.append(c_out)
+
+ if batch_first:
+ output = ivy.swapaxes(output, 0, 1)
+
+ h_outs = h_out if num_layers == 1 else ivy.concat(h_outs, axis=0)
+ c_outs = c_out if num_layers == 1 else ivy.concat(c_outs, axis=0)
+
+ if batch_sizes is not None:
+ output = _pack_padded_sequence(output, batch_sizes)[0]
+
+ return output, h_outs, c_outs
+
+
+def _lstm_cell(
+ x, init_h, init_c, kernel, recurrent_kernel, bias, recurrent_bias, batch_sizes=None
+):
+ x_shape = x.shape
+ batch_shape = x_shape[1:-1]
+ timesteps = x_shape[0]
+ input_channels = x_shape[-1]
+
+ Wi = kernel
+ Wi_x = ivy.reshape(
+ ivy.matmul(ivy.reshape(x, (-1, input_channels)), Wi)
+ + (bias if bias is not None else 0),
+ [timesteps, *batch_shape, -1],
+ )
+ Wii_x, Wif_x, Wig_x, Wio_x = ivy.split(Wi_x, num_or_size_splits=4, axis=-1)
+ Wh = recurrent_kernel
+ ht = init_h
+ ct = init_c
+ ht_list = []
+ ct_list = []
+
+ for Wii_xt, Wif_xt, Wig_xt, Wio_xt in zip(
+ ivy.unstack(Wii_x, axis=0),
+ ivy.unstack(Wif_x, axis=0),
+ ivy.unstack(Wig_x, axis=0),
+ ivy.unstack(Wio_x, axis=0),
+ ):
+ htm1 = ht
+ ctm1 = ct
+ Wh_htm1 = ivy.matmul(htm1, Wh) + (
+ recurrent_bias if recurrent_bias is not None else 0
+ )
+ Whi_htm1, Whf_htm1, Whg_htm1, Who_htm1 = ivy.split(
+ Wh_htm1, num_or_size_splits=4, axis=-1
+ )
+ it = ivy.sigmoid(Wii_xt + Whi_htm1)
+ ft = ivy.sigmoid(Wif_xt + Whf_htm1)
+ gt = ivy.tanh(Wig_xt + Whg_htm1)
+ ot = ivy.sigmoid(Wio_xt + Who_htm1)
+ ct = ft * ctm1 + it * gt
+ ht = ot * ivy.tanh(ct)
+ ct_list.append(ct)
+ ht_list.append(ht)
+
+ if batch_sizes is None:
+ c = ct_list[-1]
+ h = ht_list[-1]
+ output = ivy.concat(ht_list, axis=0)
+ else:
+ ct_list = ivy.concat(ct_list, axis=0)
+ output = ht_list = ivy.concat(ht_list, axis=0)
+ c = _extract_states(ct_list, batch_sizes)
+ h = _extract_states(ht_list, batch_sizes)
+ return output, (h, c)
+
+
+def _lstm_full(
+ input,
+ hx,
+ params,
+ has_biases,
+ num_layers,
+ dropout,
+ train,
+ bidirectional,
+ batch_first,
+):
+ return _generic_lstm(
+ input,
+ hx,
+ params,
+ has_biases,
+ num_layers,
+ dropout,
+ train,
+ bidirectional,
+ batch_first=batch_first,
+ )
+
+
+def _lstm_layer(x, hidden, weights, biases, bidirectional, batch_sizes=None):
+ if not bidirectional:
+ result, (h, c) = _lstm_cell(
+ x, *hidden, *weights, *biases, batch_sizes=batch_sizes
+ )
+ else:
+ result_fw, (h_fw, c_fw) = _lstm_cell(
+ x,
+ hidden[0][:1],
+ hidden[1][:1],
+ weights[0][0],
+ weights[1][0],
+ biases[0][0],
+ biases[1][0],
+ batch_sizes=batch_sizes,
+ )
+ x_reversed = ivy.flip(x, axis=0)
+ result_bw, (h_bw, c_bw) = _lstm_cell(
+ x_reversed,
+ hidden[0][1:],
+ hidden[1][1:],
+ weights[0][1],
+ weights[1][1],
+ biases[0][1],
+ biases[1][1],
+ batch_sizes=batch_sizes,
+ )
+ result_bw = ivy.flip(result_bw, axis=0)
+ result = ivy.concat([result_fw, result_bw], axis=len(result_fw.shape) - 1)
+ c = ivy.concat([c_fw, c_bw], axis=0)
+ h = ivy.concat([h_fw, h_bw], axis=0)
+ return result, (h, c)
+
+
+def _lstm_packed(
+ data,
+ batch_sizes,
+ hx,
+ params,
+ has_biases,
+ num_layers,
+ dropout,
+ train,
+ bidirectional,
+):
+ return _generic_lstm(
+ data,
+ hx,
+ params,
+ has_biases,
+ num_layers,
+ dropout,
+ train,
+ bidirectional,
+ batch_sizes=batch_sizes,
+ )
+
+
+def _pack_padded_sequence(input, lengths):
+ input = ivy.swapaxes(input, 0, 1)
+ data = []
+ batch_sizes = []
+ for i in range(int(max(lengths))):
+ valid_data_mask = ivy.array(lengths) > i
+ data.append(input[valid_data_mask, i])
+ batch_sizes.append(int(sum(valid_data_mask)))
+ data = ivy.concat(data)
+ batch_sizes = ivy.array(batch_sizes, dtype=ivy.int64)
+ return data, batch_sizes
+
+
+def _pad_packed_sequence(data, batch_sizes):
+ padded_data = ivy.full(
+ (len(batch_sizes), int(max(batch_sizes)), *data.shape[1:]),
+ 0,
+ dtype=data.dtype,
+ device=data.device,
+ )
+ data_offset = 0
+ for i, batch_size in enumerate(batch_sizes):
+ batch_size = int(batch_size)
+ padded_data[i, :batch_size] = data[data_offset : data_offset + batch_size]
+ data_offset += batch_size
+ lengths = ivy.sum(
+ ivy.arange(1, int(max(batch_sizes)) + 1)[:, ivy.newaxis] <= batch_sizes,
+ axis=1,
+ dtype=ivy.int64,
+ )
+ return padded_data, lengths
+
+
+def _retrieve_state(x, start, end, num_layers):
+ return x if num_layers == 1 else _slice_along_axis(x, start=start, stop=end, axis=0)
+
+
+def _transform_weights(layer_weights, layer_index):
+ weights = layer_weights[layer_index]
+ weight_ih, weight_hh, bias_ih, bias_hh = weights
+ return (
+ ivy.swapaxes(weight_ih, 0, 1),
+ ivy.swapaxes(weight_hh, 0, 1),
+ (bias_ih, bias_hh),
+ )
+
+
+def _transform_weights_no_bias(layer_weights, layer_index):
+ weights = layer_weights[layer_index]
+ weight_ih, weight_hh = weights
+ return ivy.swapaxes(weight_ih, 0, 1), ivy.swapaxes(weight_hh, 0, 1)
+
+
+# --- Main --- #
+# ------------ #
+
+
+@with_supported_device_and_dtypes(
+ {"2.1.0 and below": {"cpu": ("float32", "float64")}},
+ "torch",
+)
+@to_ivy_arrays_and_back
+def lstm(*args, **kwargs):
+ if "batch_sizes" in kwargs or (len(args) >= 4 and not isinstance(args[3], bool)):
+ return _lstm_packed(*args, **kwargs)
+ else:
+ return _lstm_full(*args, **kwargs)
+
+
+@to_ivy_arrays_and_back
+@with_supported_dtypes({"2.1.0 and below": ("float32", "float64")}, "torch")
+def multi_head_attention_forward(
+ query,
+ key,
+ value,
+ embed_dim_to_check,
+ num_heads,
+ in_proj_weight,
+ in_proj_bias,
+ bias_k,
+ bias_v,
+ add_zero_attn,
+ dropout_p,
+ out_proj_weight,
+ out_proj_bias,
+ training=True,
+ key_padding_mask=None,
+ need_weights=True,
+ attn_mask=None,
+ use_separate_proj_weight=False,
+ q_proj_weight=None,
+ k_proj_weight=None,
+ v_proj_weight=None,
+ static_k=None,
+ static_v=None,
+ average_attn_weights=True,
+ is_causal=False,
+):
+ embed_dim = query.shape[-1]
+ assert (
+ embed_dim == embed_dim_to_check
+ ), f"was expecting embedding dimension of {embed_dim_to_check}, but got {embed_dim}"
+ return ivy.multi_head_attention(
+ query,
+ key=key,
+ value=value,
+ batch_first=False,
+ num_heads=num_heads,
+ attention_mask=attn_mask,
+ in_proj_weights=in_proj_weight if not use_separate_proj_weight else None,
+ q_proj_weights=q_proj_weight,
+ k_proj_weights=k_proj_weight,
+ v_proj_weights=v_proj_weight,
+ out_proj_weights=out_proj_weight,
+ in_proj_bias=in_proj_bias,
+ out_proj_bias=out_proj_bias,
+ is_causal=is_causal and not (need_weights or key_padding_mask is not None),
+ key_padding_mask=key_padding_mask,
+ bias_k=bias_k,
+ bias_v=bias_v,
+ static_k=static_k,
+ static_v=static_v,
+ add_zero_attn=add_zero_attn,
+ return_attention_weights=need_weights,
+ average_attention_weights=average_attn_weights,
+ dropout=dropout_p,
+ training=training,
+ )
diff --git a/ivy/functional/frontends/torch/nn/functional/linear_functions.py b/ivy/functional/frontends/torch/nn/functional/linear_functions.py
index 57322d401d0f2..040cb9652212d 100644
--- a/ivy/functional/frontends/torch/nn/functional/linear_functions.py
+++ b/ivy/functional/frontends/torch/nn/functional/linear_functions.py
@@ -4,7 +4,7 @@
from ivy.functional.frontends.torch.func_wrapper import to_ivy_arrays_and_back
-@with_unsupported_dtypes({"2.0.1 and below": ("float16",)}, "torch")
+@with_unsupported_dtypes({"2.1.0 and below": ("float16",)}, "torch")
@to_ivy_arrays_and_back
def linear(input, weight, bias=None):
return ivy.linear(input, weight, bias=bias)
diff --git a/ivy/functional/frontends/torch/nn/functional/loss_functions.py b/ivy/functional/frontends/torch/nn/functional/loss_functions.py
index 16ece15e2aaf7..a274d5c5e2434 100644
--- a/ivy/functional/frontends/torch/nn/functional/loss_functions.py
+++ b/ivy/functional/frontends/torch/nn/functional/loss_functions.py
@@ -12,9 +12,7 @@
def _apply_reduction(reduction, size_average, reduce, to_reduce):
if size_average is not None or reduce is not None:
reduction = _get_reduction_string(size_average, reduce)
- return _get_reduction_method(reduction, to_reduce)
- else:
- return _get_reduction_method(reduction, to_reduce)
+ return _get_reduction_method(reduction, to_reduce)
def _get_reduction(reduction, size_average=None, reduce=None):
@@ -74,7 +72,7 @@ def _get_reduction_string(size_average, reduce):
@to_ivy_arrays_and_back
-@with_unsupported_dtypes({"2.0.1 and below": ("float16", "bfloat16")}, "torch")
+@with_unsupported_dtypes({"2.1.0 and below": ("float16", "bfloat16")}, "torch")
def binary_cross_entropy(
input, target, weight=None, size_average=None, reduce=None, reduction="mean"
):
@@ -115,7 +113,7 @@ def binary_cross_entropy_with_logits(
@to_ivy_arrays_and_back
-@with_unsupported_dtypes({"2.0.1 and below": ("float16", "bfloat16")}, "torch")
+@with_unsupported_dtypes({"2.1.0 and below": ("float16", "bfloat16")}, "torch")
def cosine_embedding_loss(
input1, input2, target, margin=0.0, size_average=None, reduce=None, reduction="mean"
):
@@ -148,10 +146,8 @@ def calculate_loss(x1, x2, target):
ivy.utils.assertions.check_true(
target.ndim + 1 == input1.ndim and target.ndim + 1 == input2.ndim,
- "{}D target tensor expects {}D input tensors, but "
- "found inputs with sizes {} and {}.".format(
- target.ndim, target.ndim + 1, list(input1.shape), list(input2.shape)
- ),
+ f"{target.ndim}D target tensor expects {target.ndim + 1}D input tensors, but "
+ f"found inputs with sizes {list(input1.shape)} and {list(input2.shape)}.",
)
ivy.utils.assertions.check_true(
@@ -163,8 +159,8 @@ def calculate_loss(x1, x2, target):
if target.ndim == 1:
ivy.utils.assertions.check_true(
target.shape[0] == input1.shape[0],
- "The size of target tensor ({}) must match the size of input tensor ({}) "
- "at non-singleton dimension 0 ".format(target.shape[0], input1.shape[0]),
+ f"The size of target tensor ({target.shape[0]}) must match the size of"
+ f" input tensor ({input1.shape[0]}) at non-singleton dimension 0 ",
)
if target.ndim == 0:
@@ -204,7 +200,7 @@ def cross_entropy(
reduction="mean",
label_smoothing=0.0,
):
- loss = ivy.cross_entropy(target, input, epsilon=label_smoothing)
+ loss = ivy.cross_entropy(target, input, epsilon=label_smoothing, reduction="none")
if ignore_index != -100:
mask = ivy.not_equal(target, ignore_index)
@@ -218,7 +214,7 @@ def cross_entropy(
@to_ivy_arrays_and_back
-@with_unsupported_dtypes({"2.0.1 and below": ("bool", "integer")}, "torch")
+@with_unsupported_dtypes({"2.1.0 and below": ("bool", "integer")}, "torch")
def gaussian_nll_loss(input, target, var, full=False, eps=1e-6, reduction="mean"):
input, target = torch_frontend.promote_types_of_torch_inputs(input, target)
target, var = torch_frontend.promote_types_of_torch_inputs(target, var)
@@ -250,7 +246,7 @@ def gaussian_nll_loss(input, target, var, full=False, eps=1e-6, reduction="mean"
@to_ivy_arrays_and_back
-@with_unsupported_dtypes({"2.0.1 and below": ("float16", "bfloat16")}, "torch")
+@with_unsupported_dtypes({"2.1.0 and below": ("float16", "bfloat16")}, "torch")
@to_ivy_arrays_and_back
def hinge_embedding_loss(
input,
@@ -285,7 +281,7 @@ def huber_loss(
@to_ivy_arrays_and_back
-@with_supported_dtypes({"2.0.1 and below": ("float32", "float64")}, "torch")
+@with_supported_dtypes({"2.1.0 and below": ("float32", "float64")}, "torch")
def kl_div(
input, target, size_average=None, reduce=None, reduction="mean", log_target=False
):
@@ -301,7 +297,7 @@ def kl_div(
@to_ivy_arrays_and_back
-@with_supported_dtypes({"2.0.1 and below": ("float", "complex")}, "torch")
+@with_supported_dtypes({"2.1.0 and below": ("float", "complex")}, "torch")
def l1_loss(
input,
target,
@@ -316,7 +312,7 @@ def l1_loss(
@to_ivy_arrays_and_back
-@with_unsupported_dtypes({"2.0.1 and below": ("float16", "bfloat16")}, "torch")
+@with_unsupported_dtypes({"2.1.0 and below": ("float16", "bfloat16")}, "torch")
def margin_ranking_loss(
input1,
input2,
@@ -335,7 +331,7 @@ def margin_ranking_loss(
@to_ivy_arrays_and_back
-@with_unsupported_dtypes({"2.0.1 and below": ("bfloat16",)}, "torch")
+@with_unsupported_dtypes({"2.1.0 and below": ("bfloat16",)}, "torch")
def mse_loss(input, target, size_average=None, reduce=None, reduction="mean"):
reduction = _get_reduction(reduction, size_average, reduce)
result = ivy.square(input - target)
@@ -344,7 +340,7 @@ def mse_loss(input, target, size_average=None, reduce=None, reduction="mean"):
@to_ivy_arrays_and_back
-@with_unsupported_dtypes({"2.0.1 and below": ("float16", "bfloat16")}, "torch")
+@with_unsupported_dtypes({"2.1.0 and below": ("float16", "bfloat16")}, "torch")
def multilabel_margin_loss(
input, target, size_average=None, reduce=None, reduction="mean"
):
@@ -364,7 +360,7 @@ def multilabel_margin_loss(
@to_ivy_arrays_and_back
-@with_unsupported_dtypes({"2.0.1 and below": ("float16", "bfloat16")}, "torch")
+@with_unsupported_dtypes({"2.1.0 and below": ("float16", "bfloat16")}, "torch")
def multilabel_soft_margin_loss(
input,
target,
@@ -394,7 +390,7 @@ def multilabel_soft_margin_loss(
@to_ivy_arrays_and_back
@with_unsupported_dtypes(
- {"2.0.1 and below": ("float16", "int8", "int16", "int32")}, "torch"
+ {"2.1.0 and below": ("float16", "int8", "int16", "int32")}, "torch"
)
def nll_loss(
input,
@@ -440,7 +436,7 @@ def pairwise_distance(x1, x2, *, p=2.0, eps=1e-06, keepdim=False):
@to_ivy_arrays_and_back
-@with_unsupported_dtypes({"2.0.1 and below": ("float16", "bfloat16")}, "torch")
+@with_unsupported_dtypes({"2.1.0 and below": ("float16", "bfloat16")}, "torch")
def poisson_nll_loss(
input,
target,
@@ -467,7 +463,7 @@ def poisson_nll_loss(
@to_ivy_arrays_and_back
-@with_unsupported_dtypes({"2.0.1 and below": ("float16", "bfloat16")}, "torch")
+@with_unsupported_dtypes({"2.1.0 and below": ("float16", "bfloat16")}, "torch")
def smooth_l1_loss(
input,
target,
@@ -480,7 +476,7 @@ def smooth_l1_loss(
@to_ivy_arrays_and_back
-@with_unsupported_dtypes({"2.0.1 and below": ("float16", "bfloat16")}, "torch")
+@with_unsupported_dtypes({"2.1.0 and below": ("float16", "bfloat16")}, "torch")
def soft_margin_loss(
input,
target,
@@ -492,7 +488,7 @@ def soft_margin_loss(
@to_ivy_arrays_and_back
-@with_unsupported_dtypes({"2.0.1 and below": ("float16", "bfloat16")}, "torch")
+@with_unsupported_dtypes({"2.1.0 and below": ("float16", "bfloat16")}, "torch")
def triplet_margin_loss(
anchor,
positive,
@@ -547,7 +543,7 @@ def pairwise_distance(x1, x2, *, p=2.0, eps=1e-06, keepdim=False):
@to_ivy_arrays_and_back
-@with_unsupported_dtypes({"2.0.1 and below": ("float16", "bfloat16")}, "torch")
+@with_unsupported_dtypes({"2.1.0 and below": ("float16", "bfloat16")}, "torch")
def triplet_margin_with_distance_loss(
anchor,
positive,
diff --git a/ivy/functional/frontends/torch/nn/functional/non_linear_activation_functions.py b/ivy/functional/frontends/torch/nn/functional/non_linear_activation_functions.py
index 79d3a7d8f1d1a..c49e44d7218f7 100644
--- a/ivy/functional/frontends/torch/nn/functional/non_linear_activation_functions.py
+++ b/ivy/functional/frontends/torch/nn/functional/non_linear_activation_functions.py
@@ -5,18 +5,21 @@
@to_ivy_arrays_and_back
+@with_unsupported_dtypes(
+ {
+ "2.1.0 and below": (
+ "complex",
+ "float16",
+ )
+ },
+ "torch",
+)
def celu(input, alpha=1.0, inplace=False):
- prod = ivy.multiply(
- alpha,
- ivy.subtract(
- ivy.exp(ivy.divide(input, alpha)),
- 1,
- ),
- )
- return ivy.add(
- ivy.maximum(0, input),
- ivy.minimum(0, prod),
- )
+ return ivy.celu(input, alpha=alpha)
+
+
+def celu_(input, alpha=1.0):
+ return celu(input, alpha=alpha, inplace=True)
@to_ivy_arrays_and_back
@@ -35,7 +38,7 @@ def elu_(input, alpha=1.0):
@to_ivy_arrays_and_back
@with_unsupported_dtypes(
{
- "2.0.1 and below": (
+ "2.1.0 and below": (
"float16",
"bfloat16",
)
@@ -60,7 +63,7 @@ def glu(input, dim=-1):
@to_ivy_arrays_and_back
-@with_unsupported_dtypes({"2.0.1 and below": ("float16",)}, "torch")
+@with_unsupported_dtypes({"2.1.0 and below": ("float16",)}, "torch")
def gumbel_softmax(logits, tau=1, hard=False, eps=1e-10, dim=-1):
gumbels = -ivy.empty_like(logits).exponential().log()
gumbels = (logits + gumbels) / tau
@@ -97,24 +100,24 @@ def hardswish(input, inplace=False):
@to_ivy_arrays_and_back
-@with_unsupported_dtypes({"2.0.1 and below": ("float16",)}, "torch")
+@with_unsupported_dtypes({"2.1.0 and below": ("float16",)}, "torch")
def hardtanh(input, min_val=-1.0, max_val=1.0, inplace=False):
less = ivy.where(ivy.less(input, min_val), min_val, input)
return ivy.where(ivy.greater(input, max_val), max_val, less).astype(input.dtype)
-@with_unsupported_dtypes({"2.0.1 and below": ("float16",)}, "torch")
+@with_unsupported_dtypes({"2.1.0 and below": ("float16",)}, "torch")
def hardtanh_(input, min_val=-1.0, max_val=1.0):
return hardtanh(input, min_val=min_val, max_val=max_val, inplace=True)
@to_ivy_arrays_and_back
-@with_unsupported_dtypes({"2.0.1 and below": ("float16",)}, "torch")
+@with_unsupported_dtypes({"2.1.0 and below": ("float16",)}, "torch")
def leaky_relu(input, negative_slope=0.01, inplace=False):
return ivy.leaky_relu(input, alpha=negative_slope)
-@with_unsupported_dtypes({"2.0.1 and below": ("float16",)}, "torch")
+@with_unsupported_dtypes({"2.1.0 and below": ("float16",)}, "torch")
def leaky_relu_(input, negative_slope=0.01):
return leaky_relu(input, negative_slope=negative_slope, inplace=True)
@@ -154,7 +157,7 @@ def local_response_norm(input, size, alpha=0.0001, beta=0.75, k=1.0):
@to_ivy_arrays_and_back
-@with_unsupported_dtypes({"2.0.1 and below": ("float16",)}, "torch")
+@with_unsupported_dtypes({"2.1.0 and below": ("float16",)}, "torch")
def log_softmax(input, dim=None, _stacklevel=3, dtype=None):
if dtype:
input = ivy.astype(ivy.array(input), ivy.as_ivy_dtype(dtype))
@@ -166,7 +169,7 @@ def log_softmax(input, dim=None, _stacklevel=3, dtype=None):
@to_ivy_arrays_and_back
@with_unsupported_dtypes(
{
- "2.0.1 and below": (
+ "2.1.0 and below": (
"float16",
"bfloat16",
)
@@ -185,67 +188,6 @@ def mish(input, inplace=False):
)
-@to_ivy_arrays_and_back
-@with_supported_dtypes({"2.0.1 and below": ("float32", "float64")}, "torch")
-def multi_head_attention_forward(
- query,
- key,
- value,
- embed_dim_to_check,
- num_heads,
- in_proj_weight,
- in_proj_bias,
- bias_k,
- bias_v,
- add_zero_attn,
- dropout_p,
- out_proj_weight,
- out_proj_bias,
- training=True,
- key_padding_mask=None,
- need_weights=True,
- attn_mask=None,
- use_separate_proj_weight=False,
- q_proj_weight=None,
- k_proj_weight=None,
- v_proj_weight=None,
- static_k=None,
- static_v=None,
- average_attn_weights=True,
- is_causal=False,
-):
- embed_dim = query.shape[-1]
- assert (
- embed_dim == embed_dim_to_check
- ), f"was expecting embedding dimension of {embed_dim_to_check}, but got {embed_dim}"
- return ivy.multi_head_attention(
- query,
- key=key,
- value=value,
- batch_first=False,
- num_heads=num_heads,
- attention_mask=attn_mask,
- in_proj_weights=in_proj_weight if not use_separate_proj_weight else None,
- q_proj_weights=q_proj_weight,
- k_proj_weights=k_proj_weight,
- v_proj_weights=v_proj_weight,
- out_proj_weights=out_proj_weight,
- in_proj_bias=in_proj_bias,
- out_proj_bias=out_proj_bias,
- is_causal=is_causal and not (need_weights or key_padding_mask is not None),
- key_padding_mask=key_padding_mask,
- bias_k=bias_k,
- bias_v=bias_v,
- static_k=static_k,
- static_v=static_v,
- add_zero_attn=add_zero_attn,
- return_attention_weights=need_weights,
- average_attention_weights=average_attn_weights,
- dropout=dropout_p,
- training=training,
- )
-
-
@to_ivy_arrays_and_back
def normalize(input, p=2.0, dim=1, eps=1e-12, out=None):
abs_square = ivy.pow(ivy.abs(input), p)
@@ -266,7 +208,7 @@ def relu(input, inplace=False):
@to_ivy_arrays_and_back
-@with_unsupported_dtypes({"2.0.1 and below": ("complex",)}, "torch")
+@with_unsupported_dtypes({"2.1.0 and below": ("complex",)}, "torch")
def relu6(input, inplace=False):
return ivy.relu6(input)
@@ -276,7 +218,7 @@ def relu_(input):
@to_ivy_arrays_and_back
-@with_unsupported_dtypes({"2.0.1 and below": ("float16",)}, "torch")
+@with_unsupported_dtypes({"2.1.0 and below": ("float16",)}, "torch")
def rrelu(input, lower=1.0 / 8, upper=1.0 / 3, training=False, inplace=False):
if training:
# alpha = ivy.random_uniform(low=lower, high=upper)
@@ -289,13 +231,13 @@ def rrelu(input, lower=1.0 / 8, upper=1.0 / 3, training=False, inplace=False):
)
-@with_unsupported_dtypes({"2.0.1 and below": ("float16",)}, "torch")
+@with_unsupported_dtypes({"2.1.0 and below": ("float16",)}, "torch")
def rrelu_(input, lower=1.0 / 8, upper=1.0 / 3, training=False):
return rrelu(input, lower=lower, upper=upper, training=training, inplace=True)
@to_ivy_arrays_and_back
-@with_supported_dtypes({"2.0.1 and below": ("float32", "float64")}, "torch")
+@with_supported_dtypes({"2.1.0 and below": ("float32", "float64")}, "torch")
def scaled_dot_product_attention(
query, key, value, attn_mask=None, dropout_p=0.0, is_causal=False, scale=None
):
@@ -316,19 +258,19 @@ def selu(input, inplace=False):
@to_ivy_arrays_and_back
-@with_unsupported_dtypes({"2.0.1 and below": ("float16",)}, "torch")
+@with_unsupported_dtypes({"2.1.0 and below": ("float16",)}, "torch")
def sigmoid(input):
return ivy.sigmoid(input)
-@with_unsupported_dtypes({"2.0.1 and below": ("float16",)}, "torch")
+@with_unsupported_dtypes({"2.1.0 and below": ("float16",)}, "torch")
@to_ivy_arrays_and_back
def silu(input, inplace=False):
return ivy.multiply(input, ivy.sigmoid(input))
@to_ivy_arrays_and_back
-@with_unsupported_dtypes({"2.0.1 and below": ("float16",)}, "torch")
+@with_unsupported_dtypes({"2.1.0 and below": ("float16",)}, "torch")
def softmax(input, dim=None, _stacklevel=3, dtype=None):
if dtype:
input = ivy.astype(ivy.array(input), ivy.as_ivy_dtype(dtype))
@@ -336,7 +278,7 @@ def softmax(input, dim=None, _stacklevel=3, dtype=None):
@to_ivy_arrays_and_back
-@with_unsupported_dtypes({"2.0.1 and below": ("float16",)}, "torch")
+@with_unsupported_dtypes({"2.1.0 and below": ("float16",)}, "torch")
def softmin(input, dim=None, dtype=None):
if dtype:
input = ivy.astype(ivy.array(input), ivy.as_ivy_dtype(dtype))
@@ -346,7 +288,7 @@ def softmin(input, dim=None, dtype=None):
@to_ivy_arrays_and_back
@with_unsupported_dtypes(
{
- "2.0.1 and below": (
+ "2.1.0 and below": (
"float16",
"bfloat16",
)
@@ -370,23 +312,23 @@ def softsign(input):
@to_ivy_arrays_and_back
-@with_unsupported_dtypes({"2.0.1 and below": ("float16",)}, "torch")
+@with_unsupported_dtypes({"2.1.0 and below": ("float16",)}, "torch")
def tanh(input):
return ivy.tanh(input)
@to_ivy_arrays_and_back
-@with_unsupported_dtypes({"2.0.1 and below": ("float16",)}, "torch")
+@with_unsupported_dtypes({"2.1.0 and below": ("float16",)}, "torch")
def tanhshrink(input):
return ivy.subtract(input, ivy.tanh(input))
@to_ivy_arrays_and_back
-@with_unsupported_dtypes({"2.0.1 and below": ("float16",)}, "torch")
+@with_unsupported_dtypes({"2.1.0 and below": ("float16",)}, "torch")
def threshold(input, threshold, value, inplace=False):
return ivy.where(ivy.greater(input, threshold), input, value)
-@with_unsupported_dtypes({"2.0.1 and below": ("float16",)}, "torch")
+@with_unsupported_dtypes({"2.1.0 and below": ("float16",)}, "torch")
def threshold_(input, threshold, value):
return threshold(input, threshold, value, inplace=True)
diff --git a/ivy/functional/frontends/torch/nn/functional/norms.py b/ivy/functional/frontends/torch/nn/functional/norms.py
index e4009fe10da68..e833142814cbe 100644
--- a/ivy/functional/frontends/torch/nn/functional/norms.py
+++ b/ivy/functional/frontends/torch/nn/functional/norms.py
@@ -5,7 +5,7 @@
@with_unsupported_dtypes(
{
- "2.0.1 and below": (
+ "2.1.0 and below": (
"bfloat16",
"float16",
)
@@ -42,7 +42,7 @@ def batch_norm(
@to_ivy_arrays_and_back
@with_unsupported_dtypes(
{
- "2.0.1 and below": (
+ "2.1.0 and below": (
"float16",
"bfloat16",
)
@@ -57,7 +57,7 @@ def group_norm(input, num_groups, weight=None, bias=None, eps=1e-05):
@with_unsupported_dtypes(
{
- "2.0.1 and below": (
+ "2.1.0 and below": (
"bfloat16",
"float16",
)
@@ -92,12 +92,12 @@ def instance_norm(
@to_ivy_arrays_and_back
-@with_unsupported_dtypes({"2.0.1 and below": ("float16", "bfloat16")}, "torch")
+@with_unsupported_dtypes({"2.1.0 and below": ("float16", "bfloat16")}, "torch")
def layer_norm(input, normalized_shape, weight=None, bias=None, eps=1e-05):
shape = ivy.shape(input)
if isinstance(normalized_shape, int) and normalized_shape == shape[-1]:
axis = [-1]
else:
- assert ivy.equal(normalized_shape, shape[-len(normalized_shape) :])
+ assert ivy.all(ivy.equal(normalized_shape, shape[-len(normalized_shape) :]))
axis = list(range(len(shape) - len(normalized_shape), len(shape)))
return ivy.layer_norm(input, axis, scale=weight, offset=bias, eps=eps)
diff --git a/ivy/functional/frontends/torch/nn/functional/pooling_functions.py b/ivy/functional/frontends/torch/nn/functional/pooling_functions.py
index 856c603c481e9..46f2dec6a5dea 100644
--- a/ivy/functional/frontends/torch/nn/functional/pooling_functions.py
+++ b/ivy/functional/frontends/torch/nn/functional/pooling_functions.py
@@ -17,13 +17,13 @@ def _broadcast_pooling_helper(x, pool_dims: str = "2d", name: str = "padding"):
dims = {"1d": 1, "2d": 2, "3d": 3}
if isinstance(x, int):
- return tuple([x for _ in range(dims[pool_dims])])
+ return tuple(x for _ in range(dims[pool_dims]))
if len(x) == 1:
- return tuple([x[0] for _ in range(dims[pool_dims])])
+ return tuple(x[0] for _ in range(dims[pool_dims]))
elif len(x) == dims[pool_dims]:
return tuple(x)
- elif len(x) != dims[pool_dims]:
+ else:
raise ValueError(
f"`{name}` must either be a single int, "
f"or a tuple of {dims[pool_dims]} ints. "
@@ -36,7 +36,7 @@ def _broadcast_pooling_helper(x, pool_dims: str = "2d", name: str = "padding"):
@with_unsupported_dtypes(
{
- "2.0.1 and below": (
+ "2.1.0 and below": (
"bfloat16",
"float16",
)
@@ -50,7 +50,7 @@ def adaptive_avg_pool1d(input, output_size):
@with_unsupported_dtypes(
{
- "2.0.1 and below": (
+ "2.1.0 and below": (
"float16",
"bfloat16",
)
@@ -64,7 +64,7 @@ def adaptive_avg_pool2d(input, output_size):
@with_unsupported_dtypes(
{
- "2.0.1 and below": (
+ "2.1.0 and below": (
"bfloat16",
"float16",
)
@@ -81,6 +81,10 @@ def adaptive_max_pool2d(
return ivy.adaptive_max_pool2d(input, output_size)
+@with_unsupported_dtypes(
+ {"2.1.0 and below": ("float16",)},
+ "torch",
+)
@to_ivy_arrays_and_back
def avg_pool1d(
input,
@@ -90,30 +94,21 @@ def avg_pool1d(
ceil_mode=False,
count_include_pad=True,
):
- if stride is None:
- stride = kernel_size
- data_format = "NCW"
- # TODO: remove the broadcasting and padding string specification when ivy.avg_pool
- # support explicit padding
- kernel_size = _broadcast_pooling_helper(kernel_size, "1d", name="kernel_size")
- padding = _broadcast_pooling_helper(padding, "1d", name="padding")
- if all(
- [pad == ivy.ceil((kernel - 1) / 2) for kernel, pad in zip(kernel_size, padding)]
- ):
- padding = "SAME"
- else:
- padding = "VALID"
return ivy.avg_pool1d(
input,
kernel_size,
- stride,
- padding,
- data_format=data_format,
+ stride if stride is not None else kernel_size,
+ [(pad, pad) for pad in padding],
+ data_format="NCW",
count_include_pad=count_include_pad,
ceil_mode=ceil_mode,
)
+@with_unsupported_dtypes(
+ {"2.1.0 and below": ("float16",)},
+ "torch",
+)
@to_ivy_arrays_and_back
def avg_pool2d(
input,
@@ -124,31 +119,22 @@ def avg_pool2d(
count_include_pad=True,
divisor_override=None,
):
- if stride is None:
- stride = kernel_size
- data_format = "NCHW"
- # TODO: remove the broadcasting and padding string specification when ivy.avg_pool
- # support explicit padding
- kernel_size = _broadcast_pooling_helper(kernel_size, "2d", name="kernel_size")
- padding = _broadcast_pooling_helper(padding, "2d", name="padding")
- if all(
- [pad == ivy.ceil((kernel - 1) / 2) for kernel, pad in zip(kernel_size, padding)]
- ):
- padding = "SAME"
- else:
- padding = "VALID"
return ivy.avg_pool2d(
input,
kernel_size,
- stride,
- padding,
- data_format=data_format,
+ stride if stride is not None else kernel_size,
+ [(pad, pad) for pad in padding],
+ data_format="NCHW",
ceil_mode=ceil_mode,
count_include_pad=count_include_pad,
divisor_override=divisor_override,
)
+@with_unsupported_dtypes(
+ {"2.1.0 and below": ("float16", "bfloat16")},
+ "torch",
+)
@to_ivy_arrays_and_back
def avg_pool3d(
input,
@@ -159,23 +145,11 @@ def avg_pool3d(
count_include_pad=True,
divisor_override=None,
):
- if stride is None:
- stride = kernel_size
- # TODO: remove the broadcasting and padding string specification when ivy.avg_pool
- # support explicit padding
- kernel_size = _broadcast_pooling_helper(kernel_size, "3d", name="kernel_size")
- padding = _broadcast_pooling_helper(padding, "3d", name="padding")
- if all(
- [pad == ivy.ceil((kernel - 1) / 2) for kernel, pad in zip(kernel_size, padding)]
- ):
- padding = "SAME"
- else:
- padding = "VALID"
return ivy.avg_pool3d(
input,
kernel_size,
- stride,
- padding,
+ stride if stride is not None else kernel_size,
+ [(pad, pad) for pad in padding],
data_format="NCDHW",
ceil_mode=ceil_mode,
count_include_pad=count_include_pad,
@@ -185,7 +159,7 @@ def avg_pool3d(
@with_unsupported_dtypes(
{
- "2.0.1 and below": (
+ "2.1.0 and below": (
"float16",
"bfloat16",
)
@@ -261,7 +235,7 @@ def max_pool1d(
)
-@with_unsupported_dtypes({"2.0.1 and below": ("float16",)}, "torch")
+@with_unsupported_dtypes({"2.1.0 and below": ("float16",)}, "torch")
@to_ivy_arrays_and_back
def max_pool2d(
input,
@@ -285,7 +259,7 @@ def max_pool2d(
)
-@with_unsupported_dtypes({"2.0.1 and below": ("float16",)}, "torch")
+@with_unsupported_dtypes({"2.1.0 and below": ("float16",)}, "torch")
@to_ivy_arrays_and_back
def max_pool3d(
input,
diff --git a/ivy/functional/frontends/torch/nn/functional/sparse_functions.py b/ivy/functional/frontends/torch/nn/functional/sparse_functions.py
index 586fd3b094a54..b50c5b16b5fba 100644
--- a/ivy/functional/frontends/torch/nn/functional/sparse_functions.py
+++ b/ivy/functional/frontends/torch/nn/functional/sparse_functions.py
@@ -33,7 +33,7 @@ def embedding(
return ret
-@with_supported_dtypes({"2.0.1 and below": ("int64",)}, "torch")
+@with_supported_dtypes({"2.1.0 and below": ("int64",)}, "torch")
@to_ivy_arrays_and_back
def one_hot(tensor, num_classes=-1):
return ivy.astype(ivy.one_hot(tensor, num_classes), tensor.dtype)
diff --git a/ivy/functional/frontends/torch/nn/functional/vision_functions.py b/ivy/functional/frontends/torch/nn/functional/vision_functions.py
index d48c0992dee71..39e5ce64eb99d 100644
--- a/ivy/functional/frontends/torch/nn/functional/vision_functions.py
+++ b/ivy/functional/frontends/torch/nn/functional/vision_functions.py
@@ -1,11 +1,9 @@
# global
-import math
# local
import ivy
from ivy import with_unsupported_dtypes, with_supported_dtypes
from ivy.functional.frontends.torch.func_wrapper import to_ivy_arrays_and_back
-from ivy.utils.exceptions import IvyNotImplementedException
# --- Helpers --- #
@@ -33,7 +31,7 @@ def _handle_padding_shape(padding, n, mode):
# ------------ #
-@with_unsupported_dtypes({"2.0.1 and below": ("float16", "bfloat16")}, "torch")
+@with_unsupported_dtypes({"2.1.0 and below": ("float16", "bfloat16")}, "torch")
@to_ivy_arrays_and_back
def affine_grid(theta, size, align_corners=False):
if len(size) == 4:
@@ -96,7 +94,7 @@ def cubic_conv2(A, x):
return ((A * x - 5 * A) * x + 8 * A) * x - 4 * A
-@with_supported_dtypes({"2.0.1 and below": ("float32", "float64")}, "torch")
+@with_supported_dtypes({"2.1.0 and below": ("float32", "float64")}, "torch")
@to_ivy_arrays_and_back
def grid_sample(
input, grid, mode="bilinear", padding_mode="zeros", align_corners=False
@@ -351,7 +349,7 @@ def grid_sample_padding(grid, padding_mode, align_corners, borders=None):
@with_unsupported_dtypes(
{
- "2.0.1 and below": (
+ "2.1.0 and below": (
"bfloat16",
"float16",
)
@@ -368,121 +366,14 @@ def interpolate(
recompute_scale_factor=None,
antialias=False,
):
- if mode in ["nearest", "area", "nearest-exact"]:
- ivy.utils.assertions.check_exists(
- align_corners,
- inverse=True,
- message=(
- "align_corners option can only be set with the interpolating modes:"
- " linear | bilinear | bicubic | trilinear"
- ),
- )
- else:
- if not ivy.exists(align_corners):
- align_corners = False
-
- dim = ivy.get_num_dims(input) - 2 # Number of spatial dimensions.
-
- if ivy.exists(size) and ivy.exists(scale_factor):
- raise ivy.utils.exceptions.IvyException(
- "only one of size or scale_factor should be defined"
- )
-
- elif ivy.exists(size) and not ivy.exists(scale_factor):
- scale_factors = None
-
- if isinstance(size, (list, tuple)):
- ivy.utils.assertions.check_equal(
- len(size),
- dim,
- inverse=False,
- message=(
- "Input and output must have the "
- "same number of spatial dimensions,"
- f" but got input with spatial dimensions of {list(input.shape[2:])}"
- f" and output size of {size}. "
- "Please provide input tensor in (N, C, d1, d2, ...,dK) format"
- " and output size in (o1, o2, ...,oK) format."
- ),
- as_array=False,
- )
- output_size = size
- else:
- output_size = [size for _ in range(dim)]
-
- elif ivy.exists(scale_factor) and not ivy.exists(size):
- output_size = None
-
- if isinstance(scale_factor, (list, tuple)):
- ivy.utils.assertions.check_equal(
- len(scale_factor),
- dim,
- inverse=False,
- message=(
- "Input and scale_factor must have the "
- "same number of spatial dimensions,"
- f" but got input with spatial dimensions of {list(input.shape[2:])}"
- f" and scale_factor of shape {scale_factor}. "
- "Please provide input tensor in (N, C, d1, d2, ...,dK) format"
- " and scale_factor in (s1, s2, ...,sK) format."
- ),
- as_array=False,
- )
- scale_factors = scale_factor
- else:
- scale_factors = [scale_factor for _ in range(dim)]
-
- else:
- ivy.utils.assertions.check_any(
- [ivy.exists(size), ivy.exists(scale_factor)],
- message="either size or scale_factor should be defined",
- as_array=False,
- )
-
- if (
- ivy.exists(size)
- and ivy.exists(recompute_scale_factor)
- and bool(recompute_scale_factor)
- ):
- raise ivy.utils.exceptions.IvyException(
- "recompute_scale_factor is not meaningful with an explicit size."
- )
-
- if ivy.exists(scale_factors):
- output_size = [
- math.floor(ivy.shape(input)[i + 2] * scale_factors[i]) for i in range(dim)
- ]
-
if (
- bool(antialias)
- and (mode not in ["bilinear", "bicubic"])
- and ivy.get_num_dims(input) == 4
+ mode not in ["linear", "bilinear", "bicubic", "trilinear"]
+ and align_corners is not None
):
raise ivy.utils.exceptions.IvyException(
- "recompute_scale_factor is not meaningful with an explicit size."
- )
-
- if ivy.get_num_dims(input) == 3 and mode == "bilinear":
- raise IvyNotImplementedException(
- "Got 3D input, but bilinear mode needs 4D input"
- )
- if ivy.get_num_dims(input) == 3 and mode == "trilinear":
- raise IvyNotImplementedException(
- "Got 3D input, but trilinear mode needs 5D input"
- )
- if ivy.get_num_dims(input) == 4 and mode == "linear":
- raise IvyNotImplementedException("Got 4D input, but linear mode needs 3D input")
- if ivy.get_num_dims(input) == 4 and mode == "trilinear":
- raise IvyNotImplementedException(
- "Got 4D input, but trilinear mode needs 5D input"
+ "align_corners option can only be set with the interpolating"
+ f"modes: linear | bilinear | bicubic | trilinear (got {mode})"
)
- if ivy.get_num_dims(input) == 5 and mode == "linear":
- raise IvyNotImplementedException("Got 5D input, but linear mode needs 3D input")
- if ivy.get_num_dims(input) == 5 and mode == "bilinear":
- raise IvyNotImplementedException(
- "Got 5D input, but bilinear mode needs 4D input"
- )
-
ivy.utils.assertions.check_elem_in_list(
ivy.get_num_dims(input),
range(3, 6),
@@ -492,9 +383,14 @@ def interpolate(
f" bicubic | trilinear | area | nearest-exact (got {mode})"
),
)
-
return ivy.interpolate(
- input, output_size, mode=mode, align_corners=align_corners, antialias=antialias
+ input,
+ size,
+ mode=mode,
+ scale_factor=scale_factor,
+ recompute_scale_factor=recompute_scale_factor,
+ align_corners=True if align_corners else False,
+ antialias=antialias,
)
@@ -519,8 +415,10 @@ def pixel_shuffle(input, upscale_factor):
ivy.utils.assertions.check_equal(
ivy.get_num_dims(input),
4,
- message="pixel_shuffle expects 4D input, but got input with sizes "
- + str(input_shape),
+ message=(
+ "pixel_shuffle expects 4D input, but got input with sizes"
+ f" {str(input_shape)}"
+ ),
as_array=False,
)
b = input_shape[0]
@@ -608,7 +506,7 @@ def reflect(x, low2, high2):
return x
-@with_unsupported_dtypes({"2.0.1 and below": ("float16", "bfloat16")}, "torch")
+@with_unsupported_dtypes({"2.1.0 and below": ("float16", "bfloat16")}, "torch")
@to_ivy_arrays_and_back
def upsample(
input,
@@ -626,7 +524,7 @@ def upsample(
)
-@with_unsupported_dtypes({"2.0.1 and below": ("float16", "bfloat16")}, "torch")
+@with_unsupported_dtypes({"2.1.0 and below": ("float16", "bfloat16")}, "torch")
@to_ivy_arrays_and_back
def upsample_bilinear(input, size=None, scale_factor=None):
return interpolate(
@@ -634,7 +532,7 @@ def upsample_bilinear(input, size=None, scale_factor=None):
)
-@with_unsupported_dtypes({"2.0.1 and below": ("float16", "bfloat16")}, "torch")
+@with_unsupported_dtypes({"2.1.0 and below": ("float16", "bfloat16")}, "torch")
@to_ivy_arrays_and_back
def upsample_nearest(input, size=None, scale_factor=None):
return interpolate(input, size=size, scale_factor=scale_factor, mode="nearest")
diff --git a/ivy/functional/frontends/torch/pointwise_ops.py b/ivy/functional/frontends/torch/pointwise_ops.py
index 899d5db6820fb..999c815825345 100644
--- a/ivy/functional/frontends/torch/pointwise_ops.py
+++ b/ivy/functional/frontends/torch/pointwise_ops.py
@@ -13,13 +13,13 @@ def abs(input, *, out=None):
return ivy.abs(input, out=out)
-@with_unsupported_dtypes({"2.0.1 and below": ("float16",)}, "torch")
+@with_unsupported_dtypes({"2.1.0 and below": ("float16",)}, "torch")
@to_ivy_arrays_and_back
def acos(input, *, out=None):
return ivy.acos(input, out=out)
-@with_unsupported_dtypes({"2.0.1 and below": ("float16",)}, "torch")
+@with_unsupported_dtypes({"2.1.0 and below": ("float16",)}, "torch")
@to_ivy_arrays_and_back
def acosh(input, *, out=None):
return ivy.acosh(input, out=out)
@@ -35,13 +35,13 @@ def add(input, other, *, alpha=1, out=None):
@to_ivy_arrays_and_back
-@with_unsupported_dtypes({"2.0.1 and below": ("float16", "bfloat16")}, "torch")
+@with_unsupported_dtypes({"2.1.0 and below": ("float16", "bfloat16")}, "torch")
def addcdiv(input, tensor1, tensor2, *, value=1, out=None):
return ivy.add(input, ivy.multiply(value, ivy.divide(tensor1, tensor2)), out=out)
@to_ivy_arrays_and_back
-@with_unsupported_dtypes({"2.0.1 and below": ("float16", "bfloat16")}, "torch")
+@with_unsupported_dtypes({"2.1.0 and below": ("float16", "bfloat16")}, "torch")
def addcmul(input, tensor1, tensor2, *, value=1, out=None):
return ivy.add(input, ivy.multiply(value, ivy.multiply(tensor1, tensor2)), out=out)
@@ -51,32 +51,32 @@ def angle(input, *, out=None):
return ivy.angle(input, out=out)
-@with_unsupported_dtypes({"2.0.1 and below": ("float16",)}, "torch")
+@with_unsupported_dtypes({"2.1.0 and below": ("float16",)}, "torch")
@to_ivy_arrays_and_back
def asin(input, *, out=None):
return ivy.asin(input, out=out)
-@with_unsupported_dtypes({"2.0.1 and below": ("float16",)}, "torch")
+@with_unsupported_dtypes({"2.1.0 and below": ("float16",)}, "torch")
@to_ivy_arrays_and_back
def asinh(input, *, out=None):
return ivy.asinh(input, out=out)
-@with_unsupported_dtypes({"2.0.1 and below": ("float16",)}, "torch")
+@with_unsupported_dtypes({"2.1.0 and below": ("float16",)}, "torch")
@to_ivy_arrays_and_back
def atan(input, *, out=None):
return ivy.atan(input, out=out)
-@with_unsupported_dtypes({"2.0.1 and below": ("float16", "bfloat16")}, "torch")
+@with_unsupported_dtypes({"2.1.0 and below": ("float16", "bfloat16")}, "torch")
@to_ivy_arrays_and_back
def atan2(input, other, *, out=None):
input, other = torch_frontend.promote_types_of_torch_inputs(input, other)
return ivy.atan2(input, other, out=out)
-@with_unsupported_dtypes({"2.0.1 and below": ("float16",)}, "torch")
+@with_unsupported_dtypes({"2.1.0 and below": ("float16",)}, "torch")
@to_ivy_arrays_and_back
def atanh(input, *, out=None):
return ivy.atanh(input, out=out)
@@ -117,13 +117,13 @@ def bitwise_xor(input, other, *, out=None):
return ivy.bitwise_xor(input, other, out=out)
-@with_unsupported_dtypes({"2.0.1 and below": ("float16",)}, "torch")
+@with_unsupported_dtypes({"2.1.0 and below": ("float16",)}, "torch")
@to_ivy_arrays_and_back
def ceil(input, *, out=None):
return ivy.ceil(input, out=out)
-@with_unsupported_dtypes({"2.0.1 and below": ("float16", "complex")}, "torch")
+@with_unsupported_dtypes({"2.1.0 and below": ("float16", "complex")}, "torch")
@to_ivy_arrays_and_back
def clamp(input, min=None, max=None, *, out=None):
ivy.utils.assertions.check_all_or_any_fn(
@@ -152,7 +152,7 @@ def copysign(input, other, *, out=None):
return ivy.copysign(input, other, out=out)
-@with_unsupported_dtypes({"2.0.1 and below": ("float16",)}, "torch")
+@with_unsupported_dtypes({"2.1.0 and below": ("float16",)}, "torch")
@to_ivy_arrays_and_back
def cos(input, *, out=None):
return ivy.cos(input, out=out)
@@ -181,31 +181,31 @@ def div(input, other, *, rounding_mode=None, out=None):
return ivy.divide(input, other, out=out)
-@with_unsupported_dtypes({"2.0.1 and below": ("float16", "complex")}, "torch")
+@with_unsupported_dtypes({"2.1.0 and below": ("float16", "complex")}, "torch")
@to_ivy_arrays_and_back
def erf(input, *, out=None):
return ivy.erf(input, out=out)
-@with_unsupported_dtypes({"2.0.1 and below": ("float16", "complex")}, "torch")
+@with_unsupported_dtypes({"2.1.0 and below": ("float16", "complex")}, "torch")
@to_ivy_arrays_and_back
def erfc(input, *, out=None):
return 1.0 - ivy.erf(input, out=out)
-@with_unsupported_dtypes({"2.0.1 and below": ("float16",)}, "torch")
+@with_unsupported_dtypes({"2.1.0 and below": ("float16",)}, "torch")
@to_ivy_arrays_and_back
def exp(input, *, out=None):
return ivy.exp(input, out=out)
-@with_unsupported_dtypes({"2.0.1 and below": ("float16",)}, "torch")
+@with_unsupported_dtypes({"2.1.0 and below": ("float16",)}, "torch")
@to_ivy_arrays_and_back
def exp2(input, out=None):
return ivy.exp2(input, out=out)
-@with_unsupported_dtypes({"2.0.1 and below": ("float16",)}, "torch")
+@with_unsupported_dtypes({"2.1.0 and below": ("float16",)}, "torch")
@to_ivy_arrays_and_back
def expm1(input, out=None):
return ivy.expm1(input, out=out)
@@ -223,7 +223,7 @@ def float_power(input, exponent, *, out=None):
return ivy.float_power(input, exponent, out=out)
-@with_unsupported_dtypes({"2.0.1 and below": ("float16",)}, "torch")
+@with_unsupported_dtypes({"2.1.0 and below": ("float16",)}, "torch")
@to_ivy_arrays_and_back
def floor(input, *, out=None):
return ivy.floor(input, out=out)
@@ -234,7 +234,7 @@ def floor_divide(input, other, *, out=None):
return ivy.floor_divide(input, other, out=out)
-@with_unsupported_dtypes({"2.0.1 and below": ("float16", "bfloat16")}, "torch")
+@with_unsupported_dtypes({"2.1.0 and below": ("float16", "bfloat16")}, "torch")
@to_ivy_arrays_and_back
def fmod(x1, x2, out=None):
return ivy.fmod(x1, x2, out=out)
@@ -245,31 +245,31 @@ def frac(input, *, out=None):
return input - ivy.sign(input) * ivy.floor(ivy.abs(input))
-@with_unsupported_dtypes({"2.0.1 and below": ("float16", "bfloat16")}, "torch")
+@with_unsupported_dtypes({"2.1.0 and below": ("float16", "bfloat16")}, "torch")
@to_ivy_arrays_and_back
def frexp(input, *, out=None):
return ivy.frexp(input, out=out)
-@with_unsupported_dtypes({"2.0.1 and below": ("bfloat16",)}, "torch")
+@with_unsupported_dtypes({"2.1.0 and below": ("bfloat16",)}, "torch")
@to_ivy_arrays_and_back
def gradient(input, *, spacing=1, dim=None, edge_order=1):
return ivy.gradient(input, spacing=spacing, edge_order=edge_order, axis=dim)
-@with_unsupported_dtypes({"2.0.1 and below": ("float16",)}, "torch")
+@with_unsupported_dtypes({"2.1.0 and below": ("float16",)}, "torch")
@to_ivy_arrays_and_back
def hypot(input, other, *, out=None):
return ivy.hypot(input, other, out=out)
-@with_unsupported_dtypes({"2.0.1 and below": ("float16",)}, "torch")
+@with_unsupported_dtypes({"2.1.0 and below": ("float16",)}, "torch")
@to_ivy_arrays_and_back
def i0(input, *, out=None):
return ivy.i0(input, out=out)
-@with_unsupported_dtypes({"2.0.1 and below": ("bfloat16",)}, "torch")
+@with_unsupported_dtypes({"2.1.0 and below": ("bfloat16",)}, "torch")
@to_ivy_arrays_and_back
def igamma(input, other, *, out=None):
return ivy.igamma(input, x=other, out=out)
@@ -280,7 +280,7 @@ def imag(input):
return ivy.imag(input)
-@with_supported_dtypes({"2.0.1 and below": ("float16", "float32", "float64")}, "torch")
+@with_supported_dtypes({"2.1.0 and below": ("float16", "float32", "float64")}, "torch")
@to_ivy_arrays_and_back
def ldexp(input, other, *, out=None):
value = ivy.pow(2, other, out=out)
@@ -288,49 +288,49 @@ def ldexp(input, other, *, out=None):
return value
-@with_unsupported_dtypes({"2.0.1 and below": ("float16", "bfloat16")}, "torch")
+@with_unsupported_dtypes({"2.1.0 and below": ("float16", "bfloat16")}, "torch")
@to_ivy_arrays_and_back
def lerp(input, end, weight, *, out=None):
return ivy.lerp(input, end, weight, out=out)
-@with_unsupported_dtypes({"2.0.1 and below": ("float16",)}, "torch")
+@with_unsupported_dtypes({"2.1.0 and below": ("float16",)}, "torch")
@to_ivy_arrays_and_back
def lgamma(input, *, out=None):
return ivy.lgamma(input, out=out)
@to_ivy_arrays_and_back
-@with_unsupported_dtypes({"2.0.1 and below": ("float16",)}, "torch")
+@with_unsupported_dtypes({"2.1.0 and below": ("float16",)}, "torch")
def log(input, *, out=None):
return ivy.log(input, out=out)
-@with_unsupported_dtypes({"2.0.1 and below": ("float16",)}, "torch")
+@with_unsupported_dtypes({"2.1.0 and below": ("float16",)}, "torch")
@to_ivy_arrays_and_back
def log10(input, *, out=None):
return ivy.log10(input, out=out)
-@with_unsupported_dtypes({"2.0.1 and below": ("float16",)}, "torch")
+@with_unsupported_dtypes({"2.1.0 and below": ("float16",)}, "torch")
@to_ivy_arrays_and_back
def log1p(input, *, out=None):
return ivy.log1p(input, out=out)
-@with_unsupported_dtypes({"2.0.1 and below": ("float16",)}, "torch")
+@with_unsupported_dtypes({"2.1.0 and below": ("float16",)}, "torch")
@to_ivy_arrays_and_back
def log2(input, *, out=None):
return ivy.log2(input, out=out)
-@with_unsupported_dtypes({"2.0.1 and below": ("float16",)}, "torch")
+@with_unsupported_dtypes({"2.1.0 and below": ("float16",)}, "torch")
@to_ivy_arrays_and_back
def logaddexp(x1, x2, out=None):
return ivy.logaddexp(x1, x2, out=out)
-@with_unsupported_dtypes({"2.0.1 and below": ("float16",)}, "torch")
+@with_unsupported_dtypes({"2.1.0 and below": ("float16",)}, "torch")
@to_ivy_arrays_and_back
def logaddexp2(x1, x2, out=None):
return ivy.logaddexp2(x1, x2, out=out)
@@ -359,13 +359,13 @@ def logical_xor(input, other, *, out=None):
return ivy.logical_xor(input, other, out=out)
-@with_unsupported_dtypes({"2.0.1 and below": ("float16", "bfloat16")}, "torch")
+@with_unsupported_dtypes({"2.1.0 and below": ("float16", "bfloat16")}, "torch")
@to_ivy_arrays_and_back
def logit(input, eps=None, *, out=None):
return ivy.logit(input, eps=eps, out=out)
-@with_unsupported_dtypes({"2.0.1 and below": ("bfloat16",)}, "torch")
+@with_unsupported_dtypes({"2.1.0 and below": ("bfloat16",)}, "torch")
@to_ivy_arrays_and_back
def masked_fill(input, mask, value):
return ivy.where(mask, value, input, out=input)
@@ -378,7 +378,7 @@ def mul(input, other, *, out=None):
@to_ivy_arrays_and_back
-@with_unsupported_dtypes({"2.0.1 and below": ("float16",)}, "torch")
+@with_unsupported_dtypes({"2.1.0 and below": ("float16",)}, "torch")
def mvlgamma(input, p, *, out=None):
ivy.assertions.check_greater(
p, 1, allow_equal=True, message="p has to be greater than or equal to 1"
@@ -393,19 +393,19 @@ def mvlgamma(input, p, *, out=None):
)
-@with_unsupported_dtypes({"2.0.1 and below": ("bfloat16",)}, "tensorflow")
+@with_unsupported_dtypes({"2.1.0 and below": ("bfloat16",)}, "tensorflow")
@to_ivy_arrays_and_back
def nan_to_num(input, nan=0.0, posinf=None, neginf=None, *, out=None):
return ivy.nan_to_num(input, nan=nan, posinf=posinf, neginf=neginf, out=out)
-@with_unsupported_dtypes({"2.0.1 and below": ("bool",)}, "torch")
+@with_unsupported_dtypes({"2.1.0 and below": ("bool",)}, "torch")
@to_ivy_arrays_and_back
def negative(input, *, out=None):
return ivy.negative(input, out=out)
-@with_unsupported_dtypes({"2.0.1 and below": ("bfloat16", "float16")}, "torch")
+@with_unsupported_dtypes({"2.1.0 and below": ("bfloat16", "float16")}, "torch")
@to_ivy_arrays_and_back
def nextafter(input, other, *, out=None):
input, other = torch_frontend.promote_types_of_torch_inputs(input, other)
@@ -417,7 +417,7 @@ def positive(input, *, out=None):
return ivy.positive(input, out=out)
-@with_unsupported_dtypes({"2.0.1 and below": ("bool",)}, "torch")
+@with_unsupported_dtypes({"2.1.0 and below": ("bool",)}, "torch")
@to_ivy_arrays_and_back
def pow(input, exponent, *, out=None):
if not ivy.is_array(exponent):
@@ -460,7 +460,7 @@ def remainder(input, other, *, out=None):
return ivy.remainder(input, other, out=out)
-@with_unsupported_dtypes({"2.0.1 and below": ("bfloat16",)}, "torch")
+@with_unsupported_dtypes({"2.1.0 and below": ("bfloat16",)}, "torch")
@to_ivy_arrays_and_back
def round(input, *, decimals=0, out=None):
m = ivy.full(input.shape, 10.0**decimals)
@@ -469,7 +469,7 @@ def round(input, *, decimals=0, out=None):
return ivy.divide(rounded, m, out=out)
-@with_unsupported_dtypes({"2.0.1 and below": ("float16",)}, "torch")
+@with_unsupported_dtypes({"2.1.0 and below": ("float16",)}, "torch")
@to_ivy_arrays_and_back
def rsqrt(input, *, out=None):
return ivy.reciprocal(ivy.sqrt(input), out=out)
@@ -488,43 +488,43 @@ def sgn(input, *, out=None):
return ivy.sign(input, out=out)
-@with_unsupported_dtypes({"2.0.1 and below": ("float16",)}, "torch")
+@with_unsupported_dtypes({"2.1.0 and below": ("float16",)}, "torch")
@to_ivy_arrays_and_back
def sigmoid(input, *, out=None):
return ivy.sigmoid(input, out=out)
-@with_unsupported_dtypes({"2.0.1 and below": ("complex",)}, "torch")
+@with_unsupported_dtypes({"2.1.0 and below": ("complex",)}, "torch")
@to_ivy_arrays_and_back
def sign(input, *, out=None):
return ivy.sign(input, out=out)
-@with_unsupported_dtypes({"2.0.1 and below": ("complex",)}, "torch")
+@with_unsupported_dtypes({"2.1.0 and below": ("complex",)}, "torch")
@to_ivy_arrays_and_back
def signbit(input, *, out=None):
return ivy.signbit(input, out=out)
-@with_unsupported_dtypes({"2.0.1 and below": ("float16",)}, "torch")
+@with_unsupported_dtypes({"2.1.0 and below": ("float16",)}, "torch")
@to_ivy_arrays_and_back
def sin(input, *, out=None):
return ivy.sin(input, out=out)
-@with_unsupported_dtypes({"2.0.1 and below": ("float16",)}, "torch")
+@with_unsupported_dtypes({"2.1.0 and below": ("float16",)}, "torch")
@to_ivy_arrays_and_back
def sinc(input, *, out=None):
return ivy.sinc(input, out=out)
-@with_unsupported_dtypes({"2.0.1 and below": ("float16",)}, "torch")
+@with_unsupported_dtypes({"2.1.0 and below": ("float16",)}, "torch")
@to_ivy_arrays_and_back
def sinh(input, *, out=None):
return ivy.sinh(input, out=out)
-@with_unsupported_dtypes({"2.0.1 and below": ("float16",)}, "torch")
+@with_unsupported_dtypes({"2.1.0 and below": ("float16",)}, "torch")
@to_ivy_arrays_and_back
def sqrt(input, *, out=None):
return ivy.sqrt(input, out=out)
@@ -541,13 +541,13 @@ def subtract(input, other, *, alpha=1, out=None):
return ivy.subtract(input, other * alpha, out=out)
-@with_unsupported_dtypes({"2.0.1 and below": ("float16",)}, "torch")
+@with_unsupported_dtypes({"2.1.0 and below": ("float16",)}, "torch")
@to_ivy_arrays_and_back
def tan(input, *, out=None):
return ivy.tan(input, out=out)
-@with_unsupported_dtypes({"2.0.1 and below": ("float16",)}, "torch")
+@with_unsupported_dtypes({"2.1.0 and below": ("float16",)}, "torch")
@to_ivy_arrays_and_back
def tanh(input, *, out=None):
return ivy.tanh(input, out=out)
@@ -559,13 +559,13 @@ def true_divide(input, other, *, out=None):
return ivy.divide(input, other, out=out)
-@with_unsupported_dtypes({"2.0.1 and below": ("float16",)}, "torch")
+@with_unsupported_dtypes({"2.1.0 and below": ("float16",)}, "torch")
@to_ivy_arrays_and_back
def trunc(input, *, out=None):
return ivy.trunc(input, out=out)
-@with_unsupported_dtypes({"2.0.1 and below": ("bfloat16",)}, "tensorflow")
+@with_unsupported_dtypes({"2.1.0 and below": ("bfloat16",)}, "tensorflow")
@to_ivy_arrays_and_back
def xlogy(input, other, *, out=None):
return ivy.xlogy(input, other, out=out)
diff --git a/ivy/functional/frontends/torch/random_sampling.py b/ivy/functional/frontends/torch/random_sampling.py
index 2ddb8de7c018d..9ce4b6822bc25 100644
--- a/ivy/functional/frontends/torch/random_sampling.py
+++ b/ivy/functional/frontends/torch/random_sampling.py
@@ -5,7 +5,7 @@
@with_supported_dtypes(
{
- "2.0.1 and below": (
+ "2.1.0 and below": (
"float32",
"float64",
)
@@ -26,7 +26,7 @@ def manual_seed(seed: int):
@with_supported_dtypes(
{
- "2.0.1 and below": (
+ "2.1.0 and below": (
"float32",
"float64",
)
@@ -49,7 +49,7 @@ def multinomial(input, num_samples, replacement=False, *, generator=None, out=No
@with_supported_dtypes(
{
- "2.0.1 and below": (
+ "2.1.0 and below": (
"float32",
"float64",
)
@@ -64,7 +64,7 @@ def normal(mean, std, *, generator=None, out=None):
@with_supported_dtypes(
{
- "2.0.1 and below": (
+ "2.1.0 and below": (
"float32",
"float64",
)
@@ -91,7 +91,7 @@ def rand(
):
if not size and "size" not in kwargs:
raise ValueError("Missing 1 required positional/keyword argument: size")
- size = kwargs["size"] if not size else size
+ size = size if size else kwargs["size"]
if (
isinstance(size, (list, tuple))
and len(size) == 1
@@ -191,7 +191,7 @@ def randn(
):
if not size and "size" not in kwargs:
raise ValueError("Missing 1 required positional/keyword argument: size")
- size = kwargs["size"] if not size else size
+ size = size if size else kwargs["size"]
if (
isinstance(size, (list, tuple))
and len(size) == 1
diff --git a/ivy/functional/frontends/torch/reduction_ops.py b/ivy/functional/frontends/torch/reduction_ops.py
index d4a5305ed6642..6e9aca464b8ed 100644
--- a/ivy/functional/frontends/torch/reduction_ops.py
+++ b/ivy/functional/frontends/torch/reduction_ops.py
@@ -32,7 +32,7 @@ def amin(input, dim=None, keepdim=False, *, out=None):
@numpy_to_torch_style_args
@to_ivy_arrays_and_back
-@with_unsupported_dtypes({"2.0.1 and below": ("float16", "bfloat16")}, "torch")
+@with_unsupported_dtypes({"2.1.0 and below": ("float16", "bfloat16")}, "torch")
def aminmax(input, *, dim=None, keepdim=False, out=None):
minmax_tuple = namedtuple("minmax", ["min", "max"])
return minmax_tuple(
@@ -51,6 +51,7 @@ def any(input, dim=None, keepdim=False, *, out=None):
return ret
+@with_unsupported_dtypes({"2.1.0 and below": ("complex", "bool")}, "torch")
@numpy_to_torch_style_args
@to_ivy_arrays_and_back
def argmax(input, dim=None, keepdim=False):
@@ -66,7 +67,7 @@ def argmin(input, dim=None, keepdim=False):
@numpy_to_torch_style_args
@to_ivy_arrays_and_back
@with_unsupported_dtypes(
- {"2.0.1 and below": ("uint8", "int8")},
+ {"2.1.0 and below": ("uint8", "int8")},
"torch",
)
def count_nonzero(input, dim=None):
@@ -161,6 +162,10 @@ def median(input, dim=None, keepdim=False, *, out=None):
@numpy_to_torch_style_args
@to_ivy_arrays_and_back
+@with_unsupported_dtypes(
+ {"2.1.0 and below": ("complex64", "complex128")},
+ "torch",
+)
def min(*input, dim=None, keepdim=False, out=None):
if len(input) == 1:
input = input[0]
@@ -191,9 +196,45 @@ def nanmean(input, dim=None, keepdim=False, *, dtype=None, out=None):
return ivy.nanmean(input, axis=dim, keepdims=keepdim, dtype=dtype, out=out)
+@numpy_to_torch_style_args
+@to_ivy_arrays_and_back
+def nanmedian(input, dim=None, keepdim=False, *, out=None):
+ if dim is None:
+ flattened_input = ivy.flatten(input)
+ sorted_input = ivy.sort(flattened_input)
+ nonnan_index = int(sorted_input.shape[0] - ivy.isnan(sorted_input).sum())
+ return sorted_input[(nonnan_index - 1) // 2]
+
+ nanmedian_tuple = namedtuple("nanmedian", ["values", "indices"])
+
+ if input.ndim == 0:
+ result = nanmedian_tuple(input, ivy.array(0))
+ else:
+ sorted_indices = ivy.argsort(input, axis=dim)
+ nonnan_index = (
+ sorted_indices.shape[dim] - ivy.isnan(input).sum(axis=1) - 1
+ ) // 2
+ nonnan_index = ivy.expand_dims(nonnan_index, axis=1)
+ nanmedian_indices = ivy.gather_nd(sorted_indices, nonnan_index, batch_dims=1)
+ nanmedian_values = ivy.take_along_axis(
+ input, ivy.expand_dims(nanmedian_indices, axis=dim), dim
+ ).squeeze(axis=dim)
+
+ if keepdim:
+ nanmedian_values = ivy.expand_dims(nanmedian_values, axis=dim)
+ nanmedian_indices = ivy.expand_dims(nanmedian_tuple, axis=dim)
+
+ result = nanmedian_tuple(nanmedian_values, nanmedian_indices)
+ if out is not None:
+ ivy.inplace_update(out[0], result.values)
+ ivy.inplace_update(out[1], result.indices)
+ return out
+ return result
+
+
@to_ivy_arrays_and_back
@with_supported_dtypes(
- {"2.0.1 and below": ("float", "int")},
+ {"2.1.0 and below": ("float", "int")},
"torch",
)
def nansum(input, dim=None, keepdim=False, *, dtype=None):
@@ -203,7 +244,7 @@ def nansum(input, dim=None, keepdim=False, *, dtype=None):
@to_ivy_arrays_and_back
@with_supported_dtypes(
- {"2.0.1 and below": ("float", "complex")},
+ {"2.1.0 and below": ("float", "complex")},
"torch",
)
def norm(input, p="fro", dim=None, keepdim=False, out=None, dtype=None):
@@ -229,7 +270,7 @@ def norm(input, p="fro", dim=None, keepdim=False, out=None, dtype=None):
@to_ivy_arrays_and_back
@with_unsupported_dtypes(
{
- "2.0.1 and below": (
+ "2.1.0 and below": (
"float16",
"bfloat16",
)
@@ -245,7 +286,7 @@ def prod(input, dim=None, keepdim=False, *, dtype=None):
@numpy_to_torch_style_args
@to_ivy_arrays_and_back
-@with_unsupported_dtypes({"2.0.1 and below": ("float16", "bfloat16")}, "torch")
+@with_unsupported_dtypes({"2.1.0 and below": ("float16", "bfloat16")}, "torch")
def quantile(input, q, dim=None, keepdim=False, *, interpolation="linear", out=None):
return ivy.quantile(
input, q, axis=dim, keepdims=keepdim, interpolation=interpolation, out=out
@@ -254,14 +295,14 @@ def quantile(input, q, dim=None, keepdim=False, *, interpolation="linear", out=N
@numpy_to_torch_style_args
@to_ivy_arrays_and_back
-@with_unsupported_dtypes({"2.0.1 and below": ("float16",)}, "torch")
+@with_unsupported_dtypes({"2.1.0 and below": ("float16",)}, "torch")
def std(input, dim=None, unbiased=True, keepdim=False, *, out=None):
return ivy.std(input, axis=dim, correction=int(unbiased), keepdims=keepdim, out=out)
@numpy_to_torch_style_args
@to_ivy_arrays_and_back
-@with_unsupported_dtypes({"2.0.1 and below": ("bfloat16",)}, "torch")
+@with_unsupported_dtypes({"2.1.0 and below": ("bfloat16",)}, "torch")
def std_mean(input, dim, unbiased, keepdim=False, *, out=None):
temp_std = ivy.std(
input, axis=dim, correction=int(unbiased), keepdims=keepdim, out=out
@@ -306,7 +347,7 @@ def unique(input, sorted=True, return_inverse=False, return_counts=False, dim=No
@with_unsupported_dtypes(
{
- "2.0.1 and below": (
+ "2.1.0 and below": (
"float16",
"complex",
)
@@ -328,7 +369,7 @@ def unique_consecutive(input, return_inverse, return_counts, dim):
@to_ivy_arrays_and_back
@with_unsupported_dtypes(
{
- "2.0.1 and below": (
+ "2.1.0 and below": (
"float16",
"bfloat16",
)
@@ -343,7 +384,7 @@ def var(input, dim, unbiased, keepdim=False, *, out=None):
@to_ivy_arrays_and_back
@with_unsupported_dtypes(
{
- "2.0.1 and below": (
+ "2.1.0 and below": (
"float16",
"bfloat16",
)
diff --git a/ivy/functional/frontends/torch/spectral_ops.py b/ivy/functional/frontends/torch/spectral_ops.py
index fe68617bdc954..8727252e94d46 100644
--- a/ivy/functional/frontends/torch/spectral_ops.py
+++ b/ivy/functional/frontends/torch/spectral_ops.py
@@ -1,5 +1,6 @@
import ivy
from ivy.functional.frontends.torch.func_wrapper import to_ivy_arrays_and_back
+from ivy.func_wrapper import with_supported_dtypes
@to_ivy_arrays_and_back
@@ -29,3 +30,32 @@ def bartlett_window(
)
return res[:-1] if periodic else res
+
+
+@to_ivy_arrays_and_back
+@with_supported_dtypes({"2.51.0 and below": ("float32", "float64")}, "torch")
+def blackman_window(
+ window_length,
+ periodic=True,
+ *,
+ dtype=None,
+ layout=None,
+ device=None,
+ requires_grad=False
+):
+ return ivy.blackman_window(window_length, periodic=periodic, dtype=dtype)
+
+
+@to_ivy_arrays_and_back
+@with_supported_dtypes({"2.51.0 and below": ("float32", "float64")}, "torch")
+def kaiser_window(
+ window_length,
+ periodic=True,
+ beta=12.0,
+ *,
+ dtype=None,
+ layout=None,
+ device=None,
+ requires_grad=False
+):
+ return ivy.kaiser_window(window_length, periodic=periodic, beta=beta, dtype=dtype)
diff --git a/ivy/functional/frontends/torch/tensor.py b/ivy/functional/frontends/torch/tensor.py
index c430c43e74369..d430edb6105c5 100644
--- a/ivy/functional/frontends/torch/tensor.py
+++ b/ivy/functional/frontends/torch/tensor.py
@@ -21,9 +21,8 @@ class Tensor:
def __init__(self, array, device=None, _init_overload=False, requires_grad=False):
if _init_overload:
self._ivy_array = (
- ivy.array(array) if not isinstance(array, ivy.Array) else array
+ array if isinstance(array, ivy.Array) else ivy.array(array)
)
-
else:
self._ivy_array = ivy.array(
array, dtype=torch_frontend.float32, device=device
@@ -100,14 +99,24 @@ def requires_grad(self):
def is_leaf(self):
return self._is_leaf
+ @property
+ def get_device(self):
+ if self.device == "cpu":
+ return -1
+ else:
+ return int(self.device.split(":")[-1])
+
# Setters #
# --------#
+ @device.setter
+ def cuda(self, device=None):
+ self.device = device
+ return self
+
@ivy_array.setter
def ivy_array(self, array):
- self._ivy_array = (
- ivy.array(array) if not isinstance(array, ivy.Array) else array
- )
+ self._ivy_array = array if isinstance(array, ivy.Array) else ivy.array(array)
@requires_grad.setter
def requires_grad(self, requires_grad):
@@ -132,19 +141,20 @@ def reshape(self, *args, shape=None):
return torch_frontend.reshape(self, args)
return torch_frontend.reshape(self)
- @with_unsupported_dtypes({"2.0.1 and below": ("bfloat16",)}, "torch")
+ @with_unsupported_dtypes({"2.1.0 and below": ("bfloat16",)}, "torch")
+ @with_unsupported_dtypes({"2.5.1 and below": ("float16",)}, "paddle")
def reshape_as(self, other):
return torch_frontend.reshape(self, other.shape)
- @with_unsupported_dtypes({"2.0.1 and below": ("bfloat16",)}, "torch")
+ @with_unsupported_dtypes({"2.1.0 and below": ("bfloat16",)}, "torch")
def add(self, other, *, alpha=1):
return torch_frontend.add(self, other, alpha=alpha)
- # @with_unsupported_dtypes({"2.0.1 and below": ("bfloat16",)}, "torch")
+ # @with_unsupported_dtypes({"2.1.0 and below": ("bfloat16",)}, "torch")
def divide(self, other, *, out=None):
return torch_frontend.divide(self, other, out=out)
- @with_unsupported_dtypes({"2.0.1 and below": ("bfloat16",)}, "torch")
+ @with_unsupported_dtypes({"2.1.0 and below": ("bfloat16",)}, "torch")
def sub(self, other, *, alpha=1):
return torch_frontend.sub(self, other, alpha=alpha)
@@ -159,105 +169,105 @@ def any(self, dim=None, keepdim=False):
def all(self, dim=None, keepdim=False):
return torch_frontend.all(self, dim=dim, keepdim=keepdim)
- @with_unsupported_dtypes({"2.0.1 and below": ("bfloat16",)}, "torch")
+ @with_unsupported_dtypes({"2.1.0 and below": ("bfloat16",)}, "torch")
def add_(self, other, *, alpha=1):
self.ivy_array = self.add(other, alpha=alpha).ivy_array
return self
- @with_unsupported_dtypes({"2.0.1 and below": ("float16",)}, "torch")
+ @with_unsupported_dtypes({"2.1.0 and below": ("float16",)}, "torch")
def addmm(self, mat1, mat2, *, beta=1, alpha=1):
return torch_frontend.addmm(self, mat1, mat2, beta=beta, alpha=alpha)
- @with_unsupported_dtypes({"2.0.1 and below": ("float16",)}, "torch")
+ @with_unsupported_dtypes({"2.1.0 and below": ("float16",)}, "torch")
def addmm_(self, mat1, mat2, *, beta=1, alpha=1):
self.ivy_array = self.addmm(mat1, mat2, beta=beta, alpha=alpha).ivy_array
return self
- @with_unsupported_dtypes({"2.0.1 and below": ("float16",)}, "torch")
+ @with_unsupported_dtypes({"2.1.0 and below": ("float16",)}, "torch")
def addmv(self, mat, vec, *, beta=1, alpha=1):
return torch_frontend.addmv(self, mat, vec, beta=beta, alpha=alpha)
- @with_unsupported_dtypes({"2.0.1 and below": ("float16",)}, "torch")
+ @with_unsupported_dtypes({"2.1.0 and below": ("float16",)}, "torch")
def addmv_(self, mat, vec, *, beta=1, alpha=1):
self.ivy_array = torch_frontend.addmv(
self, mat, vec, beta=beta, alpha=alpha
).ivy_array
return self
- @with_unsupported_dtypes({"2.0.1 and below": ("float16",)}, "torch")
+ @with_unsupported_dtypes({"2.1.0 and below": ("float16",)}, "torch")
def addbmm(self, batch1, batch2, *, beta=1, alpha=1):
return torch_frontend.addbmm(self, batch1, batch2, beta=beta, alpha=alpha)
- @with_unsupported_dtypes({"2.0.1 and below": ("float16",)}, "torch")
+ @with_unsupported_dtypes({"2.1.0 and below": ("float16",)}, "torch")
def addbmm_(self, batch1, batch2, *, beta=1, alpha=1):
self.ivy_array = self.addbmm(batch1, batch2, beta=beta, alpha=alpha).ivy_array
return self
- @with_unsupported_dtypes({"2.0.1 and below": ("bfloat16",)}, "torch")
+ @with_unsupported_dtypes({"2.1.0 and below": ("bfloat16",)}, "torch")
def subtract_(self, other, *, alpha=1):
self.ivy_array = self.sub(other, alpha=alpha).ivy_array
return self
- @with_unsupported_dtypes({"2.0.1 and below": ("float16",)}, "torch")
+ @with_unsupported_dtypes({"2.1.0 and below": ("float16",)}, "torch")
def asin(self):
return torch_frontend.asin(self)
- @with_unsupported_dtypes({"2.0.1 and below": ("float16",)}, "torch")
+ @with_unsupported_dtypes({"2.1.0 and below": ("float16",)}, "torch")
def asin_(self):
self.ivy_array = self.asin().ivy_array
return self
@numpy_to_torch_style_args
- @with_unsupported_dtypes({"2.0.1 and below": ("bfloat16",)}, "torch")
+ @with_unsupported_dtypes({"2.1.0 and below": ("bfloat16",)}, "torch")
def sum(self, dim=None, keepdim=False, *, dtype=None):
return torch_frontend.sum(self, dim=dim, keepdim=keepdim, dtype=dtype)
- @with_unsupported_dtypes({"2.0.1 and below": ("float16",)}, "torch")
+ @with_unsupported_dtypes({"2.1.0 and below": ("float16",)}, "torch")
def sin(self):
return torch_frontend.sin(self)
- @with_unsupported_dtypes({"2.0.1 and below": ("float16",)}, "torch")
+ @with_unsupported_dtypes({"2.1.0 and below": ("float16",)}, "torch")
def sin_(self):
self.ivy_array = self.sin().ivy_array
return self
- @with_unsupported_dtypes({"2.0.1 and below": ("float16",)}, "torch")
+ @with_unsupported_dtypes({"2.1.0 and below": ("float16",)}, "torch")
def sinh(self):
return torch_frontend.sinh(self)
- @with_unsupported_dtypes({"2.0.1 and below": ("float16",)}, "torch")
+ @with_unsupported_dtypes({"2.1.0 and below": ("float16",)}, "torch")
def sinh_(self):
self.ivy_array = self.sinh().ivy_array
return self
- @with_unsupported_dtypes({"2.0.1 and below": ("float16",)}, "torch")
+ @with_unsupported_dtypes({"2.1.0 and below": ("float16",)}, "torch")
def cos(self):
return torch_frontend.cos(self)
- @with_unsupported_dtypes({"2.0.1 and below": ("float16",)}, "torch")
+ @with_unsupported_dtypes({"2.1.0 and below": ("float16",)}, "torch")
def cos_(self):
self.ivy_array = self.cos().ivy_array
return self
- @with_unsupported_dtypes({"2.0.1 and below": ("float16",)}, "torch")
+ @with_unsupported_dtypes({"2.1.0 and below": ("float16",)}, "torch")
def cosh(self):
return torch_frontend.cosh(self)
- @with_unsupported_dtypes({"2.0.1 and below": ("float16", "bfloat16")}, "torch")
+ @with_unsupported_dtypes({"2.1.0 and below": ("float16",)}, "torch")
def cosh_(self):
self.ivy_array = self.cosh().ivy_array
return self
- @with_unsupported_dtypes({"2.0.1 and below": ("float16",)}, "torch")
+ @with_unsupported_dtypes({"2.1.0 and below": ("float16",)}, "torch")
def atan(self):
return torch_frontend.atan(self)
- @with_unsupported_dtypes({"2.0.1 and below": ("float16",)}, "torch")
+ @with_unsupported_dtypes({"2.1.0 and below": ("float16",)}, "torch")
def atan_(self):
self.ivy_array = self.atan().ivy_array
return self
- @with_unsupported_dtypes({"2.0.1 and below": ("float16", "bfloat16")}, "torch")
+ @with_unsupported_dtypes({"2.1.0 and below": ("float16", "bfloat16")}, "torch")
def atan2(self, other):
return torch_frontend.atan2(self, other)
@@ -303,56 +313,56 @@ def float(self, memory_format=None):
def double(self):
return self.to(torch_frontend.float64)
- @with_unsupported_dtypes({"2.0.1 and below": ("float16",)}, "torch")
+ @with_unsupported_dtypes({"2.1.0 and below": ("float16",)}, "torch")
def asinh(self):
return torch_frontend.asinh(self)
- @with_unsupported_dtypes({"2.0.1 and below": ("float16",)}, "torch")
+ @with_unsupported_dtypes({"2.1.0 and below": ("float16",)}, "torch")
def asinh_(self):
self.ivy_array = self.asinh().ivy_array
return self
- @with_unsupported_dtypes({"2.0.1 and below": ("float16",)}, "torch")
+ @with_unsupported_dtypes({"2.1.0 and below": ("float16",)}, "torch")
def tan(self):
return torch_frontend.tan(self)
- @with_unsupported_dtypes({"2.0.1 and below": ("float16",)}, "torch")
+ @with_unsupported_dtypes({"2.1.0 and below": ("float16",)}, "torch")
def tan_(self):
self.ivy_array = self.tan().ivy_array
return self
- @with_unsupported_dtypes({"2.0.1 and below": ("float16",)}, "torch")
+ @with_unsupported_dtypes({"2.1.0 and below": ("float16",)}, "torch")
def tanh(self):
return torch_frontend.tanh(self)
- @with_unsupported_dtypes({"2.0.1 and below": ("float16",)}, "torch")
+ @with_unsupported_dtypes({"2.1.0 and below": ("float16",)}, "torch")
def tanh_(self):
self.ivy_array = self.tanh().ivy_array
return self
- @with_unsupported_dtypes({"2.0.1 and below": ("float16",)}, "torch")
+ @with_unsupported_dtypes({"2.1.0 and below": ("float16",)}, "torch")
def atanh(self):
return torch_frontend.atanh(self)
- @with_unsupported_dtypes({"2.0.1 and below": ("float16",)}, "torch")
+ @with_unsupported_dtypes({"2.1.0 and below": ("float16",)}, "torch")
def atanh_(self):
self.ivy_array = self.atanh().ivy_array
return self
- @with_unsupported_dtypes({"2.0.1 and below": ("float16",)}, "torch")
+ @with_unsupported_dtypes({"2.1.0 and below": ("float16",)}, "torch")
def log(self):
return torch_frontend.log(self)
- @with_supported_dtypes({"2.0.1 and below": ("float32", "float64")}, "torch")
+ @with_supported_dtypes({"2.1.0 and below": ("float32", "float64")}, "torch")
def log2_(self):
self.ivy_array = self.log2().ivy_array
return self
- @with_unsupported_dtypes({"2.0.1 and below": ("float16", "bfloat16")}, "torch")
+ @with_unsupported_dtypes({"2.1.0 and below": ("float16", "bfloat16")}, "torch")
def logit(self):
return torch_frontend.logit(self)
- @with_unsupported_dtypes({"2.0.1 and below": ("bfloat16", "uint16")}, "torch")
+ @with_unsupported_dtypes({"2.1.0 and below": ("bfloat16", "uint16")}, "torch")
def copy_(self, other, non_blocking=False):
ivy.utils.assertions.check_one_way_broadcastable(
self.ivy_array.shape, torch_frontend.tensor(other).ivy_array.shape
@@ -360,31 +370,31 @@ def copy_(self, other, non_blocking=False):
self._ivy_array = torch_frontend.tensor(other).ivy_array
return self
- @with_unsupported_dtypes({"2.0.1 and below": ("float16",)}, "torch")
+ @with_unsupported_dtypes({"2.1.0 and below": ("float16",)}, "torch")
def log_(self):
self.ivy_array = self.log().ivy_array
return self
- @with_unsupported_dtypes({"2.0.1 and below": ("float16",)}, "torch")
+ @with_unsupported_dtypes({"2.1.0 and below": ("float16",)}, "torch")
def log2(self):
return torch_frontend.log2(self)
- @with_unsupported_dtypes({"2.0.1 and below": ("float16", "bfloat16")}, "torch")
+ @with_unsupported_dtypes({"2.1.0 and below": ("float16", "bfloat16")}, "torch")
def relu(self):
return torch_frontend_nn.relu(self)
@numpy_to_torch_style_args
- @with_unsupported_dtypes({"2.0.1 and below": ("complex",)}, "torch")
+ @with_unsupported_dtypes({"2.1.0 and below": ("complex",)}, "torch")
def amax(self, dim=None, keepdim=False):
return torch_frontend.amax(self, dim=dim, keepdim=keepdim)
@numpy_to_torch_style_args
- @with_unsupported_dtypes({"2.0.1 and below": ("complex",)}, "torch")
+ @with_unsupported_dtypes({"2.1.0 and below": ("complex",)}, "torch")
def amin(self, dim=None, keepdim=False):
return torch_frontend.amin(self, dim=dim, keepdim=keepdim)
@numpy_to_torch_style_args
- @with_unsupported_dtypes({"2.0.1 and below": ("complex", "float16")}, "torch")
+ @with_unsupported_dtypes({"2.1.0 and below": ("complex", "float16")}, "torch")
def aminmax(self, dim=None, keepdim=False):
return torch_frontend.aminmax(self, dim=dim, keepdim=keepdim)
@@ -395,7 +405,7 @@ def abs_(self):
self.ivy_array = self.abs().ivy_array
return self
- @with_unsupported_dtypes({"2.0.1 and below": ("bfloat16",)}, "torch")
+ @with_unsupported_dtypes({"2.1.0 and below": ("bfloat16",)}, "torch")
def logical_and(self, other):
return torch_frontend.logical_and(self, other)
@@ -406,7 +416,7 @@ def logical_not_(self):
self.ivy_array = ivy.astype(self.logical_not().ivy_array, self.dtype)
return self
- @with_unsupported_dtypes({"2.0.1 and below": ("bfloat16",)}, "torch")
+ @with_unsupported_dtypes({"2.1.0 and below": ("bfloat16",)}, "torch")
def logical_or(self, other):
return torch_frontend.logical_or(self, other)
@@ -416,14 +426,14 @@ def bitwise_not(self):
def bitwise_and(self, other):
return torch_frontend.bitwise_and(self, other)
- @with_supported_dtypes({"2.0.1 and below": ("integer",)}, "torch")
+ @with_supported_dtypes({"2.1.0 and below": ("integer",)}, "torch")
def bitwise_or(self, other):
return torch_frontend.bitwise_or(self, other)
def bitwise_left_shift(self, other):
return torch_frontend.bitwise_left_shift(self, other)
- @with_supported_dtypes({"2.0.1 and below": ("integer",)}, "torch")
+ @with_supported_dtypes({"2.1.0 and below": ("integer",)}, "torch")
def bitwise_or_(self, other):
self.ivy_array = self.bitwise_or(other).ivy_array
return self
@@ -451,23 +461,54 @@ def new_ones(
size, dtype=dtype, device=device, requires_grad=requires_grad
)
- @with_unsupported_dtypes({"2.0.1 and below": ("float16",)}, "torch")
+ @with_unsupported_dtypes({"2.1.0 and below": ("float16",)}, "torch")
def floor(self, *, out=None):
return torch_frontend.floor(self)
- @with_unsupported_dtypes({"2.0.1 and below": ("float16", "bfloat16")}, "torch")
+ @with_unsupported_dtypes(
+ {
+ "2.1.0 and below": (
+ "bfloat16",
+ "uint8",
+ "uint32",
+ "uint16",
+ "uint64",
+ "complex128",
+ "complex64",
+ )
+ },
+ "torch",
+ )
def not_equal(self, other, *, out=None):
return torch_frontend.not_equal(self, other, out=out)
+ @with_unsupported_dtypes(
+ {
+ "2.1.0 and below": (
+ "bfloat16",
+ "uint8",
+ "uint32",
+ "uint16",
+ "uint64",
+ "complex128",
+ "complex64",
+ )
+ },
+ "torch",
+ )
+ def not_equal_(self, other, *, out=None):
+ self.ivy_array = self.not_equal(other).ivy_array
+ return self
+
def equal(self, other):
return torch_frontend.equal(self, other)
- @with_unsupported_dtypes({"2.0.1 and below": ("float16", "complex")}, "torch")
+ @with_unsupported_dtypes({"2.1.0 and below": ("float16", "complex")}, "torch")
def erf(self, *, out=None):
return torch_frontend.erf(self, out=out)
@with_supported_dtypes(
- {"2.0.1 and below": ("float32", "float64", "bfloat16")}, "torch"
+ {"2.1.0 and below": ("float32", "float64", "bfloat16")}, "torch"
)
def erf_(self, *, out=None):
self.ivy_array = self.erf(out=out).ivy_array
@@ -567,11 +608,11 @@ def to(self, *args, **kwargs):
)
return cast_tensor
- @with_unsupported_dtypes({"2.0.1 and below": ("float16",)}, "torch")
+ @with_unsupported_dtypes({"2.1.0 and below": ("float16",)}, "torch")
def acos(self):
return torch_frontend.acos(self)
- @with_unsupported_dtypes({"2.0.1 and below": ("float16", "bfloat16")}, "torch")
+ @with_unsupported_dtypes({"2.1.0 and below": ("float16",)}, "torch")
def acos_(self):
self.ivy_array = self.acos().ivy_array
return self
@@ -591,7 +632,7 @@ def new_tensor(
_data = ivy.asarray(data, copy=True, dtype=dtype, device=device)
return torch_frontend.tensor(_data)
- @with_unsupported_dtypes({"2.0.1 and below": ("bfloat16",)}, "torch")
+ @with_unsupported_dtypes({"2.1.0 and below": ("bfloat16",)}, "torch")
def view_as(self, other):
return self.view(size=other.shape)
@@ -620,7 +661,7 @@ def detach_(self):
self.ivy_array = self.detach().ivy_array
return self
- @with_unsupported_dtypes({"2.0.1 and below": ("bfloat16", "uint16")}, "torch")
+ @with_unsupported_dtypes({"2.1.0 and below": ("uint16",)}, "torch")
@numpy_to_torch_style_args
def unsqueeze(self, dim):
return torch_frontend.unsqueeze(self, dim)
@@ -708,7 +749,7 @@ def max(self, dim=None, keepdim=False):
@with_unsupported_dtypes(
{
- "2.0.1 and below": (
+ "2.1.0 and below": (
"complex",
"bfloat16",
"bool",
@@ -734,11 +775,15 @@ def is_cuda(self):
def is_meta(self):
return "meta" in ivy.dev(self.ivy_array)
- @with_unsupported_dtypes({"2.0.1 and below": ("bfloat16",)}, "torch")
+ @with_unsupported_dtypes({"2.1.0 and below": ("uint16", "bool")}, "torch")
+ def positive(self):
+ return torch_frontend.positive(self)
+
+ @with_unsupported_dtypes({"2.1.0 and below": ("bfloat16",)}, "torch")
def pow(self, exponent):
return torch_frontend.pow(self, exponent)
- @with_unsupported_dtypes({"2.0.1 and below": ("bfloat16",)}, "torch")
+ @with_unsupported_dtypes({"2.1.0 and below": ("bfloat16",)}, "torch")
def pow_(self, exponent):
self.ivy_array = self.pow(exponent).ivy_array
return self
@@ -747,36 +792,41 @@ def size(self, dim=None):
shape = self.shape
if dim is None:
return shape
- else:
- try:
- return shape[dim]
- except IndexError:
- raise IndexError(
- "Dimension out of range (expected to be in range of [{}, {}], "
- "but got {}".format(len(shape), len(shape) - 1, dim)
- )
+ try:
+ return shape[dim]
+ except IndexError:
+ raise IndexError(
+ f"Dimension out of range (expected to be in range of [{len(shape)},"
+ f" {len(shape) - 1}], but got {dim}"
+ )
def matmul(self, other):
return torch_frontend.matmul(self, other)
+ @with_supported_dtypes(
+ {"2.1.0 and below": ("float32", "float64", "complex32", "complex64")}, "torch"
+ )
+ def matrix_power(self, n, *, out=None):
+ return torch_frontend.linalg.matrix_power(self, n, out=out)
+
def argwhere(self):
return torch_frontend.argwhere(self)
@numpy_to_torch_style_args
- @with_unsupported_dtypes({"2.0.1 and below": ("complex",)}, "torch")
+ @with_unsupported_dtypes({"2.1.0 and below": ("complex", "bool")}, "torch")
def argmax(self, dim=None, keepdim=False):
return torch_frontend.argmax(self, dim=dim, keepdim=keepdim)
@numpy_to_torch_style_args
- @with_unsupported_dtypes({"2.0.1 and below": ("complex",)}, "torch")
+ @with_unsupported_dtypes({"2.1.0 and below": ("complex",)}, "torch")
def argmin(self, dim=None, keepdim=False):
return torch_frontend.argmin(self, dim=dim, keepdim=keepdim)
- @with_unsupported_dtypes({"2.0.1 and below": ("complex",)}, "torch")
+ @with_unsupported_dtypes({"2.1.0 and below": ("complex",)}, "torch")
def argsort(self, dim=-1, descending=False):
return torch_frontend.argsort(self, dim=dim, descending=descending)
- @with_unsupported_dtypes({"2.0.1 and below": ("float16",)}, "torch")
+ @with_unsupported_dtypes({"2.1.0 and below": ("float16",)}, "torch")
def ceil(self):
return torch_frontend.ceil(self)
@@ -798,22 +848,22 @@ def permute(self, *args, dims=None):
return torch_frontend.permute(self)
@numpy_to_torch_style_args
- @with_unsupported_dtypes({"2.0.1 and below": ("float16", "bfloat16")}, "torch")
+ @with_unsupported_dtypes({"2.1.0 and below": ("float16", "bfloat16")}, "torch")
def mean(self, dim=None, keepdim=False):
return torch_frontend.mean(self, dim=dim, keepdim=keepdim)
- @with_unsupported_dtypes({"2.0.1 and below": ("bfloat16",)}, "torch")
+ @with_unsupported_dtypes({"2.1.0 and below": ("bfloat16",)}, "torch")
@numpy_to_torch_style_args
def nanmean(self, dim=None, keepdim=False):
return torch_frontend.nanmean(self, dim=dim, keepdim=keepdim)
- @with_unsupported_dtypes({"2.0.1 and below": ("bfloat16",)}, "torch")
+ @with_unsupported_dtypes({"2.1.0 and below": ("bfloat16",)}, "torch")
@numpy_to_torch_style_args
def nansum(self, dim=None, keepdim=False):
return torch_frontend.nansum(self, dim=dim, keepdim=keepdim)
@numpy_to_torch_style_args
- @with_unsupported_dtypes({"2.0.1 and below": ("float16",)}, "torch")
+ @with_unsupported_dtypes({"2.1.0 and below": ("float16",)}, "torch")
def median(self, dim=None, keepdim=False):
return torch_frontend.median(self, dim=dim, keepdim=keepdim)
@@ -831,32 +881,32 @@ def flatten(self, start_dim=0, end_dim=-1):
return torch_frontend.flatten(self, start_dim, end_dim)
@numpy_to_torch_style_args
- @with_unsupported_dtypes({"2.0.1 and below": ("float16",)}, "torch")
+ @with_unsupported_dtypes({"2.1.0 and below": ("float16",)}, "torch")
def cumsum(self, dim, *, dtype=None):
return torch_frontend.cumsum(self, dim, dtype=dtype)
@numpy_to_torch_style_args
- @with_unsupported_dtypes({"2.0.1 and below": ("float16",)}, "torch")
+ @with_unsupported_dtypes({"2.1.0 and below": ("float16",)}, "torch")
def cumsum_(self, dim, *, dtype=None):
- self.ivy_array = self.cumsum(dim, dtype).ivy_array
+ self.ivy_array = self.cumsum(dim, dtype=dtype).ivy_array
return self
- @with_unsupported_dtypes({"2.0.1 and below": ("float16", "bfloat16")}, "torch")
+ @with_unsupported_dtypes({"2.1.0 and below": ("float16", "bfloat16")}, "torch")
def inverse(self):
return torch_frontend.inverse(self)
- @with_unsupported_dtypes({"2.0.1 and below": ("bool", "bfloat16")}, "torch")
+ @with_unsupported_dtypes({"2.1.0 and below": ("bool", "bfloat16")}, "torch")
def neg(self):
return torch_frontend.negative(self)
- @with_unsupported_dtypes({"2.0.1 and below": ("bool",)}, "torch")
+ @with_unsupported_dtypes({"2.1.0 and below": ("bool",)}, "torch")
def neg_(self):
self.ivy_array = torch_frontend.negative(self).ivy_array
return self
__neg__ = neg
- @with_unsupported_dtypes({"2.0.1 and below": ("bool", "bfloat16")}, "torch")
+ @with_unsupported_dtypes({"2.1.0 and below": ("bool", "bfloat16")}, "torch")
def negative(self):
return torch_frontend.negative(self)
@@ -879,7 +929,7 @@ def type(self, dtype=None, non_blocking=False, **kwargs):
else:
return str(self.dtype)
- @with_unsupported_dtypes({"2.0.1 and below": ("bfloat16",)}, "torch")
+ @with_unsupported_dtypes({"2.1.0 and below": ("bfloat16",)}, "torch")
def type_as(self, other):
if self.dtype != other.dtype:
self.ivy_array = ivy.astype(self.ivy_array, other.dtype)
@@ -894,7 +944,7 @@ def squeeze(self, dim=None):
return torch_frontend.squeeze(self, dim)
@numpy_to_torch_style_args
- @with_unsupported_dtypes({"2.0.1 and below": ("bfloat16", "uint16")}, "torch")
+ @with_unsupported_dtypes({"2.1.0 and below": ("uint16",)}, "torch")
def squeeze_(self, dim=None):
self.ivy_array = self.squeeze(dim).ivy_array
return self
@@ -918,35 +968,35 @@ def tril_(self, diagonal=0):
def index_select(self, dim, index):
return torch_frontend.index_select(self, dim, index)
- @with_unsupported_dtypes({"2.0.1 and below": ("float16", "complex")}, "torch")
+ @with_unsupported_dtypes({"2.1.0 and below": ("float16", "complex")}, "torch")
def clamp(self, min=None, max=None):
return torch_frontend.clamp(self, min=min, max=max)
- @with_unsupported_dtypes({"2.0.1 and below": ("float16", "complex")}, "torch")
+ @with_unsupported_dtypes({"2.1.0 and below": ("float16", "complex")}, "torch")
def clamp_(self, min=None, max=None):
self.ivy_array = self.clamp(min=min, max=max).ivy_array
return self
@with_unsupported_dtypes(
- {"2.0.1 and below": ("bool", "bfloat16", "float16", "complex")}, "torch"
+ {"2.1.0 and below": ("bool", "bfloat16", "float16", "complex")}, "torch"
)
def clamp_min(self, min=None):
return torch_frontend.clamp(self, min=min)
- @with_unsupported_dtypes({"2.0.1 and below": ("float16", "bfloat16")}, "torch")
+ @with_unsupported_dtypes({"2.1.0 and below": ("float16", "bfloat16")}, "torch")
def sqrt(self):
return torch_frontend.sqrt(self)
- @with_unsupported_dtypes({"2.0.1 and below": ("float16",)}, "torch")
+ @with_unsupported_dtypes({"2.1.0 and below": ("float16",)}, "torch")
def rsqrt(self):
return torch_frontend.rsqrt(self)
- @with_unsupported_dtypes({"2.0.1 and below": ("float16", "bfloat16")}, "torch")
+ @with_unsupported_dtypes({"2.1.0 and below": ("float16", "bfloat16")}, "torch")
def rsqrt_(self):
self.ivy_array = self.rsqrt().ivy_array
return self
- @with_unsupported_dtypes({"2.0.1 and below": ("float16", "bfloat16")}, "torch")
+ @with_unsupported_dtypes({"2.1.0 and below": ("float16", "bfloat16")}, "torch")
def sqrt_(self):
self.ivy_array = self.sqrt().ivy_array
return self
@@ -957,7 +1007,7 @@ def where(self, condition, other):
def clone(self, memory_format=None):
return torch_frontend.tensor(ivy.array(self.ivy_array, copy=True))
- @with_unsupported_dtypes({"2.0.1 and below": ("float16",)}, "torch")
+ @with_unsupported_dtypes({"2.1.0 and below": ("float16",)}, "torch")
def acosh(self):
return torch_frontend.acosh(self)
@@ -970,38 +1020,38 @@ def masked_fill_(self, mask, value):
self.ivy_array = self.masked_fill(mask, value).ivy_array
return self
- @with_unsupported_dtypes({"2.0.1 and below": ("float16", "bfloat16")}, "torch")
+ @with_unsupported_dtypes({"2.1.0 and below": ("float16", "bfloat16")}, "torch")
def index_add_(self, dim, index, source, *, alpha=1):
self.ivy_array = torch_frontend.index_add(
self, dim, index, source, alpha=alpha
).ivy_array
return self
- @with_unsupported_dtypes({"2.0.1 and below": ("float16", "bfloat16")}, "torch")
+ @with_unsupported_dtypes({"2.1.0 and below": ("float16", "bfloat16")}, "torch")
def index_add(self, dim, index, source, *, alpha=1):
return torch_frontend.index_add(
self._ivy_array, dim, index, source, alpha=alpha
)
- @with_unsupported_dtypes({"2.0.1 and below": ("float16",)}, "torch")
+ @with_unsupported_dtypes({"2.1.0 and below": ("float16",)}, "torch")
def acosh_(self):
self.ivy_array = self.acosh().ivy_array
return self
- @with_unsupported_dtypes({"2.0.1 and below": ("bfloat16",)}, "torch")
+ @with_unsupported_dtypes({"2.1.0 and below": ("bfloat16",)}, "torch")
def numpy(self):
return np_frontend_array(self.ivy_array)
- @with_unsupported_dtypes({"2.0.1 and below": ("float16",)}, "torch")
+ @with_unsupported_dtypes({"2.1.0 and below": ("float16",)}, "torch")
def sigmoid(self):
return torch_frontend.sigmoid(self)
- @with_unsupported_dtypes({"2.0.1 and below": ("float16", "bfloat16")}, "torch")
+ @with_unsupported_dtypes({"2.1.0 and below": ("float16",)}, "torch")
def sigmoid_(self):
self.ivy_array = self.sigmoid().ivy_array
return self
- @with_unsupported_dtypes({"2.0.1 and below": ("float16",)}, "torch")
+ @with_unsupported_dtypes({"2.1.0 and below": ("float16",)}, "torch")
def softmax(self, dim=None, dtype=None):
return torch_frontend.nn.functional.softmax(self, dim=dim, dtype=dtype)
@@ -1033,7 +1083,7 @@ def remainder(self, other, *, out=None):
return torch_frontend.remainder(self, other, out=out)
@with_supported_dtypes(
- {"2.0.1 and below": ("float16", "float32", "float64", "bfloat16")}, "torch"
+ {"2.1.0 and below": ("float16", "float32", "float64", "bfloat16")}, "torch"
)
def reciprocal_(self):
self.ivy_array = torch_frontend.reciprocal(self).ivy_array
@@ -1051,12 +1101,12 @@ def bitwise_and_(self, other):
self.ivy_array = self.bitwise_and(other).ivy_array
return self
- @with_unsupported_dtypes({"2.0.1 and below": ("float16", "bfloat16")}, "torch")
+ @with_unsupported_dtypes({"2.1.0 and below": ("float16", "bfloat16")}, "torch")
def atan2_(self, other):
self.ivy_array = self.atan2(other).ivy_array
return self
- @with_unsupported_dtypes({"2.0.1 and below": ("bfloat16",)}, "torch")
+ @with_unsupported_dtypes({"2.1.0 and below": ("bfloat16",)}, "torch")
def fmax(self, other):
return torch_frontend.fmax(self, other)
@@ -1066,24 +1116,20 @@ def fmin(self, other):
def msort(self):
return torch_frontend.msort(self)
- @with_unsupported_dtypes(
- {"2.0.1 and below": ("float16", "bfloat16", "complex")}, "torch"
- )
+ @with_unsupported_dtypes({"2.1.0 and below": ("float16", "complex")}, "torch")
def trunc(self):
return torch_frontend.trunc(self)
- @with_unsupported_dtypes({"2.0.1 and below": ("float16", "complex")}, "torch")
+ @with_unsupported_dtypes({"2.1.0 and below": ("float16", "complex")}, "torch")
def trunc_(self):
self.ivy_array = self.trunc().ivy_array
return self
- @with_unsupported_dtypes({"2.0.1 and below": ("float16", "complex")}, "torch")
+ @with_unsupported_dtypes({"2.1.0 and below": ("float16", "complex")}, "torch")
def fix(self):
return torch_frontend.fix(self)
- @with_unsupported_dtypes(
- {"2.0.1 and below": ("float16", "bfloat16", "complex")}, "torch"
- )
+ @with_unsupported_dtypes({"2.1.0 and below": ("float16", "complex")}, "torch")
def fix_(self):
self.ivy_array = self.fix().ivy_array
return self
@@ -1094,7 +1140,11 @@ def isinf(self):
def is_complex(self):
return torch_frontend.is_complex(self._ivy_array)
- @with_unsupported_dtypes({"2.0.1 and below": ("bfloat16",)}, "torch")
+ @with_unsupported_dtypes({"2.1.0 and below": ("uint16", "bfloat16")}, "torch")
+ def is_floating_point(self):
+ return torch_frontend.is_floating_point(self._ivy_array)
+
+ @with_unsupported_dtypes({"2.1.0 and below": ("bfloat16",)}, "torch")
def isreal(self):
return torch_frontend.isreal(self._ivy_array)
@@ -1105,11 +1155,11 @@ def addr_(self, vec1, vec2, *, beta=1, alpha=1):
self.ivy_array = self.addr(vec1, vec2, beta=beta, alpha=alpha).ivy_array
return self
- @with_unsupported_dtypes({"2.0.1 and below": ("float16", "bfloat16")}, "torch")
+ @with_unsupported_dtypes({"2.1.0 and below": ("float16", "bfloat16")}, "torch")
def dot(self, tensor):
return torch_frontend.dot(self, tensor)
- @with_supported_dtypes({"2.0.1 and below": ("float32", "float64")}, "torch")
+ @with_supported_dtypes({"2.1.0 and below": ("float32", "float64")}, "torch")
def bernoulli(self, *, generator=None, out=None):
return torch_frontend.bernoulli(self._ivy_array, generator=generator, out=out)
@@ -1124,19 +1174,19 @@ def __bool__(self):
"Use a.any() or a.all()"
)
- @with_unsupported_dtypes({"2.0.1 and below": ("bfloat16",)}, "torch")
+ @with_unsupported_dtypes({"2.1.0 and below": ("bfloat16",)}, "torch")
def __add__(self, other):
return torch_frontend.add(self, other)
- @with_unsupported_dtypes({"2.0.1 and below": ("bfloat16",)}, "torch")
+ @with_unsupported_dtypes({"2.1.0 and below": ("bfloat16",)}, "torch")
def __mod__(self, other):
return torch_frontend.remainder(self, other)
- @with_unsupported_dtypes({"2.0.1 and below": ("bfloat16",)}, "torch")
+ @with_unsupported_dtypes({"2.1.0 and below": ("bfloat16",)}, "torch")
def __pow__(self, exponent):
return self.pow(exponent)
- @with_unsupported_dtypes({"2.0.1 and below": ("bfloat16",)}, "torch")
+ @with_unsupported_dtypes({"2.1.0 and below": ("bfloat16",)}, "torch")
def __rpow__(self, other):
return torch_frontend.pow(other, self)
@@ -1158,30 +1208,30 @@ def __iter__(self):
for i in range(self.shape[0]):
yield self[i]
- @with_unsupported_dtypes({"2.0.1 and below": ("bfloat16",)}, "torch")
+ @with_unsupported_dtypes({"2.1.0 and below": ("bfloat16",)}, "torch")
def __radd__(self, other):
return torch_frontend.add(other, self)
- @with_unsupported_dtypes({"2.0.1 and below": ("bfloat16",)}, "torch")
+ @with_unsupported_dtypes({"2.1.0 and below": ("bfloat16",)}, "torch")
def __mul__(self, other):
return torch_frontend.mul(self, other)
- @with_unsupported_dtypes({"2.0.1 and below": "bfloat16"}, "torch")
+ @with_unsupported_dtypes({"2.1.0 and below": "bfloat16"}, "torch")
def __matmul__(self, other):
return torch_frontend.matmul(self, other)
- @with_unsupported_dtypes({"2.0.1 and below": ("bfloat16",)}, "torch")
+ @with_unsupported_dtypes({"2.1.0 and below": ("bfloat16",)}, "torch")
def __rmul__(self, other):
return torch_frontend.mul(other, self)
- @with_unsupported_dtypes({"2.0.1 and below": ("bfloat16",)}, "torch")
+ @with_unsupported_dtypes({"2.1.0 and below": ("bfloat16",)}, "torch")
def __sub__(self, other):
return torch_frontend.subtract(self, other)
def __truediv__(self, other):
return torch_frontend.div(self, other)
- @with_unsupported_dtypes({"2.0.1 and below": ("float16", "complex")}, "torch")
+ @with_unsupported_dtypes({"2.1.0 and below": ("float16", "complex")}, "torch")
def __floordiv__(self, other):
return torch_frontend.floor_divide(self, other)
@@ -1236,38 +1286,39 @@ def __float__(self):
item = item.real
return float(item)
- @with_unsupported_dtypes({"2.0.1 and below": ("bfloat16",)}, "torch")
+ @with_unsupported_dtypes({"2.1.0 and below": ("bfloat16",)}, "torch")
def __eq__(self, other):
return torch_frontend.eq(self, other)
- @with_unsupported_dtypes({"2.0.1 and below": ("bfloat16",)}, "torch")
+ @with_unsupported_dtypes({"2.1.0 and below": ("complex",)}, "torch")
def __gt__(self, other):
return torch_frontend.greater(self, other)
- @with_unsupported_dtypes({"2.0.1 and below": ("bfloat16",)}, "torch")
+ @with_unsupported_dtypes({"2.1.0 and below": ("bfloat16",)}, "torch")
def __ge__(self, other):
return torch_frontend.greater_equal(self, other)
- @with_unsupported_dtypes({"2.0.1 and below": ("bfloat16",)}, "torch")
+ @with_unsupported_dtypes({"2.1.0 and below": ("bfloat16",)}, "torch")
def __ne__(self, other):
return self.ne(other)
- @with_unsupported_dtypes({"2.0.1 and below": ("bfloat16",)}, "torch")
+ @with_unsupported_dtypes({"2.1.0 and below": ("bfloat16",)}, "torch")
def __rsub__(self, other):
return torch_frontend.subtract(other, self)
- @with_unsupported_dtypes({"2.0.1 and below": ("bfloat16",)}, "torch")
+ @with_unsupported_dtypes({"2.1.0 and below": ("bfloat16",)}, "torch")
def __lt__(self, other):
return torch_frontend.less(self, other)
- @with_unsupported_dtypes({"2.0.1 and below": ("bfloat16",)}, "torch")
+ @with_unsupported_dtypes({"2.1.0 and below": ("bfloat16",)}, "torch")
def __le__(self, other):
return torch_frontend.less_equal(self, other)
- @with_unsupported_dtypes({"2.0.1 and below": ("bfloat16",)}, "torch")
+ @with_unsupported_dtypes({"2.1.0 and below": ("bfloat16",)}, "torch")
def __or__(self, other):
return torch_frontend.bitwise_or(self, other)
+ @with_supported_dtypes({"2.1.0 and below": ("integer", "bool")}, "torch")
def __invert__(self):
return torch_frontend.bitwise_not(self)
@@ -1301,7 +1352,7 @@ def item(self):
)
@numpy_to_torch_style_args
- @with_unsupported_dtypes({"2.0.1 and below": ("float16",)}, "torch")
+ @with_unsupported_dtypes({"2.1.0 and below": ("float16",)}, "torch")
def cumprod(self, dim, dtype):
return torch_frontend.cumprod(self, dim, dtype=dtype)
@@ -1309,26 +1360,31 @@ def cumprod(self, dim, dtype):
def count_nonzero(self, dim):
return torch_frontend.count_nonzero(self, dim=dim)
- @with_unsupported_dtypes({"2.0.1 and below": ("bfloat16", "float16")}, "torch")
+ def cov(self, /, *, correction=1, fweights=None, aweights=None):
+ return torch_frontend.cov(
+ self, correction=correction, fweights=fweights, aweights=aweights
+ )
+
+ @with_unsupported_dtypes({"2.1.0 and below": ("bfloat16", "float16")}, "torch")
def exp(self):
return torch_frontend.exp(self)
@with_unsupported_dtypes(
- {"2.0.1 and below": ("bfloat16", "float16", "complex")}, "torch"
+ {"2.1.0 and below": ("bfloat16", "float16", "complex")}, "torch"
)
def expm1(self):
return torch_frontend.expm1(self)
# remove "bfloat16" from the below decorator after fixing ivy.Array.__repr__ method
@with_unsupported_dtypes(
- {"2.0.1 and below": ("bfloat16", "float16", "complex")}, "torch"
+ {"2.1.0 and below": ("bfloat16", "float16", "complex")}, "torch"
)
def expm1_(self):
self.ivy_array = torch_frontend.expm1(self).ivy_array
return self
# fmt: off
- @with_unsupported_dtypes({"2.0.1 and below": ("int8", "int16", "int32", "int64", "uint8", "bool", "float16",)},"torch",) # noqa
+ @with_unsupported_dtypes({"2.1.0 and below": ("int8", "int16", "int32", "int64", "uint8", "bool", "float16",)},"torch",) # noqa
def exp_(self):
self.ivy_array = self.exp().ivy_array
return self
@@ -1337,33 +1393,33 @@ def exp_(self):
def mul(self, other):
return torch_frontend.mul(self, other)
- @with_unsupported_dtypes({"2.0.1 and below": ("float16",)}, "torch")
+ @with_unsupported_dtypes({"2.1.0 and below": ("float16",)}, "torch")
def ceil_(self):
self.ivy_array = torch_frontend.ceil(self).ivy_array
return self
- @with_unsupported_dtypes({"2.0.1 and below": ("bfloat16",)}, "torch")
+ @with_unsupported_dtypes({"2.1.0 and below": ("bfloat16",)}, "torch")
def mul_(self, other):
self.ivy_array = self.mul(other).ivy_array
# the return dtype is the same as the input dtype
self.ivy_array = self.to(self.dtype).ivy_array
return self
- @with_unsupported_dtypes({"2.0.1 and below": ("bfloat16", "float16")}, "torch")
+ @with_unsupported_dtypes({"2.1.0 and below": ("bfloat16", "float16")}, "torch")
def round(self, *, decimals=0):
return torch_frontend.round(self, decimals=decimals)
- @with_unsupported_dtypes({"2.0.1 and below": ("bfloat16", "float16")}, "torch")
+ @with_unsupported_dtypes({"2.1.0 and below": ("bfloat16", "float16")}, "torch")
def round_(self, *, decimals=0):
self.ivy_array = self.round(decimals=decimals).ivy_array
return self
@numpy_to_torch_style_args
- @with_unsupported_dtypes({"2.0.1 and below": ("float16", "complex")}, "torch")
+ @with_unsupported_dtypes({"2.1.0 and below": ("float16", "complex")}, "torch")
def cross(self, other, dim=-1):
return torch_frontend.cross(self, other, dim=dim)
- @with_unsupported_dtypes({"2.0.1 and below": ("float16", "bfloat16")}, "torch")
+ @with_unsupported_dtypes({"2.1.0 and below": ("float16", "bfloat16")}, "torch")
def det(self):
return torch_frontend.det(self)
@@ -1382,20 +1438,41 @@ def nonzero(self, as_tuple=False):
def mm(self, mat2):
return torch_frontend.mm(self, mat2)
- @with_unsupported_dtypes({"2.0.1 and below": ("bfloat16", "float16")}, "torch")
+ @with_unsupported_dtypes({"2.1.0 and below": ("bfloat16", "float16")}, "torch")
def square(self):
return torch_frontend.square(self._ivy_array)
- @with_unsupported_dtypes({"2.0.1 and below": ("float16", "bfloat16")}, "torch")
+ @with_supported_dtypes(
+ {
+ "2.1.0 and below": (
+ "float16",
+ "float32",
+ "float64",
+ "int16",
+ "int32",
+ "int64",
+ "uint8",
+ "int8",
+ "complex64",
+ "complex128",
+ )
+ },
+ "torch",
+ )
+ def square_(self):
+ self.ivy_array = torch_frontend.square(self._ivy_array).ivy_array
+ return self
+
+ @with_unsupported_dtypes({"2.1.0 and below": ("float16",)}, "torch")
def log10(self):
return torch_frontend.log10(self._ivy_array)
- @with_unsupported_dtypes({"2.0.1 and below": ("float16",)}, "torch")
+ @with_unsupported_dtypes({"2.1.0 and below": ("float16",)}, "torch")
def log10_(self):
self.ivy_array = self.log10().ivy_array
return self
- @with_unsupported_dtypes({"2.0.1 and below": ("bfloat16", "uint16")}, "torch")
+ @with_unsupported_dtypes({"2.1.0 and below": ("uint16",)}, "torch")
def zero_(self):
self.ivy_array = torch_frontend.zeros_like(self).ivy_array
return self
@@ -1405,7 +1482,7 @@ def short(self, memory_format=None):
return self
@numpy_to_torch_style_args
- @with_unsupported_dtypes({"2.0.1 and below": ("float16", "bfloat16")}, "torch")
+ @with_unsupported_dtypes({"2.1.0 and below": ("float16", "bfloat16")}, "torch")
def prod(self, dim=None, keepdim=False, *, dtype=None):
return torch_frontend.prod(self, dim=dim, keepdim=keepdim, dtype=dtype)
@@ -1417,7 +1494,7 @@ def div_(self, other, *, rounding_mode=None):
return self
@with_supported_dtypes(
- {"2.0.1 and below": ("float16", "float32", "float64", "bfloat16")}, "torch"
+ {"2.1.0 and below": ("float16", "float32", "float64", "bfloat16")}, "torch"
)
def true_divide_(self, other):
self.ivy_array = self.div(other, rounding_mode=None).ivy_array
@@ -1433,26 +1510,26 @@ def normal_(self, mean=0, std=1, *, generator=None):
)
return self
- @with_unsupported_dtypes({"2.0.1 and below": ("float16",)}, "torch")
+ @with_unsupported_dtypes({"2.1.0 and below": ("float16",)}, "torch")
def addcdiv(self, tensor1, tensor2, *, value=1):
return torch_frontend.addcdiv(self, tensor1, tensor2, value=value)
- @with_unsupported_dtypes({"2.0.1 and below": ("float16",)}, "torch")
+ @with_unsupported_dtypes({"2.1.0 and below": ("float16",)}, "torch")
def addcmul(self, tensor1, tensor2, *, value=1):
return torch_frontend.addcmul(self, tensor1, tensor2, value=value)
- @with_unsupported_dtypes({"2.0.1 and below": ("float16",)}, "torch")
+ @with_unsupported_dtypes({"2.1.0 and below": ("float16",)}, "torch")
def addcmul_(self, tensor1, tensor2, *, value=1):
self.ivy_array = self.addcmul(tensor1, tensor2, value=value).ivy_array
return self
sign_decorator_dtypes = ("float16", "complex", "bool")
- @with_unsupported_dtypes({"2.0.1 and below": sign_decorator_dtypes}, "torch")
+ @with_unsupported_dtypes({"2.1.0 and below": sign_decorator_dtypes}, "torch")
def sign(self):
return torch_frontend.sign(self._ivy_array)
- @with_unsupported_dtypes({"2.0.1 and below": sign_decorator_dtypes}, "torch")
+ @with_unsupported_dtypes({"2.1.0 and below": sign_decorator_dtypes}, "torch")
def sign_(self):
self.ivy_array = self.sign().ivy_array
return self
@@ -1463,11 +1540,11 @@ def std(self, dim=None, unbiased=True, keepdim=False, *, out=None):
self, dim=dim, unbiased=unbiased, keepdim=keepdim, out=out
)
- @with_unsupported_dtypes({"2.0.1 and below": ("float16", "bfloat16")}, "torch")
+ @with_unsupported_dtypes({"2.1.0 and below": ("float16", "bfloat16")}, "torch")
def fmod(self, other, *, out=None):
return torch_frontend.fmod(self, other, out=out)
- @with_unsupported_dtypes({"2.0.1 and below": ("float16", "bfloat16")}, "torch")
+ @with_unsupported_dtypes({"2.1.0 and below": ("float16", "bfloat16")}, "torch")
def fmod_(self, other):
self.ivy_array = self.fmod(other).ivy_array
return self
@@ -1478,96 +1555,96 @@ def norm(self, p="fro", dim=None, keepdim=False, dtype=None):
def tolist(self):
return self._ivy_array.to_list()
- @with_unsupported_dtypes({"2.0.1 and below": ("bfloat16",)}, "torch")
+ @with_unsupported_dtypes({"2.1.0 and below": ("bfloat16",)}, "torch")
def multiply(self, other, *, out=None):
return torch_frontend.multiply(self, other, out=out)
- @with_unsupported_dtypes({"2.0.1 and below": ("bfloat16",)}, "torch")
+ @with_unsupported_dtypes({"2.1.0 and below": ("bfloat16",)}, "torch")
def multiply_(self, other, *, out=None):
self.ivy_array = torch_frontend.multiply(self, other, out=out).ivy_array
return self
@numpy_to_torch_style_args
- @with_unsupported_dtypes({"2.0.1 and below": ("float16", "complex")}, "torch")
+ @with_unsupported_dtypes({"2.1.0 and below": ("float16", "complex")}, "torch")
def topk(self, k, dim=None, largest=True, sorted=True):
return torch_frontend.topk(self, k, dim=dim, largest=largest, sorted=sorted)
rshift_dtypes = ("float16", "bfloat16", "float32", "float64", "bool", "complex")
- @with_unsupported_dtypes({"2.0.1 and below": rshift_dtypes}, "torch")
+ @with_unsupported_dtypes({"2.1.0 and below": rshift_dtypes}, "torch")
def bitwise_right_shift(self, other, *, out=None):
return torch_frontend.bitwise_right_shift(self._ivy_array, other)
@with_supported_dtypes(
- {"2.0.1 and below": ("uint8", "int8", "int32", "int64")}, "torch"
+ {"2.1.0 and below": ("uint8", "int8", "int32", "int64")}, "torch"
)
def bitwise_right_shift_(self, other, *, out=None):
self.ivy_array = self.bitwise_right_shift(other, out=out).ivy_array
return self
- @with_unsupported_dtypes({"2.0.1 and below": ("float16", "bfloat16")}, "torch")
+ @with_unsupported_dtypes({"2.1.0 and below": ("float16", "bfloat16")}, "torch")
def logdet(self):
chol = torch_frontend.cholesky(self)
return 2 * torch_frontend.sum(
torch_frontend.log(torch_frontend.real(torch_frontend.diagonal(chol)))
)
- @with_unsupported_dtypes({"2.0.1 and below": ("float16", "bfloat16")}, "torch")
+ @with_unsupported_dtypes({"2.1.0 and below": ("float16", "bfloat16")}, "torch")
def copysign(self, other, *, out=None):
return torch_frontend.copysign(self, other, out=out)
@with_supported_dtypes(
- {"2.0.1 and below": ("float16", "float32", "float64")}, "torch"
+ {"2.1.0 and below": ("float16", "float32", "float64")}, "torch"
)
def copysign_(self, other, *, out=None):
self.ivy_array = self.copysign(other, out=out).ivy_array
return self
@with_unsupported_dtypes(
- {"2.0.1 and below": ("complex", "bfloat16", "bool")}, "torch"
+ {"2.1.0 and below": ("complex", "bfloat16", "bool")}, "torch"
)
def greater(self, other, *, out=None):
return torch_frontend.greater(self, other, out=out)
- @with_unsupported_dtypes({"2.0.1 and below": ("bfloat16", "bool")}, "torch")
+ @with_unsupported_dtypes({"2.1.0 and below": ("bfloat16", "bool")}, "torch")
def greater_(self, other):
self.ivy_array = ivy.astype(self.greater(other).ivy_array, self.dtype)
return self
@with_unsupported_dtypes(
- {"2.0.1 and below": ("complex", "bfloat16", "bool")}, "torch"
+ {"2.1.0 and below": ("complex", "bfloat16", "bool")}, "torch"
)
def greater_equal(self, other, *, out=None):
return torch_frontend.greater_equal(self, other, out=out)
- @with_unsupported_dtypes({"2.0.1 and below": ("bfloat16", "bool")}, "torch")
+ @with_unsupported_dtypes({"2.1.0 and below": ("bfloat16", "bool")}, "torch")
def greater_equal_(self, other):
self.ivy_array = ivy.astype(self.greater_equal(other).ivy_array, self.dtype)
return self
@with_unsupported_dtypes(
- {"2.0.1 and below": ("complex", "bfloat16", "bool")}, "torch"
+ {"2.1.0 and below": ("complex", "bfloat16", "bool")}, "torch"
)
def less(self, other, *, out=None):
return torch_frontend.less(self, other, out=out)
- @with_unsupported_dtypes({"2.0.1 and below": ("bfloat16", "bool")}, "torch")
+ @with_unsupported_dtypes({"2.1.0 and below": ("bfloat16", "bool")}, "torch")
def less_(self, other):
self.ivy_array = ivy.astype(self.less(other).ivy_array, self.dtype)
return self
@with_unsupported_dtypes(
- {"2.0.1 and below": ("complex", "bfloat16", "bool")}, "torch"
+ {"2.1.0 and below": ("complex", "bfloat16", "bool")}, "torch"
)
def less_equal(self, other, *, out=None):
return torch_frontend.less_equal(self, other, out=out)
- @with_unsupported_dtypes({"2.0.1 and below": ("bfloat16", "bool")}, "torch")
+ @with_unsupported_dtypes({"2.1.0 and below": ("bfloat16", "bool")}, "torch")
def less_equal_(self, other):
self.ivy_array = ivy.astype(self.less_equal(other).ivy_array, self.dtype)
return self
- @with_unsupported_dtypes({"2.0.1 and below": ("bfloat16",)}, "torch")
+ @with_unsupported_dtypes({"2.1.0 and below": ("bfloat16",)}, "torch")
def eq_(self, other):
self.ivy_array = ivy.astype(
torch_frontend.eq(self, other).ivy_array, self.dtype
@@ -1596,13 +1673,13 @@ def stride(self, dim=None):
return strides
@with_supported_dtypes(
- {"2.0.1 and below": ("float32", "float64", "bfloat16")}, "torch"
+ {"2.1.0 and below": ("float32", "float64", "bfloat16")}, "torch"
)
def log1p(self):
promoted_type = ivy.promote_types(self.dtype, "float32")
return torch_frontend.log1p(self).to(promoted_type)
- @with_supported_dtypes({"2.0.1 and below": ("float32", "float64")}, "torch")
+ @with_supported_dtypes({"2.1.0 and below": ("float32", "float64")}, "torch")
def log1p_(self):
promoted_type = ivy.promote_types(self.dtype, "float32")
self.ivy_array = torch_frontend.log1p(self).to(promoted_type).ivy_array
@@ -1622,14 +1699,14 @@ def baddbmm_(self, batch1, batch2, *, beta=1, alpha=1):
def bmm(self, mat2):
return torch_frontend.bmm(self, mat2=mat2)
- @with_unsupported_dtypes({"2.0.1 and below": ("float16",)}, "torch")
+ @with_unsupported_dtypes({"2.1.0 and below": ("float16",)}, "torch")
def floor_(self):
self.ivy_array = self.floor().ivy_array
return self
@with_unsupported_dtypes(
{
- "2.0.1 and below": (
+ "2.1.0 and below": (
"bfloat16",
"complex",
"float64",
@@ -1645,7 +1722,7 @@ def diff(self, n=1, dim=-1, prepend=None, append=None):
def diag(self, diagonal=0):
return torch_frontend.diag(self, diagonal=diagonal)
- @with_unsupported_dtypes({"2.0.1 and below": ("bfloat16",)}, "torch")
+ @with_unsupported_dtypes({"2.1.0 and below": ("bfloat16",)}, "torch")
def diagonal(self, offset=0, dim1=0, dim2=1):
return torch_frontend.diagonal(self, offset=offset, dim1=dim1, dim2=dim2)
@@ -1653,14 +1730,14 @@ def gather(self, dim, index):
return torch_frontend.gather(self, dim=dim, index=index)
@with_supported_dtypes(
- {"2.0.1 and below": ("float32", "float64", "int32", "int64")}, "torch"
+ {"2.1.0 and below": ("float32", "float64", "int32", "int64")}, "torch"
)
def scatter_add_(self, dim, index, src):
self.ivy_array = ivy.put_along_axis(self.ivy_array, index, src, dim, mode="sum")
return self
@with_supported_dtypes(
- {"2.0.1 and below": ("float32", "float64", "int32", "int64")}, "torch"
+ {"2.1.0 and below": ("float32", "float64", "int32", "int64")}, "torch"
)
def scatter_(self, dim, index, src, *, reduce=None):
if reduce is None:
@@ -1677,7 +1754,7 @@ def scatter_(self, dim, index, src, *, reduce=None):
return self
@with_supported_dtypes(
- {"2.0.1 and below": ("float32", "float64", "int32", "int64")}, "torch"
+ {"2.1.0 and below": ("float32", "float64", "int32", "int64")}, "torch"
)
def scatter_reduce_(self, dim, index, src, reduce, *, include_self=True):
if reduce == "prod":
@@ -1688,19 +1765,19 @@ def scatter_reduce_(self, dim, index, src, reduce, *, include_self=True):
return self
@with_supported_dtypes(
- {"2.0.1 and below": ("float32", "float64", "int32", "int64")}, "torch"
+ {"2.1.0 and below": ("float32", "float64", "int32", "int64")}, "torch"
)
def scatter_add(self, dim, index, src):
return torch_frontend.scatter_add(self, dim, index, src)
@with_supported_dtypes(
- {"2.0.1 and below": ("float32", "float64", "int32", "int64")}, "torch"
+ {"2.1.0 and below": ("float32", "float64", "int32", "int64")}, "torch"
)
def scatter(self, dim, index, src):
return torch_frontend.scatter_reduce(self, dim, index, src, reduce="replace")
@with_supported_dtypes(
- {"2.0.1 and below": ("float32", "float64", "int32", "int64")}, "torch"
+ {"2.1.0 and below": ("float32", "float64", "int32", "int64")}, "torch"
)
def scatter_reduce(self, dim, index, src, reduce, *, include_self=True):
return torch_frontend.scatter_reduce(self, dim, index, src, reduce=reduce)
@@ -1711,14 +1788,14 @@ def take_along_dim(self, indices, dim):
def movedim(self, source, destination):
return torch_frontend.movedim(self, source=source, destination=destination)
- @with_unsupported_dtypes({"2.0.1 and below": ("float16",)}, "torch")
+ @with_unsupported_dtypes({"2.1.0 and below": ("float16",)}, "torch")
def addcdiv_(self, tensor1, tensor2, *, value=1):
self.ivy_array = self.addcdiv(
tensor1=tensor1, tensor2=tensor2, value=value
).ivy_array
return self
- @with_unsupported_dtypes({"2.0.1 and below": ("bfloat16", "float16")}, "torch")
+ @with_unsupported_dtypes({"2.1.0 and below": ("bfloat16", "float16")}, "torch")
def cholesky(self, upper=False):
return torch_frontend.cholesky(self, upper=upper)
@@ -1757,7 +1834,7 @@ def backward(self, gradient=None, retain_graph=None, create_graph=False):
else:
next_function(_grad_list[idx])
- @with_unsupported_dtypes({"2.0.1 and below": ("float16", "bfloat16")}, "torch")
+ @with_unsupported_dtypes({"2.1.0 and below": ("float16", "bfloat16")}, "torch")
def logaddexp(self, other):
return torch_frontend.logaddexp(self, other)
@@ -1781,17 +1858,17 @@ def adjoint(self):
return torch_frontend.adjoint(self)
@with_unsupported_dtypes(
- {"2.0.1 and below": ("int16", "float16", "bfloat16")}, "torch"
+ {"2.1.0 and below": ("int16", "float16", "bfloat16")}, "torch"
)
def conj(self):
return torch_frontend.conj(self)
- @with_unsupported_dtypes({"2.0.1 and below": ("float16", "bfloat16")}, "torch")
+ @with_unsupported_dtypes({"2.1.0 and below": ("float16", "bfloat16")}, "torch")
def svd(self, some=True, compute_uv=True, *, out=None):
return torch_frontend.svd(self, some=some, compute_uv=compute_uv, out=out)
@with_unsupported_dtypes(
- {"2.0.1 and below": ("float16", "bfloat16", "float32", "float64", "complex")},
+ {"2.1.0 and below": ("float16", "bfloat16", "float32", "float64", "complex")},
"torch",
)
def gcd(self, other, *, out=None):
@@ -1799,7 +1876,7 @@ def gcd(self, other, *, out=None):
@with_unsupported_dtypes(
{
- "2.0.1 and below": (
+ "2.1.0 and below": (
"float16",
"bfloat16",
"uint16",
@@ -1819,7 +1896,7 @@ def char(self):
@with_unsupported_dtypes(
{
- "2.0.1 and below": (
+ "2.1.0 and below": (
"float16",
"bfloat16",
"float32",
@@ -1836,7 +1913,7 @@ def lcm(self, other, *, out=None):
@with_unsupported_dtypes(
{
- "2.0.1 and below": (
+ "2.1.0 and below": (
"float16",
"bfloat16",
"float32",
@@ -1857,7 +1934,7 @@ def lcm_(self, other, *, out=None):
@with_unsupported_dtypes(
{
- "2.0.1 and below": (
+ "2.1.0 and below": (
"bfloat16",
"int8",
"uint8",
@@ -1874,7 +1951,7 @@ def triu_(self, diagonal=0):
return self
@with_unsupported_dtypes(
- {"2.0.1 and below": ("float16", "bfloat16")},
+ {"2.1.0 and below": ("float16", "bfloat16")},
"torch",
)
def quantile(self, q, dim=None, keepdim=False, *, interpolation="linear", out=None):
@@ -1884,7 +1961,7 @@ def quantile(self, q, dim=None, keepdim=False, *, interpolation="linear", out=No
@with_unsupported_dtypes(
{
- "2.0.1 and below": (
+ "2.1.0 and below": (
"int8",
"int16",
"uint8",
@@ -1916,7 +1993,7 @@ def random_(
@with_unsupported_dtypes(
{
- "2.0.1 and below": (
+ "2.1.0 and below": (
"float16",
"bfloat16",
)
@@ -1928,7 +2005,7 @@ def sinc(self):
@with_supported_dtypes(
{
- "2.0.1 and below": (
+ "2.1.0 and below": (
"float32",
"float64",
"bfloat16",
@@ -1940,7 +2017,7 @@ def sinc_(self):
self.ivy_array = torch_frontend.sinc(self).ivy_array
return self
- @with_unsupported_dtypes({"2.0.1 and below": ("uint8",)}, "torch")
+ @with_unsupported_dtypes({"2.1.0 and below": ("uint8",)}, "torch")
def index_fill(self, dim, index, value):
arr = torch_frontend.moveaxis(self, dim, 0)
arr[ivy.to_list(index)] = value
@@ -1949,7 +2026,7 @@ def index_fill(self, dim, index, value):
@with_unsupported_dtypes(
{
- "2.0.1 and below": (
+ "2.1.0 and below": (
"bfloat16",
"int8",
"uint8",
@@ -1972,7 +2049,7 @@ def unique_consecutive(self, return_inverse, return_counts, dim):
@with_unsupported_dtypes(
{
- "2.0.1 and below": (
+ "2.1.0 and below": (
"uint16",
"uint32",
"uint64",
@@ -1989,7 +2066,7 @@ def cummax(self, dim):
@with_unsupported_dtypes(
{
- "2.0.1 and below": (
+ "2.1.0 and below": (
"bfloat16",
"int8",
"uint8",
@@ -2007,7 +2084,7 @@ def triu(self, diagonal=0):
return torch_frontend.triu(self, diagonal)
@with_unsupported_dtypes(
- {"2.0.1 and below": ("bfloat16",)},
+ {"2.1.0 and below": ("bfloat16",)},
"torch",
)
def xlogy_(self, *, other, out=None):
@@ -2016,7 +2093,41 @@ def xlogy_(self, *, other, out=None):
@with_unsupported_dtypes(
{
- "2.0.1 and below": (
+ "2.1.0 and below": (
+ "bfloat16",
+ "uint8",
+ "uint32",
+ "uint16",
+ "uint64",
+ "complex128",
+ "complex64",
+ )
+ },
+ "torch",
+ )
+ def ne(self, other):
+ return self.not_equal(other)
+
+ @with_unsupported_dtypes(
+ {
+ "2.1.0 and below": (
+ "bfloat16",
+ "uint8",
+ "uint32",
+ "uint16",
+ "uint64",
+ "complex128",
+ "complex64",
+ )
+ },
+ "torch",
+ )
+ def ne_(self, other):
+ return self.not_equal_(other)
+
+ @with_unsupported_dtypes(
+ {
+ "2.1.0 and below": (
"bfloat16",
"int8",
"uint8",
@@ -2036,7 +2147,7 @@ def unique(self, sorted=True, return_inverse=False, return_counts=False, dim=Non
@with_unsupported_dtypes(
{
- "2.0.1 and below": (
+ "2.1.0 and below": (
"float16",
"bfloat16",
)
@@ -2046,10 +2157,20 @@ def unique(self, sorted=True, return_inverse=False, return_counts=False, dim=Non
def xlogy(self, *, other, out=None):
return torch_frontend.xlogy(self, other, out=out)
- @with_unsupported_dtypes({"2.0.1 and below": "complex"}, "torch")
+ @with_unsupported_dtypes({"2.1.0 and below": "complex"}, "torch")
def minimum(self, other, *, out=None):
return torch_frontend.minimum(self, other=other, out=out)
+ def rad2deg(self, *, out=None):
+ return torch_frontend.rad2deg(self, out=out)
+
+ @with_supported_dtypes(
+ {"2.1.0 and below": "valid"},
+ "torch",
+ )
+ def corrcoef(self):
+ return torch_frontend.corrcoef(self)
+
# Method aliases
absolute, absolute_ = abs, abs_
clip, clip_ = clamp, clamp_
@@ -2079,12 +2200,11 @@ def minimum(self, other, *, out=None):
lt_ = less_
le = less_equal
le_ = less_equal_
- ne = not_equal
class Size(tuple):
def __new__(cls, iterable=()):
- new_iterable = list()
+ new_iterable = []
for i, item in enumerate(iterable):
if isinstance(item, int):
new_iterable.append(item)
@@ -2096,9 +2216,7 @@ def __new__(cls, iterable=()):
return super().__new__(cls, tuple(new_iterable))
def __init__(self, shape) -> None:
- self._ivy_shape = (
- ivy.shape(shape) if not isinstance(shape, ivy.Shape) else shape
- )
+ self._ivy_shape = shape if isinstance(shape, ivy.Shape) else ivy.shape(shape)
def __repr__(self):
return f'ivy.frontends.torch.Size([{", ".join(str(d) for d in self)}])'
diff --git a/ivy/functional/frontends/torch/tensor_functions.py b/ivy/functional/frontends/torch/tensor_functions.py
index 4ec803669f13a..135981f71aaee 100644
--- a/ivy/functional/frontends/torch/tensor_functions.py
+++ b/ivy/functional/frontends/torch/tensor_functions.py
@@ -31,7 +31,7 @@ def numel(input):
@to_ivy_arrays_and_back
@with_supported_dtypes(
- {"2.0.1 and below": ("float32", "float64", "int32", "int64")}, "torch"
+ {"2.1.0 and below": ("float32", "float64", "int32", "int64")}, "torch"
)
def scatter(input, dim, index, src):
return ivy.put_along_axis(input, index, src, dim, mode="replace")
@@ -39,7 +39,7 @@ def scatter(input, dim, index, src):
@to_ivy_arrays_and_back
@with_supported_dtypes(
- {"2.0.1 and below": ("float32", "float64", "int32", "int64")}, "torch"
+ {"2.1.0 and below": ("float32", "float64", "int32", "int64")}, "torch"
)
def scatter_add(input, dim, index, src):
return ivy.put_along_axis(input, index, src, dim, mode="sum")
@@ -47,7 +47,7 @@ def scatter_add(input, dim, index, src):
@to_ivy_arrays_and_back
@with_supported_dtypes(
- {"2.0.1 and below": ("float32", "float64", "int32", "int64")}, "torch"
+ {"2.1.0 and below": ("float32", "float64", "int32", "int64")}, "torch"
)
def scatter_reduce(input, dim, index, src, reduce, *, include_self=True):
mode_mappings = {
diff --git a/ivy/functional/frontends/torch/utilities.py b/ivy/functional/frontends/torch/utilities.py
index fea89a9e3af9b..da073c2720660 100644
--- a/ivy/functional/frontends/torch/utilities.py
+++ b/ivy/functional/frontends/torch/utilities.py
@@ -20,7 +20,7 @@ def _assert(condition, message):
# ------------ #
-@with_supported_dtypes({"2.0.1 and above": ("int64",)}, "torch")
+@with_supported_dtypes({"2.1.0 and above": ("int64",)}, "torch")
@to_ivy_arrays_and_back
def bincount(x, weights=None, minlength=0):
return ivy.bincount(x, weights=weights, minlength=minlength)
diff --git a/ivy/functional/frontends/torchvision/__init__.py b/ivy/functional/frontends/torchvision/__init__.py
new file mode 100644
index 0000000000000..562efb862eef2
--- /dev/null
+++ b/ivy/functional/frontends/torchvision/__init__.py
@@ -0,0 +1,23 @@
+import sys
+
+
+import ivy.functional.frontends.torch as torch
+import ivy
+from ivy.functional.frontends import set_frontend_to_specific_version
+
+
+from . import ops
+
+
+tensor = _frontend_array = torch.tensor
+
+
+# setting to specific version #
+# --------------------------- #
+
+if ivy.is_local():
+ module = ivy.utils._importlib.import_cache[__name__]
+else:
+ module = sys.modules[__name__]
+
+set_frontend_to_specific_version(module)
diff --git a/ivy/functional/frontends/torchvision/ops.py b/ivy/functional/frontends/torchvision/ops.py
new file mode 100644
index 0000000000000..589d0cb22910f
--- /dev/null
+++ b/ivy/functional/frontends/torchvision/ops.py
@@ -0,0 +1,61 @@
+import ivy
+from ivy.functional.frontends.torch.func_wrapper import to_ivy_arrays_and_back
+from ivy.func_wrapper import with_supported_dtypes, with_unsupported_device_and_dtypes
+
+
+@to_ivy_arrays_and_back
+def batched_nms(boxes, scores, idxs, iou_threshold):
+ if boxes.size == 0:
+ return ivy.array([], dtype=ivy.int64)
+ else:
+ max_coordinate = boxes.max()
+ boxes_dtype = boxes.dtype
+ offsets = idxs.astype(boxes_dtype) * (
+ max_coordinate + ivy.array(1, dtype=boxes_dtype)
+ )
+ boxes_for_nms = boxes + offsets[:, None]
+ keep = nms(boxes_for_nms, scores, iou_threshold)
+ return keep
+
+
+@to_ivy_arrays_and_back
+def box_area(boxes):
+ return ivy.prod(boxes[..., 2:] - boxes[..., :2], axis=-1)
+
+
+@with_unsupported_device_and_dtypes(
+ {
+ "2.1.0 and below": {
+ "cpu": ("float16",),
+ }
+ },
+ "torch",
+)
+@to_ivy_arrays_and_back
+def clip_boxes_to_image(boxes, size):
+ height, width = size
+ boxes_x = boxes[..., 0::2].clip(0, width)
+ boxes_y = boxes[..., 1::2].clip(0, height)
+ clipped_boxes = ivy.stack([boxes_x, boxes_y], axis=-1)
+ return clipped_boxes.reshape(boxes.shape).astype(boxes.dtype)
+
+
+@to_ivy_arrays_and_back
+def nms(boxes, scores, iou_threshold):
+ return ivy.nms(boxes, scores, iou_threshold)
+
+
+@to_ivy_arrays_and_back
+def remove_small_boxes(boxes, min_size):
+ w, h = boxes[..., 2] - boxes[..., 0], boxes[..., 3] - boxes[..., 1]
+ return ivy.nonzero((w >= min_size) & (h >= min_size))[0]
+
+
+@with_supported_dtypes({"2.1.0 and below": ("float32", "float64")}, "torch")
+@to_ivy_arrays_and_back
+def roi_align(
+ input, boxes, output_size, spatial_scale=1.0, sampling_ratio=1, aligned=False
+):
+ return ivy.roi_align(
+ input, boxes, output_size, spatial_scale, sampling_ratio, aligned
+ )
diff --git a/ivy/functional/frontends/xgboost/core.py b/ivy/functional/frontends/xgboost/core.py
index 6df989ed17a88..8e8dbf9b557f4 100644
--- a/ivy/functional/frontends/xgboost/core.py
+++ b/ivy/functional/frontends/xgboost/core.py
@@ -90,13 +90,14 @@ def num_col(self):
class Booster:
- def __init__(self, params=None, cache=None, model_file=None):
+ def __init__(self, params=None, cache=None, model_file=None, compile=False):
# cache[0] refers to input data while cache[1] refers to input target
n_feat = cache[0].shape[1]
n_inst = cache[0].shape[0]
n_output_group = ivy.unique_values(cache[1]).shape[0]
- # by default xgboost calculates the mean of a target if base_score is not provided
+ # by default xgboost calculates the mean of a target if base_score is not
+ # provided
params["base_score"] = (
cache[1].mean() if not params["base_score"] else params["base_score"]
)
@@ -111,7 +112,15 @@ def __init__(self, params=None, cache=None, model_file=None):
)
# create gbm(as for now only gblinear booster is available)
- self.gbm = GBLinear(params)
+ self.gbm = GBLinear(params, compile=compile, cache=cache)
+ self.compile = compile
+ if self.compile:
+ self._comp_binary_prediction = ivy.trace_graph(
+ _binary_prediction, backend_compile=True, static_argnums=(0,)
+ )
+
+ # invoke function to get its compiled version
+ self._comp_binary_prediction(self.gbm.obj, cache[1])
def update(self, dtrain, dlabel, iteration, fobj=None):
"""
@@ -171,9 +180,9 @@ def predict(
contributions is equal to the raw untransformed margin value of the
prediction. Note the final column is the bias term.
approx_contribs
- Approximate the contributions of each feature. Used when ``pred_contribs`` or
- ``pred_interactions`` is set to True. Changing the default of this parameter
- (False) is not recommended.
+ Approximate the contributions of each feature. Used when ``pred_contribs``
+ or ``pred_interactions`` is set to True. Changing the default of this
+ parameter (False) is not recommended.
pred_interactions
When this is True the output will be a matrix of size (nsample,
nfeats + 1, nfeats + 1) indicating the SHAP interaction values for
@@ -188,17 +197,18 @@ def predict(
feature_names are the same.
training
Whether the prediction value is used for training. This can effect `dart`
- booster, which performs dropouts during training iterations but use all trees
- for inference. If you want to obtain result with dropouts, set this parameter
- to `True`. Also, the parameter is set to true when obtaining prediction for
- custom objective function.
+ booster, which performs dropouts during training iterations but use all
+ trees for inference. If you want to obtain result with dropouts, set this
+ parameter to `True`. Also, the parameter is set to true when obtaining
+ prediction for custom objective function.
iteration_range
Specifies which layer of trees are used in prediction. For example, if a
random forest is trained with 100 rounds. Specifying `iteration_range=(10,
20)`, then only the forests built during [10, 20) (half open set) rounds are
used in this prediction. Unsupported for gblinear booster.
strict_shape
- When set to True, output shape is invariant to whether classification is used.
+ When set to True, output shape is invariant to whether classification is
+ used.
For both value and margin prediction, the output shape is (n_samples,
n_groups), n_groups == 1 when multi-class is not used. Default to False, in
which case the output shape can be (n_samples, ) if multi-class is not used.
@@ -210,9 +220,20 @@ def predict(
# currently supports prediction for binary task
# get raw predictions
pred = self.gbm.pred(data)
+ args = (self.gbm.obj, pred)
+
+ if self.compile:
+ return self._comp_binary_prediction(*args)
+ else:
+ return _binary_prediction(*args)
+
+
+# --- Helpers --- #
+# --------------- #
- # apply activation function
- pred = self.gbm.obj.pred_transform(pred)
- # apply probability thresholding
- return ivy.where(pred >= 0.5, 1.0, 0.0)
+def _binary_prediction(obj, raw_pred):
+ # apply activation function
+ pred = obj.pred_transform(raw_pred)
+ # apply probability thresholding
+ return ivy.where(pred >= 0.5, 1.0, 0.0)
diff --git a/ivy/functional/frontends/xgboost/gbm/gbm.py b/ivy/functional/frontends/xgboost/gbm/gbm.py
index a3779c99504e7..41271ea82cc56 100644
--- a/ivy/functional/frontends/xgboost/gbm/gbm.py
+++ b/ivy/functional/frontends/xgboost/gbm/gbm.py
@@ -5,26 +5,31 @@
from ivy.functional.frontends.xgboost.linear.updater_coordinate import (
coordinate_updater,
)
+from copy import deepcopy
class GBLinear:
- def __init__(self, params=None):
+ def __init__(self, params=None, compile=False, cache=None):
# we start boosting from zero
self.num_boosted_rounds = 0
# default parameter
- # xgboost provides other options for it but the way to modify it remains undocumented for Python API
+ # xgboost provides other options for it but the way to modify it remains
+ # undocumented for Python API
self.updater = coordinate_updater
- # LogisticRegression corresponds to 'binary:logistic' objective in terms of calculations
- # In xgboost LogisticClassification is used, but it simply subclasses LogisticRegression
- # redefining the method which returns the name of objective
+ # LogisticRegression corresponds to 'binary:logistic' objective in terms of
+ # calculations
+ # In xgboost LogisticClassification is used, but it simply subclasses
+ # LogisticRegression redefining the method which returns the name of objective
self.obj = LogisticRegression()
self.base_score = self.obj.prob_to_margin(params["base_score"])
- # when weights for groups are not provided this equals to a number of instances in data
- # ToDo: add weight sum calculation from provided weights, by now always assume default behaviour
+ # when weights for groups are not provided this equals to a number of instances
+ # in data
+ # TODO: add weight sum calculation from provided weights, by now always assume
+ # default behaviour
self.num_inst = params["num_instances"]
self.sum_instance_weight_ = self.num_inst
self.scale_pos_weight = (
@@ -40,14 +45,15 @@ def __init__(self, params=None):
self.num_output_group = params["num_output_group"]
self.num_feature = params["num_feature"]
- # xgboost stores weights in a vector form, but it was decided to store them as a 2D matrix here
- # it simplifies calculations while math remains the same
+ # xgboost stores weights in a vector form, but it was decided to store them as a
+ # 2D matrix here it simplifies calculations while math remains the same
# added 1 in the first dim, because xgboost stores weights and biases jointly
self.weight = ivy.zeros(
(self.num_feature + 1, self.num_output_group), dtype=ivy.float32
)
- # used to calculate convergence(comparing max difference of weights to tolerance)
- self.prev_weight = self.weight.copy()
+ # used to calculate convergence(comparing max difference of weights to
+ # tolerance)
+ self.prev_weight = deepcopy(self.weight)
# if base margin is None, use base_score instead
self.base_margin = (
@@ -59,6 +65,37 @@ def __init__(self, params=None):
self.reg_lambda_denorm = self.sum_instance_weight_ * params["reg_lambda"]
self.reg_alpha_denorm = self.sum_instance_weight_ * params["reg_alpha"]
+ # compilation block
+ self.compile = compile
+ if self.compile:
+ # don't enable native compilation for torch, bc it's already fast enough
+ # and this only increases the compilation time
+ backend_compile = True if ivy.current_backend_str() != "torch" else False
+ self._comp_pred = ivy.trace_graph(_pred, backend_compile=backend_compile)
+ self._comp_get_gradient = ivy.trace_graph(
+ _get_gradient, backend_compile=backend_compile, static_argnums=(0,)
+ )
+ self._comp_updater = ivy.trace_graph(
+ self.updater, backend_compile=backend_compile
+ )
+
+ # run each function to compile it
+ # this process doesn't affect the training
+ pred = self._comp_pred(cache[0], self.weight, self.base_margin)
+ gpair = self._comp_get_gradient(
+ self.obj, pred, cache[1], self.scale_pos_weight
+ )
+ self._comp_updater(
+ gpair,
+ cache[0],
+ self.learning_rate,
+ self.weight,
+ self.num_feature,
+ 0,
+ self.reg_alpha_denorm,
+ self.reg_lambda_denorm,
+ )
+
def boosted_rounds(self):
return self.num_boosted_rounds
@@ -80,15 +117,23 @@ def check_convergence(self):
# used to obtain raw predictions
def pred(self, data):
- return _pred(data, self.weight, self.base_margin)
+ args = (data, self.weight, self.base_margin)
+ if self.compile:
+ return self._comp_pred(*args)
+ else:
+ return _pred(*args)
def get_gradient(self, pred, label):
- return _get_gradient(self.obj, pred, label, self.scale_pos_weight)
+ args = (self.obj, pred, label, self.scale_pos_weight)
+ if self.compile:
+ return self._comp_get_gradient(*args)
+ else:
+ return _get_gradient(*args)
def do_boost(self, data, gpair, iter):
if not self.check_convergence():
self.num_boosted_rounds += 1
- self.weight = self.updater(
+ args = (
gpair,
data,
self.learning_rate,
@@ -98,6 +143,10 @@ def do_boost(self, data, gpair, iter):
self.reg_alpha_denorm,
self.reg_lambda_denorm,
)
+ if self.compile:
+ self.weight = self._comp_updater(*args)
+ else:
+ self.weight = self.updater(*args)
# --- Helpers --- #
diff --git a/ivy/functional/frontends/xgboost/sklearn.py b/ivy/functional/frontends/xgboost/sklearn.py
index 6a275622c0e5f..e2edeecb81802 100644
--- a/ivy/functional/frontends/xgboost/sklearn.py
+++ b/ivy/functional/frontends/xgboost/sklearn.py
@@ -2,6 +2,7 @@
from ivy.functional.frontends.sklearn.base import BaseEstimator as XGBModelBase
from ivy.functional.frontends.sklearn.base import ClassifierMixin as XGBClassifierBase
from .training import train
+from .core import Booster
class XGBModel(XGBModelBase):
@@ -88,6 +89,7 @@ def __init__(
self.eval_metric = eval_metric
self.early_stopping_rounds = early_stopping_rounds
self.callbacks = callbacks
+ self.compiled = False
if kwargs:
self.kwargs = kwargs
@@ -117,7 +119,7 @@ def get_params(self, deep=True):
# take random_state into account only if it's an integer
if isinstance(params["random_state"], int):
- ivy.seed(params["random_state"])
+ ivy.seed(seed_value=params["random_state"])
return params
@@ -149,6 +151,14 @@ def get_num_boosting_rounds(self):
# 100 is the default number of boosting rounds
return 100 if not self.n_estimators else self.n_estimators
+ def compile(self, X, y):
+ # set compiled flag
+ self.compiled = True
+
+ # instantiate Booster and compile funcs involved in calculations for training
+ params = self.get_xgb_params()
+ self._Booster = Booster(params, cache=[X, y], compile=True)
+
def fit(
self,
X,
@@ -222,9 +232,12 @@ def fit(
"""
# skip all the validation as we're interested in calculations for now
# ToDo: add handling for custom objective
- params = self.get_xgb_params()
-
- self._Booster = train(params, X, y, self.get_num_boosting_rounds())
+ if self.compiled:
+ for i in range(self.get_num_boosting_rounds()):
+ self._Booster.update(X, y, i)
+ else:
+ params = self.get_xgb_params()
+ self._Booster = train(params, X, y, self.get_num_boosting_rounds())
return self
@@ -259,7 +272,8 @@ def predict(
prediction
"""
- # skip the validation, as for now we simply call the predict method of underlying booster
+ # skip the validation, as for now we simply call the predict method of
+ # underlying booster
return self.get_booster().predict(
data=X,
iteration_range=iteration_range,
@@ -269,7 +283,7 @@ def predict(
class XGBClassifier(XGBModel, XGBClassifierBase):
- # as for now simply calls the init method of a parent class, because we implement a minimal
- # subset of functionality
+ # as for now simply calls the init method of a parent class, because we implement a
+ # minimal subset of functionality
def __init__(self, *, objective="binary:logistic", **kwargs):
super().__init__(objective=objective, **kwargs)
diff --git a/ivy/functional/frontends/xgboost/training.py b/ivy/functional/frontends/xgboost/training.py
index 4063985939179..cc727add4c5a6 100644
--- a/ivy/functional/frontends/xgboost/training.py
+++ b/ivy/functional/frontends/xgboost/training.py
@@ -46,8 +46,8 @@ def train(
Requires at least one item in **evals**.
The method returns the model from the last iteration (not the best one). Use
custom callback or model slicing if the best model is desired.
- If there's more than one item in **evals**, the last entry will be used for early
- stopping.
+ If there's more than one item in **evals**, the last entry will be used for
+ early stopping.
If there's more than one metric in the **eval_metric** parameter given in
**params**, the last metric will be used for early stopping.
If early stopping occurs, the model will have two additional fields:
@@ -58,11 +58,13 @@ def train(
Requires at least one item in **evals**.
If **verbose_eval** is True then the evaluation metric on the validation set is
printed at each boosting stage.
- If **verbose_eval** is an integer then the evaluation metric on the validation set
- is printed at every given **verbose_eval** boosting stage. The last boosting stage
- / the boosting stage found by using **early_stopping_rounds** is also printed.
- Example: with ``verbose_eval=4`` and at least one item in **evals**, an evaluation metric
- is printed every 4 boosting stages, instead of every boosting stage.
+ If **verbose_eval** is an integer then the evaluation metric on the validation
+ set is printed at every given **verbose_eval** boosting stage. The last boosting
+ stage / the boosting stage found by using **early_stopping_rounds** is also
+ printed.
+ Example: with ``verbose_eval=4`` and at least one item in **evals**, an
+ evaluation metric is printed every 4 boosting stages, instead of every boosting
+ stage.
xgb_model
Xgb model to be loaded before training (allows training continuation).
callbacks
diff --git a/ivy/functional/ivy/control_flow_ops.py b/ivy/functional/ivy/control_flow_ops.py
index 2096df6b2c1af..9b2dda176ad85 100644
--- a/ivy/functional/ivy/control_flow_ops.py
+++ b/ivy/functional/ivy/control_flow_ops.py
@@ -202,4 +202,4 @@ def _tuple_to_dict(t):
def _dict_to_tuple(d):
- return tuple([d[k] for k in d])
+ return tuple(d[k] for k in d)
diff --git a/ivy/functional/ivy/creation.py b/ivy/functional/ivy/creation.py
index 29bf612cb00df..44c9f370b04ed 100644
--- a/ivy/functional/ivy/creation.py
+++ b/ivy/functional/ivy/creation.py
@@ -3,6 +3,10 @@
import functools
from numbers import Number
from typing import (
+ Union,
+ Tuple,
+ Optional,
+ List,
Sequence,
Callable,
Protocol,
@@ -28,6 +32,7 @@
handle_array_like_without_promotion,
handle_device,
handle_backend_invalid,
+ temp_asarray_wrapper,
)
# Helpers #
@@ -58,7 +63,7 @@ def _asarray_handle_nestable_wrapper(*args, **kwargs):
"""
# This decorator should only be applied to ivy.asarray, so we know where
# the container must be if there is one.
- cont_fn = getattr(ivy.Container, "static_" + fn_name)
+ cont_fn = getattr(ivy.Container, f"static_{fn_name}")
if isinstance(args[0], ivy.Container):
return cont_fn(*args, **kwargs)
@@ -79,28 +84,35 @@ def _ivy_to_native(x):
for i, item in enumerate(x):
x = list(x) if isinstance(x, tuple) else x
x[i] = _ivy_to_native(item)
- else:
- if (isinstance(x, (list, tuple)) and len(x) > 0) and ivy.is_ivy_array(x[0]):
- x = ivy.to_native(x, nested=True)
- elif ivy.is_ivy_array(x):
- x = ivy.to_native(x)
+ elif (isinstance(x, (list, tuple)) and len(x) > 0) and ivy.is_ivy_array(x[0]):
+ x = ivy.to_native(x, nested=True)
+ elif ivy.is_ivy_array(x):
+ x = ivy.to_native(x)
return x
-def _shape_to_native(x):
+def _shape_to_native(x: Iterable) -> Tuple[int]:
# checks the first element of the leaf list and
# converts it to a native array if it is an ivy array
+
+ # This function is to be used with the nested_map function
+ # it was a lambda function before but was replaced with the defined function below
+ def nested_map_shape_fn(x: Iterable) -> List:
+ return x.shape if isinstance(x, ivy.Shape) else x
+
if isinstance(x, (list, tuple)) and len(x) != 0 and isinstance(x[0], (list, tuple)):
for i, item in enumerate(x):
x = list(x) if isinstance(x, tuple) else x
x[i] = _shape_to_native(item)
+
else:
if (isinstance(x, (list, tuple)) and len(x) > 0) and (
isinstance(x[0], ivy.Shape) and ivy.array_mode
):
- x = ivy.nested_map(lambda x: x.shape if isinstance(x, ivy.Shape) else x, x)
+ x = ivy.nested_map(x, nested_map_shape_fn)
elif isinstance(x, ivy.Shape) and ivy.array_mode:
x = x.shape
+
return x
@@ -261,7 +273,7 @@ def _inputs_to_native_shapes(*args, **kwargs):
class NestedSequence(Protocol[_T_co]):
- def __getitem__(self, key: int, /) -> _T_co | NestedSequence[_T_co]: ...
+ def __getitem__(self, key: int, /) -> Union[_T_co, NestedSequence[_T_co]]: ...
def __len__(self, /) -> int: ...
@@ -280,12 +292,12 @@ def __len__(self, /) -> int: ...
def arange(
start: Number,
/,
- stop: Number | None = None,
+ stop: Optional[Number] = None,
step: Number = 1,
*,
- dtype: ivy.Dtype | ivy.NativeDtype | None = None,
- device: ivy.Device | ivy.NativeDevice | None = None,
- out: ivy.Array | None = None,
+ dtype: Optional[Union[ivy.Dtype, ivy.NativeDtype]] = None,
+ device: Optional[Union[ivy.Device, ivy.NativeDevice]] = None,
+ out: Optional[ivy.Array] = None,
) -> ivy.Array:
"""
Return evenly spaced values within a given interval, with the spacing being
@@ -373,30 +385,31 @@ def arange(
)
+@temp_asarray_wrapper
@handle_backend_invalid
@handle_array_like_without_promotion
@handle_out_argument
@handle_array_function
@handle_device
def asarray(
- obj: (
- ivy.Array
- | ivy.NativeArray
- | ivy.Shape
- | ivy.NativeShape
- | bool
- | int
- | float
- | NestedSequence
- | SupportsBufferProtocol
- | np.ndarray
- ),
+ obj: Union[
+ ivy.Array,
+ ivy.NativeArray,
+ ivy.Shape,
+ ivy.NativeShape,
+ bool,
+ int,
+ float,
+ NestedSequence,
+ SupportsBufferProtocol,
+ np.ndarray,
+ ],
/,
*,
- copy: bool | None = None,
- dtype: ivy.Dtype | ivy.NativeDtype | None = None,
- device: ivy.Device | ivy.NativeDevice | None = None,
- out: ivy.Array | None = None,
+ copy: Optional[bool] = None,
+ dtype: Optional[Union[ivy.Dtype, ivy.NativeDtype]] = None,
+ device: Optional[Union[ivy.Device, ivy.NativeDevice]] = None,
+ out: Optional[ivy.Array] = None,
) -> ivy.Array:
"""
Convert the input to an array.
@@ -478,11 +491,11 @@ def asarray(
@infer_dtype
@handle_device
def zeros(
- shape: ivy.Shape | ivy.NativeShape,
+ shape: Union[ivy.Shape, ivy.NativeShape],
*,
- dtype: ivy.Dtype | ivy.NativeDtype | None = None,
- device: ivy.Device | ivy.NativeDevice | None = None,
- out: ivy.Array | None = None,
+ dtype: Optional[Union[ivy.Dtype, ivy.NativeDtype]] = None,
+ device: Optional[Union[ivy.Device, ivy.NativeDevice]] = None,
+ out: Optional[ivy.Array] = None,
) -> ivy.Array:
"""
Return a new array having a specified ``shape`` and filled with zeros.
@@ -543,11 +556,11 @@ def zeros(
@infer_dtype
@handle_device
def ones(
- shape: ivy.Shape | ivy.NativeShape,
+ shape: Union[ivy.Shape, ivy.NativeShape],
*,
- dtype: ivy.Dtype | ivy.NativeDtype | None = None,
- device: ivy.Device | ivy.NativeDevice | None = None,
- out: ivy.Array | None = None,
+ dtype: Optional[Union[ivy.Dtype, ivy.NativeDtype]] = None,
+ device: Optional[Union[ivy.Device, ivy.NativeDevice]] = None,
+ out: Optional[ivy.Array] = None,
) -> ivy.Array:
"""
Return a new array having a specified ``shape`` and filled with ones.
@@ -640,13 +653,13 @@ def ones(
@infer_dtype
@handle_device
def full_like(
- x: ivy.Array | ivy.NativeArray,
+ x: Union[ivy.Array, ivy.NativeArray],
/,
fill_value: Number,
*,
- dtype: ivy.Dtype | ivy.NativeDtype | None = None,
- device: ivy.Device | ivy.NativeDevice | None = None,
- out: ivy.Array | None = None,
+ dtype: Optional[Union[ivy.Dtype, ivy.NativeDtype]] = None,
+ device: Optional[Union[ivy.Device, ivy.NativeDevice]] = None,
+ out: Optional[ivy.Array] = None,
) -> ivy.Array:
"""
Return a new array filled with ``fill_value`` and having the same ``shape`` as an
@@ -749,12 +762,12 @@ def full_like(
@infer_dtype
@handle_device
def ones_like(
- x: ivy.Array | ivy.NativeArray,
+ x: Union[ivy.Array, ivy.NativeArray],
/,
*,
- dtype: ivy.Dtype | ivy.NativeDtype | None = None,
- device: ivy.Device | ivy.NativeDevice | None = None,
- out: ivy.Array | None = None,
+ dtype: Optional[Union[ivy.Dtype, ivy.NativeDtype]] = None,
+ device: Optional[Union[ivy.Device, ivy.NativeDevice]] = None,
+ out: Optional[ivy.Array] = None,
) -> ivy.Array:
"""
Return a new array filled with ones and having the same shape as an input array
@@ -869,12 +882,12 @@ def ones_like(
@infer_dtype
@handle_device
def zeros_like(
- x: ivy.Array | ivy.NativeArray,
+ x: Union[ivy.Array, ivy.NativeArray],
/,
*,
- dtype: ivy.Dtype | ivy.NativeDtype | None = None,
- device: ivy.Device | ivy.NativeDevice | None = None,
- out: ivy.Array | None = None,
+ dtype: Optional[Union[ivy.Dtype, ivy.NativeDtype]] = None,
+ device: Optional[Union[ivy.Device, ivy.NativeDevice]] = None,
+ out: Optional[ivy.Array] = None,
) -> ivy.Array:
"""
Return a new array filled with zeros and having the same ``shape`` as an input array
@@ -983,11 +996,11 @@ def zeros_like(
@handle_array_function
@handle_device
def tril(
- x: ivy.Array | ivy.NativeArray,
+ x: Union[ivy.Array, ivy.NativeArray],
/,
*,
k: int = 0,
- out: ivy.Array | None = None,
+ out: Optional[ivy.Array] = None,
) -> ivy.Array:
"""
Return the lower triangular part of a matrix (or a stack of matrices) ``x``.
@@ -1039,11 +1052,11 @@ def tril(
@handle_array_function
@handle_device
def triu(
- x: ivy.Array | ivy.NativeArray,
+ x: Union[ivy.Array, ivy.NativeArray],
/,
*,
k: int = 0,
- out: ivy.Array | None = None,
+ out: Optional[ivy.Array] = None,
) -> ivy.Array:
"""
Return the upper triangular part of a matrix (or a stack of matrices) ``x``.
@@ -1097,11 +1110,11 @@ def triu(
@infer_dtype
@handle_device
def empty(
- shape: ivy.Shape | ivy.NativeShape,
+ shape: Union[ivy.Shape, ivy.NativeShape],
*,
- dtype: ivy.Dtype | ivy.NativeDtype | None = None,
- device: ivy.Device | ivy.NativeDevice | None = None,
- out: ivy.Array | None = None,
+ dtype: Optional[Union[ivy.Dtype, ivy.NativeDtype]] = None,
+ device: Optional[Union[ivy.Device, ivy.NativeDevice]] = None,
+ out: Optional[ivy.Array] = None,
) -> ivy.Array:
"""
Return a new array of given shape and type, filled with zeros.
@@ -1147,12 +1160,12 @@ def empty(
@infer_dtype
@handle_device
def empty_like(
- x: ivy.Array | ivy.NativeArray,
+ x: Union[ivy.Array, ivy.NativeArray],
/,
*,
- dtype: ivy.Dtype | ivy.NativeDtype | None = None,
- device: ivy.Device | ivy.NativeDevice | None = None,
- out: ivy.Array | None = None,
+ dtype: Optional[Union[ivy.Dtype, ivy.NativeDtype]] = None,
+ device: Optional[Union[ivy.Device, ivy.NativeDevice]] = None,
+ out: Optional[ivy.Array] = None,
) -> ivy.Array:
"""
Return an uninitialized array with the same shape as an input array x.
@@ -1163,7 +1176,7 @@ def empty_like(
input array from which to derive the output array shape.
dtype
output array data type. If dtype is None, the output array data type must be
- inferred from x. Deafult: ``None``.
+ inferred from x. Default: ``None``.
device
device on which to place the created array. If device is None, the output array
device must be inferred from x. Default: ``None``.
@@ -1200,14 +1213,14 @@ def empty_like(
@handle_device
def eye(
n_rows: int,
- n_cols: int | None = None,
+ n_cols: Optional[int] = None,
/,
*,
k: int = 0,
- batch_shape: int | Sequence[int] | None = None,
- dtype: ivy.Dtype | ivy.NativeDtype | None = None,
- device: ivy.Device | ivy.NativeDevice | None = None,
- out: ivy.Array | None = None,
+ batch_shape: Optional[Union[int, Sequence[int]]] = None,
+ dtype: Optional[Union[ivy.Dtype, ivy.NativeDtype]] = None,
+ device: Optional[Union[ivy.Device, ivy.NativeDevice]] = None,
+ out: Optional[ivy.Array] = None,
) -> ivy.Array:
"""
Return a two-dimensional array with ones on the k diagonal and zeros elsewhere.
@@ -1341,16 +1354,16 @@ def eye(
@infer_dtype
@handle_device
def linspace(
- start: ivy.Array | ivy.NativeArray | float,
- stop: ivy.Array | ivy.NativeArray | float,
+ start: Union[ivy.Array, ivy.NativeArray, float],
+ stop: Union[ivy.Array, ivy.NativeArray, float],
/,
num: int,
*,
- axis: int | None = None,
+ axis: Optional[int] = None,
endpoint: bool = True,
- dtype: ivy.Dtype | ivy.NativeDtype | None = None,
- device: ivy.Device | ivy.NativeDevice | None = None,
- out: ivy.Array | None = None,
+ dtype: Optional[Union[ivy.Dtype, ivy.NativeDtype]] = None,
+ device: Optional[Union[ivy.Device, ivy.NativeDevice]] = None,
+ out: Optional[ivy.Array] = None,
) -> ivy.Array:
"""
Generate a certain number of evenly-spaced values in an interval along a given axis.
@@ -1450,11 +1463,11 @@ def linspace(
@handle_array_function
@handle_device
def meshgrid(
- *arrays: ivy.Array | ivy.NativeArray,
+ *arrays: Union[ivy.Array, ivy.NativeArray],
sparse: bool = False,
indexing: str = "xy",
- out: ivy.Array | None = None,
-) -> list[ivy.Array]:
+ out: Optional[ivy.Array] = None,
+) -> List[ivy.Array]:
"""
Return coordinate matrices from coordinate vectors.
@@ -1572,13 +1585,13 @@ def meshgrid(
@handle_array_function
@handle_device
def full(
- shape: ivy.Shape | ivy.NativeShape,
- fill_value: float | bool,
+ shape: Union[ivy.Shape, ivy.NativeShape],
+ fill_value: Union[float, bool],
/,
*,
- dtype: ivy.Dtype | ivy.NativeDtype | None = None,
- device: ivy.Device | ivy.NativeDevice | None = None,
- out: ivy.Array | None = None,
+ dtype: Optional[Union[ivy.Dtype, ivy.NativeDtype]] = None,
+ device: Optional[Union[ivy.Device, ivy.NativeDevice]] = None,
+ out: Optional[ivy.Array] = None,
) -> ivy.Array:
"""
Return a new array having a specified ``shape`` and filled with ``fill_value``.
@@ -1680,7 +1693,9 @@ def full(
@to_native_arrays_and_back
@handle_array_function
@handle_device
-def to_dlpack(x: ivy.Array | ivy.NativeArray, /, *, out: ivy.Array | None = None):
+def to_dlpack(
+ x: Union[ivy.Array, ivy.NativeArray], /, *, out: Optional[ivy.Array] = None
+):
"""
Return PyCapsule Object.
@@ -1719,16 +1734,16 @@ def to_dlpack(x: ivy.Array | ivy.NativeArray, /, *, out: ivy.Array | None = None
@handle_backend_invalid
def from_dlpack(
- x: ivy.Array | ivy.NativeArray, /, *, out: ivy.Array | None = None
+ x: Union[ivy.Array, ivy.NativeArray], /, *, out: Optional[ivy.Array] = None
) -> ivy.Array:
"""
Return a new array containing the data from another (array) object with a
- ``__dlpack__`` method.
+ ``__dlpack__`` method or PyCapsule Object.
Parameters
----------
x object
- input (array) object.
+ input (array) object with a ``__dlpack__`` method or PyCapsule Object.
out
optional output array, for writing the result to. It must have a shape that the
inputs broadcast to.
@@ -1773,11 +1788,11 @@ def from_dlpack(
@handle_array_function
@handle_device
def copy_array(
- x: ivy.Array | ivy.NativeArray,
+ x: Union[ivy.Array, ivy.NativeArray],
/,
*,
to_ivy_array: bool = True,
- out: ivy.Array | None = None,
+ out: Optional[ivy.Array] = None,
) -> ivy.Array:
"""
Copy an array.
@@ -1879,11 +1894,11 @@ def copy_array(
@handle_backend_invalid
@handle_array_like_without_promotion
def native_array(
- x: ivy.Array | ivy.NativeArray | list[Number] | tuple[Number] | np.ndarray,
+ x: Union[ivy.Array, ivy.NativeArray, List[Number], Tuple[Number], np.ndarray],
/,
*,
- dtype: ivy.Dtype | ivy.NativeDtype | None = None,
- device: ivy.Device | ivy.NativeDevice | None = None,
+ dtype: Optional[Union[ivy.Dtype, ivy.NativeDtype]] = None,
+ device: Optional[Union[ivy.Device, ivy.NativeDevice]] = None,
) -> ivy.NativeArray:
"""
Convert the input to a native array.
@@ -1939,16 +1954,16 @@ def native_array(
@handle_array_function
@handle_device
def one_hot(
- indices: ivy.Array | ivy.NativeArray,
+ indices: Union[ivy.Array, ivy.NativeArray],
depth: int,
/,
*,
- on_value: Number | None = None,
- off_value: Number | None = None,
- axis: int | None = None,
- dtype: ivy.Dtype | ivy.NativeDtype | None = None,
- device: ivy.Device | ivy.NativeDevice = None,
- out: ivy.Array | None = None,
+ on_value: Optional[Number] = None,
+ off_value: Optional[Number] = None,
+ axis: Optional[int] = None,
+ dtype: Optional[Union[ivy.Dtype, ivy.NativeDtype]] = None,
+ device: Union[ivy.Device, ivy.NativeDevice] = None,
+ out: Optional[ivy.Array] = None,
) -> ivy.Array:
"""
Return a one-hot array. The locations represented by indices in the parameter
@@ -2054,17 +2069,17 @@ def one_hot(
@infer_dtype
@handle_device
def logspace(
- start: ivy.Array | ivy.NativeArray | float,
- stop: ivy.Array | ivy.NativeArray | float,
+ start: Union[ivy.Array, ivy.NativeArray, float],
+ stop: Union[ivy.Array, ivy.NativeArray, float],
/,
num: int,
*,
base: float = 10.0,
axis: int = 0,
endpoint: bool = True,
- dtype: ivy.Dtype | ivy.NativeDtype | None = None,
- device: ivy.Device | ivy.NativeDevice | None = None,
- out: ivy.Array | None = None,
+ dtype: Optional[Union[ivy.Dtype, ivy.NativeDtype]] = None,
+ device: Optional[Union[ivy.Device, ivy.NativeDevice]] = None,
+ out: Optional[ivy.Array] = None,
) -> ivy.Array:
"""
Generate a certain number of evenly-spaced values in log space, in an interval along
@@ -2167,9 +2182,9 @@ def logspace(
@outputs_to_ivy_arrays
def frombuffer(
buffer: bytes,
- dtype: ivy.Dtype | ivy.NativeDtype | None = None,
- count: int | None = -1,
- offset: int | None = 0,
+ dtype: Optional[Union[ivy.Dtype, ivy.NativeDtype]] = None,
+ count: Optional[int] = -1,
+ offset: Optional[int] = 0,
) -> ivy.Array:
r"""
Interpret a buffer as a 1-dimensional array.
@@ -2231,12 +2246,12 @@ def frombuffer(
@handle_device
def triu_indices(
n_rows: int,
- n_cols: int | None = None,
+ n_cols: Optional[int] = None,
k: int = 0,
/,
*,
- device: ivy.Device | ivy.NativeDevice | None = None,
-) -> tuple[ivy.Array]:
+ device: Optional[Union[ivy.Device, ivy.NativeDevice]] = None,
+) -> Tuple[ivy.Array]:
"""
Return the indices of the upper triangular part of a row by col matrix in a 2-by-N
shape (tuple of two N dimensional arrays), where the first row contains row
diff --git a/ivy/functional/ivy/data_type.py b/ivy/functional/ivy/data_type.py
index 5a052a25b8bff..e0d4e592068f1 100644
--- a/ivy/functional/ivy/data_type.py
+++ b/ivy/functional/ivy/data_type.py
@@ -24,7 +24,6 @@
handle_backend_invalid,
)
from ivy.utils.exceptions import handle_exceptions
-from collections.abc import Hashable
# Helpers #
@@ -43,9 +42,8 @@ def _is_valid_dtypes_attributes(fn: Callable) -> bool:
and backend_str in fn_unsupported_dtypes
):
return False
- else:
- if isinstance(fn_unsupported_dtypes, tuple):
- return False
+ elif isinstance(fn_unsupported_dtypes, tuple):
+ return False
return True
@@ -182,6 +180,26 @@ def _nested_get(f, base_set, merge_fn, get_fn, wrapper=set):
return out
+# allow passing "integer" if all integer dtypes are supported/unsupported for e.g.
+def _expand_typesets(dtypes):
+ typesets = {
+ "valid": ivy.valid_dtypes,
+ "numeric": ivy.valid_numeric_dtypes,
+ "float": ivy.valid_float_dtypes,
+ "integer": ivy.valid_int_dtypes,
+ "unsigned": ivy.valid_uint_dtypes,
+ "complex": ivy.valid_complex_dtypes,
+ }
+ dtypes = list(dtypes)
+ typeset_list = []
+ for i, dtype in reversed(list(enumerate(dtypes))):
+ if dtype in typesets:
+ typeset_list.extend(typesets[dtype])
+ dtypes.pop(i)
+ dtypes += typeset_list
+ return dtypes
+
+
# Get the list of dtypes supported by the function
# by default returns the supported dtypes
def _get_dtypes(fn, complement=True):
@@ -206,16 +224,6 @@ def _get_dtypes(fn, complement=True):
("unsupported_dtypes", set.difference, ivy.invalid_dtypes),
]
- # allow passing "integer" if all integer dtypes are supported/unsupported for e.g.
- typesets = {
- "valid": ivy.valid_dtypes,
- "numeric": ivy.valid_numeric_dtypes,
- "float": ivy.valid_float_dtypes,
- "integer": ivy.valid_int_dtypes,
- "unsigned": ivy.valid_uint_dtypes,
- "complex": ivy.valid_complex_dtypes,
- }
-
for key, merge_fn, base in basic:
if hasattr(fn, key):
dtypes = getattr(fn, key)
@@ -223,13 +231,9 @@ def _get_dtypes(fn, complement=True):
if isinstance(dtypes, dict):
dtypes = dtypes.get(ivy.current_backend_str(), base)
ivy.utils.assertions.check_isinstance(dtypes, tuple)
- dtypes = list(dtypes)
- typeset_list = []
- for i, dtype in reversed(list(enumerate(dtypes))):
- if dtype in typesets:
- typeset_list.extend(typesets[dtype])
- dtypes.pop(i)
- dtypes = dtypes + typeset_list
+ if not dtypes:
+ dtypes = base
+ dtypes = _expand_typesets(dtypes)
supported = merge_fn(supported, set(dtypes))
if complement:
@@ -604,7 +608,7 @@ def finfo(
Returns
-------
ret
- an object having the followng attributes:
+ an object having the following attributes:
- **bits**: *int*
@@ -819,11 +823,11 @@ def result_type(
# Extra #
# ------#
-default_dtype_stack = list()
-default_float_dtype_stack = list()
-default_int_dtype_stack = list()
-default_uint_dtype_stack = list()
-default_complex_dtype_stack = list()
+default_dtype_stack = []
+default_float_dtype_stack = []
+default_int_dtype_stack = []
+default_uint_dtype_stack = []
+default_complex_dtype_stack = []
class DefaultDtype:
@@ -962,7 +966,15 @@ def is_hashable_dtype(dtype_in: Union[ivy.Dtype, ivy.NativeDtype], /) -> bool:
ret
True if data type is hashable else False
"""
- return isinstance(dtype_in, Hashable)
+ # Doing something like isinstance(dtype_in, collections.abc.Hashable)
+ # fails where the `__hash__` method is overridden to simply raise an
+ # exception.
+ # [See `tensorflow.python.trackable.data_structures.ListWrapper`]
+ try:
+ hash(dtype_in)
+ return True
+ except TypeError:
+ return False
@handle_exceptions
@@ -1823,19 +1835,13 @@ def is_bool_dtype(
elif isinstance(dtype_in, np.ndarray):
return "bool" in dtype_in.dtype.name
elif isinstance(dtype_in, Number):
- return (
- True
- if isinstance(dtype_in, (bool, np.bool)) and not isinstance(dtype_in, bool)
- else False
- )
+ return isinstance(dtype_in, (bool, np.bool_)) and not isinstance(dtype_in, bool)
elif isinstance(dtype_in, (list, tuple, dict)):
- return (
- True
- if ivy.nested_argwhere(
+ return bool(
+ ivy.nested_argwhere(
dtype_in,
- lambda x: isinstance(x, (bool, np.bool)) and x is not int,
+ lambda x: isinstance(x, (bool, np.bool_)) and x is not int,
)
- else False
)
return "bool" in ivy.as_ivy_dtype(dtype_in)
@@ -1906,11 +1912,8 @@ def is_int_dtype(
elif isinstance(dtype_in, np.ndarray):
return "int" in dtype_in.dtype.name
elif isinstance(dtype_in, Number):
- return (
- True
- if isinstance(dtype_in, (int, np.integer))
- and not isinstance(dtype_in, bool)
- else False
+ return isinstance(dtype_in, (int, np.integer)) and not isinstance(
+ dtype_in, bool
)
elif isinstance(dtype_in, (list, tuple, dict)):
@@ -1920,7 +1923,7 @@ def nested_fun(x):
or (ivy.is_array(x) and "int" in ivy.dtype(x))
) and x is not bool
- return True if ivy.nested_argwhere(dtype_in, nested_fun) else False
+ return bool(ivy.nested_argwhere(dtype_in, nested_fun))
return "int" in ivy.as_ivy_dtype(dtype_in)
@@ -1979,16 +1982,14 @@ def is_float_dtype(
elif isinstance(dtype_in, np.ndarray):
return "float" in dtype_in.dtype.name
elif isinstance(dtype_in, Number):
- return True if isinstance(dtype_in, (float, np.floating)) else False
+ return isinstance(dtype_in, (float, np.floating))
elif isinstance(dtype_in, (list, tuple, dict)):
- return (
- True
- if ivy.nested_argwhere(
+ return bool(
+ ivy.nested_argwhere(
dtype_in,
lambda x: isinstance(x, (float, np.floating))
or (ivy.is_array(x) and "float" in ivy.dtype(x)),
)
- else False
)
return "float" in as_ivy_dtype(dtype_in)
@@ -2114,6 +2115,9 @@ def promote_types(
ret
The type that both input types promote to
"""
+ # in case either is of none type
+ if not (type1 and type2):
+ return type1 if type1 else type2
query = [ivy.as_ivy_dtype(type1), ivy.as_ivy_dtype(type2)]
query = tuple(query)
if query not in ivy.promotion_table:
diff --git a/ivy/functional/ivy/device.py b/ivy/functional/ivy/device.py
index 5fb9e937c1997..3e35a572b8799 100644
--- a/ivy/functional/ivy/device.py
+++ b/ivy/functional/ivy/device.py
@@ -33,17 +33,18 @@
from ivy.func_wrapper import (
handle_out_argument,
to_native_arrays_and_back,
+ inputs_to_native_arrays,
handle_nestable,
handle_array_like_without_promotion,
handle_backend_invalid,
)
from ivy.utils.exceptions import handle_exceptions
-default_device_stack = list()
-soft_device_mode_stack = list()
-dev_handles = dict()
-split_factors = dict()
-max_chunk_sizes = dict()
+default_device_stack = []
+soft_device_mode_stack = []
+dev_handles = {}
+split_factors = {}
+max_chunk_sizes = {}
# Extra #
@@ -155,7 +156,7 @@ def _get_nvml_gpu_handle(device: Union[ivy.Device, ivy.NativeDevice], /) -> int:
def _shift_native_arrays_on_default_device(*args, **kwargs):
with ivy.ArrayMode(False):
- default_device = ivy.default_device(as_native=True)
+ default_device = ivy.default_device()
args, kwargs = ivy.nested_map(
lambda x: (
ivy.to_device(x, default_device)
@@ -164,7 +165,7 @@ def _shift_native_arrays_on_default_device(*args, **kwargs):
),
[args, kwargs],
)
- return args, kwargs, default_device
+ return args, kwargs, ivy.as_native_dev(default_device)
# Device Queries #
@@ -199,7 +200,7 @@ def get_all_ivy_arrays_on_dev(
{139740789224448:ivy.array([1,0,2])},
"""
device = ivy.as_ivy_dev(device)
- all_arrays = list()
+ all_arrays = []
for obj in gc.get_objects():
if (
obj is ivy.data_classes.array.array.Array
@@ -345,7 +346,7 @@ def unset_soft_device_mode() -> None:
@handle_exceptions
@handle_backend_invalid
@handle_nestable
-@to_native_arrays_and_back
+@inputs_to_native_arrays
def dev(
x: Union[ivy.Array, ivy.NativeArray], /, *, as_native: bool = False
) -> Union[ivy.Device, ivy.NativeDevice]:
@@ -509,8 +510,8 @@ def total_mem_on_dev(device: Union[ivy.Device, ivy.NativeDevice], /) -> float:
return psutil.virtual_memory().total / 1e9
else:
raise ivy.utils.exceptions.IvyException(
- 'Invalid device string input, must be on the form "gpu:idx" or "cpu", '
- "but found {}".format(device)
+ 'Invalid device string input, must be on the form "gpu:idx" or "cpu", but'
+ f" found {device}"
)
@@ -569,8 +570,8 @@ def used_mem_on_dev(
return (vm.total - vm.available) / 1e9
else:
raise ivy.utils.exceptions.IvyException(
- 'Invalid device string input, must be on the form "gpu:idx" or "cpu", '
- "but found {}".format(device)
+ 'Invalid device string input, must be on the form "gpu:idx" or "cpu", but'
+ f" found {device}"
)
@@ -630,8 +631,8 @@ def percent_used_mem_on_dev(
return (1 - (vm.available / vm.total)) * 100
else:
raise ivy.utils.exceptions.IvyException(
- 'Invalid device string input, must be on the form "gpu:idx" or "cpu", '
- "but found {}".format(device)
+ 'Invalid device string input, must be on the form "gpu:idx" or "cpu", but'
+ f" found {device}"
)
@@ -639,7 +640,10 @@ def percent_used_mem_on_dev(
@handle_exceptions
-def dev_util(device: Union[ivy.Device, ivy.NativeDevice], /) -> float:
+def dev_util(
+ device: Union[ivy.Device, ivy.NativeDevice],
+ /,
+) -> float:
"""
Get the current utilization (%) for a given device.
@@ -673,8 +677,8 @@ def dev_util(device: Union[ivy.Device, ivy.NativeDevice], /) -> float:
return pynvml.nvmlDeviceGetUtilizationRates(handle).gpu
else:
raise ivy.utils.exceptions.IvyException(
- 'Invalid device string input, must be on the form "gpu:idx" or "cpu", '
- "but found {}".format(device)
+ 'Invalid device string input, must be on the form "gpu:idx" or "cpu", but'
+ f" found {device}"
)
@@ -1088,9 +1092,7 @@ def split_func_call(
max_chunk_size = max_chunk_sizes[shape_key]
else:
max_chunk_size = 0
- max_dim = max(
- [inp.cont_shape[inp_ax] for inp, inp_ax in zip(inputs, input_axes)]
- )
+ max_dim = max(inp.cont_shape[inp_ax] for inp, inp_ax in zip(inputs, input_axes))
if max_dim > max_chunk_size:
max_chunk_sizes[shape_key] = max_dim
max_chunk_size = max_dim
@@ -1150,7 +1152,7 @@ def split_func_call(
return sums_or_means[0] if len(sums_or_means) == 1 else tuple(sums_or_means)
rets = [func(*i) for i in zip(*inputs_split)]
rets = [
- tuple([post_fn(r) for r in ret]) if isinstance(ret, tuple) else (post_fn(ret),)
+ tuple(post_fn(r) for r in ret) if isinstance(ret, tuple) else (post_fn(ret),)
for ret in rets
]
num_outputs = len(rets[0])
@@ -1177,9 +1179,8 @@ def _is_valid_devices_attributes(fn: Callable) -> bool:
and backend_str in fn_unsupported_devices
):
return False
- else:
- if isinstance(fn_unsupported_devices, tuple):
- return False
+ elif isinstance(fn_unsupported_devices, tuple):
+ return False
return True
@@ -1198,7 +1199,7 @@ def _get_devices(fn: Callable, complement: bool = True) -> Tuple:
supported = set(all_devices).difference(supported)
return supported
- # Their values are formated like either
+ # Their values are formatted like either
# 1. fn.supported_devices = ("cpu",)
# Could also have the "all" value for the framework
basic = [
diff --git a/ivy/functional/ivy/elementwise.py b/ivy/functional/ivy/elementwise.py
index b06424ef20d04..6a250ecb3053f 100644
--- a/ivy/functional/ivy/elementwise.py
+++ b/ivy/functional/ivy/elementwise.py
@@ -123,7 +123,6 @@ def abs(
b: ivy.array([4.5, 5.3, 0., 2.3])
}
"""
-
return ivy.current_backend(x).abs(x, out=out)
@@ -421,7 +420,7 @@ def add(
For complex floating-point operands, the real valued floating-point
special cases must independently apply to the real and
- imaginary component operation invloving real numbers as
+ imaginary component operation involving real numbers as
described in the above table. For example, let ``a = real(x1_i)``,
``c = real(x2_i)``, ``d = imag(x2_i)``,
and
@@ -515,7 +514,7 @@ def asin(
"""
Calculate an implementation-dependent approximation of the principal value of the
inverse sine, having domain ``[-1, +1]`` and codomain ``[-Ο/2, +Ο/2]`` for each
- element ``x_i`` of the input array ``x``. Each element-wise result is expressed in
+ element ``x_i`` of the input array ``x``. Each element- wise result is expressed in
radians.
**Special cases**
@@ -4212,7 +4211,7 @@ def log2(
- If ``x_i`` is ``1``, the result is ``+0``.
- If ``x_i`` is ``+infinity``, the result is ``+infinity``.
- For complex floating-point operands, special cases must be hanled as if
+ For complex floating-point operands, special cases must be handled as if
the operation is implemented using the standard change of base formula
.. math::
@@ -4246,6 +4245,37 @@ def log2(
Both the description and the type hints above assumes an array input for simplicity,
but this function is *nestable*, and therefore also accepts :class:`ivy.Container`
instances in place of any of the arguments.
+
+ Examples
+ --------
+ With :class:`ivy.Array` input:
+ >>> x = ivy.array([5.0, 1, -0.0, -6.0])
+ >>> y = ivy.log2(x)
+ >>> print(y)
+ ivy.array([2.32, 0., -inf, nan])
+ >>> x = ivy.array([[float('nan'), 1, 6.0, float('+inf')],
+ ... [+0, -2.0, -7, float('-inf')]])
+ >>> y = ivy.empty_like(x)
+ >>> ivy.log2(x, out=y)
+ >>> print(y)
+ ivy.array([[nan, 0., 2.58, inf],[inf, nan, nan, nan]])
+ >>> x = ivy.array([[float('nan'), 1, 7.0, float('+inf')],
+ ... [+0, -3.0, -8, float('-inf')]])
+ >>> ivy.log2(x, out=x)
+ >>> print(x)
+ ivy.array([[nan, 0., 2.81, inf],[inf, nan, nan, nan]])
+
+ With :class:`ivy.Container` input:
+ >>> x = ivy.Container(a=ivy.array([0.0, float('nan')]),
+ ... b=ivy.array([-0., -4.9, float('+inf')]),
+ ... c=ivy.array([8.9, 2.1, 1.]))
+ >>> y = ivy.log2(x)
+ >>> print(y)
+ {
+ a: ivy.array([-inf, nan]),
+ b: ivy.array([-inf, nan, inf]),
+ c: ivy.array([3.15, 1.07, 0.])
+ }
"""
return ivy.current_backend(x).log2(x, out=out)
@@ -4965,7 +4995,7 @@ def not_equal(
and ``x1_i`` does not equal ``x2_i``, the result is ``True``.
- In the remaining cases, the result is ``False``.
- For omplex floating-point operands, let ``a = real(x1_i)``, ``b = imag(x1_i)``,
+ For complex floating-point operands, let ``a = real(x1_i)``, ``b = imag(x1_i)``,
``c = real(x2_i)``, ``d = imag(x2_i)``, and
- If ``a``, ``b``, ``c``, or ``d`` is ``NaN``, the result is ``True``.
diff --git a/ivy/functional/ivy/experimental/activations.py b/ivy/functional/ivy/experimental/activations.py
index 55cd06ff449d2..fe15ccc31721f 100644
--- a/ivy/functional/ivy/experimental/activations.py
+++ b/ivy/functional/ivy/experimental/activations.py
@@ -63,7 +63,7 @@ def logit(
x
Input data.
eps
- When eps is None the function outpus NaN where x < 0 or x > 1.
+ When eps is None the function outputs NaN where x < 0 or x > 1.
and inf or -inf where x = 1 or x = 0, respectively.
Otherwise if eps is defined, x is clamped to [eps, 1 - eps]
complex_mode
@@ -143,10 +143,8 @@ def prelu(
n = 0
for d in x.shape:
if d == dim:
- new_shape.append(d)
n += 1
- else:
- new_shape.append(d)
+ new_shape.append(d)
if n == 1:
xs = x * slope.reshape(tuple(new_shape), out=out)
return ivy.where(x > 0, x, xs, out=out)
@@ -586,3 +584,395 @@ def hardtanh(
}
"""
return current_backend(x).hardtanh(x, max_val=max_val, min_val=min_val, out=out)
+
+
+@handle_exceptions
+@handle_backend_invalid
+@handle_nestable
+@handle_array_like_without_promotion
+@handle_out_argument
+@to_native_arrays_and_back
+@handle_array_function
+def tanhshrink(
+ x: Union[ivy.Array, ivy.NativeArray], /, *, out: Optional[ivy.Array] = None
+) -> ivy.Array:
+ """
+ Apply the tanhshrink function element-wise.
+
+ Parameters
+ ----------
+ x
+ input array.
+ out
+ optional output array, for writing the result to. It must have a shape that the
+ inputs broadcast to.
+
+ Returns
+ -------
+ ret
+ an array containing the tanhshrink activation of each element in ``x``.
+
+ Examples
+ --------
+ With :class:`ivy.Array` input:
+
+ >>> x = ivy.array([-1.0, 1.0, 2.0])
+ >>> y = ivy.tanhshrink(x)
+ >>> print(y)
+ ivy.array([-0.23840582, 0.23840582, 1.03597236])
+
+ >>> x = ivy.array([-1.0, 1.0, 2.0])
+ >>> y = x.tanhshrink()
+ >>> print(y)
+ ivy.array([-0.23840582, 0.23840582, 1.03597236])
+
+
+ >>> x = ivy.array([[-1.3, 3.8, 2.1], [1.7, 4.2, -6.6]])
+ >>> y = ivy.tanhshrink(x)
+ >>> print(y)
+ ivy.array([[-0.43827677, 2.80100036, 1.12954807],
+ [ 0.76459098, 3.20044947, -5.60000372]])
+ """
+ return current_backend(x).tanhshrink(x, out=out)
+
+
+@handle_exceptions
+@handle_backend_invalid
+@handle_nestable
+@handle_array_like_without_promotion
+@handle_out_argument
+@to_native_arrays_and_back
+@handle_array_function
+def softshrink(
+ x: Union[ivy.Array, ivy.NativeArray],
+ /,
+ *,
+ lambd: float = 0.5,
+ out: Optional[ivy.Array] = None,
+) -> ivy.Array:
+ """
+ Apply the softshrink function element-wise.
+
+ Parameters
+ ----------
+ x
+ input array.
+ lambd
+ the value of the lower bound of the linear region range.
+ out
+ optional output array, for writing the result to. It must have a shape that the
+ inputs broadcast to.
+
+ Returns
+ -------
+ ret
+ an array containing the softshrink activation of each element in ``x``.
+
+ Examples
+ --------
+ With :class:`ivy.Array` input:
+ >>> x = ivy.array([-1.0, 1.0, 2.0])
+ >>> y = ivy.softshrink(x)
+ >>> print(y)
+ ivy.array([-0.5, 0.5, 1.5])
+
+ >>> x = ivy.array([-1.0, 1.0, 2.0])
+ >>> y = x.softshrink()
+ >>> print(y)
+ ivy.array([-0.5, 0.5, 1.5])
+
+
+ >>> x = ivy.array([[-1.3, 3.8, 2.1], [1.7, 4.2, -6.6]])
+ >>> y = ivy.softshrink(x)
+ >>> print(y)
+ ivy.array([[-0.79999995, 3.29999995, 1.59999991],
+ [ 1.20000005, 3.69999981, -6.0999999 ]])
+ """
+ return current_backend(x).softshrink(x, lambd=lambd, out=out)
+
+
+def _celu_jax_like(
+ x: Union[ivy.Array, ivy.NativeArray],
+ /,
+ *,
+ fn_original: Optional[Callable] = None,
+ alpha: float = 1.0,
+ out: Optional[ivy.Array] = None,
+) -> ivy.Array:
+ # implementation of max(0, x) for complex numbers
+ complex_max = ivy.where(
+ (
+ ivy.logical_or(
+ ivy.real(x) < 0, ivy.logical_and(ivy.real(x) == 0, ivy.imag(x) < 0)
+ )
+ ),
+ ivy.astype(0.0, x.dtype),
+ x,
+ )
+
+ # implementation of min(0, x) for complex numbers
+ complex_min = ivy.where(
+ (
+ ivy.logical_or(
+ ivy.real(x) < 0, ivy.logical_and(ivy.real(x) == 0, ivy.imag(x) < 0)
+ )
+ ),
+ x,
+ ivy.astype(0.0, x.dtype),
+ )
+ return complex_max + alpha * ivy.expm1(complex_min / alpha)
+
+
+@handle_exceptions
+@handle_backend_invalid
+@handle_nestable
+@handle_array_like_without_promotion
+@handle_out_argument
+@to_native_arrays_and_back
+@handle_device
+def threshold(
+ x: Union[ivy.Array, ivy.NativeArray],
+ /,
+ *,
+ threshold: float,
+ value: float,
+ out: Optional[ivy.Array] = None,
+) -> ivy.Array:
+ """
+ Apply the threshold function element-wise.
+
+ Parameters
+ ----------
+ x
+ input array.
+ threshold
+ The value to threshold at.
+ value
+ The value to replace with.
+ out
+ optional output array, for writing the result to. It must have a shape that the
+ inputs broadcast to.
+
+ Returns
+ -------
+ ret
+ an array containing the threshold activation of each element in ``x``.
+
+ Examples
+ --------
+ With :class:`ivy.Array` input:
+ >>> x = ivy.array([-1.0, 1.0, 2.0])
+ >>> y = ivy.threshold(x,value=0.0, threshold=1.5)
+ >>> print(y)
+ ivy.array([0., 0., 2.])
+
+ >>> x = ivy.array([-1.0, 1.0, 2.0])
+ >>> x.threshold(value=0.0, threshold=1.5)
+ >>> print(y)
+ ivy.array([0., 0., 2.])
+
+
+ >>> x = ivy.array([[-1.3, 3.8, 2.1], [1.7, 4.2, -6.6]])
+ >>> y = ivy.threshold(x, value=0.0, threshold=1.5)
+ >>> print(y)
+ ivy.array([[0. , 3.79999995, 2.0999999 ],
+ [1.70000005, 4.19999981, 0. ]])
+ """
+ return current_backend(x).threshold(x, threshold=threshold, value=value, out=out)
+
+
+@handle_exceptions
+@handle_backend_invalid
+@handle_nestable
+@handle_array_like_without_promotion
+@handle_out_argument
+@to_native_arrays_and_back
+@handle_array_function
+@handle_device
+@handle_complex_input
+def celu(
+ x: Union[ivy.Array, ivy.NativeArray],
+ /,
+ *,
+ alpha: float = 1.0,
+ complex_mode: Literal["split", "magnitude", "jax"] = "jax",
+ out: Optional[ivy.Array] = None,
+) -> ivy.Array:
+ """
+ Apply the Continously Differentiable Exponential Linear Unit (CELU) activation
+ function to each element of the input.
+
+ Parameters
+ ----------
+ x
+ Input array.
+ alpha
+ The alpha value (negative slope) for the CELU formulation. Default is ``1.0``
+ complex_mode
+ optional specifier for how to handle complex data types. See
+ ``ivy.func_wrapper.handle_complex_input`` for more detail.
+ out
+ optional output array, for writing the result to. It must have a shape that the
+ inputs broadcast to.
+
+ Returns
+ -------
+ ret
+ The input array with celu applied element-wise.
+
+
+ Examples
+ --------
+ With :class:`ivy.Array` input:
+
+ >>> x = ivy.array([0.39, -0.85])
+ >>> y = ivy.celu(x)
+ >>> y
+ ivy.array([ 0.39, -0.57])
+
+ With :class:`ivy.Container` input:
+
+ >>> x = ivy.Container(a=ivy.array([0.39, -0.85]), b=ivy.array([1., -0.2]))
+ >>> y = ivy.celu(x)
+ >>> y
+ {
+ a: ivy.array([0.38999999, -0.57]),
+ b: ivy.array([1., -0.18])
+ }
+ """
+ return current_backend(x).celu(x, alpha=alpha, out=out)
+
+
+celu.jax_like = _celu_jax_like
+
+
+@handle_exceptions
+@handle_backend_invalid
+@handle_nestable
+@handle_array_like_without_promotion
+@handle_out_argument
+@to_native_arrays_and_back
+@handle_array_function
+def scaled_tanh(
+ x: Union[ivy.Array, ivy.NativeArray],
+ /,
+ *,
+ alpha: float = 1.7159,
+ beta: float = 0.67,
+ out: Optional[ivy.Array] = None,
+) -> ivy.Array:
+ """
+ Compute the scaled hyperbolic tangent (tanh) activation.
+
+ The scaled tanh activation function is defined as:
+ out = alpha * tanh(beta * x)
+
+
+ Parameters
+ ----------
+ x
+ input array.
+ alpha
+ The scaling parameter for the output.
+ Determines the amplitude of the tanh function.
+ Default: 1.7159
+ beta
+ The scaling parameter for the input.
+ Determines the slope of the tanh function.
+ Default: 0.67
+ out
+ optional output array, for writing the result to. It must have a shape that the
+ inputs broadcast to.
+
+ Returns
+ -------
+ ret
+ The input array after applying the scaled tanh activation.
+
+ Examples
+ --------
+ With :class:`ivy.Array` input:
+
+ >>> x = ivy.array([22.])
+ >>> y = ivy.scaled_tanh(x)
+ >>> y
+ ivy.array([1.71589994]))
+
+ >>> x = ivy.array([4.0, 7.0])
+ >>> y = ivy.scaled_tanh(x, alpha=1.2, beta=5)
+ >>> y
+ ivy.array([1.20000005, 1.20000005])
+
+ With :class:`ivy.Container` input:
+
+ >>> x = ivy.Container(a=ivy.array([1.2, -1.2]), b=ivy.array([4.4, -2.2]))
+ >>> y = ivy.scaled_tanh(x)
+ >>> y
+ {
+ a: ivy.array([1.14324772, -1.14324772]),
+ b: ivy.array([1.70648694, -1.54488957])
+ }
+ >>> x = ivy.Container(a=ivy.array([1.2]), b=ivy.array([4.4]))
+ >>> y = ivy.scaled_tanh(x, alpha=0.2, beta=0.5)
+ >>> y
+ {
+ a: ivy.array([0.10740992]),
+ b: ivy.array([0.19514863])
+ }
+ """
+ return current_backend(x).scaled_tanh(x, alpha=alpha, beta=beta, out=out)
+
+
+stanh = scaled_tanh
+
+
+@handle_exceptions
+@handle_backend_invalid
+@handle_nestable
+@handle_array_like_without_promotion
+@handle_out_argument
+@to_native_arrays_and_back
+@handle_array_function
+def hardshrink(
+ x: Union[ivy.Array, ivy.NativeArray],
+ /,
+ *,
+ lambd: float = 0.5,
+ out: Optional[ivy.Array] = None,
+) -> ivy.Array:
+ """
+ Apply the hardshrink function element-wise.
+
+ Parameters
+ ----------
+ x
+ input array.
+ lambd
+ the value for the Hardshrink formulation.
+ out
+ optional output array, for writing the result to. It must have a shape that the
+ inputs broadcast to.
+
+ Returns
+ -------
+ ret
+ an array containing the hardshrink activation of each element in ``x``.
+
+ Examples
+ --------
+ With :class:`ivy.Array` input:
+ >>> x = ivy.array([-1.0, 1.0, 2.0])
+ >>> y = ivy.hardshrink(x)
+ >>> print(y)
+ ivy.array([-1., 1., 2.])
+ >>> x = ivy.array([-1.0, 1.0, 2.0])
+ >>> y = x.hardshrink()
+ >>> print(y)
+ ivy.array([-0.5, 0.5, 1.5])
+ >>> x = ivy.array([[-1.3, 3.8, 2.1], [1.7, 4.2, -6.6]])
+ >>> y = ivy.hardshrink(x)
+ >>> print(y)
+ ivy.array([[-1.29999995, 3.79999995, 2.0999999 ],
+ [ 1.70000005, 4.19999981, -6.5999999 ]])
+ """
+ return current_backend(x).hardshrink(x, lambd=lambd, out=out)
diff --git a/ivy/functional/ivy/experimental/creation.py b/ivy/functional/ivy/experimental/creation.py
index 2ec7134259552..bbda425e2ccbe 100644
--- a/ivy/functional/ivy/experimental/creation.py
+++ b/ivy/functional/ivy/experimental/creation.py
@@ -515,7 +515,7 @@ def _ndenumerate(input):
for idx in _iter_product(*i):
yield idx, input[idx]
- input = ivy.array(input) if not ivy.is_ivy_array(input) else input
+ input = input if ivy.is_ivy_array(input) else ivy.array(input)
return _ndenumerate(input)
@@ -945,11 +945,12 @@ def random_parafac2(
the decomposed tensor is returned
seed
seed for generating random numbers
+
Returns
-------
ivy.Parafac2Tensor
"""
- if not all(shape[1] == shapes[0][1] for shape in shapes):
+ if any(shape[1] != shapes[0][1] for shape in shapes):
raise ValueError("All matrices must have equal number of columns.")
projection_matrices = [
@@ -1013,14 +1014,14 @@ def random_tt(
rank = list(rank)
if rank[0] != 1:
message = (
- "Provided rank[0] == {} but boundaring conditions dictatate rank[0] =="
- " rank[-1] == 1.".format(rank[0])
+ f"Provided rank[0] == {rank[0]} but boundaring conditions dictatate rank[0]"
+ " == rank[-1] == 1."
)
raise ValueError(message)
if rank[-1] != 1:
message = (
- "Provided rank[-1] == {} but boundaring conditions dictatate rank[0] =="
- " rank[-1] == 1.".format(rank[-1])
+ f"Provided rank[-1] == {rank[-1]} but boundaring conditions dictatate"
+ " rank[0] == rank[-1] == 1."
)
raise ValueError(message)
@@ -1137,3 +1138,74 @@ def mel_weight_matrix(
lower_edge_hertz,
upper_edge_hertz,
)
+
+
+# unsorted_segment_mean
+@handle_exceptions
+@handle_nestable
+@to_native_arrays_and_back
+def unsorted_segment_mean(
+ data: Union[ivy.Array, ivy.NativeArray],
+ segment_ids: Union[ivy.Array, ivy.NativeArray],
+ num_segments: Union[int, ivy.Array, ivy.NativeArray],
+) -> ivy.Array:
+ """
+ Compute the mean of elements along segments of an array. Segments are defined by an
+ integer array of segment IDs.
+
+ Parameters
+ ----------
+ data : Union[ivy.Array, ivy.NativeArray]
+ The array from which to gather values.
+
+ segment_ids : Union[ivy.Array, ivy.NativeArray]
+ Must be in the same size with the first dimension of `data`. Has to be
+ of integer data type. The index-th element of `segment_ids` array is
+ the segment identifier for the index-th element of `data`.
+
+ num_segments : Union[int, ivy.Array, ivy.NativeArray]
+ An integer or array representing the total number of distinct segment IDs.
+
+ Returns
+ -------
+ ivy.Array
+ The output array, representing the result of a segmented mean operation.
+ For each segment, it computes the mean value in `data` where `segment_ids`
+ equals to segment ID.
+ """
+ return ivy.current_backend().unsorted_segment_mean(data, segment_ids, num_segments)
+
+
+@handle_exceptions
+@handle_nestable
+@handle_array_function
+@to_native_arrays_and_back
+def polyval(
+ coeffs: Union[ivy.Array, ivy.NativeArray],
+ x: Union[ivy.Array, ivy.NativeArray],
+):
+ """
+ Evaluate and return a polynomial at specific given values.
+
+ Parameters
+ ----------
+ coeffs
+ Polynomial coefficients (including zero) from highest degree to constant term.
+ x
+ The value of the indeterminate variable at which to evaluate the polynomial.
+
+ Returns
+ -------
+ ret
+ Simplified result of substituing x in the coefficients - final value
+ of polynomial.
+
+ Examples
+ --------
+ >>> ivy.polyval([3, 0, 1], 5)
+ ivy.array(76)
+ """
+ return ivy.current_backend().polyval(
+ coeffs,
+ x,
+ )
diff --git a/ivy/functional/ivy/experimental/elementwise.py b/ivy/functional/ivy/experimental/elementwise.py
index 55747ad1089d3..09a208c346a5b 100644
--- a/ivy/functional/ivy/experimental/elementwise.py
+++ b/ivy/functional/ivy/experimental/elementwise.py
@@ -1,5 +1,5 @@
# local
-from typing import Optional, Union, Tuple, List
+from typing import Optional, Union, Tuple, List, Sequence
from numbers import Number
import ivy
from ivy.func_wrapper import (
@@ -17,6 +17,241 @@
from ivy.utils.exceptions import handle_exceptions
+@handle_backend_invalid
+@handle_nestable
+@handle_array_like_without_promotion
+@handle_out_argument
+@to_native_arrays_and_back
+@handle_array_function
+@handle_device
+def amax(
+ x: Union[ivy.Array, ivy.NativeArray],
+ /,
+ *,
+ axis: Optional[Union[int, Sequence[int]]] = None,
+ keepdims: bool = False,
+ out: Optional[ivy.Array] = None,
+) -> ivy.Array:
+ """
+ Calculate the maximum value of the input array ``x``.
+
+ .. note::
+ ``amax`` is an alias of ``max`` and both function
+ behaves similarly in every backend except PyTorch and PaddlePaddle
+ (see `PyTorch's amax function
+ documentation`_`)
+ (see `PaddlePaddle's amax function documentation`_`)
+
+ .. note::
+ When the number of elements over which to compute the maximum value is zero, the
+ maximum value is implementation-defined. Specification-compliant libraries may
+ choose to raise an error, return a sentinel value (e.g., if ``x`` is a
+ floating-point input array, return ``NaN``), or return the minimum possible
+ value for the input array ``x`` data type (e.g., if ``x`` is a floating-point
+ array, return ``-infinity``).
+
+ **Special Cases**
+
+ For floating-point operands,
+
+ - If ``x_i`` is ``NaN``, the maximum value is ``NaN``
+ (i.e., ``NaN`` values propagate).
+
+ Parameters
+ ----------
+ x
+ input array. Should have a real-valued data type.
+ axis
+ axis or axes along which maximum values must be computed. By default, the
+ maximum value must be computed over the entire array. If a tuple of integers,
+ maximum values must be computed over multiple axes. Default: ``None``.
+ keepdims
+ optional boolean, if ``True``, the reduced axes (dimensions) must be included
+ in the result as singleton dimensions, and, accordingly, the result must be
+ compatible with the input array (see `broadcasting`_).
+ Otherwise, if ``False``, the reduced axes (dimensions)
+ must not be included in the result.
+ Default: ``False``.
+ out
+ optional output array, for writing the result to.
+
+ Returns
+ -------
+ ret
+ if the maximum value was computed over the entire array, a zero-dimensional
+ array containing the maximum value; otherwise, a non-zero-dimensional array
+ containing the maximum values. The returned array must have the same data type
+ as ``x``.
+
+
+ This function conforms to the `Array API Standard
+ `_. This docstring is an extension of the
+ `docstring `_
+ in the standard.
+
+ Both the description and the type hints above assumes an array input for simplicity,
+ but this function is *nestable*, and therefore also accepts :class:`ivy.Container`
+ instances in place of any of the arguments.
+
+ Examples
+ --------
+ With :class:`ivy.Array` input:
+
+ >>> x = ivy.array([1, 2, 3])
+ >>> y = ivy.amax(x)
+ >>> print(y)
+ ivy.array(3)
+
+ >>> x = ivy.array([0, 1, 2])
+ >>> z = ivy.array([0, 0, 0])
+ >>> y = ivy.amax(x, out=z)
+ >>> print(z)
+ ivy.array(2)
+
+ >>> x = ivy.array([[0, 1, 2], [4, 6, 10]])
+ >>> y = ivy.amax(x, axis=0, keepdims=True)
+ >>> print(y)
+ ivy.array([[4, 6, 10]])
+
+ >>> x = ivy.native_array([[0, 1, 2], [4, 6, 10]])
+ >>> y = ivy.amax(x)
+ >>> print(y)
+ ivy.array(10)
+
+ With :class:`ivy.Container` input:
+
+ >>> x = ivy.Container(a=ivy.array([1, 2, 3]), b=ivy.array([2, 3, 4]))
+ >>> y = ivy.amax(x)
+ >>> print(y)
+ {
+ a: ivy.array(3),
+ b: ivy.array(4)
+ }
+ """
+ return ivy.current_backend(x).amax(x, axis=axis, keepdims=keepdims, out=out)
+
+
+@handle_backend_invalid
+@handle_nestable
+@handle_array_like_without_promotion
+@handle_out_argument
+@to_native_arrays_and_back
+@handle_array_function
+@handle_device
+def amin(
+ x: Union[ivy.Array, ivy.NativeArray],
+ /,
+ *,
+ axis: Optional[Union[int, Sequence[int]]] = None,
+ keepdims: bool = False,
+ out: Optional[ivy.Array] = None,
+) -> ivy.Array:
+ """
+ Calculate the minimum value of the input array ``x``.
+
+ .. note::
+ ``amin`` is an alias of ``min`` and both function
+ behaves similarly in every backend except PyTorch and PaddlePaddle
+ (see `PyTorch's amin function
+ documentation`_`)
+ (see `PaddlePaddle's amin function documentation`_`)
+
+ .. note::
+ When the number of elements over which to compute the minimum value is zero, the
+ minimum value is implementation-defined. Specification-compliant libraries may
+ choose to raise an error, return a sentinel value (e.g., if ``x`` is a
+ floating-point input array, return ``NaN``), or return the maximum possible value
+ for the input array ``x`` data type (e.g., if ``x`` is a floating-point array,
+ return ``+infinity``).
+
+ **Special Cases**
+
+ For floating-point operands,
+
+ - If ``x_i`` is ``NaN``, the minimum value is ``NaN``
+ (i.e., ``NaN`` values propagate).
+
+ Parameters
+ ----------
+ x
+ input array. Should have a real-valued data type.
+ axis
+ axis or axes along which minimum values must be computed. By default, the
+ minimum value must be computed over the entire array. If a tuple of integers,
+ minimum values must be computed over multiple axes. Default: ``None``.
+
+ keepdims
+ optional boolean, if ``True``, the reduced axes (dimensions) must be included
+ in the result as singleton dimensions, and, accordingly, the result must be
+ compatible with the input array (see `broadcasting`_).
+ Otherwise, if ``False``, the reduced axes (dimensions)
+ must not be included in the result.
+ Default: ``False``.
+ out
+ optional output array, for writing the result to.
+
+ Returns
+ -------
+ ret
+ if the minimum value was computed over the entire array, a zero-dimensional
+ array containing the minimum value; otherwise, a non-zero-dimensional array
+ containing the minimum values. The returned array must have the same data type
+ as ``x``.
+
+
+ This function conforms to the `Array API Standard
+ `_. This docstring is an extension of the
+ `docstring `_
+ in the standard.
+
+ Both the description and the type hints above assumes an array input for simplicity,
+ but this function is *nestable*, and therefore also accepts :class:`ivy.Container`
+ instances in place of any of the arguments.
+
+ Examples
+ --------
+ With :class:`ivy.Array` input:
+
+ >>> x = ivy.array([1, 2, 3])
+ >>> y = ivy.amin(x)
+ >>> print(y)
+ ivy.array(1)
+
+ >>> x = ivy.array([0, 1, 2])
+ >>> z = ivy.array([0, 0, 0])
+ >>> y = ivy.amin(x, out=z)
+ >>> print(z)
+ ivy.array(0)
+
+ >>> x = ivy.array([[0, 1, 2], [4, 6, 10]])
+ >>> y = ivy.amin(x, axis=0, keepdims=True)
+ >>> print(y)
+ ivy.array([[0, 1, 2]])
+
+ >>> x = ivy.native_array([[0, 1, 2], [4, 6, 10]])
+ >>> y = ivy.amin(x)
+ >>> print(y)
+ ivy.array(0)
+
+ With :class:`ivy.Container` input:
+
+ >>> x = ivy.Container(a=ivy.array([1, 2, 3]), b=ivy.array([2, 3, 4]))
+ >>> y = ivy.amin(x)
+ >>> print(y)
+ {
+ a: ivy.array(1),
+ b: ivy.array(2)
+ }
+ """
+ return ivy.current_backend(x).amin(x, axis=axis, keepdims=keepdims, out=out)
+
+
@handle_exceptions
@handle_backend_invalid
@handle_nestable
@@ -831,7 +1066,7 @@ def gradient(
Note: jax supports edge_order=1 case only
axis
dimension(s) to approximate the gradient over
- by default partial gradient is computed in every dimention
+ by default partial gradient is computed in every dimension
Returns
-------
@@ -1018,7 +1253,7 @@ def conj(
Returns
-------
ret
- an arrray of the same dtype as the input array with
+ an array of the same dtype as the input array with
the complex conjugates of the complex values present
in the input array. If x is a scalar then a scalar
will be returned.
@@ -1204,9 +1439,8 @@ def lerp(
if ivy.is_array(weight):
if ivy.dtype(weight) not in weight_allowed_types:
weight = ivy.astype(weight, "float64")
- else:
- if not isinstance(weight, float):
- weight = ivy.astype(ivy.array([weight]), "float64")
+ elif not isinstance(weight, float):
+ weight = ivy.astype(ivy.array([weight]), "float64")
return ivy.add(input, ivy.multiply(weight, ivy.subtract(end, input)), out=out)
diff --git a/ivy/functional/ivy/experimental/gradients.py b/ivy/functional/ivy/experimental/gradients.py
index e88d86c3b0f4d..8c5466de75168 100644
--- a/ivy/functional/ivy/experimental/gradients.py
+++ b/ivy/functional/ivy/experimental/gradients.py
@@ -20,3 +20,46 @@ def bind_custom_gradient_function(func, custom_grad_func):
the function
"""
return current_backend(None).bind_custom_gradient_function(func, custom_grad_func)
+
+
+def vjp(func, *primals):
+ """
+ Compute a (reverse-mode) vector-Jacobian product of `func`.
+
+ Parameters
+ ----------
+ func : callable
+ Function to be differentiated.
+ primals
+ sequence of primal values at which the Jacobian of `func` should be evaluated.
+
+ Returns
+ -------
+ ret
+ The output of `func` evaluated at `primals`. And a function from a cotangent
+ vector representing the vector-Jacobian product of fun evaluated at primals.
+ """
+ return current_backend(None).vjp(func, *primals)
+
+
+def jvp(func, primals, tangents):
+ """
+ Compute a (forward-mode) Jacobian-vector product of `func`.
+
+ Parameters
+ ----------
+ func : callable
+ Function to be differentiated.
+ primals
+ sequence of primal values at which the Jacobian of `func` should be evaluated.
+ tangents
+ sequence of tangent vectors giving the Jacobian-vector product of `func`
+ evaluated at `primals`.
+
+ Returns
+ -------
+ ret
+ The output of `func` evaluated at `primals`. And the Jacobian-vector product of
+ function evaluated at primals with tangents.
+ """
+ return current_backend(None).jvp(func, primals, tangents)
diff --git a/ivy/functional/ivy/experimental/layers.py b/ivy/functional/ivy/experimental/layers.py
index be22a45ba0966..b710032ecf31e 100644
--- a/ivy/functional/ivy/experimental/layers.py
+++ b/ivy/functional/ivy/experimental/layers.py
@@ -60,7 +60,7 @@ def max_pool1d(
indicating the per-dimension paddings. (e.g. 2, [(1, 0)])
data_format
"NWC" or "NCW". Defaults to "NWC".
- dilaton
+ dilation
The stride between elements within a sliding window, must be > 0.
ceil_mode
If True, ceil is used instead of floor to compute the output shape.
@@ -148,7 +148,7 @@ def max_pool2d(
indicating the per-dimension paddings.
data_format
NHWC" or "NCHW". Defaults to "NHWC".
- dilaton
+ dilation
The stride between elements within a sliding window, must be > 0.
ceil_mode
If True, ceil is used instead of floor to compute the output shape.
@@ -235,7 +235,7 @@ def max_pool3d(
indicating the per-dimension paddings. (e.g. 2, [(1, 0), (0, 1), (1, 1)])
data_format
"NDHWC" or "NCDHW". Defaults to "NDHWC".
- dilaton
+ dilation
The stride between elements within a sliding window, must be > 0.
ceil_mode
If True, ceil is used instead of floor to compute the output shape.
@@ -297,7 +297,7 @@ def avg_pool1d(
x: Union[ivy.Array, ivy.NativeArray],
kernel: Union[int, Tuple[int]],
strides: Union[int, Tuple[int]],
- padding: str,
+ padding: Union[str, int, List[Tuple[int, int]]],
/,
*,
data_format: str = "NWC",
@@ -380,7 +380,7 @@ def avg_pool2d(
x: Union[ivy.Array, ivy.NativeArray],
kernel: Union[int, Tuple[int], Tuple[int, int]],
strides: Union[int, Tuple[int], Tuple[int, int]],
- padding: str,
+ padding: Union[str, int, List[Tuple[int, int]]],
/,
*,
data_format: str = "NHWC",
@@ -403,7 +403,7 @@ def avg_pool2d(
The stride of the sliding window for each dimension of input.
padding
SAME" or "VALID" indicating the algorithm, or list
- indicating the per-dimensio paddings.
+ indicating the per-dimension paddings.
data_format
NHWC" or "NCHW". Defaults to "NHWC".
count_include_pad
@@ -468,7 +468,7 @@ def avg_pool3d(
x: Union[ivy.Array, ivy.NativeArray],
kernel: Union[int, Tuple[int], Tuple[int, int, int]],
strides: Union[int, Tuple[int], Tuple[int, int, int]],
- padding: str,
+ padding: Union[str, int, List[Tuple[int, int]]],
/,
*,
data_format: str = "NDHWC",
@@ -642,7 +642,7 @@ def dct(
out: Optional[Union[ivy.Array, ivy.NativeArray]] = None,
) -> Union[ivy.Array, ivy.NativeArray]:
"""
- Compute the 1D Discrete Cosine Tranformation of a given signal.
+ Compute the 1D Discrete Cosine Transformation of a given signal.
Parameters
----------
@@ -651,7 +651,7 @@ def dct(
type
The type of the dct. Must be 1, 2, 3 or 4.
n
- The lenght of the transform. If n is less than the input signal lenght,
+ The length of the transform. If n is less than the input signal length,
then x is truncated, if n is larger then x is zero-padded.
axis
The axis to compute the DCT along.
@@ -753,7 +753,7 @@ def idct(
out: Optional[Union[ivy.Array, ivy.NativeArray]] = None,
) -> Union[ivy.Array, ivy.NativeArray]:
"""
- Compute the 1D Inverse Discrete Cosine Tranformation of a given signal.
+ Compute the 1D Inverse Discrete Cosine Transformation of a given signal.
Parameters
----------
@@ -1409,9 +1409,8 @@ def _tf_area_indices(dim_index, scale):
return starting_index, ending_index, rounded_indices
-def _tf_area_interpolate(x, size, dims):
+def _tf_area_interpolate(x, size, scale, dims):
ret = ivy.zeros(x.shape[:2] + size)
- scale = ivy.divide(ivy.shape(x)[2:], size)
area = 1.0 / ivy.prod(scale)
for i, ba in enumerate(x):
for j, ch in enumerate(ba):
@@ -1487,12 +1486,11 @@ def _tf_area_interpolate(x, size, dims):
return ret
-def nearest_interpolate(x, dims, size, input_shape, exact):
+def nearest_interpolate(x, dims, size, scale, exact):
off = 0.5 if exact else 0
for d in range(dims):
- m = input_shape[d + 2]
n = size[d]
- offsets = (ivy.arange(n, dtype="float32") + off) * m / n
+ offsets = (ivy.arange(n, dtype="float32") + off) * scale[d]
offsets = ivy.astype(ivy.floor(ivy.astype(offsets, "float32")), "int32")
x = ivy.gather(x, offsets, axis=d + 2)
return x
@@ -1514,19 +1512,17 @@ def _lanczos_kernel(radius, x):
return ivy.where(ivy.bitwise_and(x >= radius, x < -radius), 0.0, out)
-def _dim_scale_factor(input_size, output_size, align_corners, scales):
- if align_corners:
- if output_size > 1:
- dim_scale_factor = (input_size - 1) / (output_size - 1)
+def _get_final_scale(input_size, output_size, align_corners, scale_factor):
+ scale = []
+ for i, (input, output) in enumerate(zip(input_size, output_size)):
+ if align_corners:
+ if output > 1:
+ scale.append((input - 1) / (output - 1))
+ else:
+ scale.append(1)
else:
- dim_scale_factor = 0.0
- else:
- dim_scale_factor = (
- input_size / (input_size * scales)
- if scales is not None
- else input_size / output_size
- )
- return dim_scale_factor
+ scale.append(1 / scale_factor[i])
+ return scale
def _mitchellcubic_kernel(x):
@@ -1542,28 +1538,17 @@ def _mitchellcubic_kernel(x):
def _compute_weight_mat(
input_size,
output_size,
- scale,
align_corners,
kernel_fn,
- antialias: bool,
- dim_scale_factor,
+ dim_scale,
):
- inv_scale = 1.0 / scale
- kernel_scale = ivy.maximum(inv_scale, 1.0) if antialias else 1.0
if not align_corners:
- sample_f = (ivy.arange(output_size) + 0.5) * dim_scale_factor - 0.5
- x = (
- ivy.abs(
- ivy.expand_dims(sample_f)
- - ivy.expand_dims(ivy.arange(input_size), axis=-1)
- )
- / kernel_scale
- )
+ sample_f = (ivy.arange(output_size) + 0.5) * dim_scale - 0.5
else:
- sample_f = ivy.arange(output_size) * dim_scale_factor
- x = ivy.abs(
- ivy.expand_dims(sample_f) - ivy.expand_dims(ivy.arange(input_size), axis=-1)
- ) / (kernel_scale)
+ sample_f = ivy.arange(output_size) * dim_scale
+ x = ivy.abs(
+ ivy.expand_dims(sample_f) - ivy.expand_dims(ivy.arange(input_size), axis=-1)
+ )
weights = kernel_fn(x)
total_weight_sum = ivy.sum(weights, axis=0, keepdims=True)
weights = ivy.where(
@@ -1611,39 +1596,31 @@ def _sum_tensors(ts):
def _upsample_bicubic2d_default(
a,
output_size,
+ scale,
align_corners,
- scale_h=None,
- scale_w=None,
):
N, C, iH, iW = a.shape
oH, oW = output_size
- def compute_scale(in_size, out_size, align_corners, scale=None):
- if align_corners:
- return (in_size - 1) / (out_size - 1) if out_size > 1 else 0
- else:
- return 1 / scale if scale is not None and scale > 0 else in_size / out_size
-
def compute_source_index(scale, dst_index, align_corners):
if align_corners:
return scale * dst_index
else:
return scale * (dst_index + 0.5) - 0.5
- height_scale = compute_scale(iH, oH, align_corners, scale_h)
- width_scale = compute_scale(iW, oW, align_corners, scale_w)
-
- N_idx = ivy.reshape(ivy.arange(N), (N, 1, 1, 1))
- C_idx = ivy.reshape(ivy.arange(C), (1, C, 1, 1))
+ N_idx = ivy.reshape(ivy.arange(N), (N, 1, 1, 1)).astype(ivy.int64)
+ C_idx = ivy.reshape(ivy.arange(C), (1, C, 1, 1)).astype(ivy.int64)
out_y = ivy.reshape(ivy.arange(oH), ((1, 1, oH, 1)))
out_x = ivy.reshape(ivy.arange(oW), ((1, 1, 1, oW)))
- real_x = compute_source_index(width_scale, out_x, align_corners)
+ scale_y, scale_x = scale
+
+ real_x = compute_source_index(scale_x, out_x, align_corners)
in_x = ivy.floor(real_x)
t_x = real_x - in_x
ix = ivy.astype(in_x, ivy.int64)
- real_y = compute_source_index(height_scale, out_y, align_corners)
+ real_y = compute_source_index(scale_y, out_y, align_corners)
in_y = ivy.floor(real_y)
t_y = real_y - in_y
iy = ivy.astype(in_y, ivy.int64)
@@ -1668,7 +1645,6 @@ def get_x_interp(y):
def area_interpolate(x, dims, size, scale):
ret = ivy.zeros(x.shape[:2] + size)
- inv_scale = ivy.divide(1.0, scale)
for i, ba in enumerate(x):
for j, ch in enumerate(ba):
if dims == 3:
@@ -1676,16 +1652,16 @@ def area_interpolate(x, dims, size, scale):
for h_dim in range(size[1]):
for w_dim in range(size[2]):
d_index = (
- int(d_dim * inv_scale[0]),
- math.ceil((d_dim + 1) * inv_scale[0]),
+ int(d_dim * scale[0]),
+ math.ceil((d_dim + 1) * scale[0]),
)
h_index = (
- int(h_dim * inv_scale[1]),
- math.ceil((h_dim + 1) * inv_scale[1]),
+ int(h_dim * scale[1]),
+ math.ceil((h_dim + 1) * scale[1]),
)
w_index = (
int(w_dim * scale[2]),
- math.ceil((w_dim + 1) * inv_scale[2]),
+ math.ceil((w_dim + 1) * scale[2]),
)
scale_z = d_index[1] - d_index[0]
scale_y = h_index[1] - h_index[0]
@@ -1702,12 +1678,12 @@ def area_interpolate(x, dims, size, scale):
for h_dim in range(size[0]):
for w_dim in range(size[1]):
h_index = (
- int(h_dim * inv_scale[0]),
- math.ceil((h_dim + 1) * inv_scale[0]),
+ int(h_dim * scale[0]),
+ math.ceil((h_dim + 1) * scale[0]),
)
w_index = (
- int(w_dim * inv_scale[1]),
- math.ceil((w_dim + 1) * inv_scale[1]),
+ int(w_dim * scale[1]),
+ math.ceil((w_dim + 1) * scale[1]),
)
scale_y = h_index[1] - h_index[0]
scale_x = w_index[1] - w_index[0]
@@ -1718,8 +1694,8 @@ def area_interpolate(x, dims, size, scale):
else:
for w_dim in range(size[0]):
w_index = (
- int(w_dim * inv_scale[0]),
- math.ceil((w_dim + 1) * inv_scale[0]),
+ int(w_dim * scale[0]),
+ math.ceil((w_dim + 1) * scale[0]),
)
scale_x = w_index[1] - w_index[0]
ret[i, j, w_dim] = ivy.sum(ch[w_index[0] : w_index[1]]) * (
@@ -1730,21 +1706,12 @@ def area_interpolate(x, dims, size, scale):
def get_interpolate_kernel(mode):
kernel_func = _triangle_kernel
- if mode == "bicubic_tensorflow":
-
- def kernel_func(inputs):
- return _cubic_kernel(inputs)
-
+ if mode == "tf_bicubic":
+ kernel_func = lambda inputs: _cubic_kernel(inputs)
elif mode == "lanczos3":
-
- def kernel_func(inputs):
- return _lanczos_kernel(3, inputs)
-
+ kernel_func = lambda inputs: _lanczos_kernel(3, inputs)
elif mode == "lanczos5":
-
- def kernel_func(inputs):
- return _lanczos_kernel(5, inputs)
-
+ kernel_func = lambda inputs: _lanczos_kernel(5, inputs)
return kernel_func
@@ -1758,26 +1725,12 @@ def generate_einsum_equation(dim):
return einsum_string
-def _interpolate_with_kernel(
- x, dims, size, scale, input_shape, align_corners, antialias, scale_factor, mode
-):
- spatial_dims = [2 + i for i in range(dims)]
+def _interpolate_with_kernel(x, dims, size, input_size, align_corners, scale, mode):
equation = generate_einsum_equation(dims)
kernel_func = get_interpolate_kernel(mode)
- output_shape = tuple(input_shape[:2]) + size
operands = []
- for i, d in enumerate(spatial_dims):
- m = input_shape[d]
- n = output_shape[d]
- dim_scale_factor = _dim_scale_factor(
- m,
- n,
- align_corners,
- scale_factor[i] if scale_factor is not None else None,
- )
- w = _compute_weight_mat(
- m, n, scale[i], align_corners, kernel_func, antialias, dim_scale_factor
- ).astype(x.dtype)
+ for m, n, s in zip(input_size, size, scale):
+ w = _compute_weight_mat(m, n, align_corners, kernel_func, s).astype(x.dtype)
operands.append(w)
return ivy.einsum(equation, x, *operands)
@@ -1801,7 +1754,7 @@ def interpolate(
"area",
"nearest_exact",
"tf_area",
- "bicubic_tensorflow",
+ "tf_bicubic",
"bicubic",
"mitchellcubic",
"lanczos3",
@@ -1810,8 +1763,8 @@ def interpolate(
] = "linear",
scale_factor: Optional[Union[Sequence[int], int]] = None,
recompute_scale_factor: Optional[bool] = None,
- align_corners: Optional[bool] = None,
- antialias: bool = False,
+ align_corners: bool = False,
+ antialias: bool = False, # ToDo: add support for antialias
out: Optional[ivy.Array] = None,
) -> ivy.Array:
"""
@@ -1836,22 +1789,23 @@ def interpolate(
- area
- tf_area
- bicubic
+ - tf_bicubic
- mitchellcubic
- lanczos3
- lanczos5
- gaussian
scale_factor
Multiplier for spatial size that defines the output size (overwriting `size`).
+ recompute_scale_factor
+ If True, then scale_factor must be provided and scale_factor is used to
+ compute the output size. The computed output size will be used to infer new
+ scales for the interpolation. If recompute_scale_factor is False, then size
+ or scale_factor will be used directly for interpolation.
align_corners
If True, the corner pixels of the input and output tensors are aligned,
and thus preserving the values at the corner pixels. If False, the corner
pixels are not aligned, and the interpolation uses edge value padding for
out-of-boundary values.
- only has an effect when mode is 'linear', 'bilinear',
- 'bicubic' or 'trilinear'. Default: False
- antialias
- If True, antialiasing is applied when downsampling an image.
- Supported modes: 'bilinear', 'bicubic'.
out
Optional output array, for writing the result to. It must
have a shape that the inputs broadcast to.
@@ -1860,132 +1814,185 @@ def interpolate(
-------
resized array
"""
- input_shape = ivy.shape(x)
- dims = len(input_shape) - 2
- size = _get_size(scale_factor, size, dims, x.shape)
- if recompute_scale_factor:
- scale_factor = None
- elif scale_factor is not None:
- scale_factor = (
- [scale_factor] * dims
- if isinstance(scale_factor, (int, float))
- else scale_factor
+ input_size = ivy.shape(x)[2:]
+ dims = len(input_size)
+ if ivy.exists(size) and ivy.exists(scale_factor):
+ raise ivy.utils.exceptions.IvyException(
+ "only one of size or scale_factor should be defined"
)
- scale_factor = (
- [scale_factor[0]] * dims
- if isinstance(scale_factor, (list, tuple)) and len(scale_factor) != dims
- else [scale_factor] * dims
+ elif ivy.exists(size) and not ivy.exists(scale_factor):
+ if isinstance(size, (list, tuple)):
+ ivy.utils.assertions.check_equal(
+ len(size),
+ dims,
+ inverse=False,
+ message=(
+ "Input and output must have the same number of spatial dimensions,"
+ f" but got input with {list(input_size)} spatial dimensions and"
+ f" output size {size}."
+ ),
+ as_array=False,
+ )
+ elif ivy.exists(scale_factor) and not ivy.exists(size):
+ if isinstance(scale_factor, (list, tuple)):
+ ivy.utils.assertions.check_equal(
+ len(scale_factor),
+ dims,
+ inverse=False,
+ message=(
+ "Input and scale_factor must have the same number of spatial"
+ f" dimensions, but got input with {list(input_size)} spatial"
+ f" dimensions and scale_factor {scale_factor}."
+ ),
+ as_array=False,
+ )
+ else:
+ raise ivy.utils.exceptions.IvyException(
+ "either size or scale_factor should be defined"
)
- scale = [ivy.divide(size[i], input_shape[i + 2]) for i in range(dims)]
- if mode in [
- "linear",
- "bilinear",
- "trilinear",
- "nd",
- "bicubic_tensorflow",
- "lanczos3",
- "lanczos5",
- ]:
- ret = _interpolate_with_kernel(
- x,
- dims,
- size,
- scale,
- input_shape,
- align_corners,
- antialias,
- scale_factor,
- mode,
+ if ivy.exists(size) and recompute_scale_factor is not None:
+ raise ivy.utils.exceptions.IvyException(
+ "recompute_scale_factor is not meaningful with an explicit size."
)
- elif mode == "bicubic":
- return _upsample_bicubic2d_default(x, size, align_corners)
- elif mode in ["nearest-exact", "nearest"]:
- ret = nearest_interpolate(x, dims, size, input_shape, mode == "nearest-exact")
- elif mode == "area":
- ret = area_interpolate(x, dims, size, scale)
- elif mode == "mitchellcubic":
- batch, channels, in_height, in_width = x.shape
- out_height, out_width = size
- scale_factor_h = out_height / in_height
- scale_factor_w = out_width / in_width
- ret = ivy.zeros((batch, channels, out_height, out_width))
- for i in range(out_height):
- for j in range(out_width):
- p_i = i / scale_factor_h
- p_j = j / scale_factor_w
- left = int(math.floor(p_j - 2))
- right = int(math.ceil(p_j + 2))
- top = int(math.floor(p_i - 2))
- bottom = int(math.ceil(p_i + 2))
- kernel_w = ivy.array(
- [
- _mitchellcubic_kernel((p_j - j) / scale_factor_w)
- for i in range(left, right)
- ]
- )
- kernel_h = ivy.array(
- [
- _mitchellcubic_kernel((p_i - i) / scale_factor_h)
- for j in range(top, bottom)
- ]
- )
- left_pad = max(0, -left)
- right_pad = max(0, right - in_width)
- top_pad = max(0, -top)
- bottom_pad = max(0, bottom - in_height)
- pad_width = [(0, 0), (0, 0)] * (len(x.shape) - 3) + [
- (top_pad, bottom_pad),
- (left_pad, right_pad),
- ]
- padded_x = ivy.pad(x, pad_width, mode="edge")
- for b in range(batch):
- for c in range(channels):
- patch = padded_x[
- b,
- c,
- top + top_pad : bottom + top_pad,
- left + left_pad : right + left_pad,
+ if ivy.get_num_dims(x) != 4 and mode == "bilinear":
+ raise ivy.utils.exceptions.IvyException(
+ f"Got {x.ndim}D input, but bilinear mode needs 4D input"
+ )
+ if ivy.get_num_dims(x) != 5 and mode == "trilinear":
+ raise ivy.utils.exceptions.IvyException(
+ f"Got {x.ndim}D input, but trilinear mode needs 5D input"
+ )
+ if ivy.get_num_dims(x) != 3 and mode == "linear":
+ raise ivy.utils.exceptions.IvyException(
+ f"Got {x.ndim}D input, but trilinear mode needs 3D input"
+ )
+ size, scale_factor = _get_size(scale_factor, size, dims, input_size)
+ ivy.utils.assertions.check_true(
+ all(s > 0 for s in size),
+ message=f"output sizes should be greater than 0, but got {size}",
+ )
+ if all(a == b for a, b in zip(size, input_size)):
+ ret = x
+ else:
+ if recompute_scale_factor:
+ scale_factor = [ivy.divide(size[i], input_size[i]) for i in range(dims)]
+ else:
+ scale_factor = [
+ 1 if input_size[i] == size[i] else scale_factor[i] for i in range(dims)
+ ]
+ scale = _get_final_scale(input_size, size, align_corners, scale_factor)
+ if mode in [
+ "linear",
+ "bilinear",
+ "trilinear",
+ "nd",
+ "tf_bicubic",
+ "lanczos3",
+ "lanczos5",
+ ]:
+ ret = _interpolate_with_kernel(
+ x,
+ dims,
+ size,
+ input_size,
+ align_corners,
+ scale,
+ mode,
+ )
+ elif mode == "bicubic":
+ ret = _upsample_bicubic2d_default(x, size, scale, align_corners)
+ elif mode in ["nearest-exact", "nearest"]:
+ ret = nearest_interpolate(x, dims, size, scale, mode == "nearest-exact")
+ elif mode == "area":
+ ret = area_interpolate(x, dims, size, scale)
+ elif mode == "mitchellcubic":
+ batch, channels, in_height, in_width = x.shape
+ out_height, out_width = size
+ scale_h, scale_w = scale
+ ret = ivy.zeros((batch, channels, out_height, out_width))
+ for i in range(out_height):
+ for j in range(out_width):
+ p_i = i * scale_h
+ p_j = j * scale_w
+ left = int(math.floor(p_j - 2))
+ right = int(math.ceil(p_j + 2))
+ top = int(math.floor(p_i - 2))
+ bottom = int(math.ceil(p_i + 2))
+ kernel_w = ivy.array(
+ [
+ _mitchellcubic_kernel((p_j - j) * scale_w)
+ for i in range(left, right)
]
- ret[b, c, i, j] = ivy.sum(
- kernel_h[:, ivy.newaxis] * patch * kernel_w[ivy.newaxis, :]
- )
- elif mode == "gaussian":
- ratio_h = size[0] / x.shape[-2]
- ratio_w = size[1] / x.shape[-1]
- sigma = max(1 / ratio_h, 1 / ratio_w) * 0.5
- kernel_size = 2 * int(math.ceil(3 * sigma)) + 1
- kernel_h = ivy.zeros((kernel_size,), dtype=x.dtype)
- kernel_w = ivy.zeros((kernel_size,), dtype=x.dtype)
- for i in range(kernel_h.size):
- kernel_h[i] = ivy.exp(-0.5 * ((i - kernel_h.size // 2) / sigma) ** 2)
- kernel_w[i] = ivy.exp(-0.5 * ((i - kernel_w.size // 2) / sigma) ** 2)
- kernel_h /= ivy.sum(kernel_h)
- kernel_w /= ivy.sum(kernel_w)
- pad_width = [(0, 0), (0, 0)] * (len(x.shape) - 3) + [
- (int(math.ceil(3 * sigma)), int(math.ceil(3 * sigma))),
- (int(math.ceil(3 * sigma)), int(math.ceil(3 * sigma))),
- ]
- padded_x = ivy.pad(x, pad_width, mode="constant")
- output_shape = x.shape[:2] + size
- ret = ivy.zeros(output_shape, dtype=x.dtype)
- for i in range(size[0]):
- for j in range(size[1]):
- p_i = int(math.floor(i / ratio_h + int(math.ceil(3 * sigma))))
- p_j = int(math.floor(j / ratio_w + int(math.ceil(3 * sigma))))
- for b in range(x.shape[0]):
- for c in range(x.shape[1]):
- patch = padded_x[
- b,
- c,
- p_i - kernel_size // 2 : p_i + kernel_size // 2 + 1,
- p_j - kernel_size // 2 : p_j + kernel_size // 2 + 1,
+ )
+ kernel_h = ivy.array(
+ [
+ _mitchellcubic_kernel((p_i - i) * scale_h)
+ for j in range(top, bottom)
]
- ret[b, c, i, j] = ivy.sum(
- kernel_h[ivy.newaxis, :] * patch * kernel_w[:, ivy.newaxis]
- )
- elif mode == "tf_area":
- ret = _tf_area_interpolate(x, size, dims)
- return ivy.astype(ret, ivy.dtype(x), out=out)
+ )
+ left_pad = max(0, -left)
+ right_pad = max(0, right - in_width)
+ top_pad = max(0, -top)
+ bottom_pad = max(0, bottom - in_height)
+ pad_width = [(0, 0), (0, 0)] * (len(x.shape) - 3) + [
+ (top_pad, bottom_pad),
+ (left_pad, right_pad),
+ ]
+ padded_x = ivy.pad(x, pad_width, mode="edge")
+ for b in range(batch):
+ for c in range(channels):
+ patch = padded_x[
+ b,
+ c,
+ top + top_pad : bottom + top_pad,
+ left + left_pad : right + left_pad,
+ ]
+ ret[b, c, i, j] = ivy.sum(
+ kernel_h[:, ivy.newaxis]
+ * patch
+ * kernel_w[ivy.newaxis, :]
+ )
+ elif mode == "gaussian":
+ ratio_h, ratio_w = scale
+ sigma = max(ratio_h, ratio_w) * 0.5
+ kernel_size = 2 * int(math.ceil(3 * sigma)) + 1
+ kernel_h = ivy.zeros((kernel_size,), dtype=x.dtype)
+ kernel_w = ivy.zeros((kernel_size,), dtype=x.dtype)
+ for i in range(kernel_h.size):
+ kernel_h[i] = ivy.exp(-0.5 * ((i - kernel_h.size // 2) / sigma) ** 2)
+ kernel_w[i] = ivy.exp(-0.5 * ((i - kernel_w.size // 2) / sigma) ** 2)
+ kernel_h /= ivy.sum(kernel_h)
+ kernel_w /= ivy.sum(kernel_w)
+ pad_width = [(0, 0), (0, 0)] * (len(x.shape) - 3) + [
+ (int(math.ceil(3 * sigma)), int(math.ceil(3 * sigma))),
+ (int(math.ceil(3 * sigma)), int(math.ceil(3 * sigma))),
+ ]
+ padded_x = ivy.pad(x, pad_width, mode="constant")
+ output_shape = x.shape[:2] + size
+ ret = ivy.zeros(output_shape, dtype=x.dtype)
+ for i in range(size[0]):
+ for j in range(size[1]):
+ p_i = int(math.floor(i * ratio_h + int(math.ceil(3 * sigma))))
+ p_j = int(math.floor(j * ratio_w + int(math.ceil(3 * sigma))))
+ for b in range(x.shape[0]):
+ for c in range(x.shape[1]):
+ patch = padded_x[
+ b,
+ c,
+ p_i - kernel_size // 2 : p_i + kernel_size // 2 + 1,
+ p_j - kernel_size // 2 : p_j + kernel_size // 2 + 1,
+ ]
+ ret[b, c, i, j] = ivy.sum(
+ kernel_h[ivy.newaxis, :]
+ * patch
+ * kernel_w[:, ivy.newaxis]
+ )
+ elif mode == "tf_area":
+ ret = _tf_area_interpolate(x, size, scale, dims)
+ ret = ivy.astype(ret, ivy.dtype(x))
+ if ivy.exists(out):
+ return ivy.inplace_update(out, ret)
+ return ret
interpolate.mixed_backend_wrappers = {
@@ -1997,7 +2004,7 @@ def interpolate(
}
-def _get_size(scale_factor, size, dims, x_shape):
+def _get_size(scale_factor, size, dims, input_shape):
if scale_factor is not None:
if isinstance(scale_factor, (float, int)):
scale_factor = [scale_factor] * dims
@@ -2005,11 +2012,12 @@ def _get_size(scale_factor, size, dims, x_shape):
scale_factor = [scale_factor[0]] * dims
size = tuple(
- [int(math.floor(x_shape[2 + i] * scale_factor[i])) for i in range(dims)]
+ int(math.floor(input_shape[i] * scale_factor[i])) for i in range(dims)
)
else:
size = (size,) * dims if isinstance(size, int) else tuple(size)
- return size
+ scale_factor = [ivy.divide(size[i], input_shape[i]) for i in range(dims)]
+ return size, scale_factor
def _output_ceil_shape(w, f, p, s):
@@ -2061,7 +2069,7 @@ def _compute_idx(in_size, out_size, device):
maxlength = in_size // out_size + 1
in_size_mod = in_size % out_size
# adaptive = True iff there are kernels with different lengths
- adaptive = not (in_size_mod == 0 or out_size % in_size_mod == 0)
+ adaptive = in_size_mod != 0 and out_size % in_size_mod != 0
if adaptive:
maxlength += 1
elif in_size_mod == 0:
@@ -2541,7 +2549,7 @@ def sliding_window(
padding=padding,
)
- if ivy.current_backend_str == "tensorflow":
+ if ivy.current_backend_str() == "tensorflow":
return ivy.current_backend(input).sliding_window(
input,
kernel_size,
@@ -2550,7 +2558,7 @@ def sliding_window(
padding=padding,
)
- if ivy.current_backend_str == "paddle":
+ if ivy.current_backend_str() == "paddle":
return ivy.current_backend(input).sliding_window(
input,
kernel_size,
@@ -2852,6 +2860,117 @@ def ifftn(
return ivy.current_backend(x).ifftn(x, s=s, axes=axes, norm=norm, out=out)
+@handle_exceptions
+@handle_backend_invalid
+@handle_nestable
+@handle_array_like_without_promotion
+@to_native_arrays_and_back
+@handle_device
+def rfft(
+ x: Union[ivy.Array, ivy.NativeArray],
+ /,
+ *,
+ n: Optional[int] = None,
+ axis: int = -1,
+ norm: Literal["backward", "ortho", "forward"] = "backward",
+ out: Optional[ivy.Array] = None,
+) -> ivy.Array:
+ """
+ Compute the one-dimensional discrete Fourier transform for real-valued input.
+
+ .. note::
+ Applying the one-dimensional inverse discrete Fourier transform for
+ real-valued input to the output of this function must return the original
+ (i.e., non-transformed) input array within numerical accuracy
+ (i.e., irfft(rfft(x)) == x), provided that the transform and inverse
+ transform are performed with the same arguments
+ (axis and normalization mode) and consistent length.
+
+ .. note::
+ If the input a contains an imaginary part, it is silently discarded.
+
+ Parameters
+ ----------
+ x
+ input array. Must have a real-valued floating-point data type.
+ n
+ length of the transformed axis of the input. If
+ - n is greater than the length of the input array, the input array
+ is zero-padded to length n.
+ - n is less than the length of the input array, the input array is
+ trimmed to length n.
+ - n is not provided, the length of the transformed axis of the
+ output must equal the length of the input along the axis specified
+ by axis. Default is ``None``.
+ axis
+ axis (dimension) over which to compute the Fourier transform.
+ If not set, the last axis (dimension) is used. Default is ``-1``.
+ norm
+ normalization mode. Should be one of the following modes:
+ - 'backward': no normalization.
+ - 'ortho': normalize by 1/sqrt(n) (i.e., make the FFT orthonormal).
+ - 'forward': normalize by 1/n.
+ Default is ``backward``.
+ out
+ Optional output array, for writing the result to. It must
+ have a shape that the inputs broadcast to.
+
+ Returns
+ -------
+ ret
+ an array transformed along the axis (dimension) indicated by axis.
+ The returned array must have a complex-valued floating-point
+ data type determined by Type Promotion Rules.
+
+ This function conforms to the `Array API Standard
+ `_. This docstring is an extension of the
+ `docstring `_
+ in the standard.
+
+ Both the description and the type hints above assumes an array input for simplicity,
+ but this function is *nestable*, and therefore also accepts :class:`ivy.Container`
+ instances in place of any of the arguments.
+
+ Examples
+ --------
+ With `ivy.Array` input:
+
+ >>> x = ivy.array([0,1,2])
+ >>> y = ivy.rfft(x)
+ >>> print(y)
+ ivy.array([ 3. +0.j , -1.5+0.8660254j])
+
+ >>> x = ivy.array([2.3,3.14,7.2])
+ >>> y = ivy.zeros(2)
+ >>> ivy.rfft(x, out=y)
+ ivy.array([12.639999+0.j , -2.87 +3.516063j])
+
+ >>> x = ivy.array([-1.2, 3.4, -5.6])
+ >>> ivy.rfft(x, n=4, out=x)
+ >>> print(x)
+ ivy.array([ -3.3999999+0.j , 4.3999996-3.4j, -10.2 +0.j ],
+ dtype=complex64)
+
+ With `ivy.Container` input:
+
+ >>> x = ivy.Container(a=ivy.array([0.,1.,2.]),
+ ... b=ivy.array([3.,4.,5.]))
+ >>> y = ivy.rfft(x)
+ >>> print(y)
+ {
+ a: ivy.array([3.+0.j, -1.5+0.8660254j]),
+ b: ivy.array([12.+0.j, -1.5+0.8660254j])
+ }
+ """
+ if axis is None:
+ axis = -1
+ if norm is None:
+ norm = "backward"
+
+ return ivy.current_backend().rfft(x, n=n, axis=axis, norm=norm, out=out)
+
+
@handle_exceptions
@handle_backend_invalid
@handle_nestable
@@ -2952,7 +3071,7 @@ def stft(
/,
*,
fft_length: Optional[int] = None,
- window_fn: Optional = None,
+ window_fn: Optional[Callable] = None,
pad_end: bool = False,
name: Optional[str] = None,
out: Optional[ivy.Array] = None,
@@ -3008,10 +3127,10 @@ def stft(
def _broadcast_pooling_helper(x, pool_dims: str = "2d", name: str = "padding"):
dims = {"1d": 1, "2d": 2, "3d": 3}
if isinstance(x, int):
- return tuple([x for _ in range(dims[pool_dims])])
+ return tuple(x for _ in range(dims[pool_dims]))
if len(x) == 1:
- return tuple([x[0] for _ in range(dims[pool_dims])])
+ return tuple(x[0] for _ in range(dims[pool_dims]))
elif len(x) == dims[pool_dims]:
return tuple(x)
diff --git a/ivy/functional/ivy/experimental/linear_algebra.py b/ivy/functional/ivy/experimental/linear_algebra.py
index e5b6cdc705641..6eccd78b3af87 100644
--- a/ivy/functional/ivy/experimental/linear_algebra.py
+++ b/ivy/functional/ivy/experimental/linear_algebra.py
@@ -465,6 +465,70 @@ def adjoint(
return current_backend(x).adjoint(x, out=out)
+@handle_exceptions
+@handle_backend_invalid
+@handle_nestable
+@handle_array_like_without_promotion
+@handle_out_argument
+@to_native_arrays_and_back
+@handle_device
+def solve_triangular(
+ x1: Union[ivy.Array, ivy.NativeArray],
+ x2: Union[ivy.Array, ivy.NativeArray],
+ /,
+ *,
+ upper: bool = True,
+ adjoint: bool = False,
+ unit_diagonal: bool = False,
+ out: Optional[ivy.Array] = None,
+) -> ivy.Array:
+ """
+ Return the unique solution to the triangular system of linear equations AX = B.
+
+ Parameters
+ ----------
+ x1
+ Triangular coefficient array A of shape (..., N, N), with no zeros on diagonal.
+ x2
+ Right-hand side array B of shape (..., N, K).
+ upper
+ Whether the input `x1` is upper triangular.
+ adjoint
+ Whether to take the adjoint (conjugate transpose) of `x1` as the matrix A.
+ unit_diagonal
+ Whether to ignore the diagonal entries of A and assume them all equal to 1.
+ out
+ Optional output array. If provided, the output array to store the result.
+
+ Returns
+ -------
+ ret
+ The solution X, which has the same shape as B.
+
+ Examples
+ --------
+ With :class:`ivy.Array` inputs:
+
+ >>> a = ivy.array([[3, 0, 0, 0],
+ ... [2, 1, 0, 0],
+ ... [1, 0, 1, 0],
+ ... [1, 1, 1, 1]], dtype=ivy.float32)
+ >>> b = ivy.array([[4],
+ ... [2],
+ ... [4],
+ ... [2]], dtype=ivy.float32)
+ >>> x = ivy.solve_triangular(a, b, upper=False)
+ >>> ivy.matmul(a, x)
+ ivy.array([[4.],
+ [2.],
+ [4.],
+ [2.]])
+ """
+ return current_backend(x1, x2).solve_triangular(
+ x1, x2, upper=upper, adjoint=adjoint, unit_diagonal=unit_diagonal, out=out
+ )
+
+
@handle_exceptions
@handle_backend_invalid
@handle_nestable
@@ -621,7 +685,7 @@ def kronecker(
return res
-# The code has been adapated from tensorly.khatri_rao
+# The code has been adapted from tensorly.khatri_rao
# https://github.com/tensorly/tensorly/blob/main/tensorly/tenalg/core_tenalg/_khatri_rao.py#L9
@handle_nestable
@handle_exceptions
@@ -817,7 +881,7 @@ def mode_dot(
return ivy.fold(res, fold_mode, new_shape, out=out)
-# The following code has been adapated from TensorLy
+# The following code has been adapted from TensorLy
# https://github.com/tensorly/tensorly/blob/main/tensorly/tenalg/core_tenalg/n_mode_product.py#L81
@handle_nestable
@handle_exceptions
@@ -942,7 +1006,7 @@ def _svd_checks(x, n_eigenvecs=None):
return n_eigenvecs, min_dim, max_dim
-# This function has been adapated from TensorLy
+# This function has been adapted from TensorLy
# https://github.com/tensorly/tensorly/blob/main/tensorly/tenalg/svd.py#L12
@handle_nestable
@handle_exceptions
@@ -1096,8 +1160,8 @@ def make_svd_non_negative(
H = ivy.soft_thresholding(H, eps)
elif nntype == "nndsvda":
avg = ivy.mean(x)
- W = ivy.where(W < eps, ivy.ones(ivy.shape(W)) * avg, W)
- H = ivy.where(H < eps, ivy.ones(ivy.shape(H)) * avg, H)
+ W = ivy.where(eps > W, ivy.ones(ivy.shape(W)) * avg, W)
+ H = ivy.where(eps > H, ivy.ones(ivy.shape(H)) * avg, H)
else:
raise ValueError(
f'Invalid nntype parameter: got {nntype} instead of one of ("nndsvd",'
@@ -1153,7 +1217,86 @@ def truncated_svd(
return S[:n_eigenvecs]
-# TODO uncommment the code below when these svd
+@handle_nestable
+@handle_exceptions
+@handle_array_like_without_promotion
+@inputs_to_ivy_arrays
+@handle_array_function
+def tensor_train(
+ input_tensor: Union[ivy.Array, ivy.NativeArray],
+ rank: Union[int, Sequence[int]],
+ /,
+ *,
+ svd: Optional[Literal["truncated_svd"]] = "truncated_svd",
+ verbose: Optional[bool] = False,
+) -> ivy.TTTensor:
+ """
+ TT decomposition via recursive SVD.
+
+ Decomposes the input into a sequence of order-3 tensors (factors)
+ Also known as Tensor-Train decomposition [1]_
+
+ Parameters
+ ----------
+ input_tensor
+ tensor to decompose
+ rank
+ maximum allowable TT rank of the factors
+ if int, then this is the same for all the factors
+ if int list, then rank[k] is the rank of the kth factor
+ svd
+ function to use to compute the SVD
+ verbose
+ level of verbosity
+
+ Returns
+ -------
+ factors
+ order-3 tensors of the TT decomposition
+
+ [1]: Ivan V. Oseledets. "Tensor-train decomposition",
+ SIAM J. Scientific Computing, 33(5):2295β2317, 2011.
+ """
+ rank = ivy.TTTensor.validate_tt_rank(ivy.shape(input_tensor), rank=rank)
+ tensor_size = input_tensor.shape
+ n_dim = len(tensor_size)
+
+ unfolding = input_tensor
+ factors = [None] * n_dim
+
+ for k in range(n_dim - 1):
+ n_row = int(rank[k] * tensor_size[k])
+ unfolding = ivy.reshape(unfolding, (n_row, -1))
+
+ (n_row, n_column) = unfolding.shape
+ current_rank = min(n_row, n_column, rank[k + 1])
+ U, S, V = _svd_interface(unfolding, n_eigenvecs=current_rank, method=svd)
+
+ rank[k + 1] = current_rank
+ factors[k] = ivy.reshape(U, (rank[k], tensor_size[k], rank[k + 1]))
+
+ if verbose is True:
+ print(
+ "TT factor " + str(k) + " computed with shape " + str(factors[k].shape)
+ )
+
+ unfolding = ivy.reshape(S, (-1, 1)) * V
+
+ (prev_rank, last_dim) = unfolding.shape
+ factors[-1] = ivy.reshape(unfolding, (prev_rank, last_dim, 1))
+
+ if verbose is True:
+ print(
+ "TT factor "
+ + str(n_dim - 1)
+ + " computed with shape "
+ + str(factors[n_dim - 1].shape)
+ )
+
+ return ivy.TTTensor(factors)
+
+
+# TODO uncomment the code below when these svd
# methods have been added
def _svd_interface(
matrix,
@@ -1261,7 +1404,7 @@ def initialize_tucker(
assert len(x.shape) >= 2
except ValueError:
raise ValueError(
- "expected x to have atleast 2 dimensions but it has only"
+ "expected x to have at least 2 dimensions but it has only"
f" {len(x.shape)} dimension(s)"
)
@@ -1310,7 +1453,7 @@ def initialize_tucker(
return (core, factors)
-# This function has been adpated from TensorLy
+# This function has been adapted from TensorLy
# https://github.com/tensorly/tensorly/blob/main/tensorly/decomposition/_tucker.py#L98
@handle_nestable
@handle_exceptions
diff --git a/ivy/functional/ivy/experimental/losses.py b/ivy/functional/ivy/experimental/losses.py
index 73feaac094b79..7e45013b52f8a 100644
--- a/ivy/functional/ivy/experimental/losses.py
+++ b/ivy/functional/ivy/experimental/losses.py
@@ -76,11 +76,11 @@ def log_poisson_loss(
"""
try:
assert true.shape == pred.shape
- except ValueError:
+ except ValueError as e:
raise ValueError(
"`pred` and `true` must have the same shape, received "
f"({pred.shape} vs {true.shape})."
- )
+ ) from e
loss = ivy.exp(pred) - pred * true
if compute_full_loss:
diff --git a/ivy/functional/ivy/experimental/manipulation.py b/ivy/functional/ivy/experimental/manipulation.py
index 1239bdefa3d46..94a6360ecc4ce 100644
--- a/ivy/functional/ivy/experimental/manipulation.py
+++ b/ivy/functional/ivy/experimental/manipulation.py
@@ -42,6 +42,12 @@ def _to_tf_padding(pad_width, ndim):
if isinstance(pad_width, Number):
pad_width = [[pad_width] * 2] * ndim
elif len(pad_width) == 2 and isinstance(pad_width[0], Number):
+ pad_width = [pad_width] * ndim
+ elif (
+ isinstance(pad_width, (list, tuple))
+ and isinstance(pad_width[0], (list, tuple))
+ and len(pad_width) < ndim
+ ):
pad_width = pad_width * ndim
return pad_width
@@ -74,7 +80,7 @@ def _to_paddle_padding(pad_width, ndim):
pad_width = [pad_width] * (2 * ndim)
else:
if len(pad_width) == 2 and isinstance(pad_width[0], Number) and ndim != 1:
- pad_width = pad_width * ndim
+ pad_width = [pad_width] * ndim
pad_width = [item for sublist in pad_width for item in sublist[::-1]][::-1]
return pad_width
@@ -584,7 +590,7 @@ def top_k(
x
The array to compute top_k for.
k
- Number of top elements to retun must not exceed the array size.
+ Number of top elements to return must not exceed the array size.
axis
The axis along which we must return the top elements default value is 1.
largest
@@ -897,16 +903,13 @@ def _set_wrap_both(padded, axis, width_pair):
def _pad_simple(array, pad_width, fill_value=None):
new_shape = tuple(
- [left + size + right for size, (left, right) in zip(array.shape, pad_width)]
+ left + size + right for size, (left, right) in zip(array.shape, pad_width)
)
padded = ivy.zeros(new_shape, dtype=array.dtype)
if fill_value is not None:
padded = ivy.ones_like(padded) * fill_value
original_area_slice = tuple(
- [
- slice(left, left + size)
- for size, (left, right) in zip(array.shape, pad_width)
- ]
+ slice(left, left + size) for size, (left, right) in zip(array.shape, pad_width)
)
padded[original_area_slice] = array
return padded, original_area_slice
@@ -951,7 +954,7 @@ def _to_dilated(x, n):
def _check_tuple_arg(arg, name, force_integer=True):
- is_scalar = ivy.isscalar if not force_integer else ivy.is_int_dtype
+ is_scalar = ivy.is_int_dtype if force_integer else ivy.isscalar
flag_assert = False
if isinstance(arg, (tuple, list)):
for nested in arg:
@@ -965,13 +968,13 @@ def _check_tuple_arg(arg, name, force_integer=True):
elif not is_scalar(arg):
flag_assert = True
if flag_assert:
- if not force_integer:
+ if force_integer:
raise ivy.utils.exceptions.IvyException(
- name + " should be scalar, tuple of scalars or tuple of scalar tuples"
+ f"{name} should be int, tuple of ints or tuple of int tuples"
)
else:
raise ivy.utils.exceptions.IvyException(
- name + " should be int, tuple of ints or tuple of int tuples"
+ f"{name} should be scalar, tuple of scalars or tuple of scalar tuples"
)
@@ -1421,7 +1424,7 @@ def atleast_1d(
Returns
-------
ret
- An array, or list of arrays, each with atleast 1D.
+ An array, or list of arrays, each with at least 1D.
Copies are made only if necessary.
Examples
@@ -1509,7 +1512,7 @@ def atleast_2d(
Returns
-------
ret
- An array, or list of arrays, each with atleast 2D.
+ An array, or list of arrays, each with at least 2D.
Copies are made only if necessary.
Examples
@@ -2032,16 +2035,16 @@ def _interior_pad(operand, padding_value, padding_config):
for axis, (low, high, _) in enumerate(padding_config):
if low > 0 and high > 0:
pad_width[axis] = (low, high)
- elif low > 0 and not high > 0:
+ elif low > 0:
pad_width[axis] = (low, 0)
- elif high > 0 and not low > 0:
+ elif high > 0:
pad_width[axis] = (0, high)
padded = ivy.constant_pad(padded, pad_width, value=padding_value)
return padded
def _interleave(a, b, axis):
- assert a.shape[axis] == b.shape[axis] or a.shape[axis] == b.shape[axis] + 1
+ assert a.shape[axis] in [b.shape[axis], b.shape[axis] + 1]
a_pad = [(0, 0, 0)] * a.ndim
b_pad = [(0, 0, 0)] * b.ndim
a_pad[axis] = (0, 1 if a.shape[axis] == b.shape[axis] else 0, 1)
@@ -2227,10 +2230,10 @@ def fill_diagonal(
end = shape[1] * shape[1]
else:
step = int(1 + (ivy.cumprod(ivy.array(shape[:-1]), axis=0)).sum())
- end = int(max_end if end > max_end else end)
+ end = int(min(end, max_end))
a = ivy.reshape(a, (-1,))
steps = ivy.arange(0, end, step)
- if isinstance(v, ivy.Array) or isinstance(v, ivy.NativeArray):
+ if isinstance(v, (ivy.Array, ivy.NativeArray)):
v = ivy.reshape(v, (-1,)).astype(a.dtype)
v = ivy.tile(v, int(ivy.ceil(len(steps) / v.shape[0])))[: len(steps)]
else:
@@ -2741,3 +2744,171 @@ def column_stack(
),
"to_skip": ("inputs_to_ivy_arrays",),
}
+
+
+@handle_exceptions
+@handle_backend_invalid
+@handle_nestable
+@handle_array_like_without_promotion
+@handle_out_argument
+@to_native_arrays_and_back
+@handle_device
+def take(
+ x: Union[int, ivy.Array, ivy.NativeArray],
+ indices: Union[int, ivy.Array, ivy.NativeArray],
+ /,
+ *,
+ axis: Optional[int] = None,
+ mode: str = "fill",
+ fill_value: Optional[Number] = None,
+ out: Optional[ivy.Array] = None,
+) -> ivy.Array:
+ """
+ Return elements of an array along an axis.
+
+ .. note::
+ Conceptually, take(x, indices, axis=3) is equivalent to x[:,:,:,indices,...];
+ however, explicit indexing via arrays of indices is not currently supported
+ in this specification due to concerns regarding __setitem__
+ and array mutation semantics.
+
+ Parameters
+ ----------
+ x
+ input array
+ indices
+ array indices. Must have an integer data type.
+ axis
+ axis over which to select values. If `axis` is negative,
+ the function must determine the axis along which to select values
+ by counting from the last dimension.
+ By default, the flattened input array is used.
+ mode
+ specifies how out-of-bounds `indices` will behave.
+ - βraiseβ β raise an error
+ - βwrapβ β wrap around
+ - βclipβ β clip to the range (all indices that are too large are
+ replaced by the index that addresses the last element along that axis.
+ Note that this disables indexing with negative numbers.)
+ - 'fill' (default) = returns invalid values (e.g. NaN)
+ for out-of bounds indices (see also fill_value below)
+ fill_value
+ fill value to return for out-of-bounds slices
+ (Defaults to NaN for inexact types,
+ the largest negative value for signed types,
+ the largest positive value for unsigned types, and True for booleans.)
+ out
+ optional output array, for writing the result to. It must
+ have a shape that the inputs broadcast to.
+
+ Returns
+ -------
+ ret
+ an array having the same data type as `x`.
+ The output array must have the same rank (i.e., number of dimensions) as `x`
+ and must have the same shape as `x`, except for the axis specified by `axis`
+ whose size must equal the number of elements in `indices`.
+
+ This function conforms to the `Array API Standard
+ `_. This docstring is an extension of the
+ `docstring `_
+ in the standard.
+
+ Both the description and the type hints above assumes an array input for simplicity,
+ but this function is *nestable*, and therefore also accepts :class:`ivy.Container`
+ instances in place of any of the arguments.
+
+ Examples
+ --------
+ With `ivy.Array` input:
+
+ >>> x = ivy.array([4,5,6])
+ >>> indices = ivy.array([2,1,0])
+ >>> y = ivy.take(x, indices)
+ >>> print(y)
+ ivy.array([6, 5, 4])
+
+ >>> x = ivy.array([4.7,5.2,6.5])
+ >>> indices = ivy.array([[0,1]])
+ >>> y = ivy.zeros_like(indices, dtype=x.dtype)
+ >>> ivy.take(x, indices, out=y)
+ >>> print(y)
+ ivy.array([[4.7, 5.2]])
+
+ >>> x = ivy.array([False, False, True])
+ >>> indices = ivy.array([[4,3,2]])
+ >>> y = ivy.zeros_like(indices, dtype=x.dtype)
+ >>> ivy.take(x, indices, out=y, mode="wrap")
+ >>> print(y)
+ ivy.array([[False, False, True]])
+
+ With `ivy.Container` input:
+
+ >>> x = ivy.Container(a=ivy.array([True,False,False]),
+ ... b=ivy.array([2.3,4.5,6.7]),
+ ... c=ivy.array([1,2,3]))
+ >>> indices = ivy.array([[1,9,2]])
+ >>> y = ivy.take(x, indices)
+ >>> print(y)
+ {
+ a: ivy.array([[False, True, False]]),
+ b: ivy.array([[4.5, nan, 6.69999981]]),
+ c: ivy.array([[2, -2147483648, 3]])
+ }
+ """
+ return ivy.current_backend().take(
+ x, indices, axis=axis, mode=mode, fill_value=fill_value, out=out
+ )
+
+
+@inputs_to_ivy_arrays
+@handle_exceptions
+@handle_device
+def trim_zeros(
+ a: Union[ivy.Array, ivy.NativeArray],
+ /,
+ *,
+ trim: Optional[str] = "fb",
+) -> ivy.Array:
+ """
+ ivy.Container instance method variant of ivy.trim_zeros. This method simply wraps
+ the function, and so the docstring for ivy.trim_zeros also applies to this method
+ with minimal changes.
+
+ Parameters
+ ----------
+ a : 1-D array
+ Input array.
+ trim : str, optional
+ A string with 'f' representing trim from front and 'b' to trim from
+ back. Default is 'fb', trim zeros from both front and back of the
+ array.
+
+ Returns
+ -------
+ 1-D array
+ The result of trimming the input. The input data type is preserved.
+
+ Examples
+ --------
+ >>> a = ivy.array([0, 0, 0, 0, 8, 3, 0, 0, 7, 1, 0])
+ >>> ivy.trim_zeros(a)
+ array([8, 3, 0, 0, 7, 1])
+ >>> ivy.trim_zeros(a, 'b')
+ array([0, 0, 0, 0, 8, 3, 0, 0, 7, 1])
+ >>> ivy.trim_zeros([0, 8, 3, 0, 0])
+ [8, 3]
+ """
+ return ivy.current_backend(a).trim_zeros(a, trim=trim)
+
+
+trim_zeros.mixed_backend_wrappers = {
+ "to_add": (
+ "handle_backend_invalid",
+ "inputs_to_native_arrays",
+ "outputs_to_ivy_arrays",
+ "handle_device",
+ ),
+ "to_skip": ("inputs_to_ivy_arrays",),
+}
diff --git a/ivy/functional/ivy/experimental/random.py b/ivy/functional/ivy/experimental/random.py
index 61e8476e5d3d1..5a653147a385a 100644
--- a/ivy/functional/ivy/experimental/random.py
+++ b/ivy/functional/ivy/experimental/random.py
@@ -277,7 +277,7 @@ def bernoulli(
out: Optional[ivy.Array] = None,
) -> ivy.Array:
"""
- Draws samples from Bernoulli distrubution paramterized by probs or logits (but not
+ Draws samples from Bernoulli distribution parameterized by probs or logits (but not
both)
Parameters
diff --git a/ivy/functional/ivy/experimental/sparse_array.py b/ivy/functional/ivy/experimental/sparse_array.py
index 3d405734c5609..4a82fb4f97ad9 100644
--- a/ivy/functional/ivy/experimental/sparse_array.py
+++ b/ivy/functional/ivy/experimental/sparse_array.py
@@ -393,7 +393,7 @@ def __init__(
if format == "coo":
self._init_coo_components(coo_indices, values, dense_shape, format)
- elif format == "csr" or format == "bsr":
+ elif format in ["csr", "bsr"]:
self._init_compressed_row_components(
crow_indices, col_indices, values, dense_shape, format
)
@@ -551,7 +551,7 @@ def __repr__(self):
f"indices={self._coo_indices}, values={self._values},"
f" dense_shape={self._dense_shape}"
)
- elif self._format == "csr" or self._format == "bsr":
+ elif self._format in ["csr", "bsr"]:
repr = (
f"crow_indices={self._crow_indices}, col_indices={self._col_indices},"
f" values={self._values}, dense_shape={self._dense_shape}"
diff --git a/ivy/functional/ivy/experimental/statistical.py b/ivy/functional/ivy/experimental/statistical.py
index 82c07f89b1853..a7c6940e15a2d 100644
--- a/ivy/functional/ivy/experimental/statistical.py
+++ b/ivy/functional/ivy/experimental/statistical.py
@@ -253,6 +253,68 @@ def nanmean(
)
+@handle_out_argument
+@handle_nestable
+@handle_backend_invalid
+@handle_exceptions
+@to_native_arrays_and_back
+@handle_device
+def nanmin(
+ x: ivy.Array,
+ /,
+ *,
+ axis: Optional[Union[Tuple[int], int]] = None,
+ keepdims: Optional[bool] = False,
+ out: Optional[ivy.Array] = None,
+ initial: Optional[Union[int, float, complex]] = None,
+ where: Optional[ivy.Array] = None,
+) -> ivy.Array:
+ """
+ Return minimum of an array or minimum along an axis, ignoring any NaNs.
+
+ Parameters
+ ----------
+ a
+ Input array.
+ axis
+ Axis or axes along which the minimum is computed.
+ The default is to compute the minimum of the flattened array.
+ out
+ optional output array, for writing the result to.
+ keepdims
+ If this is set to True, the axes which are reduced are left in the result
+ as dimensions with size one. With this option, the result will broadcast
+ correctly against the original a.
+ initial
+ The maximum value of an output element.
+ where
+ Elements to compare for the minimum
+
+ Returns
+ -------
+ ret
+ Return minimum of an array or minimum along an axis, ignoring any NaNs
+
+ Functional Examples
+ -------------------
+ >>> a = ivy.array([[1, ivy.nan], [3, 4]])
+ >>> ivy.nanmin(a)
+ 1.0
+ >>> ivy.nanmin(a, axis=1)
+ [1. 3.]
+ >>> ivy.nanmin(a, axis=0, keepdims=True)
+ [[1. 2.]]
+ """
+ return ivy.current_backend(x).nanmin(
+ x,
+ axis=axis,
+ keepdims=keepdims,
+ out=out,
+ initial=initial,
+ where=where,
+ )
+
+
@handle_exceptions
@handle_backend_invalid
@handle_nestable
diff --git a/ivy/functional/ivy/general.py b/ivy/functional/ivy/general.py
index 119ac049bee30..aa26607723101 100644
--- a/ivy/functional/ivy/general.py
+++ b/ivy/functional/ivy/general.py
@@ -45,25 +45,26 @@
)
from ivy.functional.ivy.device import dev
-FN_CACHE = dict()
+FN_CACHE = {}
INF = float("inf")
-precise_mode_stack = list()
-queue_timeout_stack = list()
-array_mode_stack = list()
-shape_array_mode_stack = list()
-nestable_mode_stack = list()
-exception_trace_mode_stack = list()
-inplace_mode_stack = list()
-trace_mode_dict = dict()
-trace_mode_dict["frontend"] = "ivy/functional/frontends"
-trace_mode_dict["ivy"] = "ivy/"
-trace_mode_dict["full"] = ""
-trace_mode_dict["none"] = ""
-show_func_wrapper_trace_mode_stack = list()
-min_denominator_stack = list()
-min_base_stack = list()
-tmp_dir_stack = list()
+precise_mode_stack = []
+queue_timeout_stack = []
+array_mode_stack = []
+shape_array_mode_stack = []
+nestable_mode_stack = []
+exception_trace_mode_stack = []
+inplace_mode_stack = []
+trace_mode_dict = {
+ "frontend": "ivy/functional/frontends",
+ "ivy": "ivy/",
+ "full": "",
+ "none": "",
+}
+show_func_wrapper_trace_mode_stack = []
+min_denominator_stack = []
+min_base_stack = []
+tmp_dir_stack = []
# Extra #
@@ -95,12 +96,12 @@ def __exit__(self, exc_type, exc_val, exc_tb):
def set_precise_mode(mode: bool) -> None:
"""
Set the mode of whether to use a promotion table that avoids any precision loss or a
- compute effecient table that avoids most wider-than-necessary promotions.
+ compute efficient table that avoids most wider-than- necessary promotions.
Parameter
---------
mode
- boolean whether to use high precision promtion table
+ boolean whether to use high precision promotion table
Examples
--------
@@ -123,7 +124,7 @@ def set_precise_mode(mode: bool) -> None:
def unset_precise_mode() -> None:
"""
Reset the mode of whether to use a promotion table that avoids any precision loss or
- a compute effecient table that avoids most wider-than-necessary promotions.
+ a compute efficient table that avoids most wider-than- necessary promotions.
Examples
--------
@@ -180,6 +181,7 @@ def __exit__(self, exc_type, exc_val, exc_tb):
def get_referrers_recursive(
item: object,
+ *,
depth: int = 0,
max_depth: int = None,
seen_set: set = None,
@@ -193,20 +195,20 @@ def get_referrers_recursive(
Parameters
----------
- item : object
+ item
The object for which referrers should be retrieved.
- depth : int, optional
+ depth
Current depth in the recursion. (default is 0)
- max_depth : int, optional
+ max_depth
Maximum depth of recursion. If `None`, there's no depth limit. (default is None)
- seen_set : set, optional
+ seen_set
Set of seen referrer IDs to prevent duplicates. (default is None)
- local_set : set, optional
+ local_set
Set of local referrer IDs to avoid redundancy. (default is None)
Returns
-------
- ivy.Container
+ ret
A container representing referrers and their sub-referrers, respecting the
`max_depth`.
@@ -238,7 +240,7 @@ def get_referrers_recursive(
for ref in gc.get_referrers(item)
if not (
isinstance(ref, dict)
- and min([k in ref for k in ["depth", "max_depth", "seen_set", "local_set"]])
+ and min(k in ref for k in ["depth", "max_depth", "seen_set", "local_set"])
)
]
@@ -533,7 +535,7 @@ def set_exception_trace_mode(mode: Literal["ivy", "full", "frontend"]) -> None:
Parameter
---------
mode
- str exeption trace mode, one of `ivy`, `full` or `frontend`
+ str exception trace mode, one of `ivy`, `full` or `frontend`
Examples
--------
@@ -1057,6 +1059,18 @@ def clip_vector_norm(
a: ivy.array([0., 0.894, 1.79]),
b: ivy.array([0.849, 1.13, 1.41])
}
+
+ With multiple :class:`ivy.Container` inputs:
+
+ >>> x = ivy.Container(a=ivy.array([0., 1., 2.]),
+ ... b=ivy.array([3., 4., 5.]))
+ >>> max_norm = ivy.Container(a=2, b=3)
+ >>> y = ivy.clip_vector_norm(x, max_norm)
+ >>> print(y)
+ {
+ a: ivy.array([0., 0.894, 1.79]),
+ b: ivy.array([2.449, 2.65, 2.83])
+ }
"""
norm = ivy.vector_norm(x, keepdims=True, ord=p)
ratio = ivy.stable_divide(max_norm, norm)
@@ -1181,7 +1195,7 @@ def fourier_encode(
Whether to space the frequency bands linearly as opposed to geometrically.
Default is ``False``.
concat
- Whether to concatenate the position, sin and cos values, or return seperately.
+ Whether to concatenate the position, sin and cos values, or return separately.
Default is ``True``.
flatten
Whether to flatten the position dimension into the batch dimension.
@@ -1225,23 +1239,22 @@ def fourier_encode(
orig_x = x
if linear:
scales = ivy.linspace(1.0, max_freq / 2, num_bands, device=dev(x))
+ elif ivy.backend == "torch" and isinstance(max_freq, float):
+ scales = ivy.logspace(
+ 0.0,
+ ivy.log(ivy.array(max_freq / 2)) / math.log(10),
+ num_bands,
+ base=10,
+ device=dev(x),
+ )
else:
- if ivy.backend == "torch" and isinstance(max_freq, float):
- scales = ivy.logspace(
- 0.0,
- ivy.log(ivy.array(max_freq / 2)) / math.log(10),
- num_bands,
- base=10,
- device=dev(x),
- )
- else:
- scales = ivy.logspace(
- 0.0,
- ivy.log(max_freq / 2) / math.log(10),
- num_bands,
- base=10,
- device=dev(x),
- )
+ scales = ivy.logspace(
+ 0.0,
+ ivy.log(max_freq / 2) / math.log(10),
+ num_bands,
+ base=10,
+ device=dev(x),
+ )
scales = ivy.astype(scales, ivy.dtype(x))
scales = scales[(*((None,) * (len(x.shape) - len(scales.shape))), Ellipsis)]
x = x * scales * math.pi
@@ -1311,9 +1324,9 @@ def value_is_nan(
False
"""
x_scalar = ivy.to_scalar(x) if ivy.is_array(x) else x
- if not x_scalar == x:
+ if x_scalar != x:
return True
- if include_infs and (x_scalar == INF or x_scalar == -INF):
+ if include_infs and (x_scalar in [INF, -INF]):
return True
return False
@@ -1541,9 +1554,7 @@ def default(
"""
with_callable = catch_exceptions or with_callable
if rev:
- tmp = x
- x = default_val
- default_val = tmp
+ x, default_val = default_val, x
if with_callable:
x_callable = callable(x)
default_callable = callable(default_val)
@@ -1639,7 +1650,7 @@ def try_else_none(fn: Callable, *args: Any, **kwargs: Any) -> Union[Callable, No
args
list of arguments.
kwargs
- dictionay of keyword arguments
+ dictionary of keyword arguments
Returns
-------
@@ -1736,12 +1747,12 @@ def match_kwargs(
>>> print(x)
[{'out': ivy.array([0., 0., 0.]), 'bias': ivy.array([0, 1, 2])}, {}]
"""
- split_kwargs = list()
+ split_kwargs = []
for receiver in receivers:
expected_kwargs = arg_names(receiver)
found_kwargs = {k: v for k, v in kwargs.items() if k in expected_kwargs}
if not allow_duplicates:
- for k in found_kwargs.keys():
+ for k in found_kwargs:
del kwargs[k]
split_kwargs.append(found_kwargs)
if len(split_kwargs) == 1:
@@ -1785,14 +1796,13 @@ def cache_fn(func: Callable) -> Callable:
"""
global FN_CACHE
if func not in FN_CACHE:
- FN_CACHE[func] = dict()
+ FN_CACHE[func] = {}
@wraps(func)
def cached_fn(*args, **kwargs):
key = "".join(
- [str(i) + ", " for i in args]
- + [" kw, "]
- + [str(i) + ", " for i in sorted(kwargs.items())]
+ ([f"{str(i)}, " for i in args] + [" kw, "])
+ + [f"{str(i)}, " for i in sorted(kwargs.items())]
)
cache = FN_CACHE[func]
if key in cache:
@@ -2172,18 +2182,28 @@ def set_min_base(val: float) -> None:
Examples
--------
+ Retrieve the minimum base
>>> x = ivy.min_base
>>> print(x)
1e-05
+ Set the minimum base to 1e-04:
>>> ivy.set_min_base(1e-04)
+
+ Retrieve the minimum base:
>>> y = ivy.min_base
>>> print(y)
1e-04
"""
global min_base_stack
+
+ # Ensure val is an instance of 'float' or 'int'
ivy.utils.assertions.check_isinstance(val, (int, float))
+
+ # Access and modify min_base_stack
min_base_stack.append(val)
+
+ # Set the min_base attribute
ivy.__setattr__("min_base", val, True)
@@ -2432,7 +2452,7 @@ def get_all_arrays_in_memory() -> List[Union[ivy.Array, ivy.NativeArray]]:
>>> x
[ivy.array([0, 1, 2])]
"""
- all_arrays = list()
+ all_arrays = []
for obj in gc.get_objects():
try:
if ivy.current_backend_str() in ["", "numpy"]:
@@ -2766,9 +2786,8 @@ def assert_supports_inplace(x: Union[ivy.Array, ivy.NativeArray], /) -> bool:
"""
ivy.utils.assertions.check_true(
ivy.supports_inplace_updates(x),
- "Inplace operations are not supported {} types with {} backend".format(
- type(x), ivy.current_backend_str()
- ),
+ f"Inplace operations are not supported {type(x)} types with"
+ f" {ivy.current_backend_str()} backend",
)
return True
@@ -2819,10 +2838,10 @@ def get_item(
ivy.array([ 4, -2, -10])
"""
if ivy.is_array(query) and ivy.is_bool_dtype(query):
- if not len(query.shape):
- if not query:
- return ivy.array([], shape=(0,), dtype=x.dtype)
- return ivy.expand_dims(x, axis=0)
+ if query.ndim == 0:
+ if query is False:
+ return ivy.zeros(shape=(0,) + x.shape, dtype=x.dtype)
+ return x[None] # eqivalent to ivy.expand_dims(x, axis=0)
query = ivy.nonzero(query, as_tuple=False)
ret = ivy.gather_nd(x, query)
else:
@@ -2925,8 +2944,8 @@ def set_item(
def _parse_query(query, x_shape):
- query = (query,) if not isinstance(query, tuple) else query
- query_ = tuple([q.to_numpy() if ivy.is_array(q) else q for q in query])
+ query = query if isinstance(query, tuple) else (query,)
+ query_ = tuple(q.to_numpy() if ivy.is_array(q) else q for q in query)
# array containing all of x's flat indices
x_ = ivy.arange(0, _numel(x_shape)).reshape(x_shape)
@@ -2956,7 +2975,7 @@ def _broadcast_to(input, target_shape):
if _numel(tuple(input.shape)) == _numel(tuple(target_shape)):
return ivy.reshape(input, target_shape)
else:
- input = ivy.expand_dims(input, axis=0) if not len(input.shape) else input
+ input = input if len(input.shape) else ivy.expand_dims(input, axis=0)
new_dims = ()
i_i = len(input.shape) - 1
for i_t in range(len(target_shape) - 1, -1, -1):
@@ -3382,7 +3401,7 @@ def scatter_nd(
indices: Union[ivy.Array, ivy.NativeArray],
updates: Union[ivy.Array, ivy.NativeArray],
/,
- shape: Optional[Union[ivy.Shape, ivy.NativeShape]] = None,
+ shape: Optional[Union[tuple, list, ivy.Array, ivy.Shape, ivy.NativeShape]] = None,
*,
reduction: str = "sum",
out: Optional[ivy.Array] = None,
@@ -3412,24 +3431,46 @@ def scatter_nd(
Examples
--------
- scatter values into an empty array, With :class:`ivy.Array` input:
+ With :class:`ivy.Array` input:
- >>> indices = ivy.array([[4], [3], [1], [7]])
- >>> updates = ivy.array([9, 10, 11, 12])
+ >>> indices = ivy.array([[4], [3], [7], [7]])
+ >>> updates = ivy.array([9, 12, 11, 10])
>>> shape = ivy.array([8])
>>> scatter = ivy.scatter_nd(indices, updates, shape)
>>> print(scatter)
- ivy.array([ 0, 11, 0, 10, 9, 0, 0, 12])
+ ivy.array([ 0, 0, 0, 12, 9, 0, 0, 21])
+
+ >>> indices = ivy.array([[0, 1], [1, 0], [1, 1], [1, 1]])
+ >>> updates = ivy.array([9, 11, 12, 10])
+ >>> shape = (2, 2)
+ >>> scatter = ivy.scatter_nd(indices, updates, shape, reduction="max")
+ >>> print(scatter)
+ ivy.array([[ 0, 9], [11, 12]])
+
+ >>> indices = ivy.array([[[0], [1]], [[2], [1]]])
+ >>> updates = ivy.array([[9, 12], [11, 10]])
+ >>> shape = [4]
+ >>> scatter = ivy.scatter_nd(indices, updates, shape, reduction="replace")
+ >>> print(scatter)
+ ivy.array([ 9, 10, 11, 0])
+
+ >>> indices = ivy.array([[[1, 1], [0, 0]], [[1, 1], [0, 0]]])
+ >>> updates = ivy.array([[-1, 12], [11, 10]])
+ >>> shape = ivy.Shape([2, 2])
+ >>> result = ivy.zeros([2, 2])
+ >>> scatter = ivy.scatter_nd(indices, updates, shape, reduction="min", out=result)
+ >>> print(result)
+ ivy.array([[ 0., 0.], [ 0., -1.]])
- With scatter into an empty array, With :class:`ivy.Container` input:
+ With :class:`ivy.Container` input:
>>> indices = ivy.Container(a=ivy.array([[4],[3],[6]]),
... b=ivy.array([[5],[1],[2]]))
>>> updates = ivy.Container(a=ivy.array([100, 200, 200]),
... b=ivy.array([20, 30, 40]))
>>> shape = ivy.Container(a=ivy.array([10]),
- ... b = ivy.array([10]))
- >>> z = ivy.scatter_nd(indices, updates, shape=shape, reduction='replace')
+ ... b=ivy.array([10]))
+ >>> z = ivy.scatter_nd(indices, updates, shape=shape)
>>> print(z)
{
a: ivy.array([0, 0, 0, 200, 100, 0, 200, 0, 0, 0]),
@@ -3443,7 +3484,7 @@ def scatter_nd(
... b=ivy.array([200, 300, 400]))
>>> z = ivy.Container(a=ivy.array([1, 2, 3, 4, 5]),
... b=ivy.array([10, 20, 30, 40, 50]))
- >>> ivy.scatter_nd(indices, updates, reduction='replace', out=z)
+ >>> ivy.scatter_nd(indices, updates, reduction="replace", out=z)
>>> print(z)
{
a: ivy.array([1, 30, 3, 20, 10]),
@@ -3656,13 +3697,41 @@ def multiprocessing(context: Optional[str] = None):
Parameters
----------
context
- The context of the multiprocessing, either fork, forkserver or spawn.
+ The context of the multiprocessing, either 'fork', 'forkserver' or 'spawn'.
Default is ``None``.
Returns
-------
ret
Multiprocessing module
+
+ Examples
+ --------
+ >>> import ivy
+
+ Using the default context (None):
+
+ >>> mp_default = ivy.multiprocessing()
+ >>> print(mp_default)
+
+
+ Specifying 'fork' as the context:
+
+ >>> mp_fork = ivy.multiprocessing(context='fork')
+ >>> print(mp_fork)
+
+
+ Specifying 'spawn' as the context:
+
+ >>> mp_spawn = ivy.multiprocessing(context='spawn')
+ >>> print(mp_spawn)
+
+
+ Specifying 'forkserver' as the context:
+
+ >>> mp_forkserver = ivy.multiprocessing(context='forkserver')
+ >>> print(mp_forkserver)
+
"""
return current_backend().multiprocessing(context)
@@ -3875,12 +3944,16 @@ def _is_valid_device_and_dtypes_attributes(fn: Callable) -> bool:
if hasattr(fn, "unsupported_device_and_dtype"):
fn_unsupported_dnd = fn.unsupported_device_and_dtype
# if it's a nested dict, unwrap for the current backend
- if isinstance(list(fn_unsupported_dnd.__get__().values())[0], dict):
+ if fn_unsupported_dnd and isinstance(
+ list(fn_unsupported_dnd.__get__().values())[0], dict
+ ):
fn_unsupported_dnd = fn_unsupported_dnd.get(backend, {})
if hasattr(fn, "supported_device_and_dtype"):
fn_supported_dnd = fn.supported_device_and_dtype
# if it's a nested dict, unwrap for the current backend
- if isinstance(list(fn_supported_dnd.__get__().values())[0], dict):
+ if fn_supported_dnd and isinstance(
+ list(fn_supported_dnd.__get__().values())[0], dict
+ ):
fn_supported_dnd = fn_supported_dnd.get(backend, {})
ivy.utils.assertions.check_false(
@@ -3939,6 +4012,26 @@ def _dnd_dict_union(a, b):
return res
+# allow passing "integer" if all integer dtypes are supported/unsupported for e.g.
+def _expand_typesets(dtypes):
+ typesets = {
+ "valid": ivy.valid_dtypes,
+ "numeric": ivy.valid_numeric_dtypes,
+ "float": ivy.valid_float_dtypes,
+ "integer": ivy.valid_int_dtypes,
+ "unsigned": ivy.valid_uint_dtypes,
+ "complex": ivy.valid_complex_dtypes,
+ }
+ dtypes = list(dtypes)
+ typeset_list = []
+ for i, dtype in reversed(list(enumerate(dtypes))):
+ if dtype in typesets:
+ typeset_list.extend(typesets[dtype])
+ dtypes.pop(i)
+ dtypes += typeset_list
+ return dtypes
+
+
def _get_devices_and_dtypes(fn, recurse=False, complement=True):
supported_devices = ivy.function_supported_devices(fn, recurse=recurse)
supported_dtypes = ivy.function_supported_dtypes(fn, recurse=recurse)
@@ -3971,7 +4064,15 @@ def _get_devices_and_dtypes(fn, recurse=False, complement=True):
if "einops" in fn.__name__ and isinstance(fn_supported_dnd, dict):
fn_supported_dnd = fn_supported_dnd.get(backend, supported)
- ivy.utils.assertions.check_isinstance(list(fn_supported_dnd.values())[0], tuple)
+ if fn_supported_dnd:
+ ivy.utils.assertions.check_isinstance(
+ list(fn_supported_dnd.values())[0], tuple
+ )
+
+ if isinstance(fn_supported_dnd, dict):
+ for device, dtypes in fn_supported_dnd.items():
+ fn_supported_dnd[device] = tuple(_expand_typesets(dtypes))
+
# dict intersection
supported = _dnd_dict_intersection(supported, fn_supported_dnd)
@@ -3981,9 +4082,15 @@ def _get_devices_and_dtypes(fn, recurse=False, complement=True):
if "einops" in fn.__name__ and isinstance(fn_unsupported_dnd, dict):
fn_unsupported_dnd = fn_unsupported_dnd.get(backend, supported)
- ivy.utils.assertions.check_isinstance(
- list(fn_unsupported_dnd.values())[0], tuple
- )
+ if fn_unsupported_dnd:
+ ivy.utils.assertions.check_isinstance(
+ list(fn_unsupported_dnd.values())[0], tuple
+ )
+
+ if isinstance(fn_unsupported_dnd, dict):
+ for device, dtypes in fn_unsupported_dnd.items():
+ fn_unsupported_dnd[device] = tuple(_expand_typesets(dtypes))
+
# dict difference
supported = _dnd_dict_difference(supported, fn_unsupported_dnd)
@@ -4000,7 +4107,7 @@ def function_supported_devices_and_dtypes(fn: Callable, recurse: bool = True) ->
"""
Return the supported combination of devices and dtypes of the current backend's
function. The function returns a dict containing the supported combination of
- devices and dtypes of the primary and compositional implementations incase of
+ devices and dtypes of the primary and compositional implementations in case of
partial mixed functions.
Parameters
@@ -4049,7 +4156,7 @@ def function_unsupported_devices_and_dtypes(fn: Callable, recurse: bool = True)
"""
Return the unsupported combination of devices and dtypes of the current backend's
function. The function returns a dict containing the unsupported combination of
- devices and dtypes of the primary and compositional implementations incase of
+ devices and dtypes of the primary and compositional implementations in case of
partial mixed functions.
Parameters
@@ -4282,6 +4389,7 @@ def is_ivy_nested_array(x: Any, /) -> bool:
----------
x
The input to check
+
Returns
-------
ret
diff --git a/ivy/functional/ivy/gradients.py b/ivy/functional/ivy/gradients.py
index 2265680a9deca..6740e800462a4 100644
--- a/ivy/functional/ivy/gradients.py
+++ b/ivy/functional/ivy/gradients.py
@@ -109,7 +109,7 @@ def _get_required_float_variables(xs, xs_grad_idxs):
Also, returns a list of duplicate index chains for the nested
structure.
"""
- if (ivy.is_ivy_container(xs) or ivy.is_array(xs)) and xs_grad_idxs == [[0]]:
+ if (ivy.is_ivy_container(xs) or ivy.is_array(xs)) and xs_grad_idxs == ((0,),):
xs_grad_idxs = None
duplicate_index_chains = _get_duplicate_index_chains(xs)
xs = _to_ivy(xs)
@@ -133,7 +133,7 @@ def map_fn(x_):
if ivy.is_array(x_):
x_ = ivy.to_ivy(x_) if ivy.is_native_array(x_) else x_
if create_var:
- x_ = _variable(x_) if not _is_variable(x_, exclusive=True) else x_
+ x_ = x_ if _is_variable(x_, exclusive=True) else _variable(x_)
if len(x_.shape) == 0:
return ivy.to_native(x_)
if reshape:
@@ -271,6 +271,47 @@ def _non_finite_to_zero(xs):
)
+def _flatten_containers(inputs):
+ """
+ Flatten containers into a single tuple of arrays.
+
+ Returns a flattened tuple of arrays and the indices of the arrays in
+ the original containers.
+ """
+ if ivy.is_array(inputs) or ivy.is_ivy_container(inputs):
+ inputs = (inputs,)
+ values = []
+ ret_idxs = []
+ for idx, input in enumerate(inputs):
+ if isinstance(input, ivy.Container):
+ grad_arr_idxs = ivy.nested_argwhere(input, lambda x: ivy.is_array(x))
+ grad_arr_values = ivy.multi_index_nest(input, grad_arr_idxs)
+ values.extend(grad_arr_values)
+ ret_idxs.append(grad_arr_idxs)
+ elif ivy.is_array(input):
+ values.append(input)
+ ret_idxs.append(None)
+ return tuple(values), ret_idxs
+
+
+def _rebuild_flattened_containers(outputs, ret_idxs):
+ """Rebuild the containers from the flattened arrays into a single tuple."""
+ rebuilt_outputs = []
+ curr_idx = 0
+ for ret_idx in ret_idxs:
+ if ret_idx is None:
+ rebuilt_outputs.append(outputs[curr_idx])
+ curr_idx += 1
+ else:
+ cont = ivy.Container()
+ num_elements = len(ret_idx)
+ cont_outputs = outputs[curr_idx : curr_idx + num_elements]
+ ivy.insert_into_nest_at_indices(cont, ret_idx, cont_outputs)
+ rebuilt_outputs.append(cont)
+ curr_idx += num_elements
+ return tuple(rebuilt_outputs)
+
+
# Private Variable Helpers #
# -------------------------#
@@ -406,8 +447,8 @@ def execute_with_gradients(
/,
*,
retain_grads: bool = False,
- xs_grad_idxs: Optional[Sequence[Sequence[Union[str, int]]]] = [[0]],
- ret_grad_idxs: Optional[Sequence[Sequence[Union[str, int]]]] = [[0]],
+ xs_grad_idxs: Sequence[Sequence[Union[str, int]]] = ((0,),),
+ ret_grad_idxs: Sequence[Sequence[Union[str, int]]] = ((0,),),
) -> Tuple[ivy.Array, ivy.Array]:
"""
Call function func with input of xs variables, and return the function result
diff --git a/ivy/functional/ivy/layers.py b/ivy/functional/ivy/layers.py
index f0e4172f818a8..3ff5ea2d4dccb 100644
--- a/ivy/functional/ivy/layers.py
+++ b/ivy/functional/ivy/layers.py
@@ -24,6 +24,19 @@
# ------#
+def _get_embed_dim(
+ in_proj_weights, q_proj_weights, k_proj_weights, v_proj_weights, query
+):
+ pre_embed_dim = query.shape[-1]
+ if ivy.exists(in_proj_weights):
+ embed_dim = in_proj_weights.shape[0] / 3
+ elif all(ivy.exists(x) for x in [q_proj_weights, k_proj_weights, v_proj_weights]):
+ embed_dim = q_proj_weights.shape[0]
+ else:
+ embed_dim = None
+ return pre_embed_dim, embed_dim
+
+
def _in_projection(
q,
k,
@@ -32,9 +45,9 @@ def _in_projection(
b=None,
):
"""
- Projects query, key and value effeciently, depending on whether we are doing self-
+ Projects query, key and value efficiently, depending on whether we are doing self-
attention (query is key is value) or cross-attention (key is value) or an attention
- where query, key and value are all diferrent.
+ where query, key and value are all different.
it is only used in
multi_head_attention layer.
@@ -385,7 +398,7 @@ def dropout(
if prob == 0 or not training:
if dtype is not None:
x = ivy.astype(x, dtype)
- return x if not ivy.exists(out) else ivy.inplace_update(out, x)
+ return ivy.inplace_update(out, x) if ivy.exists(out) else x
if noise_shape is None:
noise_shape = x.shape
else:
@@ -402,7 +415,7 @@ def dropout(
x = x * mask
if scale:
x = ivy.multiply(x, 1.0 / (1.0 - prob), out=out)
- return x if not ivy.exists(out) else ivy.inplace_update(out, x)
+ return ivy.inplace_update(out, x) if ivy.exists(out) else x
dropout.mixed_backend_wrappers = {
@@ -460,7 +473,7 @@ def scaled_dot_product_attention(
The mask input array. The mask to apply to the query-key values. Default is
None. The shape of mask input should be in *[batch_shape,num_queries,num_keys]*.
dropout_p
- Specifies the dropout probablity, if greater than 0.0, dropout is applied
+ Specifies the dropout probability, if greater than 0.0, dropout is applied
is_causal
If true, assumes causal attention masking
and errors if both `mask` and `is_causal` are set.
@@ -678,7 +691,7 @@ def scaled_dot_product_attention(
"is_causal and attn_mask cannot be set at the same time",
)
embed_dim = query.shape[-1]
- scale = 1 / (embed_dim**0.5) if not scale else scale
+ scale = scale if scale else 1 / (embed_dim**0.5)
sim = ivy.einsum("... q f, ... k f -> ... q k", query, key) * scale
sim = ivy.dropout(sim, dropout_p, training=training)
if ivy.exists(mask):
@@ -699,7 +712,7 @@ def scaled_dot_product_attention(
)
attn = ivy.softmax(sim, axis=-1)
result = ivy.einsum("... qk, ...kf -> ...qf", attn, value)
- return result if not ivy.exists(out) else ivy.inplace_update(out, result)
+ return ivy.inplace_update(out, result) if ivy.exists(out) else result
@handle_exceptions
@@ -800,9 +813,11 @@ def multi_head_attention(
bias_v
An additional bias added to the value sequence. Shape: `(E,)`.
static_k
- A static key to be used in the attention operators. Shape: `(N*num_heads, S, E//num_heads)`.
+ A static key to be used in the attention operators.
+ Shape: `(N*num_heads, S, E//num_heads)`.
static_v
- A static value to be used in the attention operators. Shape: `(N*num_heads, S, E//num_heads)`.
+ A static value to be used in the attention operators.
+ Shape: `(N*num_heads, S, E//num_heads)`.
add_zero_attn
A boolean flag indicating whether to add a batch of zeros to key and value.
return_attention_weights
@@ -841,15 +856,15 @@ def multi_head_attention(
if key is None and value is None:
key = value = query
if num_dims == 2:
- query, key, value = (ivy.expand_dims(x, axis=0) for x in [query, key, value])
+ query, key, value = [ivy.expand_dims(x, axis=0) for x in [query, key, value]]
elif not batch_first:
- query, key, value = (ivy.swapaxes(x, 0, 1) for x in [query, key, value])
+ query, key, value = [ivy.swapaxes(x, 0, 1) for x in [query, key, value]]
# project query, key and value
if ivy.exists(in_proj_weights):
q, k, v = _in_projection(query, key, value, w=in_proj_weights, b=in_proj_bias)
emb_dim = int(in_proj_weights.shape[0] / 3)
- elif all([ivy.exists(x) for x in [q_proj_weights, k_proj_weights, v_proj_weights]]):
+ elif all(ivy.exists(x) for x in [q_proj_weights, k_proj_weights, v_proj_weights]):
if ivy.exists(in_proj_bias):
b_q, b_k, b_v = ivy.split(in_proj_bias, num_or_size_splits=3)
else:
@@ -904,7 +919,7 @@ def multi_head_attention(
# get attention scores
attn_scores = ivy.matmul(q, ivy.swapaxes(k, 1, 2))
- scale = 1 / (head_dim**0.5) if not scale else scale
+ scale = scale if scale else 1 / (head_dim**0.5)
attn_scores *= scale
# mask the attention scores
@@ -1843,15 +1858,15 @@ def conv3d_transpose(
>>> x = ivy.random_normal(mean=0, std=1, shape=[1, 3, 28, 28, 3])
>>> filters = ivy.random_normal(mean=0, std=1, shape=[3, 3, 3, 3, 6])
- >>> y = ivy.conv3d_transpose(x, filters, 2, 'SAME')
+ >>> y = ivy.conv3d_transpose(x, filters, [2, 2, 2], 'SAME')
>>> print(y.shape)
ivy.Shape(1, 6, 56, 56, 6)
- >>> x = ivy.random_normal(mean=0, std=1, shape=[1, 7, 256, 256, 64])
- >>> filters = ivy.random_normal(mean=0, std=1, shape=[3, 3, 3, 64, 32])
- >>> y = ivy.conv3d_transpose(x, filters, [1, 1, 1], 'VALID')
+ >>> x = ivy.random_normal(mean=0, std=1, shape=[1, 3, 64, 64, 3])
+ >>> filters = ivy.random_normal(mean=0, std=1, shape=[3, 3, 3, 3, 6])
+ >>> y = ivy.conv3d_transpose(x, filters, [2, 2, 2], 'VALID', dilations=[1, 1, 1])
>>> print(y.shape)
- ivy.Shape(1, 9, 258, 258, 32)
+ ivy.Shape(1, 7, 129, 129, 6)
With :class:`ivy.Container` inputs:
@@ -1861,7 +1876,7 @@ def conv3d_transpose(
>>> d = ivy.random_normal(mean=0, std=1, shape=[6, 3, 3, 3, 3])
>>> x = ivy.Container(a=a, b=b)
>>> filters = ivy.Container(c=c, d=d)
- >>> y = ivy.conv3d_transpose(x, filters, 2, 'SAME')
+ >>> y = ivy.conv3d_transpose(x, filters, [2, 2, 2], 'SAME')
>>> print(y.shape)
{
a: {
@@ -1885,22 +1900,21 @@ def conv3d_transpose(
With a mix of :class:`ivy.Array` and :class:`ivy.Container` inputs:
>>> x = ivy.full((1, 6, 6, 6, 1), 2.7)
- >>> a = ivy.random_normal(mean=0, std=1, shape=[3, 3, 3, 1, 1])
- >>> b = ivy.random_normal(mean=0, std=1, shape=[3, 3, 3, 1, 1])
- >>> filters = ivy.Container(a = a, b = b)
- >>> y = ivy.conv3d_transpose(x, filters, 1, 'VALID', dilations=1)
+ >>> a = ivy.random_normal(mean=0, std=1, shape=[3, 3, 3, 1, 1])
+ >>> b = ivy.random_normal(mean=0, std=1, shape=[3, 3, 3, 1, 1])
+ >>> filters = ivy.Container(a=a, b=b)
+ >>> y = ivy.conv3d_transpose(x, filters, [1, 1, 1], 'VALID', dilations=[1, 1, 1])
>>> print(y.shape)
{
a: ivy.Shape(1, 8, 8, 8, 1),
b: ivy.Shape(1, 8, 8, 8, 1)
}
-
>>> x = ivy.full((1, 6, 6, 6, 1), 1.23)
- >>> a = ivy.array(ivy.random_normal(mean=0, std=1, shape=[3, 3, 3, 1, 1]))
- >>> b = ivy.array(ivy.random_normal(mean=0, std=1, shape=[3, 3, 3, 1, 1]))
- >>> filters = ivy.Container(a = a, b = b)
- >>> y = ivy.conv3d_transpose(x, filters, 1, 'VALID', dilations=1)
+ >>> a = ivy.array(ivy.random_normal(mean=0, std=1, shape=[3, 3, 3, 1, 1]))
+ >>> b = ivy.array(ivy.random_normal(mean=0, std=1, shape=[3, 3, 3, 1, 1]))
+ >>> filters = ivy.Container(a=a, b=b)
+ >>> y = ivy.conv3d_transpose(x, filters, [1, 1, 1], 'VALID', dilations=[1, 1, 1])
>>> print(y.shape)
{
a: ivy.Shape(1, 8, 8, 8, 1),
@@ -2065,6 +2079,68 @@ def conv_general_transpose(
-------
ret
The result of the transpose convolution operation.
+
+ Examples
+ --------
+ With :class:`ivy.Array` input:
+ >>> x = ivy.random_normal(mean=0, std=1, shape=[1, 3, 28, 28, 3])
+ >>> filters = ivy.random_normal(mean=0, std=1, shape=[3, 3, 3, 3, 6])
+ >>> y = ivy.conv3d_transpose(x, filters, [2, 2, 2], 'SAME')
+ >>> print(y.shape)
+ ivy.Shape(1, 6, 56, 56, 6)
+ >>> x = ivy.random_normal(mean=0, std=1, shape=[1, 3, 64, 64, 3])
+ >>> filters = ivy.random_normal(mean=0, std=1, shape=[3, 3, 3, 3, 6])
+ >>> y = ivy.conv3d_transpose(x, filters, [2, 2, 2], 'VALID', dilations=[1, 1, 1])
+ >>> print(y.shape)
+ ivy.Shape(1, 7, 129, 129, 6)
+ With :class: 'ivy.Container' inputs:
+ >>> a = ivy.random_normal(mean=0, std=1, shape=[1, 3, 14, 14, 3])
+ >>> b = ivy.random_normal(mean=0, std=1, shape=[1, 3, 28, 28, 3])
+ >>> c = ivy.random_normal(mean=0, std=1, shape=[6, 3, 3, 3, 3])
+ >>> d = ivy.random_normal(mean=0, std=1, shape=[6, 3, 3, 3, 3])
+ >>> x = ivy.Container(a=a, b=b)
+ >>> filters = ivy.Container(c=c, d=d)
+ >>> y = ivy.conv3d_transpose(x, filters, [2, 2, 2], 'SAME')
+ >>> print(y.shape)
+ {
+ a: {
+ c: ivy.Shape(1, 6, 28, 28, 3),
+ d: ivy.Shape(1, 6, 28, 28, 3)
+ },
+ b: {
+ c: ivy.Shape(1, 6, 56, 56, 3),
+ d: ivy.Shape(1, 6, 56, 56, 3)
+ },
+ c: {
+ c: ivy.Shape(6, 6, 6, 6, 3),
+ d: ivy.Shape(6, 6, 6, 6, 3)
+ },
+ d: {
+ c: ivy.Shape(6, 6, 6, 6, 3),
+ d: ivy.Shape(6, 6, 6, 6, 3)
+ }
+ }
+ With a mix of :class:`ivy.Array` and :class:`ivy.Container` inputs:
+ >>> x = ivy.full((1, 6, 6, 6, 1), 2.7)
+ >>> a = ivy.random_normal(mean=0, std=1, shape=[3, 3, 3, 1, 1])
+ >>> b = ivy.random_normal(mean=0, std=1, shape=[3, 3, 3, 1, 1])
+ >>> filters = ivy.Container(a=a, b=b)
+ >>> y = ivy.conv3d_transpose(x, filters, [1, 1, 1], 'VALID', dilations=[1, 1, 1])
+ >>> print(y.shape)
+ {
+ a: ivy.Shape(1, 8, 8, 8, 1),
+ b: ivy.Shape(1, 8, 8, 8, 1)
+ }
+ >>> x = ivy.full((1, 6, 6, 6, 1), 1.23)
+ >>> a = ivy.array(ivy.random_normal(mean=0, std=1, shape=[3, 3, 3, 1, 1]))
+ >>> b = ivy.array(ivy.random_normal(mean=0, std=1, shape=[3, 3, 3, 1, 1]))
+ >>> filters = ivy.Container(a=a, b=b)
+ >>> y = ivy.conv3d_transpose(x, filters, [1, 1, 1], 'VALID', dilations=[1, 1, 1])
+ >>> print(y.shape)
+ {
+ a: ivy.Shape(1, 8, 8, 8, 1),
+ b: ivy.Shape(1, 8, 8, 8, 1)
+ }
"""
return current_backend(x).conv_general_transpose(
x,
@@ -2252,7 +2328,7 @@ def lstm_update(
ct = init_c
# lstm outputs
- hts_list = list()
+ hts_list = []
# unrolled time dimension with lstm steps
for Wii_xt, Wif_xt, Wig_xt, Wio_xt in zip(
@@ -2297,25 +2373,27 @@ def _handle_padding(x, strides, filters, padding):
return pad
-def _validate_max_pool_params(kernel, strides, padding, dilation, ceil_mode, dims):
+def _validate_max_pool_params(
+ kernel, strides, padding, dilation, ceil_mode, dims, data_format
+):
if isinstance(kernel, int):
kernel = (kernel,) * dims
elif len(kernel) == 1:
kernel = (kernel[0],) * dims
- elif (len(kernel) != dims) and (len(kernel) != dims + 2):
+ elif len(kernel) not in [dims, dims + 2]:
raise ValueError(
"The kernel should be an integer, or a tuple of length"
- f" {list(set((1, dims, dims+2)))}"
+ f" {list({1, dims, dims + 2})}"
)
if isinstance(strides, int):
strides = (strides,) * dims
elif len(strides) == 1:
strides = (strides[0],) * dims
- elif (len(strides) != dims) and (len(strides) != dims + 2):
+ elif len(strides) not in [dims, dims + 2]:
raise ValueError(
"The stride should be an integer, or a tuple of length"
- f" {list(set((1, dims, dims+2)))}"
+ f" {list({1, dims, dims + 2})}"
)
if isinstance(padding, int):
@@ -2325,7 +2403,7 @@ def _validate_max_pool_params(kernel, strides, padding, dilation, ceil_mode, dim
elif isinstance(padding, tuple) and len(padding) == dims:
padding = [(padding[i],) * 2 for i in range(dims)]
elif isinstance(padding, list) and len(padding) == dims:
- if not all([isinstance(p, tuple) and len(p) == 2 for p in padding]):
+ if not all(isinstance(p, tuple) and len(p) == 2 for p in padding):
raise ValueError("Explicit padding must be a list of tuple of two integers")
if isinstance(padding, str) and padding.upper() not in ["VALID", "SAME"]:
raise ValueError(
@@ -2338,7 +2416,7 @@ def _validate_max_pool_params(kernel, strides, padding, dilation, ceil_mode, dim
dilation = (dilation[0],) * dims
elif len(dilation) != dims:
raise ValueError(
- f"Dilation must be an integer or a tuple of length {list(set((1, dims)))}"
+ f"Dilation must be an integer or a tuple of length {list({1, dims})}"
)
if min(dilation) < 1:
raise ValueError("All values of `dilation` must be positive")
@@ -2348,14 +2426,25 @@ def _validate_max_pool_params(kernel, strides, padding, dilation, ceil_mode, dim
raise ValueError("When 'padding' is 'VALID', 'ceil_mode' must be False")
assert len(kernel) == len(strides), f"len({kernel}) must equal len({strides})"
+ ret = kernel, strides, padding, dilation
+
# Account for dilation when padding > kernel/2. Not the case in torch by default.
- new_kernel = tuple(
- [dilation[i] * (kernel[i] - 1) + 1 for i in range(1, len(kernel))]
- )
+ if len(dilation) < len(kernel):
+ if data_format[:2] == "NC":
+ dilation = [1, 1, *dilation]
+ else:
+ dilation = [1, *dilation, 1]
+ elif len(dilation) > len(kernel):
+ if data_format[:2] == "NC":
+ kernel = [1, 1, *kernel]
+ else:
+ kernel = [1, *kernel, 1]
+ new_kernel = tuple(dilation[i] * (kernel[i] - 1) + 1 for i in range(1, len(kernel)))
+ new_kernel = tuple(dilation[i] * (kernel[i] - 1) + 1 for i in range(1, len(kernel)))
if isinstance(padding, list) and len(padding) == len(new_kernel):
ivy.utils.assertions.check_kernel_padding_size(new_kernel, padding)
- return kernel, strides, padding, dilation
+ return ret
def _depth_max_pooling_helper(
@@ -2665,7 +2754,7 @@ def nms(
yy2 = ivy.minimum(y2[i], y2[order[1:]])
w = ivy.maximum(0.0, xx2 - xx1) # maximum width
- h = ivy.maximum(0.0, yy2 - yy1) # maxiumum height
+ h = ivy.maximum(0.0, yy2 - yy1) # maximum height
inter = w * h
ovr = inter / (areas[i] + areas[order[1:]] - inter)
inds = ivy.nonzero(ovr <= iou_threshold)[0]
diff --git a/ivy/functional/ivy/linear_algebra.py b/ivy/functional/ivy/linear_algebra.py
index 692d24c1bf4c2..54f8f94378641 100644
--- a/ivy/functional/ivy/linear_algebra.py
+++ b/ivy/functional/ivy/linear_algebra.py
@@ -1,4 +1,5 @@
# global
+
from typing import Union, Optional, Tuple, Literal, List, Sequence
# local
@@ -1849,7 +1850,7 @@ def qr(
[ 0.00000000e+00, 9.04534034e-01, 1.80906807e+00],
[ 0.00000000e+00, 0.00000000e+00, -8.88178420e-16]])
- # Note: if `int` values are used in `x` the output for q, r varry
+ # Note: if `int` values are used in `x` the output for q, r vary
>>> x = ivy.array([[1., 2.], [3., 4.]])
>>> q = ivy.zeros_like(x)
>>> r = ivy.zeros_like(x)
@@ -2008,8 +2009,8 @@ def solve(
out: Optional[ivy.Array] = None,
) -> ivy.Array:
"""
- Return the solution to the system of linear equations represented by the well-
- determined (i.e., full rank) linear matrix equation AX = B.
+ Return the solution x to the system of linear equations represented by the well-
+ determined (i.e., full rank) linear matrix equation Ax = B.
Parameters
----------
@@ -2018,7 +2019,7 @@ def solve(
form square matrices. Must be of full rank (i.e., all rows or, equivalently,
columns must be linearly independent). Should have a floating-point data type.
x2
- ordinate (or βdependent variableβ) array B. If x2 has shape (M,), x2 is
+ ordinate (or βdependent variableβ) array B. If x2 has shape (M,1), x2 is
equivalent to an array having shape (..., M, 1). If x2 has shape (..., M, K),
each column k defines a set of ordinate values for which to compute a solution,
and shape(x2)[:-1] must be compatible with shape(x1)[:-1] (see Broadcasting).
@@ -2037,32 +2038,88 @@ def solve(
(i.e., the array corresponding to B) and must have a floating-point data
type determined by Type Promotion Rules.
-
This function conforms to the `Array API Standard
`_. This docstring is an extension of the
`docstring `_
in the standard.
- Both the description and the type hints above assumes an array input for simplicity,
+ Both the description and the type hints above assume an array input for simplicity,
but this function is *nestable*, and therefore also accepts :class:`ivy.Container`
instances in place of any of the arguments.
Examples
--------
- with :class:`ivy.Array` input:
-
- >>> x1 = ivy.array([[1., 2.],[3., 4.]])
- >>> x2 = ivy.array([5., 6.])
- >>> out = ivy.solve(x1, x2)
- >>> print(out)
- ivy.array([-4. , 4.5])
-
- >>> x1 = ivy.native_array([[1., 2.],[3., 4.]])
- >>> x2 = ivy.array([5., 6.])
- >>> z = ivy.zeros_like(x2)
- >>> ivy.solve(x1, x2, out=z)
- ivy.array([-4. , 4.5])
+ With class:`ivy.Array` input:
+ >>> A = ivy.array([[1.1, 1.2, 1.3],
+ [2.1, 2.2, 2.3],
+ [3.1, 3.2, 3.3]]),
+ >>> B = ivy.array([[1.1],
+ [2.1],
+ [3.1]]),
+ >>> x = solve(A,B);
+ >>> print(x)
+ ivy.array([[1],
+ [0],
+ [0]])
+ >>> print(x.shape)
+ (1,3)
+
+ With shape(A) = (2,3,3) and shape(B) = (2,3,1):
+ >>> A = ivy.array([[[11.1, 11.2, 11.3],
+ [12.1, 12.2, 12.3],
+ [13.1, 13.2, 13.3]],
+ [[21.1, 21.2, 21.3],
+ [22.1, 22.2, 22.3],
+ [23.1, 23.2, 23.3]]
+ ]),
+ >>> B = ivy.array([[[11.1],
+ [12.1],
+ [13.1]],
+ [[21.1],
+ [22.1],
+ [23.1]]]),
+ >>> x = solve(A,B);
+ >>> print(x)
+ ivy.array([[[1],
+ [0],
+ [0]],
+ [[1],
+ [0],
+ [0]]])
+ >>> print(x.shape)
+ (2,1,3)
+
+ With shape(A) = (3,3) and shape(B) = (3,2):
+ >>> A = ivy.array([[1.1, 1.2, 1.3],
+ [2.1, 2.2, 2.3],
+ [3.1, 3.2, 3.3]]),
+ >>> B = ivy.array([[1.1, 2.2],
+ [2.1, 4.2],
+ [3.1, 6.2]]),
+ >>> x = solve(A,B);
+ >>> print(x)
+ ivy.array([[[1],
+ [0],
+ [0]],
+ [[2],
+ [0],
+ [0]]])
+ >>> print(x.shape)
+ (2,1,3)
+
+ With class:`ivy.Container` input:
+ >>> A = ivy.array([[1.1, 1.2, 1.3],
+ [2.1, 2.2, 2.3],
+ [3.1, 3.2, 3.3]]),
+ >>> B = ivy.container(B1 = ivy.array([[1.1], [2.1], [3.1]]),
+ B2 = ivy.array([[2.2], [4.2], [6.2]]))
+ >>> x = solve(A,B);
+ >>> print(x)
+ {
+ B1:([[1],[0],[0]]),
+ B2:([[2],[0],[0]])
+ }
"""
return current_backend(x1, x2).solve(x1, x2, adjoint=adjoint, out=out)
@@ -2207,7 +2264,11 @@ def svd(
@handle_array_function
@handle_device
def svdvals(
- x: Union[ivy.Array, ivy.NativeArray], /, *, out: Optional[ivy.Array] = None
+ x: Union[ivy.Array, ivy.NativeArray],
+ /,
+ *,
+ driver: Optional[str] = None,
+ out: Optional[ivy.Array] = None,
) -> ivy.Array:
"""
Return the singular values of a matrix (or a stack of matrices) ``x``.
@@ -2217,6 +2278,10 @@ def svdvals(
x
input array having shape ``(..., M, N)`` and whose innermost two dimensions form
``MxN`` matrices.
+ driver
+ optional output array,name of the cuSOLVER method to be used. This keyword
+ argument only works on CUDA inputs.
+ Available options are: None, gesvd, gesvdj, and gesvda.Default: None.
out
optional output array, for writing the result to. It must have a shape that the
inputs broadcast to.
@@ -2330,7 +2395,7 @@ def svdvals(
b: ivy.array([23.16134834, 10.35037804, 4.31025076, 1.35769391])
}
"""
- return current_backend(x).svdvals(x, out=out)
+ return current_backend(x).svdvals(x, driver=driver, out=out)
@handle_exceptions
@@ -2475,6 +2540,14 @@ def trace(
- ``offset < 0``: off-diagonal below the main diagonal.
Default: ``0``.
+ axis1
+ axis to be used as the first axis of the 2-D sub-arrays from which the
+ diagonals should be taken.
+ Defaults to ``0.`` .
+ axis2
+ axis to be used as the second axis of the 2-D sub-arrays from which the
+ diagonals should be taken.
+ Defaults to ``1.`` .
out
optional output array, for writing the result to. It must have a shape that the
inputs broadcast to.
@@ -2511,6 +2584,14 @@ def trace(
>>> print(y)
ivy.array([3., 4.])
+ >>> x = ivy.array([[1., 2., 3.],
+ ... [4., 5., 6.],
+ ... [7., 8., 9.]])
+ >>> y = ivy.zeros(1)
+ >>> ivy.trace(x, offset=1,out=y)
+ >>> print(y)
+ ivy.array(8.)
+
With :class:`ivy.NativeArray` inputs:
>>> x = ivy.native_array([[2., 0., 3.],[3., 5., 6.]])
@@ -2521,9 +2602,9 @@ def trace(
>>> x = ivy.native_array([[0, 1, 2],
... [3, 4, 5],
... [6, 7, 8]])
- >>> y = ivy.trace(x, offset=0)
+ >>> y = ivy.trace(x, offset=1)
>>> print(y)
- ivy.array(12)
+ ivy.array(6)
With :class:`ivy.Container` inputs:
@@ -2556,6 +2637,49 @@ def trace(
a: ivy.array(6),
b: ivy.array(8)
}
+
+ With multiple ivy.Container inputs:
+
+ >>> x = ivy.Container(
+ ... a = ivy.array([[7, 1, 3],
+ ... [8, 6, 5],
+ ... [9, 7, 2]]),
+ ... b = ivy.array([[4, 3, 2],
+ ... [1, 9, 5],
+ ... [7, 0, 6]])
+ ... )
+ >>> offset = ivy.Container(a=1, b=0)
+ >>> y = ivy.trace(x, offset)
+ >>> print(y)
+ {
+ a: ivy.array(6),
+ b: ivy.array(19)
+ }
+
+ With Array instance method example:
+
+ >>> x = ivy.array([[2., 0., 11.],
+ ... [3., 5., 12.],
+ ... [1., 6., 13.],
+ ... [8., 9., 14.]])
+ >>> y = x.trace(offset=1)
+ >>> print(y)
+ ivy.array(12.)
+
+ With Container instance method example:
+
+ >>> x = ivy.Container(
+ ... a=ivy.array([[2., 0., 11.],
+ ... [3., 5., 12.]]),
+ ... b=ivy.array([[1., 6., 13.],
+ ... [8., 9., 14.]])
+ ... )
+ >>> y = x.trace(offset=0)
+ >>> print(y)
+ {
+ a: ivy.array(7.),
+ b: ivy.array(10.)
+ }
"""
return current_backend(x).trace(x, offset=offset, axis1=axis1, axis2=axis2, out=out)
diff --git a/ivy/functional/ivy/losses.py b/ivy/functional/ivy/losses.py
index 04e1c2bedbfd0..64201cf60f566 100644
--- a/ivy/functional/ivy/losses.py
+++ b/ivy/functional/ivy/losses.py
@@ -41,7 +41,7 @@ def cross_entropy(
*,
axis: int = -1,
epsilon: float = 1e-7,
- reduction: str = "sum",
+ reduction: str = "mean",
out: Optional[ivy.Array] = None,
) -> ivy.Array:
"""
@@ -97,7 +97,7 @@ def binary_cross_entropy(
*,
from_logits: bool = False,
epsilon: float = 0.0,
- reduction: str = "none",
+ reduction: str = "mean",
pos_weight: Optional[Union[ivy.Array, ivy.NativeArray]] = None,
axis: Optional[int] = None,
out: Optional[ivy.Array] = None,
@@ -278,7 +278,7 @@ def sparse_cross_entropy(
*,
axis: int = -1,
epsilon: float = 1e-7,
- reduction: str = "sum",
+ reduction: str = "mean",
out: Optional[ivy.Array] = None,
) -> ivy.Array:
"""
diff --git a/ivy/functional/ivy/meta.py b/ivy/functional/ivy/meta.py
index 8cba29098b076..771facb671158 100644
--- a/ivy/functional/ivy/meta.py
+++ b/ivy/functional/ivy/meta.py
@@ -128,7 +128,7 @@ def _train_task(
):
# init
total_cost = 0
- all_grads = list()
+ all_grads = []
# inner and outer
unique_inner = inner_v is not None
@@ -324,8 +324,8 @@ def _train_tasks_with_for_loop(
stop_gradients,
):
total_cost = 0
- updated_ivs_to_return = list()
- all_grads = list()
+ updated_ivs_to_return = []
+ all_grads = []
if isinstance(inner_v, (list, tuple)) and isinstance(
inner_v[0], (list, tuple, dict, type(None))
):
@@ -755,7 +755,7 @@ def maml_step(
callable for the inner loop cost function, receiving sub-batch, inner vars and
outer vars
outer_cost_fn
- callable for the outer loop cost function, receving task-specific sub-batch,
+ callable for the outer loop cost function, receiving task-specific sub-batch,
inner vars and outer vars. If None, the cost from the inner loop will also be
optimized in the outer loop.
variables
@@ -805,6 +805,32 @@ def maml_step(
-------
ret
The cost and the gradients with respect to the outer loop variables.
+
+ Examples
+ --------
+ With :class:`ivy.Container` input:
+
+ >>> import ivy
+ >>> from ivy.functional.ivy.gradients import _variable
+
+ >>> ivy.set_backend("torch")
+
+ >>> def inner_cost_fn(sub_batch, v):
+ ... return sub_batch.mean().x / v.mean().latent
+ >>> def outer_cost_fn(sub_batch,v):
+ ... return sub_batch.mean().x / v.mean().latent
+
+ >>> num_tasks = 2
+ >>> batch = ivy.Container({"x": ivy.arange(1, num_tasks + 1, dtype="float32")})
+ >>> variables = ivy.Container({
+ ... "latent": _variable(ivy.repeat(ivy.array([[1.0]]), num_tasks, axis=0))
+ ... })
+
+ >>> cost = ivy.maml_step(batch, inner_cost_fn, outer_cost_fn, variables, 5, 0.01)
+ >>> print(cost)
+ (ivy.array(1.40069818), {
+ latent: ivy.array([-1.13723135])
+ }, ())
"""
if num_tasks is None:
num_tasks = batch.cont_shape[0]
diff --git a/ivy/functional/ivy/nest.py b/ivy/functional/ivy/nest.py
index 8de74443df967..cfe5a0a7f537b 100644
--- a/ivy/functional/ivy/nest.py
+++ b/ivy/functional/ivy/nest.py
@@ -132,7 +132,7 @@ def set_nest_at_index(
Whether to inplace update the input nest or not
Only works if nest is a mutable type. Default is ``True``.
_result
- Placeholder for the result of the update. do not set this paramter.
+ Placeholder for the result of the update. do not set this parameter.
Returns
-------
@@ -245,14 +245,27 @@ def insert_into_nest_at_index(nest: Iterable, index: Tuple, value) -> None:
>>> print(nest)
[[1, 2], [3, 99, 4]]
"""
- if len(index) == 1:
- idx = index[0]
- if isinstance(nest, list):
- nest.insert(idx, value)
+ if isinstance(nest, (dict, ivy.Container)):
+ if len(index) == 1:
+ key = index[0]
+ if isinstance(nest, dict):
+ nest[key] = value
else:
- nest[index[0]] = value
+ key = index[0]
+ if key in nest:
+ insert_into_nest_at_index(nest[key], index[1:], value)
+ else:
+ nest[key] = {}
+ insert_into_nest_at_index(nest[key], index[1:], value)
else:
- insert_into_nest_at_index(nest[index[0]], index[1:], value)
+ if len(index) == 1:
+ idx = index[0]
+ if isinstance(nest, list):
+ nest.insert(idx, value)
+ else:
+ nest[index[0]] = value
+ else:
+ insert_into_nest_at_index(nest[index[0]], index[1:], value)
@handle_exceptions
@@ -279,7 +292,7 @@ def map_nest_at_index(
Whether to inplace update the input nest or not
Only works if nest is a mutable type. Default is ``True``.
_result
- Placeholder for the result of the update. do not set this paramter.
+ Placeholder for the result of the update. do not set this parameter.
Returns
-------
@@ -664,7 +677,7 @@ def nested_argwhere(
nest
The nest to check the leaves of.
fn
- The conditon function, returning True or False.
+ The condition function, returning True or False.
check_nests
Whether to also check the nests for the condition, not only nest leaves.
Default is ``False``.
@@ -722,7 +735,7 @@ def nested_argwhere(
]
"""
to_ignore = ivy.default(to_ignore, ())
- _index = list() if _index is None else _index
+ _index = [] if _index is None else _index
if isinstance(nest, (tuple, list)) and not isinstance(nest, to_ignore):
n = 0
_indices = []
@@ -749,21 +762,16 @@ def nested_argwhere(
)
)
if stop_after_n_found is not None and ind:
- if n < stop_after_n_found:
- n += len(ind)
- _indices += [ind]
- else:
+ if n >= stop_after_n_found:
break
- else:
- _indices += [ind]
+ n += len(ind)
+ _indices += [ind]
if stop_after_n_found is not None and n >= stop_after_n_found:
break
_indices = [idx for idxs in _indices if idxs for idx in idxs]
if check_nests and fn(nest):
_indices.append(_index)
- elif (isinstance(nest, dict) or isinstance(nest, UserDict)) and not isinstance(
- nest, to_ignore
- ):
+ elif (isinstance(nest, (dict, UserDict))) and not isinstance(nest, to_ignore):
n = 0
_indices = []
for k, v in nest.items():
@@ -789,13 +797,10 @@ def nested_argwhere(
)
)
if stop_after_n_found is not None and ind:
- if n < stop_after_n_found:
- n += len(ind)
- _indices += [ind]
- else:
+ if n >= stop_after_n_found:
break
- else:
- _indices += [ind]
+ n += len(ind)
+ _indices += [ind]
_indices = [idx for idxs in _indices if idxs for idx in idxs]
if check_nests and fn(nest):
_indices.append(_index)
@@ -837,27 +842,44 @@ def all_nested_indices(
ret
A set of indices of all elements in nest
- Both the description and the type hints above assumes an array input
- for simplicity, but this function is nestable, and therefore also
- accepts :class:ivy.Container instances in place of the arguments.
-
Examples
--------
- With :class:`Dict` input:
+ With :code:`List` input:
+
+ >>> x = [189, [863, 672], [264, 384]]
+ >>> y = ivy.all_nested_indices(x)
+ >>> print(y)
+ [[0], [1, 0], [1, 1], [2, 0], [2, 1]]
+
+ With :code:`Tuple` input:
+
+ >>> x = (189, (863, 672), (264, 384))
+ >>> y = ivy.all_nested_indices(x, include_nests=True)
+ >>> print(y)
+ [[0], [1, 0], [1, 1], [1], [2, 0], [2, 1], [2]]
+
+ With :code:`Dict` input:
>>> x = {'a': 2., 'b': [6., [15., 9.]], 'c': (7., 56.)}
>>> y = ivy.all_nested_indices(x)
>>> print(y)
[['a'], ['b', 0], ['b', 1, 0], ['b', 1, 1], ['c', 0], ['c', 1]]
+ With :class:`ivy.Array` input:
+
+ >>> x = ivy.array([[True, False], [False, False]])
+ >>> y = ivy.all_nested_indices(x)
+ >>> print(y)
+ [[]]
+
With :class:`ivy.Container` input:
- >>> x = ivy.Container(a=ivy.array([0., 1., 2.]), b=ivy.array([3., 4., 5.]))
- >>> y = ivy.all_nested_indices(x, True)
+ >>> x = ivy.Container(a=ivy.array([412, 948, 482]), b=ivy.array([168, 674, 341]))
+ >>> y = ivy.all_nested_indices(x)
>>> print(y)
[['a'], ['b']]
"""
- _index = list() if _index is None else _index
+ _index = [] if _index is None else _index
if isinstance(nest, (tuple, list)):
_indices = [
all_nested_indices(
@@ -1229,7 +1251,7 @@ def nested_any(
nest
The nest to check the leaves of.
fn
- The conditon function, returning True or False.
+ The condition function, returning True or False.
check_nests
Whether to also check the nests for the condition, not only nest leaves.
Default is ``False``.
@@ -1395,6 +1417,7 @@ def nested_multi_map(
The configuration for the nests. Default is the same as nest0.
to_ivy
convert the output to ivy_arrays. Default is ``True``
+
Returns
-------
nest containing the result of the function. The structure of the output is the
@@ -1441,7 +1464,7 @@ def nested_multi_map(
key = (
str(index) if isinstance(nest, (tuple, list)) else list(nest)[index]
)
- this_index_chain = key if index_chain == "" else (index_chain + "/" + key)
+ this_index_chain = key if index_chain == "" else f"{index_chain}/{key}"
ret = ivy.nested_multi_map(
func,
values,
@@ -1565,7 +1588,7 @@ def prune_empty(nest):
"""
valid = False
if isinstance(nest, dict):
- keys = [k for k in nest]
+ keys = list(nest)
for k in keys:
nest[k] = prune_empty(nest[k])
if nest[k] is not None:
@@ -1582,6 +1605,6 @@ def prune_empty(nest):
for i in range(len(nest) - 1, -1, -1):
if nest[i] is None:
del nest[i]
- if not valid and not (ivy.is_array(nest) or isinstance(nest, (int, float, str))):
+ if not valid and not ivy.is_array(nest) and not isinstance(nest, (int, float, str)):
return None
return nest
diff --git a/ivy/functional/ivy/norms.py b/ivy/functional/ivy/norms.py
index 7d8277e0b0906..0af316281855b 100644
--- a/ivy/functional/ivy/norms.py
+++ b/ivy/functional/ivy/norms.py
@@ -1,6 +1,5 @@
"""Collection of Ivy normalization functions."""
-
# local
from typing import List, Union, Optional
import ivy
diff --git a/ivy/functional/ivy/set.py b/ivy/functional/ivy/set.py
index d0be2ccbef5ea..0c0d916b24cc4 100644
--- a/ivy/functional/ivy/set.py
+++ b/ivy/functional/ivy/set.py
@@ -158,6 +158,8 @@ def unique_all(
def unique_inverse(
x: Union[ivy.Array, ivy.NativeArray],
/,
+ *,
+ axis: Optional[int] = None,
) -> Tuple[Union[ivy.Array, ivy.NativeArray], Union[ivy.Array, ivy.NativeArray]]:
"""
Return the unique elements of an input array ``x``, and the indices from the set of
@@ -192,8 +194,12 @@ def unique_inverse(
Parameters
----------
x
- input array. If ``x`` has more than one dimension, the function must flatten
- ``x`` and return the unique elements of the flattened array.
+ the array that will be inputted into the "unique_inverse" function
+
+ axis
+ the axis to apply unique on. If None, the unique elements of the flattened ``x``
+ are returned.
+
Returns
-------
@@ -253,7 +259,7 @@ def unique_inverse(
b: ivy.array([1, 0, 3, 1, 4, 2, 5])
}]
"""
- return ivy.current_backend(x).unique_inverse(x)
+ return ivy.current_backend(x).unique_inverse(x, axis=axis)
@handle_exceptions
diff --git a/ivy/stateful/activations.py b/ivy/stateful/activations.py
index a2a789701a64b..635aa7dc30161 100644
--- a/ivy/stateful/activations.py
+++ b/ivy/stateful/activations.py
@@ -514,6 +514,7 @@ def _forward(self, x):
Inputs to process *[batch_shape, d]*.
alpha
scaler for controlling the slope of the function for x <= 0 Default: 1.0
+
Returns
-------
ret
diff --git a/ivy/stateful/converters.py b/ivy/stateful/converters.py
index 976a6851b4b9c..cb573bfb8ee9d 100644
--- a/ivy/stateful/converters.py
+++ b/ivy/stateful/converters.py
@@ -1,4 +1,5 @@
"""Converters from Native Modules to Ivy Modules."""
+
# global
from typing import Optional, Dict, List
import re # noqa
@@ -111,19 +112,19 @@ def from_haiku_module(
"""
try:
import haiku as hk
- except ModuleNotFoundError:
+ except ModuleNotFoundError as exc:
raise ModuleNotFoundError(
"`haiku` was not found installed on your system. Please proceed "
"to install it and restart your interpreter to see the changes."
- )
+ ) from exc
try:
from haiku._src.data_structures import FlatMapping # noqa
- except (ImportError, AttributeError):
+ except (ImportError, AttributeError) as exc:
raise ImportError(
"Unable to import `FlatMapping` from `haiku`. Please check if the "
"requested attribute exists."
- )
+ ) from exc
c_args = ivy.default(constructor_args, [])
c_kwargs = ivy.default(constructor_kwargs, {})
@@ -205,19 +206,19 @@ def from_flax_module(
"""
try:
import flax # noqa
- except ModuleNotFoundError:
+ except ModuleNotFoundError as exc:
raise ModuleNotFoundError(
"`flax` was not found installed on your system. Please proceed "
"to install it and restart your interpreter to see the changes."
- )
+ ) from exc
try:
import jax
- except ModuleNotFoundError:
+ except ModuleNotFoundError as exc:
raise ModuleNotFoundError(
"`jax` was not found installed on your system. Please proceed "
"to install it and restart your interpreter to see the changes."
- )
+ ) from exc
c_args = ivy.default(constructor_args, [])
c_kwargs = ivy.default(constructor_kwargs, {})
@@ -412,11 +413,11 @@ def from_torch_module(
"""
try:
import torch # noqa
- except ModuleNotFoundError:
+ except ModuleNotFoundError as exc:
raise ModuleNotFoundError(
"`torch` was not found installed on your system. Please proceed "
"to install it and restart your interpreter to see the changes."
- )
+ ) from exc
c_args = ivy.default(constructor_args, [])
c_kwargs = ivy.default(constructor_kwargs, {})
diff --git a/ivy/stateful/helpers.py b/ivy/stateful/helpers.py
index 2a352b4a0191b..b51489772bf0b 100644
--- a/ivy/stateful/helpers.py
+++ b/ivy/stateful/helpers.py
@@ -327,9 +327,8 @@ def v_with_top_v_key_chains(self, /, *, depth=None, flatten_key_chains=False):
return ret
else:
print(
- "both self.top_v and self.v must be initialized in order to show v in "
- "top_v, "
- "but found\n\ntop_v: {}\n\nv: {}.".format(self.top_v, self.v)
+ "both self.top_v and self.v must be initialized in order to show v in"
+ f" top_v, but found\n\ntop_v: {self.top_v}\n\nv: {self.v}."
)
def mod_with_top_mod_key_chain(self, /, *, depth=None, flatten_key_chain=False):
diff --git a/ivy/stateful/initializers.py b/ivy/stateful/initializers.py
index b9e2177329f8f..1481fce608f80 100644
--- a/ivy/stateful/initializers.py
+++ b/ivy/stateful/initializers.py
@@ -84,13 +84,13 @@ def create_variables(
class Zeros(Constant):
def __init__(self):
- """Constant initalizer that fills with the constant value `0.0`."""
+ """Constant initializer that fills with the constant value `0.0`."""
super().__init__(0.0)
class Ones(Constant):
def __init__(self):
- """Constant initalizer that fills with the constant value `1.0`."""
+ """Constant initializer that fills with the constant value `1.0`."""
super().__init__(1.0)
@@ -110,7 +110,7 @@ def __init__(self, numerator, fan_mode, power, gain):
is `0` and the variance is
`(gain * numerator / fan)^power / 4`.
- This is intended as a base-class for special predefined initialzers.
+ This is intended as a base-class for special predefined initializers.
Parameters
----------
@@ -218,7 +218,7 @@ def __init__(self):
"""
Initialize Glorot uniform, also known as the Xavier uniform initializer.
- It draws values from a uniform distribtion `[-limit, limit]` where
+ It draws values from a uniform distribution `[-limit, limit]` where
`limit = sqrt(6 / (fan_in + fan_out))` where `fan_in` and `fan_out` are the
number of input and output features respectively.
"""
@@ -230,7 +230,7 @@ def __init__(self):
"""
Initialize Siren uniform for the first layer.
- It draws values from a uniform distribtion `[-limit, limit]`
+ It draws values from a uniform distribution `[-limit, limit]`
where `limit=fan_in` where `fan_in` is the number of input
features.
"""
@@ -242,7 +242,7 @@ def __init__(self, w0=30):
"""
Initialize Siren uniform initializer for the first layer.
- It draws values from a uniform distribtion `[-limit, limit]`
+ It draws values from a uniform distribution `[-limit, limit]`
where `limit=sqrt(6 / fan_in) / w0` where `fan_in` is the number
of input features.
"""
diff --git a/ivy/stateful/layers.py b/ivy/stateful/layers.py
index 835cb9b2b0c14..17db6118a9a4c 100644
--- a/ivy/stateful/layers.py
+++ b/ivy/stateful/layers.py
@@ -1,4 +1,5 @@
"""Collection of Ivy neural network layers as stateful classes."""
+
# flake8: noqa
# local
import ivy
@@ -117,8 +118,9 @@ def _forward(self, x):
return ivy.linear(x, self.v.w, bias=self.v.b if self._with_bias else None)
def extra_repr(self) -> str:
- return "in_features={}, out_features={}, with_bias={}".format(
- self._input_channels, self._output_channels, self._with_bias is True
+ return (
+ f"in_features={self._input_channels}, out_features={self._output_channels},"
+ f" with_bias={self._with_bias is True}"
)
@@ -2235,7 +2237,7 @@ def __init__(
type
The type of the dct. Must be 1, 2, 3 or 4.
n
- The length of the transform. If n is less than the input signal lenght,
+ The length of the transform. If n is less than the input signal length,
then x is truncated, if n is larger then x is zero-padded.
axis
The axis to compute the DCT along.
diff --git a/ivy/stateful/module.py b/ivy/stateful/module.py
index c1cef9142de9d..68f1c425b7658 100644
--- a/ivy/stateful/module.py
+++ b/ivy/stateful/module.py
@@ -624,7 +624,7 @@ def __call__(
Parameters
----------
v
- If given, use this container as internal varibles temporarily.
+ If given, use this container as internal variables temporarily.
Default is ``None``.
track_submod_rets
If True, will track the returns of submodules.
@@ -868,10 +868,10 @@ def __repr__(self):
if isinstance(getattr(self, key, None), Module):
mod_str = repr(getattr(self, key))
mod_str = _addindent(mod_str, 2)
- child_lines.append("(" + key + "): " + mod_str)
+ child_lines.append(f"({key}): {mod_str}")
lines = extra_lines + child_lines
- main_str = self._get_name() + "("
+ main_str = f"{self._get_name()}("
if lines:
# simple one-liner info, which most builtin Modules will use
if len(extra_lines) == 1 and not child_lines:
@@ -1078,9 +1078,8 @@ def _forward(self, *a, **kw):
a, kw = ivy.args_to_native(*a, **kw)
params_hk = self._dict_to_hk_flat_map(self.v.cont_to_dict())
ret = self._native_module.apply(params_hk, 0, *a, **kw)
- if isinstance(ret, tuple):
- return ivy.args_to_native(*ret)
- return ivy.to_native(ret)
+ nested = True if isinstance(ret, tuple) else False
+ return ivy.to_native(ret, nested=nested)
def _hk_flat_map_to_dict(self, hk_flat_map):
from haiku._src.data_structures import FlatMapping
@@ -1142,9 +1141,8 @@ def _forward(self, *a, **kw):
a, kw = ivy.args_to_native(*a, **kw)
params_fx = flax.core.freeze(self.v.cont_to_dict())
ret = self._native_module.apply(params_fx, *a, **kw)
- if isinstance(ret, tuple):
- return ivy.args_to_native(*ret)
- return ivy.to_native(ret)
+ nested = True if isinstance(ret, tuple) else False
+ return ivy.to_native(ret, nested=nested)
class _KerasIvyModule(Module):
@@ -1169,9 +1167,8 @@ def _build(self, *args, **kwargs):
def _forward(self, *a, **kw):
a, kw = ivy.args_to_native(*a, **kw)
ret = self._native_module(*a, **kw)
- if isinstance(ret, tuple):
- return ivy.args_to_native(*ret)
- return ivy.to_native(ret)
+ nested = True if isinstance(ret, tuple) else False
+ return ivy.to_native(ret, nested=nested)
class _PaddleIvyModule(Module):
@@ -1201,9 +1198,8 @@ def _build(self, *args, **kwargs):
def _forward(self, *a, **kw):
a, kw = ivy.args_to_native(*a, **kw)
ret = self._native_module(*a, **kw)
- if isinstance(ret, tuple):
- return ivy.args_to_native(*ret)
- return ivy.to_native(ret)
+ nested = True if isinstance(ret, tuple) else False
+ return ivy.to_native(ret, nested=nested)
class _TorchIvyModule(Module):
@@ -1269,6 +1265,5 @@ def _forward(self, *a, **kw):
a, kw = ivy.args_to_native(*a, **kw)
self._update_v(self.v)
ret = self._native_module(*a, **kw)
- if isinstance(ret, tuple):
- return ivy.args_to_native(*ret)
- return ivy.to_native(ret)
+ nested = True if isinstance(ret, tuple) else False
+ return ivy.to_native(ret, nested=nested)
diff --git a/ivy/stateful/optimizers.py b/ivy/stateful/optimizers.py
index fdaafbe4097ac..9670c4e5aef7a 100644
--- a/ivy/stateful/optimizers.py
+++ b/ivy/stateful/optimizers.py
@@ -417,6 +417,85 @@ def state(self):
return ivy.Container({"mw": self._mw, "vw": self._vw})
+class AdamW(Adam):
+ def __init__(
+ self,
+ lr: float = 1e-4,
+ beta1: float = 0.9,
+ beta2: float = 0.999,
+ epsilon: float = 1e-07,
+ weight_decay: float = 0.0,
+ inplace: bool = True,
+ stop_gradients: bool = True,
+ trace_on_next_step: bool = False,
+ device: Optional[Union[ivy.Device, ivy.NativeDevice]] = None,
+ ):
+ """
+ Construct an ADAMW optimizer.
+
+ Parameters
+ ----------
+ lr
+ Learning rate, default is ``1e-4``.
+ beta1
+ gradient forgetting factor, default is ``0.9``
+ beta2
+ second moment of gradient forgetting factor, default is ``0.999``
+ epsilon
+ divisor during adamw update, preventing division by zero,
+ default is ``1e-07``
+ weight_decay
+ weight decay coefficient, default is ``0.0``
+ inplace
+ Whether to update the variables in-place, or to create new variable handles.
+ This is only relevant for frameworks with stateful variables such as
+ PyTorch.
+ Default is ``True``, provided the backend framework supports it.
+ stop_gradients
+ Whether to stop the gradients of the variables after each gradient step.
+ Default is ``True``.
+ trace_on_next_step
+ Whether to trace the optimizer on the next step. Default is ``False``.
+ device
+ Device on which to create the layer's variables 'cuda:0', 'cuda:1', 'cpu'
+ etc. (Default value = None)
+ """
+ self._weight_decay = weight_decay
+ super().__init__(
+ lr,
+ beta1,
+ beta2,
+ epsilon,
+ inplace,
+ stop_gradients,
+ trace_on_next_step,
+ device,
+ )
+
+ def _step(self, v: ivy.Container, grads: ivy.Container):
+ """
+ Update nested variables container v by AdamW update step, using nested grads
+ container.
+
+ Parameters
+ ----------
+ v
+ Nested variables to update.
+ grads
+ Nested gradients to update.
+
+ Returns
+ -------
+ ret
+ The updated variables, following AdamW update step.
+ """
+ # Apply L2 regularization directly to the parameters
+ if self._weight_decay != 0:
+ grads += self._weight_decay * v
+
+ return super()._step(v, grads)
+
+
class LAMB(Optimizer):
def __init__(
self,
diff --git a/ivy/utils/assertions.py b/ivy/utils/assertions.py
index ffc034cd1d013..3ce9cb927f8be 100644
--- a/ivy/utils/assertions.py
+++ b/ivy/utils/assertions.py
@@ -22,19 +22,13 @@ def _broadcast_inputs(x1, x2):
def check_less(x1, x2, allow_equal=False, message="", as_array=True):
- def comp_fn(x1, x2):
- return ivy.any(x1 > x2), ivy.any(x1 >= x2)
-
+ comp_fn = lambda x1, x2: (ivy.any(x1 > x2), ivy.any(x1 >= x2))
if not as_array:
-
- def iter_comp_fn(x1_, x2_):
- return any(x1 > x2 for x1, x2 in zip(x1_, x2_)), any(
- x1 >= x2 for x1, x2 in zip(x1_, x2_)
- )
-
- def comp_fn(x1, x2):
- return iter_comp_fn(*_broadcast_inputs(x1, x2))
-
+ iter_comp_fn = lambda x1_, x2_: (
+ any(x1 > x2 for x1, x2 in zip(x1_, x2_)),
+ any(x1 >= x2 for x1, x2 in zip(x1_, x2_)),
+ )
+ comp_fn = lambda x1, x2: iter_comp_fn(*_broadcast_inputs(x1, x2))
gt, gt_eq = comp_fn(x1, x2)
# less_equal
if allow_equal and gt:
@@ -48,19 +42,13 @@ def comp_fn(x1, x2):
def check_greater(x1, x2, allow_equal=False, message="", as_array=True):
- def comp_fn(x1, x2):
- return ivy.any(x1 < x2), ivy.any(x1 <= x2)
-
+ comp_fn = lambda x1, x2: (ivy.any(x1 < x2), ivy.any(x1 <= x2))
if not as_array:
-
- def iter_comp_fn(x1_, x2_):
- return any(x1 < x2 for x1, x2 in zip(x1_, x2_)), any(
- x1 <= x2 for x1, x2 in zip(x1_, x2_)
- )
-
- def comp_fn(x1, x2):
- return iter_comp_fn(*_broadcast_inputs(x1, x2))
-
+ iter_comp_fn = lambda x1_, x2_: (
+ any(x1 < x2 for x1, x2 in zip(x1_, x2_)),
+ any(x1 <= x2 for x1, x2 in zip(x1_, x2_)),
+ )
+ comp_fn = lambda x1, x2: iter_comp_fn(*_broadcast_inputs(x1, x2))
lt, lt_eq = comp_fn(x1, x2)
# greater_equal
if allow_equal and lt:
@@ -75,20 +63,11 @@ def comp_fn(x1, x2):
def check_equal(x1, x2, inverse=False, message="", as_array=True):
# not_equal
- def eq_fn(x1, x2):
- return x1 == x2 if inverse else x1 != x2
-
- def comp_fn(x1, x2):
- return ivy.any(eq_fn(x1, x2))
-
+ eq_fn = lambda x1, x2: (x1 == x2 if inverse else x1 != x2)
+ comp_fn = lambda x1, x2: ivy.any(eq_fn(x1, x2))
if not as_array:
-
- def iter_comp_fn(x1_, x2_):
- return any(eq_fn(x1, x2) for x1, x2 in zip(x1_, x2_))
-
- def comp_fn(x1, x2):
- return iter_comp_fn(*_broadcast_inputs(x1, x2))
-
+ iter_comp_fn = lambda x1_, x2_: any(eq_fn(x1, x2) for x1, x2 in zip(x1_, x2_))
+ comp_fn = lambda x1, x2: iter_comp_fn(*_broadcast_inputs(x1, x2))
eq = comp_fn(x1, x2)
if inverse and eq:
raise ivy.utils.exceptions.IvyException(
@@ -157,7 +136,7 @@ def check_all_or_any_fn(
*args,
fn,
type="all",
- limit=[0],
+ limit=(0,),
message="args must exist according to type and limit given",
as_array=True,
):
@@ -203,8 +182,8 @@ def check_same_dtype(x1, x2, message=""):
# -------- #
-def check_unsorted_segment_min_valid_params(data, segment_ids, num_segments):
- if not (isinstance(num_segments, int)):
+def check_unsorted_segment_valid_params(data, segment_ids, num_segments):
+ if not isinstance(num_segments, int):
raise ValueError("num_segments must be of integer type")
valid_dtypes = [
diff --git a/ivy/utils/backend/handler.py b/ivy/utils/backend/handler.py
index 1a91f47b41564..5a58a649c19fe 100644
--- a/ivy/utils/backend/handler.py
+++ b/ivy/utils/backend/handler.py
@@ -69,9 +69,8 @@ def _prevent_access_locally(*args, **kwargs):
@functools.lru_cache
def _get_backend_for_arg(arg_module_name):
- for backend in _backend_dict:
+ for backend, module_name in _backend_dict.items():
if backend in arg_module_name:
- module_name = _backend_dict[backend]
return importlib.import_module(module_name)
@@ -207,6 +206,8 @@ def _set_module_backend(
)
backend_str = backend.current_backend_str() if backend_str is None else backend_str
for k, v in original_dict.items():
+ if k in ivy.GLOBAL_PROPS:
+ continue
compositional = k not in backend.__dict__
if compositional:
if k in invalid_dtypes and k in target.__dict__:
@@ -237,11 +238,21 @@ def _handle_backend_specific_vars(target, backend):
target.set_global_attr("RNG", target.functional.backends.jax.random.RNG)
-def convert_from_source_backend_to_numpy(variable_ids, numpy_objs, devices):
- # Dynamic Backend
- from ivy.functional.ivy.gradients import _is_variable, _variable_data
+def _data_to_new_backend(x, previous_backend):
+ device = previous_backend.dev(x.data)
+ try:
+ result = ivy.from_dlpack(previous_backend.to_dlpack(x.data))
+ result = ivy.to_device(result, device)
+ except Exception:
+ np_res = previous_backend.to_numpy(x.data)
+ result = ivy.asarray(np_res, device=device)
+ return result
+
+
+def dynamic_backend_converter(backend_stack):
+ from ivy.functional.ivy.gradients import _variable
- def _is_var(obj):
+ def _is_var(obj, backend):
if isinstance(obj, ivy.Container):
def _map_fn(x):
@@ -253,7 +264,7 @@ def _map_fn(x):
):
return False
- return _is_variable(x)
+ return backend.gradients._is_variable(x)
return obj.cont_map(lambda x, kc: _map_fn(x)).cont_all_true()
@@ -265,7 +276,7 @@ def _map_fn(x):
"jaxlib.xla_extension",
):
return False
- return _is_variable(obj)
+ return backend.gradients._is_variable(obj)
# get all ivy array instances in the project scope
container_list = [
@@ -274,7 +285,8 @@ def _map_fn(x):
if "ivy" in type(obj).__module__ and isinstance(obj, ivy.Container)
]
cont_array_idxs = ivy.nested_argwhere(
- container_list, lambda x: isinstance(x, ivy.Array)
+ container_list,
+ lambda x: isinstance(x, ivy.Array) and x.backend != ivy.current_backend_str(),
)
cont_array_vals = ivy.multi_index_nest(container_list, cont_array_idxs)
array_list = [
@@ -284,69 +296,34 @@ def _map_fn(x):
]
array_list.extend(cont_array_vals)
- # filter uninitialized arrays and arrays with other bakcends, and ensure the order
+ # filter uninitialized arrays and arrays with other backends, and ensure the order
array_list = [
arr
for arr in array_list
- if arr.__dict__ and arr.backend == ivy.current_backend_str()
+ if arr.__dict__ and arr.backend != ivy.current_backend_str()
]
- arr_ids = [id(item.data) for item in array_list]
- new_objs = dict(zip(arr_ids, array_list))
- new_objs = list(new_objs.values())
+ new_objs = [obj for obj in array_list if obj.dynamic_backend]
# now convert all ivy.Array and ivy.Container instances
- # to numpy using the current backend
+ # to the new backend
+
for obj in new_objs:
- if obj.dynamic_backend:
- numpy_objs.append(obj)
- devices.append(obj.device)
- if _is_var(obj):
- # add variable object id to set
- variable_ids.add(id(obj))
- native_var = _variable_data(obj)
- np_data = ivy.to_numpy(native_var)
+ # the following if condition avoids converting arrays that were already
+ # updated inplace i.e. are references to other arrays
+ if obj.backend != ivy.current_backend_str():
+ backend = ivy.with_backend(obj.backend, cached=True)
+ if _is_var(obj, backend):
+ native_var = backend.gradients._variable_data(obj)
+ data = _data_to_new_backend(native_var, backend)
+ new_data = _variable(data)
else:
- np_data = obj.to_numpy()
+ new_data = _data_to_new_backend(obj, backend)
if isinstance(obj, ivy.Container):
- obj.cont_inplace_update(np_data)
+ obj.cont_inplace_update(new_data)
else:
- obj._data = np_data
-
- return variable_ids, numpy_objs, devices
-
-
-def convert_from_numpy_to_target_backend(variable_ids, numpy_objs, devices):
- # Dynamic Backend
- from ivy.functional.ivy.gradients import _variable
-
- # convert all ivy.Array and ivy.Container instances from numpy
- # to native arrays using the newly set backend
- for obj, device in zip(numpy_objs, devices):
- np_arr = obj.data if isinstance(obj, ivy.Array) else obj
- # check if object was originally a variable
- if id(obj) in variable_ids:
- native_arr = ivy.nested_map(
- lambda x: current_backend().asarray(x, device=device),
- np_arr,
- include_derived=True,
- shallow=False,
- )
- new_data = _variable(native_arr)
-
- else:
- new_data = ivy.nested_map(
- lambda x: current_backend().asarray(x, device=device),
- np_arr,
- include_derived=True,
- shallow=False,
- )
-
- if isinstance(obj, ivy.Container):
- obj.cont_inplace_update(new_data)
- else:
- obj.data = new_data.data
+ obj.data = new_data.data
@prevent_access_locally
@@ -379,16 +356,6 @@ def set_backend(backend: str, dynamic: bool = False):
f"backend must be one from {list(_backend_dict.keys())}",
)
- variable_ids = set() # create an empty set to store variable object ids
- numpy_objs = [] # create an empty list to store numpy objects
- devices = [] # create an empty list to store device strings
- # created during 1st conversion step
-
- if dynamic:
- variable_ids, numpy_objs, devices = convert_from_source_backend_to_numpy(
- variable_ids, numpy_objs, devices
- )
-
# update the global dict with the new backend
with ivy.locks["backend_setter"]:
global ivy_original_dict
@@ -417,7 +384,7 @@ def set_backend(backend: str, dynamic: bool = False):
ivy.functional.__dict__[key] = ivy.__dict__[key]
if dynamic:
- convert_from_numpy_to_target_backend(variable_ids, numpy_objs, devices)
+ dynamic_backend_converter(backend_stack)
for sub_backend in ivy.available_sub_backends:
ivy.set_sub_backend(sub_backend)
if verbosity.level > 0:
@@ -536,6 +503,8 @@ def previous_backend():
# wrap backend functions if there still is a backend, and add functions
# to ivy namespace
for k, v in new_backend_dict.items():
+ if k in ivy.GLOBAL_PROPS:
+ continue
if backend_stack and k in ivy_original_dict:
v = _wrap_function(k, v, ivy_original_dict[k])
if k in ivy_original_dict:
@@ -581,7 +550,7 @@ def choose_random_backend(excluded=None):
@prevent_access_locally
def with_backend(backend: str, cached: bool = True):
# Use already compiled object
- if cached and backend in compiled_backends.keys():
+ if cached and backend in compiled_backends:
cached_backend = compiled_backends[backend][-1]
return cached_backend
with _importlib.LocalIvyImporter():
diff --git a/ivy/utils/backend/sub_backend_handler.py b/ivy/utils/backend/sub_backend_handler.py
index 01b10dc44c634..525c52ff6eacc 100644
--- a/ivy/utils/backend/sub_backend_handler.py
+++ b/ivy/utils/backend/sub_backend_handler.py
@@ -44,6 +44,7 @@ def fn_name_from_version_specific_fn_name(name, version):
version
the version of the current framework for which the support is to be
provided, the version is inferred by importing the framework
+
Returns
-------
the name of the original function which will then point to the version
@@ -52,7 +53,7 @@ def fn_name_from_version_specific_fn_name(name, version):
"""
# TODO: add tests
version = str(version)
- if version.find("+") != -1:
+ if "+" in version:
version = tuple(map(int, version[: version.index("+")].split(".")))
else:
version = tuple(map(int, version.split(".")))
@@ -93,6 +94,7 @@ def fn_name_from_version_specific_fn_name_sub_backend(
version
the version of the current framework for which the support is to be
provided, the version is inferred by importing the framework
+
Returns
-------
the name of the original function which will then point to the version
@@ -102,12 +104,12 @@ def fn_name_from_version_specific_fn_name_sub_backend(
# TODO: add tests
sub_version = str(sub_backend_version)
back_version = str(backend_version)
- if sub_version.find("+") != -1:
+ if "+" in sub_version:
sub_version = tuple(map(int, sub_version[: sub_version.index("+")].split(".")))
else:
sub_version = tuple(map(int, sub_version.split(".")))
- if back_version.find("+") != -1:
+ if "+" in back_version:
back_version = tuple(
map(int, back_version[: back_version.index("+")].split("."))
)
@@ -164,7 +166,7 @@ def set_sub_backend(sub_backend_str: str):
logging.warning("You must set a backend first")
return
- if ivy.current_backend_str() not in _backend_to_sub_backends_dict.keys():
+ if ivy.current_backend_str() not in _backend_to_sub_backends_dict:
logging.warning(
f"backend {ivy.current_backend_str()} does not have any"
" supported sub_backends"
@@ -196,7 +198,7 @@ def set_sub_backend(sub_backend_str: str):
ivy.current_sub_backends.append(sub_backend_str)
-# this is very similiar to _set_backend_as_ivy in handler.py, with a minor change
+# this is very similar to _set_backend_as_ivy in handler.py, with a minor change
def _set_sub_backend_as_ivy(
original: dict, target: ModuleType, sub_backend: ModuleType
):
diff --git a/ivy/utils/binaries.py b/ivy/utils/binaries.py
index 059e1c91e45a9..d56ec9a9c35a3 100644
--- a/ivy/utils/binaries.py
+++ b/ivy/utils/binaries.py
@@ -1,8 +1,9 @@
import os
import logging
import json
-from pip._vendor.packaging import tags
+from packaging import tags
from urllib import request
+from tqdm import tqdm
def _get_paths_from_binaries(binaries, root_dir=""):
@@ -27,9 +28,9 @@ def check_for_binaries():
if os.path.exists(binaries_path):
binaries_dict = json.load(open(binaries_path))
available_configs = json.load(open(available_configs_path))
- binaries_paths = _get_paths_from_binaries(binaries_dict)
+ binaries_paths = _get_paths_from_binaries(binaries_dict, folder_path)
# verify if all binaries are available
- for _, path in enumerate(binaries_paths):
+ for path in binaries_paths:
if not os.path.exists(path):
if initial:
config_str = "\n".join(
@@ -63,45 +64,56 @@ def cleanup_and_fetch_binaries(clean=True):
if os.path.exists(binaries_path):
binaries_dict = json.load(open(binaries_path))
available_configs = json.load(open(available_configs_path))
- binaries_exts = {
- path.split(".")[-1] for path in _get_paths_from_binaries(binaries_dict)
- }
+ binaries_paths = _get_paths_from_binaries(binaries_dict, folder_path)
+ binaries_exts = {path.split(".")[-1] for path in binaries_paths}
# clean up existing binaries
if clean:
- print("Cleaning up existing binaries...")
+ print("Cleaning up existing binaries...", end="\r")
for root, _, files in os.walk(folder_path, topdown=True):
for file in files:
if file.split(".")[-1] in binaries_exts:
os.remove(os.path.join(root, file))
+ print("Cleaning up existing binaries --> done")
print("Downloading new binaries...")
all_tags = list(tags.sys_tags())
- binaries_paths = _get_paths_from_binaries(binaries_dict)
version = os.environ["VERSION"] if "VERSION" in os.environ else "main"
terminate = False
# download binaries for the tag with highest precedence
- for tag in all_tags:
- if terminate:
- break
- for path in binaries_paths:
- module = path.split(os.sep)[1]
- if os.path.exists(path) or str(tag) not in available_configs[module]:
- continue
- folders = path.split(os.sep)
- folder_path, file_path = os.sep.join(folders[:-1]), folders[-1]
- file_name = f"{file_path[:-3]}_{tag}.so"
- search_path = f"{module}/{file_name}"
- try:
- response = request.urlopen(
- "https://github.com/unifyai/binaries/raw/"
- f"{version}/{search_path}",
- timeout=40,
- )
- os.makedirs(os.path.dirname(path), exist_ok=True)
- with open(path, "wb") as f:
- f.write(response.read())
- terminate = path == binaries_paths[-1]
- except request.HTTPError:
+ with tqdm(total=len(binaries_paths)) as pbar:
+ for tag in all_tags:
+ if terminate:
break
+ for path in binaries_paths:
+ module = path[len(folder_path) :][1:].split(os.sep)[1]
+ if (
+ os.path.exists(path)
+ or str(tag) not in available_configs[module]
+ ):
+ continue
+ folders = path.split(os.sep)
+ _, file_path = os.sep.join(folders[:-1]), folders[-1]
+ file_name = f"{file_path[:-3]}_{tag}.so"
+ search_path = f"{module}/{file_name}"
+ try:
+ response = request.urlopen(
+ "https://github.com/unifyai/binaries/raw/"
+ f"{version}/{search_path}",
+ timeout=40,
+ )
+ os.makedirs(os.path.dirname(path), exist_ok=True)
+ with open(path, "wb") as f:
+ f.write(response.read())
+ terminate = path == binaries_paths[-1]
+ pbar.update(1)
+ except request.HTTPError:
+ break
+ if terminate:
+ print("Downloaded all binaries!")
+ else:
+ print(
+ "Couldn't download all binaries. Try importing ivy to get more "
+ "details about the missing binaries."
+ )
diff --git a/ivy/utils/einsum_parser.py b/ivy/utils/einsum_parser.py
index 689f529ac9fd7..b7b20b89fc22c 100644
--- a/ivy/utils/einsum_parser.py
+++ b/ivy/utils/einsum_parser.py
@@ -209,11 +209,11 @@ def convert_interleaved_input(
symbol: get_symbol(idx) for idx, symbol in enumerate(sorted(symbol_set))
}
- except TypeError: # unhashable or uncomparable object
+ except TypeError as e: # unhashable or uncomparable object
raise TypeError(
"For this input type lists must contain either Ellipsis "
"or hashable and comparable object (e.g. int, str)."
- )
+ ) from e
subscripts = ",".join(convert_subscripts(sub, symbol_map) for sub in subscript_list)
if output_list is not None:
@@ -238,6 +238,7 @@ def legalise_einsum_expr(*operands: Any) -> str:
-------
einsum_eqn : str
Legalised einsum equation
+
Examples
--------
The operand list is simplified to reduce printing:
diff --git a/ivy/utils/einsum_path_helpers.py b/ivy/utils/einsum_path_helpers.py
new file mode 100644
index 0000000000000..c627bc0894962
--- /dev/null
+++ b/ivy/utils/einsum_path_helpers.py
@@ -0,0 +1,644 @@
+# Helper functions for einsum_path, this file has been adapted from
+# `numpy core einsumfunc.py file` here
+# https://github.com/numpy/numpy/blob/v1.26.0/numpy/core/einsumfunc.py
+
+from itertools import combinations
+
+from ivy.utils.einsum_parser import possibly_convert_to_numpy, convert_interleaved_input
+
+einsum_symbols = "abcdefghijklmnopqrstuvwxyzABCDEFGHIJKLMNOPQRSTUVWXYZ"
+einsum_symbols_set = set(einsum_symbols)
+
+
+def flop_count(idx_contraction, inner, num_terms, size_dictionary):
+ """
+ Compute the number of FLOPS in the contraction.
+
+ Parameters
+ ----------
+ idx_contraction : iterable
+ The indices involved in the contraction
+ inner : bool
+ Does this contraction require an inner product?
+ num_terms : int
+ The number of terms in a contraction
+ size_dictionary : dict
+ The size of each of the indices in idx_contraction
+
+ Returns
+ -------
+ flop_count : int
+ The total number of FLOPS required for the contraction.
+
+ Examples
+ --------
+ >>> flop_count('abc', False, 1, {'a': 2, 'b':3, 'c':5})
+ 30
+
+ >>> flop_count('abc', True, 2, {'a': 2, 'b':3, 'c':5})
+ 60
+ """
+ overall_size = compute_size_by_dict(idx_contraction, size_dictionary)
+ op_factor = max(1, num_terms - 1)
+ if inner:
+ op_factor += 1
+
+ return overall_size * op_factor
+
+
+def compute_size_by_dict(indices, idx_dict):
+ """
+ Compute the product of the elements in indices based on the dictionary idx_dict.
+
+ Parameters
+ ----------
+ indices : iterable
+ Indices to base the product on.
+ idx_dict : dictionary
+ Dictionary of index sizes
+
+ Returns
+ -------
+ ret : int
+ The resulting product.
+
+ Examples
+ --------
+ >>> compute_size_by_dict('abbc', {'a': 2, 'b':3, 'c':5})
+ 90
+ """
+ ret = 1
+ for i in indices:
+ ret *= idx_dict[i]
+ return ret
+
+
+def find_contraction(positions, input_sets, output_set):
+ """
+ Find the contraction for a given set of input and output sets.
+
+ Parameters
+ ----------
+ positions : iterable
+ Integer positions of terms used in the contraction.
+ input_sets : list
+ List of sets that represent the lhs side of the einsum subscript
+ output_set : set
+ Set that represents the rhs side of the overall einsum subscript
+
+ Returns
+ -------
+ new_result : set
+ The indices of the resulting contraction
+ remaining : list
+ List of sets that have not been contracted, the new set is appended to
+ the end of this list
+ idx_removed : set
+ Indices removed from the entire contraction
+ idx_contraction : set
+ The indices used in the current contraction
+
+ Examples
+ --------
+ # A simple dot product test case
+ >>> pos = (0, 1)
+ >>> isets = [set('ab'), set('bc')]
+ >>> oset = set('ac')
+ >>> find_contraction(pos, isets, oset)
+ ({'a', 'c'}, [{'a', 'c'}], {'b'}, {'a', 'b', 'c'})
+ # A more complex case with additional terms in the contraction
+ >>> pos = (0, 2)
+ >>> isets = [set('abd'), set('ac'), set('bdc')]
+ >>> oset = set('ac')
+ >>> find_contraction(pos, isets, oset)
+ ({'a', 'c'}, [{'a', 'c'}, {'a', 'c'}], {'b', 'd'}, {'a', 'b', 'c', 'd'})
+ """
+ idx_contract = set()
+ idx_remain = output_set.copy()
+ remaining = []
+ for ind, value in enumerate(input_sets):
+ if ind in positions:
+ idx_contract |= value
+ else:
+ remaining.append(value)
+ idx_remain |= value
+
+ new_result = idx_remain & idx_contract
+ idx_removed = idx_contract - new_result
+ remaining.append(new_result)
+
+ return (new_result, remaining, idx_removed, idx_contract)
+
+
+def optimal_path(input_sets, output_set, idx_dict, memory_limit):
+ """
+ Compute all possible pair contractions, sieves the results based on ``memory_limit``
+ and returns the lowest cost path. This algorithm scales factorial with respect to
+ the elements in the list ``input_sets``.
+
+ Parameters
+ ----------
+ input_sets : list
+ List of sets that represent the lhs side of the einsum subscript
+ output_set : set
+ Set that represents the rhs side of the overall einsum subscript
+ idx_dict : dictionary
+ Dictionary of index sizes
+ memory_limit : int
+ The maximum number of elements in a temporary array
+
+ Returns
+ -------
+ path : list
+ The optimal contraction order within the memory limit constraint.
+
+ Examples
+ --------
+ >>> isets = [set('abd'), set('ac'), set('bdc')]
+ >>> oset = set()
+ >>> idx_sizes = {'a': 1, 'b':2, 'c':3, 'd':4}
+ >>> optimal_path(isets, oset, idx_sizes, 5000)
+ [(0, 2), (0, 1)]
+ """
+ full_results = [(0, [], input_sets)]
+ for iteration in range(len(input_sets) - 1):
+ iter_results = []
+
+ # Compute all unique pairs
+ for curr in full_results:
+ cost, positions, remaining = curr
+ for con in combinations(range(len(input_sets) - iteration), 2):
+ # Find the contraction
+ cont = find_contraction(con, remaining, output_set)
+ new_result, new_input_sets, idx_removed, idx_contract = cont
+
+ # Sieve the results based on memory_limit
+ new_size = compute_size_by_dict(new_result, idx_dict)
+ if new_size > memory_limit:
+ continue
+
+ # Build (total_cost, positions, indices_remaining)
+ total_cost = cost + flop_count(
+ idx_contract, idx_removed, len(con), idx_dict
+ )
+ new_pos = positions + [con]
+ iter_results.append((total_cost, new_pos, new_input_sets))
+
+ # Update combinatorial list, if we did not find anything return best
+ # path + remaining contractions
+ if iter_results:
+ full_results = iter_results
+ else:
+ path = min(full_results, key=lambda x: x[0])[1]
+ path += [tuple(range(len(input_sets) - iteration))]
+ return path
+
+ # If we have not found anything return single einsum contraction
+ if len(full_results) == 0:
+ return [tuple(range(len(input_sets)))]
+
+ path = min(full_results, key=lambda x: x[0])[1]
+ return path
+
+
+def parse_possible_contraction(
+ positions, input_sets, output_set, idx_dict, memory_limit, path_cost, naive_cost
+):
+ """
+ Compute the cost (removed size + flops) and resultant indices for performing the
+ contraction specified by ``positions``.
+
+ Parameters
+ ----------
+ positions : tuple of int
+ The locations of the proposed tensors to contract.
+ input_sets : list of sets
+ The indices found on each tensors.
+ output_set : set
+ The output indices of the expression.
+ idx_dict : dict
+ Mapping of each index to its size.
+ memory_limit : int
+ The total allowed size for an intermediary tensor.
+ path_cost : int
+ The contraction cost so far.
+ naive_cost : int
+ The cost of the unoptimized expression.
+
+ Returns
+ -------
+ cost : (int, int)
+ A tuple containing the size of any indices removed, and the flop cost.
+ positions : tuple of int
+ The locations of the proposed tensors to contract.
+ new_input_sets : list of sets
+ The resulting new list of indices if this proposed contraction is performed.
+ """
+ # Find the contraction
+ contract = find_contraction(positions, input_sets, output_set)
+ idx_result, new_input_sets, idx_removed, idx_contract = contract
+
+ # Sieve the results based on memory_limit
+ new_size = compute_size_by_dict(idx_result, idx_dict)
+ if new_size > memory_limit:
+ return None
+
+ # Build sort tuple
+ old_sizes = (compute_size_by_dict(input_sets[p], idx_dict) for p in positions)
+ removed_size = sum(old_sizes) - new_size
+
+ # NB: removed_size used to be just the size of any removed indices i.e.:
+ # helpers.compute_size_by_dict(idx_removed, idx_dict)
+ cost = flop_count(idx_contract, idx_removed, len(positions), idx_dict)
+ sort = (-removed_size, cost)
+
+ # Sieve based on total cost as well
+ if (path_cost + cost) > naive_cost:
+ return None
+
+ # Add contraction to possible choices
+ return [sort, positions, new_input_sets]
+
+
+def update_other_results(results, best):
+ """
+ Update the positions and provisional input_sets of ``results`` based on performing
+ the contraction result ``best``. Remove any involving the tensors contracted.
+
+ Parameters
+ ----------
+ results : list
+ List of contraction results produced by ``_parse_possible_contraction``.
+ best : list
+ The best contraction of ``results`` i.e. the one that will be performed.
+
+ Returns
+ -------
+ mod_results : list
+ The list of modified results, updated with outcome of ``best`` contraction.
+ """
+ best_con = best[1]
+ bx, by = best_con
+ mod_results = []
+
+ for cost, (x, y), con_sets in results:
+ # Ignore results involving tensors just contracted
+ if x in best_con or y in best_con:
+ continue
+
+ # Update the input_sets
+ del con_sets[by - int(by > x) - int(by > y)]
+ del con_sets[bx - int(bx > x) - int(bx > y)]
+ con_sets.insert(-1, best[2][-1])
+
+ # Update the position indices
+ mod_con = x - int(x > bx) - int(x > by), y - int(y > bx) - int(y > by)
+ mod_results.append((cost, mod_con, con_sets))
+
+ return mod_results
+
+
+def greedy_path(input_sets, output_set, idx_dict, memory_limit):
+ """
+ Find the path by contracting the best pair until the input list is exhausted. The
+ best pair is found by minimizing the tuple ``(-prod(indices_removed), cost)``. What
+ this amounts to is prioritizing matrix multiplication or inner product operations,
+ then Hadamard like operations, and finally outer operations. Outer products are
+ limited by ``memory_limit``. This algorithm scales cubically with respect to the
+ number of elements in the list ``input_sets``.
+
+ Parameters
+ ----------
+ input_sets : list
+ List of sets that represent the lhs side of the einsum subscript
+ output_set : set
+ Set that represents the rhs side of the overall einsum subscript
+ idx_dict : dictionary
+ Dictionary of index sizes
+ memory_limit : int
+ The maximum number of elements in a temporary array
+
+ Returns
+ -------
+ path : list
+ The greedy contraction order within the memory limit constraint.
+
+ Examples
+ --------
+ >>> isets = [set('abd'), set('ac'), set('bdc')]
+ >>> oset = set()
+ >>> idx_sizes = {'a': 1, 'b':2, 'c':3, 'd':4}
+ >>> greedy_path(isets, oset, idx_sizes, 5000)
+ [(0, 2), (0, 1)]
+ """
+ # Handle trivial cases that leaked through
+ if len(input_sets) == 1:
+ return [(0,)]
+ elif len(input_sets) == 2:
+ return [(0, 1)]
+
+ # Build up a naive cost
+ contract = find_contraction(range(len(input_sets)), input_sets, output_set)
+ idx_result, new_input_sets, idx_removed, idx_contract = contract
+ naive_cost = flop_count(idx_contract, idx_removed, len(input_sets), idx_dict)
+
+ # Initially iterate over all pairs
+ comb_iter = combinations(range(len(input_sets)), 2)
+ known_contractions = []
+
+ path_cost = 0
+ path = []
+
+ for iteration in range(len(input_sets) - 1):
+ # Iterate over all pairs on first step, only previously found
+ # pairs on subsequent steps
+ for positions in comb_iter:
+ # Always initially ignore outer products
+ if input_sets[positions[0]].isdisjoint(input_sets[positions[1]]):
+ continue
+
+ result = parse_possible_contraction(
+ positions,
+ input_sets,
+ output_set,
+ idx_dict,
+ memory_limit,
+ path_cost,
+ naive_cost,
+ )
+ if result is not None:
+ known_contractions.append(result)
+
+ # If we do not have a inner contraction, rescan pairs including outer products
+ if len(known_contractions) == 0:
+ # Then check the outer products
+ for positions in combinations(range(len(input_sets)), 2):
+ result = parse_possible_contraction(
+ positions,
+ input_sets,
+ output_set,
+ idx_dict,
+ memory_limit,
+ path_cost,
+ naive_cost,
+ )
+ if result is not None:
+ known_contractions.append(result)
+
+ # If we still did not find any remaining contractions,
+ # default back to einsum like behavior
+ if len(known_contractions) == 0:
+ path.append(tuple(range(len(input_sets))))
+ break
+
+ # Sort based on first index
+ best = min(known_contractions, key=lambda x: x[0])
+
+ # Now propagate as many unused contractions as possible to next iteration
+ known_contractions = update_other_results(known_contractions, best)
+
+ # Next iteration only compute contractions with the new tensor
+ # All other contractions have been accounted for
+ input_sets = best[2]
+ new_tensor_pos = len(input_sets) - 1
+ comb_iter = ((i, new_tensor_pos) for i in range(new_tensor_pos))
+
+ # Update path and total cost
+ path.append(best[1])
+ path_cost += best[0][1]
+
+ return path
+
+
+def can_dot(inputs, result, idx_removed):
+ """
+ Check if we can use BLAS (np.tensordot) call and its beneficial to do so.
+
+ Parameters
+ ----------
+ inputs : list of str
+ Specifies the subscripts for summation.
+ result : str
+ Resulting summation.
+ idx_removed : set
+ Indices that are removed in the summation
+
+ Returns
+ -------
+ type : bool
+ Returns true if BLAS should and can be used, else False
+
+ Notes
+ -----
+ If the operations is BLAS level 1 or 2 and is not already aligned
+ we default back to einsum as the memory movement to copy is more
+ costly than the operation itself.
+
+ Examples
+ --------
+ # Standard GEMM operation
+ >>> can_dot(['ij', 'jk'], 'ik', set('j'))
+ True
+ # Can use the standard BLAS, but requires odd data movement
+ >>> can_dot(['ijj', 'jk'], 'ik', set('j'))
+ False
+ # DDOT where the memory is not aligned
+ >>> can_dot(['ijk', 'ikj'], '', set('ijk'))
+ False
+ """
+ # All `dot` calls remove indices
+ if len(idx_removed) == 0:
+ return False
+
+ # BLAS can only handle two operands
+ if len(inputs) != 2:
+ return False
+
+ input_left, input_right = inputs
+
+ for c in set(input_left + input_right):
+ # can't deal with repeated indices on same input or more than 2 total
+ nl, nr = input_left.count(c), input_right.count(c)
+ if (nl > 1) or (nr > 1) or (nl + nr > 2):
+ return False
+
+ # can't do implicit summation or dimension collapse e.g.
+ # "ab,bc->c" (implicitly sum over 'a')
+ # "ab,ca->ca" (take diagonal of 'a')
+ if nl + nr - 1 == int(c in result):
+ return False
+
+ # Build a few temporaries
+ set_left = set(input_left)
+ set_right = set(input_right)
+ keep_left = set_left - idx_removed
+ keep_right = set_right - idx_removed
+ rs = len(idx_removed)
+
+ # At this point we are a DOT, GEMV, or GEMM operation
+
+ # Handle inner products
+
+ # DDOT with aligned data
+ if input_left == input_right:
+ return True
+
+ # DDOT without aligned data (better to use einsum)
+ if set_left == set_right:
+ return False
+
+ # Handle the 4 possible (aligned) GEMV or GEMM cases
+
+ # GEMM or GEMV no transpose
+ if input_left[-rs:] == input_right[:rs]:
+ return True
+
+ # GEMM or GEMV transpose both
+ if input_left[:rs] == input_right[-rs:]:
+ return True
+
+ # GEMM or GEMV transpose right
+ if input_left[-rs:] == input_right[-rs:]:
+ return True
+
+ # GEMM or GEMV transpose left
+ if input_left[:rs] == input_right[:rs]:
+ return True
+
+ # Einsum is faster than GEMV if we have to copy data
+ if not keep_left or not keep_right:
+ return False
+
+ # We are a matrix-matrix product, but we need to copy data
+ return True
+
+
+def parse_einsum_input(operands, subscripts=None):
+ """
+ Reproduction of einsum c side einsum parsing in python.
+
+ Returns
+ -------
+ input_strings : str
+ Parsed input strings
+ output_string : str
+ Parsed output string
+ operands : list of array_like
+ The operands to use in the numpy contraction
+
+ Examples
+ --------
+ The operand list is simplified to reduce printing:
+
+ >>> np.random.seed(123)
+ >>> a = np.random.rand(4, 4)
+ >>> b = np.random.rand(4, 4, 4)
+ >>> parse_einsum_input(('...a,...a->...', a, b))
+ ('za,xza', 'xz', [a, b]) # may vary
+ >>> parse_einsum_input((a, [Ellipsis, 0], b, [Ellipsis, 0]))
+ ('za,xza', 'xz', [a, b]) # may vary
+ """
+ if len(operands) == 0:
+ raise ValueError("No input operands")
+
+ if subscripts:
+ subscripts = subscripts.replace(" ", "")
+ operands = [possibly_convert_to_numpy(x) for x in operands]
+ elif isinstance(operands[0], str):
+ subscripts = operands[0].replace(" ", "")
+ operands = [possibly_convert_to_numpy(x) for x in operands[1:]]
+
+ else:
+ subscripts, operands = convert_interleaved_input(operands)
+
+ # Check for proper "->"
+ if ("-" in subscripts) or (">" in subscripts):
+ invalid = (subscripts.count("-") > 1) or (subscripts.count(">") > 1)
+ if invalid or (subscripts.count("->") != 1):
+ raise ValueError("Subscripts can only contain one '->'.")
+
+ # Parse ellipses
+ if "." in subscripts:
+ used = subscripts.replace(".", "").replace(",", "").replace("->", "")
+ unused = list(einsum_symbols_set - set(used))
+ ellipse_inds = "".join(unused)
+ longest = 0
+
+ if "->" in subscripts:
+ input_tmp, output_sub = subscripts.split("->")
+ split_subscripts = input_tmp.split(",")
+ out_sub = True
+ else:
+ split_subscripts = subscripts.split(",")
+ out_sub = False
+
+ for num, sub in enumerate(split_subscripts):
+ if "." in sub:
+ if (sub.count(".") != 3) or (sub.count("...") != 1):
+ raise ValueError("Invalid Ellipses.")
+
+ # Take into account numerical values
+ if operands[num].shape == ():
+ ellipse_count = 0
+ else:
+ ellipse_count = max(operands[num].ndim, 1)
+ ellipse_count -= len(sub) - 3
+
+ if ellipse_count > longest:
+ longest = ellipse_count
+
+ if ellipse_count < 0:
+ raise ValueError("Ellipses lengths do not match.")
+ elif ellipse_count == 0:
+ split_subscripts[num] = sub.replace("...", "")
+ else:
+ rep_inds = ellipse_inds[-ellipse_count:]
+ split_subscripts[num] = sub.replace("...", rep_inds)
+
+ subscripts = ",".join(split_subscripts)
+ if longest == 0:
+ out_ellipse = ""
+ else:
+ out_ellipse = ellipse_inds[-longest:]
+
+ if out_sub:
+ subscripts += "->" + output_sub.replace("...", out_ellipse)
+ else:
+ # Special care for outputless ellipses
+ output_subscript = ""
+ tmp_subscripts = subscripts.replace(",", "")
+ for s in sorted(set(tmp_subscripts)):
+ if s not in (einsum_symbols):
+ raise ValueError("Character %s is not a valid symbol." % s)
+ if tmp_subscripts.count(s) == 1:
+ output_subscript += s
+ normal_inds = "".join(sorted(set(output_subscript) - set(out_ellipse)))
+
+ subscripts += "->" + out_ellipse + normal_inds
+
+ # Build output string if does not exist
+ if "->" in subscripts:
+ input_subscripts, output_subscript = subscripts.split("->")
+ else:
+ input_subscripts = subscripts
+ # Build output subscripts
+ tmp_subscripts = subscripts.replace(",", "")
+ output_subscript = ""
+ for s in sorted(set(tmp_subscripts)):
+ if s not in einsum_symbols:
+ raise ValueError("Character %s is not a valid symbol." % s)
+ if tmp_subscripts.count(s) == 1:
+ output_subscript += s
+
+ # Make sure output subscripts are in the input
+ for char in output_subscript:
+ if char not in input_subscripts:
+ raise ValueError("Output character %s did not appear in the input" % char)
+
+ # Make sure number operands is equivalent to the number of terms
+ if len(input_subscripts.split(",")) != len(operands):
+ raise ValueError(
+ "Number of einsum subscripts must be equal to the number of operands."
+ )
+
+ return (input_subscripts, output_subscript, operands)
diff --git a/ivy/utils/exceptions.py b/ivy/utils/exceptions.py
index 9367d74c2f04c..d95aa7c55a337 100644
--- a/ivy/utils/exceptions.py
+++ b/ivy/utils/exceptions.py
@@ -374,7 +374,7 @@ def _handle_exceptions_helper(e, cls):
# Inplace Update
# to avoid raising warnings on setting the same backend multiple times
-_inplace_warning_cache = dict()
+_inplace_warning_cache = {}
def _handle_inplace_mode(ivy_pack=None):
@@ -383,7 +383,7 @@ def _handle_inplace_mode(ivy_pack=None):
current_backend = ivy_pack.current_backend_str()
if (
current_backend != ""
- and not _inplace_warning_cache.get(current_backend, None)
+ and not _inplace_warning_cache.get(current_backend)
and not ivy_pack.native_inplace_support
and ivy_pack.inplace_mode == "lenient"
):
diff --git a/ivy/utils/inspection.py b/ivy/utils/inspection.py
index 330fd1233e80d..2fa60f6f9d839 100644
--- a/ivy/utils/inspection.py
+++ b/ivy/utils/inspection.py
@@ -15,7 +15,7 @@ def _is_optional(typ):
):
return True
except BaseException as error:
- print(f"Exception occured: {error}")
+ print(f"Exception occurred: {error}")
return False
@@ -26,7 +26,7 @@ def _is_union(typ):
if rep.startswith("Union"):
return True
except BaseException as error:
- print(f"Exception occured: {error}")
+ print(f"Exception occurred: {error}")
return False
@@ -37,7 +37,7 @@ def _is_dict(typ):
if rep.startswith("Dict"):
return True
except BaseException as error:
- print(f"Exception occured: {error}")
+ print(f"Exception occurred: {error}")
return False
@@ -48,7 +48,7 @@ def _is_iterable(typ):
if rep.startswith("List") or rep.startswith("Tuple"):
return True
except BaseException as error:
- print(f"Exception occured: {error}")
+ print(f"Exception occurred: {error}")
return False
diff --git a/ivy_tests/array_api_testing/write_array_api_tests_k_flag.py b/ivy_tests/array_api_testing/write_array_api_tests_k_flag.py
index 97525da46dd5e..02d639cda2189 100644
--- a/ivy_tests/array_api_testing/write_array_api_tests_k_flag.py
+++ b/ivy_tests/array_api_testing/write_array_api_tests_k_flag.py
@@ -14,27 +14,27 @@
# test lists
framework_tests_to_run = {
- "jax": list(),
- "numpy": list(),
- "torch": list(),
- "tensorflow": list(),
+ "jax": [],
+ "numpy": [],
+ "torch": [],
+ "tensorflow": [],
}
framework_tests_to_skip = {
- "jax": list(),
- "numpy": list(),
- "torch": list(),
- "tensorflow": list(),
+ "jax": [],
+ "numpy": [],
+ "torch": [],
+ "tensorflow": [],
}
# add from each filepath
for fpath in fpaths:
# extract contents
- with open(fpath) as file:
+ with open(fpath, "r") as file:
contents = file.read()
# update tests to run and skip
contents = [line.replace("__", "") for line in contents.split("\n")]
for framework in framework_tests_to_run:
- tests_to_run = list()
- tests_to_skip = list()
+ tests_to_run = []
+ tests_to_skip = []
for s in contents:
if s == "":
continue
@@ -44,8 +44,8 @@
and any(f in s.lower() for f in framework_tests_to_run)
):
tests_to_run += (
- ["test_" + s]
- if ("#" not in s)
+ [f"test_{s}"]
+ if "#" not in s
else ["test_" + s.split("#")[1].split(" ")[0]]
)
else:
@@ -58,7 +58,7 @@
framework_tests_to_skip[framework] = [
tts
for tts in framework_tests_to_skip[framework]
- if not max([tts in ttr for ttr in framework_tests_to_run[framework]])
+ if not max(tts in ttr for ttr in framework_tests_to_run[framework])
]
diff --git a/ivy_tests/test_ivy/conftest.py b/ivy_tests/test_ivy/conftest.py
index 2677843431571..6316ce57043c8 100644
--- a/ivy_tests/test_ivy/conftest.py
+++ b/ivy_tests/test_ivy/conftest.py
@@ -28,6 +28,7 @@
UNSET_TEST_API_CONFIG = {"list": [], "flag": []}
TEST_PARAMS_CONFIG = []
+SKIP_GROUND_TRUTH = True
UNSUPPORTED_FRAEMWORK_DEVICES = {"numpy": ["gpu", "tpu"]}
if "ARRAY_API_TESTS_MODULE" not in os.environ:
os.environ["ARRAY_API_TESTS_MODULE"] = "ivy.functional.backends.numpy"
@@ -35,7 +36,7 @@
def default_framework_mapper(fw, fw_path="/opt/fw/", set_too=False):
# do a path search, get the latest
- # so that we can get the higest version
+ # so that we can get the highest version
# available dynamically and set that for
# use by the rest of the code
# eg: torch/1.11.0 and torch/1.12.0
@@ -190,7 +191,7 @@ def pytest_configure(config):
if "/" in backend_str:
backend_str = backend_str.split("/")[0]
if (
- backend_str in UNSUPPORTED_FRAEMWORK_DEVICES.keys()
+ backend_str in UNSUPPORTED_FRAEMWORK_DEVICES
and device.partition(":")[0]
in UNSUPPORTED_FRAEMWORK_DEVICES[backend_str]
):
@@ -239,12 +240,13 @@ def pytest_generate_tests(metafunc):
# Skip backend test against groud truth backend
# This redundant and wastes resources, as we going to be comparing
# The backend against it self
+ global SKIP_GROUND_TRUTH
if hasattr(metafunc.function, "ground_truth_backend"):
test_paramters = TEST_PARAMS_CONFIG.copy()
# Find the entries that contains the ground truth backend as it's backend
for entry in test_paramters.copy():
# Entry 1 is backend_fw
- if entry[1] == metafunc.function.ground_truth_backend:
+ if entry[1] == metafunc.function.ground_truth_backend and SKIP_GROUND_TRUTH:
test_paramters.remove(entry)
metafunc.parametrize(
"on_device,backend_fw,trace_graph,implicit", test_paramters
@@ -290,10 +292,14 @@ def process_cl_flags(config) -> Dict[str, bool]:
),
"transpile": (
False,
- getopt("--with-transpile-frontend"),
+ getopt("--with-transpile"),
),
}
+ # whether to skip gt testing or not
+ global SKIP_GROUND_TRUTH
+ SKIP_GROUND_TRUTH = not tmp_config["transpile"][1]
+
# final mapping for hypothesis value generation
for k, v in tmp_config.items():
# when both flags are true
@@ -346,7 +352,7 @@ def pytest_addoption(parser):
parser.addoption("--with-instance-method-testing", action="store_true")
parser.addoption("--with-gradient-testing", action="store_true")
parser.addoption("--with-trace-testing", action="store_true")
- parser.addoption("--with-transpile-frontend", action="store_true")
+ parser.addoption("--with-transpile", action="store_true")
parser.addoption("--no-extra-testing", action="store_true")
parser.addoption(
"--my_test_dump",
diff --git a/ivy_tests/test_ivy/helpers/assertions.py b/ivy_tests/test_ivy/helpers/assertions.py
index 8ec918cce7403..539d01b0d9243 100644
--- a/ivy_tests/test_ivy/helpers/assertions.py
+++ b/ivy_tests/test_ivy/helpers/assertions.py
@@ -46,7 +46,7 @@ def assert_all_close(
f" {ret_from_gt_dtype} datatype while the backend {backend} returned a"
f" {ret_dtype} datatype"
)
- # TODO eanble
+ # TODO enable
# if ivy.is_ivy_container(ret_np) and ivy.is_ivy_container(ret_from_gt_np):
# ivy.Container.cont_multi_map(assert_all_close, [ret_np, ret_from_gt_np])
# else:
diff --git a/ivy_tests/test_ivy/helpers/function_testing.py b/ivy_tests/test_ivy/helpers/function_testing.py
index 59be9657e2ca6..4529bfd2e9a8a 100644
--- a/ivy_tests/test_ivy/helpers/function_testing.py
+++ b/ivy_tests/test_ivy/helpers/function_testing.py
@@ -59,7 +59,7 @@ def _find_instance_in_args(backend: str, args, array_indices, mask):
array_indices
Indices of arrays that exists in the args
mask
- Boolean mask for whether the corrseponding element in (args) has a
+ Boolean mask for whether the corresponding element in (args) has a
generated test_flags.native_array as False or test_flags.container as
true
@@ -264,6 +264,26 @@ def target_fn(instance, *args, **kwargs):
"the array in out argument does not contain same value as the"
" returned"
)
+ if test_flags.with_copy:
+ array_fn = ivy_backend.is_array
+ if "copy" in list(inspect.signature(target_fn).parameters.keys()):
+ kwargs["copy"] = True
+ if instance_method:
+ first_array = instance
+ else:
+ first_array = ivy_backend.func_wrapper._get_first_array(
+ *args, array_fn=array_fn, **kwargs
+ )
+ ret_, ret_np_flat_ = get_ret_and_flattened_np_array(
+ fw,
+ target_fn,
+ *args,
+ test_trace=test_flags.test_trace,
+ precision_mode=test_flags.precision_mode,
+ **kwargs,
+ )
+ assert not np.may_share_memory(first_array, ret_)
+
ret_device = None
if isinstance(ret_from_target, ivy_backend.Array): # TODO use str for now
ret_device = ivy_backend.dev(ret_from_target)
@@ -449,7 +469,15 @@ def test_function(
>>> x2 = np.array([-3, 15, 24])
>>> test_function(input_dtypes, test_flags, fw, fn_name, x1=x1, x2=x2)
"""
+ _switch_backend_context(test_flags.test_trace or test_flags.transpile)
ground_truth_backend = test_flags.ground_truth_backend
+
+ if test_flags.container[0]:
+ test_flags.with_copy = False
+
+ if test_flags.with_copy is True:
+ test_flags.with_out = False
+
if mod_backend[backend_to_test]:
# multiprocessing
proc, input_queue, output_queue = mod_backend[backend_to_test]
@@ -546,6 +574,23 @@ def test_function(
fn_name,
)
+ if test_flags.transpile:
+ if mod_backend[backend_to_test]:
+ proc, input_queue, output_queue = mod_backend[backend_to_test]
+ input_queue.put(
+ (
+ "transpile_if_required_backend",
+ backend_to_test,
+ fn_name,
+ args_np,
+ kwargs_np,
+ )
+ )
+ else:
+ _transpile_if_required_backend(
+ backend_to_test, fn_name, args=args_np, kwargs=kwargs_np
+ )
+
# Gradient test
# TODO enable back , ADD backend_to_test to the call below
@@ -616,6 +661,57 @@ def test_function(
)
+def _assert_frontend_ret(ret, for_fn=True):
+ fn_or_method = "function" if for_fn else "method"
+ if not inspect.isclass(ret):
+ is_ret_tuple = issubclass(ret.__class__, tuple)
+ else:
+ is_ret_tuple = issubclass(ret, tuple)
+ if is_ret_tuple:
+ non_frontend_idxs = ivy.nested_argwhere(
+ ret, lambda _x: not _is_frontend_array(_x) if ivy.is_array(_x) else False
+ )
+ assert not non_frontend_idxs, (
+ f"Frontend {fn_or_method} return contains non-frontend arrays at positions"
+ f" {non_frontend_idxs} (zero-based):"
+ f" {ivy.multi_index_nest(ret, non_frontend_idxs)}"
+ )
+ elif ivy.is_array(ret):
+ assert _is_frontend_array(
+ ret
+ ), f"Frontend {fn_or_method} returned non-frontend array: {ret}"
+
+
+def _transpile_if_required_backend(backend: str, fn_name: str, args=None, kwargs=None):
+ iterations = 1
+ with BackendHandler.update_backend(backend) as ivy_backend:
+ args, kwargs = ivy_backend.args_to_ivy(*args, **kwargs)
+ backend_fn = ivy.__dict__[fn_name]
+ backend_traced_fn = traced_if_required(
+ backend, backend_fn, test_trace=True, args=args, kwargs=kwargs
+ )
+
+ func_timings = []
+ for i in range(0, iterations):
+ # timing the traced_fn
+ start = time.time()
+ backend_traced_fn(*args, **kwargs)
+ end = time.time()
+ func_timings.append(end - start)
+
+ func_time = np.mean(func_timings).item()
+ backend_nodes = len(backend_traced_fn._functions)
+
+ data = {
+ "fn_name": fn_name,
+ "args": str(args),
+ "kwargs": str(kwargs),
+ "time": func_time,
+ "nodes": backend_nodes,
+ }
+ _create_transpile_report(data, backend, "report.json", True)
+
+
def test_frontend_function(
*,
input_dtypes: Union[ivy.Dtype, List[ivy.Dtype]],
@@ -674,6 +770,10 @@ def test_frontend_function(
not test_flags.with_out or not test_flags.inplace
), "only one of with_out or with_inplace can be set as True"
+ if test_flags.with_copy is True:
+ test_flags.with_out = False
+ test_flags.inplace = False
+
# split the arguments into their positional and keyword components
args_np, kwargs_np = kwargs_to_args_n_kwargs(
num_positional_args=test_flags.num_positional_args, kwargs=all_as_kwargs_np
@@ -767,157 +867,126 @@ def test_frontend_function(
frontend_array_function=(
create_frontend_array if test_flags.test_trace else None
),
- as_ivy_arrays=(not test_flags.generate_frontend_arrays),
precision_mode=test_flags.precision_mode,
**kwargs_for_test,
)
- # test if frontend array was returned
- if test_flags.generate_frontend_arrays:
- assert ivy_backend.nested_map(
- lambda x: (_is_frontend_array(x) if ivy_backend.is_array(x) else True),
- ret,
- shallow=False,
- ), f"Frontend function returned non-frontend arrays: {ret}"
+ # test if return is frontend
+ _assert_frontend_ret(ret)
- if test_flags.with_out:
+ if test_flags.with_out and "out" in list(
+ inspect.signature(frontend_fn).parameters.keys()
+ ):
if not inspect.isclass(ret):
is_ret_tuple = issubclass(ret.__class__, tuple)
else:
is_ret_tuple = issubclass(ret, tuple)
-
- if test_flags.generate_frontend_arrays:
- if is_ret_tuple:
- ret = ivy_backend.nested_map(
- lambda _x: (
- arrays_to_frontend(
- backend=backend_to_test,
- frontend_array_fn=create_frontend_array,
- )(_x)
- if not _is_frontend_array(_x)
- else _x
- ),
- ret,
- include_derived=True,
- )
- elif not _is_frontend_array(ret):
- ret = arrays_to_frontend(
- backend=backend_to_test, frontend_array_fn=create_frontend_array
- )(ret)
- else:
- if is_ret_tuple:
- ret = ivy_backend.nested_map(
- lambda _x: (
- ivy_backend.array(_x)
- if not ivy_backend.is_array(_x)
- else _x
- ),
- ret,
- include_derived=True,
- )
- elif not ivy_backend.is_array(ret):
- ret = ivy_backend.array(ret)
-
out = ret
- # pass return value to out argument
- # check if passed reference is correctly updated
- kwargs["out"] = out
if is_ret_tuple:
- if test_flags.generate_frontend_arrays:
- flatten_ret = flatten_frontend(
- ret=ret,
- backend=backend_to_test,
- frontend_array_fn=create_frontend_array,
- )
- flatten_out = flatten_frontend(
- ret=out,
- backend=backend_to_test,
- frontend_array_fn=create_frontend_array,
- )
- else:
- flatten_ret = flatten(backend=backend_to_test, ret=ret)
- flatten_out = flatten(backend=backend_to_test, ret=out)
+ flatten_ret = flatten_frontend(
+ ret=ret,
+ backend=backend_to_test,
+ frontend_array_fn=create_frontend_array,
+ )
+ flatten_out = flatten_frontend(
+ ret=out,
+ backend=backend_to_test,
+ frontend_array_fn=create_frontend_array,
+ )
for ret_array, out_array in zip(flatten_ret, flatten_out):
if ivy_backend.native_inplace_support and not any(
(ivy_backend.isscalar(ret), ivy_backend.isscalar(out))
):
- if test_flags.generate_frontend_arrays:
- assert ret_array.ivy_array.data is out_array.ivy_array.data
- else:
- assert ret_array.data is out_array.data
+ assert ret_array.ivy_array.data is out_array.ivy_array.data
assert ret_array is out_array
else:
if ivy_backend.native_inplace_support and not any(
(ivy_backend.isscalar(ret), ivy_backend.isscalar(out))
):
- if test_flags.generate_frontend_arrays:
- assert ret.ivy_array.data is out.ivy_array.data
- else:
- assert ret.data is out.data
+ assert ret.ivy_array.data is out.ivy_array.data
assert ret is out
+ elif test_flags.with_copy:
+ assert _is_frontend_array(ret)
+
+ if "copy" in list(inspect.signature(frontend_fn).parameters.keys()):
+ copy_kwargs["copy"] = True
+ first_array = ivy_backend.func_wrapper._get_first_array(
+ *copy_args,
+ array_fn=(
+ _is_frontend_array
+ if test_flags.generate_frontend_arrays
+ else ivy_backend.is_array
+ ),
+ **copy_kwargs,
+ )
+ ret_ = get_frontend_ret(
+ backend_to_test,
+ frontend_fn,
+ *copy_args,
+ test_trace=test_flags.test_trace,
+ frontend_array_function=(
+ create_frontend_array if test_flags.test_trace else None
+ ),
+ precision_mode=test_flags.precision_mode,
+ **copy_kwargs,
+ )
+ if test_flags.generate_frontend_arrays:
+ first_array = first_array.ivy_array
+ ret_ = ret_.ivy_array
+ if "bfloat16" in str(ret_.dtype):
+ ret_ = ivy_backend.astype(ret_, ivy_backend.float64)
+ if "bfloat16" in str(first_array.dtype):
+ first_array = ivy_backend.astype(first_array, ivy_backend.float64)
+ if not ivy_backend.is_native_array(first_array):
+ first_array = first_array.data
+ ret_ = ret_.data
+ if hasattr(first_array, "requires_grad"):
+ first_array.requires_grad = False
+ assert not np.may_share_memory(first_array, ret_)
elif test_flags.inplace:
- assert not isinstance(ret, tuple)
-
- if test_flags.generate_frontend_arrays and not test_flags.test_trace:
- assert _is_frontend_array(ret)
- array_fn = _is_frontend_array
- else:
- assert ivy_backend.is_array(ret)
- array_fn = ivy_backend.is_array
+ assert _is_frontend_array(ret)
if "inplace" in list(inspect.signature(frontend_fn).parameters.keys()):
# the function provides optional inplace update
- # set inplace update to be True and check
- # if returned reference is inputted reference
- # and if inputted reference's content is correctly updated
copy_kwargs["inplace"] = True
- copy_kwargs["as_ivy_arrays"] = False
- first_array = ivy_backend.func_wrapper._get_first_array(
- *copy_args, array_fn=array_fn, **copy_kwargs
- )
- ret_ = get_frontend_ret(
- backend_to_test,
- frontend_fn,
- *copy_args,
- test_trace=test_flags.test_trace,
- frontend_array_function=(
- create_frontend_array if test_flags.test_trace else None
- ),
- precision_mode=test_flags.precision_mode,
- **copy_kwargs,
- )
+ # else the function provides inplace update by default
+
+ first_array = ivy_backend.func_wrapper._get_first_array(
+ *copy_args,
+ array_fn=(
+ _is_frontend_array
+ if test_flags.generate_frontend_arrays
+ else ivy_backend.is_array
+ ),
+ **copy_kwargs,
+ )
+ ret_ = get_frontend_ret(
+ backend_to_test,
+ frontend_fn,
+ *copy_args,
+ test_trace=test_flags.test_trace,
+ frontend_array_function=(
+ create_frontend_array if test_flags.test_trace else None
+ ),
+ precision_mode=test_flags.precision_mode,
+ **copy_kwargs,
+ )
+ if test_flags.generate_frontend_arrays:
assert first_array is ret_
- else:
- # the function provides inplace update by default
- # check if returned reference is inputted reference
- copy_kwargs["as_ivy_arrays"] = False
- first_array = ivy_backend.func_wrapper._get_first_array(
- *args, array_fn=array_fn, **kwargs
- )
- ret_ = get_frontend_ret(
- frontend_fn=frontend_fn,
- backend=backend_to_test,
- precision_mode=test_flags.precision_mode,
- test_trace=test_flags.test_trace,
- frontend_array_function=(
- create_frontend_array if test_flags.test_trace else None
- ),
- *args,
- **kwargs,
- )
- assert (
- first_array is ret_
- ), f"Inplace operation failed {first_array} != {ret_}"
+ elif (
+ ivy_backend.is_native_array(first_array)
+ and ivy_backend.inplace_arrays_supported()
+ ):
+ assert first_array is ret_.ivy_array.data
+ elif ivy_backend.is_ivy_array(first_array):
+ assert first_array.data is ret_.ivy_array.data
# create NumPy args
- if test_flags.generate_frontend_arrays:
+ if test_values:
ret_np_flat = flatten_frontend_to_np(
ret=ret,
- frontend_array_fn=create_frontend_array,
backend=backend_to_test,
)
- else:
- ret_np_flat = flatten_and_to_np(ret=ret, backend=backend_to_test)
if not test_values:
ret = ivy_backend.nested_map(
@@ -976,17 +1045,14 @@ def test_frontend_function(
frontend_fw_kwargs=kwargs_frontend,
)
- if frontend_config.isscalar(frontend_ret):
- frontend_ret_np_flat = [frontend_config.to_numpy(frontend_ret)]
- else:
- # tuplify the frontend return
- if not isinstance(frontend_ret, tuple):
- frontend_ret = (frontend_ret,)
- frontend_ret_idxs = ivy.nested_argwhere(
- frontend_ret, frontend_config.is_native_array
+ if test_values:
+ frontend_ret_np_flat = flatten_frontend_fw_to_np(
+ frontend_ret,
+ frontend_config.isscalar,
+ frontend_config.is_native_array,
+ frontend_config.to_numpy,
)
- frontend_ret_flat = ivy.multi_index_nest(frontend_ret, frontend_ret_idxs)
- frontend_ret_np_flat = [frontend_config.to_numpy(x) for x in frontend_ret_flat]
+
# assuming value test will be handled manually in the test function
if not test_values:
return (
@@ -1274,7 +1340,7 @@ def test_method_backend_computation(
init_input_dtypes = ivy.default(init_input_dtypes, [])
# Constructor arguments #
- init_all_as_kwargs_np = ivy.default(init_all_as_kwargs_np, dict())
+ init_all_as_kwargs_np = ivy.default(init_all_as_kwargs_np, {})
# split the arguments into their positional and keyword components
args_np_constructor, kwargs_np_constructor = kwargs_to_args_n_kwargs(
num_positional_args=init_flags.num_positional_args,
@@ -2065,8 +2131,8 @@ def test_frontend_method(
frontend_method_data.method_name
)(*copy_args_method, **copy_kwargs_method)
assert frontend_ret_ins is copy_ins, (
- "Inplace method did not return the same instance of the frontend array,"
- " expected {}, got {}".format(copy_ins, frontend_ret_ins)
+ "Inplace method did not return the same instance of the"
+ f" frontend array, expected {copy_ins}, got {frontend_ret_ins}"
)
ret = get_frontend_ret(
backend_to_test,
@@ -2075,26 +2141,18 @@ def test_frontend_method(
frontend_array_function=(
create_frontend_array if method_flags.test_trace else None
),
- as_ivy_arrays=(not method_flags.generate_frontend_arrays),
test_trace=method_flags.test_trace,
precision_mode=method_flags.precision_mode,
**kwargs_method_ivy,
)
- if method_flags.generate_frontend_arrays:
- assert ivy_backend.nested_map(
- lambda x: _is_frontend_array(x) if ivy_backend.is_array(x) else True,
- ret,
- ), f"Frontend method returned non-frontend arrays: {ret}"
+ # test if return is frontend
+ _assert_frontend_ret(ret, for_fn=False)
- if method_flags.generate_frontend_arrays:
- ret_np_flat = flatten_frontend_to_np(
- ret=ret,
- frontend_array_fn=create_frontend_array,
- backend=backend_to_test,
- )
- else:
- ret_np_flat = flatten_and_to_np(ret=ret, backend=backend_to_test)
+ ret_np_flat = flatten_frontend_to_np(
+ ret=ret,
+ backend=backend_to_test,
+ )
# Compute the return with the native frontend framework
frontend_config = get_frontend_config(frontend)
@@ -2154,17 +2212,13 @@ def test_frontend_method(
)
if frontend == "tensorflow" and isinstance(frontend_ret, tf.TensorShape):
frontend_ret_np_flat = [np.asarray(frontend_ret, dtype=np.int32)]
- elif frontend_config.isscalar(frontend_ret):
- frontend_ret_np_flat = [np.asarray(frontend_ret)]
else:
- # tuplify the frontend return
- if not isinstance(frontend_ret, tuple):
- frontend_ret = (frontend_ret,)
- frontend_ret_idxs = ivy.nested_argwhere(
- frontend_ret, frontend_config.is_native_array
+ frontend_ret_np_flat = flatten_frontend_fw_to_np(
+ frontend_ret,
+ frontend_config.isscalar,
+ frontend_config.is_native_array,
+ frontend_config.to_numpy,
)
- frontend_ret_flat = ivy.multi_index_nest(frontend_ret, frontend_ret_idxs)
- frontend_ret_np_flat = [frontend_config.to_numpy(x) for x in frontend_ret_flat]
# assuming value test will be handled manually in the test function
if not test_values:
@@ -2193,13 +2247,13 @@ def test_frontend_method(
def _get_framework_rtol(rtols: dict, current_fw: str):
- if current_fw in rtols.keys():
+ if current_fw in rtols:
return rtols[current_fw]
return DEFAULT_RTOL
def _get_framework_atol(atols: dict, current_fw: str):
- if current_fw in atols.keys():
+ if current_fw in atols:
return atols[current_fw]
return DEFAULT_ATOL
@@ -2340,27 +2394,35 @@ def flatten(*, backend: str, ret):
def flatten_frontend(*, ret, backend: str, frontend_array_fn=None):
- """Return a flattened numpy version of the frontend arrays in ret."""
+ """Return a flattened version of the frontend arrays in ret."""
if not isinstance(ret, tuple):
ret = (ret,)
-
with BackendHandler.update_backend(backend) as ivy_backend:
ret_idxs = ivy_backend.nested_argwhere(ret, _is_frontend_array)
-
- # handle scalars
- if len(ret_idxs) == 0:
+ if len(ret_idxs) == 0: # handle scalars
ret_idxs = ivy_backend.nested_argwhere(ret, ivy_backend.isscalar)
ret_flat = ivy_backend.multi_index_nest(ret, ret_idxs)
- ret_flat = [
- frontend_array_fn(x, dtype=ivy_backend.Dtype(str(np.asarray(x).dtype)))
- for x in ret_flat
- ]
+ ret_flat = [frontend_array_fn(x) for x in ret_flat]
else:
ret_flat = ivy_backend.multi_index_nest(ret, ret_idxs)
return ret_flat
+def flatten_frontend_fw_to_np(
+ frontend_ret, isscalar_func, is_native_array_func, to_numpy_func
+):
+ if not isinstance(frontend_ret, tuple):
+ frontend_ret = (frontend_ret,)
+ frontend_ret_idxs = ivy.nested_argwhere(frontend_ret, is_native_array_func)
+ if len(frontend_ret_idxs) == 0: # handle scalars
+ frontend_ret_idxs = ivy.nested_argwhere(frontend_ret, isscalar_func)
+ frontend_ret_flat = ivy.multi_index_nest(frontend_ret, frontend_ret_idxs)
+ else:
+ frontend_ret_flat = ivy.multi_index_nest(frontend_ret, frontend_ret_idxs)
+ return [to_numpy_func(x) for x in frontend_ret_flat]
+
+
def flatten_and_to_np(*, backend: str, ret):
# flatten the return
ret_flat = flatten(backend=backend, ret=ret)
@@ -2369,15 +2431,19 @@ def flatten_and_to_np(*, backend: str, ret):
return ret
-def flatten_frontend_to_np(*, backend: str, ret, frontend_array_fn=None):
+def flatten_frontend_to_np(*, backend: str, ret):
# flatten the return
-
- ret_flat = flatten_frontend(
- ret=ret, backend=backend, frontend_array_fn=frontend_array_fn
- )
-
+ if not isinstance(ret, tuple):
+ ret = (ret,)
with BackendHandler.update_backend(backend) as ivy_backend:
- return [ivy_backend.to_numpy(x.ivy_array) for x in ret_flat]
+ ret_idxs = ivy_backend.nested_argwhere(ret, _is_frontend_array)
+ if len(ret_idxs) == 0: # handle scalars
+ ret_idxs = ivy_backend.nested_argwhere(ret, ivy_backend.isscalar)
+ ret_flat = ivy_backend.multi_index_nest(ret, ret_idxs)
+ return [ivy_backend.to_numpy(x) for x in ret_flat]
+ else:
+ ret_flat = ivy_backend.multi_index_nest(ret, ret_idxs)
+ return [ivy_backend.to_numpy(x.ivy_array) for x in ret_flat]
def get_ret_and_flattened_np_array(
@@ -2411,7 +2477,6 @@ def get_frontend_ret(
frontend_fn,
*args,
frontend_array_function=None,
- as_ivy_arrays=True,
precision_mode=False,
test_trace: bool = False,
**kwargs,
@@ -2420,26 +2485,18 @@ def get_frontend_ret(
backend, frontend_fn, test_trace=test_trace, args=args, kwargs=kwargs
)
with BackendHandler.update_backend(backend) as ivy_backend:
- if not as_ivy_arrays and test_trace:
+ if test_trace:
args, kwargs = ivy_backend.nested_map(
_frontend_array_to_ivy, (args, kwargs), include_derived={"tuple": True}
)
with ivy_backend.PreciseMode(precision_mode):
ret = frontend_fn(*args, **kwargs)
- if test_trace and frontend_array_function is not None:
- if as_ivy_arrays:
- ret = ivy_backend.nested_map(
- ivy_backend.asarray, ret, include_derived={"tuple": True}
- )
- else:
- ret = ivy_backend.nested_map(
- arrays_to_frontend(backend, frontend_array_function),
- ret,
- include_derived={"tuple": True},
- )
- elif as_ivy_arrays:
+ if test_trace:
+ assert frontend_array_function is not None
ret = ivy_backend.nested_map(
- _frontend_array_to_ivy, ret, include_derived={"tuple": True}
+ arrays_to_frontend(backend, frontend_array_function),
+ ret,
+ include_derived={"tuple": True},
)
return ret
@@ -2507,9 +2564,9 @@ def _get_transpiled_data_if_required(
"frontend_func": fn_name,
"args": str(args_for_test),
"kwargs": str(kwargs_for_test),
- "frontend_time": frontend_time,
- "frontend_fw_time": frontend_fw_time,
- "backend_nodes": backend_nodes,
+ "time": frontend_time,
+ "fw_time": frontend_fw_time,
+ "nodes": backend_nodes,
"ivy_nodes": ivy_nodes,
}
@@ -2590,10 +2647,10 @@ def args_to_frontend(
return frontend_args, frontend_kwargs
-def arrays_to_frontend(backend: str, frontend_array_fn=None):
+def arrays_to_frontend(backend: str, frontend_array_fn):
with BackendHandler.update_backend(backend) as ivy_backend:
- def _new_fn(x, *args, **kwargs):
+ def _new_fn(x):
if _is_frontend_array(x):
return x
elif ivy_backend.is_array(x):
diff --git a/ivy_tests/test_ivy/helpers/globals.py b/ivy_tests/test_ivy/helpers/globals.py
index cca30420ab260..8d1d7f2f191d2 100644
--- a/ivy_tests/test_ivy/helpers/globals.py
+++ b/ivy_tests/test_ivy/helpers/globals.py
@@ -5,7 +5,6 @@
Should not be used inside any of the test functions.
"""
-
from dataclasses import dataclass
from .pipeline_helper import get_frontend_config
@@ -38,7 +37,7 @@
"mxnet": None,
} # multiversion
-# This is used to make sure the variable is not being overriden
+# This is used to make sure the variable is not being overridden
_Notsetval = object()
CURRENT_GROUND_TRUTH_BACKEND: callable = _Notsetval
CURRENT_BACKEND: callable = _Notsetval
@@ -62,8 +61,8 @@ class TestData:
class InterruptedTest(BaseException):
"""Indicate that a test tried to write global attributes while a test is running."""
- def __init__(self, test_interruped):
- super.__init__(f"{test_interruped} was interruped during execution.")
+ def __init__(self, test_interrupted):
+ super.__init__(f"{test_interrupted} was interrupted during execution.")
# Setup
diff --git a/ivy_tests/test_ivy/helpers/hypothesis_helpers/array_helpers.py b/ivy_tests/test_ivy/helpers/hypothesis_helpers/array_helpers.py
index 1cb1f47c0de42..51a5dbcf99f8a 100644
--- a/ivy_tests/test_ivy/helpers/hypothesis_helpers/array_helpers.py
+++ b/ivy_tests/test_ivy/helpers/hypothesis_helpers/array_helpers.py
@@ -1790,22 +1790,18 @@ def arrays_for_pooling(
)
if array_dim == 3:
kernel = draw(st.tuples(st.integers(1, in_shape[1])))
- new_kernel = kernel
if return_dilation:
- new_kernel = []
dilations = []
for i in range(len(kernel)):
if kernel[i] > 1:
max_dilation = (in_shape[i + 1] - kernel[i]) // (kernel[i] - 1) + 1
dilations.append(draw(st.integers(1, max_dilation)))
- new_kernel.append(kernel[i] + (kernel[i] - 1) * (dilations[i] - 1))
else:
dilations.append(1)
- new_kernel.append(kernel[i])
if explicit_or_str_padding or only_explicit_padding:
padding = []
for i in range(array_dim - 2):
- max_pad = new_kernel[i] // 2
+ max_pad = kernel[i] // 2
padding.append(
draw(
st.tuples(
@@ -2244,7 +2240,7 @@ def create_concatenable_arrays_dtypes(
def get_first_solve_batch_matrix(draw, choose_adjoint=False):
"""
Generate non-singular left hand side of equation system possibly with a single batch
- dimension at the begining. Use get_second_solve_batch_matrix to get the right hand
+ dimension at the beginning. Use get_second_solve_batch_matrix to get the right hand
side.
Parameters
diff --git a/ivy_tests/test_ivy/helpers/hypothesis_helpers/dtype_helpers.py b/ivy_tests/test_ivy/helpers/hypothesis_helpers/dtype_helpers.py
index 9867f798f856e..31742e5519501 100644
--- a/ivy_tests/test_ivy/helpers/hypothesis_helpers/dtype_helpers.py
+++ b/ivy_tests/test_ivy/helpers/hypothesis_helpers/dtype_helpers.py
@@ -127,7 +127,7 @@ def get_dtypes(
Supported types are integer, float, valid, numeric, signed_integer, complex,
real_and_complex, float_and_complex, bool, and unsigned
index
- list indexing incase a test needs to be skipped for a particular dtype(s)
+ list indexing in case a test needs to be skipped for a particular dtype(s)
mixed_fn_compos
boolean if True, the function will return the dtypes of the compositional
implementation for mixed partial functions and if False, it will return
@@ -351,9 +351,9 @@ def array_dtypes(
else:
pairs = ivy.promotion_table.keys()
# added to avoid complex dtypes from being sampled if they are not available.
- pairs = [pair for pair in pairs if all([d in available_dtypes for d in pair])]
+ [pair for pair in pairs if all(d in available_dtypes for d in pair)]
available_dtypes = [
- pair for pair in pairs if not any([d in pair for d in unwanted_types])
+ pair for pair in pairs if not any(d in pair for d in unwanted_types)
]
dtypes = list(draw(st.sampled_from(available_dtypes)))
if num_arrays > 2:
diff --git a/ivy_tests/test_ivy/helpers/hypothesis_helpers/general_helpers.py b/ivy_tests/test_ivy/helpers/hypothesis_helpers/general_helpers.py
index 9bfc7505092b8..4b91dad3f58cf 100644
--- a/ivy_tests/test_ivy/helpers/hypothesis_helpers/general_helpers.py
+++ b/ivy_tests/test_ivy/helpers/hypothesis_helpers/general_helpers.py
@@ -430,7 +430,7 @@ def get_axis(
axis = draw(
st.one_of(*valid_strategies).filter(
lambda x: (
- all([i != axes + j for i in x for j in x])
+ all(i != axes + j for i in x for j in x)
if (isinstance(x, list) and unique and allow_neg)
else True
)
diff --git a/ivy_tests/test_ivy/helpers/multiprocessing.py b/ivy_tests/test_ivy/helpers/multiprocessing.py
index 18d589f6102c4..39234c644de27 100644
--- a/ivy_tests/test_ivy/helpers/multiprocessing.py
+++ b/ivy_tests/test_ivy/helpers/multiprocessing.py
@@ -23,6 +23,7 @@
test_method_ground_truth_computation,
test_gradient_backend_computation,
test_gradient_ground_truth_computation,
+ _transpile_if_required_backend,
)
framework_path = "/opt/fw/"
@@ -344,6 +345,9 @@ def backend_proc(input_queue, output_queue):
output_queue.put(
((None), ret_np_from_gt_flat, ret_from_gt_device, fw_list2)
)
+ if data[0] == "transpile_if_required_backend":
+ _, backend, fn_name, args_np, kwargs_np = data
+ _transpile_if_required_backend(backend, fn_name, args_np, kwargs_np)
if not data:
break
diff --git a/ivy_tests/test_ivy/helpers/test_parameter_flags.py b/ivy_tests/test_ivy/helpers/test_parameter_flags.py
index a0a398747dfa5..e123f1764db31 100644
--- a/ivy_tests/test_ivy/helpers/test_parameter_flags.py
+++ b/ivy_tests/test_ivy/helpers/test_parameter_flags.py
@@ -42,6 +42,8 @@ def _as_varaible_strategy(draw):
BuiltInplaceStrategy = DynamicFlag(st.just(False))
BuiltGradientStrategy = DynamicFlag(_gradient_strategy())
BuiltWithOutStrategy = DynamicFlag(st.booleans())
+BuiltWithCopyStrategy = DynamicFlag(st.just(False))
+BuiltCompileStrategy = DynamicFlag(st.just(False))
BuiltTraceStrategy = DynamicFlag(st.just(False))
BuiltFrontendArrayStrategy = DynamicFlag(st.booleans())
BuiltTranspileStrategy = DynamicFlag(st.just(False))
@@ -55,6 +57,7 @@ def _as_varaible_strategy(draw):
"instance_method": "BuiltInstanceStrategy",
"test_gradients": "BuiltGradientStrategy",
"with_out": "BuiltWithOutStrategy",
+ "with_copy": "BuiltWithCopyStrategy",
"inplace": "BuiltInplace",
"test_trace": "BuiltTraceStrategy",
"transpile": "BuiltTranspileStrategy",
@@ -86,23 +89,27 @@ def __init__(
ground_truth_backend,
num_positional_args,
with_out,
+ with_copy,
instance_method,
as_variable,
native_arrays,
container,
test_gradients,
test_trace,
+ transpile,
precision_mode,
):
self.ground_truth_backend = ground_truth_backend
self.num_positional_args = num_positional_args
self.with_out = with_out
+ self.with_copy = with_copy
self.instance_method = instance_method
self.native_arrays = native_arrays
self.container = container
self.as_variable = as_variable
self.test_gradients = test_gradients
self.test_trace = test_trace
+ self.transpile = transpile
self.precision_mode = precision_mode
def apply_flags(self, args_to_iterate, input_dtypes, offset, *, backend, on_device):
@@ -124,12 +131,14 @@ def __str__(self):
f"ground_truth_backend={self.ground_truth_backend}"
f"num_positional_args={self.num_positional_args}. "
f"with_out={self.with_out}. "
+ f"with_copy={self.with_copy}. "
f"instance_method={self.instance_method}. "
f"native_arrays={self.native_arrays}. "
f"container={self.container}. "
f"as_variable={self.as_variable}. "
f"test_gradients={self.test_gradients}. "
f"test_trace={self.test_trace}. "
+ f"transpile={self.transpile}. "
f"precision_mode={self.precision_mode}. "
)
@@ -145,8 +154,10 @@ def function_flags(
num_positional_args,
instance_method,
with_out,
+ with_copy,
test_gradients,
test_trace,
+ transpile,
as_variable,
native_arrays,
container_flags,
@@ -158,9 +169,11 @@ def function_flags(
ground_truth_backend=ground_truth_backend,
num_positional_args=num_positional_args,
with_out=with_out,
+ with_copy=with_copy,
instance_method=instance_method,
test_gradients=test_gradients,
test_trace=test_trace,
+ transpile=transpile,
as_variable=as_variable,
native_arrays=native_arrays,
container=container_flags,
@@ -174,6 +187,7 @@ def __init__(
self,
num_positional_args,
with_out,
+ with_copy,
inplace,
as_variable,
native_arrays,
@@ -184,6 +198,7 @@ def __init__(
):
self.num_positional_args = num_positional_args
self.with_out = with_out
+ self.with_copy = with_copy
self.inplace = inplace
self.native_arrays = native_arrays
self.as_variable = as_variable
@@ -208,6 +223,7 @@ def __str__(self):
return (
f"num_positional_args={self.num_positional_args}. "
f"with_out={self.with_out}. "
+ f"with_copy={self.with_copy}. "
f"inplace={self.inplace}. "
f"native_arrays={self.native_arrays}. "
f"as_variable={self.as_variable}. "
@@ -227,6 +243,7 @@ def frontend_function_flags(
*,
num_positional_args,
with_out,
+ with_copy,
inplace,
as_variable,
native_arrays,
@@ -240,6 +257,7 @@ def frontend_function_flags(
FrontendFunctionTestFlags,
num_positional_args=num_positional_args,
with_out=with_out,
+ with_copy=with_copy,
inplace=inplace,
as_variable=as_variable,
native_arrays=native_arrays,
diff --git a/ivy_tests/test_ivy/helpers/testing_helpers.py b/ivy_tests/test_ivy/helpers/testing_helpers.py
index 0b1f1b12c96a6..d14b66c59b421 100644
--- a/ivy_tests/test_ivy/helpers/testing_helpers.py
+++ b/ivy_tests/test_ivy/helpers/testing_helpers.py
@@ -24,6 +24,7 @@
BuiltGradientStrategy,
BuiltContainerStrategy,
BuiltWithOutStrategy,
+ BuiltWithCopyStrategy,
BuiltInplaceStrategy,
BuiltTraceStrategy,
BuiltFrontendArrayStrategy,
@@ -224,7 +225,7 @@ def _get_method_supported_devices_dtypes(
Returns
-------
- Returns a dictonary containing supported device types and its supported data types
+ Returns a dictionary containing supported device types and its supported data types
for the method
"""
supported_device_dtypes = {}
@@ -290,7 +291,7 @@ def _get_supported_devices_dtypes(fn_name: str, fn_module: str):
Returns
-------
- Returns a dictonary containing supported device types and its supported data types
+ Returns a dictionary containing supported device types and its supported data types
for the function
"""
supported_device_dtypes = {}
@@ -335,8 +336,10 @@ def handle_test(
number_positional_args=None,
test_instance_method=BuiltInstanceStrategy,
test_with_out=BuiltWithOutStrategy,
+ test_with_copy=BuiltWithCopyStrategy,
test_gradients=BuiltGradientStrategy,
test_trace=BuiltTraceStrategy,
+ transpile=BuiltTranspileStrategy,
precision_mode=BuiltPrecisionModeStrategy,
as_variable_flags=BuiltAsVariableStrategy,
native_array_flags=BuiltNativeArrayStrategy,
@@ -367,6 +370,10 @@ def handle_test(
A search strategy that generates a boolean to test the function with an `out`
parameter
+ test_with_copy
+ A search strategy that generates a boolean to test the function with an `copy`
+ parameter
+
test_gradients
A search strategy that generates a boolean to test the function with arrays as
gradients
@@ -407,8 +414,10 @@ def handle_test(
num_positional_args=number_positional_args,
instance_method=_get_runtime_flag_value(test_instance_method),
with_out=_get_runtime_flag_value(test_with_out),
+ with_copy=_get_runtime_flag_value(test_with_copy),
test_gradients=_get_runtime_flag_value(test_gradients),
test_trace=_get_runtime_flag_value(test_trace),
+ transpile=_get_runtime_flag_value(transpile),
as_variable=_get_runtime_flag_value(as_variable_flags),
native_arrays=_get_runtime_flag_value(native_array_flags),
container_flags=_get_runtime_flag_value(container_flags),
@@ -470,6 +479,7 @@ def handle_frontend_test(
aliases: List[str] = None,
number_positional_args=None,
test_with_out=BuiltWithOutStrategy,
+ test_with_copy=BuiltWithCopyStrategy,
test_inplace=BuiltInplaceStrategy,
as_variable_flags=BuiltAsVariableStrategy,
native_array_flags=BuiltNativeArrayStrategy,
@@ -503,6 +513,10 @@ def handle_frontend_test(
A search strategy that generates a boolean to test the function with an `out`
parameter
+ test_with_copy
+ A search strategy that generates a boolean to test the function with an `copy`
+ parameter
+
precision_mode
A search strategy that generates a boolean to switch between two different
precision modes supported by numpy and (torch, jax) and test the function
@@ -537,6 +551,7 @@ def handle_frontend_test(
test_flags = pf.frontend_function_flags(
num_positional_args=number_positional_args,
with_out=_get_runtime_flag_value(test_with_out),
+ with_copy=_get_runtime_flag_value(test_with_copy),
inplace=_get_runtime_flag_value(test_inplace),
as_variable=_get_runtime_flag_value(as_variable_flags),
native_arrays=_get_runtime_flag_value(native_array_flags),
@@ -762,7 +777,7 @@ def handle_frontend_method(
Name of the method
init_num_positional_args
- A search startegy that generates a number of positional arguments
+ A search strategy that generates a number of positional arguments
to be passed during instantiation of the class
init_native_arrays
@@ -782,7 +797,7 @@ def handle_frontend_method(
precision modes supported by numpy and (torch, jax) and test the function
method_num_positional_args
- A search startegy that generates a number of positional arguments
+ A search strategy that generates a number of positional arguments
to be passed during call of the class method
method_native_arrays
@@ -901,27 +916,32 @@ def seed(draw):
return draw(st.integers(min_value=0, max_value=2**8 - 1))
-def _create_transpile_report(data: dict, backend: str, file_name: str):
+def _create_transpile_report(
+ data: dict, backend: str, file_name: str, is_backend: bool = False
+):
+ backend_specific_data = ["nodes", "time", "args", "kwargs"]
+ # json report exists already
if os.path.isfile(file_name):
- with open(file_name) as outfile:
+ with open(file_name, "r") as outfile:
# Load the file's existing data
file_data = json.load(outfile)
- if file_data["backend_nodes"].get(backend, 0) > data["backend_nodes"]:
+ if file_data["nodes"].get(backend, 0) > data["nodes"]:
return
- file_data["backend_nodes"][backend] = data["backend_nodes"]
- file_data["frontend_time"][backend] = data["frontend_time"]
- file_data["args"][backend] = data["args"]
- file_data["kwargs"][backend] = data["kwargs"]
- file_data["ivy_nodes"] = data["ivy_nodes"]
- file_data["frontend_fw_time"] = data["frontend_fw_time"]
+
+ # that are backend specific
+ for key in backend_specific_data:
+ file_data[key][backend] = data[key]
+ if not is_backend:
+ # not backend specific
+ for key in ["ivy_nodes", "fw_time"]:
+ file_data[key] = data[key]
json_object = json.dumps(file_data, indent=6)
with open(file_name, "w") as outfile:
outfile.write(json_object)
return
- data["backend_nodes"] = {backend: data["backend_nodes"]}
- data["frontend_time"] = {backend: data["frontend_time"]}
- data["args"] = {backend: data["args"]}
- data["kwargs"] = {backend: data["kwargs"]}
+ # create new json report
+ for key in backend_specific_data:
+ data[key] = {backend: data[key]}
json_object = json.dumps(data, indent=6)
with open(file_name, "w") as outfile:
outfile.write(json_object)
diff --git a/ivy_tests/test_ivy/test_frontends/config/torchvision.py b/ivy_tests/test_ivy/test_frontends/config/torchvision.py
new file mode 100644
index 0000000000000..132ce1c13552b
--- /dev/null
+++ b/ivy_tests/test_ivy/test_frontends/config/torchvision.py
@@ -0,0 +1,145 @@
+from .base import FrontendConfig, SupportedDtypes, SupportedDeviecs
+import ivy
+
+
+def get_config():
+ return TorchVisionFrontendConfig()
+
+
+class TorchVisionFrontendConfig(FrontendConfig):
+ backend = ivy.with_backend("torch")
+
+ valid_devices = ["cpu", "gpu"]
+ invalid_devices = ["tpu"]
+
+ valid_dtypes = [
+ "int16",
+ "int32",
+ "int64",
+ "uint8",
+ "float16",
+ "float32",
+ "float64",
+ ]
+
+ invalid_dtypes = [
+ "int8",
+ "uint16",
+ "uint32",
+ "uint64",
+ "bfloat16",
+ "complex64",
+ "complex128",
+ "bool",
+ ]
+
+ valid_numeric_dtypes = [
+ "int16",
+ "int32",
+ "int64",
+ "uint8",
+ "float16",
+ "float32",
+ "float64",
+ ]
+
+ invalid_numeric_dtypes = [
+ "int8",
+ "uint16",
+ "uint32",
+ "uint64",
+ "bfloat16",
+ "complex64",
+ "complex128",
+ "bool",
+ ]
+
+ valid_int_dtypes = [
+ "int16",
+ "int32",
+ "int64",
+ "uint8",
+ ]
+
+ invalid_int_dtypes = [
+ "int8",
+ "uint16",
+ "uint32",
+ "uint64",
+ ]
+
+ valid_uint_dtypes = [
+ "uint8",
+ ]
+
+ invalid_uint_dtypes = [
+ "uint16",
+ "uint32",
+ "uint64",
+ ]
+
+ valid_float_dtypes = [
+ "float16",
+ "float32",
+ "float64",
+ ]
+
+ invalid_float_dtypes = [
+ "bfloat16",
+ ]
+
+ valid_complex_dtypes = []
+
+ invalid_complex_dtypes = [
+ "complex64",
+ "complex128",
+ ]
+
+ @property
+ def supported_devices(self):
+ return SupportedDeviecs(
+ valid_devices=self.valid_devices, invalid_devices=self.invalid_devices
+ )
+
+ @property
+ def supported_dtypes(self):
+ return SupportedDtypes(
+ valid_dtypes=self.valid_dtypes,
+ invalid_dtypes=self.invalid_dtypes,
+ valid_numeric_dtypes=self.valid_numeric_dtypes,
+ invalid_numeric_dtypes=self.invalid_numeric_dtypes,
+ valid_int_dtypes=self.valid_int_dtypes,
+ invalid_int_dtypes=self.invalid_int_dtypes,
+ valid_uint_dtypes=self.valid_uint_dtypes,
+ invalid_uint_dtypes=self.invalid_uint_dtypes,
+ valid_float_dtypes=self.valid_float_dtypes,
+ invalid_float_dtypes=self.invalid_float_dtypes,
+ valid_complex_dtypes=self.valid_complex_dtypes,
+ invalid_complex_dtypes=self.invalid_complex_dtypes,
+ )
+
+ @property
+ def Dtype(self):
+ return self.backend.Dtype
+
+ @property
+ def Device(self):
+ return self.backend.Device
+
+ def native_array(self, x):
+ return self.backend.native_array(x)
+
+ def is_native_array(self, x):
+ return self.backend.is_native_array(x)
+
+ def to_numpy(self, x):
+ return self.backend.to_numpy(x)
+
+ def as_native_dtype(self, dtype: str):
+ return self.backend.as_native_dtype(dtype)
+
+ def as_native_device(self, device: str):
+ return self.backend.as_native_dev(device)
+
+ def isscalar(self, x):
+ return self.backend.isscalar(x)
diff --git a/ivy_tests/test_ivy/test_frontends/test_jax/test_array.py b/ivy_tests/test_ivy/test_frontends/test_jax/test_array.py
index d02d4fb0bce90..8fa019823c2c0 100644
--- a/ivy_tests/test_ivy/test_frontends/test_jax/test_array.py
+++ b/ivy_tests/test_ivy/test_frontends/test_jax/test_array.py
@@ -177,6 +177,30 @@ def _transpose_helper(draw):
return x, xT
+# swapaxes
+@st.composite
+def dtype_x_axis(draw):
+ dtype, x, x_shape = draw(
+ helpers.dtype_and_values(
+ available_dtypes=helpers.get_dtypes("numeric"),
+ min_num_dims=1,
+ max_num_dims=5,
+ ret_shape=True,
+ )
+ )
+ axis1, axis2 = draw(
+ helpers.get_axis(
+ shape=x_shape,
+ sort_values=False,
+ unique=True,
+ min_size=2,
+ max_size=2,
+ force_tuple=True,
+ )
+ )
+ return dtype, x, axis1, axis2
+
+
# --- Main --- #
# ------------ #
@@ -2498,6 +2522,48 @@ def test_jax_array_squeeze(
)
+@handle_frontend_method(
+ class_tree=CLASS_TREE,
+ init_tree="jax.numpy.array",
+ method_name="std",
+ dtype_x_axis=helpers.dtype_values_axis(
+ available_dtypes=helpers.get_dtypes("valid")
+ ),
+ ddof=st.booleans(),
+ keepdims=st.booleans(),
+)
+def test_jax_array_std(
+ dtype_x_axis,
+ backend_fw,
+ frontend,
+ ddof,
+ keepdims,
+ frontend_method_data,
+ init_flags,
+ method_flags,
+ on_device,
+):
+ input_dtype, x, axis = dtype_x_axis
+ helpers.test_frontend_method(
+ init_input_dtypes=input_dtype,
+ backend_to_test=backend_fw,
+ frontend=frontend,
+ init_all_as_kwargs_np={
+ "object": x,
+ },
+ method_input_dtypes=input_dtype,
+ method_all_as_kwargs_np={
+ "axis": axis,
+ "ddof": ddof,
+ "keepdims": keepdims,
+ },
+ frontend_method_data=frontend_method_data,
+ init_flags=init_flags,
+ method_flags=method_flags,
+ on_device=on_device,
+ )
+
+
# var
@handle_frontend_method(
class_tree=CLASS_TREE,
@@ -2724,3 +2790,39 @@ def test_jax_sum(
on_device=on_device,
atol_=1e-04,
)
+
+
+# swapaxes
+@handle_frontend_method(
+ class_tree=CLASS_TREE,
+ init_tree="jax.numpy.array",
+ method_name="swapaxes",
+ dtype_x_axis=dtype_x_axis(),
+)
+def test_jax_swapaxes(
+ dtype_x_axis,
+ frontend,
+ frontend_method_data,
+ backend_fw,
+ init_flags,
+ method_flags,
+ on_device,
+):
+ input_dtypes, x, axis1, axis2 = dtype_x_axis
+ helpers.test_frontend_method(
+ init_input_dtypes=input_dtypes,
+ backend_to_test=backend_fw,
+ method_input_dtypes=input_dtypes,
+ init_all_as_kwargs_np={
+ "object": x[0],
+ },
+ method_all_as_kwargs_np={
+ "axis1": axis1,
+ "axis2": axis2,
+ },
+ frontend=frontend,
+ frontend_method_data=frontend_method_data,
+ init_flags=init_flags,
+ method_flags=method_flags,
+ on_device=on_device,
+ )
diff --git a/ivy_tests/test_ivy/test_frontends/test_jax/test_lax/test_linalg.py b/ivy_tests/test_ivy/test_frontends/test_jax/test_lax/test_linalg.py
index 73194a41bd519..bc5ec8da88181 100644
--- a/ivy_tests/test_ivy/test_frontends/test_jax/test_lax/test_linalg.py
+++ b/ivy_tests/test_ivy/test_frontends/test_jax/test_lax/test_linalg.py
@@ -119,6 +119,45 @@ def test_jax_eigh(
)
+# qr
+@handle_frontend_test(
+ fn_tree="jax.lax.linalg.qr",
+ dtype_and_x=helpers.dtype_and_values(
+ available_dtypes=helpers.get_dtypes("float", index=1),
+ min_num_dims=3,
+ max_num_dims=5,
+ min_dim_size=2,
+ max_dim_size=5,
+ min_value=2,
+ max_value=5,
+ ),
+ mode=st.sampled_from((True, False)),
+ test_with_out=st.just(False),
+)
+def test_jax_qr(
+ *,
+ dtype_and_x,
+ mode,
+ on_device,
+ fn_tree,
+ frontend,
+ test_flags,
+ backend_fw,
+):
+ dtype, x = dtype_and_x
+ ret, frontend_ret = helpers.test_frontend_function(
+ input_dtypes=dtype,
+ test_values=False,
+ backend_to_test=backend_fw,
+ frontend=frontend,
+ test_flags=test_flags,
+ fn_tree=fn_tree,
+ on_device=on_device,
+ x=np.asarray(x[0], dtype[0]),
+ full_matrices=mode,
+ )
+
+
# svd
@handle_frontend_test(
fn_tree="jax.lax.linalg.svd",
diff --git a/ivy_tests/test_ivy/test_frontends/test_jax/test_lax/test_operators.py b/ivy_tests/test_ivy/test_frontends/test_jax/test_lax/test_operators.py
index 6cbed7f1c3ebc..183a13d742a6c 100644
--- a/ivy_tests/test_ivy/test_frontends/test_jax/test_lax/test_operators.py
+++ b/ivy_tests/test_ivy/test_frontends/test_jax/test_lax/test_operators.py
@@ -48,7 +48,7 @@ def _arrays_idx_n_dtypes(draw):
size=num_arrays,
)
)
- xs = list()
+ xs = []
input_dtypes = draw(
helpers.array_dtypes(
available_dtypes=draw(helpers.get_dtypes("numeric")),
@@ -1114,6 +1114,38 @@ def test_jax_clamp(
)
+# complex
+@handle_frontend_test(
+ fn_tree="jax.lax.complex",
+ dtype_and_x=helpers.dtype_and_values(
+ available_dtypes=helpers.get_dtypes("numeric"),
+ num_arrays=2,
+ shared_dtype=True,
+ ),
+ test_with_out=st.just(False),
+)
+def test_jax_complex(
+ *,
+ dtype_and_x,
+ on_device,
+ fn_tree,
+ frontend,
+ test_flags,
+ backend_fw,
+):
+ input_dtype, x = dtype_and_x
+ helpers.test_frontend_function(
+ input_dtypes=input_dtype,
+ backend_to_test=backend_fw,
+ frontend=frontend,
+ test_flags=test_flags,
+ fn_tree=fn_tree,
+ on_device=on_device,
+ x=x[0],
+ y=x[1],
+ )
+
+
# concat
@handle_frontend_test(
fn_tree="jax.lax.concatenate",
@@ -1218,9 +1250,7 @@ def test_jax_conv_general_dilated(
):
dtype, x, filters, dilations, dim_num, stride, pad, fc, pref = x_f_d_other
_assume_tf_dilation_gt_1(ivy.current_backend_str(), on_device, dilations[0])
- assume(
- not (isinstance(pad, str) and not len(dilations[1]) == dilations[1].count(1))
- )
+ assume(not isinstance(pad, str) or len(dilations[1]) == dilations[1].count(1))
helpers.test_frontend_function(
input_dtypes=dtype,
backend_to_test=backend_fw,
@@ -1716,7 +1746,7 @@ def test_jax_expand_dims(
helpers.test_frontend_function(
input_dtypes=x_dtype,
frontend=frontend,
- bakcend_to_test=backend_fw,
+ backend_to_test=backend_fw,
test_flags=test_flags,
fn_tree=fn_tree,
on_device=on_device,
@@ -1889,6 +1919,40 @@ def test_jax_gt(
)
+# igamma
+@handle_frontend_test(
+ fn_tree="jax.lax.igamma",
+ dtypes_and_xs=helpers.dtype_and_values(
+ available_dtypes=helpers.get_dtypes("numeric"),
+ num_arrays=2,
+ shared_dtype=True,
+ ),
+ test_with_out=st.just(False),
+)
+def test_jax_igamma(
+ *,
+ dtypes_and_xs,
+ on_device,
+ fn_tree,
+ frontend,
+ test_flags,
+ backend_fw,
+):
+ input_dtypes, (x, y) = dtypes_and_xs
+
+ helpers.test_frontend_function(
+ input_dtypes=input_dtypes,
+ backend_to_test=backend_fw,
+ frontend=frontend,
+ test_flags=test_flags,
+ fn_tree=fn_tree,
+ on_device=on_device,
+ test_values=True,
+ x=x,
+ y=y,
+ )
+
+
# imag
@handle_frontend_test(
fn_tree="jax.lax.imag",
@@ -2643,7 +2707,7 @@ def test_jax_shift_left(
):
input_dtype, x = dtype_and_x
# negative shifts will throw an exception
- # shifts >= dtype witdth produce backend-defined behavior
+ # shifts >= dtype width produce backend-defined behavior
x[1] = np.asarray(
np.clip(x[1], 0, np.iinfo(input_dtype[1]).bits - 1), dtype=input_dtype[1]
)
@@ -2681,7 +2745,7 @@ def test_jax_shift_right_logical(
):
input_dtype, x = dtype_and_x
# negative shifts will throw an exception
- # shifts >= dtype witdth produce backend-defined behavior
+ # shifts >= dtype width produce backend-defined behavior
x[1] = np.asarray(
np.clip(x[1], 0, np.iinfo(input_dtype[1]).bits - 1), dtype=input_dtype[1]
)
diff --git a/ivy_tests/test_ivy/test_frontends/test_jax/test_nn/test_non_linear_activations.py b/ivy_tests/test_ivy/test_frontends/test_jax/test_nn/test_non_linear_activations.py
index be20daaabf52e..7a88be34962c1 100644
--- a/ivy_tests/test_ivy/test_frontends/test_jax/test_nn/test_non_linear_activations.py
+++ b/ivy_tests/test_ivy/test_frontends/test_jax/test_nn/test_non_linear_activations.py
@@ -34,7 +34,7 @@ def _dtype_indices_classes_axis(draw):
@handle_frontend_test(
fn_tree="jax.nn.celu",
dtype_and_x=helpers.dtype_and_values(
- available_dtypes=helpers.get_dtypes("float_and_integer"),
+ available_dtypes=helpers.get_dtypes("float_and_complex"),
min_value=-5,
max_value=5,
safety_factor_scale="linear",
diff --git a/ivy_tests/test_ivy/test_frontends/test_jax/test_numpy/test_creation.py b/ivy_tests/test_ivy/test_frontends/test_jax/test_numpy/test_creation.py
index c4facb3cbf363..ec3f1330f27a5 100644
--- a/ivy_tests/test_ivy/test_frontends/test_jax/test_numpy/test_creation.py
+++ b/ivy_tests/test_ivy/test_frontends/test_jax/test_numpy/test_creation.py
@@ -21,10 +21,26 @@ def _get_dtype_and_range(draw):
dim = draw(helpers.ints(min_value=2, max_value=5))
dtype = draw(helpers.get_dtypes("float", index=1, full=False))
start = draw(
- helpers.array_values(dtype=dtype[0], shape=(dim,), min_value=-50, max_value=0)
+ helpers.array_values(
+ dtype=dtype[0],
+ shape=(dim,),
+ min_value=-50,
+ max_value=0,
+ large_abs_safety_factor=4,
+ small_abs_safety_factor=4,
+ safety_factor_scale="log",
+ )
)
stop = draw(
- helpers.array_values(dtype=dtype[0], shape=(dim,), min_value=1, max_value=50)
+ helpers.array_values(
+ dtype=dtype[0],
+ shape=(dim,),
+ min_value=1,
+ max_value=50,
+ large_abs_safety_factor=4,
+ small_abs_safety_factor=4,
+ safety_factor_scale="log",
+ )
)
return dtype * 2, start, stop
@@ -83,6 +99,7 @@ def test_jax_arange(
copy=st.booleans(),
ndmin=helpers.ints(min_value=0, max_value=9),
test_with_out=st.just(True),
+ test_with_copy=st.just(True),
)
def test_jax_array(
*,
@@ -260,6 +277,7 @@ def test_jax_compress(
max_dim_size=5,
),
test_with_out=st.just(False),
+ test_with_copy=st.just(True),
)
def test_jax_copy(
dtype_and_a,
@@ -443,6 +461,63 @@ def test_jax_eye(
)
+# from_dlpack
+@handle_frontend_test(
+ fn_tree="jax.numpy.from_dlpack",
+ dtype_and_x=helpers.dtype_and_values(
+ available_dtypes=helpers.get_dtypes("numeric")
+ ),
+)
+def test_jax_from_dlpack(
+ *,
+ dtype_and_x,
+ on_device,
+ fn_tree,
+ frontend,
+ test_flags,
+ backend_fw,
+):
+ input_dtype, x = dtype_and_x
+ helpers.test_frontend_function(
+ x=x[0],
+ backend_to_test=backend_fw,
+ input_dtypes=input_dtype,
+ frontend=frontend,
+ test_flags=test_flags,
+ fn_tree=fn_tree,
+ on_device=on_device,
+ )
+
+
+@handle_frontend_test(
+ fn_tree="jax.numpy.frombuffer",
+ dtype_buffer_count_offset=_get_dtype_buffer_count_offset(),
+ test_with_out=st.just(False),
+)
+def test_jax_frombuffer(
+ *,
+ dtype_buffer_count_offset,
+ on_device,
+ fn_tree,
+ frontend,
+ backend_fw,
+ test_flags,
+):
+ input_dtype, buffer, count, offset = dtype_buffer_count_offset
+ helpers.test_frontend_function(
+ input_dtypes=input_dtype,
+ frontend=frontend,
+ test_flags=test_flags,
+ backend_to_test=backend_fw,
+ fn_tree=fn_tree,
+ on_device=on_device,
+ buffer=buffer,
+ dtype=input_dtype[0],
+ count=count,
+ offset=offset,
+ )
+
+
# full
@handle_frontend_test(
fn_tree="jax.numpy.full",
@@ -600,6 +675,42 @@ def test_jax_identity(
)
+@handle_frontend_test(
+ fn_tree="jax.numpy.in1d",
+ dtype_and_a=helpers.dtype_and_values(min_num_dims=1, max_num_dims=1),
+ dtype_and_b=helpers.dtype_and_values(min_num_dims=1, max_num_dims=1),
+ assume_unique=st.booleans(),
+ invert=st.booleans(),
+)
+def test_jax_in1d(
+ *,
+ dtype_and_a,
+ dtype_and_b,
+ assume_unique,
+ invert,
+ on_device,
+ fn_tree,
+ frontend,
+ backend_fw,
+ test_flags,
+):
+ input_dtype_a, a = dtype_and_a
+ input_dtype_b, b = dtype_and_b
+
+ helpers.test_frontend_function(
+ input_dtypes=input_dtype_a + input_dtype_b,
+ frontend=frontend,
+ test_flags=test_flags,
+ backend_to_test=backend_fw,
+ fn_tree=fn_tree,
+ on_device=on_device,
+ ar1=a[0],
+ ar2=b[0],
+ assume_unique=assume_unique,
+ invert=invert,
+ )
+
+
@handle_frontend_test(
fn_tree="jax.numpy.iterable",
dtype_and_x=helpers.dtype_and_values(
@@ -716,6 +827,7 @@ def test_jax_logspace(
sparse=st.booleans(),
indexing=st.sampled_from(["xy", "ij"]),
test_with_out=st.just(False),
+ test_with_copy=st.just(True),
)
def test_jax_meshgrid(
dtype_and_arrays,
@@ -772,96 +884,77 @@ def test_jax_ndim(
)
-# from_dlpack
+# ones
@handle_frontend_test(
- fn_tree="jax.numpy.from_dlpack",
- dtype_and_x=helpers.dtype_and_values(
- available_dtypes=helpers.get_dtypes("numeric")
+ fn_tree="jax.numpy.ones",
+ shape=helpers.get_shape(
+ allow_none=False,
+ min_num_dims=1,
+ max_num_dims=5,
+ min_dim_size=1,
+ max_dim_size=10,
),
+ dtype=helpers.get_dtypes("valid", full=False),
+ test_with_out=st.just(False),
)
-def test_jax_numpy_from_dlpack(
- *,
- dtype_and_x,
- on_device,
- fn_tree,
- frontend,
+def test_jax_ones(
+ shape,
+ dtype,
test_flags,
+ frontend,
backend_fw,
+ fn_tree,
+ on_device,
):
- input_dtype, x = dtype_and_x
helpers.test_frontend_function(
- x=x[0],
+ input_dtypes=dtype,
backend_to_test=backend_fw,
- input_dtypes=input_dtype,
frontend=frontend,
test_flags=test_flags,
fn_tree=fn_tree,
on_device=on_device,
+ shape=shape,
+ dtype=dtype[0],
)
+# ones_like
@handle_frontend_test(
- fn_tree="jax.numpy.frombuffer",
- dtype_buffer_count_offset=_get_dtype_buffer_count_offset(),
+ fn_tree="jax.numpy.ones_like",
+ dtype_and_x=helpers.dtype_and_values(
+ available_dtypes=helpers.get_dtypes("valid"),
+ ),
+ shape=helpers.get_shape(
+ allow_none=True,
+ min_num_dims=1,
+ max_num_dims=5,
+ min_dim_size=1,
+ max_dim_size=10,
+ ),
+ dtype=helpers.get_dtypes("valid", full=False),
test_with_out=st.just(False),
)
-def test_jax_numpy_frombuffer(
- *,
- dtype_buffer_count_offset,
- on_device,
- fn_tree,
+def test_jax_ones_like(
+ dtype_and_x,
+ shape,
+ dtype,
+ test_flags,
frontend,
backend_fw,
- test_flags,
+ fn_tree,
+ on_device,
):
- input_dtype, buffer, count, offset = dtype_buffer_count_offset
+ input_dtype, x = dtype_and_x
helpers.test_frontend_function(
input_dtypes=input_dtype,
- frontend=frontend,
- test_flags=test_flags,
backend_to_test=backend_fw,
- fn_tree=fn_tree,
- on_device=on_device,
- buffer=buffer,
- dtype=input_dtype[0],
- count=count,
- offset=offset,
- )
-
-
-@handle_frontend_test(
- fn_tree="jax.numpy.in1d",
- dtype_and_a=helpers.dtype_and_values(min_num_dims=1, max_num_dims=1),
- dtype_and_b=helpers.dtype_and_values(min_num_dims=1, max_num_dims=1),
- assume_unique=st.booleans(),
- invert=st.booleans(),
-)
-def test_jax_numpy_in1d(
- *,
- dtype_and_a,
- dtype_and_b,
- assume_unique,
- invert,
- on_device,
- fn_tree,
- frontend,
- backend_fw,
- test_flags,
-):
- input_dtype_a, a = dtype_and_a
- input_dtype_b, b = dtype_and_b
-
- helpers.test_frontend_function(
- input_dtypes=input_dtype_a + input_dtype_b,
frontend=frontend,
test_flags=test_flags,
- backend_to_test=backend_fw,
fn_tree=fn_tree,
on_device=on_device,
- ar1=a[0],
- ar2=b[0],
- assume_unique=assume_unique,
- invert=invert,
+ a=x[0],
+ dtype=dtype[0],
+ shape=shape,
)
@@ -881,7 +974,7 @@ def test_jax_numpy_in1d(
size=st.integers(min_value=1, max_value=100),
assume_unique=st.booleans(),
)
-def test_jax_numpy_setdiff1d(
+def test_jax_setdiff1d(
*,
dtype_and_a,
dtype_and_b,
@@ -913,80 +1006,6 @@ def test_jax_numpy_setdiff1d(
)
-# ones
-@handle_frontend_test(
- fn_tree="jax.numpy.ones",
- shape=helpers.get_shape(
- allow_none=False,
- min_num_dims=1,
- max_num_dims=5,
- min_dim_size=1,
- max_dim_size=10,
- ),
- dtype=helpers.get_dtypes("valid", full=False),
- test_with_out=st.just(False),
-)
-def test_jax_ones(
- shape,
- dtype,
- test_flags,
- frontend,
- backend_fw,
- fn_tree,
- on_device,
-):
- helpers.test_frontend_function(
- input_dtypes=dtype,
- backend_to_test=backend_fw,
- frontend=frontend,
- test_flags=test_flags,
- fn_tree=fn_tree,
- on_device=on_device,
- shape=shape,
- dtype=dtype[0],
- )
-
-
-# ones_like
-@handle_frontend_test(
- fn_tree="jax.numpy.ones_like",
- dtype_and_x=helpers.dtype_and_values(
- available_dtypes=helpers.get_dtypes("valid"),
- ),
- shape=helpers.get_shape(
- allow_none=True,
- min_num_dims=1,
- max_num_dims=5,
- min_dim_size=1,
- max_dim_size=10,
- ),
- dtype=helpers.get_dtypes("valid", full=False),
- test_with_out=st.just(False),
-)
-def test_jax_ones_like(
- dtype_and_x,
- shape,
- dtype,
- test_flags,
- frontend,
- backend_fw,
- fn_tree,
- on_device,
-):
- input_dtype, x = dtype_and_x
- helpers.test_frontend_function(
- input_dtypes=input_dtype,
- backend_to_test=backend_fw,
- frontend=frontend,
- test_flags=test_flags,
- fn_tree=fn_tree,
- on_device=on_device,
- a=x[0],
- dtype=dtype[0],
- shape=shape,
- )
-
-
# single
@handle_frontend_test(
fn_tree="jax.numpy.single",
diff --git a/ivy_tests/test_ivy/test_frontends/test_jax/test_numpy/test_indexing.py b/ivy_tests/test_ivy/test_frontends/test_jax/test_numpy/test_indexing.py
index 3e7842c3fd9e1..4d76bfcc9a139 100644
--- a/ivy_tests/test_ivy/test_frontends/test_jax/test_numpy/test_indexing.py
+++ b/ivy_tests/test_ivy/test_frontends/test_jax/test_numpy/test_indexing.py
@@ -287,7 +287,7 @@ def test_jax_mask_indices(
)
-@handle_frontend_test(fn_tree="jax.numpy.add", inputs=_helper_c_()) # dummy fn_tree
+@handle_frontend_test(fn_tree="jax.numpy.c_", inputs=_helper_c_()) # dummy fn_tree
def test_jax_numpy_c_(inputs, backend_fw):
ret_gt = c_.__getitem__(tuple(inputs))
with BackendHandler.update_backend(backend_fw):
@@ -326,7 +326,7 @@ def test_jax_numpy_indices(
)
-@handle_frontend_test(fn_tree="jax.numpy.add", inputs=_helper_r_()) # dummy fn_tree
+@handle_frontend_test(fn_tree="jax.numpy.r_", inputs=_helper_r_()) # dummy fn_tree
def test_jax_numpy_r_(inputs, backend_fw):
inputs, *_ = inputs
ret_gt = r_.__getitem__(tuple(inputs))
diff --git a/ivy_tests/test_ivy/test_frontends/test_jax/test_numpy/test_logic.py b/ivy_tests/test_ivy/test_frontends/test_jax/test_numpy/test_logic.py
index d0580c45643bb..f9a2a8c0a8f09 100644
--- a/ivy_tests/test_ivy/test_frontends/test_jax/test_numpy/test_logic.py
+++ b/ivy_tests/test_ivy/test_frontends/test_jax/test_numpy/test_logic.py
@@ -382,6 +382,36 @@ def test_jax_equal(
)
+# fromfunction
+@handle_frontend_test(
+ fn_tree="jax.numpy.fromfunction",
+ input_dtype=helpers.get_dtypes("valid"),
+ function_and_shape_and_dtype=_func_and_shape_dtype_helper(),
+ test_with_out=st.just(False),
+)
+def test_jax_fromfunction(
+ input_dtype,
+ function_and_shape_and_dtype,
+ backend_fw,
+ frontend,
+ on_device,
+ fn_tree,
+ test_flags,
+):
+ function, shape, dtype = function_and_shape_and_dtype
+ helpers.test_frontend_function(
+ input_dtypes=input_dtype,
+ backend_to_test=backend_fw,
+ frontend=frontend,
+ test_flags=test_flags,
+ fn_tree=fn_tree,
+ on_device=on_device,
+ function=function,
+ shape=shape,
+ dtype=dtype,
+ )
+
+
# greater
@handle_frontend_test(
fn_tree="jax.numpy.greater",
@@ -1078,36 +1108,6 @@ def test_jax_not_equal(
)
-# fromfunction
-@handle_frontend_test(
- fn_tree="jax.numpy.fromfunction",
- input_dtype=helpers.get_dtypes("valid"),
- function_and_shape_and_dtype=_func_and_shape_dtype_helper(),
- test_with_out=st.just(False),
-)
-def test_jax_numpy_fromfunction(
- input_dtype,
- function_and_shape_and_dtype,
- backend_fw,
- frontend,
- on_device,
- fn_tree,
- test_flags,
-):
- function, shape, dtype = function_and_shape_and_dtype
- helpers.test_frontend_function(
- input_dtypes=input_dtype,
- backend_to_test=backend_fw,
- frontend=frontend,
- test_flags=test_flags,
- fn_tree=fn_tree,
- on_device=on_device,
- function=function,
- shape=shape,
- dtype=dtype,
- )
-
-
# packbits
@handle_frontend_test(
fn_tree="jax.numpy.packbits",
@@ -1122,7 +1122,7 @@ def test_jax_numpy_fromfunction(
test_with_out=st.just(False),
bitorder=st.sampled_from(["big", "little"]),
)
-def test_jax_numpy_packbits(
+def test_jax_packbits(
dtype_x_axis,
bitorder,
frontend,
@@ -1146,38 +1146,6 @@ def test_jax_numpy_packbits(
)
-# setxor1d
-@handle_frontend_test(
- fn_tree="jax.numpy.setxor1d",
- dtypes_values=helpers.dtype_and_values(
- available_dtypes=helpers.get_dtypes("valid"), num_arrays=2, shared_dtype=True
- ),
- assume_unique=st.booleans(),
- test_with_out=st.just(False),
-)
-def test_jax_numpy_setxor1d(
- dtypes_values,
- on_device,
- fn_tree,
- frontend,
- test_flags,
- assume_unique,
- backend_fw,
-):
- x_dtypes, x = dtypes_values
- helpers.test_frontend_function(
- input_dtypes=x_dtypes,
- backend_to_test=backend_fw,
- frontend=frontend,
- test_flags=test_flags,
- fn_tree=fn_tree,
- on_device=on_device,
- ar1=x[0],
- ar2=x[1],
- assume_unique=assume_unique,
- )
-
-
@handle_frontend_test(
fn_tree="jax.numpy.right_shift",
dtype_and_x=helpers.dtype_and_values(
@@ -1210,3 +1178,35 @@ def test_jax_right_shift(
x1=xs[0],
x2=xs[1],
)
+
+
+# setxor1d
+@handle_frontend_test(
+ fn_tree="jax.numpy.setxor1d",
+ dtypes_values=helpers.dtype_and_values(
+ available_dtypes=helpers.get_dtypes("valid"), num_arrays=2, shared_dtype=True
+ ),
+ assume_unique=st.booleans(),
+ test_with_out=st.just(False),
+)
+def test_jax_setxor1d(
+ dtypes_values,
+ on_device,
+ fn_tree,
+ frontend,
+ test_flags,
+ assume_unique,
+ backend_fw,
+):
+ x_dtypes, x = dtypes_values
+ helpers.test_frontend_function(
+ input_dtypes=x_dtypes,
+ backend_to_test=backend_fw,
+ frontend=frontend,
+ test_flags=test_flags,
+ fn_tree=fn_tree,
+ on_device=on_device,
+ ar1=x[0],
+ ar2=x[1],
+ assume_unique=assume_unique,
+ )
diff --git a/ivy_tests/test_ivy/test_frontends/test_jax/test_numpy/test_manipulations.py b/ivy_tests/test_ivy/test_frontends/test_jax/test_numpy/test_manipulations.py
index a82f6d6c4a31c..2d9d150748115 100644
--- a/ivy_tests/test_ivy/test_frontends/test_jax/test_numpy/test_manipulations.py
+++ b/ivy_tests/test_ivy/test_frontends/test_jax/test_numpy/test_manipulations.py
@@ -42,7 +42,7 @@ def _arrays_idx_n_dtypes(draw):
size=num_arrays,
)
)
- xs = list()
+ xs = []
input_dtypes = draw(
helpers.array_dtypes(available_dtypes=draw(helpers.get_dtypes("valid")))
)
@@ -263,7 +263,7 @@ def _pad_helper(draw):
ndim = len(shape)
pad_width = draw(_st_tuples_or_int(ndim, min_val=0))
kwargs = {}
- if mode == "reflect" or mode == "symmetric":
+ if mode in ["reflect", "symmetric"]:
kwargs["reflect_type"] = draw(st.sampled_from(["even", "odd"]))
if mode in ["maximum", "mean", "median", "minimum"]:
kwargs["stat_length"] = draw(_st_tuples_or_int(ndim, min_val=2))
@@ -498,6 +498,30 @@ def test_jax_atleast_3d(
)
+# bartlett
+@handle_frontend_test(
+ fn_tree="jax.numpy.bartlett",
+ m=helpers.ints(min_value=0, max_value=20),
+)
+def test_jax_bartlett(
+ m,
+ frontend,
+ backend_fw,
+ test_flags,
+ fn_tree,
+ on_device,
+):
+ helpers.test_frontend_function(
+ input_dtypes=["int64"],
+ backend_to_test=backend_fw,
+ frontend=frontend,
+ test_flags=test_flags,
+ fn_tree=fn_tree,
+ on_device=on_device,
+ M=m,
+ )
+
+
# blackman
@handle_frontend_test(
fn_tree="jax.numpy.blackman",
@@ -705,6 +729,41 @@ def test_jax_concat(
)
+@handle_frontend_test(
+ fn_tree="jax.numpy.diagflat",
+ dtype_x=helpers.dtype_and_values(
+ available_dtypes=helpers.get_dtypes("float"),
+ shape=helpers.get_shape(
+ min_num_dims=1, max_num_dims=2, min_dim_size=1, max_dim_size=10
+ ),
+ small_abs_safety_factor=2.5,
+ large_abs_safety_factor=2.5,
+ safety_factor_scale="log",
+ ),
+ k=st.integers(min_value=-5, max_value=5),
+)
+def test_jax_diagflat(
+ dtype_x,
+ k,
+ frontend,
+ test_flags,
+ fn_tree,
+ backend_fw,
+ on_device,
+):
+ dtype, x = dtype_x
+ helpers.test_frontend_function(
+ input_dtypes=dtype,
+ backend_to_test=backend_fw,
+ frontend=frontend,
+ test_flags=test_flags,
+ fn_tree=fn_tree,
+ on_device=on_device,
+ v=x[0],
+ k=k,
+ )
+
+
# dsplit
@handle_frontend_test(
fn_tree="jax.numpy.dsplit",
diff --git a/ivy_tests/test_ivy/test_frontends/test_jax/test_numpy/test_mathematical_functions.py b/ivy_tests/test_ivy/test_frontends/test_jax/test_numpy/test_mathematical_functions.py
index 65be2aa2b07c3..7ff6a267a5b4b 100644
--- a/ivy_tests/test_ivy/test_frontends/test_jax/test_numpy/test_mathematical_functions.py
+++ b/ivy_tests/test_ivy/test_frontends/test_jax/test_numpy/test_mathematical_functions.py
@@ -896,8 +896,7 @@ def test_jax_diff(
axis,
):
input_dtype, x = dtype_and_x
- if axis > (x[0].ndim - 1):
- axis = x[0].ndim - 1
+ axis = min(axis, x[0].ndim - 1)
helpers.test_frontend_function(
input_dtypes=input_dtype,
test_flags=test_flags,
@@ -1062,6 +1061,53 @@ def test_jax_ediff1d(
)
+# einsum_path
+# For the optimize parameter boolean values are not added to the samples for testing
+# as it seems that Jax einsum_path function currently fails when True or False is passed
+# as optimize values. Jax einsum_path function calls opt_einsum.contract_path function,
+# and it seems that there is an open bug on their repository for boolean values.
+# Please see link to the bug https://github.com/dgasmith/opt_einsum/issues/219
+@handle_frontend_test(
+ fn_tree="jax.numpy.einsum_path",
+ eq_n_op_n_shp=helpers.einsum_helper(),
+ dtype=helpers.get_dtypes("numeric", full=False),
+ test_with_out=st.just(False),
+ optimize=st.sampled_from(["greedy", "optimal"]),
+)
+def test_jax_einsum_path(
+ *,
+ eq_n_op_n_shp,
+ dtype,
+ on_device,
+ fn_tree,
+ backend_fw,
+ frontend,
+ test_flags,
+ optimize,
+):
+ eq, operands, dtypes = eq_n_op_n_shp
+ kw = {}
+ for i, x_ in enumerate(operands):
+ dtype = dtypes[i][0]
+ kw[f"x{i}"] = np.array(x_).astype(dtype)
+ test_flags.num_positional_args = len(operands) + 1
+ ret, ret_gt = helpers.test_frontend_function(
+ input_dtypes=dtypes,
+ backend_to_test=backend_fw,
+ frontend=frontend,
+ test_flags=test_flags,
+ fn_tree=fn_tree,
+ on_device=on_device,
+ test_values=False,
+ subscripts=eq,
+ **kw,
+ optimize=optimize,
+ )
+ len(ret[0]) == len(ret_gt[0])
+ all(x == y for x, y in zip(ret[0], ret_gt[0]))
+ ret[1] == str(ret_gt[1])
+
+
# exp
@handle_frontend_test(
fn_tree="jax.numpy.exp",
@@ -1461,7 +1507,7 @@ def test_jax_frexp(
min_dim_size=1,
max_dim_size=3,
num_arrays=2,
- ).filter(lambda x: all([dtype != "uint64" for dtype in x[0]])),
+ ).filter(lambda x: all(dtype != "uint64" for dtype in x[0])),
test_with_out=st.just(False),
)
def test_jax_gcd(
@@ -1703,6 +1749,54 @@ def test_jax_inner(
)
+@handle_frontend_test(
+ fn_tree="jax.numpy.interp",
+ dtype_and_x=helpers.dtype_and_values(
+ available_dtypes=helpers.get_dtypes("float"),
+ min_num_dims=1,
+ max_num_dims=1,
+ ),
+ dtype_and_xp_fp=helpers.dtype_and_values(
+ available_dtypes=helpers.get_dtypes("float"),
+ num_arrays=2,
+ min_num_dims=1,
+ max_num_dims=1,
+ ),
+ left=st.one_of(st.floats(min_value=-1e04, max_value=1e04), st.just(np.nan)),
+ right=st.one_of(st.floats(min_value=-1e04, max_value=1e04), st.just(np.nan)),
+ test_with_out=st.just(False),
+)
+def test_jax_interp(
+ *,
+ dtype_and_x,
+ dtype_and_xp_fp,
+ left,
+ right,
+ on_device,
+ fn_tree,
+ frontend,
+ backend_fw,
+ test_flags,
+):
+ input_dtype, x = dtype_and_x
+ input_dtype2, xp_fp = dtype_and_xp_fp
+ xp = xp_fp[0]
+ fp = xp_fp[1]
+ helpers.test_frontend_function(
+ input_dtypes=[input_dtype, input_dtype2],
+ frontend=frontend,
+ backend_to_test=backend_fw,
+ test_flags=test_flags,
+ fn_tree=fn_tree,
+ on_device=on_device,
+ x=x[0],
+ xp=xp,
+ fp=fp,
+ left=left,
+ right=right,
+ )
+
+
# kron
@handle_frontend_test(
fn_tree="jax.numpy.kron",
@@ -2223,6 +2317,7 @@ def test_jax_multiply(
posinf=st.floats(min_value=5e100, max_value=5e100),
neginf=st.floats(min_value=-5e100, max_value=-5e100),
test_with_out=st.just(False),
+ test_with_copy=st.just(True),
)
def test_jax_nan_to_num(
*,
diff --git a/ivy_tests/test_ivy/test_frontends/test_jax/test_numpy/test_searching_sorting.py b/ivy_tests/test_ivy/test_frontends/test_jax/test_numpy/test_searching_sorting.py
index 1e43433c4e00e..13800e80a63d3 100644
--- a/ivy_tests/test_ivy/test_frontends/test_jax/test_numpy/test_searching_sorting.py
+++ b/ivy_tests/test_ivy/test_frontends/test_jax/test_numpy/test_searching_sorting.py
@@ -169,6 +169,42 @@ def test_jax_argwhere(
)
+# count_nonzero
+@handle_frontend_test(
+ fn_tree="jax.numpy.count_nonzero",
+ dtype_input_axis=helpers.dtype_values_axis(
+ available_dtypes=helpers.get_dtypes("valid"),
+ min_num_dims=1,
+ force_int_axis=True,
+ valid_axis=True,
+ allow_neg_axes=True,
+ ),
+ keepdims=st.booleans(),
+ test_with_out=st.just(False),
+)
+def test_jax_count_nonzero(
+ dtype_input_axis,
+ keepdims,
+ frontend,
+ test_flags,
+ fn_tree,
+ backend_fw,
+ on_device,
+):
+ input_dtype, x, axis = dtype_input_axis
+ helpers.test_frontend_function(
+ input_dtypes=input_dtype,
+ backend_to_test=backend_fw,
+ frontend=frontend,
+ test_flags=test_flags,
+ fn_tree=fn_tree,
+ on_device=on_device,
+ a=x[0],
+ axis=axis,
+ keepdims=keepdims,
+ )
+
+
# extract
@handle_frontend_test(
fn_tree="jax.numpy.extract",
diff --git a/ivy_tests/test_ivy/test_frontends/test_jax/test_numpy/test_statistical.py b/ivy_tests/test_ivy/test_frontends/test_jax/test_numpy/test_statistical.py
index c5020e7237688..28d6388d2af2c 100644
--- a/ivy_tests/test_ivy/test_frontends/test_jax/test_numpy/test_statistical.py
+++ b/ivy_tests/test_ivy/test_frontends/test_jax/test_numpy/test_statistical.py
@@ -5,7 +5,6 @@
# local
import ivy
-import ivy_tests.test_ivy.test_frontends.test_numpy.helpers as np_frontend_helpers
import ivy_tests.test_ivy.helpers as helpers
import ivy_tests.test_ivy.test_frontends.test_numpy.helpers as np_helpers
from ivy_tests.test_ivy.helpers import handle_frontend_test
@@ -106,7 +105,7 @@ def _get_castable_dtypes_values(draw, *, allow_nan=False, use_where=False):
helpers.get_castable_dtype(draw(available_dtypes), dtype[0], values[0])
)
if use_where:
- where = draw(np_frontend_helpers.where(shape=shape))
+ where = draw(np_helpers.where(shape=shape))
return [dtype1], [values], axis, dtype2, where
return [dtype1], [values], axis, dtype2
@@ -192,6 +191,55 @@ def _get_dtype_value1_value2_cov(
return [dtype], value1, value2, rowVar, bias, ddof, fweights, aweights
+@st.composite
+def _percentile_helper(draw):
+ large_abs_safety_factor = 2
+ small_abs_safety_factor = 2
+ dtype, values, axis = draw(
+ helpers.dtype_values_axis(
+ available_dtypes=helpers.get_dtypes("float"),
+ large_abs_safety_factor=large_abs_safety_factor,
+ small_abs_safety_factor=small_abs_safety_factor,
+ safety_factor_scale="log",
+ min_num_dims=1,
+ max_num_dims=5,
+ min_dim_size=2,
+ valid_axis=True,
+ allow_neg_axes=False,
+ min_axes_size=1,
+ force_int_axis=True,
+ )
+ )
+ q = draw(
+ st.one_of(
+ helpers.array_values(
+ dtype=helpers.get_dtypes("float"),
+ shape=helpers.get_shape(min_dim_size=1, max_num_dims=1, min_num_dims=1),
+ min_value=0.0,
+ max_value=100.0,
+ exclude_max=False,
+ exclude_min=False,
+ ),
+ st.floats(min_value=0.0, max_value=100.0),
+ )
+ )
+
+ interpolation_names = [
+ "linear",
+ "lower",
+ "higher",
+ "midpoint",
+ "nearest",
+ ]
+ interpolation = draw(
+ helpers.list_of_size(
+ x=st.sampled_from(interpolation_names),
+ size=1,
+ )
+ )
+ return dtype, values, axis, interpolation, q
+
+
# --- Main --- #
# ------------ #
@@ -762,7 +810,7 @@ def test_jax_nancumsum(
input_dtypes, x, axis, dtype = dtype_and_x_axis_dtype
if ivy.current_backend_str() == "torch":
assume(not test_flags.as_variable[0])
- np_frontend_helpers.test_frontend_function(
+ np_helpers.test_frontend_function(
input_dtypes=input_dtypes,
backend_to_test=backend_fw,
frontend=frontend,
@@ -965,7 +1013,7 @@ def test_jax_nanmin(
fn_tree="jax.numpy.nanstd",
dtype_and_a=_statistical_dtype_values(function="nanstd"),
dtype=helpers.get_dtypes("float", full=False, none=True),
- where=np_frontend_helpers.where(),
+ where=np_helpers.where(),
keep_dims=st.booleans(),
)
def test_jax_nanstd(
@@ -982,13 +1030,13 @@ def test_jax_nanstd(
input_dtypes, a, axis, correction = dtype_and_a
if isinstance(axis, tuple):
axis = axis[0]
- where, input_dtypes, test_flags = np_frontend_helpers.handle_where_and_array_bools(
+ where, input_dtypes, test_flags = np_helpers.handle_where_and_array_bools(
where=where,
input_dtype=input_dtypes,
test_flags=test_flags,
)
assume(np.dtype(dtype[0]) >= np.dtype(input_dtypes[0]))
- np_frontend_helpers.test_frontend_function(
+ np_helpers.test_frontend_function(
input_dtypes=input_dtypes,
backend_to_test=backend_fw,
frontend=frontend,
@@ -1075,7 +1123,7 @@ def test_jax_ptp(
keep_dims,
):
input_dtypes, x, axis, dtype = dtype_and_x_axis_dtype
- np_frontend_helpers.test_frontend_function(
+ np_helpers.test_frontend_function(
input_dtypes=input_dtypes,
backend_to_test=backend_fw,
frontend=frontend,
@@ -1272,3 +1320,28 @@ def test_jax_var(
atol=1e-3,
rtol=1e-3,
)
+
+
+@handle_frontend_test(
+ fn_tree="jax.numpy.nanpercentile",
+ dtype_and_x=_percentile_helper(),
+ keep_dims=st.booleans(),
+ test_gradients=st.just(False),
+ test_with_out=st.just(False),
+)
+def test_nanpercentile(
+ *, dtype_and_x, keep_dims, test_flags, backend_fw, fn_name, on_device
+):
+ input_dtype, x, axis, interpolation, q = dtype_and_x
+ helpers.test_function(
+ input_dtypes=input_dtype,
+ test_flags=test_flags,
+ backend_to_test=backend_fw,
+ fn_name=fn_name,
+ on_device=on_device,
+ a=x[0],
+ q=q,
+ axis=axis,
+ interpolation=interpolation[0],
+ keepdims=keep_dims,
+ )
diff --git a/ivy_tests/test_ivy/test_frontends/test_jax/test_random.py b/ivy_tests/test_ivy/test_frontends/test_jax/test_random.py
index 52e1d84278eca..ab55689f7c711 100644
--- a/ivy_tests/test_ivy/test_frontends/test_jax/test_random.py
+++ b/ivy_tests/test_ivy/test_frontends/test_jax/test_random.py
@@ -1027,7 +1027,7 @@ def test_jax_multivariate_normal(
spd = np.matmul(cov.T, cov) + np.identity(cov.shape[0])
def call():
- helpers.test_frontend_function(
+ return helpers.test_frontend_function(
input_dtypes=input_dtype + [shared_dtype],
frontend=frontend,
test_flags=test_flags,
diff --git a/ivy_tests/test_ivy/test_frontends/test_mindspore/test_numpy.py b/ivy_tests/test_ivy/test_frontends/test_mindspore/test_numpy.py
index e4d1426717a87..1cad3008612ce 100644
--- a/ivy_tests/test_ivy/test_frontends/test_mindspore/test_numpy.py
+++ b/ivy_tests/test_ivy/test_frontends/test_mindspore/test_numpy.py
@@ -21,10 +21,12 @@
ndmin=st.integers(min_value=0, max_value=5),
copy=st.booleans(),
test_with_out=st.just(False),
+ test_with_copy=st.just(True),
)
def test_mindspore_array(
dtype_and_a,
frontend,
+ backend_fw,
test_flags,
fn_tree,
on_device,
@@ -35,6 +37,7 @@ def test_mindspore_array(
helpers.test_frontend_function(
input_dtypes=dtype,
frontend=frontend,
+ backend_to_test=backend_fw,
test_flags=test_flags,
fn_tree=fn_tree,
on_device=on_device,
diff --git a/ivy_tests/test_ivy/test_frontends/test_mindspore/test_ops/test_function/test_mindspore_nn_func.py b/ivy_tests/test_ivy/test_frontends/test_mindspore/test_ops/test_function/test_mindspore_nn_func.py
index 17919064ef663..46a0d22c152cd 100644
--- a/ivy_tests/test_ivy/test_frontends/test_mindspore/test_ops/test_function/test_mindspore_nn_func.py
+++ b/ivy_tests/test_ivy/test_frontends/test_mindspore/test_ops/test_function/test_mindspore_nn_func.py
@@ -18,15 +18,13 @@
def _calculate_same_padding(kernel_size, stride, shape):
padding = tuple(
- [
- max(
- 0,
- math.ceil(((shape[i] - 1) * stride[i] + kernel_size[i] - shape[i]) / 2),
- )
- for i in range(len(kernel_size))
- ]
+ max(
+ 0,
+ math.ceil(((shape[i] - 1) * stride[i] + kernel_size[i] - shape[i]) / 2),
+ )
+ for i in range(len(kernel_size))
)
- if all([kernel_size[i] / 2 >= padding[i] for i in range(len(kernel_size))]):
+ if all(kernel_size[i] / 2 >= padding[i] for i in range(len(kernel_size))):
if _is_same_padding(padding, stride, kernel_size, shape):
return padding
return (0, 0)
@@ -34,16 +32,12 @@ def _calculate_same_padding(kernel_size, stride, shape):
def _is_same_padding(padding, stride, kernel_size, input_shape):
output_shape = tuple(
- [
- (input_shape[i] + 2 * padding[i] - kernel_size[i]) // stride[i] + 1
- for i in range(len(padding))
- ]
+ (input_shape[i] + 2 * padding[i] - kernel_size[i]) // stride[i] + 1
+ for i in range(len(padding))
)
return all(
- [
- output_shape[i] == math.ceil(input_shape[i] / stride[i])
- for i in range(len(padding))
- ]
+ output_shape[i] == math.ceil(input_shape[i] / stride[i])
+ for i in range(len(padding))
)
@@ -189,6 +183,7 @@ def test_mindspore_adaptive_avg_pool2d(
output_size,
test_flags,
frontend,
+ backend_fw,
on_device,
fn_tree,
):
@@ -196,6 +191,7 @@ def test_mindspore_adaptive_avg_pool2d(
helpers.test_frontend_function(
input_dtypes=input_dtype,
frontend=frontend,
+ backend_to_test=backend_fw,
test_flags=test_flags,
on_device=on_device,
fn_tree=fn_tree,
@@ -261,7 +257,7 @@ def test_mindspore_avg_pool2d(
# conv1d
@pytest.mark.skip("Testing pipeline not yet implemented")
@handle_frontend_test(
- fn_tree="mindspore.ops.function.nn_func.Conv1d",
+ fn_tree="mindspore.ops.function.nn_func.conv1d",
dtype_vals=_x_and_filters(dim=1),
)
def test_mindspore_conv1d(
@@ -294,7 +290,7 @@ def test_mindspore_conv1d(
@pytest.mark.skip("Testing pipeline not yet implemented")
@handle_frontend_test(
- fn_tree="mindspore.ops.function.nn_func.Conv2d",
+ fn_tree="mindspore.ops.function.nn_func.conv2d",
dtype_vals=_x_and_filters(dim=2),
)
def test_mindspore_conv2d(
@@ -327,7 +323,7 @@ def test_mindspore_conv2d(
@pytest.mark.skip("Testing pipeline not yet implemented")
@handle_frontend_test(
- fn_tree="mindspore.ops.function.nn_func.Conv3d",
+ fn_tree="mindspore.ops.function.nn_func.conv3d",
dtype_vals=_x_and_filters(dim=3),
)
def test_mindspore_conv3d(
@@ -389,12 +385,14 @@ def test_mindspore_dropout2d(
on_device,
fn_tree,
frontend,
+ backend_fw,
test_flags,
):
dtype, x = d_type_and_x
helpers.test_frontend_function(
input_dtypes=dtype,
frontend=frontend,
+ backend_to_test=backend_fw,
test_flags=test_flags,
fn_tree=fn_tree,
on_device=on_device,
@@ -433,12 +431,14 @@ def test_mindspore_dropout3d(
on_device,
fn_tree,
frontend,
+ backend_fw,
test_flags,
):
dtype, x = d_type_and_x
helpers.test_frontend_function(
input_dtypes=dtype,
frontend=frontend,
+ backend_to_test=backend_fw,
test_flags=test_flags,
fn_tree=fn_tree,
on_device=on_device,
@@ -461,6 +461,7 @@ def test_mindspore_fast_gelu(
*,
test_flags,
frontend,
+ backend_fw,
on_device,
fn_tree,
):
@@ -469,6 +470,7 @@ def test_mindspore_fast_gelu(
helpers.test_frontend_function(
input_dtypes=input_dtype,
frontend=frontend,
+ backend_to_test=backend_fw,
test_flags=test_flags,
fn_tree=fn_tree,
on_device=on_device,
@@ -551,6 +553,7 @@ def test_mindspore_interpolate(
align_corners,
recompute_scale_factor,
on_device,
+ backend_fw,
fn_tree,
frontend,
test_flags,
@@ -562,6 +565,7 @@ def test_mindspore_interpolate(
helpers.test_frontend_function(
input_dtypes=dtype,
frontend=frontend,
+ backend_to_test=backend_fw,
test_flags=test_flags,
fn_tree=fn_tree,
on_device=on_device,
@@ -604,11 +608,13 @@ def test_mindspore_kl_div(
on_device,
fn_tree,
frontend,
+ backend_fw,
test_flags,
):
helpers.test_frontend_function(
input_dtypes=p[0],
frontend=frontend,
+ backend_to_test=backend_fw,
test_flags=test_flags,
fn_tree=fn_tree,
on_device=on_device,
@@ -714,7 +720,7 @@ def test_mindspore_max_pool3d(
# pad
@pytest.mark.skip("Testing pipeline not yet implemented")
@handle_frontend_test(
- fn_tree="pad",
+ fn_tree="mindspore.ops.function.nn_func.pad",
input=helpers.dtype_and_values(
available_dtypes=helpers.get_dtypes("valid"),
num_arrays=1,
@@ -740,11 +746,13 @@ def test_mindspore_pad(
on_device,
fn_tree,
frontend,
+ backend_fw,
test_flags,
):
helpers.test_frontend_function(
input_dtypes=input[0],
frontend=frontend,
+ backend_to_test=backend_fw,
test_flags=test_flags,
fn_tree=fn_tree,
on_device=on_device,
@@ -771,12 +779,14 @@ def test_mindspore_selu(
on_device,
fn_tree,
frontend,
+ backend_fw,
test_flags,
):
input_dtype, x = dtype_and_x
helpers.test_frontend_function(
input_dtypes=input_dtype,
frontend=frontend,
+ backend_to_test=backend_fw,
test_flags=test_flags,
fn_tree=fn_tree,
on_device=on_device,
diff --git a/ivy_tests/test_ivy/test_frontends/test_mindspore/test_ops/test_mindspore_nn_func.py b/ivy_tests/test_ivy/test_frontends/test_mindspore/test_ops/test_mindspore_nn_func.py
index 555b62c8665b6..e0fc11644ad71 100644
--- a/ivy_tests/test_ivy/test_frontends/test_mindspore/test_ops/test_mindspore_nn_func.py
+++ b/ivy_tests/test_ivy/test_frontends/test_mindspore/test_ops/test_mindspore_nn_func.py
@@ -22,12 +22,14 @@ def test_mindspore_softsign(
on_device,
fn_tree,
frontend,
+ backend_fw,
test_flags,
):
input_dtype, x = dtype_and_x
helpers.test_frontend_function(
input_dtypes=input_dtype,
frontend=frontend,
+ backend_to_test=backend_fw,
test_flags=test_flags,
fn_tree=fn_tree,
on_device=on_device,
diff --git a/ivy_tests/test_ivy/test_frontends/test_numpy/helpers.py b/ivy_tests/test_ivy/test_frontends/test_numpy/helpers.py
index 8f5fbbf38c50e..878ed7e54c44d 100644
--- a/ivy_tests/test_ivy/test_frontends/test_numpy/helpers.py
+++ b/ivy_tests/test_ivy/test_frontends/test_numpy/helpers.py
@@ -43,6 +43,7 @@ def _array_and_axes_permute_helper(
minimum size of the dimension
max_dim_size
maximum size of the dimension
+
Returns
-------
A strategy that draws an array, its dtype and axes (or None).
@@ -86,7 +87,7 @@ def _flatten_frontend_return(*, ret, backend):
else:
ret_np_flat = _flatten_fw_return(ret=ret, backend=backend)
else:
- if any([not ivy_backend.is_ivy_array(x) for x in ret]):
+ if any(not ivy_backend.is_ivy_array(x) for x in ret):
ret_np_flat = helpers.flatten_frontend_to_np(backend=backend, ret=ret)
else:
ret_np_flat = _flatten_fw_return(ret=ret, backend=backend)
@@ -222,11 +223,11 @@ def _test_frontend_function_ignoring_uninitialized(*args, **kwargs):
frontend_ret_flat = [
np.where(where, x, np.zeros_like(x)) for x in frontend_ret_np_flat
]
- if "rtol" in kwargs.keys():
+ if "rtol" in kwargs:
rtol = kwargs["rtol"]
else:
rtol = 1e-4
- if "atol" in kwargs.keys():
+ if "atol" in kwargs:
atol = kwargs["atol"]
else:
atol = 1e-6
@@ -312,7 +313,7 @@ def where(draw, *, shape=None):
# noinspection PyShadowingNames
def handle_where_and_array_bools(where, input_dtype, test_flags):
- if isinstance(where, list) or isinstance(where, tuple):
+ if isinstance(where, (list, tuple)):
where = where[0]
test_flags.as_variable += [False]
test_flags.native_arrays += [False]
diff --git a/ivy_tests/test_ivy/test_frontends/test_numpy/test_creation_routines/test_from_existing_data.py b/ivy_tests/test_ivy/test_frontends/test_numpy/test_creation_routines/test_from_existing_data.py
index 3423db46edf30..3f62109f66f79 100644
--- a/ivy_tests/test_ivy/test_frontends/test_numpy/test_creation_routines/test_from_existing_data.py
+++ b/ivy_tests/test_ivy/test_frontends/test_numpy/test_creation_routines/test_from_existing_data.py
@@ -17,6 +17,7 @@
max_dim_size=5,
),
test_with_out=st.just(False),
+ test_with_copy=st.just(True),
)
def test_numpy_array(
dtype_and_a,
@@ -85,6 +86,7 @@ def test_numpy_asarray(
max_dim_size=5,
),
test_with_out=st.just(False),
+ test_with_copy=st.just(True),
)
def test_numpy_copy(
dtype_and_a,
diff --git a/ivy_tests/test_ivy/test_frontends/test_numpy/test_creation_routines/test_numerical_ranges.py b/ivy_tests/test_ivy/test_frontends/test_numpy/test_creation_routines/test_numerical_ranges.py
index 0bea5cb7070c8..75676ccfe8dad 100644
--- a/ivy_tests/test_ivy/test_frontends/test_numpy/test_creation_routines/test_numerical_ranges.py
+++ b/ivy_tests/test_ivy/test_frontends/test_numpy/test_creation_routines/test_numerical_ranges.py
@@ -210,6 +210,7 @@ def test_numpy_logspace(
sparse=st.booleans(),
indexing=st.sampled_from(["xy", "ij"]),
test_with_out=st.just(False),
+ test_with_copy=st.just(True),
)
def test_numpy_meshgrid(
*,
diff --git a/ivy_tests/test_ivy/test_frontends/test_numpy/test_fft/test_discrete_fourier_transform.py b/ivy_tests/test_ivy/test_frontends/test_numpy/test_fft/test_discrete_fourier_transform.py
index 9e121cc13268c..26506d7008684 100644
--- a/ivy_tests/test_ivy/test_frontends/test_numpy/test_fft/test_discrete_fourier_transform.py
+++ b/ivy_tests/test_ivy/test_frontends/test_numpy/test_fft/test_discrete_fourier_transform.py
@@ -109,6 +109,27 @@ def test_numpy_ifft(dtype_and_x, backend_fw, frontend, test_flags, fn_tree, on_d
)
+@handle_frontend_test(
+ fn_tree="numpy.fft.ifft2",
+ dtype_and_x=_x_and_ifft(),
+)
+def test_numpy_ifft2(dtype_and_x, backend_fw, frontend, test_flags, fn_tree, on_device):
+ input_dtype, x, dim, norm, n = dtype_and_x
+ helpers.test_frontend_function(
+ input_dtypes=input_dtype,
+ frontend=frontend,
+ backend_to_test=backend_fw,
+ test_flags=test_flags,
+ fn_tree=fn_tree,
+ on_device=on_device,
+ test_values=True,
+ a=x,
+ s=None,
+ axes=None,
+ norm=norm,
+ )
+
+
@handle_frontend_test(
fn_tree="numpy.fft.ifftn",
dtype_and_x=_x_and_ifft(),
diff --git a/ivy_tests/test_ivy/test_frontends/test_numpy/test_func_wrapper.py b/ivy_tests/test_ivy/test_frontends/test_numpy/test_func_wrapper.py
index 0df77d4cac224..d93895355b185 100644
--- a/ivy_tests/test_ivy/test_frontends/test_numpy/test_func_wrapper.py
+++ b/ivy_tests/test_ivy/test_frontends/test_numpy/test_func_wrapper.py
@@ -46,7 +46,7 @@ def _dtype_helper(draw):
def _fn(*args, check_default=False, dtype=None):
if (
check_default
- and any([not (ivy.is_array(i) or hasattr(i, "ivy_array")) for i in args])
+ and any(not (ivy.is_array(i) or hasattr(i, "ivy_array")) for i in args)
and not ivy.exists(dtype)
):
ivy.utils.assertions.check_equal(
diff --git a/ivy_tests/test_ivy/test_frontends/test_numpy/test_indexing_routines/test_generating_index_arrays.py b/ivy_tests/test_ivy/test_frontends/test_numpy/test_indexing_routines/test_generating_index_arrays.py
index b4aaed5e18e77..419ccee3d9172 100644
--- a/ivy_tests/test_ivy/test_frontends/test_numpy/test_indexing_routines/test_generating_index_arrays.py
+++ b/ivy_tests/test_ivy/test_frontends/test_numpy/test_indexing_routines/test_generating_index_arrays.py
@@ -1,6 +1,7 @@
# global
import numpy as np
from hypothesis import strategies as st
+from numpy import triu, tril
# local
import ivy_tests.test_ivy.helpers as helpers
@@ -92,6 +93,39 @@ def test_numpy_indices(
)
+@handle_frontend_test(
+ fn_tree="numpy.mask_indices",
+ n=helpers.ints(min_value=3, max_value=10),
+ mask_func=st.sampled_from([triu, tril]),
+ k=helpers.ints(min_value=-5, max_value=5),
+ input_dtype=helpers.get_dtypes("numeric"),
+ test_with_out=st.just(False),
+ number_positional_args=st.just(2),
+)
+def test_numpy_mask_indices(
+ n,
+ mask_func,
+ k,
+ input_dtype,
+ test_flags,
+ frontend,
+ backend_fw,
+ fn_tree,
+ on_device,
+):
+ helpers.test_frontend_function(
+ input_dtypes=input_dtype,
+ backend_to_test=backend_fw,
+ test_flags=test_flags,
+ frontend=frontend,
+ fn_tree=fn_tree,
+ on_device=on_device,
+ n=n,
+ mask_func=mask_func,
+ k=k,
+ )
+
+
@handle_frontend_test(
fn_tree="numpy.tril_indices",
n=helpers.ints(min_value=1, max_value=10),
diff --git a/ivy_tests/test_ivy/test_frontends/test_numpy/test_indexing_routines/test_inserting_data_into_arrays.py b/ivy_tests/test_ivy/test_frontends/test_numpy/test_indexing_routines/test_inserting_data_into_arrays.py
index 186794403dc41..a03cc96ee21a4 100644
--- a/ivy_tests/test_ivy/test_frontends/test_numpy/test_indexing_routines/test_inserting_data_into_arrays.py
+++ b/ivy_tests/test_ivy/test_frontends/test_numpy/test_indexing_routines/test_inserting_data_into_arrays.py
@@ -51,8 +51,7 @@ def _helper_r_(draw):
to_mat = draw(st.booleans())
if to_mat:
elem = draw(st.sampled_from(["c", "r"]))
- if dim > 2:
- dim = 2
+ dim = min(dim, 2)
else:
num = draw(st.integers(1, 3))
elem = ""
diff --git a/ivy_tests/test_ivy/test_frontends/test_numpy/test_manipulation_routines/test_basic_operations.py b/ivy_tests/test_ivy/test_frontends/test_numpy/test_manipulation_routines/test_basic_operations.py
index 822ccfe4b46ab..25df72b029fd5 100644
--- a/ivy_tests/test_ivy/test_frontends/test_numpy/test_manipulation_routines/test_basic_operations.py
+++ b/ivy_tests/test_ivy/test_frontends/test_numpy/test_manipulation_routines/test_basic_operations.py
@@ -42,7 +42,7 @@ def test_numpy_copyto(
frontend,
):
_, xs, casting, where = copyto_args
- if isinstance(where, list) or isinstance(where, tuple):
+ if isinstance(where, (list, tuple)):
where = where[0]
with BackendHandler.update_backend(backend_fw) as ivy_backend:
diff --git a/ivy_tests/test_ivy/test_frontends/test_numpy/test_manipulation_routines/test_padding_arrays.py b/ivy_tests/test_ivy/test_frontends/test_numpy/test_manipulation_routines/test_padding_arrays.py
index 2fa46f5db1cf2..8fe30ac7ca58f 100644
--- a/ivy_tests/test_ivy/test_frontends/test_numpy/test_manipulation_routines/test_padding_arrays.py
+++ b/ivy_tests/test_ivy/test_frontends/test_numpy/test_manipulation_routines/test_padding_arrays.py
@@ -46,7 +46,7 @@ def _pad_helper(draw):
ndim = len(shape)
pad_width = draw(_st_tuples_or_int(ndim, min_val=0))
kwargs = {}
- if mode == "reflect" or mode == "symmetric":
+ if mode in ["reflect", "symmetric"]:
kwargs["reflect_type"] = draw(st.sampled_from(["even", "odd"]))
if mode in ["maximum", "mean", "median", "minimum"]:
kwargs["stat_length"] = draw(_st_tuples_or_int(ndim, min_val=2))
diff --git a/ivy_tests/test_ivy/test_frontends/test_numpy/test_mathematical_functions/test_floating_point_routines.py b/ivy_tests/test_ivy/test_frontends/test_numpy/test_mathematical_functions/test_floating_point_routines.py
index 54924a62fb272..0e108cd4754df 100644
--- a/ivy_tests/test_ivy/test_frontends/test_numpy/test_mathematical_functions/test_floating_point_routines.py
+++ b/ivy_tests/test_ivy/test_frontends/test_numpy/test_mathematical_functions/test_floating_point_routines.py
@@ -54,6 +54,54 @@ def test_numpy_nextafter(
)
+# signbit
+@handle_frontend_test(
+ fn_tree="numpy.signbit",
+ dtypes_values_casting=np_frontend_helpers.dtypes_values_casting_dtype(
+ arr_func=[
+ lambda: helpers.dtype_and_values(
+ available_dtypes=helpers.get_dtypes("valid"),
+ shared_dtype=True,
+ )
+ ],
+ ),
+ where=np_frontend_helpers.where(),
+ number_positional_args=np_frontend_helpers.get_num_positional_args_ufunc(
+ fn_name="signbit"
+ ),
+)
+def test_numpy_signbit(
+ dtypes_values_casting,
+ where,
+ frontend,
+ test_flags,
+ backend_fw,
+ fn_tree,
+ on_device,
+):
+ input_dtypes, xs, casting, dtype = dtypes_values_casting
+ where, input_dtypes, test_flags = np_frontend_helpers.handle_where_and_array_bools(
+ where=where,
+ input_dtype=input_dtypes,
+ test_flags=test_flags,
+ )
+ np_frontend_helpers.test_frontend_function(
+ input_dtypes=input_dtypes,
+ frontend=frontend,
+ test_flags=test_flags,
+ backend_to_test=backend_fw,
+ fn_tree=fn_tree,
+ on_device=on_device,
+ x=xs[0],
+ out=None,
+ where=where,
+ casting="safe",
+ order="K",
+ dtype=dtype,
+ subok=True,
+ )
+
+
# spacing
@handle_frontend_test(
fn_tree="numpy.spacing",
diff --git a/ivy_tests/test_ivy/test_frontends/test_numpy/test_mathematical_functions/test_miscellaneous.py b/ivy_tests/test_ivy/test_frontends/test_numpy/test_mathematical_functions/test_miscellaneous.py
index 6a34e465aba4e..f596f3454031c 100644
--- a/ivy_tests/test_ivy/test_frontends/test_numpy/test_mathematical_functions/test_miscellaneous.py
+++ b/ivy_tests/test_ivy/test_frontends/test_numpy/test_mathematical_functions/test_miscellaneous.py
@@ -549,6 +549,7 @@ def test_numpy_lcm(
nan=st.floats(min_value=0, max_value=10),
copy=st.booleans(),
test_with_out=st.just(False),
+ test_with_copy=st.just(True),
)
def test_numpy_nan_to_num(
dtype_and_x,
diff --git a/ivy_tests/test_ivy/test_frontends/test_numpy/test_ndarray/test_ndarray.py b/ivy_tests/test_ivy/test_frontends/test_numpy/test_ndarray/test_ndarray.py
index 11e761c60e2ec..f19d5968df2aa 100644
--- a/ivy_tests/test_ivy/test_frontends/test_numpy/test_ndarray/test_ndarray.py
+++ b/ivy_tests/test_ivy/test_frontends/test_numpy/test_ndarray/test_ndarray.py
@@ -11,6 +11,9 @@
assert_all_close,
BackendHandler,
)
+from ivy_tests.test_ivy.test_functional.test_core.test_statistical import (
+ _statistical_dtype_values,
+)
import ivy_tests.test_ivy.test_frontends.test_numpy.helpers as np_frontend_helpers
from ivy_tests.test_ivy.test_functional.test_core.test_linalg import (
_get_first_matrix_and_dtype,
@@ -28,9 +31,6 @@
from ivy_tests.test_ivy.test_frontends.test_numpy.test_manipulation_routines.test_changing_number_of_dimensions import ( # noqa
_squeeze_helper,
)
-from ivy_tests.test_ivy.test_functional.test_core.test_statistical import (
- _statistical_dtype_values,
-)
CLASS_TREE = "ivy.functional.frontends.numpy.ndarray"
@@ -1077,6 +1077,56 @@ def test_numpy___ipow__(
)
+# __irshift__
+@handle_frontend_method(
+ class_tree=CLASS_TREE,
+ init_tree="numpy.array",
+ method_name="__irshift__",
+ dtype_and_x=helpers.dtype_and_values(
+ available_dtypes=helpers.get_dtypes("integer"),
+ num_arrays=2,
+ ),
+)
+def test_numpy___irshift__(
+ dtype_and_x,
+ frontend_method_data,
+ init_flags,
+ method_flags,
+ frontend,
+ backend_fw,
+ on_device,
+):
+ input_dtypes, x = dtype_and_x
+ max_bits = np.iinfo(input_dtypes[0]).bits
+ max_shift = max_bits - 1
+ x[1] = np.asarray(np.clip(x[1], 0, max_shift), dtype=input_dtypes[1])
+ max_value_before_shift = 2 ** (max_bits - x[1]) - 1
+ overflow_threshold = 2 ** (max_bits - 1)
+ x[0] = np.asarray(
+ np.clip(x[0], None, max_value_before_shift), dtype=input_dtypes[0]
+ )
+ if np.any(x[0] > overflow_threshold):
+ x[0] = np.clip(x[0], None, overflow_threshold)
+ if np.any(x[0] < 0):
+ x[0] = np.abs(x[0])
+ helpers.test_frontend_method(
+ init_input_dtypes=input_dtypes,
+ init_all_as_kwargs_np={
+ "object": x[0],
+ },
+ method_input_dtypes=input_dtypes,
+ backend_to_test=backend_fw,
+ method_all_as_kwargs_np={
+ "value": x[1],
+ },
+ frontend=frontend,
+ frontend_method_data=frontend_method_data,
+ init_flags=init_flags,
+ method_flags=method_flags,
+ on_device=on_device,
+ )
+
+
@handle_frontend_method(
class_tree=CLASS_TREE,
init_tree="numpy.array",
@@ -1891,90 +1941,6 @@ def test_numpy___xor__(
)
-# __getitem__
-@handle_frontend_method(
- class_tree=CLASS_TREE,
- init_tree="numpy.array",
- method_name="__getitem__",
- dtype_x_index=helpers.dtype_array_query(
- available_dtypes=helpers.get_dtypes("valid"),
- ),
-)
-def test_numpy_getitem(
- dtype_x_index,
- frontend_method_data,
- init_flags,
- method_flags,
- backend_fw,
- frontend,
- on_device,
-):
- input_dtype, x, index = dtype_x_index
- helpers.test_frontend_method(
- init_input_dtypes=[input_dtype[0]],
- init_all_as_kwargs_np={"object": x},
- method_input_dtypes=[*input_dtype[1:]],
- method_all_as_kwargs_np={"key": index},
- backend_to_test=backend_fw,
- frontend_method_data=frontend_method_data,
- init_flags=init_flags,
- method_flags=method_flags,
- frontend=frontend,
- on_device=on_device,
- )
-
-
-@handle_frontend_method(
- class_tree=CLASS_TREE,
- init_tree="numpy.array",
- method_name="__lshift__",
- dtype_and_x=helpers.dtype_and_values(
- available_dtypes=helpers.get_dtypes("integer"),
- num_arrays=2,
- max_dim_size=1,
- max_value=2**31 - 1,
- ),
-)
-def test_numpy_instance_lshift__(
- dtype_and_x,
- frontend_method_data,
- init_flags,
- method_flags,
- frontend,
- backend_fw,
- on_device,
-):
- input_dtypes, x = dtype_and_x
- max_bits = np.iinfo(input_dtypes[0]).bits
- max_shift = max_bits - 1
- x[1] = np.asarray(np.clip(x[1], 0, max_shift), dtype=input_dtypes[1])
- max_value_before_shift = 2 ** (max_bits - x[1]) - 1
- overflow_threshold = 2 ** (max_bits - 1)
- x[0] = np.asarray(
- np.clip(x[0], None, max_value_before_shift), dtype=input_dtypes[0]
- )
- if np.any(x[0] > overflow_threshold):
- x[0] = np.clip(x[0], None, overflow_threshold)
- if np.any(x[0] < 0):
- x[0] = np.abs(x[0])
- helpers.test_frontend_method(
- init_input_dtypes=input_dtypes,
- init_all_as_kwargs_np={
- "object": x[0],
- },
- method_input_dtypes=input_dtypes,
- backend_to_test=backend_fw,
- method_all_as_kwargs_np={
- "value": x[1],
- },
- frontend=frontend,
- frontend_method_data=frontend_method_data,
- init_flags=init_flags,
- method_flags=method_flags,
- on_device=on_device,
- )
-
-
@handle_frontend_method(
class_tree=CLASS_TREE,
init_tree="numpy.array",
@@ -1991,7 +1957,7 @@ def test_numpy_instance_lshift__(
keepdims=st.booleans(),
where=np_frontend_helpers.where(),
)
-def test_numpy_ndarray_all(
+def test_numpy_all(
dtype_x_axis,
keepdims,
where,
@@ -2052,7 +2018,7 @@ def test_numpy_ndarray_all(
keepdims=st.booleans(),
where=np_frontend_helpers.where(),
)
-def test_numpy_ndarray_any(
+def test_numpy_any(
dtype_x_axis,
keepdims,
where,
@@ -2111,7 +2077,7 @@ def test_numpy_ndarray_any(
),
keep_dims=st.booleans(),
)
-def test_numpy_ndarray_argmax(
+def test_numpy_argmax(
dtype_x_axis,
keep_dims,
frontend_method_data,
@@ -2153,7 +2119,7 @@ def test_numpy_ndarray_argmax(
),
keepdims=st.booleans(),
)
-def test_numpy_ndarray_argmin(
+def test_numpy_argmin(
dtype_x_axis,
keepdims,
frontend_method_data,
@@ -2195,7 +2161,7 @@ def test_numpy_ndarray_argmin(
force_int_axis=True,
),
)
-def test_numpy_ndarray_argsort(
+def test_numpy_argsort(
dtype_x_axis,
frontend_method_data,
init_flags,
@@ -2239,7 +2205,7 @@ def test_numpy_ndarray_argsort(
order=st.sampled_from(["C", "F", "A", "K"]),
copy=st.booleans(),
)
-def test_numpy_ndarray_astype(
+def test_numpy_astype(
dtypes_values_casting,
order,
copy,
@@ -2278,7 +2244,7 @@ def test_numpy_ndarray_astype(
method_name="clip",
input_and_ranges=_get_clip_inputs(),
)
-def test_numpy_ndarray_clip(
+def test_numpy_clip(
input_and_ranges,
frontend_method_data,
init_flags,
@@ -2327,7 +2293,7 @@ def test_numpy_ndarray_clip(
),
),
)
-def test_numpy_ndarray_compress(
+def test_numpy_compress(
dtype_arr_ax,
condition,
frontend_method_data,
@@ -2366,7 +2332,7 @@ def test_numpy_ndarray_compress(
available_dtypes=helpers.get_dtypes("real_and_complex"),
),
)
-def test_numpy_ndarray_conjugate(
+def test_numpy_conjugate(
dtype_and_x,
on_device,
frontend,
@@ -2401,7 +2367,7 @@ def test_numpy_ndarray_conjugate(
min_num_dims=1,
),
)
-def test_numpy_ndarray_copy(
+def test_numpy_copy(
dtype_and_x,
frontend_method_data,
init_flags,
@@ -2441,7 +2407,7 @@ def test_numpy_ndarray_copy(
),
dtype=helpers.get_dtypes("float", full=False, none=True),
)
-def test_numpy_ndarray_cumprod(
+def test_numpy_cumprod(
dtype_x_axis,
dtype,
frontend_method_data,
@@ -2479,7 +2445,7 @@ def test_numpy_ndarray_cumprod(
method_name="cumsum",
dtype_x_axis_dtype=_get_castable_dtypes_values(),
)
-def test_numpy_ndarray_cumsum(
+def test_numpy_cumsum(
dtype_x_axis_dtype,
frontend_method_data,
init_flags,
@@ -2522,7 +2488,7 @@ def test_numpy_ndarray_cumsum(
),
offset=st.integers(min_value=-2, max_value=2),
)
-def test_numpy_ndarray_diagonal(
+def test_numpy_diagonal(
dtype_x_axis,
offset,
frontend_method_data,
@@ -2560,7 +2526,7 @@ def test_numpy_ndarray_diagonal(
method_name="dot",
dtype_and_x=np_frontend_helpers._get_dtype_input_and_vectors(),
)
-def test_numpy_ndarray_dot(
+def test_numpy_dot(
dtype_and_x,
frontend_method_data,
init_flags,
@@ -2594,7 +2560,7 @@ def test_numpy_ndarray_dot(
ret_shape=True,
),
)
-def test_numpy_ndarray_dtype(dtype_x, backend_fw, frontend):
+def test_numpy_dtype(dtype_x, backend_fw, frontend):
dtype, data, shape = dtype_x
with BackendHandler.update_backend(backend_fw) as ivy_backend:
x = ivy_backend.functional.frontends.numpy.ndarray(shape, dtype[0])
@@ -2614,7 +2580,7 @@ def test_numpy_ndarray_dtype(dtype_x, backend_fw, frontend):
),
num=st.integers(min_value=1, max_value=10) | st.floats(min_value=1, max_value=10),
)
-def test_numpy_ndarray_fill(
+def test_numpy_fill(
dtype_and_x,
num,
frontend_method_data,
@@ -2650,7 +2616,7 @@ def test_numpy_ndarray_fill(
ret_shape=True,
)
)
-def test_numpy_ndarray_flat(dtype_x, backend_fw):
+def test_numpy_flat(dtype_x, backend_fw):
dtype, data, shape = dtype_x
with BackendHandler.update_backend(backend_fw) as ivy_backend:
@@ -2665,13 +2631,149 @@ def test_numpy_ndarray_flat(dtype_x, backend_fw):
)
+# __getitem__
+@handle_frontend_method(
+ class_tree=CLASS_TREE,
+ init_tree="numpy.array",
+ method_name="__getitem__",
+ dtype_x_index=helpers.dtype_array_query(
+ available_dtypes=helpers.get_dtypes("valid"),
+ ),
+)
+def test_numpy_getitem(
+ dtype_x_index,
+ frontend_method_data,
+ init_flags,
+ method_flags,
+ backend_fw,
+ frontend,
+ on_device,
+):
+ input_dtype, x, index = dtype_x_index
+ helpers.test_frontend_method(
+ init_input_dtypes=[input_dtype[0]],
+ init_all_as_kwargs_np={"object": x},
+ method_input_dtypes=[*input_dtype[1:]],
+ method_all_as_kwargs_np={"key": index},
+ backend_to_test=backend_fw,
+ frontend_method_data=frontend_method_data,
+ init_flags=init_flags,
+ method_flags=method_flags,
+ frontend=frontend,
+ on_device=on_device,
+ )
+
+
+# __ilshift__
+@handle_frontend_method(
+ class_tree=CLASS_TREE,
+ init_tree="numpy.array",
+ method_name="__ilshift__",
+ dtype_and_x=helpers.dtype_and_values(
+ available_dtypes=helpers.get_dtypes("integer"),
+ num_arrays=2,
+ max_dim_size=1,
+ max_value=2**31 - 1,
+ ),
+)
+def test_numpy_instance_ilshift__(
+ dtype_and_x,
+ frontend_method_data,
+ init_flags,
+ method_flags,
+ frontend,
+ backend_fw,
+ on_device,
+):
+ input_dtypes, x = dtype_and_x
+ max_bits = np.iinfo(input_dtypes[0]).bits
+ max_shift = max_bits - 1
+ x[1] = np.asarray(np.clip(x[1], 0, max_shift), dtype=input_dtypes[1])
+ max_value_before_shift = 2 ** (max_bits - x[1]) - 1
+ overflow_threshold = 2 ** (max_bits - 1)
+ x[0] = np.asarray(
+ np.clip(x[0], None, max_value_before_shift), dtype=input_dtypes[0]
+ )
+ if np.any(x[0] > overflow_threshold):
+ x[0] = np.clip(x[0], None, overflow_threshold)
+ if np.any(x[0] < 0):
+ x[0] = np.abs(x[0])
+ helpers.test_frontend_method(
+ init_input_dtypes=input_dtypes,
+ init_all_as_kwargs_np={
+ "object": x[0],
+ },
+ method_input_dtypes=input_dtypes,
+ backend_to_test=backend_fw,
+ method_all_as_kwargs_np={
+ "value": x[1],
+ },
+ frontend=frontend,
+ frontend_method_data=frontend_method_data,
+ init_flags=init_flags,
+ method_flags=method_flags,
+ on_device=on_device,
+ )
+
+
+@handle_frontend_method(
+ class_tree=CLASS_TREE,
+ init_tree="numpy.array",
+ method_name="__lshift__",
+ dtype_and_x=helpers.dtype_and_values(
+ available_dtypes=helpers.get_dtypes("integer"),
+ num_arrays=2,
+ max_dim_size=1,
+ max_value=2**31 - 1,
+ ),
+)
+def test_numpy_instance_lshift__(
+ dtype_and_x,
+ frontend_method_data,
+ init_flags,
+ method_flags,
+ frontend,
+ backend_fw,
+ on_device,
+):
+ input_dtypes, x = dtype_and_x
+ max_bits = np.iinfo(input_dtypes[0]).bits
+ max_shift = max_bits - 1
+ x[1] = np.asarray(np.clip(x[1], 0, max_shift), dtype=input_dtypes[1])
+ max_value_before_shift = 2 ** (max_bits - x[1]) - 1
+ overflow_threshold = 2 ** (max_bits - 1)
+ x[0] = np.asarray(
+ np.clip(x[0], None, max_value_before_shift), dtype=input_dtypes[0]
+ )
+ if np.any(x[0] > overflow_threshold):
+ x[0] = np.clip(x[0], None, overflow_threshold)
+ if np.any(x[0] < 0):
+ x[0] = np.abs(x[0])
+ helpers.test_frontend_method(
+ init_input_dtypes=input_dtypes,
+ init_all_as_kwargs_np={
+ "object": x[0],
+ },
+ method_input_dtypes=input_dtypes,
+ backend_to_test=backend_fw,
+ method_all_as_kwargs_np={
+ "value": x[1],
+ },
+ frontend=frontend,
+ frontend_method_data=frontend_method_data,
+ init_flags=init_flags,
+ method_flags=method_flags,
+ on_device=on_device,
+ )
+
+
@handle_frontend_method(
class_tree=CLASS_TREE,
init_tree="numpy.array",
method_name="item",
args_kwargs=_item_helper(),
)
-def test_numpy_ndarray_item(
+def test_numpy_item(
args_kwargs,
frontend_method_data,
init_flags,
@@ -2702,7 +2804,7 @@ def test_numpy_ndarray_item(
ret_shape=True,
),
)
-def test_numpy_ndarray_ivy_array(
+def test_numpy_ivy_array(
dtype_x,
frontend,
backend_fw,
@@ -2734,7 +2836,7 @@ def test_numpy_ndarray_ivy_array(
),
keepdims=st.booleans(),
)
-def test_numpy_ndarray_max(
+def test_numpy_max(
dtype_x_axis,
keepdims,
frontend_method_data,
@@ -2774,7 +2876,7 @@ def test_numpy_ndarray_max(
where=np_frontend_helpers.where(),
keep_dims=st.booleans(),
)
-def test_numpy_ndarray_mean(
+def test_numpy_mean(
dtype_and_x,
dtype,
where,
@@ -2829,7 +2931,7 @@ def test_numpy_ndarray_mean(
),
keepdims=st.booleans(),
)
-def test_numpy_ndarray_min(
+def test_numpy_min(
dtype_x_axis,
keepdims,
frontend_method_data,
@@ -2868,7 +2970,7 @@ def test_numpy_ndarray_min(
available_dtypes=helpers.get_dtypes("valid"),
),
)
-def test_numpy_ndarray_nonzero(
+def test_numpy_nonzero(
dtype_and_a,
frontend_method_data,
init_flags,
@@ -2904,7 +3006,7 @@ def test_numpy_ndarray_nonzero(
keep_dims=st.booleans(),
initial=st.one_of(st.floats(min_value=-100, max_value=100)),
)
-def test_numpy_ndarray_prod(
+def test_numpy_prod(
dtype_x_axis_dtype,
keep_dims,
initial,
@@ -2957,7 +3059,7 @@ def test_numpy_ndarray_prod(
ret_shape=True,
),
)
-def test_numpy_ndarray_property_ndim(dtype_x, backend_fw):
+def test_numpy_property_ndim(dtype_x, backend_fw):
dtype, data, shape = dtype_x
with BackendHandler.update_backend(backend_fw) as ivy_backend:
x = ivy_backend.functional.frontends.numpy.ndarray(shape, dtype[0])
@@ -2975,7 +3077,7 @@ def test_numpy_ndarray_property_ndim(dtype_x, backend_fw):
valid_axis=True,
),
)
-def test_numpy_ndarray_ptp(
+def test_numpy_ptp(
dtype_x_axis,
frontend_method_data,
init_flags,
@@ -3011,7 +3113,7 @@ def test_numpy_ndarray_ptp(
available_dtypes=helpers.get_dtypes("valid"),
),
)
-def test_numpy_ndarray_ravel(
+def test_numpy_ravel(
dtype_and_a,
frontend_method_data,
init_flags,
@@ -3050,7 +3152,7 @@ def test_numpy_ndarray_ravel(
repeats=helpers.ints(min_value=2, max_value=5),
axis=helpers.ints(min_value=-1, max_value=1),
)
-def test_numpy_ndarray_repeat(
+def test_numpy_repeat(
dtype_and_x,
repeats,
axis,
@@ -3089,7 +3191,7 @@ def test_numpy_ndarray_repeat(
dtypes_x_shape=dtypes_x_reshape(),
order=st.sampled_from(["C", "F", "A"]),
)
-def test_numpy_ndarray_reshape(
+def test_numpy_reshape(
dtypes_x_shape,
order,
frontend_method_data,
@@ -3132,7 +3234,7 @@ def test_numpy_ndarray_reshape(
),
decimals=st.integers(min_value=0, max_value=3),
)
-def test_numpy_ndarray_round(
+def test_numpy_round(
dtype_and_x,
decimals,
frontend_method_data,
@@ -3173,7 +3275,7 @@ def test_numpy_ndarray_round(
),
side=st.sampled_from(["left", "right"]),
)
-def test_numpy_ndarray_searchsorted(
+def test_numpy_searchsorted(
dtype_x_v,
side,
frontend_method_data,
@@ -3214,7 +3316,7 @@ def test_numpy_ndarray_searchsorted(
available_dtypes=helpers.get_dtypes("valid"),
),
)
-def test_numpy_ndarray_setitem(
+def test_numpy_setitem(
dtypes_x_index_val,
frontend_method_data,
init_flags,
@@ -3244,7 +3346,7 @@ def test_numpy_ndarray_setitem(
ret_shape=True,
),
)
-def test_numpy_ndarray_shape(
+def test_numpy_shape(
dtype_x,
backend_fw,
):
@@ -3263,7 +3365,7 @@ def test_numpy_ndarray_shape(
ret_shape=True,
),
)
-def test_numpy_ndarray_size(
+def test_numpy_size(
dtype_x,
):
dtype, data, shape = dtype_x
@@ -3284,7 +3386,7 @@ def test_numpy_ndarray_size(
force_int_axis=True,
),
)
-def test_numpy_ndarray_sort(
+def test_numpy_sort(
dtype_x_axis,
frontend_method_data,
init_flags,
@@ -3333,7 +3435,7 @@ def test_numpy_ndarray_sort(
),
axis=_squeeze_helper(),
)
-def test_numpy_ndarray_squeeze(
+def test_numpy_squeeze(
dtype_and_x,
axis,
frontend_method_data,
@@ -3376,7 +3478,7 @@ def test_numpy_ndarray_squeeze(
keepdims=st.booleans(),
where=np_frontend_helpers.where(),
)
-def test_numpy_ndarray_std(
+def test_numpy_std(
dtype_x_axis,
keepdims,
where,
@@ -3428,7 +3530,7 @@ def test_numpy_ndarray_std(
keep_dims=st.booleans(),
initial=st.one_of(st.floats(min_value=-100, max_value=100)),
)
-def test_numpy_ndarray_sum(
+def test_numpy_sum(
dtype_x_axis_dtype,
keep_dims,
initial,
@@ -3479,7 +3581,7 @@ def test_numpy_ndarray_sum(
method_name="swapaxes",
dtype_x_and_axes=dtype_values_and_axes(),
)
-def test_numpy_ndarray_swapaxes(
+def test_numpy_swapaxes(
dtype_x_and_axes,
frontend,
frontend_method_data,
@@ -3516,7 +3618,7 @@ def test_numpy_ndarray_swapaxes(
),
order=st.sampled_from(["C", "F"]),
)
-def test_numpy_ndarray_tobytes(
+def test_numpy_tobytes(
dtype_x,
order,
backend_fw,
@@ -3544,7 +3646,7 @@ def test_numpy_ndarray_tobytes(
max_size=50,
),
)
-def test_numpy_ndarray_tofile(
+def test_numpy_tofile(
dtype_and_x,
path,
frontend_method_data,
@@ -3582,7 +3684,7 @@ def test_numpy_ndarray_tofile(
available_dtypes=helpers.get_dtypes("valid"),
),
)
-def test_numpy_ndarray_tolist(
+def test_numpy_tolist(
dtype_and_x,
frontend_method_data,
init_flags,
@@ -3620,7 +3722,7 @@ def test_numpy_ndarray_tolist(
max_dim_size=10,
),
)
-def test_numpy_ndarray_transpose(
+def test_numpy_transpose(
array_and_axes,
frontend_method_data,
init_flags,
@@ -3648,6 +3750,52 @@ def test_numpy_ndarray_transpose(
)
+# var
+@handle_frontend_method(
+ class_tree=CLASS_TREE,
+ init_tree="numpy.array",
+ method_name="var",
+ dtype_x_axis=_statistical_dtype_values(function="var"),
+ dtype=helpers.get_dtypes("valid", full=False, none=True),
+ where=np_frontend_helpers.where(),
+ keepdims=st.booleans(),
+)
+def test_numpy_var(
+ dtype_x_axis,
+ frontend_method_data,
+ init_flags,
+ method_flags,
+ frontend,
+ backend_fw,
+ on_device,
+ keepdims,
+ where,
+ dtype,
+):
+ input_dtypes, x, axis = dtype_x_axis
+ helpers.test_frontend_method(
+ init_input_dtypes=input_dtypes,
+ method_input_dtypes=input_dtypes,
+ init_all_as_kwargs_np={
+ "object": x[0],
+ },
+ method_all_as_kwargs_np={
+ "axis": axis,
+ "dtype": dtype,
+ "keepdims": keepdims,
+ "where": where,
+ },
+ frontend=frontend,
+ backend_to_test=backend_fw,
+ frontend_method_data=frontend_method_data,
+ init_flags=init_flags,
+ method_flags=method_flags,
+ rtol_=1e-2,
+ atol_=1e-2,
+ on_device=on_device,
+ )
+
+
# view
@handle_frontend_method(
class_tree=CLASS_TREE,
@@ -3657,7 +3805,7 @@ def test_numpy_ndarray_transpose(
available_dtypes=helpers.get_dtypes("valid"),
),
)
-def test_numpy_ndarray_view(
+def test_numpy_view(
dtype_and_x,
frontend_method_data,
init_flags,
diff --git a/ivy_tests/test_ivy/test_frontends/test_numpy/test_random/test_functions.py b/ivy_tests/test_ivy/test_frontends/test_numpy/test_random/test_functions.py
index df6e88d78bace..ed12dacf5bdc8 100644
--- a/ivy_tests/test_ivy/test_frontends/test_numpy/test_random/test_functions.py
+++ b/ivy_tests/test_ivy/test_frontends/test_numpy/test_random/test_functions.py
@@ -971,6 +971,33 @@ def test_numpy_standard_cauchy(
)
+@handle_frontend_test(
+ fn_tree="numpy.random.standard_exponential",
+ input_dtypes=helpers.get_dtypes("float", index=2),
+ size=helpers.get_shape(allow_none=True),
+ test_with_out=st.just(False),
+)
+def test_numpy_standard_exponential(
+ input_dtypes,
+ frontend,
+ test_flags,
+ backend_fw,
+ fn_tree,
+ on_device,
+ size,
+):
+ helpers.test_frontend_function(
+ input_dtypes=input_dtypes,
+ backend_to_test=backend_fw,
+ frontend=frontend,
+ test_flags=test_flags,
+ fn_tree=fn_tree,
+ on_device=on_device,
+ test_values=False,
+ size=size,
+ )
+
+
@handle_frontend_test(
fn_tree="numpy.random.standard_gamma",
shape_dtypes=helpers.get_dtypes("float", full=False),
diff --git a/ivy_tests/test_ivy/test_frontends/test_onnx/test_elementwise.py b/ivy_tests/test_ivy/test_frontends/test_onnx/test_elementwise.py
index 50cf23e71c94d..0f997b69770f1 100644
--- a/ivy_tests/test_ivy/test_frontends/test_onnx/test_elementwise.py
+++ b/ivy_tests/test_ivy/test_frontends/test_onnx/test_elementwise.py
@@ -28,12 +28,14 @@ def test_onnx_abs(
on_device,
fn_tree,
frontend,
+ backend_fw,
test_flags,
):
input_dtype, x = dtype_and_x
helpers.test_frontend_function(
input_dtypes=input_dtype,
frontend=frontend,
+ backend_to_test=backend_fw,
test_flags=test_flags,
fn_tree=fn_tree,
on_device=on_device,
@@ -81,10 +83,12 @@ def test_onnx_acos(
on_device,
fn_tree,
frontend,
+ backend_fw,
test_flags,
):
input_dtype, x = dtype_and_x
helpers.test_frontend_function(
+ backend_to_test=backend_fw,
input_dtypes=input_dtype,
frontend=frontend,
test_flags=test_flags,
@@ -131,10 +135,12 @@ def test_onnx_acosh(
on_device,
fn_tree,
frontend,
+ backend_fw,
test_flags,
):
input_dtype, x = dtype_and_x
helpers.test_frontend_function(
+ backend_to_test=backend_fw,
input_dtypes=input_dtype,
frontend=frontend,
test_flags=test_flags,
@@ -187,12 +193,14 @@ def test_onnx_add(
on_device,
fn_tree,
frontend,
+ backend_fw,
test_flags,
):
input_dtype, x = dtype_and_x
helpers.test_frontend_function(
input_dtypes=input_dtype,
frontend=frontend,
+ backend_to_test=backend_fw,
test_flags=test_flags,
fn_tree=fn_tree,
on_device=on_device,
@@ -246,12 +254,14 @@ def test_onnx_asin(
on_device,
fn_tree,
frontend,
+ backend_fw,
test_flags,
):
input_dtype, x = dtype_and_x
helpers.test_frontend_function(
input_dtypes=input_dtype,
frontend=frontend,
+ backend_to_test=backend_fw,
test_flags=test_flags,
fn_tree=fn_tree,
on_device=on_device,
diff --git a/ivy_tests/test_ivy/test_frontends/test_paddle/test_creation.py b/ivy_tests/test_ivy/test_frontends/test_paddle/test_creation.py
index 054f4980e17f7..cc0165ae4f6a1 100644
--- a/ivy_tests/test_ivy/test_frontends/test_paddle/test_creation.py
+++ b/ivy_tests/test_ivy/test_frontends/test_paddle/test_creation.py
@@ -99,6 +99,7 @@ def test_paddle_assign(
@handle_frontend_test(
fn_tree="paddle.clone",
dtype_and_x=helpers.dtype_and_values(available_dtypes=helpers.get_dtypes("valid")),
+ test_with_copy=st.just(True),
)
def test_paddle_clone(
*,
diff --git a/ivy_tests/test_ivy/test_frontends/test_paddle/test_fft.py b/ivy_tests/test_ivy/test_frontends/test_paddle/test_fft.py
index 269473214f479..fcff4153a6409 100644
--- a/ivy_tests/test_ivy/test_frontends/test_paddle/test_fft.py
+++ b/ivy_tests/test_ivy/test_frontends/test_paddle/test_fft.py
@@ -4,6 +4,9 @@
# local
import ivy_tests.test_ivy.helpers as helpers
from ivy_tests.test_ivy.helpers import handle_frontend_test
+from ivy_tests.test_ivy.test_functional.test_experimental.test_nn.test_layers import (
+ _x_and_ifftn,
+)
# Custom Hypothesis strategy for generating sequences of 2 integers
@@ -225,6 +228,32 @@ def test_paddle_ifft(
)
+# ifftn
+@handle_frontend_test(
+ fn_tree="paddle.fft.ifftn",
+ dtype_and_x=_x_and_ifftn(),
+)
+def test_paddle_ifftn(
+ dtype_and_x,
+ frontend,
+ backend_fw,
+ test_flags,
+ fn_tree,
+):
+ dtype, x, s, axes, norm = dtype_and_x
+ helpers.test_frontend_function(
+ input_dtypes=dtype,
+ frontend=frontend,
+ backend_to_test=backend_fw,
+ test_flags=test_flags,
+ fn_tree=fn_tree,
+ x=x,
+ s=s,
+ axes=axes,
+ norm=norm,
+ )
+
+
@handle_frontend_test(
fn_tree="paddle.fft.ifftshift",
dtype_x_axis=helpers.dtype_values_axis(
@@ -258,6 +287,50 @@ def test_paddle_ifftshift(
)
+@handle_frontend_test(
+ fn_tree="paddle.fft.ihfft2",
+ dtype_x_axis=helpers.dtype_values_axis(
+ available_dtypes=["float64", "float32", "int64", "int32"],
+ min_value=-10,
+ max_value=10,
+ min_num_dims=2,
+ max_num_dims=2,
+ shape=st.tuples(
+ st.integers(min_value=2, max_value=10),
+ st.integers(min_value=2, max_value=10),
+ ),
+ ),
+ s=st.one_of(
+ st.lists(st.integers(min_value=2, max_value=10), min_size=2, max_size=2),
+ ),
+ axes=st.just([-2, -1]),
+ norm=st.sampled_from(["backward", "ortho", "forward"]),
+)
+def test_paddle_ihfft2(
+ dtype_x_axis,
+ s,
+ axes,
+ norm,
+ frontend,
+ backend_fw,
+ test_flags,
+ fn_tree,
+):
+ input_dtypes, x, axis_ = dtype_x_axis
+
+ helpers.test_frontend_function(
+ input_dtypes=input_dtypes,
+ frontend=frontend,
+ backend_to_test=backend_fw,
+ test_flags=test_flags,
+ fn_tree=fn_tree,
+ x=x[0],
+ s=s,
+ axes=axes,
+ norm=norm,
+ )
+
+
@handle_frontend_test(
fn_tree="paddle.fft.irfft",
dtype_x_axis=helpers.dtype_values_axis(
diff --git a/ivy_tests/test_ivy/test_frontends/test_paddle/test_linalg.py b/ivy_tests/test_ivy/test_frontends/test_paddle/test_linalg.py
index 99e9e3feaef72..cc3923059d942 100644
--- a/ivy_tests/test_ivy/test_frontends/test_paddle/test_linalg.py
+++ b/ivy_tests/test_ivy/test_frontends/test_paddle/test_linalg.py
@@ -171,6 +171,17 @@ def _transpose_helper(draw):
return dtype, x, perm
+@st.composite
+def dims_and_offset(draw, shape):
+ shape_actual = draw(shape)
+ dim1 = draw(helpers.get_axis(shape=shape, force_int=True))
+ dim2 = draw(helpers.get_axis(shape=shape, force_int=True))
+ offset = draw(
+ st.integers(min_value=-shape_actual[dim1], max_value=shape_actual[dim1])
+ )
+ return dim1, dim2, offset
+
+
# Helpers #
# ------ #
@@ -688,6 +699,86 @@ def test_paddle_eigvalsh(
)
+# diagonal
+@handle_frontend_test(
+ fn_tree="paddle.diagonal",
+ dtype_and_values=helpers.dtype_and_values(
+ available_dtypes=helpers.get_dtypes("valid"),
+ shape=st.shared(helpers.get_shape(min_num_dims=2), key="shape"),
+ ),
+ axis_and_offset=dims_and_offset(
+ shape=st.shared(helpers.get_shape(min_num_dims=2), key="shape")
+ ),
+)
+def test_paddle_linalg_diagonal(
+ dtype_and_values,
+ axis_and_offset,
+ on_device,
+ fn_tree,
+ frontend,
+ test_flags,
+ backend_fw,
+):
+ input_dtype, value = dtype_and_values
+ axis1, axis2, offset = axis_and_offset
+ input = value[0]
+ num_dims = len(np.shape(input))
+ assume(axis1 != axis2)
+ if axis1 < 0:
+ assume(axis1 + num_dims != axis2)
+ if axis2 < 0:
+ assume(axis1 != axis2 + num_dims)
+ helpers.test_frontend_function(
+ input_dtypes=input_dtype,
+ on_device=on_device,
+ frontend=frontend,
+ backend_to_test=backend_fw,
+ test_flags=test_flags,
+ fn_tree=fn_tree,
+ x=input,
+ offset=offset,
+ axis1=axis1,
+ axis2=axis2,
+ )
+
+
+@handle_frontend_test(
+ fn_tree="paddle.lu_unpack",
+ dtype_x=_get_dtype_and_square_matrix(real_and_complex_only=True),
+ p=st.lists(st.floats(1, 5), max_size=5),
+ unpack_datas=st.booleans(),
+ unpack_pivots=st.booleans(),
+)
+def test_paddle_lu_unpack(
+ *,
+ dtype_x,
+ p,
+ unpack_datas,
+ unpack_pivots,
+ on_device,
+ fn_tree,
+ frontend,
+ test_flags,
+ backend_fw,
+):
+ dtype, x = dtype_x
+ x = np.array(x[0], dtype=dtype[0])
+ helpers.test_frontend_function(
+ input_dtypes=dtype,
+ backend_to_test=backend_fw,
+ frontend=frontend,
+ test_flags=test_flags,
+ fn_tree=fn_tree,
+ on_device=on_device,
+ lu_data=x,
+ lu_pivots=p,
+ unpack_datas=unpack_datas,
+ unpack_pivots=unpack_pivots,
+ rtol=1e-03,
+ atol=1e-03,
+ )
+
+
# matmul
@handle_frontend_test(
fn_tree="paddle.matmul",
diff --git a/ivy_tests/test_ivy/test_frontends/test_paddle/test_manipulation.py b/ivy_tests/test_ivy/test_frontends/test_paddle/test_manipulation.py
index 84e747400de97..413f799ef4d9d 100644
--- a/ivy_tests/test_ivy/test_frontends/test_paddle/test_manipulation.py
+++ b/ivy_tests/test_ivy/test_frontends/test_paddle/test_manipulation.py
@@ -436,6 +436,41 @@ def test_paddle_gather(
)
+# gather_nd
+@handle_frontend_test(
+ fn_tree="paddle.gather_nd",
+ dtype_x_index=helpers.array_indices_axis(
+ array_dtypes=helpers.get_dtypes("valid"),
+ indices_dtypes=["int64"],
+ min_num_dims=5,
+ max_num_dims=10,
+ min_dim_size=1,
+ max_dim_size=5,
+ indices_same_dims=False,
+ ),
+)
+def test_paddle_gather_nd(
+ *,
+ dtype_x_index,
+ on_device,
+ backend_fw,
+ fn_tree,
+ frontend,
+ test_flags,
+):
+ input_dtypes, x, index, _, _ = dtype_x_index
+ helpers.test_frontend_function(
+ input_dtypes=input_dtypes,
+ frontend=frontend,
+ backend_to_test=backend_fw,
+ test_flags=test_flags,
+ fn_tree=fn_tree,
+ on_device=on_device,
+ x=x,
+ index=index,
+ )
+
+
# repeat_interleave
@handle_frontend_test(
fn_tree="paddle.repeat_interleave",
@@ -721,6 +756,32 @@ def test_paddle_tile(
)
+@handle_frontend_test(
+ fn_tree="paddle.tolist",
+ dtype_and_x=helpers.dtype_and_values(available_dtypes=helpers.get_dtypes("valid")),
+ test_with_out=st.just(False),
+)
+def test_paddle_tolist(
+ *,
+ dtype_and_x,
+ on_device,
+ fn_tree,
+ backend_fw,
+ frontend,
+ test_flags,
+):
+ x_dtype, x = dtype_and_x
+ helpers.test_frontend_function(
+ input_dtypes=x_dtype,
+ backend_to_test=backend_fw,
+ frontend=frontend,
+ test_flags=test_flags,
+ fn_tree=fn_tree,
+ on_device=on_device,
+ x=x[0],
+ )
+
+
# unbind
@handle_frontend_test(
fn_tree="paddle.unbind",
diff --git a/ivy_tests/test_ivy/test_frontends/test_paddle/test_math.py b/ivy_tests/test_ivy/test_frontends/test_paddle/test_math.py
index 6d2023351d1d6..c4b40e6a715d9 100644
--- a/ivy_tests/test_ivy/test_frontends/test_paddle/test_math.py
+++ b/ivy_tests/test_ivy/test_frontends/test_paddle/test_math.py
@@ -1,6 +1,8 @@
# global
from hypothesis import strategies as st, assume
+import hypothesis.extra.numpy as nph
import numpy as np
+import sys
# local
import ivy_tests.test_ivy.helpers as helpers
@@ -15,6 +17,33 @@
# --------------- #
+@st.composite
+def _draw_paddle_diagonal(draw):
+ _dtype, _x = draw(
+ helpers.dtype_and_values(
+ available_dtypes=helpers.get_dtypes("numeric"),
+ min_num_dims=2,
+ max_num_dims=10,
+ min_dim_size=1,
+ max_dim_size=50,
+ )
+ )
+
+ offset = (draw(helpers.ints(min_value=-10, max_value=50)),)
+ axes = (
+ draw(
+ st.lists(
+ helpers.ints(min_value=-(len(_x)), max_value=len(_x)),
+ min_size=len(_x) + 1,
+ max_size=len(_x) + 1,
+ unique=True,
+ ).filter(lambda axes: axes[0] % 2 != axes[1] % 2)
+ ),
+ )
+
+ return _dtype, _x[0], offset[0], axes[0]
+
+
@st.composite
def _test_paddle_take_helper(draw):
mode = draw(st.sampled_from(["raise", "clip", "wrap"]))
@@ -201,6 +230,42 @@ def test_paddle_addmm(
)
+# all
+@handle_frontend_test(
+ fn_tree="paddle.all",
+ dtype_and_x=helpers.dtype_values_axis(
+ available_dtypes=["bool"],
+ valid_axis=True,
+ allow_neg_axes=True,
+ force_int_axis=True,
+ min_num_dims=1,
+ ),
+ keepdim=st.booleans(),
+)
+def test_paddle_all(
+ *,
+ dtype_and_x,
+ keepdim,
+ on_device,
+ fn_tree,
+ frontend,
+ test_flags,
+ backend_fw,
+):
+ input_dtype, x, axis = dtype_and_x
+ helpers.test_frontend_function(
+ input_dtypes=input_dtype,
+ frontend=frontend,
+ test_flags=test_flags,
+ fn_tree=fn_tree,
+ on_device=on_device,
+ backend_to_test=backend_fw,
+ x=x[0],
+ axis=axis,
+ keepdim=keepdim,
+ )
+
+
# amax
@handle_frontend_test(
fn_tree="paddle.amax",
@@ -474,6 +539,34 @@ def test_paddle_atanh(
)
+# broadcast_shape
+@handle_frontend_test(
+ fn_tree="paddle.broadcast_shape",
+ input_shapes_x=nph.mutually_broadcastable_shapes(
+ num_shapes=2, min_dims=1, max_dims=5, min_side=1, max_side=5
+ ),
+)
+def test_paddle_broadcast_shape(
+ *,
+ input_shapes_x,
+ on_device,
+ fn_tree,
+ frontend,
+ backend_fw,
+ test_flags,
+):
+ helpers.test_frontend_function(
+ input_dtypes=["int32", "int64"],
+ frontend=frontend,
+ backend_to_test=backend_fw,
+ test_flags=test_flags,
+ fn_tree=fn_tree,
+ on_device=on_device,
+ x_shape=input_shapes_x[0][0],
+ y_shape=input_shapes_x[0][1],
+ )
+
+
# ceil
@handle_frontend_test(
fn_tree="paddle.ceil",
@@ -653,6 +746,41 @@ def test_paddle_cumprod(
)
+@handle_frontend_test(
+ fn_tree="paddle.cumsum",
+ dtype_and_x=helpers.dtype_values_axis(
+ available_dtypes=helpers.get_dtypes("valid"),
+ valid_axis=True,
+ force_int_axis=True,
+ min_num_dims=1,
+ min_value=-5,
+ max_value=5,
+ ),
+)
+def test_paddle_cumsum(
+ *,
+ dtype_and_x,
+ on_device,
+ fn_tree,
+ frontend,
+ backend_fw,
+ test_flags,
+):
+ input_dtype, x, axis = dtype_and_x
+ helpers.test_frontend_function(
+ input_dtypes=input_dtype,
+ frontend=frontend,
+ backend_to_test=backend_fw,
+ test_flags=test_flags,
+ fn_tree=fn_tree,
+ on_device=on_device,
+ x=x[0],
+ axis=axis,
+ # rtol=1e-04,
+ # atol=1e-04,
+ )
+
+
# deg2rad
@handle_frontend_test(
fn_tree="paddle.deg2rad",
@@ -681,6 +809,32 @@ def test_paddle_deg2rad(
)
+# diagonal
+@handle_frontend_test(fn_tree="paddle.diagonal", data=_draw_paddle_diagonal())
+def test_paddle_diagonal(
+ *,
+ data,
+ on_device,
+ fn_tree,
+ frontend,
+ backend_fw,
+ test_flags,
+):
+ _dtype, _x, offset, axes = data
+ helpers.test_frontend_function(
+ input_dtypes=_dtype,
+ frontend=frontend,
+ backend_to_test=backend_fw,
+ test_flags=test_flags,
+ fn_tree=fn_tree,
+ on_device=on_device,
+ x=_x,
+ offset=offset,
+ axis1=axes[0],
+ axis2=axes[1],
+ )
+
+
# diff
@handle_frontend_test(
fn_tree="paddle.diff",
@@ -1170,6 +1324,45 @@ def test_paddle_inner(
)
+# inverse
+@handle_frontend_test(
+ fn_tree="paddle.inverse",
+ dtype_and_x=helpers.dtype_and_values(
+ available_dtypes=helpers.get_dtypes("float"),
+ min_value=-100.0,
+ max_value=100.0,
+ shape=helpers.ints(min_value=2, max_value=10).map(lambda x: tuple([x, x])),
+ ).filter(
+ lambda x: "float16" not in x[0]
+ and "bfloat16" not in x[0]
+ and np.linalg.det(np.asarray(x[1][0])) != 0
+ and np.linalg.cond(x[1][0]) < 1 / sys.float_info.epsilon
+ ),
+ test_with_out=st.just(False),
+)
+def test_paddle_inverse(
+ *,
+ dtype_and_x,
+ on_device,
+ fn_tree,
+ frontend,
+ test_flags,
+ backend_fw,
+):
+ dtype, x = dtype_and_x
+ helpers.test_frontend_function(
+ input_dtypes=dtype,
+ backend_to_test=backend_fw,
+ rtol=1e-01,
+ atol=1e-01,
+ frontend=frontend,
+ test_flags=test_flags,
+ fn_tree=fn_tree,
+ on_device=on_device,
+ x=x[0],
+ )
+
+
# isfinite
@handle_frontend_test(
fn_tree="paddle.isfinite",
diff --git a/ivy_tests/test_ivy/test_frontends/test_paddle/test_nn/test_functional/test_activation.py b/ivy_tests/test_ivy/test_frontends/test_paddle/test_nn/test_functional/test_activation.py
index 48c3ef820ea6b..a95ae21b3bf0f 100644
--- a/ivy_tests/test_ivy/test_frontends/test_paddle/test_nn/test_functional/test_activation.py
+++ b/ivy_tests/test_ivy/test_frontends/test_paddle/test_nn/test_functional/test_activation.py
@@ -35,9 +35,9 @@ def _generate_prelu_arrays(draw):
@handle_frontend_test(
fn_tree="paddle.nn.functional.celu",
dtype_and_x=helpers.dtype_and_values(
- available_dtypes=helpers.get_dtypes("valid"),
+ available_dtypes=helpers.get_dtypes("float"),
),
- alpha=helpers.ints(min_value=1, max_value=10),
+ alpha=helpers.floats(min_value=0.1, max_value=1.0),
)
def test_paddle_celu(
*,
diff --git a/ivy_tests/test_ivy/test_frontends/test_paddle/test_nn/test_functional/test_common.py b/ivy_tests/test_ivy/test_frontends/test_paddle/test_nn/test_functional/test_common.py
index 14dedc3b6db4a..f020caefc0d57 100644
--- a/ivy_tests/test_ivy/test_frontends/test_paddle/test_nn/test_functional/test_common.py
+++ b/ivy_tests/test_ivy/test_frontends/test_paddle/test_nn/test_functional/test_common.py
@@ -34,7 +34,7 @@ def _interp_args(draw, mode=None, mode_list=None):
"trilinear",
"nearest-exact",
"tf_area",
- "bicubic_tensorflow",
+ "tf_bicubic",
"lanczos3",
"lanczos5",
"mitchellcubic",
@@ -46,7 +46,7 @@ def _interp_args(draw, mode=None, mode_list=None):
"bilinear",
"trilinear",
"nearest-exact",
- "bicubic_tensorflow",
+ "tf_bicubic",
"lanczos3",
"lanczos5",
]
@@ -69,7 +69,7 @@ def _interp_args(draw, mode=None, mode_list=None):
"nearest-exact",
"area",
"tf_area",
- "bicubic_tensorflow",
+ "tf_bicubic",
"lanczos3",
"lanczos5",
"mitchellcubic",
@@ -86,7 +86,7 @@ def _interp_args(draw, mode=None, mode_list=None):
num_dims = 3
elif mode in [
"bilinear",
- "bicubic_tensorflow",
+ "tf_bicubic",
"bicubic",
"mitchellcubic",
"gaussian",
@@ -217,37 +217,6 @@ def paddle_unfold_handler(draw, dtype):
# ------------ #
-# linear
-@handle_frontend_test(
- fn_tree="paddle.nn.functional.common.linear",
- dtype_x_weight_bias=_x_and_linear(
- dtypes=helpers.get_dtypes("valid", full=False),
- ),
-)
-def test_linear(
- *,
- dtype_x_weight_bias,
- on_device,
- fn_tree,
- backend_fw,
- frontend,
- test_flags,
-):
- dtype, x, weight, bias = dtype_x_weight_bias
- weight = ivy.swapaxes(weight, -1, -2)
- helpers.test_frontend_function(
- input_dtypes=dtype,
- frontend=frontend,
- backend_to_test=backend_fw,
- test_flags=test_flags,
- fn_tree=fn_tree,
- on_device=on_device,
- x=x,
- weight=weight,
- bias=bias,
- )
-
-
# Cosine Similarity
@handle_frontend_test(
fn_tree="paddle.nn.functional.common.cosine_similarity",
@@ -458,32 +427,34 @@ def test_paddle_interpolate(
)
+# linear
@handle_frontend_test(
- fn_tree="paddle.nn.functional.common.zeropad2d",
- d_type_and_x_paddings=_zero2pad(),
- dataformat=st.sampled_from(["NCHW", "NHWC"]),
+ fn_tree="paddle.nn.functional.common.linear",
+ dtype_x_weight_bias=_x_and_linear(
+ dtypes=helpers.get_dtypes("valid", full=False),
+ ),
)
-def test_paddle_zeropad2d(
+def test_paddle_linear(
*,
- d_type_and_x_paddings,
+ dtype_x_weight_bias,
on_device,
fn_tree,
+ backend_fw,
frontend,
test_flags,
- backend_fw,
- dataformat,
):
- dtype, x, padding = d_type_and_x_paddings
+ dtype, x, weight, bias = dtype_x_weight_bias
+ weight = ivy.swapaxes(weight, -1, -2)
helpers.test_frontend_function(
input_dtypes=dtype,
- backend_to_test=backend_fw,
frontend=frontend,
+ backend_to_test=backend_fw,
test_flags=test_flags,
fn_tree=fn_tree,
on_device=on_device,
- x=x[0],
- padding=padding,
- data_format=dataformat,
+ x=x,
+ weight=weight,
+ bias=bias,
)
@@ -491,7 +462,7 @@ def test_paddle_zeropad2d(
fn_tree="paddle.nn.functional.common.unfold",
dtype_inputs=paddle_unfold_handler(dtype=helpers.get_dtypes("valid", full=False)),
)
-def test_unfold(
+def test_paddle_unfold(
*,
dtype_inputs,
on_device,
@@ -514,3 +485,32 @@ def test_unfold(
paddings=paddings,
dilations=dilations,
)
+
+
+@handle_frontend_test(
+ fn_tree="paddle.nn.functional.common.zeropad2d",
+ d_type_and_x_paddings=_zero2pad(),
+ dataformat=st.sampled_from(["NCHW", "NHWC"]),
+)
+def test_paddle_zeropad2d(
+ *,
+ d_type_and_x_paddings,
+ on_device,
+ fn_tree,
+ frontend,
+ test_flags,
+ backend_fw,
+ dataformat,
+):
+ dtype, x, padding = d_type_and_x_paddings
+ helpers.test_frontend_function(
+ input_dtypes=dtype,
+ backend_to_test=backend_fw,
+ frontend=frontend,
+ test_flags=test_flags,
+ fn_tree=fn_tree,
+ on_device=on_device,
+ x=x[0],
+ padding=padding,
+ data_format=dataformat,
+ )
diff --git a/ivy_tests/test_ivy/test_frontends/test_paddle/test_nn/test_functional/test_pooling.py b/ivy_tests/test_ivy/test_frontends/test_paddle/test_nn/test_functional/test_pooling.py
index 881592b4e143a..129f4f502591c 100644
--- a/ivy_tests/test_ivy/test_frontends/test_paddle/test_nn/test_functional/test_pooling.py
+++ b/ivy_tests/test_ivy/test_frontends/test_paddle/test_nn/test_functional/test_pooling.py
@@ -276,6 +276,60 @@ def test_paddle_avg_pool2d(
)
+# max_pool2d
+@handle_frontend_test(
+ fn_tree="paddle.nn.functional.pooling.max_pool2d",
+ dtype_x_k_s=helpers.arrays_for_pooling(
+ min_dims=4, max_dims=4, min_side=2, max_side=4
+ ),
+ ceil_mode=st.sampled_from([True]),
+ data_format=st.sampled_from(["NCHW", "NHWC"]),
+)
+def test_paddle_max_pool2d(
+ dtype_x_k_s,
+ ceil_mode,
+ data_format,
+ *,
+ test_flags,
+ backend_fw,
+ frontend,
+ fn_tree,
+ on_device,
+):
+ input_dtype, x, kernel, stride, padding = dtype_x_k_s
+
+ if data_format == "NCHW":
+ x[0] = x[0].reshape(
+ (x[0].shape[0], x[0].shape[3], x[0].shape[1], x[0].shape[2])
+ )
+ if len(stride) == 1:
+ stride = (stride[0], stride[0])
+ if padding == "SAME":
+ padding = test_pooling_functions.calculate_same_padding(
+ kernel, stride, x[0].shape[2:]
+ )
+ else:
+ padding = (0, 0)
+
+ if padding == "VALID" and ceil_mode:
+ ceil_mode = False
+
+ helpers.test_frontend_function(
+ input_dtypes=input_dtype,
+ test_flags=test_flags,
+ backend_to_test=backend_fw,
+ frontend=frontend,
+ fn_tree=fn_tree,
+ on_device=on_device,
+ x=x[0],
+ kernel_size=kernel,
+ stride=stride,
+ padding=padding,
+ ceil_mode=ceil_mode,
+ data_format=data_format,
+ )
+
+
# max_unpool1d
@handle_frontend_test(
fn_tree="paddle.nn.functional.max_unpool1d",
diff --git a/ivy_tests/test_ivy/test_frontends/test_paddle/test_random.py b/ivy_tests/test_ivy/test_frontends/test_paddle/test_random.py
index a9c2e27164924..693be50047aad 100644
--- a/ivy_tests/test_ivy/test_frontends/test_paddle/test_random.py
+++ b/ivy_tests/test_ivy/test_frontends/test_paddle/test_random.py
@@ -7,6 +7,64 @@
from ivy_tests.test_ivy.helpers import handle_frontend_test
+# --- Helpers --- #
+# --------------- #
+
+
+@st.composite
+def _multinomial_helper(draw):
+ input_dtype_and_x = draw(
+ helpers.dtype_and_values(
+ available_dtypes=helpers.get_dtypes("valid"),
+ shape=helpers.get_shape(min_num_dims=1, max_num_dims=2, min_dim_size=2),
+ )
+ )
+ num_samples = draw(st.integers(min_value=1, max_value=10))
+ if num_samples > 2:
+ replacement = True
+ else:
+ replacement = draw(st.booleans())
+
+ input_dtype, x = input_dtype_and_x
+
+ total = sum(x)
+ x = [arr / total for arr in x]
+
+ return input_dtype, x, num_samples, replacement
+
+
+# --- Main --- #
+# ------------ #
+
+
+# multinomial
+@handle_frontend_test(
+ fn_tree="paddle.tensor.random.multinomial",
+ input_dtype_and_x=_multinomial_helper(),
+)
+def test_paddle_multinomial(
+ input_dtype_and_x,
+ test_flags,
+ frontend,
+ backend_fw,
+ fn_tree,
+ on_device,
+):
+ input_dtype, x, num_samples, replacement = input_dtype_and_x
+ helpers.test_frontend_function(
+ input_dtypes=input_dtype,
+ test_flags=test_flags,
+ frontend=frontend,
+ backend_to_test=backend_fw,
+ fn_tree=fn_tree,
+ on_device=on_device,
+ test_values=False,
+ x=x[0],
+ num_samples=num_samples,
+ replacement=replacement,
+ )
+
+
@handle_frontend_test(
fn_tree="paddle.normal",
input_dtypes=st.sampled_from([["float32"], ["float64"]]),
diff --git a/ivy_tests/test_ivy/test_frontends/test_paddle/test_search.py b/ivy_tests/test_ivy/test_frontends/test_paddle/test_search.py
index 44301295765cc..0df93fb0f3095 100644
--- a/ivy_tests/test_ivy/test_frontends/test_paddle/test_search.py
+++ b/ivy_tests/test_ivy/test_frontends/test_paddle/test_search.py
@@ -149,6 +149,37 @@ def test_paddle_argsort(
)
+@handle_frontend_test(
+ fn_tree="paddle.index_sample",
+ array_indices_axis=helpers.array_indices_axis(
+ array_dtypes=helpers.get_dtypes("valid"),
+ indices_dtypes=helpers.get_dtypes("integer"),
+ min_num_dims=2,
+ max_num_dims=2,
+ disable_random_axis=True,
+ ),
+)
+def test_paddle_index_sample(
+ *,
+ array_indices_axis,
+ frontend,
+ test_flags,
+ fn_tree,
+ backend_fw,
+):
+ dtype, x, index = array_indices_axis
+ if index.ndim == 2 and index.shape[0] == x.shape[0]:
+ helpers.test_frontend_function(
+ input_dtypes=dtype,
+ backend_to_test=backend_fw,
+ frontend=frontend,
+ test_flags=test_flags,
+ fn_tree=fn_tree,
+ x=x,
+ index=index,
+ )
+
+
# kthvalue
@handle_frontend_test(
fn_tree="paddle.kthvalue",
diff --git a/ivy_tests/test_ivy/test_frontends/test_paddle/test_stat.py b/ivy_tests/test_ivy/test_frontends/test_paddle/test_stat.py
index a7b41e0baf820..f8ccfc5b7ac2c 100644
--- a/ivy_tests/test_ivy/test_frontends/test_paddle/test_stat.py
+++ b/ivy_tests/test_ivy/test_frontends/test_paddle/test_stat.py
@@ -86,6 +86,7 @@ def test_paddle_nanmedian(
dtype_x_and_axis,
keepdim,
frontend,
+ backend_fw,
test_flags,
fn_tree,
):
@@ -93,6 +94,7 @@ def test_paddle_nanmedian(
helpers.test_frontend_function(
input_dtypes=input_dtypes,
frontend=frontend,
+ backend_to_test=backend_fw,
test_flags=test_flags,
fn_tree=fn_tree,
x=x[0],
diff --git a/ivy_tests/test_ivy/test_frontends/test_paddle/test_tensor/test_tensor.py b/ivy_tests/test_ivy/test_frontends/test_paddle/test_tensor/test_tensor.py
index 362f7f8df059a..ff869ce9ca229 100644
--- a/ivy_tests/test_ivy/test_frontends/test_paddle/test_tensor/test_tensor.py
+++ b/ivy_tests/test_ivy/test_frontends/test_paddle/test_tensor/test_tensor.py
@@ -16,6 +16,12 @@
from ivy_tests.test_ivy.test_functional.test_core.test_statistical import (
_statistical_dtype_values,
)
+from ivy_tests.test_ivy.test_frontends.test_torch.test_blas_and_lapack_ops import (
+ _get_dtype_and_3dbatch_matrices,
+)
+from ivy_tests.test_ivy.test_frontends.test_paddle.test_manipulation import (
+ _tile_helper,
+)
CLASS_TREE = "ivy.functional.frontends.paddle.Tensor"
@@ -148,6 +154,29 @@ def _get_dtype_and_square_matrix(draw):
return dtype, mat
+# bmm helper function
+@st.composite
+def _get_dtype_and_values_bmm(draw):
+ # arrays x and y of sizes (b, m, k) and (b, k, n) respectively
+ b = draw(helpers.ints(min_value=1, max_value=10))
+ k = draw(helpers.ints(min_value=1, max_value=10))
+ m = draw(helpers.ints(min_value=1, max_value=10))
+ n = draw(helpers.ints(min_value=1, max_value=10))
+ dtype = draw(helpers.get_dtypes("float", index=1, full=False))
+ x = draw(
+ helpers.array_values(
+ dtype=dtype[0], shape=(b, m, k), min_value=-10, max_value=10
+ )
+ )
+ y = draw(
+ helpers.array_values(
+ dtype=dtype[0], shape=(b, k, n), min_value=-10, max_value=10
+ )
+ )
+ return dtype, x, y
+
+
+# lerp helper function
@st.composite
def _get_dtype_and_values_for_lerp(draw):
is_tensor = draw(st.booleans())
@@ -196,10 +225,88 @@ def _reshape_helper(draw):
return dtypes, x, reshape_shape
+# diagonal
+@st.composite
+def dims_and_offset(draw, shape):
+ shape_actual = draw(shape)
+ dim1 = draw(helpers.get_axis(shape=shape, force_int=True))
+ dim2 = draw(helpers.get_axis(shape=shape, force_int=True))
+ offset = draw(
+ st.integers(min_value=-shape_actual[dim1], max_value=shape_actual[dim1])
+ )
+ return dim1, dim2, offset
+
+
+# expand helper function
+@st.composite
+def dtypes_x_shape(draw):
+ dtypes, x = draw(
+ helpers.dtype_and_values(
+ min_dim_size=1,
+ min_num_dims=1,
+ available_dtypes=["float32"],
+ shape=st.shared(
+ helpers.get_shape(
+ min_num_dims=1,
+ max_num_dims=6,
+ ),
+ key="shape",
+ ),
+ )
+ )
+ shape = draw(
+ st.shared(
+ helpers.get_shape(
+ min_num_dims=1,
+ max_num_dims=6,
+ ),
+ key="shape",
+ )
+ )
+ return dtypes, x, shape
+
+
# --- Main --- #
# ------------ #
+# __add__
+@handle_frontend_method(
+ class_tree=CLASS_TREE,
+ init_tree="paddle.to_tensor",
+ method_name="__add__",
+ dtype_and_x=helpers.dtype_and_values(
+ available_dtypes=helpers.get_dtypes("valid"), num_arrays=2, shared_dtype=True
+ ),
+)
+def test_paddle___add__(
+ dtype_and_x,
+ frontend_method_data,
+ init_flags,
+ method_flags,
+ frontend,
+ on_device,
+ backend_fw,
+):
+ input_dtype, x = dtype_and_x
+ helpers.test_frontend_method(
+ init_input_dtypes=input_dtype,
+ backend_to_test=backend_fw,
+ init_all_as_kwargs_np={
+ "data": x[0],
+ },
+ method_input_dtypes=input_dtype,
+ method_all_as_kwargs_np={
+ "y": x[1],
+ },
+ frontend_method_data=frontend_method_data,
+ init_flags=init_flags,
+ method_flags=method_flags,
+ frontend=frontend,
+ on_device=on_device,
+ )
+
+
# __setitem__
@handle_frontend_method(
class_tree=CLASS_TREE,
@@ -304,30 +411,32 @@ def test_paddle__reshape(
)
-# is_floating_point
+# abs
@handle_frontend_method(
class_tree=CLASS_TREE,
init_tree="paddle.to_tensor",
- method_name="is_floating_point",
+ method_name="abs",
dtype_and_x=helpers.dtype_and_values(
- available_dtypes=["int16", "int32", "int64", "float32", "float64"],
+ available_dtypes=helpers.get_dtypes("float"),
),
)
-def test_paddle_is_floating_point(
+def test_paddle_abs(
dtype_and_x,
frontend_method_data,
init_flags,
method_flags,
frontend,
- backend_fw,
on_device,
+ backend_fw,
):
input_dtype, x = dtype_and_x
helpers.test_frontend_method(
init_input_dtypes=input_dtype,
- init_all_as_kwargs_np={"data": x[0]},
- method_input_dtypes=input_dtype,
backend_to_test=backend_fw,
+ init_all_as_kwargs_np={
+ "data": x[0],
+ },
+ method_input_dtypes=input_dtype,
method_all_as_kwargs_np={},
frontend_method_data=frontend_method_data,
init_flags=init_flags,
@@ -337,16 +446,16 @@ def test_paddle_is_floating_point(
)
-# __add__
+# acosh
@handle_frontend_method(
class_tree=CLASS_TREE,
init_tree="paddle.to_tensor",
- method_name="__add__",
+ method_name="acosh",
dtype_and_x=helpers.dtype_and_values(
- available_dtypes=helpers.get_dtypes("valid"), num_arrays=2, shared_dtype=True
+ available_dtypes=helpers.get_dtypes("float"),
),
)
-def test_paddle_tensor___add__(
+def test_paddle_acosh(
dtype_and_x,
frontend_method_data,
init_flags,
@@ -363,9 +472,7 @@ def test_paddle_tensor___add__(
"data": x[0],
},
method_input_dtypes=input_dtype,
- method_all_as_kwargs_np={
- "y": x[1],
- },
+ method_all_as_kwargs_np={},
frontend_method_data=frontend_method_data,
init_flags=init_flags,
method_flags=method_flags,
@@ -374,16 +481,17 @@ def test_paddle_tensor___add__(
)
-# abs
+# add_
@handle_frontend_method(
class_tree=CLASS_TREE,
init_tree="paddle.to_tensor",
- method_name="abs",
+ method_name="add_",
dtype_and_x=helpers.dtype_and_values(
- available_dtypes=helpers.get_dtypes("float"),
+ available_dtypes=helpers.get_dtypes("valid"), num_arrays=2, shared_dtype=True
),
+ test_inplace=st.just(True),
)
-def test_paddle_tensor_abs(
+def test_paddle_add_(
dtype_and_x,
frontend_method_data,
init_flags,
@@ -400,7 +508,7 @@ def test_paddle_tensor_abs(
"data": x[0],
},
method_input_dtypes=input_dtype,
- method_all_as_kwargs_np={},
+ method_all_as_kwargs_np={"y": x[1]},
frontend_method_data=frontend_method_data,
init_flags=init_flags,
method_flags=method_flags,
@@ -409,16 +517,17 @@ def test_paddle_tensor_abs(
)
-# acosh
@handle_frontend_method(
class_tree=CLASS_TREE,
init_tree="paddle.to_tensor",
- method_name="acosh",
+ method_name="add_n",
dtype_and_x=helpers.dtype_and_values(
available_dtypes=helpers.get_dtypes("float"),
+ num_arrays=helpers.ints(min_value=1, max_value=5),
+ shared_dtype=True,
),
)
-def test_paddle_tensor_acosh(
+def test_paddle_add_n(
dtype_and_x,
frontend_method_data,
init_flags,
@@ -431,11 +540,9 @@ def test_paddle_tensor_acosh(
helpers.test_frontend_method(
init_input_dtypes=input_dtype,
backend_to_test=backend_fw,
- init_all_as_kwargs_np={
- "data": x[0],
- },
+ init_all_as_kwargs_np={"inputs": x},
method_input_dtypes=input_dtype,
- method_all_as_kwargs_np={},
+ method_all_as_kwargs_np={"inputs": x},
frontend_method_data=frontend_method_data,
init_flags=init_flags,
method_flags=method_flags,
@@ -444,18 +551,32 @@ def test_paddle_tensor_acosh(
)
-# add_
+# addmm
@handle_frontend_method(
class_tree=CLASS_TREE,
init_tree="paddle.to_tensor",
- method_name="add_",
- dtype_and_x=helpers.dtype_and_values(
- available_dtypes=helpers.get_dtypes("valid"), num_arrays=2, shared_dtype=True
+ method_name="addmm",
+ dtype_input_xy=_get_dtype_and_3dbatch_matrices(with_input=True, input_3d=True),
+ beta=st.floats(
+ min_value=-5,
+ max_value=5,
+ allow_nan=False,
+ allow_subnormal=False,
+ allow_infinity=False,
+ ),
+ alpha=st.floats(
+ min_value=-5,
+ max_value=5,
+ allow_nan=False,
+ allow_subnormal=False,
+ allow_infinity=False,
),
- test_inplace=st.just(True),
)
-def test_paddle_tensor_add_(
- dtype_and_x,
+def test_paddle_addmm(
+ *,
+ dtype_input_xy,
+ beta,
+ alpha,
frontend_method_data,
init_flags,
method_flags,
@@ -463,15 +584,15 @@ def test_paddle_tensor_add_(
on_device,
backend_fw,
):
- input_dtype, x = dtype_and_x
+ input_dtype, input, x, y = dtype_input_xy
helpers.test_frontend_method(
init_input_dtypes=input_dtype,
backend_to_test=backend_fw,
init_all_as_kwargs_np={
- "data": x[0],
+ "data": input[0],
},
method_input_dtypes=input_dtype,
- method_all_as_kwargs_np={"y": x[1]},
+ method_all_as_kwargs_np={"x": x[0], "y": y[0], "beta": beta, "alpha": alpha},
frontend_method_data=frontend_method_data,
init_flags=init_flags,
method_flags=method_flags,
@@ -494,7 +615,7 @@ def test_paddle_tensor_add_(
),
keep_dims=st.booleans(),
)
-def test_paddle_tensor_all(
+def test_paddle_all(
dtype_x_axis,
keep_dims,
frontend_method_data,
@@ -538,7 +659,7 @@ def test_paddle_tensor_all(
# atol=1e-08,
# equal_nan=st.booleans(),
)
-def test_paddle_tensor_allclose(
+def test_paddle_allclose(
dtype_and_x,
# rtol,
# atol,
@@ -580,7 +701,7 @@ def test_paddle_tensor_allclose(
available_dtypes=["float64", "complex64", "complex128"],
),
)
-def test_paddle_tensor_angle(
+def test_paddle_angle(
dtype_and_x,
frontend_method_data,
init_flags,
@@ -620,7 +741,7 @@ def test_paddle_tensor_angle(
),
keep_dims=st.booleans(),
)
-def test_paddle_tensor_any(
+def test_paddle_any(
dtype_x_axis,
keep_dims,
frontend_method_data,
@@ -664,7 +785,7 @@ def test_paddle_tensor_any(
),
keep_dims=st.booleans(),
)
-def test_paddle_tensor_argmax(
+def test_paddle_argmax(
dtype_x_axis,
keep_dims,
frontend_method_data,
@@ -708,7 +829,7 @@ def test_paddle_tensor_argmax(
),
keep_dims=st.booleans(),
)
-def test_paddle_tensor_argmin(
+def test_paddle_argmin(
dtype_x_axis,
keep_dims,
on_device,
@@ -752,7 +873,7 @@ def test_paddle_tensor_argmin(
),
descending=st.booleans(),
)
-def test_paddle_tensor_argsort(
+def test_paddle_argsort(
dtype_x_axis,
descending,
frontend_method_data,
@@ -789,7 +910,7 @@ def test_paddle_tensor_argsort(
method_name="as_complex",
dtypes_and_x=_get_as_complex_inputs_(),
)
-def test_paddle_tensor_as_complex(
+def test_paddle_as_complex(
dtypes_and_x,
frontend_method_data,
init_flags,
@@ -823,7 +944,7 @@ def test_paddle_tensor_as_complex(
num_arrays=1,
),
)
-def test_paddle_tensor_as_real(
+def test_paddle_as_real(
dtype_and_x,
frontend_method_data,
init_flags,
@@ -858,7 +979,7 @@ def test_paddle_tensor_as_real(
available_dtypes=helpers.get_dtypes("float"),
),
)
-def test_paddle_tensor_asin(
+def test_paddle_asin(
dtype_and_x,
frontend_method_data,
init_flags,
@@ -893,7 +1014,7 @@ def test_paddle_tensor_asin(
available_dtypes=helpers.get_dtypes("float"),
),
)
-def test_paddle_tensor_asinh(
+def test_paddle_asinh(
dtype_and_x,
frontend_method_data,
init_flags,
@@ -929,7 +1050,7 @@ def test_paddle_tensor_asinh(
),
dtype=st.one_of(helpers.get_dtypes("valid")),
)
-def test_paddle_tensor_astype(
+def test_paddle_astype(
dtype_and_x,
dtype,
frontend_method_data,
@@ -969,7 +1090,7 @@ def test_paddle_tensor_astype(
available_dtypes=helpers.get_dtypes("float"),
),
)
-def test_paddle_tensor_atan(
+def test_paddle_atan(
dtype_and_x,
frontend_method_data,
init_flags,
@@ -1004,7 +1125,7 @@ def test_paddle_tensor_atan(
available_dtypes=helpers.get_dtypes("valid"), num_arrays=2, shared_dtype=True
),
)
-def test_paddle_tensor_bitwise_and(
+def test_paddle_bitwise_and(
dtypes_and_x,
frontend_method_data,
init_flags,
@@ -1037,7 +1158,7 @@ def test_paddle_tensor_bitwise_and(
available_dtypes=helpers.get_dtypes("valid"),
),
)
-def test_paddle_tensor_bitwise_not(
+def test_paddle_bitwise_not(
dtype_and_x,
frontend_method_data,
init_flags,
@@ -1071,7 +1192,7 @@ def test_paddle_tensor_bitwise_not(
available_dtypes=helpers.get_dtypes("valid"), num_arrays=2, shared_dtype=True
),
)
-def test_paddle_tensor_bitwise_or(
+def test_paddle_bitwise_or(
dtypes_and_x,
frontend_method_data,
init_flags,
@@ -1104,7 +1225,7 @@ def test_paddle_tensor_bitwise_or(
available_dtypes=helpers.get_dtypes("valid"), num_arrays=2, shared_dtype=True
),
)
-def test_paddle_tensor_bitwise_xor(
+def test_paddle_bitwise_xor(
dtypes_and_x,
frontend_method_data,
init_flags,
@@ -1128,6 +1249,37 @@ def test_paddle_tensor_bitwise_xor(
)
+# bmm
+@handle_frontend_method(
+ class_tree=CLASS_TREE,
+ init_tree="paddle.to_tensor",
+ method_name="bmm",
+ dtype_and_x=_get_dtype_and_values_bmm(),
+)
+def test_paddle_bmm(
+ dtype_and_x,
+ frontend_method_data,
+ init_flags,
+ method_flags,
+ frontend,
+ on_device,
+ backend_fw,
+):
+ input_dtype, x, y = dtype_and_x
+ helpers.test_frontend_method(
+ init_input_dtypes=input_dtype,
+ backend_to_test=backend_fw,
+ init_all_as_kwargs_np={"data": x},
+ method_input_dtypes=input_dtype,
+ method_all_as_kwargs_np={"y": y},
+ frontend_method_data=frontend_method_data,
+ init_flags=init_flags,
+ method_flags=method_flags,
+ frontend=frontend,
+ on_device=on_device,
+ )
+
+
# cast
@handle_frontend_method(
class_tree=CLASS_TREE,
@@ -1138,7 +1290,7 @@ def test_paddle_tensor_bitwise_xor(
),
dtype=helpers.get_dtypes("valid", full=False),
)
-def test_paddle_tensor_cast(
+def test_paddle_cast(
dtype_and_x,
dtype,
frontend_method_data,
@@ -1178,7 +1330,7 @@ def test_paddle_tensor_cast(
available_dtypes=helpers.get_dtypes("float"),
),
)
-def test_paddle_tensor_ceil(
+def test_paddle_ceil(
dtype_and_x,
frontend_method_data,
init_flags,
@@ -1214,7 +1366,7 @@ def test_paddle_tensor_ceil(
),
test_inplace=st.just(True),
)
-def test_paddle_tensor_ceil_(
+def test_paddle_ceil_(
dtype_and_x,
frontend_method_data,
init_flags,
@@ -1248,7 +1400,7 @@ def test_paddle_tensor_ceil_(
dtype_and_x=_get_dtype_and_square_matrix(),
upper=st.booleans(),
)
-def test_paddle_tensor_cholesky(
+def test_paddle_cholesky(
dtype_and_x,
upper,
frontend_method_data,
@@ -1283,7 +1435,7 @@ def test_paddle_tensor_cholesky(
method_name="clip",
input_and_ranges=_get_clip_inputs(),
)
-def test_paddle_tensor_clip(
+def test_paddle_clip(
input_and_ranges,
frontend,
frontend_method_data,
@@ -1317,7 +1469,7 @@ def test_paddle_tensor_clip(
input_and_ranges=_get_clip_inputs_(),
test_inplace=st.just(True),
)
-def test_paddle_tensor_clip_(
+def test_paddle_clip_(
input_and_ranges,
frontend,
frontend_method_data,
@@ -1357,7 +1509,7 @@ def test_paddle_tensor_clip_(
dtype_and_x=_get_dtype_and_matrix_non_singular(dtypes=["float32", "float64"]),
p=st.sampled_from([None, "fro", "nuc", np.inf, -np.inf, 1, -1, 2, -2]),
)
-def test_paddle_tensor_cond(
+def test_paddle_cond(
dtype_and_x,
p,
frontend_method_data,
@@ -1393,7 +1545,7 @@ def test_paddle_tensor_cond(
available_dtypes=helpers.get_dtypes("numeric"),
),
)
-def test_paddle_tensor_conj(
+def test_paddle_conj(
dtype_and_x,
frontend_method_data,
init_flags,
@@ -1428,7 +1580,7 @@ def test_paddle_tensor_conj(
available_dtypes=helpers.get_dtypes("float"),
),
)
-def test_paddle_tensor_cos(
+def test_paddle_cos(
dtype_and_x,
frontend_method_data,
init_flags,
@@ -1463,7 +1615,7 @@ def test_paddle_tensor_cos(
available_dtypes=helpers.get_dtypes("float"),
),
)
-def test_paddle_tensor_cosh(
+def test_paddle_cosh(
dtype_and_x,
frontend_method_data,
init_flags,
@@ -1502,7 +1654,7 @@ def test_paddle_tensor_cosh(
max_value=5,
),
)
-def test_paddle_tensor_cumprod(
+def test_paddle_cumprod(
dtype_x_axis,
frontend_method_data,
init_flags,
@@ -1541,7 +1693,7 @@ def test_paddle_tensor_cumprod(
max_value=5,
),
)
-def test_paddle_tensor_cumsum(
+def test_paddle_cumsum(
dtype_x_axis,
frontend_method_data,
init_flags,
@@ -1576,7 +1728,7 @@ def test_paddle_tensor_cumsum(
available_dtypes=helpers.get_dtypes("float"),
),
)
-def test_paddle_tensor_deg2rad(
+def test_paddle_deg2rad(
dtype_and_x,
frontend_method_data,
init_flags,
@@ -1611,7 +1763,7 @@ def test_paddle_tensor_deg2rad(
available_dtypes=helpers.get_dtypes("valid", prune_function=False)
).filter(lambda x: "bfloat16" not in x[0]),
)
-def test_paddle_tensor_device(
+def test_paddle_device(
dtype_x,
):
_, data = dtype_x
@@ -1622,6 +1774,56 @@ def test_paddle_tensor_device(
)
+# diagonal
+@handle_frontend_method(
+ class_tree=CLASS_TREE,
+ init_tree="paddle.to_tensor",
+ method_name="diagonal",
+ dtype_and_values=helpers.dtype_and_values(
+ available_dtypes=helpers.get_dtypes("valid"),
+ shape=st.shared(helpers.get_shape(min_num_dims=2), key="shape"),
+ ),
+ dims_and_offset=dims_and_offset(
+ shape=st.shared(helpers.get_shape(min_num_dims=2), key="shape")
+ ),
+)
+def test_paddle_diagonal(
+ dtype_and_values,
+ dims_and_offset,
+ frontend,
+ frontend_method_data,
+ backend_fw,
+ init_flags,
+ method_flags,
+ on_device,
+):
+ input_dtype, value = dtype_and_values
+ dim1, dim2, offset = dims_and_offset
+ input = value[0]
+ num_dims = len(np.shape(input))
+ assume(dim1 != dim2)
+ if dim1 < 0:
+ assume(dim1 + num_dims != dim2)
+ if dim2 < 0:
+ assume(dim1 != dim2 + num_dims)
+ helpers.test_frontend_method(
+ init_input_dtypes=[input_dtype[0]],
+ init_all_as_kwargs_np={"x": input},
+ method_input_dtypes=[input_dtype[0]],
+ method_all_as_kwargs_np={
+ "offset": offset,
+ "axis1": dim1,
+ "axis2": dim2,
+ },
+ frontend=frontend,
+ frontend_method_data=frontend_method_data,
+ backend_to_test=backend_fw,
+ init_flags=init_flags,
+ method_flags=method_flags,
+ on_device=on_device,
+ )
+
+
# digamma
@handle_frontend_method(
class_tree=CLASS_TREE,
@@ -1633,7 +1835,7 @@ def test_paddle_tensor_device(
max_value=1e5,
),
)
-def test_paddle_tensor_digamma(
+def test_paddle_digamma(
dtype_and_x,
frontend_method_data,
init_flags,
@@ -1668,7 +1870,7 @@ def test_paddle_tensor_digamma(
available_dtypes=helpers.get_dtypes("valid"),
),
)
-def test_paddle_tensor_dim(
+def test_paddle_dim(
dtype_and_x,
frontend_method_data,
init_flags,
@@ -1707,7 +1909,7 @@ def test_paddle_tensor_dim(
small_abs_safety_factor=32,
),
)
-def test_paddle_tensor_divide(
+def test_paddle_divide(
dtypes_and_x,
frontend_method_data,
init_flags,
@@ -1736,7 +1938,7 @@ def test_paddle_tensor_divide(
available_dtypes=helpers.get_dtypes("valid", prune_function=False)
).filter(lambda x: "bfloat16" not in x[0]),
)
-def test_paddle_tensor_dtype(
+def test_paddle_dtype(
dtype_x,
):
dtype, data = dtype_x
@@ -1752,7 +1954,7 @@ def test_paddle_tensor_dtype(
method_name="eigvals",
dtype_and_x=_get_dtype_and_square_matrix(),
)
-def test_paddle_tensor_eigvals(
+def test_paddle_eigvals(
dtype_and_x,
frontend_method_data,
init_flags,
@@ -1809,7 +2011,7 @@ def test_paddle_tensor_eigvals(
shared_dtype=True,
),
)
-def test_paddle_tensor_equal(
+def test_paddle_equal(
dtypes_and_x,
frontend_method_data,
init_flags,
@@ -1848,7 +2050,7 @@ def test_paddle_tensor_equal(
small_abs_safety_factor=32,
),
)
-def test_paddle_tensor_equal_all(
+def test_paddle_equal_all(
dtypes_and_x,
frontend_method_data,
init_flags,
@@ -1881,7 +2083,7 @@ def test_paddle_tensor_equal_all(
available_dtypes=helpers.get_dtypes("valid"),
),
)
-def test_paddle_tensor_erf(
+def test_paddle_erf(
dtype_and_x,
frontend_method_data,
init_flags,
@@ -1916,7 +2118,7 @@ def test_paddle_tensor_erf(
available_dtypes=helpers.get_dtypes("float"),
),
)
-def test_paddle_tensor_exp(
+def test_paddle_exp(
dtype_and_x,
frontend_method_data,
init_flags,
@@ -1952,7 +2154,7 @@ def test_paddle_tensor_exp(
),
test_inplace=st.just(True),
)
-def test_paddle_tensor_exp_(
+def test_paddle_exp_(
dtype_and_x,
frontend_method_data,
init_flags,
@@ -1978,6 +2180,50 @@ def test_paddle_tensor_exp_(
)
+# fill_
+@handle_frontend_method(
+ class_tree=CLASS_TREE,
+ init_tree="paddle.to_tensor",
+ method_name="fill_",
+ dtype_and_x=helpers.dtype_and_values(
+ available_dtypes=helpers.get_dtypes("valid"),
+ allow_inf=False,
+ ),
+ dtype_v=helpers.dtype_and_values(
+ available_dtypes=helpers.get_dtypes("valid"),
+ shape=(1,),
+ min_value=0,
+ max_value=10,
+ ),
+)
+def test_paddle_fill_(
+ dtype_and_x,
+ dtype_v,
+ frontend_method_data,
+ init_flags,
+ method_flags,
+ frontend,
+ on_device,
+ backend_fw,
+):
+ input_dtype, x = dtype_and_x
+ value_dtype, v = dtype_v
+ helpers.test_frontend_method(
+ init_input_dtypes=input_dtype,
+ backend_to_test=backend_fw,
+ init_all_as_kwargs_np={
+ "data": x[0],
+ },
+ method_input_dtypes=value_dtype,
+ method_all_as_kwargs_np={"value": v[0].item()},
+ frontend_method_data=frontend_method_data,
+ init_flags=init_flags,
+ method_flags=method_flags,
+ frontend=frontend,
+ on_device=on_device,
+ )
+
+
# floor
@handle_frontend_method(
class_tree=CLASS_TREE,
@@ -1987,7 +2233,7 @@ def test_paddle_tensor_exp_(
available_dtypes=helpers.get_dtypes("float"),
),
)
-def test_paddle_tensor_floor(
+def test_paddle_floor(
dtype_and_x,
frontend_method_data,
init_flags,
@@ -2023,7 +2269,7 @@ def test_paddle_tensor_floor(
),
test_inplace=st.just(True),
)
-def test_paddle_tensor_floor_(
+def test_paddle_floor_(
dtype_and_x,
frontend_method_data,
init_flags,
@@ -2064,7 +2310,7 @@ def test_paddle_tensor_floor_(
safety_factor_scale="linear",
),
)
-def test_paddle_tensor_floor_divide(
+def test_paddle_floor_divide(
dtypes_and_x,
frontend_method_data,
init_flags,
@@ -2098,7 +2344,7 @@ def test_paddle_tensor_floor_divide(
available_dtypes=helpers.get_dtypes("float"), num_arrays=2, shared_dtype=True
),
)
-def test_paddle_tensor_fmax(
+def test_paddle_fmax(
dtypes_and_x,
frontend_method_data,
init_flags,
@@ -2130,7 +2376,7 @@ def test_paddle_tensor_fmax(
available_dtypes=helpers.get_dtypes("float"), num_arrays=2, shared_dtype=True
),
)
-def test_paddle_tensor_fmin(
+def test_paddle_fmin(
dtypes_and_x,
frontend_method_data,
init_flags,
@@ -2154,21 +2400,20 @@ def test_paddle_tensor_fmin(
)
-# greater_than
+# frac
@handle_frontend_method(
class_tree=CLASS_TREE,
init_tree="paddle.to_tensor",
- method_name="greater_than",
- dtypes_and_x=helpers.dtype_and_values(
- available_dtypes=helpers.get_dtypes("valid"),
- num_arrays=2,
- shared_dtype=True,
- safety_factor_scale="log",
- small_abs_safety_factor=32,
+ method_name="frac",
+ dtype_and_x=helpers.dtype_and_values(
+ available_dtypes=helpers.get_dtypes(kind="valid"),
+ num_arrays=1,
+ max_value=1e6,
+ min_value=-1e6,
),
)
-def test_paddle_tensor_greater_than(
- dtypes_and_x,
+def test_paddle_frac(
+ dtype_and_x,
frontend_method_data,
init_flags,
method_flags,
@@ -2176,13 +2421,15 @@ def test_paddle_tensor_greater_than(
on_device,
backend_fw,
):
- input_dtype, x = dtypes_and_x
+ input_dtype, x = dtype_and_x
helpers.test_frontend_method(
init_input_dtypes=input_dtype,
backend_to_test=backend_fw,
- init_all_as_kwargs_np={"data": x[0]},
+ init_all_as_kwargs_np={
+ "data": x[0],
+ },
method_input_dtypes=input_dtype,
- method_all_as_kwargs_np={"y": x[1]},
+ method_all_as_kwargs_np={},
frontend_method_data=frontend_method_data,
init_flags=init_flags,
method_flags=method_flags,
@@ -2191,17 +2438,17 @@ def test_paddle_tensor_greater_than(
)
-# imag
+# gather
@handle_frontend_method(
class_tree=CLASS_TREE,
init_tree="paddle.to_tensor",
- method_name="imag",
- dtype_and_x=helpers.dtype_and_values(
- available_dtypes=helpers.get_dtypes("valid"),
+ method_name="gather",
+ dtypes_and_x=helpers.dtype_and_values(
+ available_dtypes=helpers.get_dtypes("float"), num_arrays=2, shared_dtype=True
),
)
-def test_paddle_tensor_imag(
- dtype_and_x,
+def test_paddle_gather(
+ dtypes_and_x,
frontend_method_data,
init_flags,
method_flags,
@@ -2209,15 +2456,13 @@ def test_paddle_tensor_imag(
on_device,
backend_fw,
):
- input_dtype, x = dtype_and_x
+ input_dtype, x = dtypes_and_x
helpers.test_frontend_method(
init_input_dtypes=input_dtype,
backend_to_test=backend_fw,
- init_all_as_kwargs_np={
- "data": x[0],
- },
+ init_all_as_kwargs_np={"data": x[0]},
method_input_dtypes=input_dtype,
- method_all_as_kwargs_np={},
+ method_all_as_kwargs_np={"y": x[1]},
frontend_method_data=frontend_method_data,
init_flags=init_flags,
method_flags=method_flags,
@@ -2226,21 +2471,93 @@ def test_paddle_tensor_imag(
)
-# inner
+# greater_than
@handle_frontend_method(
class_tree=CLASS_TREE,
init_tree="paddle.to_tensor",
- method_name="inner",
- dtype_and_x=helpers.dtype_and_values(
+ method_name="greater_than",
+ dtypes_and_x=helpers.dtype_and_values(
available_dtypes=helpers.get_dtypes("valid"),
- min_value=-10,
- max_value=10,
num_arrays=2,
shared_dtype=True,
+ safety_factor_scale="log",
+ small_abs_safety_factor=32,
),
)
-def test_paddle_tensor_inner(
- dtype_and_x,
+def test_paddle_greater_than(
+ dtypes_and_x,
+ frontend_method_data,
+ init_flags,
+ method_flags,
+ frontend,
+ on_device,
+ backend_fw,
+):
+ input_dtype, x = dtypes_and_x
+ helpers.test_frontend_method(
+ init_input_dtypes=input_dtype,
+ backend_to_test=backend_fw,
+ init_all_as_kwargs_np={"data": x[0]},
+ method_input_dtypes=input_dtype,
+ method_all_as_kwargs_np={"y": x[1]},
+ frontend_method_data=frontend_method_data,
+ init_flags=init_flags,
+ method_flags=method_flags,
+ frontend=frontend,
+ on_device=on_device,
+ )
+
+
+# imag
+@handle_frontend_method(
+ class_tree=CLASS_TREE,
+ init_tree="paddle.to_tensor",
+ method_name="imag",
+ dtype_and_x=helpers.dtype_and_values(
+ available_dtypes=helpers.get_dtypes("valid"),
+ ),
+)
+def test_paddle_imag(
+ dtype_and_x,
+ frontend_method_data,
+ init_flags,
+ method_flags,
+ frontend,
+ on_device,
+ backend_fw,
+):
+ input_dtype, x = dtype_and_x
+ helpers.test_frontend_method(
+ init_input_dtypes=input_dtype,
+ backend_to_test=backend_fw,
+ init_all_as_kwargs_np={
+ "data": x[0],
+ },
+ method_input_dtypes=input_dtype,
+ method_all_as_kwargs_np={},
+ frontend_method_data=frontend_method_data,
+ init_flags=init_flags,
+ method_flags=method_flags,
+ frontend=frontend,
+ on_device=on_device,
+ )
+
+
+# inner
+@handle_frontend_method(
+ class_tree=CLASS_TREE,
+ init_tree="paddle.to_tensor",
+ method_name="inner",
+ dtype_and_x=helpers.dtype_and_values(
+ available_dtypes=helpers.get_dtypes("valid"),
+ min_value=-10,
+ max_value=10,
+ num_arrays=2,
+ shared_dtype=True,
+ ),
+)
+def test_paddle_inner(
+ dtype_and_x,
frontend_method_data,
init_flags,
method_flags,
@@ -2265,6 +2582,39 @@ def test_paddle_tensor_inner(
)
+# is_floating_point
+@handle_frontend_method(
+ class_tree=CLASS_TREE,
+ init_tree="paddle.to_tensor",
+ method_name="is_floating_point",
+ dtype_and_x=helpers.dtype_and_values(
+ available_dtypes=["int16", "int32", "int64", "float32", "float64"],
+ ),
+)
+def test_paddle_is_floating_point(
+ dtype_and_x,
+ frontend_method_data,
+ init_flags,
+ method_flags,
+ frontend,
+ backend_fw,
+ on_device,
+):
+ input_dtype, x = dtype_and_x
+ helpers.test_frontend_method(
+ init_input_dtypes=input_dtype,
+ init_all_as_kwargs_np={"data": x[0]},
+ method_input_dtypes=input_dtype,
+ backend_to_test=backend_fw,
+ method_all_as_kwargs_np={},
+ frontend_method_data=frontend_method_data,
+ init_flags=init_flags,
+ method_flags=method_flags,
+ frontend=frontend,
+ on_device=on_device,
+ )
+
+
# is_tensor
@handle_frontend_method(
class_tree=CLASS_TREE,
@@ -2275,7 +2625,7 @@ def test_paddle_tensor_inner(
num_arrays=1,
),
)
-def test_paddle_tensor_is_tensor(
+def test_paddle_is_tensor(
dtype_and_x,
frontend_method_data,
init_flags,
@@ -2310,7 +2660,7 @@ def test_paddle_tensor_is_tensor(
available_dtypes=helpers.get_dtypes("valid"), num_arrays=2, shared_dtype=True
),
)
-def test_paddle_tensor_isclose(
+def test_paddle_isclose(
dtypes_and_x,
frontend_method_data,
init_flags,
@@ -2343,7 +2693,7 @@ def test_paddle_tensor_isclose(
available_dtypes=helpers.get_dtypes("valid"),
),
)
-def test_paddle_tensor_isfinite(
+def test_paddle_isfinite(
dtype_and_x,
frontend_method_data,
init_flags,
@@ -2378,7 +2728,7 @@ def test_paddle_tensor_isfinite(
available_dtypes=helpers.get_dtypes("valid"),
),
)
-def test_paddle_tensor_isinf(
+def test_paddle_isinf(
dtype_and_x,
frontend_method_data,
init_flags,
@@ -2413,7 +2763,7 @@ def test_paddle_tensor_isinf(
available_dtypes=helpers.get_dtypes("float"),
),
)
-def test_paddle_tensor_isnan(
+def test_paddle_isnan(
dtype_and_x,
frontend_method_data,
init_flags,
@@ -2446,7 +2796,7 @@ def test_paddle_tensor_isnan(
method_name="lerp",
dtypes_and_x=_get_dtype_and_values_for_lerp(),
)
-def test_paddle_tensor_lerp(
+def test_paddle_lerp(
dtypes_and_x,
frontend_method_data,
init_flags,
@@ -2483,7 +2833,7 @@ def test_paddle_tensor_lerp(
),
test_inplace=st.just(True),
)
-def test_paddle_tensor_lerp_(
+def test_paddle_lerp_(
dtypes_and_x,
frontend_method_data,
init_flags,
@@ -2525,7 +2875,7 @@ def test_paddle_tensor_lerp_(
shared_dtype=True,
),
)
-def test_paddle_tensor_less_equal(
+def test_paddle_less_equal(
dtype_and_x,
frontend_method_data,
init_flags,
@@ -2558,7 +2908,7 @@ def test_paddle_tensor_less_equal(
available_dtypes=helpers.get_dtypes("valid"), num_arrays=2, shared_dtype=True
),
)
-def test_paddle_tensor_less_than(
+def test_paddle_less_than(
dtypes_and_x,
frontend_method_data,
init_flags,
@@ -2591,7 +2941,7 @@ def test_paddle_tensor_less_than(
available_dtypes=helpers.get_dtypes("float"),
),
)
-def test_paddle_tensor_log(
+def test_paddle_log(
dtype_and_x,
frontend_method_data,
init_flags,
@@ -2626,7 +2976,7 @@ def test_paddle_tensor_log(
available_dtypes=helpers.get_dtypes("float"),
),
)
-def test_paddle_tensor_log10(
+def test_paddle_log10(
dtype_and_x,
frontend_method_data,
init_flags,
@@ -2661,7 +3011,7 @@ def test_paddle_tensor_log10(
available_dtypes=helpers.get_dtypes("valid"), num_arrays=2, shared_dtype=True
),
)
-def test_paddle_tensor_logical_and(
+def test_paddle_logical_and(
dtypes_and_x,
frontend_method_data,
init_flags,
@@ -2694,7 +3044,7 @@ def test_paddle_tensor_logical_and(
available_dtypes=helpers.get_dtypes("valid"),
),
)
-def test_paddle_tensor_logical_not(
+def test_paddle_logical_not(
dtype_and_x,
frontend_method_data,
init_flags,
@@ -2729,7 +3079,7 @@ def test_paddle_tensor_logical_not(
available_dtypes=helpers.get_dtypes("valid"), num_arrays=2, shared_dtype=True
),
)
-def test_paddle_tensor_logical_or(
+def test_paddle_logical_or(
dtypes_and_x,
frontend_method_data,
init_flags,
@@ -2762,7 +3112,7 @@ def test_paddle_tensor_logical_or(
available_dtypes=helpers.get_dtypes("valid"), num_arrays=2, shared_dtype=True
),
)
-def test_paddle_tensor_logical_xor(
+def test_paddle_logical_xor(
dtypes_and_x,
frontend_method_data,
init_flags,
@@ -2800,7 +3150,7 @@ def test_paddle_tensor_logical_xor(
),
keep_dims=st.booleans(),
)
-def test_paddle_tensor_max(
+def test_paddle_max(
dtype_x_axis,
keep_dims,
frontend_method_data,
@@ -2838,7 +3188,7 @@ def test_paddle_tensor_max(
dtype_and_x=_statistical_dtype_values(function="mean"),
keepdim=st.booleans(),
)
-def test_paddle_tensor_mean(
+def test_paddle_mean(
dtype_and_x,
keepdim,
frontend,
@@ -2876,7 +3226,43 @@ def test_paddle_tensor_mean(
shared_dtype=True,
),
)
-def test_paddle_tensor_minimum(
+def test_paddle_minimum(
+ dtype_and_x,
+ frontend_method_data,
+ init_flags,
+ method_flags,
+ frontend,
+ on_device,
+ backend_fw,
+):
+ input_dtype, x = dtype_and_x
+ helpers.test_frontend_method(
+ init_input_dtypes=input_dtype,
+ backend_to_test=backend_fw,
+ init_all_as_kwargs_np={"data": x[0]},
+ method_input_dtypes=input_dtype,
+ method_all_as_kwargs_np={"y": x[1]},
+ frontend_method_data=frontend_method_data,
+ init_flags=init_flags,
+ method_flags=method_flags,
+ frontend=frontend,
+ on_device=on_device,
+ )
+
+
+@handle_frontend_method(
+ class_tree=CLASS_TREE,
+ init_tree="paddle.to_tensor",
+ method_name="mod",
+ dtype_and_x=helpers.dtype_and_values(
+ available_dtypes=helpers.get_dtypes("valid"),
+ num_arrays=2,
+ shared_dtype=True,
+ min_value=0,
+ exclude_min=True,
+ ),
+)
+def test_paddle_mod(
dtype_and_x,
frontend_method_data,
init_flags,
@@ -2910,7 +3296,7 @@ def test_paddle_tensor_minimum(
shared_dtype=True,
),
)
-def test_paddle_tensor_multiply(
+def test_paddle_multiply(
dtype_and_x,
frontend_method_data,
init_flags,
@@ -2943,7 +3329,7 @@ def test_paddle_tensor_multiply(
available_dtypes=helpers.get_dtypes("valid", prune_function=False),
).filter(lambda x: "bfloat16" not in x[0]),
)
-def test_paddle_tensor_ndim(
+def test_paddle_ndim(
dtype_x,
):
_, data = dtype_x
@@ -2963,7 +3349,7 @@ def test_paddle_tensor_ndim(
allow_inf=False,
),
)
-def test_paddle_tensor_neg(
+def test_paddle_neg(
dtype_and_x,
frontend,
frontend_method_data,
@@ -3000,7 +3386,7 @@ def test_paddle_tensor_neg(
allow_inf=True,
),
)
-def test_paddle_tensor_nonzero(
+def test_paddle_nonzero(
dtype_and_x,
frontend_method_data,
init_flags,
@@ -3026,6 +3412,47 @@ def test_paddle_tensor_nonzero(
)
+# not_equal
+@handle_frontend_method(
+ class_tree=CLASS_TREE,
+ init_tree="paddle.to_tensor",
+ method_name="not_equal",
+ dtype_and_x=helpers.dtype_and_values(
+ available_dtypes=helpers.get_dtypes(kind="valid"),
+ num_arrays=2,
+ shared_dtype=True,
+ ),
+)
+def test_paddle_not_equal(
+ dtype_and_x,
+ frontend_method_data,
+ init_flags,
+ method_flags,
+ frontend,
+ on_device,
+ backend_fw,
+):
+ input_dtype, x = dtype_and_x
+ helpers.test_frontend_method(
+ init_input_dtypes=input_dtype,
+ backend_to_test=backend_fw,
+ init_all_as_kwargs_np={
+ "x": x[0],
+ },
+ method_input_dtypes=input_dtype,
+ method_all_as_kwargs_np={
+ "y": x[1],
+ },
+ frontend_method_data=frontend_method_data,
+ init_flags=init_flags,
+ method_flags=method_flags,
+ frontend=frontend,
+ on_device=on_device,
+ rtol_=1e-02,
+ atol_=1e-02,
+ )
+
+
@handle_frontend_method(
class_tree=CLASS_TREE,
init_tree="paddle.to_tensor",
@@ -3035,7 +3462,7 @@ def test_paddle_tensor_nonzero(
min_num_dims=1,
),
)
-def test_paddle_tensor_numel(
+def test_paddle_numel(
dtype_and_x,
frontend_method_data,
init_flags,
@@ -3072,7 +3499,7 @@ def test_paddle_tensor_numel(
min_dim_size=2,
),
)
-def test_paddle_tensor_numpy(
+def test_paddle_numpy(
dtype_and_x,
frontend_method_data,
init_flags,
@@ -3110,7 +3537,7 @@ def test_paddle_tensor_numpy(
shared_dtype=True,
),
)
-def test_paddle_tensor_pow(
+def test_paddle_pow(
dtypes_and_x,
frontend_method_data,
init_flags,
@@ -3150,7 +3577,7 @@ def test_paddle_tensor_pow(
),
keep_dims=st.booleans(),
)
-def test_paddle_tensor_prod(
+def test_paddle_prod(
dtype_x_axis,
keep_dims,
frontend_method_data,
@@ -3188,7 +3615,7 @@ def test_paddle_tensor_prod(
available_dtypes=helpers.get_dtypes("float"),
),
)
-def test_paddle_tensor_rad2deg(
+def test_paddle_rad2deg(
dtype_and_x,
frontend_method_data,
init_flags,
@@ -3225,7 +3652,7 @@ def test_paddle_tensor_rad2deg(
allow_inf=True,
),
)
-def test_paddle_tensor_real(
+def test_paddle_real(
dtype_and_x,
frontend_method_data,
init_flags,
@@ -3260,7 +3687,7 @@ def test_paddle_tensor_real(
available_dtypes=helpers.get_dtypes("valid"),
),
)
-def test_paddle_tensor_reciprocal(
+def test_paddle_reciprocal(
dtype_and_x,
frontend_method_data,
init_flags,
@@ -3295,7 +3722,7 @@ def test_paddle_tensor_reciprocal(
available_dtypes=helpers.get_dtypes("valid"),
),
)
-def test_paddle_tensor_reciprocal_(
+def test_paddle_reciprocal_(
dtype_and_x,
frontend_method_data,
init_flags,
@@ -3336,7 +3763,7 @@ def test_paddle_tensor_reciprocal_(
shared_dtype=True,
),
)
-def test_paddle_tensor_remainder(
+def test_paddle_remainder(
dtype_and_x,
frontend_method_data,
init_flags,
@@ -3372,7 +3799,7 @@ def test_paddle_tensor_remainder(
),
test_inplace=st.just(True),
)
-def test_paddle_tensor_remainder_(
+def test_paddle_remainder_(
dtype_and_x,
frontend_method_data,
init_flags,
@@ -3413,7 +3840,7 @@ def test_paddle_tensor_remainder_(
max_dim_size=10,
),
)
-def test_paddle_tensor_rot90(
+def test_paddle_rot90(
dtype_m_k_axes,
frontend_method_data,
init_flags,
@@ -3452,7 +3879,7 @@ def test_paddle_tensor_rot90(
available_dtypes=helpers.get_dtypes("valid"),
),
)
-def test_paddle_tensor_round_(
+def test_paddle_round_(
dtype_and_x,
frontend_method_data,
init_flags,
@@ -3487,7 +3914,7 @@ def test_paddle_tensor_round_(
available_dtypes=helpers.get_dtypes("valid"),
),
)
-def test_paddle_tensor_rsqrt(
+def test_paddle_rsqrt(
dtype_and_x,
frontend_method_data,
init_flags,
@@ -3523,7 +3950,7 @@ def test_paddle_tensor_rsqrt(
),
test_inplace=st.just(True),
)
-def test_paddle_tensor_rsqrt_(
+def test_paddle_rsqrt_(
dtype_and_x,
frontend_method_data,
init_flags,
@@ -3555,7 +3982,7 @@ def test_paddle_tensor_rsqrt_(
ret_shape=True,
).filter(lambda x: "bfloat16" not in x[0]),
)
-def test_paddle_tensor_shape(dtype_x):
+def test_paddle_shape(dtype_x):
_, data, shape = dtype_x
x = Tensor(data[0])
ivy.utils.assertions.check_equal(
@@ -3571,7 +3998,7 @@ def test_paddle_tensor_shape(dtype_x):
available_dtypes=helpers.get_dtypes("float"),
),
)
-def test_paddle_tensor_sign(
+def test_paddle_sign(
dtype_and_x,
frontend_method_data,
init_flags,
@@ -3606,7 +4033,7 @@ def test_paddle_tensor_sign(
available_dtypes=helpers.get_dtypes("float"),
),
)
-def test_paddle_tensor_sin(
+def test_paddle_sin(
dtype_and_x,
frontend_method_data,
init_flags,
@@ -3641,7 +4068,7 @@ def test_paddle_tensor_sin(
available_dtypes=helpers.get_dtypes("float"),
),
)
-def test_paddle_tensor_sinh(
+def test_paddle_sinh(
dtype_and_x,
frontend_method_data,
init_flags,
@@ -3681,7 +4108,7 @@ def test_paddle_tensor_sinh(
),
descending=st.booleans(),
)
-def test_paddle_tensor_sort(
+def test_paddle_sort(
dtype_x_axis,
descending,
frontend_method_data,
@@ -3711,6 +4138,41 @@ def test_paddle_tensor_sort(
)
+# split
+@handle_frontend_method(
+ class_tree=CLASS_TREE,
+ init_tree="paddle.to_tensor",
+ method_name="split",
+ dtype_and_x=helpers.dtype_and_values(
+ available_dtypes=helpers.get_dtypes("valid"),
+ ),
+)
+def test_paddle_split(
+ dtype_and_x,
+ frontend_method_data,
+ init_flags,
+ method_flags,
+ frontend,
+ on_device,
+ backend_fw,
+):
+ input_dtype, x = dtype_and_x
+ helpers.test_frontend_method(
+ init_input_dtypes=input_dtype,
+ backend_to_test=backend_fw,
+ init_all_as_kwargs_np={
+ "data": x[0],
+ },
+ method_input_dtypes=input_dtype,
+ method_all_as_kwargs_np={},
+ frontend_method_data=frontend_method_data,
+ init_flags=init_flags,
+ method_flags=method_flags,
+ frontend=frontend,
+ on_device=on_device,
+ )
+
+
# sqrt
@handle_frontend_method(
class_tree=CLASS_TREE,
@@ -3720,7 +4182,7 @@ def test_paddle_tensor_sort(
available_dtypes=helpers.get_dtypes("valid"),
),
)
-def test_paddle_tensor_sqrt(
+def test_paddle_sqrt(
dtype_and_x,
frontend_method_data,
init_flags,
@@ -3756,7 +4218,7 @@ def test_paddle_tensor_sqrt(
),
test_inplace=st.just(True),
)
-def test_paddle_tensor_sqrt_(
+def test_paddle_sqrt_(
dtype_x,
frontend,
frontend_method_data,
@@ -3789,7 +4251,7 @@ def test_paddle_tensor_sqrt_(
available_dtypes=helpers.get_dtypes("float"),
),
)
-def test_paddle_tensor_square(
+def test_paddle_square(
dtype_and_x,
frontend_method_data,
init_flags,
@@ -3831,7 +4293,7 @@ def test_paddle_tensor_square(
),
test_inplace=st.just(True),
)
-def test_paddle_tensor_squeeze_(
+def test_paddle_squeeze_(
dtype_value,
axis,
frontend_method_data,
@@ -3871,7 +4333,7 @@ def test_paddle_tensor_squeeze_(
scale_a=st.floats(1e-5, 1e5),
scale_b=st.floats(1e-5, 1e5),
)
-def test_paddle_tensor_stanh(
+def test_paddle_stanh(
dtype_and_x,
frontend_method_data,
scale_a,
@@ -3910,7 +4372,7 @@ def test_paddle_tensor_stanh(
dtype_and_x=_statistical_dtype_values(function="std"),
keepdim=st.booleans(),
)
-def test_paddle_tensor_std(
+def test_paddle_std(
dtype_and_x,
keepdim,
frontend,
@@ -3948,7 +4410,7 @@ def test_paddle_tensor_std(
available_dtypes=helpers.get_dtypes("float"), num_arrays=2, shared_dtype=True
),
)
-def test_paddle_tensor_subtract(
+def test_paddle_subtract(
dtypes_and_x,
frontend_method_data,
init_flags,
@@ -3982,7 +4444,7 @@ def test_paddle_tensor_subtract(
),
test_inplace=st.just(True),
)
-def test_paddle_tensor_subtract_(
+def test_paddle_subtract_(
dtypes_and_x,
frontend_method_data,
init_flags,
@@ -4006,6 +4468,42 @@ def test_paddle_tensor_subtract_(
)
+# t
+@handle_frontend_method(
+ class_tree=CLASS_TREE,
+ init_tree="paddle.to_tensor",
+ method_name="t",
+ dtype_and_x=helpers.dtype_and_values(
+ available_dtypes=helpers.get_dtypes("valid"),
+ max_num_dims=2,
+ ),
+)
+def test_paddle_t(
+ dtype_and_x,
+ frontend_method_data,
+ init_flags,
+ method_flags,
+ frontend,
+ on_device,
+ backend_fw,
+):
+ input_dtype, x = dtype_and_x
+ helpers.test_frontend_method(
+ init_input_dtypes=input_dtype,
+ init_all_as_kwargs_np={
+ "data": x[0],
+ },
+ backend_to_test=backend_fw,
+ method_input_dtypes=input_dtype,
+ method_all_as_kwargs_np={},
+ frontend_method_data=frontend_method_data,
+ init_flags=init_flags,
+ method_flags=method_flags,
+ frontend=frontend,
+ on_device=on_device,
+ )
+
+
# tanh
@handle_frontend_method(
class_tree=CLASS_TREE,
@@ -4015,7 +4513,7 @@ def test_paddle_tensor_subtract_(
available_dtypes=helpers.get_dtypes("valid"),
),
)
-def test_paddle_tensor_tanh(
+def test_paddle_tanh(
dtype_and_x,
frontend_method_data,
init_flags,
@@ -4050,7 +4548,7 @@ def test_paddle_tensor_tanh(
available_dtypes=helpers.get_dtypes("valid"),
),
)
-def test_paddle_tensor_tanh_(
+def test_paddle_tanh_(
dtype_and_x,
frontend_method_data,
init_flags,
@@ -4076,6 +4574,116 @@ def test_paddle_tensor_tanh_(
)
+# expand
+@handle_frontend_method(
+ class_tree=CLASS_TREE,
+ init_tree="paddle.to_tensor",
+ method_name="expand",
+ dtype_x_shape=dtypes_x_shape(),
+)
+def test_paddle_tensor_expand(
+ dtype_x_shape,
+ frontend_method_data,
+ init_flags,
+ method_flags,
+ frontend,
+ on_device,
+ backend_fw,
+):
+ input_dtype, x, shape = dtype_x_shape
+ helpers.test_frontend_method(
+ init_input_dtypes=input_dtype,
+ backend_to_test=backend_fw,
+ init_all_as_kwargs_np={
+ "data": x[0],
+ },
+ method_input_dtypes=input_dtype,
+ method_all_as_kwargs_np={
+ "shape": shape,
+ },
+ frontend_method_data=frontend_method_data,
+ init_flags=init_flags,
+ method_flags=method_flags,
+ frontend=frontend,
+ on_device=on_device,
+ )
+
+
+@handle_frontend_method(
+ class_tree=CLASS_TREE,
+ init_tree="paddle.to_tensor",
+ method_name="heaviside",
+ dtype_and_x=helpers.dtype_and_values(
+ available_dtypes=helpers.get_dtypes("float"),
+ num_arrays=2,
+ allow_inf=False,
+ large_abs_safety_factor=2,
+ small_abs_safety_factor=2,
+ safety_factor_scale="log",
+ shared_dtype=True,
+ ),
+)
+def test_paddle_tensor_heaviside(
+ dtype_and_x,
+ frontend_method_data,
+ init_flags,
+ method_flags,
+ frontend,
+ backend_fw,
+ on_device,
+):
+ input_dtype, x = dtype_and_x
+ helpers.test_frontend_method(
+ init_input_dtypes=input_dtype,
+ backend_to_test=backend_fw,
+ init_all_as_kwargs_np={
+ "x": x[0],
+ },
+ method_input_dtypes=input_dtype,
+ method_all_as_kwargs_np={
+ "y": x[1],
+ },
+ init_flags=init_flags,
+ method_flags=method_flags,
+ frontend_method_data=frontend_method_data,
+ frontend=frontend,
+ on_device=on_device,
+ )
+
+
+# tile
+@handle_frontend_method(
+ class_tree=CLASS_TREE,
+ init_tree="paddle.to_tensor",
+ method_name="tile",
+ dt_x_repeats=_tile_helper(),
+)
+def test_paddle_tensor_tile(
+ dt_x_repeats,
+ frontend,
+ backend_fw,
+ frontend_method_data,
+ init_flags,
+ method_flags,
+ on_device,
+):
+ input_dtypes, x, repeats = dt_x_repeats
+ helpers.test_frontend_method(
+ init_input_dtypes=input_dtypes,
+ init_all_as_kwargs_np={"data": x[0]},
+ method_input_dtypes=input_dtypes,
+ method_all_as_kwargs_np={
+ "repeat_times": repeats,
+ },
+ frontend=frontend,
+ frontend_method_data=frontend_method_data,
+ init_flags=init_flags,
+ backend_to_test=backend_fw,
+ method_flags=method_flags,
+ on_device=on_device,
+ )
+
+
# topk
@handle_frontend_method(
class_tree=CLASS_TREE,
@@ -4091,7 +4699,7 @@ def test_paddle_tensor_tanh_(
sorted=st.booleans(),
largest=st.booleans(),
)
-def test_paddle_tensor_topk(
+def test_paddle_topk(
dtype_x_and_axis,
k,
sorted,
@@ -4144,7 +4752,7 @@ def test_paddle_tensor_topk(
axis1=st.integers(min_value=0, max_value=0),
axis2=st.integers(min_value=1, max_value=1),
)
-def test_paddle_tensor_trace(
+def test_paddle_trace(
dtype_and_x,
offset,
axis1,
@@ -4185,7 +4793,7 @@ def test_paddle_tensor_trace(
available_dtypes=helpers.get_dtypes("valid"),
),
)
-def test_paddle_tensor_trunc(
+def test_paddle_trunc(
dtype_and_x,
frontend_method_data,
init_flags,
@@ -4226,7 +4834,50 @@ def test_paddle_tensor_trunc(
max_axis=0,
),
)
-def test_paddle_tensor_unbind(
+def test_paddle_unbind(
+ dtype_x_axis,
+ frontend_method_data,
+ init_flags,
+ method_flags,
+ frontend,
+ on_device,
+ backend_fw,
+):
+ input_dtypes, x, axis = dtype_x_axis
+ helpers.test_frontend_method(
+ init_input_dtypes=input_dtypes,
+ backend_to_test=backend_fw,
+ init_all_as_kwargs_np={
+ "data": x[0],
+ },
+ method_input_dtypes=input_dtypes,
+ method_all_as_kwargs_np={
+ "axis": axis,
+ },
+ frontend=frontend,
+ frontend_method_data=frontend_method_data,
+ init_flags=init_flags,
+ method_flags=method_flags,
+ on_device=on_device,
+ )
+
+
+# unique_consecutive
+@handle_frontend_method(
+ class_tree=CLASS_TREE,
+ init_tree="paddle.to_tensor",
+ method_name="unique_consecutive",
+ dtype_x_axis=helpers.dtype_values_axis(
+ available_dtypes=helpers.get_dtypes("valid"),
+ min_num_dims=2,
+ max_num_dims=4,
+ max_dim_size=1,
+ force_int_axis=True,
+ min_axis=-1,
+ max_axis=0,
+ ),
+)
+def test_paddle_unique_consecutive(
dtype_x_axis,
frontend_method_data,
init_flags,
@@ -4269,7 +4920,7 @@ def test_paddle_tensor_unbind(
force_int=True,
),
)
-def test_paddle_tensor_unsqueeze(
+def test_paddle_unsqueeze(
dtype_value,
axis,
frontend_method_data,
@@ -4314,7 +4965,7 @@ def test_paddle_tensor_unsqueeze(
),
test_inplace=st.just(True),
)
-def test_paddle_tensor_unsqueeze_(
+def test_paddle_unsqueeze_(
dtype_value,
axis,
frontend_method_data,
@@ -4351,7 +5002,7 @@ def test_paddle_tensor_unsqueeze_(
dtype_and_x=_statistical_dtype_values(function="var"),
keepdim=st.booleans(),
)
-def test_paddle_tensor_var(
+def test_paddle_var(
dtype_and_x,
keepdim,
frontend,
@@ -4391,7 +5042,7 @@ def test_paddle_tensor_var(
),
test_inplace=st.just(True),
)
-def test_paddle_tensor_zero_(
+def test_paddle_zero_(
dtype_and_x,
frontend_method_data,
init_flags,
diff --git a/ivy_tests/test_ivy/test_frontends/test_pandas/test_dataframe.py b/ivy_tests/test_ivy/test_frontends/test_pandas/test_dataframe.py
index 074137130b30e..cd079b2375f49 100644
--- a/ivy_tests/test_ivy/test_frontends/test_pandas/test_dataframe.py
+++ b/ivy_tests/test_ivy/test_frontends/test_pandas/test_dataframe.py
@@ -24,6 +24,7 @@ def test_pandas_series_abs(
frontend_method_data,
init_flags,
method_flags,
+ backend_fw,
on_device,
):
# todo add castable dtypes for output
@@ -39,6 +40,7 @@ def test_pandas_series_abs(
init_flags=init_flags,
method_flags=method_flags,
frontend=frontend,
+ backend_to_test=backend_fw,
on_device=on_device,
)
@@ -138,6 +140,7 @@ def test_pandas_series_to_numpy(
na_values,
copy,
frontend_method_data,
+ backend_fw,
init_flags,
method_flags,
on_device,
@@ -157,5 +160,6 @@ def test_pandas_series_to_numpy(
init_flags=init_flags,
method_flags=method_flags,
frontend=frontend,
+ backend_to_test=backend_fw,
on_device=on_device,
)
diff --git a/ivy_tests/test_ivy/test_frontends/test_pandas/test_series.py b/ivy_tests/test_ivy/test_frontends/test_pandas/test_series.py
index 796045cb93a76..bbb520bb280b3 100644
--- a/ivy_tests/test_ivy/test_frontends/test_pandas/test_series.py
+++ b/ivy_tests/test_ivy/test_frontends/test_pandas/test_series.py
@@ -21,6 +21,7 @@
def test_pandas_series_abs(
dtype_x,
frontend,
+ backend_fw,
frontend_method_data,
init_flags,
method_flags,
@@ -38,6 +39,7 @@ def test_pandas_series_abs(
init_flags=init_flags,
method_flags=method_flags,
frontend=frontend,
+ backend_to_test=backend_fw,
on_device=on_device,
)
diff --git a/ivy_tests/test_ivy/test_frontends/test_scipy/test_linalg/test_linalg.py b/ivy_tests/test_ivy/test_frontends/test_scipy/test_linalg/test_linalg.py
index d73718550615a..a8e405bcbe01b 100644
--- a/ivy_tests/test_ivy/test_frontends/test_scipy/test_linalg/test_linalg.py
+++ b/ivy_tests/test_ivy/test_frontends/test_scipy/test_linalg/test_linalg.py
@@ -56,7 +56,7 @@ def _generate_eigh_tridiagonal_args(draw):
select_range = [-100, 100]
eigvals_only = draw(st.booleans())
- tol = draw(st.floats(1e-5, 1e-3) | st.just(None))
+ tol = draw(st.floats(1e-5, 1e-3))
return dtype, alpha, beta, eigvals_only, select, select_range, tol
diff --git a/ivy_tests/test_ivy/test_frontends/test_sklearn/test_utils/test_multiclass.py b/ivy_tests/test_ivy/test_frontends/test_sklearn/test_utils/test_multiclass.py
index 28c93f02fa8a2..f989c8d21d1d3 100644
--- a/ivy_tests/test_ivy/test_frontends/test_sklearn/test_utils/test_multiclass.py
+++ b/ivy_tests/test_ivy/test_frontends/test_sklearn/test_utils/test_multiclass.py
@@ -5,7 +5,7 @@
# not suitable for usual frontend testing
@pytest.mark.parametrize(
- "y, label",
+ ("y", "label"),
[
([1.2], "continuous"),
([1], "binary"),
diff --git a/ivy_tests/test_ivy/test_frontends/test_sklearn/test_utils/test_validation.py b/ivy_tests/test_ivy/test_frontends/test_sklearn/test_utils/test_validation.py
index 19b865e1df85f..ec6258248bcea 100644
--- a/ivy_tests/test_ivy/test_frontends/test_sklearn/test_utils/test_validation.py
+++ b/ivy_tests/test_ivy/test_frontends/test_sklearn/test_utils/test_validation.py
@@ -8,6 +8,7 @@
dtype_and_x=helpers.dtype_and_values(
available_dtypes=helpers.get_dtypes("valid"),
),
+ test_with_copy=st.just(True),
)
def test_sklearn_as_float_array(
dtype_and_x,
diff --git a/ivy_tests/test_ivy/test_frontends/test_tensorflow/test_general_functions.py b/ivy_tests/test_ivy/test_frontends/test_tensorflow/test_general_functions.py
index 9fe8772271444..2fa0da6a6c272 100644
--- a/ivy_tests/test_ivy/test_frontends/test_tensorflow/test_general_functions.py
+++ b/ivy_tests/test_ivy/test_frontends/test_tensorflow/test_general_functions.py
@@ -1050,6 +1050,7 @@ def test_tensorflow_gather_nd(
available_dtypes=helpers.get_dtypes("numeric"),
),
test_with_out=st.just(False),
+ test_with_copy=st.just(True),
)
def test_tensorflow_identity(
dtype_and_x,
@@ -1078,6 +1079,7 @@ def test_tensorflow_identity(
available_dtypes=helpers.get_dtypes("valid"), max_num_dims=5
),
test_with_out=st.just(False),
+ test_with_copy=st.just(True),
)
def test_tensorflow_identity_n(
dtype_and_x,
@@ -2036,6 +2038,50 @@ def test_tensorflow_strided_slice(
raise e
+# tensor_scatter_nd_add
+@handle_frontend_test(
+ fn_tree="tensorflow.tensor_scatter_nd_add",
+ all_arguments=_multiple_shape_helper(),
+ tensor=helpers.array_values(
+ dtype=helpers.get_dtypes("numeric"), shape=(8,), min_value=2, max_value=49
+ ),
+ indices=helpers.array_values(
+ dtype=helpers.get_dtypes("integer"), shape=(4, 1), min_value=0, max_value=7
+ ),
+ updates=helpers.array_values(
+ dtype=helpers.get_dtypes("integer"),
+ shape=(4,),
+ min_value=9,
+ max_value=12,
+ ),
+)
+def test_tensorflow_tensor_scatter_nd_add(
+ *,
+ all_arguments,
+ tensor,
+ indices,
+ updates,
+ frontend,
+ test_flags,
+ fn_tree,
+ on_device,
+ backend_fw,
+):
+ input_dtype, input_matrix, dt_and_multiples = all_arguments
+ dt_mul, multiples = dt_and_multiples
+ helpers.test_frontend_function(
+ input_dtypes=input_dtype + dt_mul,
+ frontend=frontend,
+ backend_to_test=backend_fw,
+ test_flags=test_flags,
+ fn_tree=fn_tree,
+ on_device=on_device,
+ tensor=tensor[0],
+ indices=indices[0],
+ updates=updates[0],
+ )
+
+
@handle_frontend_test(fn_tree="tensorflow.tile", all_arguments=_multiple_shape_helper())
def test_tensorflow_tile(
*,
diff --git a/ivy_tests/test_ivy/test_frontends/test_tensorflow/test_keras/test_activations.py b/ivy_tests/test_ivy/test_frontends/test_tensorflow/test_keras/test_activations.py
index d75cb2079ef85..c0b7eaa4a2ce9 100644
--- a/ivy_tests/test_ivy/test_frontends/test_tensorflow/test_keras/test_activations.py
+++ b/ivy_tests/test_ivy/test_frontends/test_tensorflow/test_keras/test_activations.py
@@ -18,7 +18,7 @@ def get_callable_functions(
module_name: str,
):
module = sys.modules[module_name]
- fn_list = list()
+ fn_list = []
for fn_name in dir(module):
obj = getattr(module, fn_name)
if callable(obj):
diff --git a/ivy_tests/test_ivy/test_frontends/test_tensorflow/test_linalg.py b/ivy_tests/test_ivy/test_frontends/test_tensorflow/test_linalg.py
index f535c0c5792bb..fdc93ffc80933 100644
--- a/ivy_tests/test_ivy/test_frontends/test_tensorflow/test_linalg.py
+++ b/ivy_tests/test_ivy/test_frontends/test_tensorflow/test_linalg.py
@@ -157,52 +157,6 @@ def _get_second_matrix(draw):
# ------------ #
-# qr
-@handle_frontend_test(
- fn_tree="tensorflow.linalg.qr",
- dtype_and_x=helpers.dtype_and_values(
- available_dtypes=helpers.get_dtypes("float"),
- min_value=0,
- max_value=10,
- shape=helpers.ints(min_value=2, max_value=5).map(lambda x: tuple([x, x])),
- ),
-)
-def test_qr(
- *,
- dtype_and_x,
- frontend,
- test_flags,
- fn_tree,
- on_device,
- backend_fw,
-):
- dtype, x = dtype_and_x
- x = np.asarray(x[0], dtype=dtype[0])
- x = np.matmul(x.T, x) + np.identity(x.shape[0]) * 1e-3
- ret, frontend_ret = helpers.test_frontend_function(
- input_dtypes=dtype,
- backend_to_test=backend_fw,
- frontend=frontend,
- test_flags=test_flags,
- fn_tree=fn_tree,
- on_device=on_device,
- test_values=False,
- atol=1e-03,
- rtol=1e-05,
- input=x,
- )
- ret = [ivy.to_numpy(x) for x in ret]
- frontend_ret = [np.asarray(x) for x in frontend_ret]
-
- assert_all_close(
- ret_np=ret[0],
- ret_from_gt_np=frontend_ret[0],
- rtol=1e-2,
- atol=1e-2,
- ground_truth_backend=frontend,
- )
-
-
# adjoint
@handle_frontend_test(
fn_tree="tensorflow.linalg.adjoint",
@@ -937,6 +891,52 @@ def test_tensorflow_pinv(
)
+# qr
+@handle_frontend_test(
+ fn_tree="tensorflow.linalg.qr",
+ dtype_and_x=helpers.dtype_and_values(
+ available_dtypes=helpers.get_dtypes("float"),
+ min_value=0,
+ max_value=10,
+ shape=helpers.ints(min_value=2, max_value=5).map(lambda x: tuple([x, x])),
+ ),
+)
+def test_tensorflow_qr(
+ *,
+ dtype_and_x,
+ frontend,
+ test_flags,
+ fn_tree,
+ on_device,
+ backend_fw,
+):
+ dtype, x = dtype_and_x
+ x = np.asarray(x[0], dtype=dtype[0])
+ x = np.matmul(x.T, x) + np.identity(x.shape[0]) * 1e-3
+ ret, frontend_ret = helpers.test_frontend_function(
+ input_dtypes=dtype,
+ backend_to_test=backend_fw,
+ frontend=frontend,
+ test_flags=test_flags,
+ fn_tree=fn_tree,
+ on_device=on_device,
+ test_values=False,
+ atol=1e-03,
+ rtol=1e-05,
+ input=x,
+ )
+ ret = [ivy.to_numpy(x) for x in ret]
+ frontend_ret = [np.asarray(x) for x in frontend_ret]
+
+ assert_all_close(
+ ret_np=ret[0],
+ ret_from_gt_np=frontend_ret[0],
+ rtol=1e-2,
+ atol=1e-2,
+ ground_truth_backend=frontend,
+ )
+
+
# Tests for tensorflow.linalg.set_diag function's frontend
@handle_frontend_test(
fn_tree="tensorflow.linalg.set_diag",
diff --git a/ivy_tests/test_ivy/test_frontends/test_tensorflow/test_math.py b/ivy_tests/test_ivy/test_frontends/test_tensorflow/test_math.py
index 0c1b908abde9d..21fbc1c7a8000 100644
--- a/ivy_tests/test_ivy/test_frontends/test_tensorflow/test_math.py
+++ b/ivy_tests/test_ivy/test_frontends/test_tensorflow/test_math.py
@@ -1478,6 +1478,37 @@ def test_tensorflow_less_equal(
)
+# lgamma
+@handle_frontend_test(
+ fn_tree="tensorflow.math.lgamma",
+ dtype_and_x=helpers.dtype_and_values(
+ available_dtypes=helpers.get_dtypes("valid"),
+ safety_factor_scale="log",
+ ),
+ test_with_out=st.just(False),
+)
+def test_tensorflow_lgamma(
+ *,
+ dtype_and_x,
+ on_device,
+ fn_tree,
+ backend_fw,
+ frontend,
+ test_flags,
+):
+ input_dtype, xs = dtype_and_x
+ helpers.test_frontend_function(
+ input_dtypes=input_dtype,
+ frontend=frontend,
+ backend_to_test=backend_fw,
+ test_flags=test_flags,
+ fn_tree=fn_tree,
+ on_device=on_device,
+ rtol=1e-04,
+ x=xs[0],
+ )
+
+
# log
@handle_frontend_test(
fn_tree="tensorflow.math.log",
@@ -3072,6 +3103,38 @@ def test_tensorflow_unsorted_segment_mean(
)
+# unsorted_segment_min
+@handle_frontend_test(
+ fn_tree="tensorflow.math.unsorted_segment_min",
+ data=helpers.array_values(dtype=ivy.int32, shape=(5, 6), min_value=1, max_value=9),
+ segment_ids=helpers.array_values(
+ dtype=ivy.int32, shape=(5,), min_value=0, max_value=4
+ ),
+ test_with_out=st.just(False),
+)
+def test_tensorflow_unsorted_segment_min(
+ *,
+ data,
+ segment_ids,
+ frontend,
+ test_flags,
+ fn_tree,
+ backend_fw,
+ on_device,
+):
+ helpers.test_frontend_function(
+ input_dtypes=["int32", "int64"],
+ frontend=frontend,
+ backend_to_test=backend_fw,
+ test_flags=test_flags,
+ fn_tree=fn_tree,
+ on_device=on_device,
+ data=data,
+ segment_ids=segment_ids,
+ num_segments=np.max(segment_ids) + 1,
+ )
+
+
# unsorted_segment_sqrt_n
@handle_frontend_test(
fn_tree="tensorflow.math.unsorted_segment_sqrt_n",
diff --git a/ivy_tests/test_ivy/test_frontends/test_tensorflow/test_nn.py b/ivy_tests/test_ivy/test_frontends/test_tensorflow/test_nn.py
index d317cf5d90ca5..c90ef72de9b48 100644
--- a/ivy_tests/test_ivy/test_frontends/test_tensorflow/test_nn.py
+++ b/ivy_tests/test_ivy/test_frontends/test_tensorflow/test_nn.py
@@ -1497,6 +1497,40 @@ def test_tensorflow_max_pool2d(
)
+# max_pool3d
+@handle_frontend_test(
+ fn_tree="tensorflow.nn.max_pool3d",
+ data_format=st.sampled_from(["NDHWC", "NCDHW"]),
+ x_k_s_p=helpers.arrays_for_pooling(min_dims=5, max_dims=5, min_side=1, max_side=4),
+ test_with_out=st.just(False),
+)
+def test_tensorflow_max_pool3d(
+ *,
+ x_k_s_p,
+ data_format,
+ frontend,
+ test_flags,
+ fn_tree,
+ backend_fw,
+ on_device,
+):
+ input_dtype, x, ksize, strides, padding = x_k_s_p
+ data_format = data_format
+ helpers.test_frontend_function(
+ input_dtypes=input_dtype,
+ backend_to_test=backend_fw,
+ frontend=frontend,
+ test_flags=test_flags,
+ fn_tree=fn_tree,
+ on_device=on_device,
+ input=x[0],
+ ksize=ksize,
+ strides=strides,
+ padding=padding,
+ data_format=data_format,
+ )
+
+
# moments
@handle_frontend_test(
fn_tree="tensorflow.nn.moments",
diff --git a/ivy_tests/test_ivy/test_frontends/test_tensorflow/test_raw_ops.py b/ivy_tests/test_ivy/test_frontends/test_tensorflow/test_raw_ops.py
index eccdd054aa54a..b3fe2d66ab1ba 100644
--- a/ivy_tests/test_ivy/test_frontends/test_tensorflow/test_raw_ops.py
+++ b/ivy_tests/test_ivy/test_frontends/test_tensorflow/test_raw_ops.py
@@ -73,7 +73,7 @@ def _arrays_idx_n_dtypes(draw):
size=num_arrays,
)
)
- xs = list()
+ xs = []
input_dtypes = draw(
helpers.array_dtypes(
available_dtypes=draw(helpers.get_dtypes("float")), shared_dtype=True
@@ -276,6 +276,12 @@ def _squeeze_helper(draw):
return [axis] if axis is not None else axis
+@st.composite
+def df(draw, data_format):
+ data_format = draw(data_format)
+ return data_format
+
+
# Reverse
@st.composite
def reverse_helper(draw):
@@ -1193,6 +1199,35 @@ def test_tensorflow_ConcatV2(
)
+# Conj
+@handle_frontend_test(
+ fn_tree="tensorflow.raw_ops.Conj",
+ dtype_and_xs=helpers.dtype_and_values(
+ available_dtypes=helpers.get_dtypes("complex"),
+ ),
+ test_with_out=st.just(False),
+)
+def test_tensorflow_Conj( # NOQA
+ *,
+ dtype_and_xs,
+ frontend,
+ test_flags,
+ fn_tree,
+ backend_fw,
+ on_device,
+):
+ input_dtype, xs = dtype_and_xs
+ helpers.test_frontend_function(
+ input_dtypes=input_dtype,
+ backend_to_test=backend_fw,
+ frontend=frontend,
+ test_flags=test_flags,
+ fn_tree=fn_tree,
+ on_device=on_device,
+ input=xs[0],
+ )
+
+
# Conv2D
@handle_frontend_test(
fn_tree="tensorflow.raw_ops.Conv2D",
@@ -1856,6 +1891,45 @@ def test_tensorflow_FFT2D(
)
+# FFT3D
+@handle_frontend_test(
+ fn_tree="tensorflow.raw_ops.FFT3D",
+ dtype_and_x=helpers.dtype_and_values(
+ available_dtypes=helpers.get_dtypes("complex"),
+ min_value=-1e5,
+ max_value=1e5,
+ min_num_dims=3,
+ max_num_dims=5,
+ min_dim_size=2,
+ max_dim_size=5,
+ large_abs_safety_factor=2.5,
+ small_abs_safety_factor=2.5,
+ safety_factor_scale="log",
+ ),
+)
+def test_tensorflow_FFT3D(
+ *,
+ dtype_and_x,
+ frontend,
+ test_flags,
+ fn_tree,
+ backend_fw,
+ on_device,
+):
+ dtype, x = dtype_and_x
+ helpers.test_frontend_function(
+ input_dtypes=dtype,
+ backend_to_test=backend_fw,
+ frontend=frontend,
+ test_flags=test_flags,
+ fn_tree=fn_tree,
+ on_device=on_device,
+ input=x[0],
+ rtol=1e-02,
+ atol=1e-02,
+ )
+
+
# fill
@handle_frontend_test(
fn_tree="tensorflow.raw_ops.Fill",
@@ -2769,6 +2843,41 @@ def test_tensorflow_Max( # NOQA
)
+# MaxPool3D
+@handle_frontend_test(
+ fn_tree="tensorflow.raw_ops.MaxPool3D",
+ aliases=["tensorflow.nn.max_pool3d"],
+ data_format=st.sampled_from(["NDHWC", "NCDHW"]),
+ x_k_s_p=helpers.arrays_for_pooling(min_dims=5, max_dims=5, min_side=1, max_side=5),
+ test_with_out=st.just(False),
+)
+def test_tensorflow_MaxPool3D(
+ *,
+ x_k_s_p,
+ data_format,
+ frontend,
+ test_flags,
+ fn_tree,
+ backend_fw,
+ on_device,
+):
+ input_dtype, x, ksize, strides, padding = x_k_s_p
+ data_format = data_format
+ helpers.test_frontend_function(
+ input_dtypes=input_dtype,
+ backend_to_test=backend_fw,
+ frontend=frontend,
+ test_flags=test_flags,
+ fn_tree=fn_tree,
+ on_device=on_device,
+ input=x[0],
+ ksize=ksize,
+ strides=strides,
+ padding=padding,
+ data_format=data_format,
+ )
+
+
# Maximum
@handle_frontend_test(
fn_tree="tensorflow.raw_ops.Maximum",
@@ -2911,6 +3020,38 @@ def test_tensorflow_Minimum( # NOQA
)
+# Mod
+@handle_frontend_test(
+ fn_tree="tensorflow.raw_ops.Mod",
+ dtype_and_x=helpers.dtype_and_values(
+ available_dtypes=helpers.get_dtypes("float"),
+ num_arrays=2,
+ shared_dtype=True,
+ ),
+ test_with_out=st.just(False),
+)
+def test_tensorflow_Mod( # NOQA
+ *,
+ dtype_and_x,
+ frontend,
+ test_flags,
+ fn_tree,
+ backend_fw,
+ on_device,
+):
+ input_dtype, xs = dtype_and_x
+ helpers.test_frontend_function(
+ input_dtypes=input_dtype,
+ backend_to_test=backend_fw,
+ frontend=frontend,
+ test_flags=test_flags,
+ fn_tree=fn_tree,
+ on_device=on_device,
+ x=xs[0],
+ y=xs[1],
+ )
+
+
@handle_frontend_test(
fn_tree="tensorflow.raw_ops.Mul",
dtype_and_x=helpers.dtype_and_values(
diff --git a/ivy_tests/test_ivy/test_frontends/test_tensorflow/test_tensor.py b/ivy_tests/test_ivy/test_frontends/test_tensorflow/test_tensor.py
index 30655e5736998..66acd2020de79 100644
--- a/ivy_tests/test_ivy/test_frontends/test_tensorflow/test_tensor.py
+++ b/ivy_tests/test_ivy/test_frontends/test_tensorflow/test_tensor.py
@@ -916,7 +916,7 @@ def test_tensorflow__pow__(
on_device,
):
input_dtype, x = dtype_and_x
- if x[1].dtype == "int32" or x[1].dtype == "int64":
+ if x[1].dtype in ["int32", "int64"]:
if x[1].ndim == 0:
if x[1] < 0:
x[1] *= -1
@@ -1470,7 +1470,7 @@ def test_tensorflow__xor__(
available_dtypes=helpers.get_dtypes("valid", prune_function=False)
),
)
-def test_tensorflow_tensor_device(
+def test_tensorflow_device(
dtype_x,
backend_fw,
):
@@ -1487,7 +1487,7 @@ def test_tensorflow_tensor_device(
available_dtypes=helpers.get_dtypes("valid", prune_function=False),
),
)
-def test_tensorflow_tensor_dtype(
+def test_tensorflow_dtype(
dtype_x,
backend_fw,
):
@@ -1508,7 +1508,7 @@ def test_tensorflow_tensor_dtype(
min_dim_size=1,
),
)
-def test_tensorflow_tensor_get_shape(
+def test_tensorflow_get_shape(
dtype_and_x,
frontend,
frontend_method_data,
@@ -1539,7 +1539,7 @@ def test_tensorflow_tensor_get_shape(
available_dtypes=helpers.get_dtypes("valid", prune_function=False)
),
)
-def test_tensorflow_tensor_ivy_array(
+def test_tensorflow_ivy_array(
dtype_x,
backend_fw,
):
@@ -1565,7 +1565,7 @@ def test_tensorflow_tensor_ivy_array(
max_num_dims=5,
),
)
-def test_tensorflow_tensor_set_shape(
+def test_tensorflow_set_shape(
dtype_and_x,
frontend,
frontend_method_data,
@@ -1595,7 +1595,7 @@ def test_tensorflow_tensor_set_shape(
ret_shape=True,
),
)
-def test_tensorflow_tensor_shape(
+def test_tensorflow_shape(
dtype_x,
backend_fw,
):
diff --git a/ivy_tests/test_ivy/test_frontends/test_tensorflow/test_tensorarray.py b/ivy_tests/test_ivy/test_frontends/test_tensorflow/test_tensorarray.py
new file mode 100644
index 0000000000000..fe1f72681a7b5
--- /dev/null
+++ b/ivy_tests/test_ivy/test_frontends/test_tensorflow/test_tensorarray.py
@@ -0,0 +1,308 @@
+# global
+from hypothesis import strategies as st, given
+import numpy as np
+import tensorflow as tf
+
+# local
+import ivy_tests.test_ivy.helpers as helpers
+from ivy_tests.test_ivy.helpers import BackendHandler
+
+
+# --- Helpers --- #
+# --------------- #
+
+
+def _helper_init_tensorarray(backend_fw, l_kwargs, fn=None):
+ id_write, kwargs = l_kwargs
+ with BackendHandler.update_backend(backend_fw) as ivy_backend:
+ local_importer = ivy_backend.utils.dynamic_import
+ tf_frontend = local_importer.import_module(
+ "ivy.functional.frontends.tensorflow"
+ )
+ ta = tf_frontend.tensor.TensorArray(**kwargs)
+ ta_gt = tf.TensorArray(**kwargs)
+ if fn == "unstack":
+ ta_gt = ta_gt.unstack(tf.constant(id_write))
+ ta = ta.unstack(tf_frontend.constant(id_write))
+ elif fn == "split":
+ ta_gt = ta_gt.split(**id_write)
+ ta = ta.split(**id_write)
+ elif fn == "scatter":
+ indices, value = [*zip(*id_write)]
+ ta_gt = ta_gt.scatter(indices, tf.cast(tf.stack(value), dtype=ta_gt.dtype))
+ value = tf_frontend.stack(list(map(tf_frontend.constant, value)))
+ ta = ta.scatter(indices, tf_frontend.cast(value, ta.dtype))
+ else:
+ for id, write in id_write:
+ ta_gt = ta_gt.write(id, tf.constant(write))
+ ta = ta.write(id, tf_frontend.constant(write))
+ return ta_gt, ta
+
+
+@st.composite
+def _helper_random_tensorarray(draw, fn=None):
+ size = draw(st.integers(1, 10))
+ dynamic_size = draw(st.booleans())
+ clear_after_read = draw(st.booleans())
+ infer_shape = draw(st.booleans())
+ element_shape = draw(helpers.get_shape())
+ element_shape = draw(st.one_of(st.just(None), st.just(element_shape)))
+ shape = None
+ if (
+ infer_shape
+ or element_shape is not None
+ or fn in ["scatter", "stack", "gather", "concat"]
+ ):
+ if fn == "concat":
+ element_shape = None
+ infer_shape = False
+ shape = list(draw(helpers.get_shape(min_num_dims=1)))
+ elif element_shape is None:
+ shape = draw(helpers.get_shape())
+ else:
+ shape = element_shape
+ dtype = draw(helpers.get_dtypes(full=False, prune_function=False))[0]
+ if fn in ["stack", "concat"]:
+ ids_to_write = [True for i in range(size)]
+ else:
+ ids_to_write = [draw(st.booleans()) for i in range(size)]
+ if sum(ids_to_write) == 0:
+ ids_to_write[draw(st.integers(0, size - 1))] = True
+ kwargs = {
+ "dtype": dtype,
+ "size": size,
+ "dynamic_size": dynamic_size,
+ "clear_after_read": clear_after_read,
+ "infer_shape": infer_shape,
+ "element_shape": element_shape,
+ }
+ id_write = []
+ for id, flag in enumerate(ids_to_write):
+ if fn == "concat":
+ shape[0] = draw(st.integers(1, 10))
+ if flag:
+ write = np.array(
+ draw(
+ helpers.array_values(
+ dtype=dtype,
+ shape=shape if shape is not None else helpers.get_shape(),
+ )
+ )
+ )
+ id_write.append((id, write))
+ if fn != "gather":
+ return id_write, kwargs
+ else:
+ ids = []
+ for id, _ in id_write:
+ if draw(st.booleans()):
+ ids.append(id)
+ if not ids:
+ ids.append(id)
+ return id_write, kwargs, ids
+
+
+@st.composite
+def _helper_split(draw):
+ shape = draw(helpers.get_shape(min_num_dims=1))
+ dtype = draw(helpers.get_dtypes(full=False, prune_function=False))[0]
+ value = draw(helpers.array_values(dtype=dtype, shape=shape))
+ dynamic_size = draw(st.booleans())
+ if dynamic_size:
+ size = draw(st.integers(1, shape[0] + 5))
+ else:
+ size = shape[0]
+ total = 0
+ length = []
+ for i in range(shape[0]):
+ length.append(draw(st.integers(0, shape[0] - total)))
+ total += length[-1]
+ if total != shape[0]:
+ length[-1] += shape[0] - total
+ return {"value": value, "lengths": length}, {
+ "dtype": dtype,
+ "size": size,
+ "dynamic_size": dynamic_size,
+ }
+
+
+@st.composite
+def _helper_unstack(draw):
+ shape = draw(helpers.get_shape(min_num_dims=1))
+ size = draw(st.integers(1, 10))
+ dynamic_size = draw(st.booleans()) if size >= shape[0] else True
+ dtype = draw(helpers.get_dtypes(full=False, prune_function=False))[0]
+ tensor = draw(helpers.array_values(dtype=dtype, shape=shape))
+ kwargs = {"dtype": dtype, "size": size, "dynamic_size": dynamic_size}
+ return tensor, kwargs
+
+
+# --- Main --- #
+# ------------ #
+
+
+@given(l_kwargs=_helper_random_tensorarray())
+def test_tensorflow_close(
+ l_kwargs,
+ backend_fw,
+):
+ ta_gt, ta = _helper_init_tensorarray(backend_fw, l_kwargs)
+ ta.close()
+ ta_gt.close()
+ assert np.array(ta.size()) == 0
+ assert np.array(ta_gt.size()) == 0
+
+
+@given(l_kwargs=_helper_random_tensorarray(fn="concat"))
+def test_tensorflow_concat(
+ l_kwargs,
+ backend_fw,
+):
+ ta_gt, ta = _helper_init_tensorarray(backend_fw, l_kwargs)
+ helpers.value_test(
+ ret_np_from_gt_flat=ta_gt.concat().numpy().flatten(),
+ ret_np_flat=np.array(ta.concat()).flatten(),
+ backend=backend_fw,
+ )
+
+
+@given(l_kwargs=_helper_random_tensorarray())
+def test_tensorflow_dtype(
+ l_kwargs,
+ backend_fw,
+):
+ ta_gt, ta = _helper_init_tensorarray(backend_fw, l_kwargs)
+ assert ta_gt.dtype == ta.dtype.ivy_dtype
+
+
+@given(l_kwargs=_helper_random_tensorarray())
+def test_tensorflow_dynamic_size(
+ l_kwargs,
+ backend_fw,
+):
+ ta_gt, ta = _helper_init_tensorarray(backend_fw, l_kwargs)
+ assert ta_gt.dynamic_size == ta.dynamic_size
+
+
+@given(l_kwargs=_helper_random_tensorarray())
+def test_tensorflow_element_shape(
+ l_kwargs,
+ backend_fw,
+):
+ ta_gt, ta = _helper_init_tensorarray(backend_fw, l_kwargs)
+ assert ta_gt.element_shape == ta.element_shape
+
+
+@given(l_kwargs=_helper_random_tensorarray())
+def test_tensorflow_flow(
+ l_kwargs,
+ backend_fw,
+):
+ ta_gt, ta = _helper_init_tensorarray(backend_fw, l_kwargs)
+ assert ta_gt.flow == ta.flow
+
+
+@given(l_kwargs=_helper_random_tensorarray(fn="gather"))
+def test_tensorflow_gather(
+ l_kwargs,
+ backend_fw,
+):
+ ta_gt, ta = _helper_init_tensorarray(backend_fw, l_kwargs[:2])
+ *_, indices = l_kwargs
+ helpers.value_test(
+ ret_np_from_gt_flat=ta_gt.gather(indices).numpy().flatten(),
+ ret_np_flat=np.array(ta.gather(indices)).flatten(),
+ backend=backend_fw,
+ )
+
+
+@given(l_kwargs=_helper_random_tensorarray())
+def test_tensorflow_handle(
+ l_kwargs,
+ backend_fw,
+):
+ ta_gt, ta = _helper_init_tensorarray(backend_fw, l_kwargs)
+ assert ta_gt.handle == ta.handle
+
+
+# test for read and write methods
+@given(l_kwargs=_helper_random_tensorarray())
+def test_tensorflow_read(
+ l_kwargs,
+ backend_fw,
+):
+ ta_gt, ta = _helper_init_tensorarray(backend_fw, l_kwargs)
+ id_read, _ = l_kwargs
+ for id, read in id_read:
+ helpers.value_test(
+ ret_np_from_gt_flat=ta_gt.read(id).numpy().flatten(),
+ ret_np_flat=np.array(ta.read(id)).flatten(),
+ backend=backend_fw,
+ )
+
+
+@given(l_kwargs=_helper_random_tensorarray(fn="scatter"))
+def test_tensorflow_scatter(
+ l_kwargs,
+ backend_fw,
+):
+ id_read, _ = l_kwargs
+ ta_gt, ta = _helper_init_tensorarray(backend_fw, l_kwargs, "scatter")
+ for id, read in id_read:
+ helpers.value_test(
+ ret_np_from_gt_flat=ta_gt.read(id).numpy().flatten(),
+ ret_np_flat=np.array(ta.read(id)).flatten(),
+ backend=backend_fw,
+ )
+
+
+@given(l_kwargs=_helper_random_tensorarray())
+def test_tensorflow_size(
+ l_kwargs,
+ backend_fw,
+):
+ ta_gt, ta = _helper_init_tensorarray(backend_fw, l_kwargs)
+ helpers.value_test(
+ ret_np_from_gt_flat=ta_gt.size().numpy().flatten(),
+ ret_np_flat=np.array(ta.size()).flatten(),
+ backend=backend_fw,
+ )
+
+
+@given(
+ kwargs_v_l=_helper_split(),
+)
+def test_tensorflow_split(kwargs_v_l, backend_fw):
+ ta_gt, ta = _helper_init_tensorarray(backend_fw, kwargs_v_l, "split")
+ for id in range(ta_gt.size()):
+ helpers.value_test(
+ ret_np_from_gt_flat=ta_gt.read(id).numpy().flatten(),
+ ret_np_flat=np.array(ta.read(id)).flatten(),
+ backend=backend_fw,
+ )
+
+
+@given(l_kwargs=_helper_random_tensorarray(fn="stack"))
+def test_tensorflow_stack(
+ l_kwargs,
+ backend_fw,
+):
+ ta_gt, ta = _helper_init_tensorarray(backend_fw, l_kwargs)
+ helpers.value_test(
+ ret_np_from_gt_flat=ta_gt.stack().numpy().flatten(),
+ ret_np_flat=np.array(ta.stack()).flatten(),
+ backend=backend_fw,
+ )
+
+
+@given(l_kwargs=_helper_unstack())
+def test_tensorflow_unstack(
+ l_kwargs,
+ backend_fw,
+):
+ ta_gt, ta = _helper_init_tensorarray(backend_fw, l_kwargs, "unstack")
+ helpers.value_test(
+ ret_np_from_gt_flat=ta_gt.stack().numpy().flatten(),
+ ret_np_flat=np.array(ta.stack()).flatten(),
+ backend=backend_fw,
+ )
diff --git a/ivy_tests/test_ivy/test_frontends/test_tensorflow/test_tensorshape.py b/ivy_tests/test_ivy/test_frontends/test_tensorflow/test_tensorshape.py
new file mode 100644
index 0000000000000..044f5601897e6
--- /dev/null
+++ b/ivy_tests/test_ivy/test_frontends/test_tensorflow/test_tensorshape.py
@@ -0,0 +1,80 @@
+# global
+from hypothesis import strategies as st
+
+# local
+import ivy
+import ivy_tests.test_ivy.helpers as helpers
+from ivy_tests.test_ivy.helpers import handle_frontend_method
+import pytest
+
+CLASS_TREE = "ivy.functional.frontends.tensorflow.tensor.TensorShape"
+
+
+# __add__
+@pytest.mark.skip("TODO: test needs implementing correctly")
+@handle_frontend_method(
+ class_tree=CLASS_TREE,
+ init_tree="tensorflow.TensorShape",
+ method_name="__add__",
+ shape_list=helpers.list_of_size(x=st.sampled_from([0, 1, 2, 3, 4]), size=3),
+ other_list=helpers.list_of_size(x=st.sampled_from([0, 1, 2, 3, 4]), size=3),
+)
+def test_tensorflow__add__(
+ shape_list,
+ other_list,
+ frontend,
+ frontend_method_data,
+ init_flags,
+ method_flags,
+ on_device,
+ backend_fw,
+):
+ helpers.test_frontend_method(
+ init_input_dtypes=[ivy.int64],
+ backend_to_test=backend_fw,
+ init_all_as_kwargs_np={
+ "dims": shape_list,
+ },
+ method_input_dtypes=[ivy.int64],
+ method_all_as_kwargs_np={
+ "other": other_list,
+ },
+ frontend=frontend,
+ frontend_method_data=frontend_method_data,
+ init_flags=init_flags,
+ method_flags=method_flags,
+ on_device=on_device,
+ )
+
+
+# __bool__
+@pytest.mark.skip("TODO: test needs implementing correctly")
+@handle_frontend_method(
+ class_tree=CLASS_TREE,
+ init_tree="tensorflow.TensorShape",
+ method_name="__bool__",
+ shape_list=helpers.list_of_size(x=st.sampled_from([0, 1, 2, 3, 4]), size=3),
+)
+def test_tensorflow__bool__(
+ shape_list,
+ frontend,
+ frontend_method_data,
+ init_flags,
+ method_flags,
+ backend_fw,
+ on_device,
+):
+ helpers.test_frontend_method(
+ init_input_dtypes=[ivy.int64],
+ backend_to_test=backend_fw,
+ init_all_as_kwargs_np={
+ "dims": shape_list,
+ },
+ method_input_dtypes=[ivy.int64],
+ method_all_as_kwargs_np={},
+ frontend=frontend,
+ frontend_method_data=frontend_method_data,
+ init_flags=init_flags,
+ method_flags=method_flags,
+ on_device=on_device,
+ )
diff --git a/ivy_tests/test_ivy/test_frontends/test_torch/test_creation_ops.py b/ivy_tests/test_ivy/test_frontends/test_torch/test_creation_ops.py
index d27dca99f63e2..859ddfa5df3cf 100644
--- a/ivy_tests/test_ivy/test_frontends/test_torch/test_creation_ops.py
+++ b/ivy_tests/test_ivy/test_frontends/test_torch/test_creation_ops.py
@@ -150,61 +150,6 @@ def _start_stop_step(draw):
# ------------ #
-# complex
-@handle_frontend_test(
- fn_tree="torch.complex",
- dtype_and_x=helpers.dtype_and_values(available_dtypes=helpers.get_dtypes("float")),
-)
-def test_complex(
- *,
- dtype_and_x,
- on_device,
- fn_tree,
- frontend,
- test_flags,
- backend_fw,
-):
- input_dtype, input = dtype_and_x
- helpers.test_frontend_function(
- input_dtypes=input_dtype,
- backend_to_test=backend_fw,
- frontend=frontend,
- test_flags=test_flags,
- fn_tree=fn_tree,
- on_device=on_device,
- real=input[0],
- imag=input[0],
- )
-
-
-# polar
-@handle_frontend_test(
- fn_tree="torch.polar",
- dtype_and_x=helpers.dtype_and_values(available_dtypes=helpers.get_dtypes("float")),
- test_with_out=st.just(False),
-)
-def test_polar(
- *,
- dtype_and_x,
- on_device,
- fn_tree,
- frontend,
- test_flags,
- backend_fw,
-):
- input_dtype, input = dtype_and_x
- helpers.test_frontend_function(
- input_dtypes=input_dtype,
- backend_to_test=backend_fw,
- frontend=frontend,
- test_flags=test_flags,
- fn_tree=fn_tree,
- on_device=on_device,
- abs=input[0],
- angle=input[0],
- )
-
-
# arange
@handle_frontend_test(
fn_tree="torch.arange",
@@ -318,6 +263,7 @@ def test_torch_as_tensor(
available_dtypes=helpers.get_dtypes("numeric")
),
dtype=helpers.get_dtypes("numeric", full=False),
+ test_with_copy=st.just(True),
)
def test_torch_asarray(
*,
@@ -343,6 +289,33 @@ def test_torch_asarray(
)
+# complex
+@handle_frontend_test(
+ fn_tree="torch.complex",
+ dtype_and_x=helpers.dtype_and_values(available_dtypes=helpers.get_dtypes("float")),
+)
+def test_torch_complex(
+ *,
+ dtype_and_x,
+ on_device,
+ fn_tree,
+ frontend,
+ test_flags,
+ backend_fw,
+):
+ input_dtype, input = dtype_and_x
+ helpers.test_frontend_function(
+ input_dtypes=input_dtype,
+ backend_to_test=backend_fw,
+ frontend=frontend,
+ test_flags=test_flags,
+ fn_tree=fn_tree,
+ on_device=on_device,
+ real=input[0],
+ imag=input[0],
+ )
+
+
# empty
@handle_frontend_test(
fn_tree="torch.empty",
@@ -777,6 +750,34 @@ def test_torch_ones_like(
)
+# polar
+@handle_frontend_test(
+ fn_tree="torch.polar",
+ dtype_and_x=helpers.dtype_and_values(available_dtypes=helpers.get_dtypes("float")),
+ test_with_out=st.just(False),
+)
+def test_torch_polar(
+ *,
+ dtype_and_x,
+ on_device,
+ fn_tree,
+ frontend,
+ test_flags,
+ backend_fw,
+):
+ input_dtype, input = dtype_and_x
+ helpers.test_frontend_function(
+ input_dtypes=input_dtype,
+ backend_to_test=backend_fw,
+ frontend=frontend,
+ test_flags=test_flags,
+ fn_tree=fn_tree,
+ on_device=on_device,
+ abs=input[0],
+ angle=input[0],
+ )
+
+
# range
@handle_frontend_test(
fn_tree="torch.range",
diff --git a/ivy_tests/test_ivy/test_frontends/test_torch/test_func_wrapper.py b/ivy_tests/test_ivy/test_frontends/test_torch/test_func_wrapper.py
index fad1aacd51cfd..4f731ff5d246c 100644
--- a/ivy_tests/test_ivy/test_frontends/test_torch/test_func_wrapper.py
+++ b/ivy_tests/test_ivy/test_frontends/test_torch/test_func_wrapper.py
@@ -18,10 +18,10 @@
# --------------- #
-def _fn(*args, dtype=None, check_default=False):
+def _fn(*args, dtype=None, check_default=False, inplace=False):
if (
check_default
- and all([not (ivy.is_array(i) or hasattr(i, "ivy_array")) for i in args])
+ and all(not (ivy.is_array(i) or hasattr(i, "ivy_array")) for i in args)
and not ivy.exists(dtype)
):
ivy.utils.assertions.check_equal(
@@ -107,26 +107,51 @@ def test_torch_numpy_to_torch_style_args(dim, keepdim, input, other):
available_dtypes=helpers.get_dtypes("valid", prune_function=False)
).filter(lambda x: "bfloat16" not in x[0]),
dtype=helpers.get_dtypes("valid", none=True, full=False, prune_function=False),
+ generate_type=st.sampled_from(["frontend", "ivy", "native"]),
+ inplace=st.booleans(),
)
-def test_torch_outputs_to_frontend_arrays(dtype_and_x, dtype, backend_fw):
+def test_torch_outputs_to_frontend_arrays(
+ dtype_and_x,
+ dtype,
+ generate_type,
+ inplace,
+ backend_fw,
+):
x_dtype, x = dtype_and_x
ivy.set_backend(backend_fw)
- # check for ivy array
- input_ivy = ivy.array(x[0], dtype=x_dtype[0])
- if not len(input_ivy.shape):
- scalar_input_ivy = ivy.to_scalar(input_ivy)
- outputs_to_frontend_arrays(_fn)(
- scalar_input_ivy, scalar_input_ivy, check_default=True, dtype=dtype
- )
+ x = ivy.array(x[0], dtype=x_dtype[0])
+ if generate_type == "frontend":
+ x = Tensor(x)
+ elif generate_type == "native":
+ x = x.data
+
+ if not len(x.shape):
+ scalar_x = ivy.to_scalar(x.ivy_array if isinstance(x, Tensor) else x)
outputs_to_frontend_arrays(_fn)(
- scalar_input_ivy, input_ivy, check_default=True, dtype=dtype
+ scalar_x, scalar_x, check_default=True, dtype=dtype
)
- output = outputs_to_frontend_arrays(_fn)(input_ivy, check_default=True, dtype=dtype)
+ outputs_to_frontend_arrays(_fn)(scalar_x, x, check_default=True, dtype=dtype)
+ output = outputs_to_frontend_arrays(_fn)(
+ x, check_default=True, dtype=dtype, inplace=inplace
+ )
assert isinstance(output, Tensor)
- assert str(input_ivy.dtype) == str(output.dtype)
- assert ivy.all(input_ivy == output.ivy_array)
+ if inplace:
+ if generate_type == "frontend":
+ assert x is output
+ elif generate_type == "native":
+ assert x is output.ivy_array.data
+ else:
+ assert x is output.ivy_array
+ else:
+ assert ivy.as_ivy_dtype(x.dtype) == ivy.as_ivy_dtype(output.dtype)
+ if generate_type == "frontend":
+ assert ivy.all(x.ivy_array == output.ivy_array)
+ elif generate_type == "native":
+ assert ivy.all(x == output.ivy_array.data)
+ else:
+ assert ivy.all(x == output.ivy_array)
assert ivy.default_float_dtype_stack == ivy.default_int_dtype_stack == []
diff --git a/ivy_tests/test_ivy/test_frontends/test_torch/test_indexing_slicing_joining_mutating_ops.py b/ivy_tests/test_ivy/test_frontends/test_torch/test_indexing_slicing_joining_mutating_ops.py
index 43fa078b04deb..f08400ec8e6c3 100644
--- a/ivy_tests/test_ivy/test_frontends/test_torch/test_indexing_slicing_joining_mutating_ops.py
+++ b/ivy_tests/test_ivy/test_frontends/test_torch/test_indexing_slicing_joining_mutating_ops.py
@@ -1,4 +1,6 @@
# global
+import random
+
from hypothesis import strategies as st
import math
@@ -6,13 +8,11 @@
# local
import ivy
import ivy_tests.test_ivy.helpers as helpers
+import ivy_tests.test_ivy.helpers.globals as test_globals
from ivy_tests.test_ivy.helpers import handle_frontend_test
from ivy_tests.test_ivy.test_functional.test_core.test_manipulation import _get_splits
-from ivy_tests.test_ivy.test_functional.test_core.test_searching import (
- _broadcastable_trio,
-)
-from ivy_tests.test_ivy.test_functional.test_core.test_manipulation import ( # noqa
- _get_splits,
+from ivy_tests.array_api_testing.test_array_api.array_api_tests import (
+ hypothesis_helpers as hh,
)
@@ -72,7 +72,7 @@ def _arrays_dim_idx_n_dtypes(draw):
)
)
- xs = list()
+ xs = []
available_input_types = draw(helpers.get_dtypes("numeric"))
available_input_types.remove("float16") # half summation unstable in backends
input_dtypes = draw(
@@ -130,7 +130,7 @@ def _arrays_dim_idx_n_dtypes_extend(
)
)
- xs = list()
+ xs = []
available_input_types = draw(helpers.get_dtypes(support_dtypes))
unstabled_dtypes = ["float16"]
@@ -182,7 +182,7 @@ def _arrays_idx_n_dtypes(draw):
size=num_arrays,
)
)
- xs = list()
+ xs = []
input_dtypes = draw(
helpers.array_dtypes(available_dtypes=draw(helpers.get_dtypes("float")))
)
@@ -218,6 +218,63 @@ def _chunk_helper(draw):
return dtype, x, axis, chunks
+# diagonal_scatter
+@st.composite
+def _diag_x_y_offset_axes(draw):
+ currentshape = random.randint(2, 4)
+
+ if test_globals.CURRENT_BACKEND == "paddle":
+ currentshape = 2
+
+ offset = draw(
+ helpers.ints(min_value=-(currentshape - 1), max_value=currentshape - 1)
+ )
+ available_input_types = draw(helpers.get_dtypes("float"))
+
+ available_input_types = helpers.array_dtypes(available_dtypes=available_input_types)
+
+ dtype, x = draw(
+ helpers.dtype_and_values(
+ min_num_dims=currentshape,
+ max_num_dims=currentshape,
+ min_dim_size=currentshape,
+ max_dim_size=currentshape,
+ num_arrays=1,
+ available_dtypes=available_input_types,
+ ),
+ )
+
+ diagonal_shape = draw(
+ helpers.get_shape(
+ min_num_dims=currentshape - 1,
+ max_num_dims=currentshape - 1,
+ min_dim_size=currentshape,
+ max_dim_size=currentshape,
+ ),
+ )
+ diagonal_shape = diagonal_shape[:-1] + (diagonal_shape[-1] - abs(offset),)
+ y = draw(
+ helpers.array_values(
+ shape=diagonal_shape,
+ dtype=available_input_types,
+ exclude_min=False,
+ )
+ )
+
+ prohibited_pairs = {(2, -1), (-2, 1), (1, -2), (-1, 2)}
+
+ axes = draw(
+ st.lists(
+ helpers.ints(min_value=-2, max_value=1), min_size=2, max_size=2, unique=True
+ ).filter(
+ lambda axes: (axes[0] % 2 != axes[1] % 2)
+ and tuple(axes) not in prohibited_pairs,
+ )
+ )
+
+ return dtype, x, y, offset, axes
+
+
@st.composite
def _dtype_input_dim_start_length(draw):
_shape = draw(helpers.get_shape(min_num_dims=1, min_dim_size=1))
@@ -278,6 +335,31 @@ def _dtypes_input_mask(draw):
return _dtype, _x, _mask
+@st.composite
+def _where_helper(draw):
+ shape_1, shape_2 = draw(hh.two_broadcastable_shapes())
+ dtype_x1, x1 = draw(
+ helpers.dtype_and_values(
+ available_dtypes=helpers.get_dtypes("valid"),
+ shape=shape_1,
+ )
+ )
+ dtype_x2, x2 = draw(
+ helpers.dtype_and_values(
+ available_dtypes=helpers.get_dtypes("valid"),
+ shape=shape_1,
+ shared_dtype=True,
+ )
+ )
+ _, cond = draw(
+ helpers.dtype_and_values(
+ available_dtypes=["bool"],
+ shape=shape_2,
+ )
+ )
+ return ["bool", *dtype_x1, *dtype_x2], [cond[0], x1[0], x2[0]]
+
+
# reshape
@st.composite
def dtypes_x_reshape(draw):
@@ -495,6 +577,35 @@ def test_torch_conj(
)
+@handle_frontend_test(
+ fn_tree="torch.diagonal_scatter", dtype_and_values=_diag_x_y_offset_axes()
+)
+def test_torch_diagonal_scatter(
+ *,
+ dtype_and_values,
+ on_device,
+ fn_tree,
+ frontend,
+ test_flags,
+ backend_fw,
+):
+ input_dtype, value, src, offset, axes = dtype_and_values
+
+ helpers.test_frontend_function(
+ input_dtypes=input_dtype,
+ backend_to_test=backend_fw,
+ frontend=frontend,
+ test_flags=test_flags,
+ fn_tree=fn_tree,
+ on_device=on_device,
+ input=value[0],
+ src=src,
+ offset=offset,
+ dim1=axes[0],
+ dim2=axes[1],
+ )
+
+
# dsplit
@handle_frontend_test(
fn_tree="torch.dsplit",
@@ -1702,7 +1813,7 @@ def test_torch_vstack(
@handle_frontend_test(
fn_tree="torch.where",
- broadcastables=_broadcastable_trio(),
+ broadcastables=_where_helper(),
only_cond=st.booleans(),
)
def test_torch_where(
@@ -1715,7 +1826,7 @@ def test_torch_where(
backend_fw,
on_device,
):
- cond, xs, dtypes = broadcastables
+ dtypes, arrays = broadcastables
if only_cond:
helpers.test_frontend_function(
@@ -1725,18 +1836,18 @@ def test_torch_where(
test_flags=test_flags,
fn_tree=fn_tree,
on_device=on_device,
- condition=xs[0],
+ condition=arrays[0],
)
else:
helpers.test_frontend_function(
- input_dtypes=["bool"] + dtypes,
+ input_dtypes=dtypes,
+ backend_to_test=backend_fw,
frontend=frontend,
test_flags=test_flags,
fn_tree=fn_tree,
on_device=on_device,
- condition=cond,
- input=xs[0],
- other=xs[1],
- backend_to_test=backend_fw,
+ condition=arrays[0],
+ input=arrays[1],
+ other=arrays[2],
)
diff --git a/ivy_tests/test_ivy/test_frontends/test_torch/test_linalg.py b/ivy_tests/test_ivy/test_frontends/test_torch/test_linalg.py
index 45c624d17c354..40e88dbcda2cc 100644
--- a/ivy_tests/test_ivy/test_frontends/test_torch/test_linalg.py
+++ b/ivy_tests/test_ivy/test_frontends/test_torch/test_linalg.py
@@ -63,7 +63,7 @@ def _generate_multi_dot_dtype_and_arrays(draw):
@st.composite
def _get_axis_and_p(draw):
p = draw(st.sampled_from(["fro", "nuc", 1, 2, -1, -2, float("inf"), -float("inf")]))
- if p == "fro" or p == "nuc":
+ if p in ["fro", "nuc"]:
max_axes_size = 2
min_axes_size = 2
else:
@@ -1156,10 +1156,12 @@ def test_torch_svd(
@handle_frontend_test(
fn_tree="torch.linalg.svdvals",
dtype_and_x=_get_dtype_and_matrix(batch=True),
+ driver=st.sampled_from([None, "gesvd", "gesvdj", "gesvda"]),
)
def test_torch_svdvals(
*,
dtype_and_x,
+ driver,
on_device,
fn_tree,
frontend,
@@ -1174,6 +1176,7 @@ def test_torch_svdvals(
test_flags=test_flags,
fn_tree=fn_tree,
on_device=on_device,
+ driver=driver,
A=x[0],
)
diff --git a/ivy_tests/test_ivy/test_frontends/test_torch/test_miscellaneous_ops.py b/ivy_tests/test_ivy/test_frontends/test_torch/test_miscellaneous_ops.py
index 992c6a7689d1e..340fe6bed6930 100644
--- a/ivy_tests/test_ivy/test_frontends/test_torch/test_miscellaneous_ops.py
+++ b/ivy_tests/test_ivy/test_frontends/test_torch/test_miscellaneous_ops.py
@@ -516,6 +516,7 @@ def test_torch_cartesian_prod(
dtype_and_x=helpers.dtype_and_values(
available_dtypes=helpers.get_dtypes("valid"),
),
+ test_with_copy=st.just(True),
)
def test_torch_clone(
*,
@@ -944,12 +945,10 @@ def test_torch_diff(
@handle_frontend_test(
fn_tree="torch.einsum",
eq_n_op_n_shp=helpers.einsum_helper(),
- dtype=helpers.get_dtypes("numeric", full=False),
)
def test_torch_einsum(
*,
eq_n_op_n_shp,
- dtype,
on_device,
fn_tree,
frontend,
@@ -959,7 +958,7 @@ def test_torch_einsum(
eq, operands, dtypes = eq_n_op_n_shp
kw = {}
for i, x_ in enumerate(operands):
- dtype = dtypes[i][0]
+ dtype = dtypes[i]
kw[f"x{i}"] = np.array(x_).astype(dtype)
test_flags.num_positional_args = len(operands) + 1
helpers.test_frontend_function(
@@ -1024,6 +1023,7 @@ def test_torch_flatten(
shape=st.shared(helpers.get_shape(min_num_dims=1), key="shape"),
force_tuple=True,
),
+ test_with_copy=st.just(True),
)
def test_torch_flip(
*,
@@ -1055,6 +1055,7 @@ def test_torch_flip(
available_dtypes=helpers.get_dtypes("float"),
shape=helpers.get_shape(min_num_dims=2),
),
+ test_with_copy=st.just(True),
)
def test_torch_fliplr(
*,
@@ -1084,6 +1085,7 @@ def test_torch_fliplr(
available_dtypes=helpers.get_dtypes("float"),
shape=helpers.get_shape(min_num_dims=1),
),
+ test_with_copy=st.just(True),
)
def test_torch_flipud(
*,
@@ -1814,10 +1816,12 @@ def test_torch_view_as_real(
fn_tree,
frontend,
test_flags,
+ backend_fw,
):
input_dtype, x = dtype_and_x
helpers.test_frontend_function(
input_dtypes=input_dtype,
+ backend_to_test=backend_fw,
frontend=frontend,
test_flags=test_flags,
fn_tree=fn_tree,
diff --git a/ivy_tests/test_ivy/test_frontends/test_torch/test_nn/test_functional/test_convolution_functions.py b/ivy_tests/test_ivy/test_frontends/test_torch/test_nn/test_functional/test_convolution_functions.py
index d2e95a4269d04..d61a1440719e1 100644
--- a/ivy_tests/test_ivy/test_frontends/test_torch/test_nn/test_functional/test_convolution_functions.py
+++ b/ivy_tests/test_ivy/test_frontends/test_torch/test_nn/test_functional/test_convolution_functions.py
@@ -3,7 +3,6 @@
from hypothesis import strategies as st, assume
# local
-import ivy
import ivy_tests.test_ivy.helpers as helpers
from ivy_tests.test_ivy.helpers import handle_frontend_test
from ivy_tests.test_ivy.test_functional.test_nn.test_layers import (
@@ -44,7 +43,7 @@ def _fold_helper(draw, dim=2):
)
)
if vals.shape[0] == 1: # un-batched inputs are also supported
- vals = draw(st.one_of(st.just(vals), st.just(ivy.squeeze(vals, axis=0))))
+ vals = draw(st.sampled_from([vals, vals[0]]))
return dtype, vals, kernel_size, output_shape, dilation, stride, padding
diff --git a/ivy_tests/test_ivy/test_frontends/test_torch/test_nn/test_functional/test_layer_functions.py b/ivy_tests/test_ivy/test_frontends/test_torch/test_nn/test_functional/test_layer_functions.py
new file mode 100644
index 0000000000000..32f7508490e1b
--- /dev/null
+++ b/ivy_tests/test_ivy/test_frontends/test_torch/test_nn/test_functional/test_layer_functions.py
@@ -0,0 +1,281 @@
+# global
+from hypothesis import assume, strategies as st
+import numpy as np
+
+# local
+import ivy
+from ivy.functional.ivy.layers import _get_embed_dim
+from ivy.functional.frontends.torch.nn.functional.layer_functions import (
+ _pack_padded_sequence,
+)
+from ivy_tests.test_ivy import helpers
+from ivy_tests.test_ivy.helpers import handle_frontend_test
+from ivy_tests.test_ivy.test_functional.test_nn.test_layers import _mha_helper
+
+
+# --- Helpers --- #
+# --------------- #
+
+
+@st.composite
+def _lstm_helper(draw):
+ dtype = draw(helpers.get_dtypes("valid", full=False))
+
+ has_biases = draw(st.booleans())
+ bidirectional = draw(st.booleans())
+ dropout = draw(st.floats(min_value=0, max_value=0.99))
+ train = (
+ draw(st.booleans()) and not dropout
+ ) # not yet supported by original function
+ packed = draw(st.booleans())
+
+ batch_first = draw(st.booleans()) and not packed
+ num_batches = draw(st.integers(min_value=1, max_value=5))
+ num_layers = draw(st.integers(min_value=1, max_value=3))
+ num_directions = 2 if bidirectional else 1
+ seq_size = draw(st.integers(min_value=1, max_value=5))
+ in_size = draw(st.integers(min_value=1, max_value=3))
+ hidden_size = draw(st.integers(min_value=1, max_value=3))
+
+ input = draw(
+ helpers.array_values(
+ dtype=dtype[0],
+ shape=(
+ (num_batches, seq_size, in_size)
+ if batch_first
+ else (seq_size, num_batches, in_size)
+ ),
+ min_value=0,
+ max_value=1,
+ )
+ )
+
+ init_h = draw(
+ helpers.array_values(
+ dtype=dtype[0],
+ shape=(num_directions * num_layers, num_batches, hidden_size),
+ min_value=0,
+ max_value=1,
+ )
+ )
+ init_c = draw(
+ helpers.array_values(
+ dtype=dtype[0],
+ shape=(num_directions * num_layers, num_batches, hidden_size),
+ min_value=0,
+ max_value=1,
+ )
+ )
+
+ all_weights = []
+ for k in range(num_layers):
+ for _ in range(num_directions):
+ weight_ih = draw(
+ helpers.array_values(
+ dtype=dtype[0],
+ shape=(
+ (4 * hidden_size, in_size)
+ if k == 0
+ else (4 * hidden_size, num_directions * hidden_size)
+ ),
+ min_value=0,
+ max_value=1,
+ )
+ )
+ weight_hh = draw(
+ helpers.array_values(
+ dtype=dtype[0],
+ shape=(4 * hidden_size, hidden_size),
+ min_value=0,
+ max_value=1,
+ )
+ )
+ all_weights += [weight_ih, weight_hh]
+ if has_biases:
+ bias_ih = draw(
+ helpers.array_values(
+ dtype=dtype[0],
+ shape=(4 * hidden_size,),
+ min_value=0,
+ max_value=1,
+ )
+ )
+ bias_hh = draw(
+ helpers.array_values(
+ dtype=dtype[0],
+ shape=(4 * hidden_size,),
+ min_value=0,
+ max_value=1,
+ )
+ )
+ all_weights += [bias_ih, bias_hh]
+
+ if packed:
+ batch_sizes = [seq_size]
+ batch_sizes += draw(
+ st.lists(
+ st.integers(min_value=1, max_value=seq_size),
+ min_size=num_batches - 1,
+ max_size=num_batches - 1,
+ )
+ )
+ batch_sizes = np.array(draw(st.permutations(batch_sizes)))
+ input, batch_sizes = [
+ ivy.to_numpy(p) for p in _pack_padded_sequence(input, batch_sizes)
+ ]
+ else:
+ batch_sizes = None
+
+ initial_states = init_h, init_c
+ all_weights = tuple(all_weights)
+ if batch_sizes is not None:
+ dtypes = dtype + ["int64"]
+ kwargs = {
+ "data": input,
+ "batch_sizes": batch_sizes,
+ "hx": initial_states,
+ "params": all_weights,
+ "has_biases": has_biases,
+ "num_layers": num_layers,
+ "dropout": dropout,
+ "train": train,
+ "bidirectional": bidirectional,
+ }
+ else:
+ dtypes = dtype
+ kwargs = {
+ "input": input,
+ "hx": initial_states,
+ "params": all_weights,
+ "has_biases": has_biases,
+ "num_layers": num_layers,
+ "dropout": dropout,
+ "train": train,
+ "bidirectional": bidirectional,
+ "batch_first": batch_first,
+ }
+ return dtypes, kwargs
+
+
+# --- Main --- #
+# ------------ #
+
+
+# lstm
+@handle_frontend_test(
+ fn_tree="torch.lstm",
+ dtypes_kwargs=_lstm_helper(),
+ test_with_out=st.just(False),
+)
+def test_torch_lstm(
+ *,
+ dtypes_kwargs,
+ on_device,
+ fn_tree,
+ frontend,
+ test_flags,
+ backend_fw,
+):
+ dtypes, kwargs = dtypes_kwargs
+ # Todo: Debug the function to have this case passing as well
+ assume("batch_sizes" not in kwargs or not kwargs["bidirectional"])
+ helpers.test_frontend_function(
+ input_dtypes=dtypes,
+ backend_to_test=backend_fw,
+ frontend=frontend,
+ test_flags=test_flags,
+ fn_tree=fn_tree,
+ on_device=on_device,
+ **kwargs,
+ )
+
+
+# multi_head_attention_forward
+@handle_frontend_test(
+ fn_tree="torch.nn.functional.multi_head_attention_forward",
+ dtype_mha_args=_mha_helper(same_pre_embed_dim=True, batch_second=True).filter(
+ lambda args: args[10] is not None
+ and (not args[22] or args[5] is not None)
+ and len(set(_get_embed_dim(*args[6:10], args[1]))) == 1
+ ),
+ test_with_out=st.just(False),
+)
+def test_torch_multi_head_attention_forward(
+ *,
+ on_device,
+ fn_tree,
+ frontend,
+ test_flags,
+ dtype_mha_args,
+ backend_fw,
+):
+ (
+ dtype,
+ q,
+ k,
+ v,
+ heads,
+ attn_mask,
+ in_proj_weight,
+ q_proj_weight,
+ k_proj_weight,
+ v_proj_weight,
+ out_proj_weight,
+ in_proj_bias,
+ out_proj_bias,
+ key_padding_mask,
+ bias_k,
+ bias_v,
+ static_k,
+ static_v,
+ _,
+ add_zero_attn,
+ dropout_p,
+ training,
+ is_causal,
+ need_weights,
+ average_attn_weights,
+ batch_first,
+ ) = dtype_mha_args
+ if k is None and v is None:
+ k = v = q
+ # re-order the dtypes to match the order of the frontend arguments, not the order
+ # of ivy.multi_head_attention's arguments given by _mha_helper
+ kwargs = {
+ "query": q,
+ "key": k,
+ "value": v,
+ "embed_dim_to_check": q.shape[-1],
+ "num_heads": heads,
+ "in_proj_weight": in_proj_weight,
+ "in_proj_bias": in_proj_bias,
+ "bias_k": bias_k,
+ "bias_v": bias_v,
+ "add_zero_attn": add_zero_attn,
+ "dropout_p": dropout_p,
+ "out_proj_weight": out_proj_weight,
+ "out_proj_bias": out_proj_bias,
+ "training": training,
+ "key_padding_mask": key_padding_mask,
+ "need_weights": need_weights,
+ "attn_mask": attn_mask,
+ "use_separate_proj_weight": in_proj_weight is None,
+ "q_proj_weight": q_proj_weight,
+ "k_proj_weight": k_proj_weight,
+ "v_proj_weight": v_proj_weight,
+ "static_k": static_k,
+ "static_v": static_v,
+ "average_attn_weights": average_attn_weights,
+ "is_causal": is_causal,
+ }
+ helpers.test_frontend_function(
+ input_dtypes=[str(r.dtype) for r in kwargs.values() if ivy.is_array(r)],
+ backend_to_test=backend_fw,
+ frontend=frontend,
+ test_flags=test_flags,
+ fn_tree=fn_tree,
+ atol=1e-03,
+ on_device=on_device,
+ test_values=not training or dropout_p == 0.0,
+ **kwargs,
+ )
diff --git a/ivy_tests/test_ivy/test_frontends/test_torch/test_nn/test_functional/test_loss_functions.py b/ivy_tests/test_ivy/test_frontends/test_torch/test_nn/test_functional/test_loss_functions.py
index 7ced14b897bc6..13abfcfcee803 100644
--- a/ivy_tests/test_ivy/test_frontends/test_torch/test_nn/test_functional/test_loss_functions.py
+++ b/ivy_tests/test_ivy/test_frontends/test_torch/test_nn/test_functional/test_loss_functions.py
@@ -685,11 +685,13 @@ def test_torch_multilabel_margin_loss(
reduce,
test_flags,
fn_tree,
+ backend_fw,
frontend,
on_device,
):
input_dtype, x = dtype_and_inputs
helpers.test_frontend_function(
+ backend_to_test=backend_fw,
input_dtypes=input_dtype,
frontend=frontend,
test_flags=test_flags,
diff --git a/ivy_tests/test_ivy/test_frontends/test_torch/test_nn/test_functional/test_non_linear_activation_functions.py b/ivy_tests/test_ivy/test_frontends/test_torch/test_nn/test_functional/test_non_linear_activation_functions.py
index 010ced1773e0f..2c9dc0c529024 100644
--- a/ivy_tests/test_ivy/test_frontends/test_torch/test_nn/test_functional/test_non_linear_activation_functions.py
+++ b/ivy_tests/test_ivy/test_frontends/test_torch/test_nn/test_functional/test_non_linear_activation_functions.py
@@ -4,9 +4,7 @@
# local
import ivy_tests.test_ivy.helpers as helpers
-from ivy.functional.backends.torch.layers import _get_embed_dim
from ivy_tests.test_ivy.helpers import handle_frontend_test
-from ivy_tests.test_ivy.test_functional.test_nn.test_layers import _mha_helper
# --- Helpers --- #
@@ -107,8 +105,9 @@ def _x_and_scaled_attention(draw, dtypes):
fn_tree="torch.nn.functional.celu",
dtype_and_input=helpers.dtype_and_values(
available_dtypes=helpers.get_dtypes("float"),
+ min_num_dims=1,
),
- alpha=helpers.floats(min_value=0.1, max_value=1.0, exclude_min=True),
+ alpha=helpers.floats(min_value=0.1, max_value=1.0),
test_inplace=st.booleans(),
test_with_out=st.just(False),
)
@@ -122,7 +121,7 @@ def test_torch_celu(
test_flags,
backend_fw,
):
- input_dtype, input = dtype_and_input
+ input_dtype, x = dtype_and_input
_filter_dtypes(input_dtype)
helpers.test_frontend_function(
input_dtypes=input_dtype,
@@ -131,7 +130,44 @@ def test_torch_celu(
test_flags=test_flags,
fn_tree=fn_tree,
on_device=on_device,
- input=input[0],
+ input=x[0],
+ rtol=1e-02,
+ atol=1e-02,
+ alpha=alpha,
+ )
+
+
+# celu_
+@handle_frontend_test(
+ fn_tree="torch.nn.functional.celu_",
+ dtype_and_input=helpers.dtype_and_values(
+ available_dtypes=helpers.get_dtypes("float"),
+ min_num_dims=1,
+ ),
+ alpha=helpers.floats(min_value=0.1, max_value=1.0),
+ test_inplace=st.just(True),
+ test_with_out=st.just(False),
+)
+def test_torch_celu_(
+ *,
+ dtype_and_input,
+ alpha,
+ on_device,
+ fn_tree,
+ frontend,
+ test_flags,
+ backend_fw,
+):
+ input_dtype, x = dtype_and_input
+ _filter_dtypes(input_dtype)
+ helpers.test_frontend_function(
+ input_dtypes=input_dtype,
+ backend_to_test=backend_fw,
+ frontend=frontend,
+ test_flags=test_flags,
+ fn_tree=fn_tree,
+ on_device=on_device,
+ input=x[0],
alpha=alpha,
)
@@ -686,97 +722,6 @@ def test_torch_mish(
)
-# multi_head_attention_forward
-@handle_frontend_test(
- fn_tree="torch.nn.functional.multi_head_attention_forward",
- dtype_mha_args=_mha_helper(same_pre_embed_dim=True, batch_second=True).filter(
- lambda args: args[10] is not None
- and (not args[22] or args[5] is not None)
- and len(set(_get_embed_dim(*args[6:10], args[1]))) == 1
- ),
- test_with_out=st.just(False),
-)
-def test_torch_multi_head_attention_forward(
- *,
- on_device,
- fn_tree,
- frontend,
- test_flags,
- dtype_mha_args,
- backend_fw,
-):
- (
- dtype,
- q,
- k,
- v,
- heads,
- attn_mask,
- in_proj_weight,
- q_proj_weight,
- k_proj_weight,
- v_proj_weight,
- out_proj_weight,
- in_proj_bias,
- out_proj_bias,
- key_padding_mask,
- bias_k,
- bias_v,
- static_k,
- static_v,
- _,
- add_zero_attn,
- dropout_p,
- training,
- is_causal,
- need_weights,
- average_attn_weights,
- batch_first,
- ) = dtype_mha_args
- if k is None and v is None:
- k = v = q
- # re-order the dtypes to match the order of the frontend arguments, not the order
- # of ivy.multi_head_attention's arguments given by _mha_helper
- kwargs = {
- "query": q,
- "key": k,
- "value": v,
- "embed_dim_to_check": q.shape[-1],
- "num_heads": heads,
- "in_proj_weight": in_proj_weight,
- "in_proj_bias": in_proj_bias,
- "bias_k": bias_k,
- "bias_v": bias_v,
- "add_zero_attn": add_zero_attn,
- "dropout_p": dropout_p,
- "out_proj_weight": out_proj_weight,
- "out_proj_bias": out_proj_bias,
- "training": training,
- "key_padding_mask": key_padding_mask,
- "need_weights": need_weights,
- "attn_mask": attn_mask,
- "use_separate_proj_weight": in_proj_weight is None,
- "q_proj_weight": q_proj_weight,
- "k_proj_weight": k_proj_weight,
- "v_proj_weight": v_proj_weight,
- "static_k": static_k,
- "static_v": static_v,
- "average_attn_weights": average_attn_weights,
- "is_causal": is_causal,
- }
- helpers.test_frontend_function(
- input_dtypes=[str(r.dtype) for r in kwargs.values() if ivy.is_array(r)],
- backend_to_test=backend_fw,
- frontend=frontend,
- test_flags=test_flags,
- fn_tree=fn_tree,
- atol=1e-03,
- on_device=on_device,
- test_values=not training or dropout_p == 0.0,
- **kwargs,
- )
-
-
# normalize
@handle_frontend_test(
fn_tree="torch.nn.functional.normalize",
@@ -1169,7 +1114,8 @@ def test_torch_softmax(
input=x[0],
dim=axis,
_stacklevel=3,
- dtype=ivy.as_ivy_dtype(dtypes[0]),
+ dtype=dtypes[0],
+ atol=1e-03,
)
ivy.previous_backend()
diff --git a/ivy_tests/test_ivy/test_frontends/test_torch/test_nn/test_functional/test_pooling_functions.py b/ivy_tests/test_ivy/test_frontends/test_torch/test_nn/test_functional/test_pooling_functions.py
index 8d940f3f91acd..a18769d922b1d 100644
--- a/ivy_tests/test_ivy/test_frontends/test_torch/test_nn/test_functional/test_pooling_functions.py
+++ b/ivy_tests/test_ivy/test_frontends/test_torch/test_nn/test_functional/test_pooling_functions.py
@@ -9,15 +9,13 @@
def calculate_same_padding(kernel_size, stride, shape):
padding = tuple(
- [
- max(
- 0,
- math.ceil(((shape[i] - 1) * stride[i] + kernel_size[i] - shape[i]) / 2),
- )
- for i in range(len(kernel_size))
- ]
+ max(
+ 0,
+ math.ceil(((shape[i] - 1) * stride[i] + kernel_size[i] - shape[i]) / 2),
+ )
+ for i in range(len(kernel_size))
)
- if all([kernel_size[i] / 2 >= padding[i] for i in range(len(kernel_size))]):
+ if all(kernel_size[i] / 2 >= padding[i] for i in range(len(kernel_size))):
if is_same_padding(padding, stride, kernel_size, shape):
return padding
return [0] * len(shape)
@@ -25,16 +23,12 @@ def calculate_same_padding(kernel_size, stride, shape):
def is_same_padding(padding, stride, kernel_size, input_shape):
output_shape = tuple(
- [
- (input_shape[i] + 2 * padding[i] - kernel_size[i]) // stride[i] + 1
- for i in range(len(padding))
- ]
+ (input_shape[i] + 2 * padding[i] - kernel_size[i]) // stride[i] + 1
+ for i in range(len(padding))
)
return all(
- [
- output_shape[i] == math.ceil(input_shape[i] / stride[i])
- for i in range(len(padding))
- ]
+ output_shape[i] == math.ceil(input_shape[i] / stride[i])
+ for i in range(len(padding))
)
@@ -193,14 +187,7 @@ def test_torch_avg_pool1d(
on_device,
):
input_dtype, x, kernel_size, stride, padding = dtype_x_k_s
- # TODO: remove the processing of padding attribute when ivy.avg_pool
- # support explicit padding
- x_shape = [x[0].shape[2]]
- padding = [pad[i] for i, pad in enumerate(padding)]
- # figuring out the exact kernel_size for SAME and VALID padding
- # As ivy.avg_pool1d doesn't support explicit padding scheme
- if not sum(padding) == 0:
- padding = calculate_same_padding(kernel_size, stride, x_shape)
+ padding = [pad[0] for pad in padding]
helpers.test_frontend_function(
input_dtypes=input_dtype,
backend_to_test=backend_fw,
@@ -248,7 +235,7 @@ def test_torch_avg_pool2d(
# support explicit padding
padding = [pad[i] for i, pad in enumerate(padding)]
x_shape = x[0].shape[2:]
- if not sum(padding) == 0:
+ if sum(padding) != 0:
padding = calculate_same_padding(kernel_size, [stride[0]] * 2, x_shape)
helpers.test_frontend_function(
input_dtypes=input_dtype,
@@ -300,7 +287,7 @@ def test_torch_avg_pool3d(
# support explicit padding
x_shape = x[0].shape[2:]
padding = [pad[0] for pad in padding]
- if not sum(padding) == 0:
+ if sum(padding) != 0:
stride_broad = (stride[0],) * 3 if len(stride) == 1 else stride
padding = calculate_same_padding(kernel_size, stride_broad, x_shape)
helpers.test_frontend_function(
diff --git a/ivy_tests/test_ivy/test_frontends/test_torch/test_nn/test_functional/test_vision_functions.py b/ivy_tests/test_ivy/test_frontends/test_torch/test_nn/test_functional/test_vision_functions.py
index 9c34752b567e5..912c3e82599be 100644
--- a/ivy_tests/test_ivy/test_frontends/test_torch/test_nn/test_functional/test_vision_functions.py
+++ b/ivy_tests/test_ivy/test_frontends/test_torch/test_nn/test_functional/test_vision_functions.py
@@ -238,7 +238,7 @@ def test_torch_grid_sample(
@handle_frontend_test(
fn_tree="torch.nn.functional.interpolate",
dtype_and_input_and_other=_interp_args(
- mode_list=["linear", "bilinear", "trilinear", "nearest", "area"],
+ mode_list="torch",
),
number_positional_args=st.just(2),
)
@@ -260,6 +260,8 @@ def test_torch_interpolate(
scale_factor,
recompute_scale_factor,
) = dtype_and_input_and_other
+ if mode not in ["linear", "bilinear", "bicubic", "trilinear"]:
+ align_corners = None
helpers.test_frontend_function(
input_dtypes=input_dtype,
backend_to_test=backend_fw,
@@ -267,8 +269,7 @@ def test_torch_interpolate(
test_flags=test_flags,
fn_tree=fn_tree,
on_device=on_device,
- rtol=1e-01,
- atol=1e-01,
+ atol=1e-03,
input=x[0],
size=size,
scale_factor=scale_factor,
diff --git a/ivy_tests/test_ivy/test_frontends/test_torch/test_pointwise_ops.py b/ivy_tests/test_ivy/test_frontends/test_torch/test_pointwise_ops.py
index a18f1bfc9f288..678aaad47bd17 100644
--- a/ivy_tests/test_ivy/test_frontends/test_torch/test_pointwise_ops.py
+++ b/ivy_tests/test_ivy/test_frontends/test_torch/test_pointwise_ops.py
@@ -5,13 +5,12 @@
# local
import ivy
import ivy_tests.test_ivy.helpers as helpers
+from ivy_tests.array_api_testing.test_array_api.array_api_tests import (
+ hypothesis_helpers as hh,
+)
from ivy_tests.test_ivy.helpers import handle_frontend_test
from ivy_tests.test_ivy.test_functional.test_core.test_elementwise import pow_helper
-from ivy_tests.test_ivy.test_functional.test_core.test_searching import (
- _broadcastable_trio,
-)
-
# --- Helpers --- #
# --------------- #
@@ -87,14 +86,26 @@ def _get_clip_inputs(draw):
@st.composite
def _masked_fill_helper(draw):
- cond, xs, dtypes = draw(_broadcastable_trio())
- if ivy.is_uint_dtype(dtypes[0]):
- fill_value = draw(helpers.ints(min_value=0, max_value=5))
- elif ivy.is_int_dtype(dtypes[0]):
- fill_value = draw(helpers.ints(min_value=-5, max_value=5))
- else:
- fill_value = draw(helpers.floats(min_value=-5, max_value=5))
- return dtypes[0], xs[0], cond, fill_value
+ shape_1, shape_2 = draw(hh.two_broadcastable_shapes())
+ dtype, x = draw(
+ helpers.dtype_and_values(
+ available_dtypes=helpers.get_dtypes("valid"),
+ shape=shape_1,
+ )
+ )
+ _, mask = draw(
+ helpers.dtype_and_values(
+ dtype=["bool"],
+ shape=shape_2,
+ )
+ )
+ _, fill_value = draw(
+ helpers.dtype_and_values(
+ dtype=dtype,
+ shape=(),
+ )
+ )
+ return dtype[0], x[0], mask[0], fill_value[0]
# --- Main --- #
@@ -721,7 +732,7 @@ def test_torch_bitwise_left_shift(
):
input_dtype, x = dtype_and_x
# negative shifts will throw an exception
- # shifts >= dtype witdth produce backend-defined behavior
+ # shifts >= dtype width produce backend-defined behavior
x[1] = np.asarray(
np.clip(x[1], 0, np.iinfo(input_dtype[1]).bits - 1), dtype=input_dtype[1]
)
@@ -813,7 +824,7 @@ def test_torch_bitwise_right_shift(
):
input_dtype, x = dtype_and_x
# negative shifts will throw an exception
- # shifts >= dtype witdth produce backend-defined behavior
+ # shifts >= dtype width produce backend-defined behavior
x[1] = np.asarray(
np.clip(x[1], 0, np.iinfo(input_dtype[1]).bits - 1), dtype=input_dtype[1]
)
@@ -2109,7 +2120,6 @@ def test_torch_masked_fill(
test_flags=test_flags,
fn_tree=fn_tree,
on_device=on_device,
- rtol=1e-03,
input=x,
mask=mask,
value=val,
diff --git a/ivy_tests/test_ivy/test_frontends/test_torch/test_random_sampling.py b/ivy_tests/test_ivy/test_frontends/test_torch/test_random_sampling.py
index 7d021c4db6a05..2b54f57e84078 100644
--- a/ivy_tests/test_ivy/test_frontends/test_torch/test_random_sampling.py
+++ b/ivy_tests/test_ivy/test_frontends/test_torch/test_random_sampling.py
@@ -344,7 +344,7 @@ def test_torch_randint(
backend_fw,
):
def call():
- helpers.test_frontend_function(
+ return helpers.test_frontend_function(
input_dtypes=dtype,
backend_to_test=backend_fw,
frontend=frontend,
diff --git a/ivy_tests/test_ivy/test_frontends/test_torch/test_reduction_ops.py b/ivy_tests/test_ivy/test_frontends/test_torch/test_reduction_ops.py
index 98fd3b4793061..9d3f52db9a672 100644
--- a/ivy_tests/test_ivy/test_frontends/test_torch/test_reduction_ops.py
+++ b/ivy_tests/test_ivy/test_frontends/test_torch/test_reduction_ops.py
@@ -21,7 +21,7 @@
@st.composite
def _get_axis_and_p(draw, kind="valid"):
p = draw(st.sampled_from(["fro", "nuc", 1, 2, -1, -2, float("inf"), -float("inf")]))
- if p == "fro" or p == "nuc":
+ if p in ["fro", "nuc"]:
max_axes_size = 2
min_axes_size = 2
else:
@@ -243,11 +243,9 @@ def test_torch_any(
@handle_frontend_test(
fn_tree="torch.argmax",
dtype_input_axis=helpers.dtype_values_axis(
- available_dtypes=helpers.get_dtypes("numeric"),
+ available_dtypes=helpers.get_dtypes("valid"),
force_int_axis=True,
- min_num_dims=1,
- min_axis=-1,
- max_axis=0,
+ valid_axis=True,
),
keepdims=st.booleans(),
)
@@ -672,6 +670,40 @@ def test_torch_nanmean(
)
+@handle_frontend_test(
+ fn_tree="torch.nanmedian",
+ dtype_input_axis=helpers.dtype_values_axis(
+ available_dtypes=helpers.get_dtypes("numeric"),
+ min_num_dims=1,
+ valid_axis=True,
+ force_int_axis=True,
+ ),
+ keepdim=st.booleans(),
+)
+def test_torch_nanmedian(
+ *,
+ dtype_input_axis,
+ keepdim,
+ on_device,
+ fn_tree,
+ frontend,
+ test_flags,
+ backend_fw,
+):
+ input_dtype, input, dim = dtype_input_axis
+ helpers.test_frontend_function(
+ input_dtypes=input_dtype,
+ backend_to_test=backend_fw,
+ frontend=frontend,
+ test_flags=test_flags,
+ fn_tree=fn_tree,
+ on_device=on_device,
+ input=input[0],
+ dim=dim,
+ keepdim=keepdim,
+ )
+
+
@handle_frontend_test(
fn_tree="torch.nansum",
dtype_and_x=_get_castable_dtype(
diff --git a/ivy_tests/test_ivy/test_frontends/test_torch/test_spectral_ops.py b/ivy_tests/test_ivy/test_frontends/test_torch/test_spectral_ops.py
index a1a7024f75a68..6e6376537f430 100644
--- a/ivy_tests/test_ivy/test_frontends/test_torch/test_spectral_ops.py
+++ b/ivy_tests/test_ivy/test_frontends/test_torch/test_spectral_ops.py
@@ -1,5 +1,4 @@
from hypothesis import strategies as st
-
import ivy_tests.test_ivy.helpers as helpers
from ivy_tests.test_ivy.helpers import handle_frontend_test
@@ -33,3 +32,70 @@ def test_torch_bartlett_window(
rtol=1e-02,
atol=1e-02,
)
+
+
+@handle_frontend_test(
+ window_length=helpers.ints(min_value=1, max_value=100),
+ dtype=helpers.get_dtypes("float", full=False),
+ fn_tree="torch.blackman_window",
+ periodic=st.booleans(),
+)
+def test_torch_blackman_window(
+ *,
+ window_length,
+ dtype,
+ periodic,
+ on_device,
+ fn_tree,
+ frontend,
+ backend_fw,
+ test_flags,
+):
+ helpers.test_frontend_function(
+ input_dtypes=[],
+ on_device=on_device,
+ frontend=frontend,
+ backend_to_test=backend_fw,
+ test_flags=test_flags,
+ fn_tree=fn_tree,
+ window_length=window_length,
+ periodic=periodic,
+ dtype=dtype[0],
+ rtol=1e-02,
+ atol=1e-02,
+ )
+
+
+@handle_frontend_test(
+ window_length=helpers.ints(min_value=1, max_value=100),
+ dtype=helpers.get_dtypes("float", full=False),
+ fn_tree="torch.kaiser_window",
+ periodic=st.booleans(),
+ beta=helpers.floats(min_value=1, max_value=20),
+)
+def test_torch_kaiser_window(
+ *,
+ window_length,
+ dtype,
+ periodic,
+ beta,
+ on_device,
+ fn_tree,
+ frontend,
+ backend_fw,
+ test_flags,
+):
+ helpers.test_frontend_function(
+ input_dtypes=[],
+ on_device=on_device,
+ frontend=frontend,
+ backend_to_test=backend_fw,
+ test_flags=test_flags,
+ fn_tree=fn_tree,
+ window_length=window_length,
+ periodic=periodic,
+ beta=beta,
+ dtype=dtype[0],
+ rtol=1e-02,
+ atol=1e-02,
+ )
diff --git a/ivy_tests/test_ivy/test_frontends/test_torch/test_tensor.py b/ivy_tests/test_ivy/test_frontends/test_torch/test_tensor.py
index 62fa9f91eb0d0..1a824bf209d64 100644
--- a/ivy_tests/test_ivy/test_frontends/test_torch/test_tensor.py
+++ b/ivy_tests/test_ivy/test_frontends/test_torch/test_tensor.py
@@ -36,6 +36,7 @@
)
from ivy_tests.test_ivy.test_frontends.test_torch.test_miscellaneous_ops import ( # noqa
dtype_value1_value2_axis,
+ _get_dtype_value1_value2_cov,
)
from ivy_tests.test_ivy.test_frontends.test_torch.test_linalg import ( # noqa
_get_dtype_and_matrix,
@@ -114,7 +115,7 @@ def _arrays_dim_idx_n_dtypes(draw):
)
)
- xs = list()
+ xs = []
available_input_types = draw(helpers.get_dtypes("numeric"))
input_dtypes = draw(
helpers.array_dtypes(
@@ -706,11 +707,8 @@ def test_torch___getitem__(
init_tree="torch.tensor",
method_name="__gt__",
dtype_and_x=helpers.dtype_and_values(
- available_dtypes=helpers.get_dtypes("float"),
+ available_dtypes=helpers.get_dtypes("valid"),
num_arrays=2,
- min_value=-1e04,
- max_value=1e04,
- allow_inf=False,
),
)
def test_torch___gt__(
@@ -723,22 +721,28 @@ def test_torch___gt__(
backend_fw,
):
input_dtype, x = dtype_and_x
- helpers.test_frontend_method(
- init_input_dtypes=input_dtype,
- backend_to_test=backend_fw,
- init_all_as_kwargs_np={
- "data": x[0],
- },
- method_input_dtypes=input_dtype,
- method_all_as_kwargs_np={
- "other": x[1],
- },
- frontend_method_data=frontend_method_data,
- init_flags=init_flags,
- method_flags=method_flags,
- frontend=frontend,
- on_device=on_device,
- )
+ try:
+ helpers.test_frontend_method(
+ init_input_dtypes=input_dtype,
+ backend_to_test=backend_fw,
+ init_all_as_kwargs_np={
+ "data": x[0],
+ },
+ method_input_dtypes=input_dtype,
+ method_all_as_kwargs_np={
+ "other": x[1],
+ },
+ frontend_method_data=frontend_method_data,
+ init_flags=init_flags,
+ method_flags=method_flags,
+ frontend=frontend,
+ on_device=on_device,
+ )
+ except RuntimeError as e:
+ if "overflow" in e:
+ assume(False)
+ else:
+ raise
# __invert__
@@ -746,10 +750,7 @@ def test_torch___gt__(
class_tree=CLASS_TREE,
init_tree="torch.tensor",
method_name="__invert__",
- dtype_and_x=helpers.dtype_and_values(
- available_dtypes=helpers.get_dtypes("integer"),
- num_arrays=1,
- ),
+ dtype_and_x=helpers.dtype_and_values(),
)
def test_torch___invert__(
dtype_and_x,
@@ -1439,81 +1440,89 @@ def test_torch__array__(
)
+@given(
+ dtype_x=helpers.dtype_and_values(
+ available_dtypes=helpers.get_dtypes("valid", prune_function=False),
+ ),
+ requires_grad=st.booleans(),
+)
+def test_torch__requires_grad(
+ dtype_x,
+ requires_grad,
+ backend_fw,
+):
+ ivy.set_backend(backend_fw)
+ _, data = dtype_x
+ x = Tensor(data[0])
+ assert not x._requires_grad
+ x.requires_grad_()
+ assert x._requires_grad
+ x.requires_grad_(requires_grad)
+ assert x._requires_grad == requires_grad
+ ivy.previous_backend()
+
+
+# abs
@handle_frontend_method(
class_tree=CLASS_TREE,
init_tree="torch.tensor",
- method_name="baddbmm_",
- dtype_and_matrices=_get_dtype_and_3dbatch_matrices(with_input=True, input_3d=True),
- beta=st.floats(
- min_value=-5,
- max_value=5,
- allow_nan=False,
- allow_subnormal=False,
- allow_infinity=False,
- ),
- alpha=st.floats(
- min_value=-5,
- max_value=5,
- allow_nan=False,
- allow_subnormal=False,
- allow_infinity=False,
+ method_name="abs",
+ dtype_and_x=helpers.dtype_and_values(
+ available_dtypes=helpers.get_dtypes("float"),
),
- test_inplace=st.just(True),
)
-def test_torch_baddbmm_(
- dtype_and_matrices,
- beta,
- alpha,
- frontend,
+def test_torch_abs(
+ dtype_and_x,
frontend_method_data,
init_flags,
method_flags,
+ frontend,
on_device,
+ backend_fw,
):
- input_dtype, x, batch1, batch2 = dtype_and_matrices
+ input_dtype, x = dtype_and_x
helpers.test_frontend_method(
init_input_dtypes=input_dtype,
- init_all_as_kwargs_np={"data": x[0]},
- method_input_dtypes=input_dtype,
- method_all_as_kwargs_np={
- "batch1": batch1,
- "batch2": batch2,
- "beta": beta,
- "alpha": alpha,
+ backend_to_test=backend_fw,
+ init_all_as_kwargs_np={
+ "data": x[0],
},
- frontend=frontend,
+ method_input_dtypes=input_dtype,
+ method_all_as_kwargs_np={},
frontend_method_data=frontend_method_data,
init_flags=init_flags,
method_flags=method_flags,
+ frontend=frontend,
on_device=on_device,
)
-# char
+# abs_
@handle_frontend_method(
class_tree=CLASS_TREE,
init_tree="torch.tensor",
- method_name="char",
- dtype_x=helpers.dtype_and_values(
- available_dtypes=helpers.get_dtypes("valid"),
- min_value=-128,
- max_value=127,
+ method_name="abs_",
+ dtype_and_x=helpers.dtype_and_values(
+ available_dtypes=helpers.get_dtypes("float"),
),
+ test_inplace=st.just(True),
)
-def test_torch_char(
- dtype_x,
- frontend,
+def test_torch_abs_(
+ dtype_and_x,
frontend_method_data,
init_flags,
method_flags,
+ frontend,
on_device,
backend_fw,
):
- input_dtype, x = dtype_x
+ input_dtype, x = dtype_and_x
helpers.test_frontend_method(
init_input_dtypes=input_dtype,
backend_to_test=backend_fw,
- init_all_as_kwargs_np={"data": x[0]},
+ init_all_as_kwargs_np={
+ "data": x[0],
+ },
method_input_dtypes=input_dtype,
method_all_as_kwargs_np={},
frontend_method_data=frontend_method_data,
@@ -1524,82 +1533,71 @@ def test_torch_char(
)
-# index_fill
+# acos
@handle_frontend_method(
class_tree=CLASS_TREE,
init_tree="torch.tensor",
- method_name="index_fill",
- dtype_indices_axis=helpers.array_indices_axis(
- array_dtypes=helpers.get_dtypes("numeric"),
- indices_dtypes=["int64"],
- min_num_dims=1,
- max_num_dims=5,
- min_dim_size=1,
- max_dim_size=10,
- first_dimension_only=True,
- indices_same_dims=False,
+ method_name="acos",
+ dtype_and_x=helpers.dtype_and_values(
+ available_dtypes=helpers.get_dtypes("float"),
+ allow_inf=False,
),
- value=st.floats(min_value=-100, max_value=100),
)
-def test_torch_index_fill(
- dtype_indices_axis,
- value,
- frontend,
+def test_torch_acos(
+ dtype_and_x,
frontend_method_data,
init_flags,
method_flags,
+ frontend,
on_device,
backend_fw,
):
- input_dtypes, x, indices, axis, _ = dtype_indices_axis
- if indices.ndim != 1:
- indices = ivy.flatten(indices)
+ input_dtype, x = dtype_and_x
helpers.test_frontend_method(
- init_input_dtypes=[input_dtypes[0]],
+ init_input_dtypes=input_dtype,
backend_to_test=backend_fw,
- init_all_as_kwargs_np={"data": x},
- method_input_dtypes=[input_dtypes[1]],
- method_all_as_kwargs_np={
- "dim": axis,
- "index": indices,
- "value": value,
+ init_all_as_kwargs_np={
+ "data": x[0],
},
- frontend=frontend,
+ method_input_dtypes=input_dtype,
+ method_all_as_kwargs_np={},
frontend_method_data=frontend_method_data,
init_flags=init_flags,
method_flags=method_flags,
+ frontend=frontend,
on_device=on_device,
)
-# nansum
+# acos_
@handle_frontend_method(
class_tree=CLASS_TREE,
init_tree="torch.tensor",
- method_name="nansum",
- dtype_x=helpers.dtype_and_values(
+ method_name="acos_",
+ dtype_and_x=helpers.dtype_and_values(
+ min_value=-1.0,
+ max_value=1.0,
available_dtypes=helpers.get_dtypes("float"),
- min_value=-1e04,
- max_value=1e04,
),
+ test_inplace=st.just(True),
)
-def test_torch_instance_nansum(
- dtype_x,
- frontend,
+def test_torch_acos_(
+ dtype_and_x,
frontend_method_data,
init_flags,
method_flags,
+ frontend,
on_device,
backend_fw,
):
- input_dtype, x = dtype_x
+ input_dtype, x = dtype_and_x
helpers.test_frontend_method(
init_input_dtypes=input_dtype,
backend_to_test=backend_fw,
init_all_as_kwargs_np={
"data": x[0],
},
- method_input_dtypes=input_dtype,
+ method_input_dtypes=[],
method_all_as_kwargs_np={},
frontend_method_data=frontend_method_data,
init_flags=init_flags,
@@ -1609,168 +1607,194 @@ def test_torch_instance_nansum(
)
-# scatter
+# acosh
@handle_frontend_method(
class_tree=CLASS_TREE,
init_tree="torch.tensor",
- method_name="scatter",
- args=put_along_axis_helper(),
+ method_name="acosh",
+ dtype_and_x=helpers.dtype_and_values(
+ min_value=1.0,
+ available_dtypes=helpers.get_dtypes("float"),
+ ),
)
-def test_torch_instance_scatter(
- args,
- frontend,
+def test_torch_acosh(
+ dtype_and_x,
frontend_method_data,
init_flags,
method_flags,
+ frontend,
on_device,
backend_fw,
):
- input_dtypes, x, indices, values, axis = args
+ input_dtype, x = dtype_and_x
helpers.test_frontend_method(
- init_input_dtypes=[input_dtypes[0]],
+ init_input_dtypes=input_dtype,
backend_to_test=backend_fw,
init_all_as_kwargs_np={
- "data": x,
- },
- method_input_dtypes=["int64", input_dtypes[0]],
- method_all_as_kwargs_np={
- "dim": axis,
- "index": indices,
- "src": values,
+ "data": x[0],
},
- frontend=frontend,
+ method_input_dtypes=[],
+ method_all_as_kwargs_np={},
frontend_method_data=frontend_method_data,
init_flags=init_flags,
method_flags=method_flags,
+ frontend=frontend,
on_device=on_device,
)
-# scatter_
+# acosh_
@handle_frontend_method(
class_tree=CLASS_TREE,
init_tree="torch.tensor",
- method_name="scatter_",
- args=put_along_axis_helper(),
- reduce=st.sampled_from(["add", "multiply"]),
+ method_name="acosh_",
+ dtype_and_x=helpers.dtype_and_values(
+ min_value=1.0,
+ available_dtypes=helpers.get_dtypes("float"),
+ ),
+ test_inplace=st.just(True),
)
-def test_torch_instance_scatter_(
- args,
- reduce,
- frontend,
+def test_torch_acosh_(
+ dtype_and_x,
frontend_method_data,
init_flags,
method_flags,
+ frontend,
on_device,
backend_fw,
):
- input_dtypes, x, indices, values, axis = args
+ input_dtype, x = dtype_and_x
helpers.test_frontend_method(
- init_input_dtypes=[input_dtypes[0]],
+ init_input_dtypes=input_dtype,
backend_to_test=backend_fw,
init_all_as_kwargs_np={
- "data": x,
- },
- method_input_dtypes=["int64", input_dtypes[0]],
- method_all_as_kwargs_np={
- "dim": axis,
- "index": indices,
- "src": values,
- "reduce": reduce,
+ "data": x[0],
},
- frontend=frontend,
+ method_input_dtypes=[],
+ method_all_as_kwargs_np={},
frontend_method_data=frontend_method_data,
init_flags=init_flags,
method_flags=method_flags,
+ frontend=frontend,
on_device=on_device,
)
-# scatter_add
+# add
@handle_frontend_method(
class_tree=CLASS_TREE,
init_tree="torch.tensor",
- method_name="scatter_add",
- args=put_along_axis_helper(),
-)
-def test_torch_instance_scatter_add(
- args,
- frontend,
- frontend_method_data,
- init_flags,
- method_flags,
- on_device,
- backend_fw,
+ method_name="add",
+ dtype_and_x=helpers.dtype_and_values(
+ available_dtypes=helpers.get_dtypes("float"),
+ num_arrays=2,
+ min_value=-1e04,
+ max_value=1e04,
+ allow_inf=False,
+ ),
+ alpha=st.floats(min_value=-1e04, max_value=1e04, allow_infinity=False),
+)
+def test_torch_add(
+ dtype_and_x,
+ alpha,
+ frontend,
+ frontend_method_data,
+ init_flags,
+ method_flags,
+ on_device,
+ backend_fw,
):
- input_dtypes, x, indices, values, axis = args
+ input_dtype, x = dtype_and_x
helpers.test_frontend_method(
- init_input_dtypes=[input_dtypes[0]],
+ init_input_dtypes=input_dtype,
backend_to_test=backend_fw,
init_all_as_kwargs_np={
- "data": x,
+ "data": x[0],
},
- method_input_dtypes=["int64", input_dtypes[0]],
+ method_input_dtypes=input_dtype,
method_all_as_kwargs_np={
- "dim": axis,
- "index": indices,
- "src": values,
+ "other": x[1],
+ "alpha": alpha,
},
- frontend=frontend,
frontend_method_data=frontend_method_data,
init_flags=init_flags,
method_flags=method_flags,
+ frontend=frontend,
+ atol_=1e-02,
on_device=on_device,
)
-# scatter_add_
+# add_
@handle_frontend_method(
class_tree=CLASS_TREE,
init_tree="torch.tensor",
- method_name="scatter_add_",
- args=put_along_axis_helper(),
+ method_name="add_",
+ dtype_and_x=helpers.dtype_and_values(
+ available_dtypes=helpers.get_dtypes("float"),
+ num_arrays=2,
+ min_value=-1e04,
+ max_value=1e04,
+ allow_inf=False,
+ ),
+ alpha=st.floats(min_value=-1e04, max_value=1e04, allow_infinity=False),
+ test_inplace=st.just(True),
)
-def test_torch_instance_scatter_add_(
- args,
- frontend,
+def test_torch_add_(
+ dtype_and_x,
+ alpha,
frontend_method_data,
init_flags,
method_flags,
+ frontend,
on_device,
backend_fw,
):
- input_dtypes, x, indices, values, axis = args
+ input_dtype, x = dtype_and_x
helpers.test_frontend_method(
- init_input_dtypes=[input_dtypes[0]],
+ init_input_dtypes=[input_dtype[0]],
backend_to_test=backend_fw,
init_all_as_kwargs_np={
- "data": x,
+ "data": x[0],
},
- method_input_dtypes=["int64", input_dtypes[0]],
+ method_input_dtypes=input_dtype,
method_all_as_kwargs_np={
- "dim": axis,
- "index": indices,
- "src": values,
+ "other": x[1],
+ "alpha": alpha,
},
- frontend=frontend,
frontend_method_data=frontend_method_data,
init_flags=init_flags,
method_flags=method_flags,
+ frontend=frontend,
on_device=on_device,
)
-# scatter_reduce
+# addbmm
@handle_frontend_method(
class_tree=CLASS_TREE,
init_tree="torch.tensor",
- method_name="scatter_reduce",
- args=put_along_axis_helper(),
- mode=st.sampled_from(["sum", "prod", "amin", "amax"]),
+ method_name="addbmm",
+ dtype_and_matrices=_get_dtype_and_3dbatch_matrices(with_input=True),
+ beta=st.floats(
+ min_value=-5,
+ max_value=5,
+ allow_nan=False,
+ allow_subnormal=False,
+ allow_infinity=False,
+ ),
+ alpha=st.floats(
+ min_value=-5,
+ max_value=5,
+ allow_nan=False,
+ allow_subnormal=False,
+ allow_infinity=False,
+ ),
)
-def test_torch_instance_scatter_reduce(
- args,
- mode,
+def test_torch_addbmm(
+ dtype_and_matrices,
+ beta,
+ alpha,
frontend,
frontend_method_data,
init_flags,
@@ -1778,39 +1802,55 @@ def test_torch_instance_scatter_reduce(
on_device,
backend_fw,
):
- input_dtypes, x, indices, values, axis = args
+ input_dtype, x, batch1, batch2 = dtype_and_matrices
helpers.test_frontend_method(
- init_input_dtypes=[input_dtypes[0]],
+ init_input_dtypes=input_dtype,
backend_to_test=backend_fw,
init_all_as_kwargs_np={
"data": x,
},
- method_input_dtypes=["int64", input_dtypes[0]],
+ method_input_dtypes=input_dtype,
method_all_as_kwargs_np={
- "dim": axis,
- "index": indices,
- "src": values,
- "reduce": mode,
+ "batch1": batch1,
+ "batch2": batch2,
+ "beta": beta,
+ "alpha": alpha,
},
- frontend=frontend,
frontend_method_data=frontend_method_data,
init_flags=init_flags,
method_flags=method_flags,
+ frontend=frontend,
+ atol_=1e-02,
on_device=on_device,
)
-# scatter_reduce_
+# addbmm_
@handle_frontend_method(
class_tree=CLASS_TREE,
init_tree="torch.tensor",
- method_name="scatter_reduce_",
- args=put_along_axis_helper(),
- mode=st.sampled_from(["sum", "prod", "amin", "amax"]),
+ method_name="addbmm_",
+ dtype_and_matrices=_get_dtype_and_3dbatch_matrices(with_input=True),
+ beta=st.floats(
+ min_value=-5,
+ max_value=5,
+ allow_nan=False,
+ allow_subnormal=False,
+ allow_infinity=False,
+ ),
+ alpha=st.floats(
+ min_value=-5,
+ max_value=5,
+ allow_nan=False,
+ allow_subnormal=False,
+ allow_infinity=False,
+ ),
+ test_inplace=st.just(True),
)
-def test_torch_instance_scatter_reduce_(
- args,
- mode,
+def test_torch_addbmm_(
+ dtype_and_matrices,
+ beta,
+ alpha,
frontend,
frontend_method_data,
init_flags,
@@ -1818,111 +1858,141 @@ def test_torch_instance_scatter_reduce_(
on_device,
backend_fw,
):
- input_dtypes, x, indices, values, axis = args
+ input_dtype, x, batch1, batch2 = dtype_and_matrices
helpers.test_frontend_method(
- init_input_dtypes=[input_dtypes[0]],
+ init_input_dtypes=input_dtype,
backend_to_test=backend_fw,
init_all_as_kwargs_np={
"data": x,
},
- method_input_dtypes=["int64", input_dtypes[0]],
+ method_input_dtypes=input_dtype,
method_all_as_kwargs_np={
- "dim": axis,
- "index": indices,
- "src": values,
- "reduce": mode,
+ "batch1": batch1,
+ "batch2": batch2,
+ "beta": beta,
+ "alpha": alpha,
},
- frontend=frontend,
frontend_method_data=frontend_method_data,
init_flags=init_flags,
method_flags=method_flags,
+ frontend=frontend,
+ atol_=1e-02,
on_device=on_device,
)
+# addcdiv
@handle_frontend_method(
class_tree=CLASS_TREE,
init_tree="torch.tensor",
- method_name="sinc",
+ method_name="addcdiv",
dtype_and_x=helpers.dtype_and_values(
available_dtypes=helpers.get_dtypes("float"),
+ num_arrays=3,
+ large_abs_safety_factor=2.5,
+ small_abs_safety_factor=2.5,
+ safety_factor_scale="log",
+ shared_dtype=True,
),
+ value=st.floats(min_value=-100, max_value=100),
)
-def test_torch_instance_sinc(
- *,
+def test_torch_addcdiv(
dtype_and_x,
+ value,
frontend,
- backend_fw,
frontend_method_data,
init_flags,
method_flags,
on_device,
+ backend_fw,
):
input_dtype, x = dtype_and_x
+ assume(not np.any(np.isclose(x[2], 0)))
+
helpers.test_frontend_method(
init_input_dtypes=input_dtype,
- init_all_as_kwargs_np={
- "data": x[0],
- },
+ backend_to_test=backend_fw,
+ init_all_as_kwargs_np={"data": x[0]},
method_input_dtypes=input_dtype,
- method_all_as_kwargs_np={},
+ method_all_as_kwargs_np={
+ "tensor1": x[1],
+ "tensor2": x[2],
+ "value": value,
+ },
frontend_method_data=frontend_method_data,
init_flags=init_flags,
method_flags=method_flags,
frontend=frontend,
- backend_to_test=backend_fw,
on_device=on_device,
+ atol_=1e-03,
)
-# sinc_
@handle_frontend_method(
class_tree=CLASS_TREE,
init_tree="torch.tensor",
- method_name="sinc_",
+ method_name="addcdiv_",
dtype_and_x=helpers.dtype_and_values(
- available_dtypes=helpers.get_dtypes("valid"),
+ available_dtypes=helpers.get_dtypes("float"),
+ num_arrays=3,
+ large_abs_safety_factor=2.5,
+ small_abs_safety_factor=2.5,
+ safety_factor_scale="log",
+ shared_dtype=True,
),
+ value=st.floats(min_value=-100, max_value=100),
test_inplace=st.just(True),
)
-def test_torch_instance_sinc_(
- *,
+def test_torch_addcdiv_(
dtype_and_x,
+ value,
frontend,
- backend_fw,
frontend_method_data,
init_flags,
method_flags,
on_device,
+ backend_fw,
):
input_dtype, x = dtype_and_x
+ assume(not np.any(np.isclose(x[2], 0)))
+
helpers.test_frontend_method(
init_input_dtypes=input_dtype,
- init_all_as_kwargs_np={
- "data": x[0],
- },
+ backend_to_test=backend_fw,
+ init_all_as_kwargs_np={"data": x[0]},
method_input_dtypes=input_dtype,
- method_all_as_kwargs_np={},
+ method_all_as_kwargs_np={
+ "tensor1": x[1],
+ "tensor2": x[2],
+ "value": value,
+ },
frontend_method_data=frontend_method_data,
init_flags=init_flags,
method_flags=method_flags,
frontend=frontend,
- backend_to_test=backend_fw,
on_device=on_device,
+ atol_=1e-03,
)
-# isnan
+# addcmul
@handle_frontend_method(
class_tree=CLASS_TREE,
init_tree="torch.tensor",
- method_name="isnan",
- dtype_x=helpers.dtype_and_values(
- available_dtypes=helpers.get_dtypes("valid"),
+ method_name="addcmul",
+ dtype_and_x=helpers.dtype_and_values(
+ available_dtypes=helpers.get_dtypes("float"),
+ num_arrays=3,
+ large_abs_safety_factor=2.5,
+ small_abs_safety_factor=2.5,
+ safety_factor_scale="log",
+ shared_dtype=True,
),
+ value=st.floats(min_value=-100, max_value=100),
)
-def test_torch_isnan(
- dtype_x,
+def test_torch_addcmul(
+ dtype_and_x,
+ value,
frontend,
frontend_method_data,
init_flags,
@@ -1930,322 +2000,470 @@ def test_torch_isnan(
on_device,
backend_fw,
):
- input_dtype, x = dtype_x
+ input_dtype, x = dtype_and_x
+
helpers.test_frontend_method(
init_input_dtypes=input_dtype,
backend_to_test=backend_fw,
init_all_as_kwargs_np={"data": x[0]},
method_input_dtypes=input_dtype,
- method_all_as_kwargs_np={},
+ method_all_as_kwargs_np={
+ "tensor1": x[1],
+ "tensor2": x[2],
+ "value": value,
+ },
frontend_method_data=frontend_method_data,
init_flags=init_flags,
method_flags=method_flags,
frontend=frontend,
on_device=on_device,
+ atol_=1e-02,
)
-# rsqrt_
+# addcmul_
@handle_frontend_method(
class_tree=CLASS_TREE,
init_tree="torch.tensor",
- method_name="rsqrt_",
+ method_name="addcmul_",
dtype_and_x=helpers.dtype_and_values(
available_dtypes=helpers.get_dtypes("float"),
+ num_arrays=3,
+ large_abs_safety_factor=2.5,
+ small_abs_safety_factor=2.5,
+ safety_factor_scale="log",
+ shared_dtype=True,
),
+ value=st.floats(min_value=-100, max_value=100),
test_inplace=st.just(True),
)
-def test_torch_rsqrt_(
+def test_torch_addcmul_(
dtype_and_x,
+ value,
+ frontend,
frontend_method_data,
init_flags,
method_flags,
- frontend,
on_device,
backend_fw,
):
input_dtype, x = dtype_and_x
+
helpers.test_frontend_method(
init_input_dtypes=input_dtype,
backend_to_test=backend_fw,
- init_all_as_kwargs_np={
- "data": x[0],
- },
+ init_all_as_kwargs_np={"data": x[0]},
method_input_dtypes=input_dtype,
- method_all_as_kwargs_np={},
+ method_all_as_kwargs_np={
+ "tensor1": x[1],
+ "tensor2": x[2],
+ "value": value,
+ },
frontend_method_data=frontend_method_data,
init_flags=init_flags,
method_flags=method_flags,
frontend=frontend,
on_device=on_device,
+ atol_=1e-02,
)
-@given(
- dtype_x=helpers.dtype_and_values(
- available_dtypes=helpers.get_dtypes("valid", prune_function=False),
+# addmm
+@handle_frontend_method(
+ class_tree=CLASS_TREE,
+ init_tree="torch.tensor",
+ method_name="addmm",
+ dtype_and_matrices=_get_dtype_input_and_matrices(with_input=True),
+ beta=st.floats(
+ min_value=-5,
+ max_value=5,
+ allow_nan=False,
+ allow_subnormal=False,
+ allow_infinity=False,
+ ),
+ alpha=st.floats(
+ min_value=-5,
+ max_value=5,
+ allow_nan=False,
+ allow_subnormal=False,
+ allow_infinity=False,
),
- requires_grad=st.booleans(),
)
-def test_torch_tensor__requires_grad(
- dtype_x,
- requires_grad,
+def test_torch_addmm(
+ dtype_and_matrices,
+ beta,
+ alpha,
+ frontend,
+ frontend_method_data,
+ init_flags,
+ method_flags,
+ on_device,
backend_fw,
):
- ivy.set_backend(backend_fw)
- _, data = dtype_x
- x = Tensor(data[0])
- assert not x._requires_grad
- x.requires_grad_()
- assert x._requires_grad
- x.requires_grad_(requires_grad)
- assert x._requires_grad == requires_grad
- ivy.previous_backend()
+ input_dtype, x, mat1, mat2 = dtype_and_matrices
+ helpers.test_frontend_method(
+ init_input_dtypes=input_dtype,
+ backend_to_test=backend_fw,
+ init_all_as_kwargs_np={
+ "data": x,
+ },
+ method_input_dtypes=input_dtype,
+ method_all_as_kwargs_np={
+ "mat1": mat1,
+ "mat2": mat2,
+ "beta": beta,
+ "alpha": alpha,
+ },
+ frontend_method_data=frontend_method_data,
+ init_flags=init_flags,
+ method_flags=method_flags,
+ frontend=frontend,
+ atol_=1e-02,
+ on_device=on_device,
+ )
-# abs
+# addmm_
@handle_frontend_method(
class_tree=CLASS_TREE,
init_tree="torch.tensor",
- method_name="abs",
- dtype_and_x=helpers.dtype_and_values(
- available_dtypes=helpers.get_dtypes("float"),
+ method_name="addmm_",
+ dtype_and_matrices=_get_dtype_input_and_matrices(with_input=True),
+ beta=st.floats(
+ min_value=-5,
+ max_value=5,
+ allow_nan=False,
+ allow_subnormal=False,
+ allow_infinity=False,
+ ),
+ alpha=st.floats(
+ min_value=-5,
+ max_value=5,
+ allow_nan=False,
+ allow_subnormal=False,
+ allow_infinity=False,
),
+ test_inplace=st.just(True),
)
-def test_torch_tensor_abs(
- dtype_and_x,
+def test_torch_addmm_(
+ dtype_and_matrices,
+ beta,
+ alpha,
+ frontend,
frontend_method_data,
init_flags,
method_flags,
- frontend,
on_device,
backend_fw,
):
- input_dtype, x = dtype_and_x
+ input_dtype, x, mat1, mat2 = dtype_and_matrices
helpers.test_frontend_method(
init_input_dtypes=input_dtype,
backend_to_test=backend_fw,
init_all_as_kwargs_np={
- "data": x[0],
+ "data": x,
},
method_input_dtypes=input_dtype,
- method_all_as_kwargs_np={},
+ method_all_as_kwargs_np={
+ "mat1": mat1,
+ "mat2": mat2,
+ "beta": beta,
+ "alpha": alpha,
+ },
frontend_method_data=frontend_method_data,
init_flags=init_flags,
method_flags=method_flags,
frontend=frontend,
+ atol_=1e-02,
on_device=on_device,
)
-# abs_
+# addmv
@handle_frontend_method(
class_tree=CLASS_TREE,
init_tree="torch.tensor",
- method_name="abs_",
- dtype_and_x=helpers.dtype_and_values(
- available_dtypes=helpers.get_dtypes("float"),
+ method_name="addmv",
+ dtype_and_matrices=_get_dtype_input_and_mat_vec(with_input=True),
+ beta=st.floats(
+ min_value=-5,
+ max_value=5,
+ allow_nan=False,
+ allow_subnormal=False,
+ allow_infinity=False,
+ ),
+ alpha=st.floats(
+ min_value=-5,
+ max_value=5,
+ allow_nan=False,
+ allow_subnormal=False,
+ allow_infinity=False,
),
- test_inplace=st.just(True),
)
-def test_torch_tensor_abs_(
- dtype_and_x,
+def test_torch_addmv(
+ dtype_and_matrices,
+ beta,
+ alpha,
+ frontend,
frontend_method_data,
init_flags,
method_flags,
- frontend,
on_device,
backend_fw,
):
- input_dtype, x = dtype_and_x
+ input_dtype, x, mat, vec = dtype_and_matrices
helpers.test_frontend_method(
init_input_dtypes=input_dtype,
backend_to_test=backend_fw,
init_all_as_kwargs_np={
- "data": x[0],
+ "data": x,
},
method_input_dtypes=input_dtype,
- method_all_as_kwargs_np={},
+ method_all_as_kwargs_np={
+ "mat": mat,
+ "vec": vec,
+ "beta": beta,
+ "alpha": alpha,
+ },
frontend_method_data=frontend_method_data,
init_flags=init_flags,
method_flags=method_flags,
frontend=frontend,
+ atol_=1e-02,
on_device=on_device,
)
-# acos
+# addmv_
@handle_frontend_method(
class_tree=CLASS_TREE,
init_tree="torch.tensor",
- method_name="acos",
- dtype_and_x=helpers.dtype_and_values(
- available_dtypes=helpers.get_dtypes("float"),
- allow_inf=False,
+ method_name="addmv_",
+ dtype_and_matrices=_get_dtype_input_and_mat_vec(with_input=True),
+ beta=st.floats(
+ min_value=-5,
+ max_value=5,
+ allow_nan=False,
+ allow_subnormal=False,
+ allow_infinity=False,
),
+ alpha=st.floats(
+ min_value=-5,
+ max_value=5,
+ allow_nan=False,
+ allow_subnormal=False,
+ allow_infinity=False,
+ ),
+ test_inplace=st.just(True),
)
-def test_torch_tensor_acos(
- dtype_and_x,
+def test_torch_addmv_(
+ dtype_and_matrices,
+ beta,
+ alpha,
+ frontend,
frontend_method_data,
init_flags,
method_flags,
- frontend,
on_device,
backend_fw,
):
- input_dtype, x = dtype_and_x
+ input_dtype, x, mat, vec = dtype_and_matrices
helpers.test_frontend_method(
init_input_dtypes=input_dtype,
- backend_to_test=backend_fw,
init_all_as_kwargs_np={
- "data": x[0],
+ "data": x,
},
method_input_dtypes=input_dtype,
- method_all_as_kwargs_np={},
+ backend_to_test=backend_fw,
+ method_all_as_kwargs_np={
+ "mat": mat,
+ "vec": vec,
+ "beta": beta,
+ "alpha": alpha,
+ },
frontend_method_data=frontend_method_data,
init_flags=init_flags,
method_flags=method_flags,
frontend=frontend,
+ atol_=1e-02,
on_device=on_device,
)
-# acos_
+# addr
@handle_frontend_method(
class_tree=CLASS_TREE,
init_tree="torch.tensor",
- method_name="acos_",
- dtype_and_x=helpers.dtype_and_values(
- min_value=-1.0,
- max_value=1.0,
- available_dtypes=helpers.get_dtypes("float"),
+ method_name="addr",
+ dtype_and_vecs=_get_dtype_input_and_vectors(with_input=True),
+ beta=st.floats(
+ min_value=-5,
+ max_value=5,
+ allow_nan=False,
+ allow_subnormal=False,
+ allow_infinity=False,
+ ),
+ alpha=st.floats(
+ min_value=-5,
+ max_value=5,
+ allow_nan=False,
+ allow_subnormal=False,
+ allow_infinity=False,
),
- test_inplace=st.just(True),
)
-def test_torch_tensor_acos_(
- dtype_and_x,
+def test_torch_addr(
+ dtype_and_vecs,
+ beta,
+ alpha,
+ frontend,
frontend_method_data,
init_flags,
method_flags,
- frontend,
on_device,
backend_fw,
):
- input_dtype, x = dtype_and_x
+ dtype, input, vec1, vec2 = dtype_and_vecs
helpers.test_frontend_method(
- init_input_dtypes=input_dtype,
+ init_input_dtypes=dtype,
backend_to_test=backend_fw,
init_all_as_kwargs_np={
- "data": x[0],
+ "data": input,
+ },
+ method_input_dtypes=dtype,
+ method_all_as_kwargs_np={
+ "vec1": vec1,
+ "vec2": vec2,
+ "beta": beta,
+ "alpha": alpha,
},
- method_input_dtypes=[],
- method_all_as_kwargs_np={},
frontend_method_data=frontend_method_data,
init_flags=init_flags,
method_flags=method_flags,
frontend=frontend,
+ atol_=1e-02,
on_device=on_device,
)
-# acosh
+# addr_
@handle_frontend_method(
class_tree=CLASS_TREE,
init_tree="torch.tensor",
- method_name="acosh",
- dtype_and_x=helpers.dtype_and_values(
- min_value=1.0,
- available_dtypes=helpers.get_dtypes("float"),
+ method_name="addr_",
+ dtype_and_vecs=_get_dtype_input_and_vectors(with_input=True),
+ beta=st.floats(
+ min_value=-5,
+ max_value=5,
+ allow_nan=False,
+ allow_subnormal=False,
+ allow_infinity=False,
+ ),
+ alpha=st.floats(
+ min_value=-5,
+ max_value=5,
+ allow_nan=False,
+ allow_subnormal=False,
+ allow_infinity=False,
),
+ test_inplace=st.just(True),
)
-def test_torch_tensor_acosh(
- dtype_and_x,
+def test_torch_addr_(
+ dtype_and_vecs,
+ beta,
+ alpha,
+ frontend,
frontend_method_data,
init_flags,
method_flags,
- frontend,
on_device,
backend_fw,
):
- input_dtype, x = dtype_and_x
+ dtype, input, vec1, vec2 = dtype_and_vecs
helpers.test_frontend_method(
- init_input_dtypes=input_dtype,
+ init_input_dtypes=dtype,
backend_to_test=backend_fw,
init_all_as_kwargs_np={
- "data": x[0],
+ "data": input,
+ },
+ method_input_dtypes=dtype,
+ method_all_as_kwargs_np={
+ "vec1": vec1,
+ "vec2": vec2,
+ "beta": beta,
+ "alpha": alpha,
},
- method_input_dtypes=[],
- method_all_as_kwargs_np={},
frontend_method_data=frontend_method_data,
init_flags=init_flags,
method_flags=method_flags,
frontend=frontend,
+ atol_=1e-02,
on_device=on_device,
)
-# acosh_
@handle_frontend_method(
class_tree=CLASS_TREE,
init_tree="torch.tensor",
- method_name="acosh_",
- dtype_and_x=helpers.dtype_and_values(
- min_value=1.0,
- available_dtypes=helpers.get_dtypes("float"),
+ method_name="adjoint",
+ dtype_and_values=helpers.dtype_and_values(
+ available_dtypes=helpers.get_dtypes("real_and_complex"),
+ min_num_dims=2,
+ min_dim_size=2,
),
- test_inplace=st.just(True),
)
-def test_torch_tensor_acosh_(
- dtype_and_x,
+def test_torch_adjoint(
+ dtype_and_values,
+ frontend,
frontend_method_data,
init_flags,
method_flags,
- frontend,
on_device,
backend_fw,
):
- input_dtype, x = dtype_and_x
+ input_dtype, values = dtype_and_values
+
helpers.test_frontend_method(
init_input_dtypes=input_dtype,
backend_to_test=backend_fw,
init_all_as_kwargs_np={
- "data": x[0],
+ "data": values[0],
},
- method_input_dtypes=[],
+ method_input_dtypes=input_dtype,
method_all_as_kwargs_np={},
- frontend_method_data=frontend_method_data,
init_flags=init_flags,
method_flags=method_flags,
+ frontend_method_data=frontend_method_data,
frontend=frontend,
on_device=on_device,
)
-# add
+# all
@handle_frontend_method(
class_tree=CLASS_TREE,
init_tree="torch.tensor",
- method_name="add",
- dtype_and_x=helpers.dtype_and_values(
- available_dtypes=helpers.get_dtypes("float"),
- num_arrays=2,
+ method_name="all",
+ dtype_input_axis=helpers.dtype_values_axis(
+ available_dtypes=helpers.get_dtypes("numeric"),
+ min_num_dims=1,
min_value=-1e04,
max_value=1e04,
- allow_inf=False,
+ valid_axis=True,
+ force_int_axis=True,
),
- alpha=st.floats(min_value=-1e04, max_value=1e04, allow_infinity=False),
+ keepdim=st.booleans(),
)
-def test_torch_tensor_add(
- dtype_and_x,
- alpha,
- frontend,
+def test_torch_all(
+ dtype_input_axis,
+ keepdim,
frontend_method_data,
init_flags,
method_flags,
+ frontend,
on_device,
backend_fw,
):
- input_dtype, x = dtype_and_x
+ input_dtype, x, axis = dtype_input_axis
helpers.test_frontend_method(
init_input_dtypes=input_dtype,
backend_to_test=backend_fw,
@@ -2254,36 +2472,32 @@ def test_torch_tensor_add(
},
method_input_dtypes=input_dtype,
method_all_as_kwargs_np={
- "other": x[1],
- "alpha": alpha,
+ "dim": axis,
+ "keepdim": keepdim,
},
frontend_method_data=frontend_method_data,
init_flags=init_flags,
method_flags=method_flags,
frontend=frontend,
- atol_=1e-02,
on_device=on_device,
)
-# add_
+# amax
@handle_frontend_method(
class_tree=CLASS_TREE,
init_tree="torch.tensor",
- method_name="add_",
- dtype_and_x=helpers.dtype_and_values(
- available_dtypes=helpers.get_dtypes("float"),
- num_arrays=2,
- min_value=-1e04,
- max_value=1e04,
- allow_inf=False,
+ method_name="amax",
+ dtype_x_axis=helpers.dtype_values_axis(
+ available_dtypes=helpers.get_dtypes("numeric"),
+ valid_axis=True,
+ force_int_axis=True,
),
- alpha=st.floats(min_value=-1e04, max_value=1e04, allow_infinity=False),
- test_inplace=st.just(True),
+ keepdim=st.booleans(),
)
-def test_torch_tensor_add_(
- dtype_and_x,
- alpha,
+def test_torch_amax(
+ dtype_x_axis,
+ keepdim,
frontend_method_data,
init_flags,
method_flags,
@@ -2291,17 +2505,17 @@ def test_torch_tensor_add_(
on_device,
backend_fw,
):
- input_dtype, x = dtype_and_x
+ input_dtype, x, axis = dtype_x_axis
helpers.test_frontend_method(
- init_input_dtypes=[input_dtype[0]],
+ init_input_dtypes=input_dtype,
backend_to_test=backend_fw,
init_all_as_kwargs_np={
"data": x[0],
},
method_input_dtypes=input_dtype,
method_all_as_kwargs_np={
- "other": x[1],
- "alpha": alpha,
+ "dim": axis,
+ "keepdim": keepdim,
},
frontend_method_data=frontend_method_data,
init_flags=init_flags,
@@ -2311,135 +2525,94 @@ def test_torch_tensor_add_(
)
-# addbmm
+# amin
@handle_frontend_method(
class_tree=CLASS_TREE,
init_tree="torch.tensor",
- method_name="addbmm",
- dtype_and_matrices=_get_dtype_and_3dbatch_matrices(with_input=True),
- beta=st.floats(
- min_value=-5,
- max_value=5,
- allow_nan=False,
- allow_subnormal=False,
- allow_infinity=False,
- ),
- alpha=st.floats(
- min_value=-5,
- max_value=5,
- allow_nan=False,
- allow_subnormal=False,
- allow_infinity=False,
+ method_name="amin",
+ dtype_x_axis=helpers.dtype_values_axis(
+ available_dtypes=helpers.get_dtypes("numeric"),
+ valid_axis=True,
+ force_int_axis=True,
),
+ keepdim=st.booleans(),
)
-def test_torch_tensor_addbmm(
- dtype_and_matrices,
- beta,
- alpha,
- frontend,
+def test_torch_amin(
+ dtype_x_axis,
+ keepdim,
frontend_method_data,
init_flags,
method_flags,
+ frontend,
on_device,
backend_fw,
):
- input_dtype, x, batch1, batch2 = dtype_and_matrices
+ input_dtype, x, axis = dtype_x_axis
helpers.test_frontend_method(
init_input_dtypes=input_dtype,
backend_to_test=backend_fw,
init_all_as_kwargs_np={
- "data": x,
+ "data": x[0],
},
method_input_dtypes=input_dtype,
method_all_as_kwargs_np={
- "batch1": batch1,
- "batch2": batch2,
- "beta": beta,
- "alpha": alpha,
+ "dim": axis,
+ "keepdim": keepdim,
},
frontend_method_data=frontend_method_data,
init_flags=init_flags,
method_flags=method_flags,
frontend=frontend,
- atol_=1e-02,
on_device=on_device,
)
-# addbmm_
+# aminmax
@handle_frontend_method(
class_tree=CLASS_TREE,
init_tree="torch.tensor",
- method_name="addbmm_",
- dtype_and_matrices=_get_dtype_and_3dbatch_matrices(with_input=True),
- beta=st.floats(
- min_value=-5,
- max_value=5,
- allow_nan=False,
- allow_subnormal=False,
- allow_infinity=False,
- ),
- alpha=st.floats(
- min_value=-5,
- max_value=5,
- allow_nan=False,
- allow_subnormal=False,
- allow_infinity=False,
+ method_name="aminmax",
+ dtype_input_axis=helpers.dtype_and_values(
+ available_dtypes=helpers.get_dtypes("numeric"),
),
- test_inplace=st.just(True),
)
-def test_torch_tensor_addbmm_(
- dtype_and_matrices,
- beta,
- alpha,
- frontend,
+def test_torch_aminmax(
+ dtype_input_axis,
frontend_method_data,
init_flags,
method_flags,
+ frontend,
on_device,
backend_fw,
):
- input_dtype, x, batch1, batch2 = dtype_and_matrices
+ input_dtype, x = dtype_input_axis
helpers.test_frontend_method(
init_input_dtypes=input_dtype,
backend_to_test=backend_fw,
init_all_as_kwargs_np={
- "data": x,
+ "data": x[0],
},
method_input_dtypes=input_dtype,
- method_all_as_kwargs_np={
- "batch1": batch1,
- "batch2": batch2,
- "beta": beta,
- "alpha": alpha,
- },
+ method_all_as_kwargs_np={},
frontend_method_data=frontend_method_data,
init_flags=init_flags,
method_flags=method_flags,
frontend=frontend,
- atol_=1e-02,
on_device=on_device,
)
-# addcdiv
+# angle
@handle_frontend_method(
class_tree=CLASS_TREE,
init_tree="torch.tensor",
- method_name="addcdiv",
- dtype_and_x=helpers.dtype_and_values(
- available_dtypes=helpers.get_dtypes("float"),
- num_arrays=3,
- large_abs_safety_factor=2.5,
- small_abs_safety_factor=2.5,
- safety_factor_scale="log",
- shared_dtype=True,
+ method_name="angle",
+ dtype_and_values=helpers.dtype_and_values(
+ available_dtypes=["float64", "complex64", "complex128"],
),
- value=st.floats(min_value=-100, max_value=100),
)
-def test_torch_tensor_addcdiv(
- dtype_and_x,
- value,
+def test_torch_angle(
+ dtype_and_values,
frontend,
frontend_method_data,
init_flags,
@@ -2447,93 +2620,84 @@ def test_torch_tensor_addcdiv(
on_device,
backend_fw,
):
- input_dtype, x = dtype_and_x
- assume(not np.any(np.isclose(x[2], 0)))
+ input_dtype, values = dtype_and_values
helpers.test_frontend_method(
init_input_dtypes=input_dtype,
backend_to_test=backend_fw,
- init_all_as_kwargs_np={"data": x[0]},
- method_input_dtypes=input_dtype,
- method_all_as_kwargs_np={
- "tensor1": x[1],
- "tensor2": x[2],
- "value": value,
+ init_all_as_kwargs_np={
+ "data": values[0],
},
- frontend_method_data=frontend_method_data,
+ method_input_dtypes=input_dtype,
+ method_all_as_kwargs_np={},
init_flags=init_flags,
method_flags=method_flags,
+ frontend_method_data=frontend_method_data,
frontend=frontend,
on_device=on_device,
- atol_=1e-03,
)
+# any
@handle_frontend_method(
class_tree=CLASS_TREE,
init_tree="torch.tensor",
- method_name="addcdiv_",
- dtype_and_x=helpers.dtype_and_values(
- available_dtypes=helpers.get_dtypes("float"),
- num_arrays=3,
- large_abs_safety_factor=2.5,
- small_abs_safety_factor=2.5,
- safety_factor_scale="log",
- shared_dtype=True,
+ method_name="any",
+ dtype_input_axis=helpers.dtype_values_axis(
+ available_dtypes=helpers.get_dtypes("numeric"),
+ min_num_dims=1,
+ min_value=-1e04,
+ max_value=1e04,
+ valid_axis=True,
+ force_int_axis=True,
),
- value=st.floats(min_value=-100, max_value=100),
- test_inplace=st.just(True),
+ keepdim=st.booleans(),
)
-def test_torch_tensor_addcdiv_(
- dtype_and_x,
- value,
- frontend,
+def test_torch_any(
+ dtype_input_axis,
+ keepdim,
frontend_method_data,
init_flags,
method_flags,
+ frontend,
on_device,
backend_fw,
):
- input_dtype, x = dtype_and_x
- assume(not np.any(np.isclose(x[2], 0)))
-
+ input_dtype, x, axis = dtype_input_axis
helpers.test_frontend_method(
init_input_dtypes=input_dtype,
backend_to_test=backend_fw,
- init_all_as_kwargs_np={"data": x[0]},
+ init_all_as_kwargs_np={
+ "data": x[0],
+ },
method_input_dtypes=input_dtype,
method_all_as_kwargs_np={
- "tensor1": x[1],
- "tensor2": x[2],
- "value": value,
+ "dim": axis,
+ "keepdim": keepdim,
},
frontend_method_data=frontend_method_data,
init_flags=init_flags,
method_flags=method_flags,
frontend=frontend,
on_device=on_device,
- atol_=1e-03,
)
-# addcmul
+# write test for torch instance apply_
+
+
@handle_frontend_method(
class_tree=CLASS_TREE,
init_tree="torch.tensor",
- method_name="addcmul",
- dtype_and_x=helpers.dtype_and_values(
+ method_name="apply_",
+ dtype_and_values=helpers.dtype_and_values(
available_dtypes=helpers.get_dtypes("float"),
- num_arrays=3,
- large_abs_safety_factor=2.5,
- small_abs_safety_factor=2.5,
- safety_factor_scale="log",
- shared_dtype=True,
+ num_arrays=1,
),
- value=st.floats(min_value=-100, max_value=100),
+ test_inplace=st.just(True),
)
-def test_torch_tensor_addcmul(
- dtype_and_x,
- value,
+def test_torch_apply_(
+ dtype_and_values,
frontend,
frontend_method_data,
init_flags,
@@ -2541,462 +2705,340 @@ def test_torch_tensor_addcmul(
on_device,
backend_fw,
):
- input_dtype, x = dtype_and_x
+ def func(x):
+ return x + 1
+
+ input_dtype, values = dtype_and_values
helpers.test_frontend_method(
init_input_dtypes=input_dtype,
backend_to_test=backend_fw,
- init_all_as_kwargs_np={"data": x[0]},
+ init_all_as_kwargs_np={
+ "data": values[0],
+ },
method_input_dtypes=input_dtype,
method_all_as_kwargs_np={
- "tensor1": x[1],
- "tensor2": x[2],
- "value": value,
+ "callable": func,
},
- frontend_method_data=frontend_method_data,
init_flags=init_flags,
method_flags=method_flags,
+ frontend_method_data=frontend_method_data,
frontend=frontend,
on_device=on_device,
- atol_=1e-02,
)
-# addcmul_
+# arccos
@handle_frontend_method(
class_tree=CLASS_TREE,
init_tree="torch.tensor",
- method_name="addcmul_",
+ method_name="arccos",
dtype_and_x=helpers.dtype_and_values(
+ min_value=-1.0,
+ max_value=1.0,
available_dtypes=helpers.get_dtypes("float"),
- num_arrays=3,
- large_abs_safety_factor=2.5,
- small_abs_safety_factor=2.5,
- safety_factor_scale="log",
- shared_dtype=True,
),
- value=st.floats(min_value=-100, max_value=100),
- test_inplace=st.just(True),
)
-def test_torch_tensor_addcmul_(
+def test_torch_arccos(
dtype_and_x,
- value,
- frontend,
frontend_method_data,
init_flags,
method_flags,
+ frontend,
on_device,
backend_fw,
):
input_dtype, x = dtype_and_x
-
helpers.test_frontend_method(
init_input_dtypes=input_dtype,
backend_to_test=backend_fw,
- init_all_as_kwargs_np={"data": x[0]},
- method_input_dtypes=input_dtype,
- method_all_as_kwargs_np={
- "tensor1": x[1],
- "tensor2": x[2],
- "value": value,
+ init_all_as_kwargs_np={
+ "data": x[0],
},
+ method_input_dtypes=[],
+ method_all_as_kwargs_np={},
frontend_method_data=frontend_method_data,
init_flags=init_flags,
method_flags=method_flags,
frontend=frontend,
on_device=on_device,
- atol_=1e-02,
)
-# addmm
+# arccos_
@handle_frontend_method(
class_tree=CLASS_TREE,
init_tree="torch.tensor",
- method_name="addmm",
- dtype_and_matrices=_get_dtype_input_and_matrices(with_input=True),
- beta=st.floats(
- min_value=-5,
- max_value=5,
- allow_nan=False,
- allow_subnormal=False,
- allow_infinity=False,
- ),
- alpha=st.floats(
- min_value=-5,
- max_value=5,
- allow_nan=False,
- allow_subnormal=False,
- allow_infinity=False,
+ method_name="arccos_",
+ dtype_and_x=helpers.dtype_and_values(
+ min_value=-1.0,
+ max_value=1.0,
+ available_dtypes=helpers.get_dtypes("float"),
),
+ test_inplace=st.just(True),
)
-def test_torch_tensor_addmm(
- dtype_and_matrices,
- beta,
- alpha,
- frontend,
+def test_torch_arccos_(
+ dtype_and_x,
frontend_method_data,
init_flags,
method_flags,
+ frontend,
on_device,
backend_fw,
):
- input_dtype, x, mat1, mat2 = dtype_and_matrices
+ input_dtype, x = dtype_and_x
helpers.test_frontend_method(
init_input_dtypes=input_dtype,
backend_to_test=backend_fw,
init_all_as_kwargs_np={
- "data": x,
- },
- method_input_dtypes=input_dtype,
- method_all_as_kwargs_np={
- "mat1": mat1,
- "mat2": mat2,
- "beta": beta,
- "alpha": alpha,
+ "data": x[0],
},
+ method_input_dtypes=[],
+ method_all_as_kwargs_np={},
frontend_method_data=frontend_method_data,
init_flags=init_flags,
method_flags=method_flags,
frontend=frontend,
- atol_=1e-02,
on_device=on_device,
)
-# addmm_
+# arccosh
@handle_frontend_method(
class_tree=CLASS_TREE,
init_tree="torch.tensor",
- method_name="addmm_",
- dtype_and_matrices=_get_dtype_input_and_matrices(with_input=True),
- beta=st.floats(
- min_value=-5,
- max_value=5,
- allow_nan=False,
- allow_subnormal=False,
- allow_infinity=False,
- ),
- alpha=st.floats(
- min_value=-5,
- max_value=5,
- allow_nan=False,
- allow_subnormal=False,
- allow_infinity=False,
+ method_name="arccosh",
+ dtype_and_x=helpers.dtype_and_values(
+ min_value=-1.0,
+ max_value=1.0,
+ available_dtypes=helpers.get_dtypes("float"),
),
- test_inplace=st.just(True),
)
-def test_torch_tensor_addmm_(
- dtype_and_matrices,
- beta,
- alpha,
- frontend,
+def test_torch_arccosh(
+ dtype_and_x,
frontend_method_data,
init_flags,
method_flags,
+ frontend,
on_device,
backend_fw,
):
- input_dtype, x, mat1, mat2 = dtype_and_matrices
+ input_dtype, x = dtype_and_x
helpers.test_frontend_method(
init_input_dtypes=input_dtype,
backend_to_test=backend_fw,
init_all_as_kwargs_np={
- "data": x,
- },
- method_input_dtypes=input_dtype,
- method_all_as_kwargs_np={
- "mat1": mat1,
- "mat2": mat2,
- "beta": beta,
- "alpha": alpha,
+ "data": x[0],
},
+ method_input_dtypes=[],
+ method_all_as_kwargs_np={},
frontend_method_data=frontend_method_data,
init_flags=init_flags,
method_flags=method_flags,
frontend=frontend,
- atol_=1e-02,
on_device=on_device,
)
-# addmv
+# arccosh_
@handle_frontend_method(
class_tree=CLASS_TREE,
init_tree="torch.tensor",
- method_name="addmv",
- dtype_and_matrices=_get_dtype_input_and_mat_vec(with_input=True),
- beta=st.floats(
- min_value=-5,
- max_value=5,
- allow_nan=False,
- allow_subnormal=False,
- allow_infinity=False,
- ),
- alpha=st.floats(
- min_value=-5,
- max_value=5,
- allow_nan=False,
- allow_subnormal=False,
- allow_infinity=False,
+ method_name="arccosh_",
+ dtype_and_x=helpers.dtype_and_values(
+ min_value=-1.0,
+ max_value=1.0,
+ available_dtypes=helpers.get_dtypes("float"),
),
+ test_inplace=st.just(True),
)
-def test_torch_tensor_addmv(
- dtype_and_matrices,
- beta,
- alpha,
- frontend,
+def test_torch_arccosh_(
+ dtype_and_x,
frontend_method_data,
init_flags,
method_flags,
+ frontend,
on_device,
backend_fw,
):
- input_dtype, x, mat, vec = dtype_and_matrices
+ input_dtype, x = dtype_and_x
helpers.test_frontend_method(
init_input_dtypes=input_dtype,
backend_to_test=backend_fw,
init_all_as_kwargs_np={
- "data": x,
- },
- method_input_dtypes=input_dtype,
- method_all_as_kwargs_np={
- "mat": mat,
- "vec": vec,
- "beta": beta,
- "alpha": alpha,
+ "data": x[0],
},
+ method_input_dtypes=[],
+ method_all_as_kwargs_np={},
frontend_method_data=frontend_method_data,
init_flags=init_flags,
method_flags=method_flags,
frontend=frontend,
- atol_=1e-02,
on_device=on_device,
)
-# addmv_
+# arcsin
@handle_frontend_method(
class_tree=CLASS_TREE,
init_tree="torch.tensor",
- method_name="addmv_",
- dtype_and_matrices=_get_dtype_input_and_mat_vec(with_input=True),
- beta=st.floats(
- min_value=-5,
- max_value=5,
- allow_nan=False,
- allow_subnormal=False,
- allow_infinity=False,
- ),
- alpha=st.floats(
- min_value=-5,
- max_value=5,
- allow_nan=False,
- allow_subnormal=False,
- allow_infinity=False,
+ method_name="arcsin",
+ dtype_and_x=helpers.dtype_and_values(
+ available_dtypes=helpers.get_dtypes("float"),
+ allow_inf=False,
),
- test_inplace=st.just(True),
)
-def test_torch_tensor_addmv_(
- dtype_and_matrices,
- beta,
- alpha,
- frontend,
+def test_torch_arcsin(
+ dtype_and_x,
frontend_method_data,
init_flags,
method_flags,
+ frontend,
on_device,
backend_fw,
):
- input_dtype, x, mat, vec = dtype_and_matrices
+ input_dtype, x = dtype_and_x
helpers.test_frontend_method(
init_input_dtypes=input_dtype,
+ backend_to_test=backend_fw,
init_all_as_kwargs_np={
- "data": x,
+ "data": x[0],
},
method_input_dtypes=input_dtype,
- backend_to_test=backend_fw,
- method_all_as_kwargs_np={
- "mat": mat,
- "vec": vec,
- "beta": beta,
- "alpha": alpha,
- },
+ method_all_as_kwargs_np={},
frontend_method_data=frontend_method_data,
init_flags=init_flags,
method_flags=method_flags,
frontend=frontend,
- atol_=1e-02,
on_device=on_device,
)
-# addr
+# arcsin_
@handle_frontend_method(
class_tree=CLASS_TREE,
init_tree="torch.tensor",
- method_name="addr",
- dtype_and_vecs=_get_dtype_input_and_vectors(with_input=True),
- beta=st.floats(
- min_value=-5,
- max_value=5,
- allow_nan=False,
- allow_subnormal=False,
- allow_infinity=False,
- ),
- alpha=st.floats(
- min_value=-5,
- max_value=5,
- allow_nan=False,
- allow_subnormal=False,
- allow_infinity=False,
+ method_name="arcsin_",
+ dtype_and_x=helpers.dtype_and_values(
+ min_value=-1.0,
+ max_value=1.0,
+ available_dtypes=helpers.get_dtypes("float"),
),
+ test_inplace=st.just(True),
)
-def test_torch_tensor_addr(
- dtype_and_vecs,
- beta,
- alpha,
- frontend,
+def test_torch_arcsin_(
+ dtype_and_x,
frontend_method_data,
init_flags,
method_flags,
+ frontend,
on_device,
backend_fw,
):
- dtype, input, vec1, vec2 = dtype_and_vecs
+ input_dtype, x = dtype_and_x
helpers.test_frontend_method(
- init_input_dtypes=dtype,
+ init_input_dtypes=input_dtype,
backend_to_test=backend_fw,
init_all_as_kwargs_np={
- "data": input,
- },
- method_input_dtypes=dtype,
- method_all_as_kwargs_np={
- "vec1": vec1,
- "vec2": vec2,
- "beta": beta,
- "alpha": alpha,
+ "data": x[0],
},
+ method_input_dtypes=[],
+ method_all_as_kwargs_np={},
frontend_method_data=frontend_method_data,
init_flags=init_flags,
method_flags=method_flags,
frontend=frontend,
- atol_=1e-02,
on_device=on_device,
)
-# addr_
+# arcsinh
@handle_frontend_method(
class_tree=CLASS_TREE,
init_tree="torch.tensor",
- method_name="addr_",
- dtype_and_vecs=_get_dtype_input_and_vectors(with_input=True),
- beta=st.floats(
- min_value=-5,
- max_value=5,
- allow_nan=False,
- allow_subnormal=False,
- allow_infinity=False,
- ),
- alpha=st.floats(
- min_value=-5,
- max_value=5,
- allow_nan=False,
- allow_subnormal=False,
- allow_infinity=False,
+ method_name="arcsinh",
+ dtype_and_x=helpers.dtype_and_values(
+ min_value=-1.0,
+ max_value=1.0,
+ available_dtypes=helpers.get_dtypes("float"),
),
- test_inplace=st.just(True),
)
-def test_torch_tensor_addr_(
- dtype_and_vecs,
- beta,
- alpha,
- frontend,
+def test_torch_arcsinh(
+ dtype_and_x,
frontend_method_data,
init_flags,
method_flags,
+ frontend,
on_device,
backend_fw,
):
- dtype, input, vec1, vec2 = dtype_and_vecs
+ input_dtype, x = dtype_and_x
helpers.test_frontend_method(
- init_input_dtypes=dtype,
+ init_input_dtypes=input_dtype,
backend_to_test=backend_fw,
init_all_as_kwargs_np={
- "data": input,
- },
- method_input_dtypes=dtype,
- method_all_as_kwargs_np={
- "vec1": vec1,
- "vec2": vec2,
- "beta": beta,
- "alpha": alpha,
+ "data": x[0],
},
+ method_input_dtypes=[],
+ method_all_as_kwargs_np={},
frontend_method_data=frontend_method_data,
init_flags=init_flags,
method_flags=method_flags,
frontend=frontend,
- atol_=1e-02,
on_device=on_device,
)
+# arcsinh_
@handle_frontend_method(
class_tree=CLASS_TREE,
init_tree="torch.tensor",
- method_name="adjoint",
- dtype_and_values=helpers.dtype_and_values(
- available_dtypes=helpers.get_dtypes("real_and_complex"),
- min_num_dims=2,
- min_dim_size=2,
+ method_name="arcsinh_",
+ dtype_and_x=helpers.dtype_and_values(
+ min_value=-1.0,
+ max_value=1.0,
+ available_dtypes=helpers.get_dtypes("float"),
),
+ test_inplace=st.just(True),
)
-def test_torch_tensor_adjoint(
- dtype_and_values,
- frontend,
+def test_torch_arcsinh_(
+ dtype_and_x,
frontend_method_data,
init_flags,
method_flags,
+ frontend,
on_device,
backend_fw,
):
- input_dtype, values = dtype_and_values
-
+ input_dtype, x = dtype_and_x
helpers.test_frontend_method(
init_input_dtypes=input_dtype,
backend_to_test=backend_fw,
init_all_as_kwargs_np={
- "data": values[0],
+ "data": x[0],
},
- method_input_dtypes=input_dtype,
+ method_input_dtypes=[],
method_all_as_kwargs_np={},
+ frontend_method_data=frontend_method_data,
init_flags=init_flags,
method_flags=method_flags,
- frontend_method_data=frontend_method_data,
frontend=frontend,
on_device=on_device,
)
-# all
+# arctan
@handle_frontend_method(
class_tree=CLASS_TREE,
init_tree="torch.tensor",
- method_name="all",
- dtype_input_axis=helpers.dtype_values_axis(
- available_dtypes=helpers.get_dtypes("numeric"),
- min_num_dims=1,
- min_value=-1e04,
- max_value=1e04,
- valid_axis=True,
- force_int_axis=True,
+ method_name="arctan",
+ dtype_and_x=helpers.dtype_and_values(
+ available_dtypes=helpers.get_dtypes("float"),
+ allow_inf=False,
),
- keepdim=st.booleans(),
)
-def test_torch_tensor_all(
- dtype_input_axis,
- keepdim,
+def test_torch_arctan(
+ dtype_and_x,
frontend_method_data,
init_flags,
method_flags,
@@ -3004,7 +3046,7 @@ def test_torch_tensor_all(
on_device,
backend_fw,
):
- input_dtype, x, axis = dtype_input_axis
+ input_dtype, x = dtype_and_x
helpers.test_frontend_method(
init_input_dtypes=input_dtype,
backend_to_test=backend_fw,
@@ -3012,10 +3054,7 @@ def test_torch_tensor_all(
"data": x[0],
},
method_input_dtypes=input_dtype,
- method_all_as_kwargs_np={
- "dim": axis,
- "keepdim": keepdim,
- },
+ method_all_as_kwargs_np={},
frontend_method_data=frontend_method_data,
init_flags=init_flags,
method_flags=method_flags,
@@ -3024,21 +3063,18 @@ def test_torch_tensor_all(
)
-# amax
+# arctan2
@handle_frontend_method(
class_tree=CLASS_TREE,
init_tree="torch.tensor",
- method_name="amax",
- dtype_x_axis=helpers.dtype_values_axis(
- available_dtypes=helpers.get_dtypes("numeric"),
- valid_axis=True,
- force_int_axis=True,
+ method_name="arctan2",
+ dtype_and_x=helpers.dtype_and_values(
+ available_dtypes=helpers.get_dtypes("float"),
+ num_arrays=2,
),
- keepdim=st.booleans(),
)
-def test_torch_tensor_amax(
- dtype_x_axis,
- keepdim,
+def test_torch_arctan2(
+ dtype_and_x,
frontend_method_data,
init_flags,
method_flags,
@@ -3046,7 +3082,7 @@ def test_torch_tensor_amax(
on_device,
backend_fw,
):
- input_dtype, x, axis = dtype_x_axis
+ input_dtype, x = dtype_and_x
helpers.test_frontend_method(
init_input_dtypes=input_dtype,
backend_to_test=backend_fw,
@@ -3055,8 +3091,7 @@ def test_torch_tensor_amax(
},
method_input_dtypes=input_dtype,
method_all_as_kwargs_np={
- "dim": axis,
- "keepdim": keepdim,
+ "other": x[1],
},
frontend_method_data=frontend_method_data,
init_flags=init_flags,
@@ -3066,21 +3101,18 @@ def test_torch_tensor_amax(
)
-# amin
+# arctan2_
@handle_frontend_method(
class_tree=CLASS_TREE,
init_tree="torch.tensor",
- method_name="amin",
- dtype_x_axis=helpers.dtype_values_axis(
- available_dtypes=helpers.get_dtypes("numeric"),
- valid_axis=True,
- force_int_axis=True,
+ method_name="arctan2_",
+ dtype_and_x=helpers.dtype_and_values(
+ available_dtypes=helpers.get_dtypes("float"),
+ num_arrays=2,
),
- keepdim=st.booleans(),
)
-def test_torch_tensor_amin(
- dtype_x_axis,
- keepdim,
+def test_torch_arctan2_(
+ dtype_and_x,
frontend_method_data,
init_flags,
method_flags,
@@ -3088,7 +3120,7 @@ def test_torch_tensor_amin(
on_device,
backend_fw,
):
- input_dtype, x, axis = dtype_x_axis
+ input_dtype, x = dtype_and_x
helpers.test_frontend_method(
init_input_dtypes=input_dtype,
backend_to_test=backend_fw,
@@ -3097,8 +3129,7 @@ def test_torch_tensor_amin(
},
method_input_dtypes=input_dtype,
method_all_as_kwargs_np={
- "dim": axis,
- "keepdim": keepdim,
+ "other": x[1],
},
frontend_method_data=frontend_method_data,
init_flags=init_flags,
@@ -3108,17 +3139,19 @@ def test_torch_tensor_amin(
)
-# aminmax
+# arctan_
@handle_frontend_method(
class_tree=CLASS_TREE,
init_tree="torch.tensor",
- method_name="aminmax",
- dtype_input_axis=helpers.dtype_and_values(
- available_dtypes=helpers.get_dtypes("numeric"),
+ method_name="arctan_",
+ dtype_and_x=helpers.dtype_and_values(
+ available_dtypes=helpers.get_dtypes("float"),
+ allow_inf=False,
),
+ test_inplace=st.just(True),
)
-def test_torch_tensor_aminmax(
- dtype_input_axis,
+def test_torch_arctan_(
+ dtype_and_x,
frontend_method_data,
init_flags,
method_flags,
@@ -3126,7 +3159,7 @@ def test_torch_tensor_aminmax(
on_device,
backend_fw,
):
- input_dtype, x = dtype_input_axis
+ input_dtype, x = dtype_and_x
helpers.test_frontend_method(
init_input_dtypes=input_dtype,
backend_to_test=backend_fw,
@@ -3143,58 +3176,57 @@ def test_torch_tensor_aminmax(
)
-# angle
+# arctanh
@handle_frontend_method(
class_tree=CLASS_TREE,
init_tree="torch.tensor",
- method_name="angle",
- dtype_and_values=helpers.dtype_and_values(
- available_dtypes=["float64", "complex64", "complex128"],
+ method_name="arctanh",
+ dtype_and_x=helpers.dtype_and_values(
+ min_value=-1.0,
+ max_value=1.0,
+ available_dtypes=helpers.get_dtypes("float"),
),
)
-def test_torch_tensor_angle(
- dtype_and_values,
- frontend,
+def test_torch_arctanh(
+ dtype_and_x,
frontend_method_data,
init_flags,
method_flags,
+ frontend,
on_device,
+ backend_fw,
):
- input_dtype, values = dtype_and_values
-
+ input_dtype, x = dtype_and_x
helpers.test_frontend_method(
init_input_dtypes=input_dtype,
+ backend_to_test=backend_fw,
init_all_as_kwargs_np={
- "data": values[0],
+ "data": x[0],
},
- method_input_dtypes=input_dtype,
+ method_input_dtypes=[],
method_all_as_kwargs_np={},
+ frontend_method_data=frontend_method_data,
init_flags=init_flags,
method_flags=method_flags,
- frontend_method_data=frontend_method_data,
frontend=frontend,
on_device=on_device,
)
-# any
+# arctanh_
@handle_frontend_method(
class_tree=CLASS_TREE,
init_tree="torch.tensor",
- method_name="any",
- dtype_input_axis=helpers.dtype_values_axis(
- available_dtypes=helpers.get_dtypes("numeric"),
- min_num_dims=1,
- min_value=-1e04,
- max_value=1e04,
- valid_axis=True,
- force_int_axis=True,
+ method_name="arctanh_",
+ dtype_and_x=helpers.dtype_and_values(
+ min_value=-1.0,
+ max_value=1.0,
+ available_dtypes=helpers.get_dtypes("float"),
),
- keepdim=st.booleans(),
+ test_inplace=st.just(True),
)
-def test_torch_tensor_any(
- dtype_input_axis,
- keepdim,
+def test_torch_arctanh_(
+ dtype_and_x,
frontend_method_data,
init_flags,
method_flags,
@@ -3202,18 +3234,15 @@ def test_torch_tensor_any(
on_device,
backend_fw,
):
- input_dtype, x, axis = dtype_input_axis
+ input_dtype, x = dtype_and_x
helpers.test_frontend_method(
init_input_dtypes=input_dtype,
backend_to_test=backend_fw,
init_all_as_kwargs_np={
"data": x[0],
},
- method_input_dtypes=input_dtype,
- method_all_as_kwargs_np={
- "dim": axis,
- "keepdim": keepdim,
- },
+ method_input_dtypes=[],
+ method_all_as_kwargs_np={},
frontend_method_data=frontend_method_data,
init_flags=init_flags,
method_flags=method_flags,
@@ -3222,64 +3251,70 @@ def test_torch_tensor_any(
)
-# write test for torch instance apply_
-
-
+# argmax
@handle_frontend_method(
class_tree=CLASS_TREE,
- init_tree="torch.tensor",
- method_name="apply_",
- dtype_and_values=helpers.dtype_and_values(
- available_dtypes=helpers.get_dtypes("float"),
- num_arrays=1,
+ init_tree="torch.tensor",
+ method_name="argmax",
+ dtype_input_axis=helpers.dtype_values_axis(
+ available_dtypes=helpers.get_dtypes("valid"),
+ force_int_axis=True,
+ valid_axis=True,
),
- test_inplace=st.just(True),
+ keepdim=st.booleans(),
)
-def test_torch_tensor_apply_(
- dtype_and_values,
- frontend,
+def test_torch_argmax(
+ dtype_input_axis,
+ keepdim,
frontend_method_data,
init_flags,
method_flags,
+ frontend,
on_device,
backend_fw,
):
- def func(x):
- return x + 1
-
- input_dtype, values = dtype_and_values
-
+ input_dtype, x, axis = dtype_input_axis
helpers.test_frontend_method(
init_input_dtypes=input_dtype,
backend_to_test=backend_fw,
init_all_as_kwargs_np={
- "data": values[0],
+ "data": x[0],
},
method_input_dtypes=input_dtype,
method_all_as_kwargs_np={
- "callable": func,
+ "dim": axis,
+ "keepdim": keepdim,
},
+ frontend_method_data=frontend_method_data,
init_flags=init_flags,
method_flags=method_flags,
- frontend_method_data=frontend_method_data,
frontend=frontend,
on_device=on_device,
)
-# arccos
+# argmin
@handle_frontend_method(
class_tree=CLASS_TREE,
init_tree="torch.tensor",
- method_name="arccos",
- dtype_and_x=helpers.dtype_and_values(
- min_value=-1.0,
- max_value=1.0,
- available_dtypes=helpers.get_dtypes("float"),
+ method_name="argmin",
+ dtype_input_axis=helpers.dtype_values_axis(
+ available_dtypes=helpers.get_dtypes("numeric"),
+ force_int_axis=True,
+ min_num_dims=1,
+ max_num_dims=3,
+ min_dim_size=1,
+ max_dim_size=3,
+ min_value=1,
+ max_value=5,
+ valid_axis=True,
+ allow_neg_axes=True,
),
+ keepdim=st.booleans(),
)
-def test_torch_tensor_arccos(
- dtype_and_x,
+def test_torch_argmin(
+ dtype_input_axis,
+ keepdim,
frontend_method_data,
init_flags,
method_flags,
@@ -3287,15 +3322,18 @@ def test_torch_tensor_arccos(
on_device,
backend_fw,
):
- input_dtype, x = dtype_and_x
+ input_dtype, x, axis = dtype_input_axis
helpers.test_frontend_method(
init_input_dtypes=input_dtype,
backend_to_test=backend_fw,
init_all_as_kwargs_np={
"data": x[0],
},
- method_input_dtypes=[],
- method_all_as_kwargs_np={},
+ method_input_dtypes=input_dtype,
+ method_all_as_kwargs_np={
+ "dim": axis,
+ "keepdim": keepdim,
+ },
frontend_method_data=frontend_method_data,
init_flags=init_flags,
method_flags=method_flags,
@@ -3304,20 +3342,28 @@ def test_torch_tensor_arccos(
)
-# arccos_
+# argsort
@handle_frontend_method(
class_tree=CLASS_TREE,
init_tree="torch.tensor",
- method_name="arccos_",
- dtype_and_x=helpers.dtype_and_values(
- min_value=-1.0,
- max_value=1.0,
- available_dtypes=helpers.get_dtypes("float"),
+ method_name="argsort",
+ dtype_input_axis=helpers.dtype_values_axis(
+ available_dtypes=helpers.get_dtypes("numeric"),
+ force_int_axis=True,
+ min_num_dims=1,
+ max_num_dims=3,
+ min_dim_size=1,
+ max_dim_size=3,
+ min_value=1,
+ max_value=5,
+ valid_axis=True,
+ allow_neg_axes=True,
),
- test_inplace=st.just(True),
+ descending=st.booleans(),
)
-def test_torch_tensor_arccos_(
- dtype_and_x,
+def test_torch_argsort(
+ dtype_input_axis,
+ descending,
frontend_method_data,
init_flags,
method_flags,
@@ -3325,15 +3371,18 @@ def test_torch_tensor_arccos_(
on_device,
backend_fw,
):
- input_dtype, x = dtype_and_x
+ input_dtype, x, axis = dtype_input_axis
helpers.test_frontend_method(
init_input_dtypes=input_dtype,
backend_to_test=backend_fw,
init_all_as_kwargs_np={
"data": x[0],
},
- method_input_dtypes=[],
- method_all_as_kwargs_np={},
+ method_input_dtypes=input_dtype,
+ method_all_as_kwargs_np={
+ "dim": axis,
+ "descending": descending,
+ },
frontend_method_data=frontend_method_data,
init_flags=init_flags,
method_flags=method_flags,
@@ -3342,18 +3391,16 @@ def test_torch_tensor_arccos_(
)
-# arccosh
+# argwhere
@handle_frontend_method(
class_tree=CLASS_TREE,
init_tree="torch.tensor",
- method_name="arccosh",
+ method_name="argwhere",
dtype_and_x=helpers.dtype_and_values(
- min_value=-1.0,
- max_value=1.0,
- available_dtypes=helpers.get_dtypes("float"),
+ available_dtypes=helpers.get_dtypes("valid"),
),
)
-def test_torch_tensor_arccosh(
+def test_torch_argwhere(
dtype_and_x,
frontend_method_data,
init_flags,
@@ -3369,7 +3416,7 @@ def test_torch_tensor_arccosh(
init_all_as_kwargs_np={
"data": x[0],
},
- method_input_dtypes=[],
+ method_input_dtypes=input_dtype,
method_all_as_kwargs_np={},
frontend_method_data=frontend_method_data,
init_flags=init_flags,
@@ -3379,55 +3426,51 @@ def test_torch_tensor_arccosh(
)
-# arccosh_
@handle_frontend_method(
class_tree=CLASS_TREE,
init_tree="torch.tensor",
- method_name="arccosh_",
- dtype_and_x=helpers.dtype_and_values(
- min_value=-1.0,
- max_value=1.0,
- available_dtypes=helpers.get_dtypes("float"),
- ),
- test_inplace=st.just(True),
+ method_name="as_strided",
+ dtype_x_and_other=_as_strided_helper(),
)
-def test_torch_tensor_arccosh_(
- dtype_and_x,
+def test_torch_as_strided(
+ dtype_x_and_other,
+ frontend,
frontend_method_data,
init_flags,
method_flags,
- frontend,
on_device,
backend_fw,
):
- input_dtype, x = dtype_and_x
+ input_dtype, x, size, stride, offset = dtype_x_and_other
helpers.test_frontend_method(
init_input_dtypes=input_dtype,
backend_to_test=backend_fw,
- init_all_as_kwargs_np={
- "data": x[0],
+ init_all_as_kwargs_np={"data": x[0]},
+ method_input_dtypes=input_dtype,
+ method_all_as_kwargs_np={
+ "size": size,
+ "stride": stride,
+ "storage_offset": offset,
},
- method_input_dtypes=[],
- method_all_as_kwargs_np={},
+ frontend=frontend,
frontend_method_data=frontend_method_data,
init_flags=init_flags,
method_flags=method_flags,
- frontend=frontend,
on_device=on_device,
)
-# arcsin
+# asin
@handle_frontend_method(
class_tree=CLASS_TREE,
init_tree="torch.tensor",
- method_name="arcsin",
+ method_name="asin",
dtype_and_x=helpers.dtype_and_values(
available_dtypes=helpers.get_dtypes("float"),
allow_inf=False,
),
)
-def test_torch_tensor_arcsin(
+def test_torch_asin(
dtype_and_x,
frontend_method_data,
init_flags,
@@ -3453,19 +3496,18 @@ def test_torch_tensor_arcsin(
)
-# arcsin_
+# asin_
@handle_frontend_method(
class_tree=CLASS_TREE,
init_tree="torch.tensor",
- method_name="arcsin_",
+ method_name="asin_",
dtype_and_x=helpers.dtype_and_values(
min_value=-1.0,
max_value=1.0,
available_dtypes=helpers.get_dtypes("float"),
),
- test_inplace=st.just(True),
)
-def test_torch_tensor_arcsin_(
+def test_torch_asin_(
dtype_and_x,
frontend_method_data,
init_flags,
@@ -3491,18 +3533,17 @@ def test_torch_tensor_arcsin_(
)
-# arcsinh
+# asinh
@handle_frontend_method(
class_tree=CLASS_TREE,
init_tree="torch.tensor",
- method_name="arcsinh",
+ method_name="asinh",
dtype_and_x=helpers.dtype_and_values(
- min_value=-1.0,
- max_value=1.0,
available_dtypes=helpers.get_dtypes("float"),
+ allow_inf=False,
),
)
-def test_torch_tensor_arcsinh(
+def test_torch_asinh(
dtype_and_x,
frontend_method_data,
init_flags,
@@ -3518,29 +3559,30 @@ def test_torch_tensor_arcsinh(
init_all_as_kwargs_np={
"data": x[0],
},
- method_input_dtypes=[],
+ method_input_dtypes=input_dtype,
method_all_as_kwargs_np={},
frontend_method_data=frontend_method_data,
init_flags=init_flags,
method_flags=method_flags,
frontend=frontend,
+ rtol_=1e-2,
+ atol_=1e-2,
on_device=on_device,
)
-# arcsinh_
+# asinh_
@handle_frontend_method(
class_tree=CLASS_TREE,
init_tree="torch.tensor",
- method_name="arcsinh_",
+ method_name="asinh_",
dtype_and_x=helpers.dtype_and_values(
- min_value=-1.0,
- max_value=1.0,
available_dtypes=helpers.get_dtypes("float"),
+ allow_inf=False,
),
test_inplace=st.just(True),
)
-def test_torch_tensor_arcsinh_(
+def test_torch_asinh_(
dtype_and_x,
frontend_method_data,
init_flags,
@@ -3556,27 +3598,29 @@ def test_torch_tensor_arcsinh_(
init_all_as_kwargs_np={
"data": x[0],
},
- method_input_dtypes=[],
+ method_input_dtypes=input_dtype,
method_all_as_kwargs_np={},
frontend_method_data=frontend_method_data,
init_flags=init_flags,
method_flags=method_flags,
frontend=frontend,
+ rtol_=1e-2,
+ atol_=1e-2,
on_device=on_device,
)
-# arctan
+# atan
@handle_frontend_method(
class_tree=CLASS_TREE,
init_tree="torch.tensor",
- method_name="arctan",
+ method_name="atan",
dtype_and_x=helpers.dtype_and_values(
available_dtypes=helpers.get_dtypes("float"),
allow_inf=False,
),
)
-def test_torch_tensor_arctan(
+def test_torch_atan(
dtype_and_x,
frontend_method_data,
init_flags,
@@ -3602,17 +3646,17 @@ def test_torch_tensor_arctan(
)
-# arctan2
+# atan2
@handle_frontend_method(
class_tree=CLASS_TREE,
init_tree="torch.tensor",
- method_name="arctan2",
+ method_name="atan2",
dtype_and_x=helpers.dtype_and_values(
available_dtypes=helpers.get_dtypes("float"),
num_arrays=2,
),
)
-def test_torch_tensor_arctan2(
+def test_torch_atan2(
dtype_and_x,
frontend_method_data,
init_flags,
@@ -3640,17 +3684,18 @@ def test_torch_tensor_arctan2(
)
-# arctan2_
+# atan2_
@handle_frontend_method(
class_tree=CLASS_TREE,
init_tree="torch.tensor",
- method_name="arctan2_",
+ method_name="atan2_",
dtype_and_x=helpers.dtype_and_values(
available_dtypes=helpers.get_dtypes("float"),
num_arrays=2,
),
+ test_inplace=st.just(True),
)
-def test_torch_tensor_arctan2_(
+def test_torch_atan2_(
dtype_and_x,
frontend_method_data,
init_flags,
@@ -3678,18 +3723,18 @@ def test_torch_tensor_arctan2_(
)
-# arctan_
+# atan_
@handle_frontend_method(
class_tree=CLASS_TREE,
init_tree="torch.tensor",
- method_name="arctan_",
+ method_name="atan_",
dtype_and_x=helpers.dtype_and_values(
available_dtypes=helpers.get_dtypes("float"),
allow_inf=False,
),
test_inplace=st.just(True),
)
-def test_torch_tensor_arctan_(
+def test_torch_atan_(
dtype_and_x,
frontend_method_data,
init_flags,
@@ -3705,7 +3750,7 @@ def test_torch_tensor_arctan_(
init_all_as_kwargs_np={
"data": x[0],
},
- method_input_dtypes=input_dtype,
+ method_input_dtypes=[],
method_all_as_kwargs_np={},
frontend_method_data=frontend_method_data,
init_flags=init_flags,
@@ -3715,18 +3760,18 @@ def test_torch_tensor_arctan_(
)
-# arctanh
+# atanh
@handle_frontend_method(
class_tree=CLASS_TREE,
init_tree="torch.tensor",
- method_name="arctanh",
+ method_name="atanh",
dtype_and_x=helpers.dtype_and_values(
min_value=-1.0,
max_value=1.0,
available_dtypes=helpers.get_dtypes("float"),
),
)
-def test_torch_tensor_arctanh(
+def test_torch_atanh(
dtype_and_x,
frontend_method_data,
init_flags,
@@ -3752,11 +3797,11 @@ def test_torch_tensor_arctanh(
)
-# arctanh_
+# atanh_
@handle_frontend_method(
class_tree=CLASS_TREE,
init_tree="torch.tensor",
- method_name="arctanh_",
+ method_name="atanh_",
dtype_and_x=helpers.dtype_and_values(
min_value=-1.0,
max_value=1.0,
@@ -3764,7 +3809,7 @@ def test_torch_tensor_arctanh(
),
test_inplace=st.just(True),
)
-def test_torch_tensor_arctanh_(
+def test_torch_atanh_(
dtype_and_x,
frontend_method_data,
init_flags,
@@ -3790,145 +3835,206 @@ def test_torch_tensor_arctanh_(
)
-# argmax
+@given(
+ dtype_x=helpers.dtype_and_values(
+ available_dtypes=helpers.get_dtypes("float", prune_function=False),
+ num_arrays=3,
+ min_value=-1e3,
+ max_value=1e3,
+ ).filter(lambda x: all(dt == "float32" for dt in x[0])),
+)
+def test_torch_backward(
+ dtype_x,
+ backend_fw,
+):
+ ivy.set_backend(backend_fw)
+ if ivy.current_backend_str() == "numpy":
+ ivy.warnings.warn("Gradient calculation unavailable for numpy backend")
+ return
+ if ivy.current_backend_str() == "paddle":
+ ivy.warnings.warn("torch.Tensor.backward() unavailable for paddle backend")
+ return
+ _, values = dtype_x
+ x = Tensor(values[0], requires_grad=True)
+ y = Tensor(values[1], requires_grad=True)
+ z = Tensor(values[2], requires_grad=True)
+ a = x + y.pow(2)
+ b = z * a
+ c = b.sum()
+ c.backward()
+ x_torch = torch.tensor(values[0], requires_grad=True, dtype=torch.float32)
+ y_torch = torch.tensor(values[1], requires_grad=True, dtype=torch.float32)
+ z_torch = torch.tensor(values[2], requires_grad=True, dtype=torch.float32)
+ a_torch = x_torch + y_torch.pow(2)
+ b_torch = z_torch * a_torch
+ c_torch = b_torch.sum()
+ c_torch.backward()
+ helpers.assertions.value_test(
+ ret_np_flat=helpers.flatten_and_to_np(
+ ret=x._grads.ivy_array, backend=backend_fw
+ ),
+ ret_np_from_gt_flat=helpers.flatten_and_to_np(
+ ret=ivy.to_ivy(x_torch.grad.numpy()), backend=backend_fw
+ ),
+ rtol=1e-3,
+ atol=1e-3,
+ backend="torch",
+ )
+ helpers.assertions.value_test(
+ ret_np_flat=helpers.flatten_and_to_np(
+ ret=y._grads.ivy_array, backend=backend_fw
+ ),
+ ret_np_from_gt_flat=helpers.flatten_and_to_np(
+ ret=ivy.to_ivy(y_torch.grad.numpy()), backend=backend_fw
+ ),
+ rtol=1e-3,
+ atol=1e-3,
+ backend="torch",
+ )
+ helpers.assertions.value_test(
+ ret_np_flat=helpers.flatten_and_to_np(
+ ret=z._grads.ivy_array, backend=backend_fw
+ ),
+ ret_np_from_gt_flat=helpers.flatten_and_to_np(
+ ret=ivy.to_ivy(z_torch.grad.numpy()), backend=backend_fw
+ ),
+ rtol=1e-3,
+ atol=1e-3,
+ backend="torch",
+ )
+
+
@handle_frontend_method(
class_tree=CLASS_TREE,
init_tree="torch.tensor",
- method_name="argmax",
- dtype_input_axis=helpers.dtype_values_axis(
- available_dtypes=helpers.get_dtypes("numeric"),
- force_int_axis=True,
- min_num_dims=1,
- max_num_dims=3,
- min_dim_size=1,
- max_dim_size=3,
- min_value=1,
+ method_name="baddbmm",
+ dtype_and_matrices=_get_dtype_and_3dbatch_matrices(with_input=True, input_3d=True),
+ beta=st.floats(
+ min_value=-5,
max_value=5,
- valid_axis=True,
- allow_neg_axes=True,
+ allow_nan=False,
+ allow_subnormal=False,
+ allow_infinity=False,
+ ),
+ alpha=st.floats(
+ min_value=-5,
+ max_value=5,
+ allow_nan=False,
+ allow_subnormal=False,
+ allow_infinity=False,
),
- keepdim=st.booleans(),
)
-def test_torch_tensor_argmax(
- dtype_input_axis,
- keepdim,
+def test_torch_baddbmm(
+ dtype_and_matrices,
+ beta,
+ alpha,
+ frontend,
frontend_method_data,
init_flags,
method_flags,
- frontend,
on_device,
backend_fw,
):
- input_dtype, x, axis = dtype_input_axis
+ input_dtype, x, batch1, batch2 = dtype_and_matrices
helpers.test_frontend_method(
init_input_dtypes=input_dtype,
backend_to_test=backend_fw,
- init_all_as_kwargs_np={
- "data": x[0],
- },
+ init_all_as_kwargs_np={"data": x[0]},
method_input_dtypes=input_dtype,
method_all_as_kwargs_np={
- "dim": axis,
- "keepdim": keepdim,
+ "batch1": batch1,
+ "batch2": batch2,
+ "beta": beta,
+ "alpha": alpha,
},
+ frontend=frontend,
frontend_method_data=frontend_method_data,
init_flags=init_flags,
method_flags=method_flags,
- frontend=frontend,
on_device=on_device,
)
-# argmin
@handle_frontend_method(
class_tree=CLASS_TREE,
init_tree="torch.tensor",
- method_name="argmin",
- dtype_input_axis=helpers.dtype_values_axis(
- available_dtypes=helpers.get_dtypes("numeric"),
- force_int_axis=True,
- min_num_dims=1,
- max_num_dims=3,
- min_dim_size=1,
- max_dim_size=3,
- min_value=1,
+ method_name="baddbmm_",
+ dtype_and_matrices=_get_dtype_and_3dbatch_matrices(with_input=True, input_3d=True),
+ beta=st.floats(
+ min_value=-5,
max_value=5,
- valid_axis=True,
- allow_neg_axes=True,
+ allow_nan=False,
+ allow_subnormal=False,
+ allow_infinity=False,
),
- keepdim=st.booleans(),
+ alpha=st.floats(
+ min_value=-5,
+ max_value=5,
+ allow_nan=False,
+ allow_subnormal=False,
+ allow_infinity=False,
+ ),
+ test_inplace=st.just(True),
)
-def test_torch_tensor_argmin(
- dtype_input_axis,
- keepdim,
+def test_torch_baddbmm_(
+ dtype_and_matrices,
+ beta,
+ alpha,
+ frontend,
frontend_method_data,
init_flags,
method_flags,
- frontend,
on_device,
backend_fw,
):
- input_dtype, x, axis = dtype_input_axis
+ input_dtype, x, batch1, batch2 = dtype_and_matrices
helpers.test_frontend_method(
init_input_dtypes=input_dtype,
backend_to_test=backend_fw,
- init_all_as_kwargs_np={
- "data": x[0],
- },
+ init_all_as_kwargs_np={"data": x[0]},
method_input_dtypes=input_dtype,
method_all_as_kwargs_np={
- "dim": axis,
- "keepdim": keepdim,
+ "batch1": batch1,
+ "batch2": batch2,
+ "beta": beta,
+ "alpha": alpha,
},
+ frontend=frontend,
frontend_method_data=frontend_method_data,
init_flags=init_flags,
method_flags=method_flags,
- frontend=frontend,
on_device=on_device,
)
-# argsort
+# bernoulli
@handle_frontend_method(
class_tree=CLASS_TREE,
init_tree="torch.tensor",
- method_name="argsort",
- dtype_input_axis=helpers.dtype_values_axis(
- available_dtypes=helpers.get_dtypes("numeric"),
- force_int_axis=True,
- min_num_dims=1,
- max_num_dims=3,
- min_dim_size=1,
- max_dim_size=3,
- min_value=1,
- max_value=5,
- valid_axis=True,
- allow_neg_axes=True,
+ method_name="bernoulli",
+ dtype_and_x=helpers.dtype_and_values(
+ available_dtypes=helpers.get_dtypes("valid"),
),
- descending=st.booleans(),
+ test_with_out=st.just(True),
)
-def test_torch_tensor_argsort(
- dtype_input_axis,
- descending,
+def test_torch_bernoulli(
+ dtype_and_x,
+ frontend,
frontend_method_data,
init_flags,
method_flags,
- frontend,
on_device,
backend_fw,
):
- input_dtype, x, axis = dtype_input_axis
+ input_dtype, x = dtype_and_x
helpers.test_frontend_method(
init_input_dtypes=input_dtype,
backend_to_test=backend_fw,
init_all_as_kwargs_np={
- "data": x[0],
+ "input": x[0],
},
method_input_dtypes=input_dtype,
- method_all_as_kwargs_np={
- "dim": axis,
- "descending": descending,
- },
+ method_all_as_kwargs_np={"generator": x[1], "out": x[2]},
frontend_method_data=frontend_method_data,
init_flags=init_flags,
method_flags=method_flags,
@@ -3937,16 +4043,17 @@ def test_torch_tensor_argsort(
)
-# argwhere
+# bitwise_and
@handle_frontend_method(
class_tree=CLASS_TREE,
init_tree="torch.tensor",
- method_name="argwhere",
+ method_name="bitwise_and",
dtype_and_x=helpers.dtype_and_values(
- available_dtypes=helpers.get_dtypes("valid"),
+ available_dtypes=helpers.get_dtypes("integer"),
+ num_arrays=2,
),
)
-def test_torch_tensor_argwhere(
+def test_torch_bitwise_and(
dtype_and_x,
frontend_method_data,
init_flags,
@@ -3963,60 +4070,29 @@ def test_torch_tensor_argwhere(
"data": x[0],
},
method_input_dtypes=input_dtype,
- method_all_as_kwargs_np={},
- frontend_method_data=frontend_method_data,
- init_flags=init_flags,
- method_flags=method_flags,
- frontend=frontend,
- on_device=on_device,
- )
-
-
-@handle_frontend_method(
- class_tree=CLASS_TREE,
- init_tree="torch.tensor",
- method_name="as_strided",
- dtype_x_and_other=_as_strided_helper(),
-)
-def test_torch_tensor_as_strided(
- dtype_x_and_other,
- frontend,
- frontend_method_data,
- init_flags,
- method_flags,
- on_device,
- backend_fw,
-):
- input_dtype, x, size, stride, offset = dtype_x_and_other
- helpers.test_frontend_method(
- init_input_dtypes=input_dtype,
- backend_to_test=backend_fw,
- init_all_as_kwargs_np={"data": x[0]},
- method_input_dtypes=input_dtype,
method_all_as_kwargs_np={
- "size": size,
- "stride": stride,
- "storage_offset": offset,
+ "other": x[1],
},
- frontend=frontend,
frontend_method_data=frontend_method_data,
init_flags=init_flags,
method_flags=method_flags,
+ frontend=frontend,
on_device=on_device,
)
-# asin
+# bitwise_and_
@handle_frontend_method(
class_tree=CLASS_TREE,
init_tree="torch.tensor",
- method_name="asin",
+ method_name="bitwise_and_",
dtype_and_x=helpers.dtype_and_values(
- available_dtypes=helpers.get_dtypes("float"),
- allow_inf=False,
+ available_dtypes=helpers.get_dtypes("integer"),
+ num_arrays=2,
),
+ test_inplace=st.just(True),
)
-def test_torch_tensor_asin(
+def test_torch_bitwise_and_(
dtype_and_x,
frontend_method_data,
init_flags,
@@ -4033,7 +4109,9 @@ def test_torch_tensor_asin(
"data": x[0],
},
method_input_dtypes=input_dtype,
- method_all_as_kwargs_np={},
+ method_all_as_kwargs_np={
+ "other": x[1],
+ },
frontend_method_data=frontend_method_data,
init_flags=init_flags,
method_flags=method_flags,
@@ -4042,18 +4120,17 @@ def test_torch_tensor_asin(
)
-# asin_
+# bitwise_left_shift
@handle_frontend_method(
class_tree=CLASS_TREE,
init_tree="torch.tensor",
- method_name="asin_",
+ method_name="bitwise_left_shift",
dtype_and_x=helpers.dtype_and_values(
- min_value=-1.0,
- max_value=1.0,
- available_dtypes=helpers.get_dtypes("float"),
+ available_dtypes=helpers.get_dtypes("integer"),
+ num_arrays=2,
),
)
-def test_torch_tensor_asin_(
+def test_torch_bitwise_left_shift(
dtype_and_x,
frontend_method_data,
init_flags,
@@ -4069,8 +4146,10 @@ def test_torch_tensor_asin_(
init_all_as_kwargs_np={
"data": x[0],
},
- method_input_dtypes=[],
- method_all_as_kwargs_np={},
+ method_input_dtypes=input_dtype,
+ method_all_as_kwargs_np={
+ "other": x[1],
+ },
frontend_method_data=frontend_method_data,
init_flags=init_flags,
method_flags=method_flags,
@@ -4079,17 +4158,17 @@ def test_torch_tensor_asin_(
)
-# asinh
+# bitwise_not
@handle_frontend_method(
class_tree=CLASS_TREE,
init_tree="torch.tensor",
- method_name="asinh",
+ method_name="bitwise_not",
dtype_and_x=helpers.dtype_and_values(
- available_dtypes=helpers.get_dtypes("float"),
- allow_inf=False,
+ available_dtypes=helpers.get_dtypes("integer"),
+ num_arrays=2,
),
)
-def test_torch_tensor_asinh(
+def test_torch_bitwise_not(
dtype_and_x,
frontend_method_data,
init_flags,
@@ -4106,29 +4185,27 @@ def test_torch_tensor_asinh(
"data": x[0],
},
method_input_dtypes=input_dtype,
- method_all_as_kwargs_np={},
frontend_method_data=frontend_method_data,
init_flags=init_flags,
method_flags=method_flags,
+ method_all_as_kwargs_np={},
frontend=frontend,
- rtol_=1e-2,
- atol_=1e-2,
on_device=on_device,
)
-# asinh_
+# bitwise_not_
@handle_frontend_method(
class_tree=CLASS_TREE,
init_tree="torch.tensor",
- method_name="asinh_",
+ method_name="bitwise_not_",
dtype_and_x=helpers.dtype_and_values(
- available_dtypes=helpers.get_dtypes("float"),
- allow_inf=False,
+ available_dtypes=helpers.get_dtypes("integer"),
+ num_arrays=2,
),
test_inplace=st.just(True),
)
-def test_torch_tensor_asinh_(
+def test_torch_bitwise_not_(
dtype_and_x,
frontend_method_data,
init_flags,
@@ -4145,28 +4222,26 @@ def test_torch_tensor_asinh_(
"data": x[0],
},
method_input_dtypes=input_dtype,
- method_all_as_kwargs_np={},
frontend_method_data=frontend_method_data,
init_flags=init_flags,
method_flags=method_flags,
+ method_all_as_kwargs_np={},
frontend=frontend,
- rtol_=1e-2,
- atol_=1e-2,
on_device=on_device,
)
-# atan
+# bitwise_or
@handle_frontend_method(
class_tree=CLASS_TREE,
init_tree="torch.tensor",
- method_name="atan",
+ method_name="bitwise_or",
dtype_and_x=helpers.dtype_and_values(
- available_dtypes=helpers.get_dtypes("float"),
- allow_inf=False,
+ available_dtypes=helpers.get_dtypes("valid"),
+ num_arrays=2,
),
)
-def test_torch_tensor_atan(
+def test_torch_bitwise_or(
dtype_and_x,
frontend_method_data,
init_flags,
@@ -4183,7 +4258,9 @@ def test_torch_tensor_atan(
"data": x[0],
},
method_input_dtypes=input_dtype,
- method_all_as_kwargs_np={},
+ method_all_as_kwargs_np={
+ "other": x[1],
+ },
frontend_method_data=frontend_method_data,
init_flags=init_flags,
method_flags=method_flags,
@@ -4192,17 +4269,18 @@ def test_torch_tensor_atan(
)
-# atan2
+# bitwise_or_
@handle_frontend_method(
class_tree=CLASS_TREE,
init_tree="torch.tensor",
- method_name="atan2",
+ method_name="bitwise_or_",
dtype_and_x=helpers.dtype_and_values(
- available_dtypes=helpers.get_dtypes("float"),
+ available_dtypes=helpers.get_dtypes("valid"),
num_arrays=2,
),
+ test_inplace=st.just(True),
)
-def test_torch_tensor_atan2(
+def test_torch_bitwise_or_(
dtype_and_x,
frontend_method_data,
init_flags,
@@ -4230,18 +4308,18 @@ def test_torch_tensor_atan2(
)
-# atan2_
+# bitwise right shift
@handle_frontend_method(
class_tree=CLASS_TREE,
init_tree="torch.tensor",
- method_name="atan2_",
+ method_name="bitwise_right_shift",
dtype_and_x=helpers.dtype_and_values(
- available_dtypes=helpers.get_dtypes("float"),
+ available_dtypes=helpers.get_dtypes("valid"),
num_arrays=2,
+ shared_dtype=True,
),
- test_inplace=st.just(True),
)
-def test_torch_tensor_atan2_(
+def test_torch_bitwise_right_shift(
dtype_and_x,
frontend_method_data,
init_flags,
@@ -4251,6 +4329,11 @@ def test_torch_tensor_atan2_(
backend_fw,
):
input_dtype, x = dtype_and_x
+ # negative shifts will throw an exception
+ # shifts >= dtype width produce backend-defined behavior
+ x[1] = np.asarray(
+ np.clip(x[1], 0, np.iinfo(input_dtype[1]).bits - 1), dtype=input_dtype[1]
+ )
helpers.test_frontend_method(
init_input_dtypes=input_dtype,
backend_to_test=backend_fw,
@@ -4269,18 +4352,18 @@ def test_torch_tensor_atan2_(
)
-# atan_
+# bitwise_right_shift_
@handle_frontend_method(
class_tree=CLASS_TREE,
init_tree="torch.tensor",
- method_name="atan_",
+ method_name="bitwise_right_shift_",
dtype_and_x=helpers.dtype_and_values(
- available_dtypes=helpers.get_dtypes("float"),
- allow_inf=False,
+ available_dtypes=helpers.get_dtypes("valid"),
+ num_arrays=2,
+ shared_dtype=True,
),
- test_inplace=st.just(True),
)
-def test_torch_tensor_atan_(
+def test_torch_bitwise_right_shift_(
dtype_and_x,
frontend_method_data,
init_flags,
@@ -4290,14 +4373,21 @@ def test_torch_tensor_atan_(
backend_fw,
):
input_dtype, x = dtype_and_x
+ # negative shifts will throw an exception
+ # shifts >= dtype width produce backend-defined behavior
+ x[1] = np.asarray(
+ np.clip(x[1], 0, np.iinfo(input_dtype[1]).bits - 1), dtype=input_dtype[1]
+ )
helpers.test_frontend_method(
init_input_dtypes=input_dtype,
backend_to_test=backend_fw,
init_all_as_kwargs_np={
"data": x[0],
},
- method_input_dtypes=[],
- method_all_as_kwargs_np={},
+ method_input_dtypes=input_dtype,
+ method_all_as_kwargs_np={
+ "other": x[1],
+ },
frontend_method_data=frontend_method_data,
init_flags=init_flags,
method_flags=method_flags,
@@ -4306,18 +4396,17 @@ def test_torch_tensor_atan_(
)
-# atanh
+# bitwise_xor
@handle_frontend_method(
class_tree=CLASS_TREE,
init_tree="torch.tensor",
- method_name="atanh",
+ method_name="bitwise_xor",
dtype_and_x=helpers.dtype_and_values(
- min_value=-1.0,
- max_value=1.0,
- available_dtypes=helpers.get_dtypes("float"),
+ available_dtypes=st.one_of(st.just(("bool",)), helpers.get_dtypes("integer")),
+ num_arrays=2,
),
)
-def test_torch_tensor_atanh(
+def test_torch_bitwise_xor(
dtype_and_x,
frontend_method_data,
init_flags,
@@ -4333,8 +4422,10 @@ def test_torch_tensor_atanh(
init_all_as_kwargs_np={
"data": x[0],
},
- method_input_dtypes=[],
- method_all_as_kwargs_np={},
+ method_input_dtypes=input_dtype,
+ method_all_as_kwargs_np={
+ "other": x[1],
+ },
frontend_method_data=frontend_method_data,
init_flags=init_flags,
method_flags=method_flags,
@@ -4343,19 +4434,18 @@ def test_torch_tensor_atanh(
)
-# atanh_
+# bitwise_xor_
@handle_frontend_method(
class_tree=CLASS_TREE,
init_tree="torch.tensor",
- method_name="atanh_",
+ method_name="bitwise_xor_",
dtype_and_x=helpers.dtype_and_values(
- min_value=-1.0,
- max_value=1.0,
- available_dtypes=helpers.get_dtypes("float"),
+ available_dtypes=st.one_of(st.just(("bool",)), helpers.get_dtypes("integer")),
+ num_arrays=2,
),
test_inplace=st.just(True),
)
-def test_torch_tensor_atanh_(
+def test_torch_bitwise_xor_(
dtype_and_x,
frontend_method_data,
init_flags,
@@ -4371,8 +4461,10 @@ def test_torch_tensor_atanh_(
init_all_as_kwargs_np={
"data": x[0],
},
- method_input_dtypes=[],
- method_all_as_kwargs_np={},
+ method_input_dtypes=input_dtype,
+ method_all_as_kwargs_np={
+ "other": x[1],
+ },
frontend_method_data=frontend_method_data,
init_flags=init_flags,
method_flags=method_flags,
@@ -4381,142 +4473,52 @@ def test_torch_tensor_atanh_(
)
-@given(
- dtype_x=helpers.dtype_and_values(
- available_dtypes=helpers.get_dtypes("float", prune_function=False),
- num_arrays=3,
- min_value=-1e3,
- max_value=1e3,
- ).filter(lambda x: all(dt == "float32" for dt in x[0])),
-)
-def test_torch_tensor_backward(
- dtype_x,
- backend_fw,
-):
- ivy.set_backend(backend_fw)
- if ivy.current_backend_str() == "numpy":
- ivy.warnings.warn("Gradient calculation unavailable for numpy backend")
- return
- if ivy.current_backend_str() == "paddle":
- ivy.warnings.warn("torch.Tensor.backward() unavailable for paddle backend")
- return
- _, values = dtype_x
- x = Tensor(values[0], requires_grad=True)
- y = Tensor(values[1], requires_grad=True)
- z = Tensor(values[2], requires_grad=True)
- a = x + y.pow(2)
- b = z * a
- c = b.sum()
- c.backward()
- x_torch = torch.tensor(values[0], requires_grad=True, dtype=torch.float32)
- y_torch = torch.tensor(values[1], requires_grad=True, dtype=torch.float32)
- z_torch = torch.tensor(values[2], requires_grad=True, dtype=torch.float32)
- a_torch = x_torch + y_torch.pow(2)
- b_torch = z_torch * a_torch
- c_torch = b_torch.sum()
- c_torch.backward()
- helpers.assertions.value_test(
- ret_np_flat=helpers.flatten_and_to_np(
- ret=x._grads.ivy_array, backend=backend_fw
- ),
- ret_np_from_gt_flat=helpers.flatten_and_to_np(
- ret=ivy.to_ivy(x_torch.grad.numpy()), backend=backend_fw
- ),
- rtol=1e-3,
- atol=1e-3,
- backend="torch",
- )
- helpers.assertions.value_test(
- ret_np_flat=helpers.flatten_and_to_np(
- ret=y._grads.ivy_array, backend=backend_fw
- ),
- ret_np_from_gt_flat=helpers.flatten_and_to_np(
- ret=ivy.to_ivy(y_torch.grad.numpy()), backend=backend_fw
- ),
- rtol=1e-3,
- atol=1e-3,
- backend="torch",
- )
- helpers.assertions.value_test(
- ret_np_flat=helpers.flatten_and_to_np(
- ret=z._grads.ivy_array, backend=backend_fw
- ),
- ret_np_from_gt_flat=helpers.flatten_and_to_np(
- ret=ivy.to_ivy(z_torch.grad.numpy()), backend=backend_fw
- ),
- rtol=1e-3,
- atol=1e-3,
- backend="torch",
- )
-
-
@handle_frontend_method(
class_tree=CLASS_TREE,
init_tree="torch.tensor",
- method_name="baddbmm",
+ method_name="bmm",
dtype_and_matrices=_get_dtype_and_3dbatch_matrices(with_input=True, input_3d=True),
- beta=st.floats(
- min_value=-5,
- max_value=5,
- allow_nan=False,
- allow_subnormal=False,
- allow_infinity=False,
- ),
- alpha=st.floats(
- min_value=-5,
- max_value=5,
- allow_nan=False,
- allow_subnormal=False,
- allow_infinity=False,
- ),
)
-def test_torch_tensor_baddbmm(
+def test_torch_bmm(
dtype_and_matrices,
- beta,
- alpha,
+ backend_fw,
frontend,
frontend_method_data,
init_flags,
method_flags,
on_device,
- backend_fw,
):
- input_dtype, x, batch1, batch2 = dtype_and_matrices
+ input_dtype, _, x, mat2 = dtype_and_matrices
helpers.test_frontend_method(
init_input_dtypes=input_dtype,
- backend_to_test=backend_fw,
- init_all_as_kwargs_np={"data": x[0]},
+ init_all_as_kwargs_np={"data": x},
method_input_dtypes=input_dtype,
- method_all_as_kwargs_np={
- "batch1": batch1,
- "batch2": batch2,
- "beta": beta,
- "alpha": alpha,
- },
+ method_all_as_kwargs_np={"mat2": mat2},
frontend=frontend,
frontend_method_data=frontend_method_data,
init_flags=init_flags,
method_flags=method_flags,
on_device=on_device,
+ backend_to_test=backend_fw,
)
-# bernoulli
+# bool
@handle_frontend_method(
class_tree=CLASS_TREE,
init_tree="torch.tensor",
- method_name="bernoulli",
+ method_name="bool",
dtype_and_x=helpers.dtype_and_values(
- available_dtypes=helpers.get_dtypes("valid"),
+ available_dtypes=helpers.get_dtypes("integer"),
),
- test_with_out=st.just(True),
)
-def test_torch_tensor_bernoulli(
+def test_torch_bool(
dtype_and_x,
- frontend,
frontend_method_data,
init_flags,
method_flags,
+ frontend,
+ on_device,
backend_fw,
):
input_dtype, x = dtype_and_x
@@ -4524,28 +4526,28 @@ def test_torch_tensor_bernoulli(
init_input_dtypes=input_dtype,
backend_to_test=backend_fw,
init_all_as_kwargs_np={
- "input": x[0],
+ "data": x[0],
},
method_input_dtypes=input_dtype,
- method_all_as_kwargs_np={"generator": x[1], "out": x[2]},
+ method_all_as_kwargs_np={},
frontend_method_data=frontend_method_data,
init_flags=init_flags,
method_flags=method_flags,
frontend=frontend,
+ on_device=on_device,
)
-# bitwise_and
+# byte
@handle_frontend_method(
class_tree=CLASS_TREE,
init_tree="torch.tensor",
- method_name="bitwise_and",
+ method_name="byte",
dtype_and_x=helpers.dtype_and_values(
- available_dtypes=helpers.get_dtypes("integer"),
- num_arrays=2,
+ available_dtypes=helpers.get_dtypes("valid"),
),
)
-def test_torch_tensor_bitwise_and(
+def test_torch_byte(
dtype_and_x,
frontend_method_data,
init_flags,
@@ -4562,9 +4564,7 @@ def test_torch_tensor_bitwise_and(
"data": x[0],
},
method_input_dtypes=input_dtype,
- method_all_as_kwargs_np={
- "other": x[1],
- },
+ method_all_as_kwargs_np={},
frontend_method_data=frontend_method_data,
init_flags=init_flags,
method_flags=method_flags,
@@ -4573,18 +4573,16 @@ def test_torch_tensor_bitwise_and(
)
-# bitwise_and_
+# ceil
@handle_frontend_method(
class_tree=CLASS_TREE,
init_tree="torch.tensor",
- method_name="bitwise_and_",
+ method_name="ceil",
dtype_and_x=helpers.dtype_and_values(
- available_dtypes=helpers.get_dtypes("integer"),
- num_arrays=2,
+ available_dtypes=helpers.get_dtypes("float"),
),
- test_inplace=st.just(True),
)
-def test_torch_tensor_bitwise_and_(
+def test_torch_ceil(
dtype_and_x,
frontend_method_data,
init_flags,
@@ -4601,9 +4599,7 @@ def test_torch_tensor_bitwise_and_(
"data": x[0],
},
method_input_dtypes=input_dtype,
- method_all_as_kwargs_np={
- "other": x[1],
- },
+ method_all_as_kwargs_np={},
frontend_method_data=frontend_method_data,
init_flags=init_flags,
method_flags=method_flags,
@@ -4612,17 +4608,17 @@ def test_torch_tensor_bitwise_and_(
)
-# bitwise_left_shift
+# ceil_
@handle_frontend_method(
class_tree=CLASS_TREE,
init_tree="torch.tensor",
- method_name="bitwise_left_shift",
+ method_name="ceil_",
dtype_and_x=helpers.dtype_and_values(
- available_dtypes=helpers.get_dtypes("integer"),
- num_arrays=2,
+ available_dtypes=helpers.get_dtypes("float"),
),
+ test_inplace=st.just(True),
)
-def test_torch_tensor_bitwise_left_shift(
+def test_torch_ceil_(
dtype_and_x,
frontend_method_data,
init_flags,
@@ -4639,9 +4635,7 @@ def test_torch_tensor_bitwise_left_shift(
"data": x[0],
},
method_input_dtypes=input_dtype,
- method_all_as_kwargs_np={
- "other": x[1],
- },
+ method_all_as_kwargs_np={},
frontend_method_data=frontend_method_data,
init_flags=init_flags,
method_flags=method_flags,
@@ -4650,63 +4644,112 @@ def test_torch_tensor_bitwise_left_shift(
)
-# bitwise_not
+# char
@handle_frontend_method(
class_tree=CLASS_TREE,
init_tree="torch.tensor",
- method_name="bitwise_not",
- dtype_and_x=helpers.dtype_and_values(
- available_dtypes=helpers.get_dtypes("integer"),
- num_arrays=2,
+ method_name="char",
+ dtype_x=helpers.dtype_and_values(
+ available_dtypes=helpers.get_dtypes("valid"),
+ min_value=-128,
+ max_value=127,
),
)
-def test_torch_tensor_bitwise_not(
- dtype_and_x,
+def test_torch_char(
+ dtype_x,
+ frontend,
frontend_method_data,
init_flags,
method_flags,
+ on_device,
+ backend_fw,
+):
+ input_dtype, x = dtype_x
+ helpers.test_frontend_method(
+ init_input_dtypes=input_dtype,
+ backend_to_test=backend_fw,
+ init_all_as_kwargs_np={"data": x[0]},
+ method_input_dtypes=input_dtype,
+ method_all_as_kwargs_np={},
+ frontend_method_data=frontend_method_data,
+ init_flags=init_flags,
+ method_flags=method_flags,
+ frontend=frontend,
+ on_device=on_device,
+ )
+
+
+@handle_frontend_method(
+ class_tree=CLASS_TREE,
+ init_tree="torch.tensor",
+ method_name="cholesky",
+ dtype_and_x=_get_dtype_and_matrix(square=True),
+ upper=st.booleans(),
+)
+def test_torch_cholesky(
+ dtype_and_x,
+ upper,
frontend,
+ frontend_method_data,
+ init_flags,
+ method_flags,
on_device,
backend_fw,
):
input_dtype, x = dtype_and_x
+ x = x[0]
+ # make symmetric positive-definite
+ x = np.matmul(x.swapaxes(-1, -2), x) + np.identity(x.shape[-1]) * 1e-3
+
helpers.test_frontend_method(
init_input_dtypes=input_dtype,
backend_to_test=backend_fw,
init_all_as_kwargs_np={
- "data": x[0],
+ "data": x,
},
method_input_dtypes=input_dtype,
+ method_all_as_kwargs_np={
+ "upper": upper,
+ },
frontend_method_data=frontend_method_data,
init_flags=init_flags,
method_flags=method_flags,
- method_all_as_kwargs_np={},
frontend=frontend,
on_device=on_device,
+ rtol_=1e-2,
)
-# bitwise_not_
+# chunk
+@pytest.mark.skip("Testing takes a lot of time")
@handle_frontend_method(
class_tree=CLASS_TREE,
init_tree="torch.tensor",
- method_name="bitwise_not_",
- dtype_and_x=helpers.dtype_and_values(
- available_dtypes=helpers.get_dtypes("integer"),
- num_arrays=2,
+ method_name="chunk",
+ dtype_x_dim=helpers.dtype_values_axis(
+ available_dtypes=helpers.get_dtypes("float"),
+ min_num_dims=1,
+ min_value=-1e04,
+ max_value=1e04,
+ force_int_axis=True,
+ valid_axis=True,
+ ),
+ chunks=st.integers(
+ min_value=1,
+ max_value=5,
),
- test_inplace=st.just(True),
)
-def test_torch_tensor_bitwise_not_(
- dtype_and_x,
+def test_torch_chunk(
+ dtype_x_dim,
+ chunks,
+ frontend,
frontend_method_data,
init_flags,
method_flags,
- frontend,
on_device,
backend_fw,
):
- input_dtype, x = dtype_and_x
+ input_dtype, x, dim = dtype_x_dim
helpers.test_frontend_method(
init_input_dtypes=input_dtype,
backend_to_test=backend_fw,
@@ -4714,35 +4757,35 @@ def test_torch_tensor_bitwise_not_(
"data": x[0],
},
method_input_dtypes=input_dtype,
+ method_all_as_kwargs_np={
+ "chunks": chunks,
+ "dim": dim,
+ },
frontend_method_data=frontend_method_data,
init_flags=init_flags,
method_flags=method_flags,
- method_all_as_kwargs_np={},
frontend=frontend,
on_device=on_device,
)
-# bitwise_or
+# clamp
@handle_frontend_method(
class_tree=CLASS_TREE,
init_tree="torch.tensor",
- method_name="bitwise_or",
- dtype_and_x=helpers.dtype_and_values(
- available_dtypes=helpers.get_dtypes("valid"),
- num_arrays=2,
- ),
+ method_name="clamp",
+ dtype_and_x_min_max=_get_clamp_inputs(),
)
-def test_torch_tensor_bitwise_or(
- dtype_and_x,
+def test_torch_clamp(
+ dtype_and_x_min_max,
+ frontend,
frontend_method_data,
init_flags,
method_flags,
- frontend,
on_device,
backend_fw,
):
- input_dtype, x = dtype_and_x
+ input_dtype, x, min, max = dtype_and_x_min_max
helpers.test_frontend_method(
init_input_dtypes=input_dtype,
backend_to_test=backend_fw,
@@ -4750,9 +4793,7 @@ def test_torch_tensor_bitwise_or(
"data": x[0],
},
method_input_dtypes=input_dtype,
- method_all_as_kwargs_np={
- "other": x[1],
- },
+ method_all_as_kwargs_np={"min": min, "max": max},
frontend_method_data=frontend_method_data,
init_flags=init_flags,
method_flags=method_flags,
@@ -4761,27 +4802,24 @@ def test_torch_tensor_bitwise_or(
)
-# bitwise_or_
+# clamp_
@handle_frontend_method(
class_tree=CLASS_TREE,
init_tree="torch.tensor",
- method_name="bitwise_or_",
- dtype_and_x=helpers.dtype_and_values(
- available_dtypes=helpers.get_dtypes("valid"),
- num_arrays=2,
- ),
+ method_name="clamp_",
+ dtype_and_x_min_max=_get_clamp_inputs(),
test_inplace=st.just(True),
)
-def test_torch_tensor_bitwise_or_(
- dtype_and_x,
+def test_torch_clamp_(
+ dtype_and_x_min_max,
+ frontend,
frontend_method_data,
init_flags,
method_flags,
- frontend,
on_device,
backend_fw,
):
- input_dtype, x = dtype_and_x
+ input_dtype, x, min, max = dtype_and_x_min_max
helpers.test_frontend_method(
init_input_dtypes=input_dtype,
backend_to_test=backend_fw,
@@ -4789,9 +4827,7 @@ def test_torch_tensor_bitwise_or_(
"data": x[0],
},
method_input_dtypes=input_dtype,
- method_all_as_kwargs_np={
- "other": x[1],
- },
+ method_all_as_kwargs_np={"min": min, "max": max},
frontend_method_data=frontend_method_data,
init_flags=init_flags,
method_flags=method_flags,
@@ -4800,41 +4836,31 @@ def test_torch_tensor_bitwise_or_(
)
-# bitwise right shift
@handle_frontend_method(
class_tree=CLASS_TREE,
init_tree="torch.tensor",
- method_name="bitwise_right_shift",
- dtype_and_x=helpers.dtype_and_values(
- available_dtypes=helpers.get_dtypes("valid"),
- num_arrays=2,
- shared_dtype=True,
- ),
+ method_name="clamp_min",
+ input_and_ranges=_get_clip_min_inputs(),
)
-def test_torch_tensor_bitwise_right_shift(
- dtype_and_x,
+def test_torch_clamp_min(
+ input_and_ranges,
frontend_method_data,
init_flags,
- method_flags,
+ backend_fw,
frontend,
on_device,
- backend_fw,
+ method_flags,
):
- input_dtype, x = dtype_and_x
- # negative shifts will throw an exception
- # shifts >= dtype witdth produce backend-defined behavior
- x[1] = np.asarray(
- np.clip(x[1], 0, np.iinfo(input_dtype[1]).bits - 1), dtype=input_dtype[1]
- )
+ x_dtype, x, min = input_and_ranges
helpers.test_frontend_method(
- init_input_dtypes=input_dtype,
+ init_input_dtypes=x_dtype,
backend_to_test=backend_fw,
init_all_as_kwargs_np={
"data": x[0],
},
- method_input_dtypes=input_dtype,
+ method_input_dtypes=x_dtype,
method_all_as_kwargs_np={
- "other": x[1],
+ "min": min,
},
frontend_method_data=frontend_method_data,
init_flags=init_flags,
@@ -4844,32 +4870,23 @@ def test_torch_tensor_bitwise_right_shift(
)
-# bitwise_right_shift_
+# clip
@handle_frontend_method(
class_tree=CLASS_TREE,
init_tree="torch.tensor",
- method_name="bitwise_right_shift_",
- dtype_and_x=helpers.dtype_and_values(
- available_dtypes=helpers.get_dtypes("valid"),
- num_arrays=2,
- shared_dtype=True,
- ),
+ method_name="clip",
+ input_and_ranges=_get_clamp_inputs(),
)
-def test_torch_tensor_bitwise_right_shift_(
- dtype_and_x,
+def test_torch_clip(
+ input_and_ranges,
+ frontend,
frontend_method_data,
init_flags,
method_flags,
- frontend,
on_device,
backend_fw,
):
- input_dtype, x = dtype_and_x
- # negative shifts will throw an exception
- # shifts >= dtype witdth produce backend-defined behavior
- x[1] = np.asarray(
- np.clip(x[1], 0, np.iinfo(input_dtype[1]).bits - 1), dtype=input_dtype[1]
- )
+ input_dtype, x, min, max = input_and_ranges
helpers.test_frontend_method(
init_input_dtypes=input_dtype,
backend_to_test=backend_fw,
@@ -4877,9 +4894,7 @@ def test_torch_tensor_bitwise_right_shift_(
"data": x[0],
},
method_input_dtypes=input_dtype,
- method_all_as_kwargs_np={
- "other": x[1],
- },
+ method_all_as_kwargs_np={"min": min, "max": max},
frontend_method_data=frontend_method_data,
init_flags=init_flags,
method_flags=method_flags,
@@ -4888,26 +4903,23 @@ def test_torch_tensor_bitwise_right_shift_(
)
-# bitwise_xor
+# clip_
@handle_frontend_method(
class_tree=CLASS_TREE,
init_tree="torch.tensor",
- method_name="bitwise_xor",
- dtype_and_x=helpers.dtype_and_values(
- available_dtypes=st.one_of(st.just(("bool",)), helpers.get_dtypes("integer")),
- num_arrays=2,
- ),
+ method_name="clip_",
+ input_and_ranges=_get_clamp_inputs(),
)
-def test_torch_tensor_bitwise_xor(
- dtype_and_x,
+def test_torch_clip_(
+ input_and_ranges,
+ frontend,
frontend_method_data,
init_flags,
method_flags,
- frontend,
on_device,
backend_fw,
):
- input_dtype, x = dtype_and_x
+ input_dtype, x, min, max = input_and_ranges
helpers.test_frontend_method(
init_input_dtypes=input_dtype,
backend_to_test=backend_fw,
@@ -4915,9 +4927,7 @@ def test_torch_tensor_bitwise_xor(
"data": x[0],
},
method_input_dtypes=input_dtype,
- method_all_as_kwargs_np={
- "other": x[1],
- },
+ method_all_as_kwargs_np={"min": min, "max": max},
frontend_method_data=frontend_method_data,
init_flags=init_flags,
method_flags=method_flags,
@@ -4926,18 +4936,17 @@ def test_torch_tensor_bitwise_xor(
)
-# bitwise_xor_
+# clone
@handle_frontend_method(
class_tree=CLASS_TREE,
init_tree="torch.tensor",
- method_name="bitwise_xor_",
+ method_name="clone",
dtype_and_x=helpers.dtype_and_values(
- available_dtypes=st.one_of(st.just(("bool",)), helpers.get_dtypes("integer")),
- num_arrays=2,
+ available_dtypes=helpers.get_dtypes("valid"),
+ num_arrays=1,
),
- test_inplace=st.just(True),
)
-def test_torch_tensor_bitwise_xor_(
+def test_torch_clone(
dtype_and_x,
frontend_method_data,
init_flags,
@@ -4954,9 +4963,7 @@ def test_torch_tensor_bitwise_xor_(
"data": x[0],
},
method_input_dtypes=input_dtype,
- method_all_as_kwargs_np={
- "other": x[1],
- },
+ method_all_as_kwargs_np={},
frontend_method_data=frontend_method_data,
init_flags=init_flags,
method_flags=method_flags,
@@ -4965,16 +4972,15 @@ def test_torch_tensor_bitwise_xor_(
)
-# bool
@handle_frontend_method(
class_tree=CLASS_TREE,
init_tree="torch.tensor",
- method_name="bool",
+ method_name="conj",
dtype_and_x=helpers.dtype_and_values(
- available_dtypes=helpers.get_dtypes("integer"),
+ available_dtypes=helpers.get_dtypes("float_and_complex")
),
)
-def test_torch_tensor_bool(
+def test_torch_conj(
dtype_and_x,
frontend_method_data,
init_flags,
@@ -5000,16 +5006,17 @@ def test_torch_tensor_bool(
)
-# byte
+# contiguous
@handle_frontend_method(
class_tree=CLASS_TREE,
init_tree="torch.tensor",
- method_name="byte",
+ method_name="contiguous",
dtype_and_x=helpers.dtype_and_values(
- available_dtypes=helpers.get_dtypes("valid"),
+ available_dtypes=helpers.get_dtypes("float"),
+ allow_inf=False,
),
)
-def test_torch_tensor_byte(
+def test_torch_contiguous(
dtype_and_x,
frontend_method_data,
init_flags,
@@ -5035,16 +5042,18 @@ def test_torch_tensor_byte(
)
-# ceil
+# copy_
@handle_frontend_method(
class_tree=CLASS_TREE,
init_tree="torch.tensor",
- method_name="ceil",
+ method_name="copy_",
dtype_and_x=helpers.dtype_and_values(
- available_dtypes=helpers.get_dtypes("float"),
+ available_dtypes=helpers.get_dtypes("valid"),
+ num_arrays=2,
),
+ test_inplace=st.just(True),
)
-def test_torch_tensor_ceil(
+def test_torch_copy_(
dtype_and_x,
frontend_method_data,
init_flags,
@@ -5061,7 +5070,9 @@ def test_torch_tensor_ceil(
"data": x[0],
},
method_input_dtypes=input_dtype,
- method_all_as_kwargs_np={},
+ method_all_as_kwargs_np={
+ "other": x[1],
+ },
frontend_method_data=frontend_method_data,
init_flags=init_flags,
method_flags=method_flags,
@@ -5070,17 +5081,18 @@ def test_torch_tensor_ceil(
)
-# ceil_
+# copysign
@handle_frontend_method(
class_tree=CLASS_TREE,
init_tree="torch.tensor",
- method_name="ceil_",
+ method_name="copysign",
dtype_and_x=helpers.dtype_and_values(
available_dtypes=helpers.get_dtypes("float"),
+ min_num_dims=1,
+ num_arrays=2,
),
- test_inplace=st.just(True),
)
-def test_torch_tensor_ceil_(
+def test_torch_copysign(
dtype_and_x,
frontend_method_data,
init_flags,
@@ -5097,7 +5109,9 @@ def test_torch_tensor_ceil_(
"data": x[0],
},
method_input_dtypes=input_dtype,
- method_all_as_kwargs_np={},
+ method_all_as_kwargs_np={
+ "other": x[1],
+ },
frontend_method_data=frontend_method_data,
init_flags=init_flags,
method_flags=method_flags,
@@ -5106,77 +5120,66 @@ def test_torch_tensor_ceil_(
)
+# copysign_
@handle_frontend_method(
class_tree=CLASS_TREE,
init_tree="torch.tensor",
- method_name="cholesky",
- dtype_and_x=_get_dtype_and_matrix(square=True),
- upper=st.booleans(),
+ method_name="copysign_",
+ dtype_and_x=helpers.dtype_and_values(
+ available_dtypes=helpers.get_dtypes("valid"),
+ min_num_dims=1,
+ num_arrays=2,
+ ),
+ test_inplace=st.just(True),
)
-def test_torch_tensor_cholesky(
+def test_torch_copysign_(
dtype_and_x,
- upper,
- frontend,
frontend_method_data,
init_flags,
method_flags,
+ frontend,
on_device,
backend_fw,
):
input_dtype, x = dtype_and_x
- x = x[0]
- # make symmetric positive-definite
- x = np.matmul(x.swapaxes(-1, -2), x) + np.identity(x.shape[-1]) * 1e-3
-
helpers.test_frontend_method(
init_input_dtypes=input_dtype,
backend_to_test=backend_fw,
init_all_as_kwargs_np={
- "data": x,
+ "data": x[0],
},
method_input_dtypes=input_dtype,
method_all_as_kwargs_np={
- "upper": upper,
+ "other": x[1],
},
frontend_method_data=frontend_method_data,
init_flags=init_flags,
method_flags=method_flags,
frontend=frontend,
on_device=on_device,
- rtol_=1e-2,
)
-# chunk
-@pytest.mark.skip("Testing takes a lot of time")
+# cos
@handle_frontend_method(
class_tree=CLASS_TREE,
init_tree="torch.tensor",
- method_name="chunk",
- dtype_x_dim=helpers.dtype_values_axis(
+ method_name="cos",
+ dtype_and_x=helpers.dtype_and_values(
available_dtypes=helpers.get_dtypes("float"),
- min_num_dims=1,
- min_value=-1e04,
- max_value=1e04,
- force_int_axis=True,
- valid_axis=True,
- ),
- chunks=st.integers(
- min_value=1,
- max_value=5,
+ allow_inf=False,
),
)
-def test_torch_tensor_chunk(
- dtype_x_dim,
- chunks,
- frontend,
+def test_torch_cos(
+ dtype_and_x,
frontend_method_data,
init_flags,
method_flags,
+ frontend,
on_device,
backend_fw,
):
- input_dtype, x, dim = dtype_x_dim
+ input_dtype, x = dtype_and_x
helpers.test_frontend_method(
init_input_dtypes=input_dtype,
backend_to_test=backend_fw,
@@ -5184,10 +5187,7 @@ def test_torch_tensor_chunk(
"data": x[0],
},
method_input_dtypes=input_dtype,
- method_all_as_kwargs_np={
- "chunks": chunks,
- "dim": dim,
- },
+ method_all_as_kwargs_np={},
frontend_method_data=frontend_method_data,
init_flags=init_flags,
method_flags=method_flags,
@@ -5196,31 +5196,35 @@ def test_torch_tensor_chunk(
)
-# clamp
+# cos_
@handle_frontend_method(
class_tree=CLASS_TREE,
init_tree="torch.tensor",
- method_name="clamp",
- dtype_and_x_min_max=_get_clamp_inputs(),
+ method_name="cos_",
+ dtype_and_x=helpers.dtype_and_values(
+ available_dtypes=helpers.get_dtypes("float"),
+ allow_inf=False,
+ ),
+ test_inplace=st.just(True),
)
-def test_torch_tensor_clamp(
- dtype_and_x_min_max,
- frontend,
+def test_torch_cos_(
+ dtype_and_x,
frontend_method_data,
init_flags,
method_flags,
+ frontend,
on_device,
backend_fw,
):
- input_dtype, x, min, max = dtype_and_x_min_max
+ input_dtype, x = dtype_and_x
helpers.test_frontend_method(
init_input_dtypes=input_dtype,
backend_to_test=backend_fw,
init_all_as_kwargs_np={
- "data": x[0],
+ "data": list(x[0]) if isinstance(x[0], int) else x[0],
},
method_input_dtypes=input_dtype,
- method_all_as_kwargs_np={"min": min, "max": max},
+ method_all_as_kwargs_np={},
frontend_method_data=frontend_method_data,
init_flags=init_flags,
method_flags=method_flags,
@@ -5229,24 +5233,26 @@ def test_torch_tensor_clamp(
)
-# clamp_
+# cosh
@handle_frontend_method(
class_tree=CLASS_TREE,
init_tree="torch.tensor",
- method_name="clamp_",
- dtype_and_x_min_max=_get_clamp_inputs(),
- test_inplace=st.just(True),
+ method_name="cosh",
+ dtype_and_x=helpers.dtype_and_values(
+ available_dtypes=helpers.get_dtypes("float"),
+ allow_inf=False,
+ ),
)
-def test_torch_tensor_clamp_(
- dtype_and_x_min_max,
- frontend,
+def test_torch_cosh(
+ dtype_and_x,
frontend_method_data,
init_flags,
method_flags,
+ frontend,
on_device,
backend_fw,
):
- input_dtype, x, min, max = dtype_and_x_min_max
+ input_dtype, x = dtype_and_x
helpers.test_frontend_method(
init_input_dtypes=input_dtype,
backend_to_test=backend_fw,
@@ -5254,7 +5260,7 @@ def test_torch_tensor_clamp_(
"data": x[0],
},
method_input_dtypes=input_dtype,
- method_all_as_kwargs_np={"min": min, "max": max},
+ method_all_as_kwargs_np={},
frontend_method_data=frontend_method_data,
init_flags=init_flags,
method_flags=method_flags,
@@ -5263,57 +5269,71 @@ def test_torch_tensor_clamp_(
)
+# cosh_
@handle_frontend_method(
class_tree=CLASS_TREE,
init_tree="torch.tensor",
- method_name="clamp_min",
- input_and_ranges=_get_clip_min_inputs(),
+ method_name="cosh_",
+ dtype_and_x=helpers.dtype_and_values(
+ available_dtypes=helpers.get_dtypes("float"),
+ allow_inf=False,
+ ),
+ test_inplace=st.just(True),
)
-def test_torch_tensor_clamp_min(
- input_and_ranges,
+def test_torch_cosh_(
+ dtype_and_x,
frontend_method_data,
init_flags,
- backend_fw,
+ method_flags,
frontend,
on_device,
- method_flags,
+ backend_fw,
):
- x_dtype, x, min = input_and_ranges
+ input_dtype, x = dtype_and_x
helpers.test_frontend_method(
- init_input_dtypes=x_dtype,
+ init_input_dtypes=input_dtype,
backend_to_test=backend_fw,
init_all_as_kwargs_np={
"data": x[0],
},
- method_input_dtypes=x_dtype,
- method_all_as_kwargs_np={
- "min": min,
- },
+ method_input_dtypes=input_dtype,
+ method_all_as_kwargs_np={},
frontend_method_data=frontend_method_data,
init_flags=init_flags,
method_flags=method_flags,
frontend=frontend,
on_device=on_device,
+ rtol_=1e-2,
+ atol_=1e-2,
)
-# clip
+# count_nonzero
@handle_frontend_method(
class_tree=CLASS_TREE,
init_tree="torch.tensor",
- method_name="clip",
- input_and_ranges=_get_clamp_inputs(),
+ method_name="count_nonzero",
+ dtype_value=helpers.dtype_and_values(
+ available_dtypes=helpers.get_dtypes("valid"),
+ shape=st.shared(helpers.get_shape(min_num_dims=1), key="shape"),
+ ),
+ dim=helpers.get_axis(
+ shape=st.shared(helpers.get_shape(), key="shape"),
+ allow_neg=True,
+ force_int=True,
+ ),
)
-def test_torch_tensor_clip(
- input_and_ranges,
- frontend,
+def test_torch_count_nonzero(
+ dtype_value,
+ dim,
frontend_method_data,
init_flags,
method_flags,
+ frontend,
on_device,
backend_fw,
):
- input_dtype, x, min, max = input_and_ranges
+ input_dtype, x = dtype_value
helpers.test_frontend_method(
init_input_dtypes=input_dtype,
backend_to_test=backend_fw,
@@ -5321,7 +5341,7 @@ def test_torch_tensor_clip(
"data": x[0],
},
method_input_dtypes=input_dtype,
- method_all_as_kwargs_np={"min": min, "max": max},
+ method_all_as_kwargs_np={"dim": dim},
frontend_method_data=frontend_method_data,
init_flags=init_flags,
method_flags=method_flags,
@@ -5330,51 +5350,76 @@ def test_torch_tensor_clip(
)
-# clip_
+# cov
@handle_frontend_method(
class_tree=CLASS_TREE,
init_tree="torch.tensor",
- method_name="clip_",
- input_and_ranges=_get_clamp_inputs(),
+ method_name="cov",
+ dtype_and_x=_get_dtype_value1_value2_cov(
+ available_dtypes=helpers.get_dtypes("float"),
+ min_num_dims=2,
+ max_num_dims=2,
+ min_dim_size=2,
+ max_dim_size=5,
+ min_value=1,
+ max_value=1e10,
+ abs_smallest_val=0.01,
+ large_abs_safety_factor=2,
+ safety_factor_scale="log",
+ ),
)
-def test_torch_tensor_clip_(
- input_and_ranges,
- frontend,
+def test_torch_cov(
+ dtype_and_x,
frontend_method_data,
init_flags,
method_flags,
+ frontend,
on_device,
backend_fw,
):
- input_dtype, x, min, max = input_and_ranges
+ input_dtype, x, correction, fweights, aweights = dtype_and_x
helpers.test_frontend_method(
- init_input_dtypes=input_dtype,
+ init_input_dtypes=["float64", "int64", "float64"],
backend_to_test=backend_fw,
init_all_as_kwargs_np={
- "data": x[0],
+ "data": x,
+ },
+ method_input_dtypes=["int64", "float64"],
+ method_all_as_kwargs_np={
+ "correction": correction,
+ "fweights": fweights,
+ "aweights": aweights,
},
- method_input_dtypes=input_dtype,
- method_all_as_kwargs_np={"min": min, "max": max},
frontend_method_data=frontend_method_data,
init_flags=init_flags,
method_flags=method_flags,
frontend=frontend,
on_device=on_device,
+ rtol_=1e-2,
+ atol_=1e-2,
)
-# clone
+# cross
@handle_frontend_method(
class_tree=CLASS_TREE,
init_tree="torch.tensor",
- method_name="clone",
- dtype_and_x=helpers.dtype_and_values(
- available_dtypes=helpers.get_dtypes("valid"),
- num_arrays=1,
+ method_name="cross",
+ dtype_input_other_dim=dtype_value1_value2_axis(
+ available_dtypes=helpers.get_dtypes("numeric"),
+ min_num_dims=1,
+ max_num_dims=10,
+ min_dim_size=3,
+ max_dim_size=3,
+ min_value=-1e10,
+ max_value=1e10,
+ abs_smallest_val=0.01,
+ large_abs_safety_factor=2,
+ safety_factor_scale="log",
),
)
-def test_torch_tensor_clone(
- dtype_and_x,
+def test_torch_cross(
+ dtype_input_other_dim,
frontend_method_data,
init_flags,
method_flags,
@@ -5382,33 +5427,65 @@ def test_torch_tensor_clone(
on_device,
backend_fw,
):
- input_dtype, x = dtype_and_x
+ dtype, input, other, dim = dtype_input_other_dim
helpers.test_frontend_method(
- init_input_dtypes=input_dtype,
+ init_input_dtypes=dtype,
backend_to_test=backend_fw,
init_all_as_kwargs_np={
- "data": x[0],
+ "data": input,
+ },
+ method_input_dtypes=dtype,
+ method_all_as_kwargs_np={
+ "other": other,
+ "dim": dim,
},
- method_input_dtypes=input_dtype,
- method_all_as_kwargs_np={},
frontend_method_data=frontend_method_data,
init_flags=init_flags,
method_flags=method_flags,
frontend=frontend,
on_device=on_device,
+ rtol_=1e-2,
+ atol_=1e-2,
)
+@given(
+ dtype_x=helpers.dtype_and_values(
+ available_dtypes=helpers.get_dtypes("valid", prune_function=False),
+ ).filter(
+ lambda x: "bfloat16" not in x[0]
+ and "uint16" not in x[0]
+ and "uint32" not in x[0]
+ and "uint64" not in x[0]
+ ),
+)
+def test_torch_cuda(dtype_x, backend_fw):
+ ivy.set_backend(backend_fw)
+ _, data = dtype_x
+ x = Tensor(data[0], device="gpu:0")
+ device = "gpu:0"
+ ivy.utils.assertions.check_equal(x.cuda, device, as_array=False)
+ ivy.previous_backend()
+
+
+# cummax
@handle_frontend_method(
class_tree=CLASS_TREE,
init_tree="torch.tensor",
- method_name="conj",
- dtype_and_x=helpers.dtype_and_values(
- available_dtypes=helpers.get_dtypes("float_and_complex")
+ method_name="cummax",
+ dtype_value=helpers.dtype_and_values(
+ available_dtypes=helpers.get_dtypes("valid"),
+ shape=st.shared(helpers.get_shape(min_num_dims=1), key="shape"),
+ ),
+ dim=helpers.get_axis(
+ shape=st.shared(helpers.get_shape(), key="shape"),
+ allow_neg=False,
+ force_int=True,
),
)
-def test_torch_tensor_conj(
- dtype_and_x,
+def test_torch_cummax(
+ dtype_value,
+ dim,
frontend_method_data,
init_flags,
method_flags,
@@ -5416,7 +5493,7 @@ def test_torch_tensor_conj(
on_device,
backend_fw,
):
- input_dtype, x = dtype_and_x
+ input_dtype, x = dtype_value
helpers.test_frontend_method(
init_input_dtypes=input_dtype,
backend_to_test=backend_fw,
@@ -5424,7 +5501,7 @@ def test_torch_tensor_conj(
"data": x[0],
},
method_input_dtypes=input_dtype,
- method_all_as_kwargs_np={},
+ method_all_as_kwargs_np={"dim": dim},
frontend_method_data=frontend_method_data,
init_flags=init_flags,
method_flags=method_flags,
@@ -5433,18 +5510,26 @@ def test_torch_tensor_conj(
)
-# contiguous
+# cumprod
@handle_frontend_method(
class_tree=CLASS_TREE,
init_tree="torch.tensor",
- method_name="contiguous",
- dtype_and_x=helpers.dtype_and_values(
- available_dtypes=helpers.get_dtypes("float"),
- allow_inf=False,
+ method_name="cumprod",
+ dtype_value=helpers.dtype_and_values(
+ available_dtypes=helpers.get_dtypes("valid"),
+ shape=st.shared(helpers.get_shape(min_num_dims=1), key="shape"),
+ ),
+ dim=helpers.get_axis(
+ shape=st.shared(helpers.get_shape(), key="shape"),
+ allow_neg=True,
+ force_int=True,
),
+ dtypes=_dtypes(),
)
-def test_torch_tensor_contiguous(
- dtype_and_x,
+def test_torch_cumprod(
+ dtype_value,
+ dim,
+ dtypes,
frontend_method_data,
init_flags,
method_flags,
@@ -5452,15 +5537,18 @@ def test_torch_tensor_contiguous(
on_device,
backend_fw,
):
- input_dtype, x = dtype_and_x
+ input_dtype, x = dtype_value
helpers.test_frontend_method(
init_input_dtypes=input_dtype,
backend_to_test=backend_fw,
init_all_as_kwargs_np={
"data": x[0],
},
- method_input_dtypes=input_dtype,
- method_all_as_kwargs_np={},
+ method_input_dtypes=dtypes,
+ method_all_as_kwargs_np={
+ "dim": dim,
+ "dtype": dtypes[0],
+ },
frontend_method_data=frontend_method_data,
init_flags=init_flags,
method_flags=method_flags,
@@ -5469,19 +5557,26 @@ def test_torch_tensor_contiguous(
)
-# copy_
+# cumsum
@handle_frontend_method(
class_tree=CLASS_TREE,
init_tree="torch.tensor",
- method_name="copy_",
- dtype_and_x=helpers.dtype_and_values(
+ method_name="cumsum",
+ dtype_value=helpers.dtype_and_values(
available_dtypes=helpers.get_dtypes("valid"),
- num_arrays=2,
+ shape=st.shared(helpers.get_shape(min_num_dims=1), key="shape"),
),
- test_inplace=st.just(True),
+ dim=helpers.get_axis(
+ shape=st.shared(helpers.get_shape(), key="shape"),
+ allow_neg=True,
+ force_int=True,
+ ),
+ dtypes=_dtypes(),
)
-def test_torch_tensor_copy_(
- dtype_and_x,
+def test_torch_cumsum(
+ dtype_value,
+ dim,
+ dtypes,
frontend_method_data,
init_flags,
method_flags,
@@ -5489,16 +5584,17 @@ def test_torch_tensor_copy_(
on_device,
backend_fw,
):
- input_dtype, x = dtype_and_x
+ input_dtype, x = dtype_value
helpers.test_frontend_method(
init_input_dtypes=input_dtype,
backend_to_test=backend_fw,
init_all_as_kwargs_np={
"data": x[0],
},
- method_input_dtypes=input_dtype,
+ method_input_dtypes=dtypes,
method_all_as_kwargs_np={
- "other": x[1],
+ "dim": dim,
+ "dtype": dtypes[0],
},
frontend_method_data=frontend_method_data,
init_flags=init_flags,
@@ -5508,19 +5604,25 @@ def test_torch_tensor_copy_(
)
-# copysign
+# cumsum_
@handle_frontend_method(
class_tree=CLASS_TREE,
init_tree="torch.tensor",
- method_name="copysign",
- dtype_and_x=helpers.dtype_and_values(
- available_dtypes=helpers.get_dtypes("float"),
- min_num_dims=1,
- num_arrays=2,
+ method_name="cumsum_",
+ dtype_value=helpers.dtype_and_values(
+ available_dtypes=helpers.get_dtypes("numeric"),
+ shape=st.shared(helpers.get_shape(min_num_dims=1), key="shape"),
+ ),
+ dim=helpers.get_axis(
+ shape=st.shared(helpers.get_shape(), key="shape"),
+ allow_neg=True,
+ force_int=True,
),
+ test_inplace=st.just(True),
)
-def test_torch_tensor_copysign(
- dtype_and_x,
+def test_torch_cumsum_(
+ dtype_value,
+ dim,
frontend_method_data,
init_flags,
method_flags,
@@ -5528,7 +5630,7 @@ def test_torch_tensor_copysign(
on_device,
backend_fw,
):
- input_dtype, x = dtype_and_x
+ input_dtype, x = dtype_value
helpers.test_frontend_method(
init_input_dtypes=input_dtype,
backend_to_test=backend_fw,
@@ -5537,7 +5639,8 @@ def test_torch_tensor_copysign(
},
method_input_dtypes=input_dtype,
method_all_as_kwargs_np={
- "other": x[1],
+ "dim": dim,
+ "dtype": input_dtype[0],
},
frontend_method_data=frontend_method_data,
init_flags=init_flags,
@@ -5546,20 +5649,15 @@ def test_torch_tensor_copysign(
on_device=on_device,
)
-
-# copysign_
-@handle_frontend_method(
- class_tree=CLASS_TREE,
- init_tree="torch.tensor",
- method_name="copysign_",
- dtype_and_x=helpers.dtype_and_values(
- available_dtypes=helpers.get_dtypes("valid"),
- min_num_dims=1,
- num_arrays=2,
- ),
- test_inplace=st.just(True),
+
+# det
+@handle_frontend_method(
+ class_tree=CLASS_TREE,
+ init_tree="torch.tensor",
+ method_name="det",
+ dtype_and_x=_get_dtype_and_matrix(square=True, batch=True),
)
-def test_torch_tensor_copysign_(
+def test_torch_det(
dtype_and_x,
frontend_method_data,
init_flags,
@@ -5573,12 +5671,10 @@ def test_torch_tensor_copysign_(
init_input_dtypes=input_dtype,
backend_to_test=backend_fw,
init_all_as_kwargs_np={
- "data": x[0],
+ "data": x,
},
method_input_dtypes=input_dtype,
- method_all_as_kwargs_np={
- "other": x[1],
- },
+ method_all_as_kwargs_np={},
frontend_method_data=frontend_method_data,
init_flags=init_flags,
method_flags=method_flags,
@@ -5587,17 +5683,16 @@ def test_torch_tensor_copysign_(
)
-# cos
+# detach
@handle_frontend_method(
class_tree=CLASS_TREE,
init_tree="torch.tensor",
- method_name="cos",
+ method_name="detach",
dtype_and_x=helpers.dtype_and_values(
- available_dtypes=helpers.get_dtypes("float"),
- allow_inf=False,
+ available_dtypes=helpers.get_dtypes("valid"),
),
)
-def test_torch_tensor_cos(
+def test_torch_detach(
dtype_and_x,
frontend_method_data,
init_flags,
@@ -5623,18 +5718,17 @@ def test_torch_tensor_cos(
)
-# cos_
+# detach_
@handle_frontend_method(
class_tree=CLASS_TREE,
init_tree="torch.tensor",
- method_name="cos_",
+ method_name="detach_",
dtype_and_x=helpers.dtype_and_values(
- available_dtypes=helpers.get_dtypes("float"),
- allow_inf=False,
+ available_dtypes=helpers.get_dtypes("valid"),
),
test_inplace=st.just(True),
)
-def test_torch_tensor_cos_(
+def test_torch_detach_(
dtype_and_x,
frontend_method_data,
init_flags,
@@ -5648,7 +5742,7 @@ def test_torch_tensor_cos_(
init_input_dtypes=input_dtype,
backend_to_test=backend_fw,
init_all_as_kwargs_np={
- "data": list(x[0]) if isinstance(x[0], int) else x[0],
+ "data": x[0],
},
method_input_dtypes=input_dtype,
method_all_as_kwargs_np={},
@@ -5660,18 +5754,38 @@ def test_torch_tensor_cos_(
)
-# cosh
+@given(
+ dtype_x=helpers.dtype_and_values(
+ available_dtypes=helpers.get_dtypes("valid", prune_function=False)
+ ).filter(lambda x: "bfloat16" not in x[0]),
+)
+def test_torch_device(
+ dtype_x,
+ backend_fw,
+):
+ ivy.set_backend(backend_fw)
+ _, data = dtype_x
+ x = Tensor(data[0])
+ x.ivy_array = data[0]
+ ivy.utils.assertions.check_equal(
+ x.device, ivy.dev(ivy.array(data[0])), as_array=False
+ )
+ ivy.previous_backend()
+
+
@handle_frontend_method(
class_tree=CLASS_TREE,
init_tree="torch.tensor",
- method_name="cosh",
- dtype_and_x=helpers.dtype_and_values(
+ method_name="diag",
+ dtype_and_values=helpers.dtype_and_values(
available_dtypes=helpers.get_dtypes("float"),
- allow_inf=False,
+ shape=st.shared(helpers.get_shape(min_num_dims=1, max_num_dims=2), key="shape"),
),
+ diagonal=st.integers(min_value=-100, max_value=100),
)
-def test_torch_tensor_cosh(
- dtype_and_x,
+def test_torch_diag(
+ dtype_and_values,
+ diagonal,
frontend_method_data,
init_flags,
method_flags,
@@ -5679,15 +5793,17 @@ def test_torch_tensor_cosh(
on_device,
backend_fw,
):
- input_dtype, x = dtype_and_x
+ input_dtype, values = dtype_and_values
helpers.test_frontend_method(
init_input_dtypes=input_dtype,
backend_to_test=backend_fw,
init_all_as_kwargs_np={
- "data": x[0],
+ "data": values[0],
},
method_input_dtypes=input_dtype,
- method_all_as_kwargs_np={},
+ method_all_as_kwargs_np={
+ "diagonal": diagonal,
+ },
frontend_method_data=frontend_method_data,
init_flags=init_flags,
method_flags=method_flags,
@@ -5696,63 +5812,85 @@ def test_torch_tensor_cosh(
)
-# cosh_
@handle_frontend_method(
class_tree=CLASS_TREE,
init_tree="torch.tensor",
- method_name="cosh_",
- dtype_and_x=helpers.dtype_and_values(
- available_dtypes=helpers.get_dtypes("float"),
- allow_inf=False,
+ method_name="diagonal",
+ dtype_and_values=helpers.dtype_and_values(
+ available_dtypes=helpers.get_dtypes("valid"),
+ shape=st.shared(helpers.get_shape(min_num_dims=2), key="shape"),
+ ),
+ dims_and_offset=dims_and_offset(
+ shape=st.shared(helpers.get_shape(min_num_dims=2), key="shape")
),
- test_inplace=st.just(True),
)
-def test_torch_tensor_cosh_(
- dtype_and_x,
+def test_torch_diagonal(
+ dtype_and_values,
+ dims_and_offset,
+ frontend,
frontend_method_data,
+ backend_fw,
init_flags,
method_flags,
- frontend,
on_device,
- backend_fw,
):
- input_dtype, x = dtype_and_x
+ input_dtype, value = dtype_and_values
+ dim1, dim2, offset = dims_and_offset
+ input = value[0]
+ num_dims = len(np.shape(input))
+ assume(dim1 != dim2)
+ if dim1 < 0:
+ assume(dim1 + num_dims != dim2)
+ if dim2 < 0:
+ assume(dim1 != dim2 + num_dims)
helpers.test_frontend_method(
- init_input_dtypes=input_dtype,
- backend_to_test=backend_fw,
- init_all_as_kwargs_np={
- "data": x[0],
+ init_input_dtypes=[input_dtype[0]],
+ init_all_as_kwargs_np={"data": input},
+ method_input_dtypes=[input_dtype[0]],
+ method_all_as_kwargs_np={
+ "offset": offset,
+ "dim1": dim1,
+ "dim2": dim2,
},
- method_input_dtypes=input_dtype,
- method_all_as_kwargs_np={},
+ frontend=frontend,
frontend_method_data=frontend_method_data,
+ backend_to_test=backend_fw,
init_flags=init_flags,
method_flags=method_flags,
- frontend=frontend,
on_device=on_device,
- rtol_=1e-2,
- atol_=1e-2,
)
-# count_nonzero
+# diff
@handle_frontend_method(
class_tree=CLASS_TREE,
init_tree="torch.tensor",
- method_name="count_nonzero",
- dtype_value=helpers.dtype_and_values(
- available_dtypes=helpers.get_dtypes("valid"),
- shape=st.shared(helpers.get_shape(min_num_dims=1), key="shape"),
+ method_name="diff",
+ dtype_n_x_n_axis=helpers.dtype_values_axis(
+ available_dtypes=st.shared(helpers.get_dtypes("valid"), key="dtype"),
+ min_num_dims=1,
+ min_value=-1e09,
+ max_value=1e09,
+ valid_axis=True,
+ force_int_axis=True,
),
- dim=helpers.get_axis(
- shape=st.shared(helpers.get_shape(), key="shape"),
- allow_neg=True,
- force_int=True,
+ n=st.integers(min_value=0, max_value=5),
+ dtype_prepend=helpers.dtype_and_values(
+ available_dtypes=st.shared(helpers.get_dtypes("numeric"), key="dtype"),
+ min_num_dims=1,
+ max_num_dims=1,
+ ),
+ dtype_append=helpers.dtype_and_values(
+ available_dtypes=st.shared(helpers.get_dtypes("numeric"), key="dtype"),
+ min_num_dims=1,
+ max_num_dims=1,
),
)
-def test_torch_tensor_count_nonzero(
- dtype_value,
- dim,
+def test_torch_diff(
+ dtype_n_x_n_axis,
+ n,
+ dtype_prepend,
+ dtype_append,
frontend_method_data,
init_flags,
method_flags,
@@ -5760,7 +5898,9 @@ def test_torch_tensor_count_nonzero(
on_device,
backend_fw,
):
- input_dtype, x = dtype_value
+ input_dtype, x, axis = dtype_n_x_n_axis
+ _, prepend = dtype_prepend
+ _, append = dtype_append
helpers.test_frontend_method(
init_input_dtypes=input_dtype,
backend_to_test=backend_fw,
@@ -5768,7 +5908,12 @@ def test_torch_tensor_count_nonzero(
"data": x[0],
},
method_input_dtypes=input_dtype,
- method_all_as_kwargs_np={"dim": dim},
+ method_all_as_kwargs_np={
+ "n": n,
+ "dim": axis,
+ "prepend": prepend[0],
+ "append": append[0],
+ },
frontend_method_data=frontend_method_data,
init_flags=init_flags,
method_flags=method_flags,
@@ -5777,26 +5922,17 @@ def test_torch_tensor_count_nonzero(
)
-# cross
+# dim
@handle_frontend_method(
class_tree=CLASS_TREE,
init_tree="torch.tensor",
- method_name="cross",
- dtype_input_other_dim=dtype_value1_value2_axis(
+ method_name="dim",
+ dtype_and_x=helpers.dtype_and_values(
available_dtypes=helpers.get_dtypes("numeric"),
- min_num_dims=1,
- max_num_dims=10,
- min_dim_size=3,
- max_dim_size=3,
- min_value=-1e10,
- max_value=1e10,
- abs_smallest_val=0.01,
- large_abs_safety_factor=2,
- safety_factor_scale="log",
),
)
-def test_torch_tensor_cross(
- dtype_input_other_dim,
+def test_torch_dim(
+ dtype_and_x,
frontend_method_data,
init_flags,
method_flags,
@@ -5804,62 +5940,59 @@ def test_torch_tensor_cross(
on_device,
backend_fw,
):
- dtype, input, other, dim = dtype_input_other_dim
+ input_dtype, x = dtype_and_x
helpers.test_frontend_method(
- init_input_dtypes=dtype,
+ init_input_dtypes=input_dtype,
backend_to_test=backend_fw,
init_all_as_kwargs_np={
- "data": input,
- },
- method_input_dtypes=dtype,
- method_all_as_kwargs_np={
- "other": other,
- "dim": dim,
+ "data": x[0],
},
+ method_input_dtypes=[],
+ method_all_as_kwargs_np={},
frontend_method_data=frontend_method_data,
init_flags=init_flags,
method_flags=method_flags,
frontend=frontend,
on_device=on_device,
- rtol_=1e-2,
- atol_=1e-2,
)
-# cummax
+# div
@handle_frontend_method(
class_tree=CLASS_TREE,
init_tree="torch.tensor",
- method_name="cummax",
- dtype_value=helpers.dtype_and_values(
- available_dtypes=helpers.get_dtypes("valid"),
- shape=st.shared(helpers.get_shape(min_num_dims=1), key="shape"),
- ),
- dim=helpers.get_axis(
- shape=st.shared(helpers.get_shape(), key="shape"),
- allow_neg=False,
- force_int=True,
+ method_name="div",
+ dtype_and_x=helpers.dtype_and_values(
+ available_dtypes=helpers.get_dtypes("numeric"),
+ num_arrays=2,
+ large_abs_safety_factor=2.5,
+ small_abs_safety_factor=2.5,
+ safety_factor_scale="log",
),
+ rounding_mode=st.sampled_from(["floor", "trunc"]) | st.none(),
)
-def test_torch_tensor_cummax(
- dtype_value,
- dim,
+def test_torch_div(
+ dtype_and_x,
+ rounding_mode,
+ frontend,
frontend_method_data,
init_flags,
method_flags,
- frontend,
on_device,
backend_fw,
):
- input_dtype, x = dtype_value
+ input_dtype, x = dtype_and_x
+ assume(not np.any(np.isclose(x[1], 0)))
+
helpers.test_frontend_method(
init_input_dtypes=input_dtype,
backend_to_test=backend_fw,
- init_all_as_kwargs_np={
- "data": x[0],
- },
+ init_all_as_kwargs_np={"data": x[0]},
method_input_dtypes=input_dtype,
- method_all_as_kwargs_np={"dim": dim},
+ method_all_as_kwargs_np={
+ "other": x[1],
+ "rounding_mode": rounding_mode,
+ },
frontend_method_data=frontend_method_data,
init_flags=init_flags,
method_flags=method_flags,
@@ -5868,44 +6001,42 @@ def test_torch_tensor_cummax(
)
-# cumprod
+# div_
@handle_frontend_method(
class_tree=CLASS_TREE,
init_tree="torch.tensor",
- method_name="cumprod",
- dtype_value=helpers.dtype_and_values(
- available_dtypes=helpers.get_dtypes("valid"),
- shape=st.shared(helpers.get_shape(min_num_dims=1), key="shape"),
- ),
- dim=helpers.get_axis(
- shape=st.shared(helpers.get_shape(), key="shape"),
- allow_neg=True,
- force_int=True,
+ method_name="div_",
+ dtype_and_x=helpers.dtype_and_values(
+ available_dtypes=helpers.get_dtypes("numeric"),
+ num_arrays=2,
+ large_abs_safety_factor=2.5,
+ small_abs_safety_factor=2.5,
+ safety_factor_scale="log",
),
- dtypes=_dtypes(),
+ rounding_mode=st.sampled_from(["floor", "trunc"]) | st.none(),
+ test_inplace=st.just(True),
)
-def test_torch_tensor_cumprod(
- dtype_value,
- dim,
- dtypes,
+def test_torch_div_(
+ dtype_and_x,
+ rounding_mode,
+ frontend,
frontend_method_data,
init_flags,
method_flags,
- frontend,
on_device,
backend_fw,
):
- input_dtype, x = dtype_value
+ input_dtype, x = dtype_and_x
+ assume(not np.any(np.isclose(x[1], 0)))
+
helpers.test_frontend_method(
init_input_dtypes=input_dtype,
backend_to_test=backend_fw,
- init_all_as_kwargs_np={
- "data": x[0],
- },
- method_input_dtypes=dtypes,
+ init_all_as_kwargs_np={"data": x[0]},
+ method_input_dtypes=input_dtype,
method_all_as_kwargs_np={
- "dim": dim,
- "dtype": dtypes[0],
+ "other": x[1],
+ "rounding_mode": rounding_mode,
},
frontend_method_data=frontend_method_data,
init_flags=init_flags,
@@ -5915,72 +6046,60 @@ def test_torch_tensor_cumprod(
)
-# cumsum
+# divide
@handle_frontend_method(
class_tree=CLASS_TREE,
init_tree="torch.tensor",
- method_name="cumsum",
- dtype_value=helpers.dtype_and_values(
+ method_name="divide",
+ dtype_and_x=helpers.dtype_and_values(
available_dtypes=helpers.get_dtypes("valid"),
- shape=st.shared(helpers.get_shape(min_num_dims=1), key="shape"),
- ),
- dim=helpers.get_axis(
- shape=st.shared(helpers.get_shape(), key="shape"),
- allow_neg=True,
- force_int=True,
+ num_arrays=2,
+ min_value=-1e04,
+ max_value=1e04,
+ allow_inf=False,
),
- dtypes=_dtypes(),
)
-def test_torch_tensor_cumsum(
- dtype_value,
- dim,
- dtypes,
+def test_torch_divide(
+ dtype_and_x,
+ frontend,
frontend_method_data,
init_flags,
method_flags,
- frontend,
on_device,
backend_fw,
):
- input_dtype, x = dtype_value
+ input_dtype, x = dtype_and_x
helpers.test_frontend_method(
init_input_dtypes=input_dtype,
backend_to_test=backend_fw,
init_all_as_kwargs_np={
"data": x[0],
},
- method_input_dtypes=dtypes,
+ method_input_dtypes=input_dtype,
method_all_as_kwargs_np={
- "dim": dim,
- "dtype": dtypes[0],
+ "other": x[1],
},
frontend_method_data=frontend_method_data,
init_flags=init_flags,
method_flags=method_flags,
frontend=frontend,
+ atol_=1e-02,
on_device=on_device,
)
-# cumsum_
@handle_frontend_method(
class_tree=CLASS_TREE,
init_tree="torch.tensor",
- method_name="cumsum_",
- dtype_value=helpers.dtype_and_values(
- available_dtypes=helpers.get_dtypes("numeric"),
- shape=st.shared(helpers.get_shape(min_num_dims=1), key="shape"),
- ),
- dim=helpers.get_axis(
- shape=st.shared(helpers.get_shape(), key="shape"),
- allow_neg=True,
- force_int=True,
+ method_name="dot",
+ dtype_and_x=helpers.dtype_and_values(
+ available_dtypes=helpers.get_dtypes("float"),
+ num_arrays=2,
+ shape=(1,),
),
- test_inplace=st.just(True),
)
-def test_torch_tensor_cumsum_(
- dtype_value,
- dim,
+def test_torch_dot(
+ dtype_and_x,
frontend_method_data,
init_flags,
method_flags,
@@ -5988,7 +6107,7 @@ def test_torch_tensor_cumsum_(
on_device,
backend_fw,
):
- input_dtype, x = dtype_value
+ input_dtype, x = dtype_and_x
helpers.test_frontend_method(
init_input_dtypes=input_dtype,
backend_to_test=backend_fw,
@@ -5997,8 +6116,7 @@ def test_torch_tensor_cumsum_(
},
method_input_dtypes=input_dtype,
method_all_as_kwargs_np={
- "dim": dim,
- "dtype": input_dtype[0],
+ "tensor": x[1],
},
frontend_method_data=frontend_method_data,
init_flags=init_flags,
@@ -6008,28 +6126,28 @@ def test_torch_tensor_cumsum_(
)
-# det
@handle_frontend_method(
class_tree=CLASS_TREE,
init_tree="torch.tensor",
- method_name="det",
- dtype_and_x=_get_dtype_and_matrix(square=True, batch=True),
+ method_name="double",
+ dtype_and_x=helpers.dtype_and_values(
+ available_dtypes=helpers.get_dtypes("valid"),
+ ),
)
-def test_torch_tensor_det(
+def test_torch_double(
dtype_and_x,
frontend_method_data,
init_flags,
method_flags,
frontend,
- on_device,
backend_fw,
+ on_device,
):
input_dtype, x = dtype_and_x
helpers.test_frontend_method(
init_input_dtypes=input_dtype,
- backend_to_test=backend_fw,
init_all_as_kwargs_np={
- "data": x,
+ "data": x[0],
},
method_input_dtypes=input_dtype,
method_all_as_kwargs_np={},
@@ -6037,21 +6155,31 @@ def test_torch_tensor_det(
init_flags=init_flags,
method_flags=method_flags,
frontend=frontend,
+ backend_to_test=backend_fw,
on_device=on_device,
)
-# detach
+# dsplit
@handle_frontend_method(
class_tree=CLASS_TREE,
init_tree="torch.tensor",
- method_name="detach",
- dtype_and_x=helpers.dtype_and_values(
+ method_name="dsplit",
+ dtype_value=helpers.dtype_and_values(
available_dtypes=helpers.get_dtypes("valid"),
+ shape=st.shared(helpers.get_shape(min_num_dims=3), key="value_shape"),
+ ),
+ indices_or_sections=_get_splits(
+ min_num_dims=3,
+ axis=2,
+ allow_none=False,
+ allow_array_indices=False,
+ is_mod_split=True,
),
)
-def test_torch_tensor_detach(
- dtype_and_x,
+def test_torch_dsplit(
+ dtype_value,
+ indices_or_sections,
frontend_method_data,
init_flags,
method_flags,
@@ -6059,15 +6187,15 @@ def test_torch_tensor_detach(
on_device,
backend_fw,
):
- input_dtype, x = dtype_and_x
+ input_dtype, x = dtype_value
helpers.test_frontend_method(
init_input_dtypes=input_dtype,
backend_to_test=backend_fw,
init_all_as_kwargs_np={
"data": x[0],
},
- method_input_dtypes=input_dtype,
- method_all_as_kwargs_np={},
+ method_input_dtypes=[],
+ method_all_as_kwargs_np={"indices_or_sections": indices_or_sections},
frontend_method_data=frontend_method_data,
init_flags=init_flags,
method_flags=method_flags,
@@ -6076,17 +6204,34 @@ def test_torch_tensor_detach(
)
-# detach_
+@given(
+ dtype_x=helpers.dtype_and_values(
+ available_dtypes=helpers.get_dtypes("valid", prune_function=False)
+ ).filter(lambda x: "bfloat16" not in x[0]),
+)
+def test_torch_dtype(dtype_x, backend_fw):
+ ivy.set_backend(backend_fw)
+ dtype, data = dtype_x
+ x = Tensor(data[0])
+ x.ivy_array = data[0]
+ ivy.utils.assertions.check_equal(x.dtype, dtype[0], as_array=False)
+ ivy.previous_backend()
+
+
@handle_frontend_method(
class_tree=CLASS_TREE,
init_tree="torch.tensor",
- method_name="detach_",
+ method_name="eq_",
dtype_and_x=helpers.dtype_and_values(
- available_dtypes=helpers.get_dtypes("valid"),
+ available_dtypes=helpers.get_dtypes("float"),
+ num_arrays=2,
+ min_value=-1e04,
+ max_value=1e04,
+ allow_inf=False,
),
test_inplace=st.just(True),
)
-def test_torch_tensor_detach_(
+def test_torch_eq_(
dtype_and_x,
frontend_method_data,
init_flags,
@@ -6103,7 +6248,9 @@ def test_torch_tensor_detach_(
"data": x[0],
},
method_input_dtypes=input_dtype,
- method_all_as_kwargs_np={},
+ method_all_as_kwargs_np={
+ "other": x[1],
+ },
frontend_method_data=frontend_method_data,
init_flags=init_flags,
method_flags=method_flags,
@@ -6112,153 +6259,105 @@ def test_torch_tensor_detach_(
)
-@given(
- dtype_x=helpers.dtype_and_values(
- available_dtypes=helpers.get_dtypes("valid", prune_function=False)
- ).filter(lambda x: "bfloat16" not in x[0]),
-)
-def test_torch_tensor_device(
- dtype_x,
- backend_fw,
-):
- ivy.set_backend(backend_fw)
- _, data = dtype_x
- x = Tensor(data[0])
- x.ivy_array = data[0]
- ivy.utils.assertions.check_equal(
- x.device, ivy.dev(ivy.array(data[0])), as_array=False
- )
- ivy.previous_backend()
-
-
+# equal
@handle_frontend_method(
class_tree=CLASS_TREE,
init_tree="torch.tensor",
- method_name="diag",
- dtype_and_values=helpers.dtype_and_values(
- available_dtypes=helpers.get_dtypes("float"),
- shape=st.shared(helpers.get_shape(min_num_dims=1, max_num_dims=2), key="shape"),
+ method_name="equal",
+ dtype_and_x=helpers.dtype_and_values(
+ available_dtypes=helpers.get_dtypes("numeric"),
+ num_arrays=2,
+ shared_dtype=True,
+ min_num_dims=1,
+ min_value=-1e04,
+ max_value=1e04,
),
- diagonal=st.integers(min_value=-100, max_value=100),
)
-def test_torch_tensor_diag(
- dtype_and_values,
- diagonal,
+def test_torch_equal(
+ dtype_and_x,
+ frontend,
frontend_method_data,
init_flags,
method_flags,
- frontend,
on_device,
backend_fw,
):
- input_dtype, values = dtype_and_values
+ input_dtype, x = dtype_and_x
helpers.test_frontend_method(
init_input_dtypes=input_dtype,
backend_to_test=backend_fw,
init_all_as_kwargs_np={
- "data": values[0],
+ "data": x[0],
},
method_input_dtypes=input_dtype,
method_all_as_kwargs_np={
- "diagonal": diagonal,
+ "other": x[1],
},
frontend_method_data=frontend_method_data,
init_flags=init_flags,
method_flags=method_flags,
frontend=frontend,
+ atol_=1e-04,
+ rtol_=1e-04,
on_device=on_device,
)
+# erf
@handle_frontend_method(
class_tree=CLASS_TREE,
init_tree="torch.tensor",
- method_name="diagonal",
- dtype_and_values=helpers.dtype_and_values(
- available_dtypes=helpers.get_dtypes("valid"),
- shape=st.shared(helpers.get_shape(min_num_dims=2), key="shape"),
- ),
- dims_and_offset=dims_and_offset(
- shape=st.shared(helpers.get_shape(min_num_dims=2), key="shape")
+ method_name="erf",
+ dtype_and_x=helpers.dtype_and_values(
+ available_dtypes=helpers.get_dtypes("float"),
),
)
-def test_torch_tensor_diagonal(
- dtype_and_values,
- dims_and_offset,
- frontend,
+def test_torch_erf(
+ dtype_and_x,
frontend_method_data,
- backend_fw,
init_flags,
method_flags,
+ frontend,
on_device,
+ backend_fw,
):
- input_dtype, value = dtype_and_values
- dim1, dim2, offset = dims_and_offset
- input = value[0]
- num_dims = len(np.shape(input))
- assume(dim1 != dim2)
- if dim1 < 0:
- assume(dim1 + num_dims != dim2)
- if dim2 < 0:
- assume(dim1 != dim2 + num_dims)
+ input_dtype, x = dtype_and_x
helpers.test_frontend_method(
- init_input_dtypes=[input_dtype[0]],
- init_all_as_kwargs_np={"data": input},
- method_input_dtypes=[input_dtype[0]],
- method_all_as_kwargs_np={
- "offset": offset,
- "dim1": dim1,
- "dim2": dim2,
+ init_input_dtypes=input_dtype,
+ backend_to_test=backend_fw,
+ init_all_as_kwargs_np={
+ "data": x[0],
},
- frontend=frontend,
+ method_input_dtypes=input_dtype,
+ method_all_as_kwargs_np={},
frontend_method_data=frontend_method_data,
- backend_to_test=backend_fw,
init_flags=init_flags,
method_flags=method_flags,
+ frontend=frontend,
on_device=on_device,
)
-# diff
+# erf_
@handle_frontend_method(
class_tree=CLASS_TREE,
init_tree="torch.tensor",
- method_name="diff",
- dtype_n_x_n_axis=helpers.dtype_values_axis(
- available_dtypes=st.shared(helpers.get_dtypes("valid"), key="dtype"),
- min_num_dims=1,
- min_value=-1e09,
- max_value=1e09,
- valid_axis=True,
- force_int_axis=True,
- ),
- n=st.integers(min_value=0, max_value=5),
- dtype_prepend=helpers.dtype_and_values(
- available_dtypes=st.shared(helpers.get_dtypes("numeric"), key="dtype"),
- min_num_dims=1,
- max_num_dims=1,
- ),
- dtype_append=helpers.dtype_and_values(
- available_dtypes=st.shared(helpers.get_dtypes("numeric"), key="dtype"),
- min_num_dims=1,
- max_num_dims=1,
+ method_name="erf_",
+ dtype_and_x=helpers.dtype_and_values(
+ available_dtypes=helpers.get_dtypes("valid"),
),
+ test_inplace=st.just(True),
)
-def test_torch_tensor_diff(
- dtype_n_x_n_axis,
- n,
- dtype_prepend,
- dtype_append,
+def test_torch_erf_(
+ dtype_and_x,
frontend_method_data,
init_flags,
method_flags,
frontend,
on_device,
- backend_fw,
-):
- input_dtype, x, axis = dtype_n_x_n_axis
- _, prepend = dtype_prepend
- _, append = dtype_append
+ backend_fw,
+):
+ input_dtype, x = dtype_and_x
helpers.test_frontend_method(
init_input_dtypes=input_dtype,
backend_to_test=backend_fw,
@@ -6266,12 +6365,7 @@ def test_torch_tensor_diff(
"data": x[0],
},
method_input_dtypes=input_dtype,
- method_all_as_kwargs_np={
- "n": n,
- "dim": axis,
- "prepend": prepend[0],
- "append": append[0],
- },
+ method_all_as_kwargs_np={},
frontend_method_data=frontend_method_data,
init_flags=init_flags,
method_flags=method_flags,
@@ -6280,16 +6374,16 @@ def test_torch_tensor_diff(
)
-# dim
+# exp
@handle_frontend_method(
class_tree=CLASS_TREE,
init_tree="torch.tensor",
- method_name="dim",
+ method_name="exp",
dtype_and_x=helpers.dtype_and_values(
available_dtypes=helpers.get_dtypes("numeric"),
),
)
-def test_torch_tensor_dim(
+def test_torch_exp(
dtype_and_x,
frontend_method_data,
init_flags,
@@ -6305,7 +6399,7 @@ def test_torch_tensor_dim(
init_all_as_kwargs_np={
"data": x[0],
},
- method_input_dtypes=[],
+ method_input_dtypes=input_dtype,
method_all_as_kwargs_np={},
frontend_method_data=frontend_method_data,
init_flags=init_flags,
@@ -6315,42 +6409,34 @@ def test_torch_tensor_dim(
)
-# div
+# exp_
@handle_frontend_method(
class_tree=CLASS_TREE,
init_tree="torch.tensor",
- method_name="div",
+ method_name="exp_",
dtype_and_x=helpers.dtype_and_values(
available_dtypes=helpers.get_dtypes("numeric"),
- num_arrays=2,
- large_abs_safety_factor=2.5,
- small_abs_safety_factor=2.5,
- safety_factor_scale="log",
),
- rounding_mode=st.sampled_from(["floor", "trunc"]) | st.none(),
+ test_inplace=st.just(True),
)
-def test_torch_tensor_div(
+def test_torch_exp_(
dtype_and_x,
- rounding_mode,
- frontend,
frontend_method_data,
init_flags,
method_flags,
+ frontend,
on_device,
backend_fw,
):
input_dtype, x = dtype_and_x
- assume(not np.any(np.isclose(x[1], 0)))
-
helpers.test_frontend_method(
init_input_dtypes=input_dtype,
backend_to_test=backend_fw,
- init_all_as_kwargs_np={"data": x[0]},
- method_input_dtypes=input_dtype,
- method_all_as_kwargs_np={
- "other": x[1],
- "rounding_mode": rounding_mode,
+ init_all_as_kwargs_np={
+ "data": x[0],
},
+ method_input_dtypes=input_dtype,
+ method_all_as_kwargs_np={},
frontend_method_data=frontend_method_data,
init_flags=init_flags,
method_flags=method_flags,
@@ -6359,43 +6445,43 @@ def test_torch_tensor_div(
)
-# div_
@handle_frontend_method(
class_tree=CLASS_TREE,
init_tree="torch.tensor",
- method_name="div_",
- dtype_and_x=helpers.dtype_and_values(
- available_dtypes=helpers.get_dtypes("numeric"),
- num_arrays=2,
- large_abs_safety_factor=2.5,
- small_abs_safety_factor=2.5,
- safety_factor_scale="log",
- ),
- rounding_mode=st.sampled_from(["floor", "trunc"]) | st.none(),
- test_inplace=st.just(True),
+ method_name="expand",
+ dtype_x_shape=_expand_helper(),
+ unpack_shape=st.booleans(),
)
-def test_torch_tensor_div_(
- dtype_and_x,
- rounding_mode,
- frontend,
+def test_torch_expand(
+ dtype_x_shape,
+ unpack_shape,
frontend_method_data,
init_flags,
method_flags,
+ frontend,
on_device,
backend_fw,
):
- input_dtype, x = dtype_and_x
- assume(not np.any(np.isclose(x[1], 0)))
-
+ input_dtype, x, shape = dtype_x_shape
+ if unpack_shape:
+ method_flags.num_positional_args = len(shape) + 1
+ size = {}
+ i = 0
+ for x_ in shape:
+ size[f"x{i}"] = x_
+ i += 1
+ else:
+ size = {
+ "size": shape,
+ }
helpers.test_frontend_method(
init_input_dtypes=input_dtype,
backend_to_test=backend_fw,
- init_all_as_kwargs_np={"data": x[0]},
- method_input_dtypes=input_dtype,
- method_all_as_kwargs_np={
- "other": x[1],
- "rounding_mode": rounding_mode,
+ init_all_as_kwargs_np={
+ "data": x[0],
},
+ method_input_dtypes=input_dtype,
+ method_all_as_kwargs_np=size,
frontend_method_data=frontend_method_data,
init_flags=init_flags,
method_flags=method_flags,
@@ -6404,29 +6490,25 @@ def test_torch_tensor_div_(
)
-# divide
+# expand_as
@handle_frontend_method(
class_tree=CLASS_TREE,
init_tree="torch.tensor",
- method_name="divide",
- dtype_and_x=helpers.dtype_and_values(
- available_dtypes=helpers.get_dtypes("valid"),
- num_arrays=2,
- min_value=-1e04,
- max_value=1e04,
- allow_inf=False,
+ method_name="expand_as",
+ dtype_x=helpers.dtype_and_values(
+ available_dtypes=helpers.get_dtypes("valid"), num_arrays=2
),
)
-def test_torch_tensor_divide(
- dtype_and_x,
- frontend,
+def test_torch_expand_as(
+ dtype_x,
frontend_method_data,
init_flags,
method_flags,
+ frontend,
on_device,
backend_fw,
):
- input_dtype, x = dtype_and_x
+ input_dtype, x = dtype_x
helpers.test_frontend_method(
init_input_dtypes=input_dtype,
backend_to_test=backend_fw,
@@ -6441,22 +6523,20 @@ def test_torch_tensor_divide(
init_flags=init_flags,
method_flags=method_flags,
frontend=frontend,
- atol_=1e-02,
on_device=on_device,
)
+# expm1
@handle_frontend_method(
class_tree=CLASS_TREE,
init_tree="torch.tensor",
- method_name="dot",
+ method_name="expm1",
dtype_and_x=helpers.dtype_and_values(
- available_dtypes=helpers.get_dtypes("float"),
- num_arrays=2,
- shape=(1,),
+ available_dtypes=helpers.get_dtypes("numeric"),
),
)
-def test_torch_tensor_dot(
+def test_torch_expm1(
dtype_and_x,
frontend_method_data,
init_flags,
@@ -6473,9 +6553,7 @@ def test_torch_tensor_dot(
"data": x[0],
},
method_input_dtypes=input_dtype,
- method_all_as_kwargs_np={
- "tensor": x[1],
- },
+ method_all_as_kwargs_np={},
frontend_method_data=frontend_method_data,
init_flags=init_flags,
method_flags=method_flags,
@@ -6484,26 +6562,29 @@ def test_torch_tensor_dot(
)
+# expm1_
@handle_frontend_method(
class_tree=CLASS_TREE,
init_tree="torch.tensor",
- method_name="double",
+ method_name="expm1_",
dtype_and_x=helpers.dtype_and_values(
- available_dtypes=helpers.get_dtypes("valid"),
+ available_dtypes=helpers.get_dtypes("float"),
),
+ test_inplace=st.just(True),
)
-def test_torch_tensor_double(
+def test_torch_expm1_(
dtype_and_x,
frontend_method_data,
init_flags,
method_flags,
frontend,
- backend_fw,
on_device,
+ backend_fw,
):
input_dtype, x = dtype_and_x
helpers.test_frontend_method(
init_input_dtypes=input_dtype,
+ backend_to_test=backend_fw,
init_all_as_kwargs_np={
"data": x[0],
},
@@ -6513,31 +6594,24 @@ def test_torch_tensor_double(
init_flags=init_flags,
method_flags=method_flags,
frontend=frontend,
- backend_to_test=backend_fw,
on_device=on_device,
)
-# dsplit
+# fill_
@handle_frontend_method(
class_tree=CLASS_TREE,
init_tree="torch.tensor",
- method_name="dsplit",
- dtype_value=helpers.dtype_and_values(
- available_dtypes=helpers.get_dtypes("valid"),
- shape=st.shared(helpers.get_shape(min_num_dims=3), key="value_shape"),
- ),
- indices_or_sections=_get_splits(
- min_num_dims=3,
- axis=2,
- allow_none=False,
- allow_array_indices=False,
- is_mod_split=True,
+ method_name="fill_",
+ dtype_and_x=helpers.dtype_and_values(
+ available_dtypes=helpers.get_dtypes("float"),
),
+ value=helpers.floats(min_value=1, max_value=10),
+ test_inplace=st.just(True),
)
-def test_torch_tensor_dsplit(
- dtype_value,
- indices_or_sections,
+def test_torch_fill_(
+ dtype_and_x,
+ value,
frontend_method_data,
init_flags,
method_flags,
@@ -6545,15 +6619,17 @@ def test_torch_tensor_dsplit(
on_device,
backend_fw,
):
- input_dtype, x = dtype_value
+ input_dtype, x = dtype_and_x
helpers.test_frontend_method(
init_input_dtypes=input_dtype,
backend_to_test=backend_fw,
init_all_as_kwargs_np={
"data": x[0],
},
- method_input_dtypes=[],
- method_all_as_kwargs_np={"indices_or_sections": indices_or_sections},
+ method_input_dtypes=input_dtype,
+ method_all_as_kwargs_np={
+ "value": value,
+ },
frontend_method_data=frontend_method_data,
init_flags=init_flags,
method_flags=method_flags,
@@ -6562,35 +6638,18 @@ def test_torch_tensor_dsplit(
)
-@given(
- dtype_x=helpers.dtype_and_values(
- available_dtypes=helpers.get_dtypes("valid", prune_function=False)
- ).filter(lambda x: "bfloat16" not in x[0]),
-)
-def test_torch_tensor_dtype(dtype_x, backend_fw):
- ivy.set_backend(backend_fw)
- dtype, data = dtype_x
- x = Tensor(data[0])
- x.ivy_array = data[0]
- ivy.utils.assertions.check_equal(x.dtype, dtype[0], as_array=False)
- ivy.previous_backend()
-
-
+# fix
@handle_frontend_method(
class_tree=CLASS_TREE,
init_tree="torch.tensor",
- method_name="eq_",
- dtype_and_x=helpers.dtype_and_values(
- available_dtypes=helpers.get_dtypes("float"),
- num_arrays=2,
- min_value=-1e04,
- max_value=1e04,
- allow_inf=False,
+ method_name="fix",
+ dtype_value=helpers.dtype_and_values(
+ available_dtypes=helpers.get_dtypes("numeric"),
+ shape=st.shared(helpers.get_shape(min_num_dims=1), key="shape"),
),
- test_inplace=st.just(True),
)
-def test_torch_tensor_eq_(
- dtype_and_x,
+def test_torch_fix(
+ dtype_value,
frontend_method_data,
init_flags,
method_flags,
@@ -6598,7 +6657,7 @@ def test_torch_tensor_eq_(
on_device,
backend_fw,
):
- input_dtype, x = dtype_and_x
+ input_dtype, x = dtype_value
helpers.test_frontend_method(
init_input_dtypes=input_dtype,
backend_to_test=backend_fw,
@@ -6606,9 +6665,7 @@ def test_torch_tensor_eq_(
"data": x[0],
},
method_input_dtypes=input_dtype,
- method_all_as_kwargs_np={
- "other": x[1],
- },
+ method_all_as_kwargs_np={},
frontend_method_data=frontend_method_data,
init_flags=init_flags,
method_flags=method_flags,
@@ -6617,30 +6674,27 @@ def test_torch_tensor_eq_(
)
-# equal
+# fix_
@handle_frontend_method(
class_tree=CLASS_TREE,
init_tree="torch.tensor",
- method_name="equal",
- dtype_and_x=helpers.dtype_and_values(
+ method_name="fix_",
+ dtype_value=helpers.dtype_and_values(
available_dtypes=helpers.get_dtypes("numeric"),
- num_arrays=2,
- shared_dtype=True,
- min_num_dims=1,
- min_value=-1e04,
- max_value=1e04,
+ shape=st.shared(helpers.get_shape(min_num_dims=1), key="shape"),
),
+ test_inplace=st.just(True),
)
-def test_torch_tensor_equal(
- dtype_and_x,
- frontend,
+def test_torch_fix_(
+ dtype_value,
frontend_method_data,
init_flags,
method_flags,
+ frontend,
on_device,
backend_fw,
):
- input_dtype, x = dtype_and_x
+ input_dtype, x = dtype_value
helpers.test_frontend_method(
init_input_dtypes=input_dtype,
backend_to_test=backend_fw,
@@ -6648,30 +6702,35 @@ def test_torch_tensor_equal(
"data": x[0],
},
method_input_dtypes=input_dtype,
- method_all_as_kwargs_np={
- "other": x[1],
- },
+ method_all_as_kwargs_np={},
frontend_method_data=frontend_method_data,
init_flags=init_flags,
method_flags=method_flags,
frontend=frontend,
- atol_=1e-04,
- rtol_=1e-04,
on_device=on_device,
)
-# erf
+# flatten
@handle_frontend_method(
class_tree=CLASS_TREE,
init_tree="torch.tensor",
- method_name="erf",
- dtype_and_x=helpers.dtype_and_values(
- available_dtypes=helpers.get_dtypes("float"),
+ method_name="flatten",
+ dtype_value=helpers.dtype_and_values(
+ available_dtypes=helpers.get_dtypes("valid"),
+ shape=st.shared(helpers.get_shape(), key="shape"),
+ ),
+ axes=helpers.get_axis(
+ shape=st.shared(helpers.get_shape(), key="shape"),
+ min_size=2,
+ max_size=2,
+ unique=False,
+ force_tuple=True,
),
)
-def test_torch_tensor_erf(
- dtype_and_x,
+def test_torch_flatten(
+ dtype_value,
+ axes,
frontend_method_data,
init_flags,
method_flags,
@@ -6679,7 +6738,7 @@ def test_torch_tensor_erf(
on_device,
backend_fw,
):
- input_dtype, x = dtype_and_x
+ input_dtype, x = dtype_value
helpers.test_frontend_method(
init_input_dtypes=input_dtype,
backend_to_test=backend_fw,
@@ -6687,7 +6746,10 @@ def test_torch_tensor_erf(
"data": x[0],
},
method_input_dtypes=input_dtype,
- method_all_as_kwargs_np={},
+ method_all_as_kwargs_np={
+ "start_dim": axes[0],
+ "end_dim": axes[1],
+ },
frontend_method_data=frontend_method_data,
init_flags=init_flags,
method_flags=method_flags,
@@ -6696,18 +6758,17 @@ def test_torch_tensor_erf(
)
-# erf_
+# flip
@handle_frontend_method(
class_tree=CLASS_TREE,
init_tree="torch.tensor",
- method_name="erf_",
- dtype_and_x=helpers.dtype_and_values(
- available_dtypes=helpers.get_dtypes("valid"),
+ method_name="flip",
+ dtype_values_axis=_array_idxes_n_dtype(
+ available_dtypes=helpers.get_dtypes("float"),
),
- test_inplace=st.just(True),
)
-def test_torch_tensor_erf_(
- dtype_and_x,
+def test_torch_flip(
+ dtype_values_axis,
frontend_method_data,
init_flags,
method_flags,
@@ -6715,15 +6776,17 @@ def test_torch_tensor_erf_(
on_device,
backend_fw,
):
- input_dtype, x = dtype_and_x
+ x, idxes, dtype = dtype_values_axis
helpers.test_frontend_method(
- init_input_dtypes=input_dtype,
+ init_input_dtypes=dtype,
backend_to_test=backend_fw,
init_all_as_kwargs_np={
"data": x[0],
},
- method_input_dtypes=input_dtype,
- method_all_as_kwargs_np={},
+ method_input_dtypes=dtype,
+ method_all_as_kwargs_np={
+ "dims": idxes,
+ },
frontend_method_data=frontend_method_data,
init_flags=init_flags,
method_flags=method_flags,
@@ -6732,16 +6795,17 @@ def test_torch_tensor_erf_(
)
-# exp
+# fliplr
@handle_frontend_method(
class_tree=CLASS_TREE,
init_tree="torch.tensor",
- method_name="exp",
+ method_name="fliplr",
dtype_and_x=helpers.dtype_and_values(
- available_dtypes=helpers.get_dtypes("numeric"),
+ available_dtypes=helpers.get_dtypes("float"),
+ min_num_dims=2,
),
)
-def test_torch_tensor_exp(
+def test_torch_fliplr(
dtype_and_x,
frontend_method_data,
init_flags,
@@ -6750,14 +6814,14 @@ def test_torch_tensor_exp(
on_device,
backend_fw,
):
- input_dtype, x = dtype_and_x
+ dtype, x = dtype_and_x
helpers.test_frontend_method(
- init_input_dtypes=input_dtype,
+ init_input_dtypes=dtype,
backend_to_test=backend_fw,
init_all_as_kwargs_np={
"data": x[0],
},
- method_input_dtypes=input_dtype,
+ method_input_dtypes=dtype,
method_all_as_kwargs_np={},
frontend_method_data=frontend_method_data,
init_flags=init_flags,
@@ -6767,18 +6831,16 @@ def test_torch_tensor_exp(
)
-# exp_
@handle_frontend_method(
class_tree=CLASS_TREE,
init_tree="torch.tensor",
- method_name="exp_",
- dtype_and_x=helpers.dtype_and_values(
- available_dtypes=helpers.get_dtypes("numeric"),
+ method_name="float",
+ dtype_x=helpers.dtype_and_values(
+ available_dtypes=helpers.get_dtypes("valid"),
),
- test_inplace=st.just(True),
)
-def test_torch_tensor_exp_(
- dtype_and_x,
+def test_torch_float(
+ dtype_x,
frontend_method_data,
init_flags,
method_flags,
@@ -6786,7 +6848,7 @@ def test_torch_tensor_exp_(
on_device,
backend_fw,
):
- input_dtype, x = dtype_and_x
+ input_dtype, x = dtype_x
helpers.test_frontend_method(
init_input_dtypes=input_dtype,
backend_to_test=backend_fw,
@@ -6803,16 +6865,17 @@ def test_torch_tensor_exp_(
)
+# floor
@handle_frontend_method(
class_tree=CLASS_TREE,
init_tree="torch.tensor",
- method_name="expand",
- dtype_x_shape=_expand_helper(),
- unpack_shape=st.booleans(),
+ method_name="floor",
+ dtype_and_x=helpers.dtype_and_values(
+ available_dtypes=helpers.get_dtypes("float"),
+ ),
)
-def test_torch_tensor_expand(
- dtype_x_shape,
- unpack_shape,
+def test_torch_floor(
+ dtype_and_x,
frontend_method_data,
init_flags,
method_flags,
@@ -6820,18 +6883,7 @@ def test_torch_tensor_expand(
on_device,
backend_fw,
):
- input_dtype, x, shape = dtype_x_shape
- if unpack_shape:
- method_flags.num_positional_args = len(shape) + 1
- size = {}
- i = 0
- for x_ in shape:
- size[f"x{i}"] = x_
- i += 1
- else:
- size = {
- "size": shape,
- }
+ input_dtype, x = dtype_and_x
helpers.test_frontend_method(
init_input_dtypes=input_dtype,
backend_to_test=backend_fw,
@@ -6839,7 +6891,7 @@ def test_torch_tensor_expand(
"data": x[0],
},
method_input_dtypes=input_dtype,
- method_all_as_kwargs_np=size,
+ method_all_as_kwargs_np={},
frontend_method_data=frontend_method_data,
init_flags=init_flags,
method_flags=method_flags,
@@ -6848,17 +6900,17 @@ def test_torch_tensor_expand(
)
-# expand_as
@handle_frontend_method(
class_tree=CLASS_TREE,
init_tree="torch.tensor",
- method_name="expand_as",
- dtype_x=helpers.dtype_and_values(
- available_dtypes=helpers.get_dtypes("valid"), num_arrays=2
+ method_name="floor_",
+ dtype_and_x=helpers.dtype_and_values(
+ available_dtypes=helpers.get_dtypes("float"),
),
+ test_inplace=st.just(True),
)
-def test_torch_tensor_expand_as(
- dtype_x,
+def test_torch_floor_(
+ dtype_and_x,
frontend_method_data,
init_flags,
method_flags,
@@ -6866,7 +6918,7 @@ def test_torch_tensor_expand_as(
on_device,
backend_fw,
):
- input_dtype, x = dtype_x
+ input_dtype, x = dtype_and_x
helpers.test_frontend_method(
init_input_dtypes=input_dtype,
backend_to_test=backend_fw,
@@ -6874,9 +6926,7 @@ def test_torch_tensor_expand_as(
"data": x[0],
},
method_input_dtypes=input_dtype,
- method_all_as_kwargs_np={
- "other": x[1],
- },
+ method_all_as_kwargs_np={},
frontend_method_data=frontend_method_data,
init_flags=init_flags,
method_flags=method_flags,
@@ -6885,16 +6935,17 @@ def test_torch_tensor_expand_as(
)
-# expm1
+# fmax
@handle_frontend_method(
class_tree=CLASS_TREE,
init_tree="torch.tensor",
- method_name="expm1",
+ method_name="fmax",
dtype_and_x=helpers.dtype_and_values(
- available_dtypes=helpers.get_dtypes("numeric"),
+ available_dtypes=helpers.get_dtypes("float"),
+ num_arrays=2,
),
)
-def test_torch_tensor_expm1(
+def test_torch_fmax(
dtype_and_x,
frontend_method_data,
init_flags,
@@ -6911,7 +6962,9 @@ def test_torch_tensor_expm1(
"data": x[0],
},
method_input_dtypes=input_dtype,
- method_all_as_kwargs_np={},
+ method_all_as_kwargs_np={
+ "other": x[1],
+ },
frontend_method_data=frontend_method_data,
init_flags=init_flags,
method_flags=method_flags,
@@ -6920,17 +6973,17 @@ def test_torch_tensor_expm1(
)
-# expm1_
+# fmin
@handle_frontend_method(
class_tree=CLASS_TREE,
init_tree="torch.tensor",
- method_name="expm1_",
+ method_name="fmin",
dtype_and_x=helpers.dtype_and_values(
available_dtypes=helpers.get_dtypes("float"),
+ num_arrays=2,
),
- test_inplace=st.just(True),
)
-def test_torch_tensor_expm1_(
+def test_torch_fmin(
dtype_and_x,
frontend_method_data,
init_flags,
@@ -6947,7 +7000,9 @@ def test_torch_tensor_expm1_(
"data": x[0],
},
method_input_dtypes=input_dtype,
- method_all_as_kwargs_np={},
+ method_all_as_kwargs_np={
+ "other": x[1],
+ },
frontend_method_data=frontend_method_data,
init_flags=init_flags,
method_flags=method_flags,
@@ -6956,24 +7011,26 @@ def test_torch_tensor_expm1_(
)
-# fill_
+# fmod
@handle_frontend_method(
class_tree=CLASS_TREE,
init_tree="torch.tensor",
- method_name="fill_",
+ method_name="fmod",
dtype_and_x=helpers.dtype_and_values(
available_dtypes=helpers.get_dtypes("float"),
+ num_arrays=2,
+ shared_dtype=True,
+ min_num_dims=1,
+ min_value=-100,
+ max_value=100,
),
- value=helpers.floats(min_value=1, max_value=10),
- test_inplace=st.just(True),
)
-def test_torch_tensor_fill_(
+def test_torch_fmod(
dtype_and_x,
- value,
+ frontend,
frontend_method_data,
init_flags,
method_flags,
- frontend,
on_device,
backend_fw,
):
@@ -6981,122 +7038,120 @@ def test_torch_tensor_fill_(
helpers.test_frontend_method(
init_input_dtypes=input_dtype,
backend_to_test=backend_fw,
- init_all_as_kwargs_np={
- "data": x[0],
- },
+ init_all_as_kwargs_np={"data": x[0]},
method_input_dtypes=input_dtype,
- method_all_as_kwargs_np={
- "value": value,
- },
+ method_all_as_kwargs_np={"other": x[1]},
+ frontend=frontend,
frontend_method_data=frontend_method_data,
init_flags=init_flags,
method_flags=method_flags,
- frontend=frontend,
on_device=on_device,
)
-# fix
+# fmod_
@handle_frontend_method(
class_tree=CLASS_TREE,
init_tree="torch.tensor",
- method_name="fix",
- dtype_value=helpers.dtype_and_values(
- available_dtypes=helpers.get_dtypes("numeric"),
- shape=st.shared(helpers.get_shape(min_num_dims=1), key="shape"),
+ method_name="fmod_",
+ dtype_and_x=helpers.dtype_and_values(
+ available_dtypes=helpers.get_dtypes("float"),
+ num_arrays=2,
+ shared_dtype=True,
+ min_num_dims=1,
+ min_value=-100,
+ max_value=100,
),
+ test_inplace=st.just(True),
)
-def test_torch_tensor_fix(
- dtype_value,
+def test_torch_fmod_(
+ dtype_and_x,
+ frontend,
frontend_method_data,
init_flags,
method_flags,
- frontend,
on_device,
backend_fw,
):
- input_dtype, x = dtype_value
+ input_dtype, x = dtype_and_x
helpers.test_frontend_method(
init_input_dtypes=input_dtype,
backend_to_test=backend_fw,
- init_all_as_kwargs_np={
- "data": x[0],
- },
+ init_all_as_kwargs_np={"data": x[0]},
method_input_dtypes=input_dtype,
- method_all_as_kwargs_np={},
+ method_all_as_kwargs_np={"other": x[1]},
+ frontend=frontend,
frontend_method_data=frontend_method_data,
init_flags=init_flags,
method_flags=method_flags,
- frontend=frontend,
on_device=on_device,
)
-# fix_
@handle_frontend_method(
class_tree=CLASS_TREE,
init_tree="torch.tensor",
- method_name="fix_",
- dtype_value=helpers.dtype_and_values(
- available_dtypes=helpers.get_dtypes("numeric"),
- shape=st.shared(helpers.get_shape(min_num_dims=1), key="shape"),
+ method_name="gather",
+ params_indices_others=helpers.array_indices_axis(
+ array_dtypes=helpers.get_dtypes("valid"),
+ indices_dtypes=["int64"],
+ indices_same_dims=True,
),
- test_inplace=st.just(True),
)
-def test_torch_tensor_fix_(
- dtype_value,
+def test_torch_gather(
+ params_indices_others,
+ frontend,
frontend_method_data,
init_flags,
method_flags,
- frontend,
on_device,
backend_fw,
):
- input_dtype, x = dtype_value
+ input_dtypes, x, indices, axis, batch_dims = params_indices_others
helpers.test_frontend_method(
- init_input_dtypes=input_dtype,
+ init_input_dtypes=[input_dtypes[0]],
backend_to_test=backend_fw,
- init_all_as_kwargs_np={
- "data": x[0],
+ init_all_as_kwargs_np={"data": x},
+ method_input_dtypes=[input_dtypes[1]],
+ method_all_as_kwargs_np={
+ "dim": axis,
+ "index": indices,
},
- method_input_dtypes=input_dtype,
- method_all_as_kwargs_np={},
+ frontend=frontend,
frontend_method_data=frontend_method_data,
init_flags=init_flags,
method_flags=method_flags,
- frontend=frontend,
on_device=on_device,
)
-# flatten
+# gcd
@handle_frontend_method(
class_tree=CLASS_TREE,
init_tree="torch.tensor",
- method_name="flatten",
- dtype_value=helpers.dtype_and_values(
- available_dtypes=helpers.get_dtypes("valid"),
- shape=st.shared(helpers.get_shape(), key="shape"),
- ),
- axes=helpers.get_axis(
- shape=st.shared(helpers.get_shape(), key="shape"),
- min_size=2,
- max_size=2,
- unique=False,
- force_tuple=True,
+ method_name="gcd",
+ dtype_and_x=helpers.dtype_and_values(
+ available_dtypes=helpers.get_dtypes("integer"),
+ min_value=-100,
+ max_value=100,
+ min_num_dims=1,
+ max_num_dims=3,
+ min_dim_size=1,
+ max_dim_size=3,
+ num_arrays=2,
+ shared_dtype=True,
),
)
-def test_torch_tensor_flatten(
- dtype_value,
- axes,
+def test_torch_gcd(
+ dtype_and_x,
+ frontend,
frontend_method_data,
init_flags,
method_flags,
- frontend,
on_device,
backend_fw,
):
- input_dtype, x = dtype_value
+ input_dtype, x = dtype_and_x
helpers.test_frontend_method(
init_input_dtypes=input_dtype,
backend_to_test=backend_fw,
@@ -7105,28 +7160,79 @@ def test_torch_tensor_flatten(
},
method_input_dtypes=input_dtype,
method_all_as_kwargs_np={
- "start_dim": axes[0],
- "end_dim": axes[1],
+ "other": x[1],
},
+ frontend=frontend,
frontend_method_data=frontend_method_data,
init_flags=init_flags,
method_flags=method_flags,
- frontend=frontend,
on_device=on_device,
)
-# flip
+@given(
+ dtype_x=helpers.dtype_and_values(
+ available_dtypes=helpers.get_dtypes("valid", prune_function=False)
+ ).filter(
+ lambda x: "bfloat16" not in x[0]
+ and "uint16" not in x[0]
+ and "uint32" not in x[0]
+ and "uint64" not in x[0]
+ ),
+)
+def test_torch_get_device(
+ dtype_x,
+ backend_fw,
+):
+ ivy.set_backend(backend_fw)
+ _, data = dtype_x
+ x = Tensor(data[0])
+ ivy.utils.assertions.check_equal(x.get_device, -1, as_array=False)
+ x = Tensor(data[0], "gpu:0")
+ ivy.utils.assertions.check_equal(x.get_device, 0, as_array=False)
+ x = Tensor(data[0], "tpu:3")
+ ivy.utils.assertions.check_equal(x.get_device, 3, as_array=False)
+ ivy.previous_backend()
+
+
+def test_torch_grad(backend_fw):
+ ivy.set_backend(backend_fw)
+ x = Tensor(ivy.array([1.0, 2.0, 3.0]))
+ grads = ivy.array([1.0, 2.0, 3.0])
+ x._grads = grads
+ assert ivy.array_equal(x.grad, grads)
+ ivy.previous_backend()
+
+
+def test_torch_grad_fn(backend_fw):
+ ivy.set_backend(backend_fw)
+ x = Tensor(ivy.array([3.0]), requires_grad=True)
+ ivy.utils.assertions.check_equal(x.grad_fn, None, as_array=False)
+ y = x.pow(2)
+ ivy.utils.assertions.check_equal(y.grad_fn, "PowBackward", as_array=False)
+ ivy.utils.assertions.check_equal(
+ y.grad_fn.next_functions[0], "AccumulateGrad", as_array=False
+ )
+ z = y.detach()
+ ivy.utils.assertions.check_equal(z.grad_fn, None, as_array=False)
+ ivy.previous_backend()
+
+
+# greater
@handle_frontend_method(
class_tree=CLASS_TREE,
init_tree="torch.tensor",
- method_name="flip",
- dtype_values_axis=_array_idxes_n_dtype(
- available_dtypes=helpers.get_dtypes("float"),
+ method_name="greater",
+ dtype_and_x=helpers.dtype_and_values(
+ available_dtypes=helpers.get_dtypes("valid"),
+ num_arrays=2,
+ min_value=-1e04,
+ max_value=1e04,
+ allow_inf=False,
),
)
-def test_torch_tensor_flip(
- dtype_values_axis,
+def test_torch_greater(
+ dtype_and_x,
frontend_method_data,
init_flags,
method_flags,
@@ -7134,16 +7240,16 @@ def test_torch_tensor_flip(
on_device,
backend_fw,
):
- x, idxes, dtype = dtype_values_axis
+ input_dtype, x = dtype_and_x
helpers.test_frontend_method(
- init_input_dtypes=dtype,
+ init_input_dtypes=input_dtype,
backend_to_test=backend_fw,
init_all_as_kwargs_np={
"data": x[0],
},
- method_input_dtypes=dtype,
+ method_input_dtypes=input_dtype,
method_all_as_kwargs_np={
- "dims": idxes,
+ "other": x[1],
},
frontend_method_data=frontend_method_data,
init_flags=init_flags,
@@ -7153,17 +7259,21 @@ def test_torch_tensor_flip(
)
-# fliplr
+# greater_
@handle_frontend_method(
class_tree=CLASS_TREE,
init_tree="torch.tensor",
- method_name="fliplr",
+ method_name="greater_",
dtype_and_x=helpers.dtype_and_values(
- available_dtypes=helpers.get_dtypes("float"),
- min_num_dims=2,
+ available_dtypes=helpers.get_dtypes("valid"),
+ num_arrays=2,
+ min_value=-1e04,
+ max_value=1e04,
+ allow_inf=False,
),
+ test_inplace=st.just(True),
)
-def test_torch_tensor_fliplr(
+def test_torch_greater_(
dtype_and_x,
frontend_method_data,
init_flags,
@@ -7172,15 +7282,17 @@ def test_torch_tensor_fliplr(
on_device,
backend_fw,
):
- dtype, x = dtype_and_x
+ input_dtype, x = dtype_and_x
helpers.test_frontend_method(
- init_input_dtypes=dtype,
+ init_input_dtypes=input_dtype,
backend_to_test=backend_fw,
init_all_as_kwargs_np={
"data": x[0],
},
- method_input_dtypes=dtype,
- method_all_as_kwargs_np={},
+ method_input_dtypes=input_dtype,
+ method_all_as_kwargs_np={
+ "other": x[1],
+ },
frontend_method_data=frontend_method_data,
init_flags=init_flags,
method_flags=method_flags,
@@ -7189,16 +7301,21 @@ def test_torch_tensor_fliplr(
)
+# greater_equal
@handle_frontend_method(
class_tree=CLASS_TREE,
init_tree="torch.tensor",
- method_name="float",
- dtype_x=helpers.dtype_and_values(
+ method_name="greater_equal",
+ dtype_and_x=helpers.dtype_and_values(
available_dtypes=helpers.get_dtypes("valid"),
+ num_arrays=2,
+ min_value=-1e04,
+ max_value=1e04,
+ allow_inf=False,
),
)
-def test_torch_tensor_float(
- dtype_x,
+def test_torch_greater_equal(
+ dtype_and_x,
frontend_method_data,
init_flags,
method_flags,
@@ -7206,7 +7323,7 @@ def test_torch_tensor_float(
on_device,
backend_fw,
):
- input_dtype, x = dtype_x
+ input_dtype, x = dtype_and_x
helpers.test_frontend_method(
init_input_dtypes=input_dtype,
backend_to_test=backend_fw,
@@ -7214,7 +7331,9 @@ def test_torch_tensor_float(
"data": x[0],
},
method_input_dtypes=input_dtype,
- method_all_as_kwargs_np={},
+ method_all_as_kwargs_np={
+ "other": x[1],
+ },
frontend_method_data=frontend_method_data,
init_flags=init_flags,
method_flags=method_flags,
@@ -7223,16 +7342,21 @@ def test_torch_tensor_float(
)
-# floor
+# greater_equal_
@handle_frontend_method(
class_tree=CLASS_TREE,
init_tree="torch.tensor",
- method_name="floor",
+ method_name="greater_equal_",
dtype_and_x=helpers.dtype_and_values(
- available_dtypes=helpers.get_dtypes("float"),
+ available_dtypes=helpers.get_dtypes("valid"),
+ num_arrays=2,
+ min_value=-1e04,
+ max_value=1e04,
+ allow_inf=False,
),
+ test_inplace=st.just(True),
)
-def test_torch_tensor_floor(
+def test_torch_greater_equal_(
dtype_and_x,
frontend_method_data,
init_flags,
@@ -7249,7 +7373,9 @@ def test_torch_tensor_floor(
"data": x[0],
},
method_input_dtypes=input_dtype,
- method_all_as_kwargs_np={},
+ method_all_as_kwargs_np={
+ "other": x[1],
+ },
frontend_method_data=frontend_method_data,
init_flags=init_flags,
method_flags=method_flags,
@@ -7258,16 +7384,16 @@ def test_torch_tensor_floor(
)
+# half
@handle_frontend_method(
class_tree=CLASS_TREE,
init_tree="torch.tensor",
- method_name="floor_",
+ method_name="half",
dtype_and_x=helpers.dtype_and_values(
- available_dtypes=helpers.get_dtypes("float"),
+ available_dtypes=helpers.get_dtypes("valid"),
),
- test_inplace=st.just(True),
)
-def test_torch_tensor_floor_(
+def test_torch_half(
dtype_and_x,
frontend_method_data,
init_flags,
@@ -7293,56 +7419,63 @@ def test_torch_tensor_floor_(
)
-# fmax
@handle_frontend_method(
class_tree=CLASS_TREE,
init_tree="torch.tensor",
- method_name="fmax",
- dtype_and_x=helpers.dtype_and_values(
+ method_name="heaviside",
+ dtype_and_values=helpers.dtype_and_values(
available_dtypes=helpers.get_dtypes("float"),
num_arrays=2,
),
)
-def test_torch_tensor_fmax(
- dtype_and_x,
+def test_torch_heaviside(
+ dtype_and_values,
+ frontend,
frontend_method_data,
init_flags,
method_flags,
- frontend,
on_device,
backend_fw,
):
- input_dtype, x = dtype_and_x
+ input_dtype, values = dtype_and_values
helpers.test_frontend_method(
init_input_dtypes=input_dtype,
backend_to_test=backend_fw,
init_all_as_kwargs_np={
- "data": x[0],
+ "data": values[0],
},
method_input_dtypes=input_dtype,
method_all_as_kwargs_np={
- "other": x[1],
+ "values": values[1],
},
- frontend_method_data=frontend_method_data,
init_flags=init_flags,
method_flags=method_flags,
+ frontend_method_data=frontend_method_data,
frontend=frontend,
on_device=on_device,
)
-# fmin
+# hsplit
@handle_frontend_method(
class_tree=CLASS_TREE,
init_tree="torch.tensor",
- method_name="fmin",
- dtype_and_x=helpers.dtype_and_values(
- available_dtypes=helpers.get_dtypes("float"),
- num_arrays=2,
+ method_name="hsplit",
+ dtype_value=helpers.dtype_and_values(
+ available_dtypes=helpers.get_dtypes("valid"),
+ shape=st.shared(helpers.get_shape(min_num_dims=2), key="value_shape"),
+ ),
+ indices_or_sections=_get_splits(
+ min_num_dims=1,
+ axis=1,
+ allow_none=False,
+ allow_array_indices=False,
+ is_mod_split=True,
),
)
-def test_torch_tensor_fmin(
- dtype_and_x,
+def test_torch_hsplit(
+ dtype_value,
+ indices_or_sections,
frontend_method_data,
init_flags,
method_flags,
@@ -7350,17 +7483,15 @@ def test_torch_tensor_fmin(
on_device,
backend_fw,
):
- input_dtype, x = dtype_and_x
+ input_dtype, x = dtype_value
helpers.test_frontend_method(
init_input_dtypes=input_dtype,
backend_to_test=backend_fw,
init_all_as_kwargs_np={
"data": x[0],
},
- method_input_dtypes=input_dtype,
- method_all_as_kwargs_np={
- "other": x[1],
- },
+ method_input_dtypes=[],
+ method_all_as_kwargs_np={"indices_or_sections": indices_or_sections},
frontend_method_data=frontend_method_data,
init_flags=init_flags,
method_flags=method_flags,
@@ -7369,95 +7500,132 @@ def test_torch_tensor_fmin(
)
-# fmod
+@given(
+ dtype_x=helpers.dtype_and_values(
+ available_dtypes=helpers.get_dtypes("complex", prune_function=False)
+ ),
+)
+def test_torch_imag(dtype_x, backend_fw):
+ ivy.set_backend(backend_fw)
+ _, data = dtype_x
+ x = Tensor(data[0])
+ x.ivy_array = data[0]
+ ivy.utils.assertions.check_equal(x.imag, ivy.imag(data[0]))
+ ivy.previous_backend()
+
+
@handle_frontend_method(
class_tree=CLASS_TREE,
init_tree="torch.tensor",
- method_name="fmod",
- dtype_and_x=helpers.dtype_and_values(
- available_dtypes=helpers.get_dtypes("float"),
- num_arrays=2,
- shared_dtype=True,
- min_num_dims=1,
- min_value=-100,
- max_value=100,
- ),
+ method_name="index_add",
+ xs_dtypes_dim_idx=_arrays_dim_idx_n_dtypes(),
+ alpha=st.integers(min_value=1, max_value=2),
)
-def test_torch_tensor_fmod(
- dtype_and_x,
- frontend,
+def test_torch_index_add(
+ *,
+ xs_dtypes_dim_idx,
+ alpha,
frontend_method_data,
init_flags,
method_flags,
on_device,
+ frontend,
backend_fw,
):
- input_dtype, x = dtype_and_x
+ xs, input_dtypes, axis, indices = xs_dtypes_dim_idx
+ if xs[0].shape[axis] < xs[1].shape[axis]:
+ source, input = xs
+ else:
+ input, source = xs
helpers.test_frontend_method(
- init_input_dtypes=input_dtype,
+ init_input_dtypes=[input_dtypes[0]],
backend_to_test=backend_fw,
- init_all_as_kwargs_np={"data": x[0]},
- method_input_dtypes=input_dtype,
- method_all_as_kwargs_np={"other": x[1]},
+ init_all_as_kwargs_np={
+ "data": input,
+ },
+ method_input_dtypes=["int64", input_dtypes[1]],
+ method_all_as_kwargs_np={
+ "dim": axis,
+ "index": indices,
+ "source": source,
+ "alpha": alpha,
+ },
frontend=frontend,
frontend_method_data=frontend_method_data,
init_flags=init_flags,
method_flags=method_flags,
on_device=on_device,
+ rtol_=1e-03,
)
-# fmod_
+# index_add
@handle_frontend_method(
class_tree=CLASS_TREE,
init_tree="torch.tensor",
- method_name="fmod_",
- dtype_and_x=helpers.dtype_and_values(
- available_dtypes=helpers.get_dtypes("float"),
- num_arrays=2,
- shared_dtype=True,
- min_num_dims=1,
- min_value=-100,
- max_value=100,
- ),
+ method_name="index_add_",
+ xs_dtypes_dim_idx=_arrays_dim_idx_n_dtypes(),
+ alpha=st.integers(min_value=1, max_value=2),
test_inplace=st.just(True),
)
-def test_torch_tensor_fmod_(
- dtype_and_x,
- frontend,
+def test_torch_index_add_(
+ *,
+ xs_dtypes_dim_idx,
+ alpha,
frontend_method_data,
init_flags,
method_flags,
on_device,
+ frontend,
backend_fw,
):
- input_dtype, x = dtype_and_x
+ xs, input_dtypes, axis, indices = xs_dtypes_dim_idx
+ if xs[0].shape[axis] < xs[1].shape[axis]:
+ source, input = xs
+ else:
+ input, source = xs
helpers.test_frontend_method(
- init_input_dtypes=input_dtype,
+ init_input_dtypes=[input_dtypes[0]],
backend_to_test=backend_fw,
- init_all_as_kwargs_np={"data": x[0]},
- method_input_dtypes=input_dtype,
- method_all_as_kwargs_np={"other": x[1]},
+ init_all_as_kwargs_np={
+ "data": input,
+ },
+ method_input_dtypes=["int64", input_dtypes[1]],
+ method_all_as_kwargs_np={
+ "dim": axis,
+ "index": indices,
+ "source": source,
+ "alpha": alpha,
+ },
frontend=frontend,
frontend_method_data=frontend_method_data,
init_flags=init_flags,
method_flags=method_flags,
on_device=on_device,
+ rtol_=1e-03,
)
+# index_fill
@handle_frontend_method(
class_tree=CLASS_TREE,
init_tree="torch.tensor",
- method_name="gather",
- params_indices_others=helpers.array_indices_axis(
- array_dtypes=helpers.get_dtypes("valid"),
+ method_name="index_fill",
+ dtype_indices_axis=helpers.array_indices_axis(
+ array_dtypes=helpers.get_dtypes("numeric"),
indices_dtypes=["int64"],
- indices_same_dims=True,
+ min_num_dims=1,
+ max_num_dims=5,
+ min_dim_size=1,
+ max_dim_size=10,
+ first_dimension_only=True,
+ indices_same_dims=False,
),
+ value=st.floats(min_value=-100, max_value=100),
)
-def test_torch_tensor_gather(
- params_indices_others,
+def test_torch_index_fill(
+ dtype_indices_axis,
+ value,
frontend,
frontend_method_data,
init_flags,
@@ -7465,7 +7633,9 @@ def test_torch_tensor_gather(
on_device,
backend_fw,
):
- input_dtypes, x, indices, axis, batch_dims = params_indices_others
+ input_dtypes, x, indices, axis, _ = dtype_indices_axis
+ if indices.ndim != 1:
+ indices = ivy.flatten(indices)
helpers.test_frontend_method(
init_input_dtypes=[input_dtypes[0]],
backend_to_test=backend_fw,
@@ -7474,97 +7644,67 @@ def test_torch_tensor_gather(
method_all_as_kwargs_np={
"dim": axis,
"index": indices,
+ "value": value,
},
frontend=frontend,
frontend_method_data=frontend_method_data,
init_flags=init_flags,
method_flags=method_flags,
- on_device=on_device,
- )
-
-
-# gcd
-@handle_frontend_method(
- class_tree=CLASS_TREE,
- init_tree="torch.tensor",
- method_name="gcd",
- dtype_and_x=helpers.dtype_and_values(
- available_dtypes=helpers.get_dtypes("integer"),
- min_value=-100,
- max_value=100,
- min_num_dims=1,
- max_num_dims=3,
- min_dim_size=1,
- max_dim_size=3,
- num_arrays=2,
- shared_dtype=True,
+ on_device=on_device,
+ )
+
+
+# index_select
+@handle_frontend_method(
+ class_tree=CLASS_TREE,
+ init_tree="torch.tensor",
+ method_name="index_select",
+ params_indices_others=helpers.array_indices_axis(
+ array_dtypes=helpers.get_dtypes("valid"),
+ indices_dtypes=["int64"],
+ max_num_dims=1,
+ indices_same_dims=True,
),
)
-def test_torch_tensor_gcd(
- dtype_and_x,
- frontend,
+def test_torch_index_select(
+ params_indices_others,
frontend_method_data,
init_flags,
method_flags,
+ frontend,
on_device,
backend_fw,
):
- input_dtype, x = dtype_and_x
+ input_dtypes, input, indices, axis, batch_dims = params_indices_others
helpers.test_frontend_method(
- init_input_dtypes=input_dtype,
+ init_input_dtypes=[input_dtypes[0]],
backend_to_test=backend_fw,
init_all_as_kwargs_np={
- "data": x[0],
+ "data": input,
},
- method_input_dtypes=input_dtype,
+ method_input_dtypes=[input_dtypes[1]],
method_all_as_kwargs_np={
- "other": x[1],
+ "dim": axis,
+ "index": indices,
},
- frontend=frontend,
frontend_method_data=frontend_method_data,
init_flags=init_flags,
method_flags=method_flags,
+ frontend=frontend,
on_device=on_device,
)
-def test_torch_tensor_grad(backend_fw):
- ivy.set_backend(backend_fw)
- x = Tensor(ivy.array([1.0, 2.0, 3.0]))
- grads = ivy.array([1.0, 2.0, 3.0])
- x._grads = grads
- assert ivy.array_equal(x.grad, grads)
- ivy.previous_backend()
-
-
-def test_torch_tensor_grad_fn(backend_fw):
- ivy.set_backend(backend_fw)
- x = Tensor(ivy.array([3.0]), requires_grad=True)
- ivy.utils.assertions.check_equal(x.grad_fn, None, as_array=False)
- y = x.pow(2)
- ivy.utils.assertions.check_equal(y.grad_fn, "PowBackward", as_array=False)
- ivy.utils.assertions.check_equal(
- y.grad_fn.next_functions[0], "AccumulateGrad", as_array=False
- )
- z = y.detach()
- ivy.utils.assertions.check_equal(z.grad_fn, None, as_array=False)
- ivy.previous_backend()
-
-
-# greater
+# int
@handle_frontend_method(
class_tree=CLASS_TREE,
init_tree="torch.tensor",
- method_name="greater",
+ method_name="int",
dtype_and_x=helpers.dtype_and_values(
- available_dtypes=helpers.get_dtypes("valid"),
- num_arrays=2,
- min_value=-1e04,
- max_value=1e04,
- allow_inf=False,
+ available_dtypes=helpers.get_dtypes("integer"),
),
)
-def test_torch_tensor_greater(
+def test_torch_int(
dtype_and_x,
frontend_method_data,
init_flags,
@@ -7581,9 +7721,7 @@ def test_torch_tensor_greater(
"data": x[0],
},
method_input_dtypes=input_dtype,
- method_all_as_kwargs_np={
- "other": x[1],
- },
+ method_all_as_kwargs_np={},
frontend_method_data=frontend_method_data,
init_flags=init_flags,
method_flags=method_flags,
@@ -7592,21 +7730,17 @@ def test_torch_tensor_greater(
)
-# greater_
+# inverse
@handle_frontend_method(
class_tree=CLASS_TREE,
init_tree="torch.tensor",
- method_name="greater_",
+ method_name="inverse",
dtype_and_x=helpers.dtype_and_values(
- available_dtypes=helpers.get_dtypes("valid"),
- num_arrays=2,
- min_value=-1e04,
- max_value=1e04,
- allow_inf=False,
- ),
- test_inplace=st.just(True),
+ available_dtypes=helpers.get_dtypes("float"),
+ min_num_dims=2,
+ ).filter(lambda s: s[1][0].shape[-1] == s[1][0].shape[-2]),
)
-def test_torch_tensor_greater_(
+def test_torch_inverse(
dtype_and_x,
frontend_method_data,
init_flags,
@@ -7623,9 +7757,7 @@ def test_torch_tensor_greater_(
"data": x[0],
},
method_input_dtypes=input_dtype,
- method_all_as_kwargs_np={
- "other": x[1],
- },
+ method_all_as_kwargs_np={},
frontend_method_data=frontend_method_data,
init_flags=init_flags,
method_flags=method_flags,
@@ -7634,20 +7766,16 @@ def test_torch_tensor_greater_(
)
-# greater_equal
+# is_complex
@handle_frontend_method(
class_tree=CLASS_TREE,
init_tree="torch.tensor",
- method_name="greater_equal",
+ method_name="is_complex",
dtype_and_x=helpers.dtype_and_values(
available_dtypes=helpers.get_dtypes("valid"),
- num_arrays=2,
- min_value=-1e04,
- max_value=1e04,
- allow_inf=False,
),
)
-def test_torch_tensor_greater_equal(
+def test_torch_is_complex(
dtype_and_x,
frontend_method_data,
init_flags,
@@ -7660,13 +7788,9 @@ def test_torch_tensor_greater_equal(
helpers.test_frontend_method(
init_input_dtypes=input_dtype,
backend_to_test=backend_fw,
- init_all_as_kwargs_np={
- "data": x[0],
- },
+ init_all_as_kwargs_np={"data": x[0]},
method_input_dtypes=input_dtype,
- method_all_as_kwargs_np={
- "other": x[1],
- },
+ method_all_as_kwargs_np={},
frontend_method_data=frontend_method_data,
init_flags=init_flags,
method_flags=method_flags,
@@ -7675,21 +7799,35 @@ def test_torch_tensor_greater_equal(
)
-# greater_equal_
+@given(
+ dtype_x=helpers.dtype_and_values(
+ available_dtypes=helpers.get_dtypes("valid", prune_function=False)
+ ).filter(lambda x: "bfloat16" not in x[0]),
+)
+def test_torch_is_cuda(
+ dtype_x,
+ backend_fw,
+):
+ ivy.set_backend(backend_fw)
+ _, data = dtype_x
+ x = Tensor(data[0])
+ x.ivy_array = data[0]
+ ivy.utils.assertions.check_equal(
+ x.is_cuda, "gpu" in ivy.dev(ivy.array(data[0])), as_array=False
+ )
+ ivy.previous_backend()
+
+
+# is_floating_point
@handle_frontend_method(
class_tree=CLASS_TREE,
init_tree="torch.tensor",
- method_name="greater_equal_",
+ method_name="is_floating_point",
dtype_and_x=helpers.dtype_and_values(
available_dtypes=helpers.get_dtypes("valid"),
- num_arrays=2,
- min_value=-1e04,
- max_value=1e04,
- allow_inf=False,
),
- test_inplace=st.just(True),
)
-def test_torch_tensor_greater_equal_(
+def test_torch_is_floating_point(
dtype_and_x,
frontend_method_data,
init_flags,
@@ -7702,13 +7840,9 @@ def test_torch_tensor_greater_equal_(
helpers.test_frontend_method(
init_input_dtypes=input_dtype,
backend_to_test=backend_fw,
- init_all_as_kwargs_np={
- "data": x[0],
- },
+ init_all_as_kwargs_np={"data": x[0]},
method_input_dtypes=input_dtype,
- method_all_as_kwargs_np={
- "other": x[1],
- },
+ method_all_as_kwargs_np={},
frontend_method_data=frontend_method_data,
init_flags=init_flags,
method_flags=method_flags,
@@ -7717,16 +7851,68 @@ def test_torch_tensor_greater_equal_(
)
-# half
+@given(
+ requires_grad=st.booleans(),
+)
+def test_torch_is_leaf(requires_grad, backend_fw):
+ ivy.set_backend(backend_fw)
+ x = Tensor(ivy.array([3.0]), requires_grad=requires_grad)
+ ivy.utils.assertions.check_equal(x.is_leaf, True, as_array=False)
+ y = x.pow(2)
+ ivy.utils.assertions.check_equal(y.is_leaf, not requires_grad, as_array=False)
+ z = y.detach()
+ ivy.utils.assertions.check_equal(z.is_leaf, True, as_array=False)
+ ivy.previous_backend()
+
+
+@given(
+ dtype_x=helpers.dtype_and_values(
+ available_dtypes=helpers.get_dtypes("valid", prune_function=False)
+ ).filter(lambda x: "bfloat16" not in x[0]),
+)
+def test_torch_is_meta(
+ dtype_x,
+ backend_fw,
+):
+ ivy.set_backend(backend_fw)
+ _, data = dtype_x
+ x = Tensor(data[0])
+ x.ivy_array = data[0]
+ ivy.utils.assertions.check_equal(
+ x.is_meta, "meta" in ivy.dev(ivy.array(data[0])), as_array=False
+ )
+ ivy.previous_backend()
+
+
+@given(
+ dtype_x=helpers.dtype_and_values(
+ available_dtypes=helpers.get_dtypes("valid", prune_function=False)
+ ).filter(lambda x: "bfloat16" not in x[0]),
+)
+def test_torch_is_quantized(
+ dtype_x,
+ backend_fw,
+):
+ ivy.set_backend(backend_fw)
+ _, data = dtype_x
+ x = Tensor(data[0])
+ x.ivy_array = data[0]
+ ivy.utils.assertions.check_equal(
+ x.is_quantized, "q" in ivy.dtype(ivy.array(data[0])), as_array=False
+ )
+ ivy.previous_backend()
+
+
+# isinf
@handle_frontend_method(
class_tree=CLASS_TREE,
init_tree="torch.tensor",
- method_name="half",
+ method_name="isinf",
dtype_and_x=helpers.dtype_and_values(
available_dtypes=helpers.get_dtypes("valid"),
),
)
-def test_torch_tensor_half(
+def test_torch_isinf(
dtype_and_x,
frontend_method_data,
init_flags,
@@ -7739,9 +7925,7 @@ def test_torch_tensor_half(
helpers.test_frontend_method(
init_input_dtypes=input_dtype,
backend_to_test=backend_fw,
- init_all_as_kwargs_np={
- "data": x[0],
- },
+ init_all_as_kwargs_np={"data": x[0]},
method_input_dtypes=input_dtype,
method_all_as_kwargs_np={},
frontend_method_data=frontend_method_data,
@@ -7752,17 +7936,17 @@ def test_torch_tensor_half(
)
+# isnan
@handle_frontend_method(
class_tree=CLASS_TREE,
init_tree="torch.tensor",
- method_name="heaviside",
- dtype_and_values=helpers.dtype_and_values(
- available_dtypes=helpers.get_dtypes("float"),
- num_arrays=2,
+ method_name="isnan",
+ dtype_x=helpers.dtype_and_values(
+ available_dtypes=helpers.get_dtypes("valid"),
),
)
-def test_torch_tensor_heaviside(
- dtype_and_values,
+def test_torch_isnan(
+ dtype_x,
frontend,
frontend_method_data,
init_flags,
@@ -7770,45 +7954,32 @@ def test_torch_tensor_heaviside(
on_device,
backend_fw,
):
- input_dtype, values = dtype_and_values
+ input_dtype, x = dtype_x
helpers.test_frontend_method(
init_input_dtypes=input_dtype,
backend_to_test=backend_fw,
- init_all_as_kwargs_np={
- "data": values[0],
- },
+ init_all_as_kwargs_np={"data": x[0]},
method_input_dtypes=input_dtype,
- method_all_as_kwargs_np={
- "values": values[1],
- },
+ method_all_as_kwargs_np={},
+ frontend_method_data=frontend_method_data,
init_flags=init_flags,
method_flags=method_flags,
- frontend_method_data=frontend_method_data,
frontend=frontend,
on_device=on_device,
)
-# hsplit
+# isreal
@handle_frontend_method(
class_tree=CLASS_TREE,
init_tree="torch.tensor",
- method_name="hsplit",
- dtype_value=helpers.dtype_and_values(
- available_dtypes=helpers.get_dtypes("valid"),
- shape=st.shared(helpers.get_shape(min_num_dims=2), key="value_shape"),
- ),
- indices_or_sections=_get_splits(
- min_num_dims=1,
- axis=1,
- allow_none=False,
- allow_array_indices=False,
- is_mod_split=True,
+ method_name="isreal",
+ dtype_and_x=helpers.dtype_and_values(
+ available_dtypes=helpers.get_dtypes("numeric"),
),
)
-def test_torch_tensor_hsplit(
- dtype_value,
- indices_or_sections,
+def test_torch_isreal(
+ dtype_and_x,
frontend_method_data,
init_flags,
method_flags,
@@ -7816,15 +7987,13 @@ def test_torch_tensor_hsplit(
on_device,
backend_fw,
):
- input_dtype, x = dtype_value
+ input_dtype, x = dtype_and_x
helpers.test_frontend_method(
init_input_dtypes=input_dtype,
backend_to_test=backend_fw,
- init_all_as_kwargs_np={
- "data": x[0],
- },
- method_input_dtypes=[],
- method_all_as_kwargs_np={"indices_or_sections": indices_or_sections},
+ init_all_as_kwargs_np={"data": x[0]},
+ method_input_dtypes=input_dtype,
+ method_all_as_kwargs_np={},
frontend_method_data=frontend_method_data,
init_flags=init_flags,
method_flags=method_flags,
@@ -7835,124 +8004,132 @@ def test_torch_tensor_hsplit(
@given(
dtype_x=helpers.dtype_and_values(
- available_dtypes=helpers.get_dtypes("complex", prune_function=False)
- ),
+ available_dtypes=helpers.get_dtypes("valid", prune_function=False)
+ ).filter(lambda x: "bfloat16" not in x[0]),
)
-def test_torch_tensor_imag(dtype_x, backend_fw):
- ivy.set_backend(backend_fw)
+def test_torch_ivy_array(
+ dtype_x,
+ backend_fw,
+):
_, data = dtype_x
+ ivy.set_backend(backend_fw)
x = Tensor(data[0])
x.ivy_array = data[0]
- ivy.utils.assertions.check_equal(x.imag, ivy.imag(data[0]))
- ivy.previous_backend()
+ ret = helpers.flatten_and_to_np(ret=x.ivy_array.data, backend=backend_fw)
+ ret_gt = helpers.flatten_and_to_np(ret=data[0], backend=backend_fw)
+ helpers.value_test(
+ ret_np_flat=ret,
+ ret_np_from_gt_flat=ret_gt,
+ backend="torch",
+ )
+# lcm
@handle_frontend_method(
class_tree=CLASS_TREE,
init_tree="torch.tensor",
- method_name="index_add",
- xs_dtypes_dim_idx=_arrays_dim_idx_n_dtypes(),
- alpha=st.integers(min_value=1, max_value=2),
+ method_name="lcm",
+ dtype_and_x=helpers.dtype_and_values(
+ available_dtypes=helpers.get_dtypes("integer"),
+ num_arrays=2,
+ min_value=-100,
+ max_value=100,
+ min_num_dims=1,
+ max_num_dims=3,
+ min_dim_size=1,
+ max_dim_size=3,
+ shared_dtype=True,
+ ),
)
-def test_torch_tensor_index_add(
- *,
- xs_dtypes_dim_idx,
- alpha,
+def test_torch_lcm(
+ dtype_and_x,
+ frontend,
frontend_method_data,
init_flags,
method_flags,
on_device,
- frontend,
backend_fw,
):
- xs, input_dtypes, axis, indices = xs_dtypes_dim_idx
- if xs[0].shape[axis] < xs[1].shape[axis]:
- source, input = xs
- else:
- input, source = xs
+ input_dtype, x = dtype_and_x
helpers.test_frontend_method(
- init_input_dtypes=[input_dtypes[0]],
+ init_input_dtypes=input_dtype,
backend_to_test=backend_fw,
init_all_as_kwargs_np={
- "data": input,
+ "data": x[0],
},
- method_input_dtypes=["int64", input_dtypes[1]],
+ method_input_dtypes=input_dtype,
method_all_as_kwargs_np={
- "dim": axis,
- "index": indices,
- "source": source,
- "alpha": alpha,
+ "other": x[1],
},
frontend=frontend,
frontend_method_data=frontend_method_data,
init_flags=init_flags,
method_flags=method_flags,
on_device=on_device,
- rtol_=1e-03,
)
-# index_add
+# lcm_
@handle_frontend_method(
class_tree=CLASS_TREE,
init_tree="torch.tensor",
- method_name="index_add_",
- xs_dtypes_dim_idx=_arrays_dim_idx_n_dtypes(),
- alpha=st.integers(min_value=1, max_value=2),
+ method_name="lcm_",
+ dtype_and_x=helpers.dtype_and_values(
+ available_dtypes=helpers.get_dtypes("integer"),
+ num_arrays=2,
+ min_value=-100,
+ max_value=100,
+ min_num_dims=1,
+ max_num_dims=3,
+ min_dim_size=1,
+ max_dim_size=3,
+ shared_dtype=True,
+ ),
test_inplace=st.just(True),
)
-def test_torch_tensor_index_add_(
- *,
- xs_dtypes_dim_idx,
- alpha,
+def test_torch_lcm_(
+ dtype_and_x,
+ frontend,
frontend_method_data,
init_flags,
method_flags,
on_device,
- frontend,
backend_fw,
):
- xs, input_dtypes, axis, indices = xs_dtypes_dim_idx
- if xs[0].shape[axis] < xs[1].shape[axis]:
- source, input = xs
- else:
- input, source = xs
+ input_dtype, x = dtype_and_x
helpers.test_frontend_method(
- init_input_dtypes=[input_dtypes[0]],
+ init_input_dtypes=input_dtype,
backend_to_test=backend_fw,
init_all_as_kwargs_np={
- "data": input,
+ "data": x[0],
},
- method_input_dtypes=["int64", input_dtypes[1]],
+ method_input_dtypes=input_dtype,
method_all_as_kwargs_np={
- "dim": axis,
- "index": indices,
- "source": source,
- "alpha": alpha,
+ "other": x[1],
},
frontend=frontend,
frontend_method_data=frontend_method_data,
init_flags=init_flags,
method_flags=method_flags,
on_device=on_device,
- rtol_=1e-03,
)
-# index_select
+# less
@handle_frontend_method(
class_tree=CLASS_TREE,
init_tree="torch.tensor",
- method_name="index_select",
- params_indices_others=helpers.array_indices_axis(
- array_dtypes=helpers.get_dtypes("valid"),
- indices_dtypes=["int64"],
- max_num_dims=1,
- indices_same_dims=True,
+ method_name="less",
+ dtype_and_x=helpers.dtype_and_values(
+ available_dtypes=helpers.get_dtypes("valid"),
+ num_arrays=2,
+ min_value=-1e04,
+ max_value=1e04,
+ allow_inf=False,
),
)
-def test_torch_tensor_index_select(
- params_indices_others,
+def test_torch_less(
+ dtype_and_x,
frontend_method_data,
init_flags,
method_flags,
@@ -7960,17 +8137,16 @@ def test_torch_tensor_index_select(
on_device,
backend_fw,
):
- input_dtypes, input, indices, axis, batch_dims = params_indices_others
+ input_dtype, x = dtype_and_x
helpers.test_frontend_method(
- init_input_dtypes=[input_dtypes[0]],
+ init_input_dtypes=input_dtype,
backend_to_test=backend_fw,
init_all_as_kwargs_np={
- "data": input,
+ "data": x[0],
},
- method_input_dtypes=[input_dtypes[1]],
+ method_input_dtypes=input_dtype,
method_all_as_kwargs_np={
- "dim": axis,
- "index": indices,
+ "other": x[1],
},
frontend_method_data=frontend_method_data,
init_flags=init_flags,
@@ -7980,46 +8156,62 @@ def test_torch_tensor_index_select(
)
+# less_
@handle_frontend_method(
class_tree=CLASS_TREE,
init_tree="torch.tensor",
- method_name="bmm",
- dtype_and_matrices=_get_dtype_and_3dbatch_matrices(with_input=True, input_3d=True),
+ method_name="less_",
+ dtype_and_x=helpers.dtype_and_values(
+ available_dtypes=helpers.get_dtypes("valid"),
+ num_arrays=2,
+ min_value=-1e04,
+ max_value=1e04,
+ allow_inf=False,
+ ),
+ test_inplace=st.just(True),
)
-def test_torch_tensor_instance_bmm(
- dtype_and_matrices,
- backend_fw,
- frontend,
+def test_torch_less_(
+ dtype_and_x,
frontend_method_data,
init_flags,
method_flags,
+ frontend,
on_device,
+ backend_fw,
):
- input_dtype, _, x, mat2 = dtype_and_matrices
+ input_dtype, x = dtype_and_x
helpers.test_frontend_method(
init_input_dtypes=input_dtype,
- init_all_as_kwargs_np={"data": x},
+ backend_to_test=backend_fw,
+ init_all_as_kwargs_np={
+ "data": x[0],
+ },
method_input_dtypes=input_dtype,
- method_all_as_kwargs_np={"mat2": mat2},
- frontend=frontend,
+ method_all_as_kwargs_np={
+ "other": x[1],
+ },
frontend_method_data=frontend_method_data,
init_flags=init_flags,
method_flags=method_flags,
+ frontend=frontend,
on_device=on_device,
- backend_to_test=backend_fw,
)
-# int
+# less_equal
@handle_frontend_method(
class_tree=CLASS_TREE,
init_tree="torch.tensor",
- method_name="int",
+ method_name="less_equal",
dtype_and_x=helpers.dtype_and_values(
- available_dtypes=helpers.get_dtypes("integer"),
+ available_dtypes=helpers.get_dtypes("valid"),
+ num_arrays=2,
+ min_value=-1e04,
+ max_value=1e04,
+ allow_inf=False,
),
)
-def test_torch_tensor_int(
+def test_torch_less_equal(
dtype_and_x,
frontend_method_data,
init_flags,
@@ -8036,7 +8228,9 @@ def test_torch_tensor_int(
"data": x[0],
},
method_input_dtypes=input_dtype,
- method_all_as_kwargs_np={},
+ method_all_as_kwargs_np={
+ "other": x[1],
+ },
frontend_method_data=frontend_method_data,
init_flags=init_flags,
method_flags=method_flags,
@@ -8045,17 +8239,21 @@ def test_torch_tensor_int(
)
-# inverse
+# less_equal_
@handle_frontend_method(
class_tree=CLASS_TREE,
init_tree="torch.tensor",
- method_name="inverse",
+ method_name="less_equal_",
dtype_and_x=helpers.dtype_and_values(
- available_dtypes=helpers.get_dtypes("float"),
- min_num_dims=2,
- ).filter(lambda s: s[1][0].shape[-1] == s[1][0].shape[-2]),
+ available_dtypes=helpers.get_dtypes("valid"),
+ num_arrays=2,
+ min_value=-1e04,
+ max_value=1e04,
+ allow_inf=False,
+ ),
+ test_inplace=st.just(True),
)
-def test_torch_tensor_inverse(
+def test_torch_less_equal_(
dtype_and_x,
frontend_method_data,
init_flags,
@@ -8072,7 +8270,9 @@ def test_torch_tensor_inverse(
"data": x[0],
},
method_input_dtypes=input_dtype,
- method_all_as_kwargs_np={},
+ method_all_as_kwargs_np={
+ "other": x[1],
+ },
frontend_method_data=frontend_method_data,
init_flags=init_flags,
method_flags=method_flags,
@@ -8081,16 +8281,17 @@ def test_torch_tensor_inverse(
)
-# is_complex
+# log
@handle_frontend_method(
class_tree=CLASS_TREE,
init_tree="torch.tensor",
- method_name="is_complex",
+ method_name="log",
dtype_and_x=helpers.dtype_and_values(
- available_dtypes=helpers.get_dtypes("valid"),
+ available_dtypes=helpers.get_dtypes("float"),
+ allow_inf=False,
),
)
-def test_torch_tensor_is_complex(
+def test_torch_log(
dtype_and_x,
frontend_method_data,
init_flags,
@@ -8099,102 +8300,34 @@ def test_torch_tensor_is_complex(
on_device,
backend_fw,
):
- input_dtype, x = dtype_and_x
- helpers.test_frontend_method(
- init_input_dtypes=input_dtype,
- backend_to_test=backend_fw,
- init_all_as_kwargs_np={"data": x[0]},
- method_input_dtypes=input_dtype,
- method_all_as_kwargs_np={},
- frontend_method_data=frontend_method_data,
- init_flags=init_flags,
- method_flags=method_flags,
- frontend=frontend,
- on_device=on_device,
- )
-
-
-@given(
- dtype_x=helpers.dtype_and_values(
- available_dtypes=helpers.get_dtypes("valid", prune_function=False)
- ).filter(lambda x: "bfloat16" not in x[0]),
-)
-def test_torch_tensor_is_cuda(
- dtype_x,
- backend_fw,
-):
- ivy.set_backend(backend_fw)
- _, data = dtype_x
- x = Tensor(data[0])
- x.ivy_array = data[0]
- ivy.utils.assertions.check_equal(
- x.is_cuda, "gpu" in ivy.dev(ivy.array(data[0])), as_array=False
- )
- ivy.previous_backend()
-
-
-@given(
- requires_grad=st.booleans(),
-)
-def test_torch_tensor_is_leaf(requires_grad, backend_fw):
- ivy.set_backend(backend_fw)
- x = Tensor(ivy.array([3.0]), requires_grad=requires_grad)
- ivy.utils.assertions.check_equal(x.is_leaf, True, as_array=False)
- y = x.pow(2)
- ivy.utils.assertions.check_equal(y.is_leaf, not requires_grad, as_array=False)
- z = y.detach()
- ivy.utils.assertions.check_equal(z.is_leaf, True, as_array=False)
- ivy.previous_backend()
-
-
-@given(
- dtype_x=helpers.dtype_and_values(
- available_dtypes=helpers.get_dtypes("valid", prune_function=False)
- ).filter(lambda x: "bfloat16" not in x[0]),
-)
-def test_torch_tensor_is_meta(
- dtype_x,
- backend_fw,
-):
- ivy.set_backend(backend_fw)
- _, data = dtype_x
- x = Tensor(data[0])
- x.ivy_array = data[0]
- ivy.utils.assertions.check_equal(
- x.is_meta, "meta" in ivy.dev(ivy.array(data[0])), as_array=False
- )
- ivy.previous_backend()
-
-
-@given(
- dtype_x=helpers.dtype_and_values(
- available_dtypes=helpers.get_dtypes("valid", prune_function=False)
- ).filter(lambda x: "bfloat16" not in x[0]),
-)
-def test_torch_tensor_is_quantized(
- dtype_x,
- backend_fw,
-):
- ivy.set_backend(backend_fw)
- _, data = dtype_x
- x = Tensor(data[0])
- x.ivy_array = data[0]
- ivy.utils.assertions.check_equal(
- x.is_quantized, "q" in ivy.dtype(ivy.array(data[0])), as_array=False
+ input_dtype, x = dtype_and_x
+ helpers.test_frontend_method(
+ init_input_dtypes=input_dtype,
+ backend_to_test=backend_fw,
+ init_all_as_kwargs_np={
+ "data": x[0],
+ },
+ method_input_dtypes=input_dtype,
+ method_all_as_kwargs_np={},
+ frontend_method_data=frontend_method_data,
+ init_flags=init_flags,
+ method_flags=method_flags,
+ frontend=frontend,
+ on_device=on_device,
)
- ivy.previous_backend()
-# isinf
+# log10
@handle_frontend_method(
class_tree=CLASS_TREE,
init_tree="torch.tensor",
- method_name="isinf",
+ method_name="log10",
dtype_and_x=helpers.dtype_and_values(
- available_dtypes=helpers.get_dtypes("valid"),
+ available_dtypes=helpers.get_dtypes("float"),
+ allow_inf=False,
),
)
-def test_torch_tensor_isinf(
+def test_torch_log10(
dtype_and_x,
frontend_method_data,
init_flags,
@@ -8207,7 +8340,9 @@ def test_torch_tensor_isinf(
helpers.test_frontend_method(
init_input_dtypes=input_dtype,
backend_to_test=backend_fw,
- init_all_as_kwargs_np={"data": x[0]},
+ init_all_as_kwargs_np={
+ "data": x[0],
+ },
method_input_dtypes=input_dtype,
method_all_as_kwargs_np={},
frontend_method_data=frontend_method_data,
@@ -8218,16 +8353,18 @@ def test_torch_tensor_isinf(
)
-# isreal
+# log10_ tests
@handle_frontend_method(
class_tree=CLASS_TREE,
init_tree="torch.tensor",
- method_name="isreal",
+ method_name="log10_",
dtype_and_x=helpers.dtype_and_values(
- available_dtypes=helpers.get_dtypes("numeric"),
+ available_dtypes=helpers.get_dtypes("float"),
+ allow_inf=False,
),
+ test_inplace=st.just(True),
)
-def test_torch_tensor_isreal(
+def test_torch_log10_(
dtype_and_x,
frontend_method_data,
init_flags,
@@ -8240,7 +8377,9 @@ def test_torch_tensor_isreal(
helpers.test_frontend_method(
init_input_dtypes=input_dtype,
backend_to_test=backend_fw,
- init_all_as_kwargs_np={"data": x[0]},
+ init_all_as_kwargs_np={
+ "data": x[0],
+ },
method_input_dtypes=input_dtype,
method_all_as_kwargs_np={},
frontend_method_data=frontend_method_data,
@@ -8251,47 +8390,52 @@ def test_torch_tensor_isreal(
)
-@given(
+@handle_frontend_method(
+ class_tree=CLASS_TREE,
+ init_tree="torch.tensor",
+ method_name="log1p",
dtype_x=helpers.dtype_and_values(
- available_dtypes=helpers.get_dtypes("valid", prune_function=False)
- ).filter(lambda x: "bfloat16" not in x[0]),
+ available_dtypes=helpers.get_dtypes("valid"),
+ max_value=1e37,
+ ),
)
-def test_torch_tensor_ivy_array(
+def test_torch_log1p(
dtype_x,
+ frontend,
+ frontend_method_data,
+ init_flags,
+ method_flags,
+ on_device,
backend_fw,
):
- _, data = dtype_x
- ivy.set_backend(backend_fw)
- x = Tensor(data[0])
- x.ivy_array = data[0]
- ret = helpers.flatten_and_to_np(ret=x.ivy_array.data, backend=backend_fw)
- ret_gt = helpers.flatten_and_to_np(ret=data[0], backend=backend_fw)
- helpers.value_test(
- ret_np_flat=ret,
- ret_np_from_gt_flat=ret_gt,
- backend="torch",
+ input_dtype, x = dtype_x
+ helpers.test_frontend_method(
+ init_input_dtypes=input_dtype,
+ backend_to_test=backend_fw,
+ init_all_as_kwargs_np={"data": x[0]},
+ method_input_dtypes=input_dtype,
+ method_all_as_kwargs_np={},
+ frontend=frontend,
+ frontend_method_data=frontend_method_data,
+ init_flags=init_flags,
+ method_flags=method_flags,
+ on_device=on_device,
)
-# lcm
+# log1p_
@handle_frontend_method(
class_tree=CLASS_TREE,
init_tree="torch.tensor",
- method_name="lcm",
- dtype_and_x=helpers.dtype_and_values(
- available_dtypes=helpers.get_dtypes("integer"),
- num_arrays=2,
- min_value=-100,
- max_value=100,
- min_num_dims=1,
- max_num_dims=3,
- min_dim_size=1,
- max_dim_size=3,
- shared_dtype=True,
+ method_name="log1p_",
+ dtype_x=helpers.dtype_and_values(
+ available_dtypes=helpers.get_dtypes("valid"),
+ max_value=1e37,
),
+ test_inplace=st.just(True),
)
-def test_torch_tensor_lcm(
- dtype_and_x,
+def test_torch_log1p_(
+ dtype_x,
frontend,
frontend_method_data,
init_flags,
@@ -8299,17 +8443,13 @@ def test_torch_tensor_lcm(
on_device,
backend_fw,
):
- input_dtype, x = dtype_and_x
+ input_dtype, x = dtype_x
helpers.test_frontend_method(
init_input_dtypes=input_dtype,
backend_to_test=backend_fw,
- init_all_as_kwargs_np={
- "data": x[0],
- },
+ init_all_as_kwargs_np={"data": x[0]},
method_input_dtypes=input_dtype,
- method_all_as_kwargs_np={
- "other": x[1],
- },
+ method_all_as_kwargs_np={},
frontend=frontend,
frontend_method_data=frontend_method_data,
init_flags=init_flags,
@@ -8318,30 +8458,22 @@ def test_torch_tensor_lcm(
)
-# lcm_
+# log2
@handle_frontend_method(
class_tree=CLASS_TREE,
init_tree="torch.tensor",
- method_name="lcm_",
+ method_name="log2",
dtype_and_x=helpers.dtype_and_values(
- available_dtypes=helpers.get_dtypes("integer"),
- num_arrays=2,
- min_value=-100,
- max_value=100,
- min_num_dims=1,
- max_num_dims=3,
- min_dim_size=1,
- max_dim_size=3,
- shared_dtype=True,
+ available_dtypes=helpers.get_dtypes("float"),
+ allow_inf=False,
),
- test_inplace=st.just(True),
)
-def test_torch_tensor_lcm_(
+def test_torch_log2(
dtype_and_x,
- frontend,
frontend_method_data,
init_flags,
method_flags,
+ frontend,
on_device,
backend_fw,
):
@@ -8353,31 +8485,27 @@ def test_torch_tensor_lcm_(
"data": x[0],
},
method_input_dtypes=input_dtype,
- method_all_as_kwargs_np={
- "other": x[1],
- },
- frontend=frontend,
+ method_all_as_kwargs_np={},
frontend_method_data=frontend_method_data,
init_flags=init_flags,
method_flags=method_flags,
+ frontend=frontend,
on_device=on_device,
)
-# less
+# log2_
@handle_frontend_method(
class_tree=CLASS_TREE,
init_tree="torch.tensor",
- method_name="less",
+ method_name="log2_",
dtype_and_x=helpers.dtype_and_values(
available_dtypes=helpers.get_dtypes("valid"),
- num_arrays=2,
- min_value=-1e04,
- max_value=1e04,
allow_inf=False,
),
+ test_inplace=st.just(True),
)
-def test_torch_tensor_less(
+def test_torch_log2_(
dtype_and_x,
frontend_method_data,
init_flags,
@@ -8394,9 +8522,7 @@ def test_torch_tensor_less(
"data": x[0],
},
method_input_dtypes=input_dtype,
- method_all_as_kwargs_np={
- "other": x[1],
- },
+ method_all_as_kwargs_np={},
frontend_method_data=frontend_method_data,
init_flags=init_flags,
method_flags=method_flags,
@@ -8405,21 +8531,18 @@ def test_torch_tensor_less(
)
-# less_
+# log_
@handle_frontend_method(
class_tree=CLASS_TREE,
init_tree="torch.tensor",
- method_name="less_",
+ method_name="log_",
dtype_and_x=helpers.dtype_and_values(
- available_dtypes=helpers.get_dtypes("valid"),
- num_arrays=2,
- min_value=-1e04,
- max_value=1e04,
+ available_dtypes=helpers.get_dtypes("float"),
allow_inf=False,
),
test_inplace=st.just(True),
)
-def test_torch_tensor_less_(
+def test_torch_log_(
dtype_and_x,
frontend_method_data,
init_flags,
@@ -8436,9 +8559,7 @@ def test_torch_tensor_less_(
"data": x[0],
},
method_input_dtypes=input_dtype,
- method_all_as_kwargs_np={
- "other": x[1],
- },
+ method_all_as_kwargs_np={},
frontend_method_data=frontend_method_data,
init_flags=init_flags,
method_flags=method_flags,
@@ -8447,20 +8568,21 @@ def test_torch_tensor_less_(
)
-# less_equal
+# logaddexp
@handle_frontend_method(
class_tree=CLASS_TREE,
init_tree="torch.tensor",
- method_name="less_equal",
+ method_name="logaddexp",
dtype_and_x=helpers.dtype_and_values(
- available_dtypes=helpers.get_dtypes("valid"),
+ available_dtypes=helpers.get_dtypes("float"),
num_arrays=2,
- min_value=-1e04,
- max_value=1e04,
- allow_inf=False,
+ min_num_dims=1,
+ min_value=-100,
+ max_value=100,
+ shared_dtype=True,
),
)
-def test_torch_tensor_less_equal(
+def test_torch_logaddexp(
dtype_and_x,
frontend_method_data,
init_flags,
@@ -8488,21 +8610,14 @@ def test_torch_tensor_less_equal(
)
-# less_equal_
+# logdet
@handle_frontend_method(
class_tree=CLASS_TREE,
init_tree="torch.tensor",
- method_name="less_equal_",
- dtype_and_x=helpers.dtype_and_values(
- available_dtypes=helpers.get_dtypes("valid"),
- num_arrays=2,
- min_value=-1e04,
- max_value=1e04,
- allow_inf=False,
- ),
- test_inplace=st.just(True),
+ method_name="logdet",
+ dtype_and_x=_get_dtype_and_matrix(square=True, batch=True),
)
-def test_torch_tensor_less_equal_(
+def test_torch_logdet(
dtype_and_x,
frontend_method_data,
init_flags,
@@ -8512,16 +8627,16 @@ def test_torch_tensor_less_equal_(
backend_fw,
):
input_dtype, x = dtype_and_x
+ dtype, x = dtype_and_x
+ x = np.matmul(x.T, x) + np.identity(x.shape[0])
helpers.test_frontend_method(
init_input_dtypes=input_dtype,
backend_to_test=backend_fw,
init_all_as_kwargs_np={
- "data": x[0],
+ "data": x,
},
method_input_dtypes=input_dtype,
- method_all_as_kwargs_np={
- "other": x[1],
- },
+ method_all_as_kwargs_np={},
frontend_method_data=frontend_method_data,
init_flags=init_flags,
method_flags=method_flags,
@@ -8530,17 +8645,17 @@ def test_torch_tensor_less_equal_(
)
-# log
+# logical_and
@handle_frontend_method(
class_tree=CLASS_TREE,
init_tree="torch.tensor",
- method_name="log",
+ method_name="logical_and",
dtype_and_x=helpers.dtype_and_values(
- available_dtypes=helpers.get_dtypes("float"),
- allow_inf=False,
+ available_dtypes=helpers.get_dtypes("valid"),
+ num_arrays=2,
),
)
-def test_torch_tensor_log(
+def test_torch_logical_and(
dtype_and_x,
frontend_method_data,
init_flags,
@@ -8557,7 +8672,9 @@ def test_torch_tensor_log(
"data": x[0],
},
method_input_dtypes=input_dtype,
- method_all_as_kwargs_np={},
+ method_all_as_kwargs_np={
+ "other": x[1],
+ },
frontend_method_data=frontend_method_data,
init_flags=init_flags,
method_flags=method_flags,
@@ -8566,17 +8683,16 @@ def test_torch_tensor_log(
)
-# log10
+# logical_not
@handle_frontend_method(
class_tree=CLASS_TREE,
init_tree="torch.tensor",
- method_name="log10",
+ method_name="logical_not",
dtype_and_x=helpers.dtype_and_values(
- available_dtypes=helpers.get_dtypes("float"),
- allow_inf=False,
+ available_dtypes=helpers.get_dtypes("valid"), num_arrays=1
),
)
-def test_torch_tensor_log10(
+def test_torch_logical_not(
dtype_and_x,
frontend_method_data,
init_flags,
@@ -8602,18 +8718,19 @@ def test_torch_tensor_log10(
)
-# log10_ tests
+# logical_not_
@handle_frontend_method(
class_tree=CLASS_TREE,
init_tree="torch.tensor",
- method_name="log10_",
+ method_name="logical_not_",
dtype_and_x=helpers.dtype_and_values(
- available_dtypes=helpers.get_dtypes("float"),
- allow_inf=False,
+ available_dtypes=helpers.get_dtypes("valid"),
+ num_arrays=1,
+ large_abs_safety_factor=12,
),
test_inplace=st.just(True),
)
-def test_torch_tensor_log10_(
+def test_torch_logical_not_(
dtype_and_x,
frontend_method_data,
init_flags,
@@ -8639,85 +8756,92 @@ def test_torch_tensor_log10_(
)
+# logical_or
@handle_frontend_method(
class_tree=CLASS_TREE,
init_tree="torch.tensor",
- method_name="log1p",
- dtype_x=helpers.dtype_and_values(
+ method_name="logical_or",
+ dtype_and_x=helpers.dtype_and_values(
available_dtypes=helpers.get_dtypes("valid"),
- max_value=1e37,
+ num_arrays=2,
),
)
-def test_torch_tensor_log1p(
- dtype_x,
- frontend,
+def test_torch_logical_or(
+ dtype_and_x,
frontend_method_data,
init_flags,
method_flags,
+ frontend,
on_device,
backend_fw,
):
- input_dtype, x = dtype_x
+ input_dtype, x = dtype_and_x
helpers.test_frontend_method(
init_input_dtypes=input_dtype,
backend_to_test=backend_fw,
- init_all_as_kwargs_np={"data": x[0]},
+ init_all_as_kwargs_np={
+ "data": x[0],
+ },
method_input_dtypes=input_dtype,
- method_all_as_kwargs_np={},
- frontend=frontend,
+ method_all_as_kwargs_np={
+ "other": x[1],
+ },
frontend_method_data=frontend_method_data,
init_flags=init_flags,
method_flags=method_flags,
+ frontend=frontend,
on_device=on_device,
)
-# log1p_
+# logit
@handle_frontend_method(
class_tree=CLASS_TREE,
init_tree="torch.tensor",
- method_name="log1p_",
- dtype_x=helpers.dtype_and_values(
- available_dtypes=helpers.get_dtypes("valid"),
- max_value=1e37,
+ method_name="logit",
+ dtype_and_x=helpers.dtype_and_values(
+ available_dtypes=helpers.get_dtypes("float"),
+ allow_inf=False,
+ min_num_dims=1,
+ min_dim_size=1,
),
- test_inplace=st.just(True),
)
-def test_torch_tensor_log1p_(
- dtype_x,
- frontend,
+def test_torch_logit(
+ dtype_and_x,
frontend_method_data,
init_flags,
method_flags,
+ frontend,
on_device,
backend_fw,
):
- input_dtype, x = dtype_x
+ input_dtype, x = dtype_and_x
helpers.test_frontend_method(
init_input_dtypes=input_dtype,
backend_to_test=backend_fw,
- init_all_as_kwargs_np={"data": x[0]},
+ init_all_as_kwargs_np={
+ "data": x[0],
+ },
method_input_dtypes=input_dtype,
method_all_as_kwargs_np={},
- frontend=frontend,
frontend_method_data=frontend_method_data,
init_flags=init_flags,
method_flags=method_flags,
+ frontend=frontend,
on_device=on_device,
)
-# log2
+# long
@handle_frontend_method(
class_tree=CLASS_TREE,
init_tree="torch.tensor",
- method_name="log2",
+ method_name="long",
dtype_and_x=helpers.dtype_and_values(
- available_dtypes=helpers.get_dtypes("float"),
- allow_inf=False,
+ available_dtypes=helpers.get_dtypes("integer"),
),
)
-def test_torch_tensor_log2(
+def test_torch_long(
dtype_and_x,
frontend_method_data,
init_flags,
@@ -8743,19 +8867,15 @@ def test_torch_tensor_log2(
)
-# log2_
+# masked_fill
@handle_frontend_method(
class_tree=CLASS_TREE,
init_tree="torch.tensor",
- method_name="log2_",
- dtype_and_x=helpers.dtype_and_values(
- available_dtypes=helpers.get_dtypes("valid"),
- allow_inf=False,
- ),
- test_inplace=st.just(True),
+ method_name="masked_fill",
+ x_mask_val=_masked_fill_helper(),
)
-def test_torch_tensor_log2_(
- dtype_and_x,
+def test_torch_masked_fill(
+ x_mask_val,
frontend_method_data,
init_flags,
method_flags,
@@ -8763,15 +8883,18 @@ def test_torch_tensor_log2_(
on_device,
backend_fw,
):
- input_dtype, x = dtype_and_x
+ dtype, x, mask, val = x_mask_val
helpers.test_frontend_method(
- init_input_dtypes=input_dtype,
+ init_input_dtypes=[dtype],
backend_to_test=backend_fw,
init_all_as_kwargs_np={
- "data": x[0],
+ "data": x,
+ },
+ method_input_dtypes=["bool", dtype],
+ method_all_as_kwargs_np={
+ "mask": mask,
+ "value": val,
},
- method_input_dtypes=input_dtype,
- method_all_as_kwargs_np={},
frontend_method_data=frontend_method_data,
init_flags=init_flags,
method_flags=method_flags,
@@ -8780,19 +8903,15 @@ def test_torch_tensor_log2_(
)
-# log_
+# matmul
@handle_frontend_method(
class_tree=CLASS_TREE,
init_tree="torch.tensor",
- method_name="log_",
- dtype_and_x=helpers.dtype_and_values(
- available_dtypes=helpers.get_dtypes("float"),
- allow_inf=False,
- ),
- test_inplace=st.just(True),
+ method_name="matmul",
+ dtype_tensor1_tensor2=_get_dtype_and_multiplicative_matrices(),
)
-def test_torch_tensor_log_(
- dtype_and_x,
+def test_torch_matmul(
+ dtype_tensor1_tensor2,
frontend_method_data,
init_flags,
method_flags,
@@ -8800,15 +8919,15 @@ def test_torch_tensor_log_(
on_device,
backend_fw,
):
- input_dtype, x = dtype_and_x
+ dtype, tensor1, tensor2 = dtype_tensor1_tensor2
helpers.test_frontend_method(
- init_input_dtypes=input_dtype,
+ init_input_dtypes=dtype,
backend_to_test=backend_fw,
init_all_as_kwargs_np={
- "data": x[0],
+ "data": tensor1,
},
- method_input_dtypes=input_dtype,
- method_all_as_kwargs_np={},
+ method_input_dtypes=dtype,
+ method_all_as_kwargs_np={"other": tensor2},
frontend_method_data=frontend_method_data,
init_flags=init_flags,
method_flags=method_flags,
@@ -8817,22 +8936,17 @@ def test_torch_tensor_log_(
)
-# logaddexp
+# matrix_power
@handle_frontend_method(
class_tree=CLASS_TREE,
init_tree="torch.tensor",
- method_name="logaddexp",
- dtype_and_x=helpers.dtype_and_values(
- available_dtypes=helpers.get_dtypes("float"),
- num_arrays=2,
- min_num_dims=1,
- min_value=-100,
- max_value=100,
- shared_dtype=True,
- ),
+ method_name="matrix_power",
+ dtype_x=_get_dtype_and_matrix(square=True, invertible=True),
+ n=helpers.ints(min_value=2, max_value=5),
)
-def test_torch_tensor_logaddexp(
- dtype_and_x,
+def test_torch_matrix_power(
+ dtype_x,
+ n,
frontend_method_data,
init_flags,
method_flags,
@@ -8840,7 +8954,7 @@ def test_torch_tensor_logaddexp(
on_device,
backend_fw,
):
- input_dtype, x = dtype_and_x
+ input_dtype, x = dtype_x
helpers.test_frontend_method(
init_input_dtypes=input_dtype,
backend_to_test=backend_fw,
@@ -8849,7 +8963,7 @@ def test_torch_tensor_logaddexp(
},
method_input_dtypes=input_dtype,
method_all_as_kwargs_np={
- "other": x[1],
+ "n": n,
},
frontend_method_data=frontend_method_data,
init_flags=init_flags,
@@ -8859,15 +8973,17 @@ def test_torch_tensor_logaddexp(
)
-# logdet
+# max
@handle_frontend_method(
class_tree=CLASS_TREE,
init_tree="torch.tensor",
- method_name="logdet",
- dtype_and_x=_get_dtype_and_matrix(square=True, batch=True),
+ method_name="max",
+ dtype_x=helpers.dtype_and_values(
+ available_dtypes=helpers.get_dtypes("float"),
+ ),
)
-def test_torch_tensor_logdet(
- dtype_and_x,
+def test_torch_max(
+ dtype_x,
frontend_method_data,
init_flags,
method_flags,
@@ -8875,14 +8991,12 @@ def test_torch_tensor_logdet(
on_device,
backend_fw,
):
- input_dtype, x = dtype_and_x
- dtype, x = dtype_and_x
- x = np.matmul(x.T, x) + np.identity(x.shape[0])
+ input_dtype, x = dtype_x
helpers.test_frontend_method(
init_input_dtypes=input_dtype,
backend_to_test=backend_fw,
init_all_as_kwargs_np={
- "data": x,
+ "data": x[0],
},
method_input_dtypes=input_dtype,
method_all_as_kwargs_np={},
@@ -8894,17 +9008,17 @@ def test_torch_tensor_logdet(
)
-# logical_and
+# maximum
@handle_frontend_method(
class_tree=CLASS_TREE,
init_tree="torch.tensor",
- method_name="logical_and",
+ method_name="maximum",
dtype_and_x=helpers.dtype_and_values(
available_dtypes=helpers.get_dtypes("valid"),
num_arrays=2,
),
)
-def test_torch_tensor_logical_and(
+def test_torch_maximum(
dtype_and_x,
frontend_method_data,
init_flags,
@@ -8932,25 +9046,29 @@ def test_torch_tensor_logical_and(
)
-# logical_not
+# mean
@handle_frontend_method(
class_tree=CLASS_TREE,
init_tree="torch.tensor",
- method_name="logical_not",
- dtype_and_x=helpers.dtype_and_values(
- available_dtypes=helpers.get_dtypes("valid"), num_arrays=1
+ method_name="mean",
+ dtype_and_x=_statistical_dtype_values(
+ function="mean",
+ min_value=-1e04,
+ max_value=1e04,
),
+ keepdims=st.booleans(),
)
-def test_torch_tensor_logical_not(
+def test_torch_mean(
dtype_and_x,
+ keepdims,
+ frontend,
frontend_method_data,
init_flags,
method_flags,
- frontend,
on_device,
backend_fw,
):
- input_dtype, x = dtype_and_x
+ input_dtype, x, axis = dtype_and_x
helpers.test_frontend_method(
init_input_dtypes=input_dtype,
backend_to_test=backend_fw,
@@ -8958,7 +9076,10 @@ def test_torch_tensor_logical_not(
"data": x[0],
},
method_input_dtypes=input_dtype,
- method_all_as_kwargs_np={},
+ method_all_as_kwargs_np={
+ "dim": axis,
+ "keepdim": keepdims,
+ },
frontend_method_data=frontend_method_data,
init_flags=init_flags,
method_flags=method_flags,
@@ -8967,28 +9088,68 @@ def test_torch_tensor_logical_not(
)
-# logical_not_
+# median
@handle_frontend_method(
class_tree=CLASS_TREE,
init_tree="torch.tensor",
- method_name="logical_not_",
- dtype_and_x=helpers.dtype_and_values(
- available_dtypes=helpers.get_dtypes("valid"),
- num_arrays=1,
- large_abs_safety_factor=12,
+ method_name="median",
+ dtype_input_axis=helpers.dtype_values_axis(
+ available_dtypes=helpers.get_dtypes("float"),
+ min_num_dims=1,
+ valid_axis=True,
+ force_int_axis=True,
),
- test_inplace=st.just(True),
+ keepdim=st.booleans(),
)
-def test_torch_tensor_logical_not_(
- dtype_and_x,
+def test_torch_median(
+ dtype_input_axis,
+ keepdim,
+ frontend,
frontend_method_data,
init_flags,
method_flags,
+ on_device,
+ backend_fw,
+):
+ input_dtype, x, axis = dtype_input_axis
+ helpers.test_frontend_method(
+ init_input_dtypes=input_dtype,
+ backend_to_test=backend_fw,
+ init_all_as_kwargs_np={
+ "data": x[0],
+ },
+ method_input_dtypes=input_dtype,
+ method_all_as_kwargs_np={
+ "dim": axis,
+ "keepdim": keepdim,
+ },
+ frontend_method_data=frontend_method_data,
+ init_flags=init_flags,
+ method_flags=method_flags,
+ frontend=frontend,
+ on_device=on_device,
+ )
+
+
+# min
+@handle_frontend_method(
+ class_tree=CLASS_TREE,
+ init_tree="torch.tensor",
+ method_name="min",
+ dtype_x=helpers.dtype_and_values(
+ available_dtypes=helpers.get_dtypes("float"),
+ ),
+)
+def test_torch_min(
+ dtype_x,
frontend,
+ frontend_method_data,
+ init_flags,
+ method_flags,
on_device,
backend_fw,
):
- input_dtype, x = dtype_and_x
+ input_dtype, x = dtype_x
helpers.test_frontend_method(
init_input_dtypes=input_dtype,
backend_to_test=backend_fw,
@@ -9005,17 +9166,17 @@ def test_torch_tensor_logical_not_(
)
-# logical_or
+# minimum
@handle_frontend_method(
class_tree=CLASS_TREE,
init_tree="torch.tensor",
- method_name="logical_or",
+ method_name="minimum",
dtype_and_x=helpers.dtype_and_values(
available_dtypes=helpers.get_dtypes("valid"),
num_arrays=2,
),
)
-def test_torch_tensor_logical_or(
+def test_torch_minimum(
dtype_and_x,
frontend_method_data,
init_flags,
@@ -9043,20 +9204,15 @@ def test_torch_tensor_logical_or(
)
-# logit
+# mm
@handle_frontend_method(
class_tree=CLASS_TREE,
init_tree="torch.tensor",
- method_name="logit",
- dtype_and_x=helpers.dtype_and_values(
- available_dtypes=helpers.get_dtypes("float"),
- allow_inf=False,
- min_num_dims=1,
- min_dim_size=1,
- ),
+ method_name="mm",
+ dtype_xy=_get_dtype_input_and_matrices(),
)
-def test_torch_tensor_logit(
- dtype_and_x,
+def test_torch_mm(
+ dtype_xy,
frontend_method_data,
init_flags,
method_flags,
@@ -9064,15 +9220,17 @@ def test_torch_tensor_logit(
on_device,
backend_fw,
):
- input_dtype, x = dtype_and_x
+ dtype, x, y = dtype_xy
helpers.test_frontend_method(
- init_input_dtypes=input_dtype,
+ init_input_dtypes=dtype,
backend_to_test=backend_fw,
init_all_as_kwargs_np={
- "data": x[0],
+ "data": x,
+ },
+ method_input_dtypes=dtype,
+ method_all_as_kwargs_np={
+ "mat2": y,
},
- method_input_dtypes=input_dtype,
- method_all_as_kwargs_np={},
frontend_method_data=frontend_method_data,
init_flags=init_flags,
method_flags=method_flags,
@@ -9081,50 +9239,96 @@ def test_torch_tensor_logit(
)
-# long
@handle_frontend_method(
class_tree=CLASS_TREE,
init_tree="torch.tensor",
- method_name="long",
- dtype_and_x=helpers.dtype_and_values(
- available_dtypes=helpers.get_dtypes("integer"),
+ method_name="movedim",
+ dtype_and_input=helpers.dtype_and_values(
+ available_dtypes=helpers.get_dtypes("float"),
+ min_value=-100,
+ max_value=100,
+ shape=st.shared(
+ helpers.get_shape(
+ min_num_dims=1,
+ max_num_dims=3,
+ min_dim_size=1,
+ max_dim_size=3,
+ ),
+ key="a_s_d",
+ ),
+ ),
+ source=helpers.get_axis(
+ allow_none=False,
+ unique=True,
+ shape=st.shared(
+ helpers.get_shape(
+ min_num_dims=1,
+ max_num_dims=3,
+ min_dim_size=1,
+ max_dim_size=3,
+ ),
+ key="a_s_d",
+ ),
+ min_size=1,
+ force_int=True,
+ ),
+ destination=helpers.get_axis(
+ allow_none=False,
+ unique=True,
+ shape=st.shared(
+ helpers.get_shape(
+ min_num_dims=1,
+ max_num_dims=3,
+ min_dim_size=1,
+ max_dim_size=3,
+ ),
+ key="a_s_d",
+ ),
+ min_size=1,
+ force_int=True,
),
)
-def test_torch_tensor_long(
- dtype_and_x,
+def test_torch_movedim(
+ dtype_and_input,
+ source,
+ destination,
+ frontend,
frontend_method_data,
init_flags,
method_flags,
- frontend,
on_device,
backend_fw,
):
- input_dtype, x = dtype_and_x
+ input_dtype, value = dtype_and_input
helpers.test_frontend_method(
init_input_dtypes=input_dtype,
backend_to_test=backend_fw,
- init_all_as_kwargs_np={
- "data": x[0],
- },
+ init_all_as_kwargs_np={"data": value[0]},
method_input_dtypes=input_dtype,
- method_all_as_kwargs_np={},
+ method_all_as_kwargs_np={
+ "source": source,
+ "destination": destination,
+ },
+ frontend=frontend,
frontend_method_data=frontend_method_data,
init_flags=init_flags,
method_flags=method_flags,
- frontend=frontend,
on_device=on_device,
)
-# masked_fill
+# msort
@handle_frontend_method(
class_tree=CLASS_TREE,
init_tree="torch.tensor",
- method_name="masked_fill",
- x_mask_val=_masked_fill_helper(),
+ method_name="msort",
+ dtype_value=helpers.dtype_and_values(
+ available_dtypes=["float32", "float64", "int32", "int64"],
+ shape=st.shared(helpers.get_shape(min_num_dims=1), key="shape"),
+ ),
)
-def test_torch_tensor_masked_fill(
- x_mask_val,
+def test_torch_msort(
+ dtype_value,
frontend_method_data,
init_flags,
method_flags,
@@ -9132,18 +9336,15 @@ def test_torch_tensor_masked_fill(
on_device,
backend_fw,
):
- dtype, x, mask, val = x_mask_val
+ input_dtype, x = dtype_value
helpers.test_frontend_method(
- init_input_dtypes=[dtype],
+ init_input_dtypes=input_dtype,
backend_to_test=backend_fw,
init_all_as_kwargs_np={
- "data": x,
- },
- method_input_dtypes=["bool", dtype],
- method_all_as_kwargs_np={
- "mask": mask,
- "value": val,
+ "data": x[0],
},
+ method_input_dtypes=input_dtype,
+ method_all_as_kwargs_np={},
frontend_method_data=frontend_method_data,
init_flags=init_flags,
method_flags=method_flags,
@@ -9152,15 +9353,18 @@ def test_torch_tensor_masked_fill(
)
-# matmul
+# mul
@handle_frontend_method(
class_tree=CLASS_TREE,
init_tree="torch.tensor",
- method_name="matmul",
- dtype_tensor1_tensor2=_get_dtype_and_multiplicative_matrices(),
+ method_name="mul",
+ dtype_and_x=helpers.dtype_and_values(
+ available_dtypes=helpers.get_dtypes("numeric"),
+ num_arrays=2,
+ ),
)
-def test_torch_tensor_matmul(
- dtype_tensor1_tensor2,
+def test_torch_mul(
+ dtype_and_x,
frontend_method_data,
init_flags,
method_flags,
@@ -9168,15 +9372,17 @@ def test_torch_tensor_matmul(
on_device,
backend_fw,
):
- dtype, tensor1, tensor2 = dtype_tensor1_tensor2
+ input_dtype, x = dtype_and_x
helpers.test_frontend_method(
- init_input_dtypes=dtype,
+ init_input_dtypes=input_dtype,
backend_to_test=backend_fw,
init_all_as_kwargs_np={
- "data": tensor1,
+ "data": x[0],
+ },
+ method_input_dtypes=input_dtype,
+ method_all_as_kwargs_np={
+ "other": x[1],
},
- method_input_dtypes=dtype,
- method_all_as_kwargs_np={"other": tensor2},
frontend_method_data=frontend_method_data,
init_flags=init_flags,
method_flags=method_flags,
@@ -9185,17 +9391,20 @@ def test_torch_tensor_matmul(
)
-# max
+# mul_
@handle_frontend_method(
class_tree=CLASS_TREE,
init_tree="torch.tensor",
- method_name="max",
- dtype_x=helpers.dtype_and_values(
- available_dtypes=helpers.get_dtypes("float"),
+ method_name="mul_",
+ dtype_and_x=helpers.dtype_and_values(
+ available_dtypes=helpers.get_dtypes("numeric"),
+ num_arrays=2,
+ shared_dtype=True,
),
+ test_inplace=st.just(True),
)
-def test_torch_tensor_max(
- dtype_x,
+def test_torch_mul_(
+ dtype_and_x,
frontend_method_data,
init_flags,
method_flags,
@@ -9203,7 +9412,7 @@ def test_torch_tensor_max(
on_device,
backend_fw,
):
- input_dtype, x = dtype_x
+ input_dtype, x = dtype_and_x
helpers.test_frontend_method(
init_input_dtypes=input_dtype,
backend_to_test=backend_fw,
@@ -9211,7 +9420,9 @@ def test_torch_tensor_max(
"data": x[0],
},
method_input_dtypes=input_dtype,
- method_all_as_kwargs_np={},
+ method_all_as_kwargs_np={
+ "other": x[1],
+ },
frontend_method_data=frontend_method_data,
init_flags=init_flags,
method_flags=method_flags,
@@ -9220,17 +9431,17 @@ def test_torch_tensor_max(
)
-# maximum
+# multiply
@handle_frontend_method(
class_tree=CLASS_TREE,
init_tree="torch.tensor",
- method_name="maximum",
+ method_name="multiply",
dtype_and_x=helpers.dtype_and_values(
- available_dtypes=helpers.get_dtypes("valid"),
+ available_dtypes=helpers.get_dtypes("numeric"),
num_arrays=2,
),
)
-def test_torch_tensor_maximum(
+def test_torch_multiply(
dtype_and_x,
frontend_method_data,
init_flags,
@@ -9258,29 +9469,27 @@ def test_torch_tensor_maximum(
)
-# mean
+# multiply_
@handle_frontend_method(
class_tree=CLASS_TREE,
init_tree="torch.tensor",
- method_name="mean",
- dtype_and_x=_statistical_dtype_values(
- function="mean",
- min_value=-1e04,
- max_value=1e04,
+ method_name="multiply_",
+ dtype_and_x=helpers.dtype_and_values(
+ available_dtypes=helpers.get_dtypes("numeric"),
+ num_arrays=2,
),
- keepdims=st.booleans(),
+ test_inplace=st.just(True),
)
-def test_torch_tensor_mean(
+def test_torch_multiply_(
dtype_and_x,
- keepdims,
- frontend,
frontend_method_data,
init_flags,
method_flags,
+ frontend,
on_device,
backend_fw,
):
- input_dtype, x, axis = dtype_and_x
+ input_dtype, x = dtype_and_x
helpers.test_frontend_method(
init_input_dtypes=input_dtype,
backend_to_test=backend_fw,
@@ -9289,8 +9498,7 @@ def test_torch_tensor_mean(
},
method_input_dtypes=input_dtype,
method_all_as_kwargs_np={
- "dim": axis,
- "keepdim": keepdims,
+ "other": x[1],
},
frontend_method_data=frontend_method_data,
init_flags=init_flags,
@@ -9300,22 +9508,19 @@ def test_torch_tensor_mean(
)
-# median
+# nanmean
@handle_frontend_method(
class_tree=CLASS_TREE,
init_tree="torch.tensor",
- method_name="median",
- dtype_input_axis=helpers.dtype_values_axis(
+ method_name="nanmean",
+ dtype_x=helpers.dtype_and_values(
available_dtypes=helpers.get_dtypes("float"),
- min_num_dims=1,
- valid_axis=True,
- force_int_axis=True,
+ min_value=-1e04,
+ max_value=1e04,
),
- keepdim=st.booleans(),
)
-def test_torch_tensor_median(
- dtype_input_axis,
- keepdim,
+def test_torch_nanmean(
+ dtype_x,
frontend,
frontend_method_data,
init_flags,
@@ -9323,7 +9528,7 @@ def test_torch_tensor_median(
on_device,
backend_fw,
):
- input_dtype, x, axis = dtype_input_axis
+ input_dtype, x = dtype_x
helpers.test_frontend_method(
init_input_dtypes=input_dtype,
backend_to_test=backend_fw,
@@ -9331,10 +9536,7 @@ def test_torch_tensor_median(
"data": x[0],
},
method_input_dtypes=input_dtype,
- method_all_as_kwargs_np={
- "dim": axis,
- "keepdim": keepdim,
- },
+ method_all_as_kwargs_np={},
frontend_method_data=frontend_method_data,
init_flags=init_flags,
method_flags=method_flags,
@@ -9343,16 +9545,18 @@ def test_torch_tensor_median(
)
-# min
+# nansum
@handle_frontend_method(
class_tree=CLASS_TREE,
init_tree="torch.tensor",
- method_name="min",
+ method_name="nansum",
dtype_x=helpers.dtype_and_values(
available_dtypes=helpers.get_dtypes("float"),
+ min_value=-1e04,
+ max_value=1e04,
),
)
-def test_torch_tensor_min(
+def test_torch_nansum(
dtype_x,
frontend,
frontend_method_data,
@@ -9378,53 +9582,65 @@ def test_torch_tensor_min(
)
-# minimum
@handle_frontend_method(
class_tree=CLASS_TREE,
init_tree="torch.tensor",
- method_name="minimum",
- dtype_and_x=helpers.dtype_and_values(
- available_dtypes=helpers.get_dtypes("valid"),
- num_arrays=2,
- ),
+ method_name="narrow",
+ dtype_input_dim_start_length=_dtype_input_dim_start_length(),
)
-def test_torch_tensor_minimum(
- dtype_and_x,
+def test_torch_narrow(
+ dtype_input_dim_start_length,
+ frontend,
frontend_method_data,
init_flags,
method_flags,
- frontend,
on_device,
backend_fw,
):
- input_dtype, x = dtype_and_x
+ (input_dtype, x, dim, start, length) = dtype_input_dim_start_length
helpers.test_frontend_method(
init_input_dtypes=input_dtype,
backend_to_test=backend_fw,
- init_all_as_kwargs_np={
- "data": x[0],
- },
+ init_all_as_kwargs_np={"data": x[0]},
method_input_dtypes=input_dtype,
method_all_as_kwargs_np={
- "other": x[1],
+ "dim": dim,
+ "start": start,
+ "length": length,
},
+ frontend=frontend,
frontend_method_data=frontend_method_data,
init_flags=init_flags,
method_flags=method_flags,
- frontend=frontend,
on_device=on_device,
)
-# mm
+@given(
+ dtype_x=helpers.dtype_and_values(
+ available_dtypes=helpers.get_dtypes("valid", prune_function=False),
+ ret_shape=True,
+ ).filter(lambda x: "bfloat16" not in x[0]),
+)
+def test_torch_ndim(dtype_x, backend_fw):
+ ivy.set_backend(backend_fw)
+ dtype, data, shape = dtype_x
+ x = Tensor(data[0])
+ ivy.utils.assertions.check_equal(x.ndim, data[0].ndim, as_array=False)
+ ivy.previous_backend()
+
+
+# ndimension
@handle_frontend_method(
class_tree=CLASS_TREE,
init_tree="torch.tensor",
- method_name="mm",
- dtype_xy=_get_dtype_input_and_matrices(),
+ method_name="ndimension",
+ dtype_and_x=helpers.dtype_and_values(
+ available_dtypes=helpers.get_dtypes("numeric"),
+ ),
)
-def test_torch_tensor_mm(
- dtype_xy,
+def test_torch_ndimension(
+ dtype_and_x,
frontend_method_data,
init_flags,
method_flags,
@@ -9432,123 +9648,86 @@ def test_torch_tensor_mm(
on_device,
backend_fw,
):
- dtype, x, y = dtype_xy
+ input_dtype, x = dtype_and_x
helpers.test_frontend_method(
- init_input_dtypes=dtype,
+ init_input_dtypes=input_dtype,
backend_to_test=backend_fw,
init_all_as_kwargs_np={
- "data": x,
- },
- method_input_dtypes=dtype,
- method_all_as_kwargs_np={
- "mat2": y,
+ "data": x[0],
},
+ method_input_dtypes=[],
+ method_all_as_kwargs_np={},
frontend_method_data=frontend_method_data,
init_flags=init_flags,
- method_flags=method_flags,
- frontend=frontend,
- on_device=on_device,
- )
-
-
-@handle_frontend_method(
- class_tree=CLASS_TREE,
- init_tree="torch.tensor",
- method_name="movedim",
- dtype_and_input=helpers.dtype_and_values(
- available_dtypes=helpers.get_dtypes("float"),
- min_value=-100,
- max_value=100,
- shape=st.shared(
- helpers.get_shape(
- min_num_dims=1,
- max_num_dims=3,
- min_dim_size=1,
- max_dim_size=3,
- ),
- key="a_s_d",
- ),
- ),
- source=helpers.get_axis(
- allow_none=False,
- unique=True,
- shape=st.shared(
- helpers.get_shape(
- min_num_dims=1,
- max_num_dims=3,
- min_dim_size=1,
- max_dim_size=3,
- ),
- key="a_s_d",
- ),
- min_size=1,
- force_int=True,
- ),
- destination=helpers.get_axis(
- allow_none=False,
- unique=True,
- shape=st.shared(
- helpers.get_shape(
- min_num_dims=1,
- max_num_dims=3,
- min_dim_size=1,
- max_dim_size=3,
- ),
- key="a_s_d",
- ),
- min_size=1,
- force_int=True,
+ method_flags=method_flags,
+ frontend=frontend,
+ on_device=on_device,
+ )
+
+
+# ne
+@handle_frontend_method(
+ class_tree=CLASS_TREE,
+ init_tree="torch.tensor",
+ method_name="ne",
+ dtype_and_x=helpers.dtype_and_values(
+ available_dtypes=helpers.get_dtypes("float"),
+ num_arrays=2,
+ min_value=-1e04,
+ max_value=1e04,
+ allow_inf=False,
),
)
-def test_torch_tensor_movedim(
- dtype_and_input,
- source,
- destination,
- frontend,
+def test_torch_ne(
+ dtype_and_x,
frontend_method_data,
init_flags,
method_flags,
+ frontend,
on_device,
backend_fw,
):
- input_dtype, value = dtype_and_input
+ input_dtype, x = dtype_and_x
helpers.test_frontend_method(
init_input_dtypes=input_dtype,
backend_to_test=backend_fw,
- init_all_as_kwargs_np={"data": value[0]},
+ init_all_as_kwargs_np={
+ "data": x[0],
+ },
method_input_dtypes=input_dtype,
method_all_as_kwargs_np={
- "source": source,
- "destination": destination,
+ "other": x[1],
},
- frontend=frontend,
frontend_method_data=frontend_method_data,
init_flags=init_flags,
method_flags=method_flags,
+ frontend=frontend,
on_device=on_device,
)
-# msort
@handle_frontend_method(
class_tree=CLASS_TREE,
init_tree="torch.tensor",
- method_name="msort",
- dtype_value=helpers.dtype_and_values(
- available_dtypes=["float32", "float64", "int32", "int64"],
- shape=st.shared(helpers.get_shape(min_num_dims=1), key="shape"),
+ method_name="ne_",
+ dtype_and_x=helpers.dtype_and_values(
+ available_dtypes=helpers.get_dtypes("float"),
+ num_arrays=2,
+ min_value=-1e04,
+ max_value=1e04,
+ allow_inf=False,
),
)
-def test_torch_tensor_msort(
- dtype_value,
+def test_torch_ne_(
+ dtype_and_x,
+ frontend,
frontend_method_data,
init_flags,
method_flags,
- frontend,
on_device,
backend_fw,
):
- input_dtype, x = dtype_value
+ input_dtype, x = dtype_and_x
helpers.test_frontend_method(
init_input_dtypes=input_dtype,
backend_to_test=backend_fw,
@@ -9556,31 +9735,36 @@ def test_torch_tensor_msort(
"data": x[0],
},
method_input_dtypes=input_dtype,
- method_all_as_kwargs_np={},
+ method_all_as_kwargs_np={
+ "other": x[1],
+ },
frontend_method_data=frontend_method_data,
init_flags=init_flags,
method_flags=method_flags,
frontend=frontend,
+ atol_=1e-02,
on_device=on_device,
)
-# mul
+# neg
@handle_frontend_method(
class_tree=CLASS_TREE,
init_tree="torch.tensor",
- method_name="mul",
+ method_name="neg",
dtype_and_x=helpers.dtype_and_values(
- available_dtypes=helpers.get_dtypes("numeric"),
- num_arrays=2,
+ available_dtypes=helpers.get_dtypes("float"),
+ min_value=-1e04,
+ max_value=1e04,
+ allow_inf=False,
),
)
-def test_torch_tensor_mul(
+def test_torch_neg(
dtype_and_x,
+ frontend,
frontend_method_data,
init_flags,
method_flags,
- frontend,
on_device,
backend_fw,
):
@@ -9592,9 +9776,7 @@ def test_torch_tensor_mul(
"data": x[0],
},
method_input_dtypes=input_dtype,
- method_all_as_kwargs_np={
- "other": x[1],
- },
+ method_all_as_kwargs_np={},
frontend_method_data=frontend_method_data,
init_flags=init_flags,
method_flags=method_flags,
@@ -9603,24 +9785,25 @@ def test_torch_tensor_mul(
)
-# mul_
+# neg_
@handle_frontend_method(
class_tree=CLASS_TREE,
init_tree="torch.tensor",
- method_name="mul_",
+ method_name="neg_",
dtype_and_x=helpers.dtype_and_values(
- available_dtypes=helpers.get_dtypes("numeric"),
- num_arrays=2,
- shared_dtype=True,
+ available_dtypes=helpers.get_dtypes("valid"),
+ min_value=-1e04,
+ max_value=1e04,
+ allow_inf=False,
),
test_inplace=st.just(True),
)
-def test_torch_tensor_mul_(
+def test_torch_neg_(
dtype_and_x,
+ frontend,
frontend_method_data,
init_flags,
method_flags,
- frontend,
on_device,
backend_fw,
):
@@ -9632,9 +9815,7 @@ def test_torch_tensor_mul_(
"data": x[0],
},
method_input_dtypes=input_dtype,
- method_all_as_kwargs_np={
- "other": x[1],
- },
+ method_all_as_kwargs_np={},
frontend_method_data=frontend_method_data,
init_flags=init_flags,
method_flags=method_flags,
@@ -9643,17 +9824,19 @@ def test_torch_tensor_mul_(
)
-# multiply
+# negative
@handle_frontend_method(
class_tree=CLASS_TREE,
init_tree="torch.tensor",
- method_name="multiply",
+ method_name="negative",
dtype_and_x=helpers.dtype_and_values(
- available_dtypes=helpers.get_dtypes("numeric"),
- num_arrays=2,
+ available_dtypes=helpers.get_dtypes("float"),
+ min_value=-1e04,
+ max_value=1e04,
+ allow_inf=False,
),
)
-def test_torch_tensor_multiply(
+def test_torch_negative(
dtype_and_x,
frontend_method_data,
init_flags,
@@ -9670,9 +9853,7 @@ def test_torch_tensor_multiply(
"data": x[0],
},
method_input_dtypes=input_dtype,
- method_all_as_kwargs_np={
- "other": x[1],
- },
+ method_all_as_kwargs_np={},
frontend_method_data=frontend_method_data,
init_flags=init_flags,
method_flags=method_flags,
@@ -9681,19 +9862,22 @@ def test_torch_tensor_multiply(
)
-# multiply_
+# new_empty (not actually intuitive for testing)
@handle_frontend_method(
class_tree=CLASS_TREE,
init_tree="torch.tensor",
- method_name="multiply_",
+ method_name="new_empty",
dtype_and_x=helpers.dtype_and_values(
available_dtypes=helpers.get_dtypes("numeric"),
- num_arrays=2,
),
- test_inplace=st.just(True),
+ size=helpers.get_shape(
+ min_num_dims=1,
+ max_num_dims=3,
+ ),
)
-def test_torch_tensor_multiply_(
+def test_torch_new_empty(
dtype_and_x,
+ size,
frontend_method_data,
init_flags,
method_flags,
@@ -9703,14 +9887,14 @@ def test_torch_tensor_multiply_(
):
input_dtype, x = dtype_and_x
helpers.test_frontend_method(
- init_input_dtypes=input_dtype,
+ init_input_dtypes=[input_dtype[0]],
backend_to_test=backend_fw,
init_all_as_kwargs_np={
- "data": x[0],
+ "data": x,
},
- method_input_dtypes=input_dtype,
+ method_input_dtypes=[ivy.int32],
method_all_as_kwargs_np={
- "other": x[1],
+ "size": size,
},
frontend_method_data=frontend_method_data,
init_flags=init_flags,
@@ -9720,35 +9904,34 @@ def test_torch_tensor_multiply_(
)
-# nanmean
+# new_full
@handle_frontend_method(
class_tree=CLASS_TREE,
init_tree="torch.tensor",
- method_name="nanmean",
- dtype_x=helpers.dtype_and_values(
- available_dtypes=helpers.get_dtypes("float"),
- min_value=-1e04,
- max_value=1e04,
- ),
+ method_name="new_full",
+ dtype_and_x=_fill_value_and_size(max_num_dims=3),
)
-def test_torch_tensor_nanmean(
- dtype_x,
- frontend,
+def test_torch_new_full(
+ dtype_and_x,
frontend_method_data,
init_flags,
method_flags,
+ frontend,
on_device,
backend_fw,
):
- input_dtype, x = dtype_x
+ input_dtype, x = dtype_and_x
helpers.test_frontend_method(
- init_input_dtypes=input_dtype,
+ init_input_dtypes=[input_dtype[0]],
backend_to_test=backend_fw,
init_all_as_kwargs_np={
"data": x[0],
},
- method_input_dtypes=input_dtype,
- method_all_as_kwargs_np={},
+ method_input_dtypes=[input_dtype[1]],
+ method_all_as_kwargs_np={
+ "size": x[1],
+ "fill_value": x[2],
+ },
frontend_method_data=frontend_method_data,
init_flags=init_flags,
method_flags=method_flags,
@@ -9757,64 +9940,67 @@ def test_torch_tensor_nanmean(
)
+# new_ones
@handle_frontend_method(
class_tree=CLASS_TREE,
init_tree="torch.tensor",
- method_name="narrow",
- dtype_input_dim_start_length=_dtype_input_dim_start_length(),
+ method_name="new_ones",
+ dtype_and_x=helpers.dtype_and_values(available_dtypes=helpers.get_dtypes("float")),
+ size=helpers.get_shape(
+ allow_none=False,
+ min_num_dims=1,
+ max_num_dims=5,
+ min_dim_size=1,
+ max_dim_size=10,
+ ),
+ dtypes=_dtypes(),
+ requires_grad=_requires_grad(),
)
-def test_torch_tensor_narrow(
- dtype_input_dim_start_length,
- frontend,
+def test_torch_new_ones(
+ dtype_and_x,
+ size,
+ dtypes,
+ requires_grad,
+ on_device,
frontend_method_data,
init_flags,
method_flags,
- on_device,
+ frontend,
backend_fw,
):
- (input_dtype, x, dim, start, length) = dtype_input_dim_start_length
+ input_dtype, x = dtype_and_x
helpers.test_frontend_method(
init_input_dtypes=input_dtype,
backend_to_test=backend_fw,
- init_all_as_kwargs_np={"data": x[0]},
- method_input_dtypes=input_dtype,
+ init_all_as_kwargs_np={
+ "data": x[0],
+ },
+ method_input_dtypes=dtypes,
method_all_as_kwargs_np={
- "dim": dim,
- "start": start,
- "length": length,
+ "size": size,
+ "dtype": dtypes[0],
+ "requires_grad": requires_grad,
+ "device": on_device,
},
- frontend=frontend,
frontend_method_data=frontend_method_data,
init_flags=init_flags,
method_flags=method_flags,
+ frontend=frontend,
on_device=on_device,
)
-@given(
- dtype_x=helpers.dtype_and_values(
- available_dtypes=helpers.get_dtypes("valid", prune_function=False),
- ret_shape=True,
- ).filter(lambda x: "bfloat16" not in x[0]),
-)
-def test_torch_tensor_ndim(dtype_x, backend_fw):
- ivy.set_backend(backend_fw)
- dtype, data, shape = dtype_x
- x = Tensor(data[0])
- ivy.utils.assertions.check_equal(x.ndim, data[0].ndim, as_array=False)
- ivy.previous_backend()
-
-
-# ndimension
+# new_tensor
@handle_frontend_method(
class_tree=CLASS_TREE,
init_tree="torch.tensor",
- method_name="ndimension",
+ method_name="new_tensor",
dtype_and_x=helpers.dtype_and_values(
available_dtypes=helpers.get_dtypes("numeric"),
+ num_arrays=2,
),
)
-def test_torch_tensor_ndimension(
+def test_torch_new_tensor(
dtype_and_x,
frontend_method_data,
init_flags,
@@ -9825,13 +10011,16 @@ def test_torch_tensor_ndimension(
):
input_dtype, x = dtype_and_x
helpers.test_frontend_method(
- init_input_dtypes=input_dtype,
+ init_input_dtypes=[input_dtype[0]],
backend_to_test=backend_fw,
init_all_as_kwargs_np={
"data": x[0],
},
- method_input_dtypes=[],
- method_all_as_kwargs_np={},
+ method_input_dtypes=[input_dtype[1]],
+ method_all_as_kwargs_np={
+ "data": x[1],
+ "dtype": input_dtype[1],
+ },
frontend_method_data=frontend_method_data,
init_flags=init_flags,
method_flags=method_flags,
@@ -9840,26 +10029,32 @@ def test_torch_tensor_ndimension(
)
-# ne
+# new_zeros
@handle_frontend_method(
class_tree=CLASS_TREE,
init_tree="torch.tensor",
- method_name="ne",
- dtype_and_x=helpers.dtype_and_values(
- available_dtypes=helpers.get_dtypes("float"),
- num_arrays=2,
- min_value=-1e04,
- max_value=1e04,
- allow_inf=False,
+ method_name="new_zeros",
+ dtype_and_x=helpers.dtype_and_values(available_dtypes=helpers.get_dtypes("valid")),
+ size=helpers.get_shape(
+ allow_none=False,
+ min_num_dims=1,
+ max_num_dims=5,
+ min_dim_size=1,
+ max_dim_size=10,
),
+ dtypes=_dtypes(),
+ requires_grad=_requires_grad(),
)
-def test_torch_tensor_ne(
+def test_torch_new_zeros(
dtype_and_x,
+ size,
+ dtypes,
+ requires_grad,
+ on_device,
frontend_method_data,
init_flags,
method_flags,
frontend,
- on_device,
backend_fw,
):
input_dtype, x = dtype_and_x
@@ -9869,9 +10064,12 @@ def test_torch_tensor_ne(
init_all_as_kwargs_np={
"data": x[0],
},
- method_input_dtypes=input_dtype,
+ method_input_dtypes=dtypes,
method_all_as_kwargs_np={
- "other": x[1],
+ "size": size,
+ "dtype": dtypes[0],
+ "requires_grad": requires_grad,
+ "device": on_device,
},
frontend_method_data=frontend_method_data,
init_flags=init_flags,
@@ -9881,28 +10079,25 @@ def test_torch_tensor_ne(
)
-# neg
+# nonzero
@handle_frontend_method(
class_tree=CLASS_TREE,
init_tree="torch.tensor",
- method_name="neg",
- dtype_and_x=helpers.dtype_and_values(
- available_dtypes=helpers.get_dtypes("float"),
- min_value=-1e04,
- max_value=1e04,
- allow_inf=False,
+ method_name="nonzero",
+ dtype_and_values=helpers.dtype_and_values(
+ available_dtypes=helpers.get_dtypes("numeric"),
),
)
-def test_torch_tensor_neg(
- dtype_and_x,
- frontend,
+def test_torch_nonzero(
+ dtype_and_values,
frontend_method_data,
init_flags,
method_flags,
+ frontend,
on_device,
backend_fw,
):
- input_dtype, x = dtype_and_x
+ input_dtype, x = dtype_and_values
helpers.test_frontend_method(
init_input_dtypes=input_dtype,
backend_to_test=backend_fw,
@@ -9919,21 +10114,19 @@ def test_torch_tensor_neg(
)
-# neg_
+# norm
@handle_frontend_method(
class_tree=CLASS_TREE,
init_tree="torch.tensor",
- method_name="neg_",
- dtype_and_x=helpers.dtype_and_values(
- available_dtypes=helpers.get_dtypes("valid"),
- min_value=-1e04,
- max_value=1e04,
- allow_inf=False,
- ),
- test_inplace=st.just(True),
+ method_name="norm",
+ p_dtype_x_axis=_get_axis_and_p(),
+ keepdim=st.booleans(),
+ dtype=helpers.get_dtypes("valid", full=False),
)
-def test_torch_tensor_neg_(
- dtype_and_x,
+def test_torch_norm(
+ p_dtype_x_axis,
+ keepdim,
+ dtype,
frontend,
frontend_method_data,
init_flags,
@@ -9941,201 +10134,218 @@ def test_torch_tensor_neg_(
on_device,
backend_fw,
):
- input_dtype, x = dtype_and_x
+ p, values = p_dtype_x_axis
+ input_dtype, x, axis = values
helpers.test_frontend_method(
init_input_dtypes=input_dtype,
backend_to_test=backend_fw,
- init_all_as_kwargs_np={
- "data": x[0],
- },
+ init_all_as_kwargs_np={"data": x[0]},
method_input_dtypes=input_dtype,
- method_all_as_kwargs_np={},
+ method_all_as_kwargs_np={
+ "p": p,
+ "dim": axis,
+ "keepdim": keepdim,
+ "dtype": dtype[0],
+ },
+ frontend=frontend,
frontend_method_data=frontend_method_data,
init_flags=init_flags,
method_flags=method_flags,
- frontend=frontend,
on_device=on_device,
)
-# negative
+# normal_
@handle_frontend_method(
class_tree=CLASS_TREE,
init_tree="torch.tensor",
- method_name="negative",
+ method_name="normal_",
dtype_and_x=helpers.dtype_and_values(
available_dtypes=helpers.get_dtypes("float"),
- min_value=-1e04,
- max_value=1e04,
- allow_inf=False,
),
+ mean=helpers.floats(min_value=-1, max_value=1),
+ std=helpers.floats(min_value=0, max_value=1),
)
-def test_torch_tensor_negative(
+def test_torch_normal_(
dtype_and_x,
+ mean,
+ std,
+ frontend,
frontend_method_data,
init_flags,
method_flags,
- frontend,
on_device,
backend_fw,
):
- input_dtype, x = dtype_and_x
- helpers.test_frontend_method(
- init_input_dtypes=input_dtype,
- backend_to_test=backend_fw,
- init_all_as_kwargs_np={
- "data": x[0],
- },
- method_input_dtypes=input_dtype,
- method_all_as_kwargs_np={},
- frontend_method_data=frontend_method_data,
- init_flags=init_flags,
- method_flags=method_flags,
- frontend=frontend,
- on_device=on_device,
- )
+ dtype, x = dtype_and_x
+
+ def call():
+ return helpers.test_frontend_method(
+ init_input_dtypes=dtype,
+ backend_to_test=backend_fw,
+ init_all_as_kwargs_np={"data": x[0]},
+ method_input_dtypes=dtype,
+ method_all_as_kwargs_np={
+ "mean": mean,
+ "std": std,
+ },
+ frontend_method_data=frontend_method_data,
+ init_flags=init_flags,
+ method_flags=method_flags,
+ frontend=frontend,
+ on_device=on_device,
+ test_values=False,
+ )
+
+ ret = call()
+ if not ivy.exists(ret):
+ return
-# new_empty (not actually intuitive for testing)
+ ret_np, ret_from_np = ret
+ ret_np = helpers.flatten_and_to_np(ret=ret_np)
+ ret_from_np = helpers.flatten_and_to_np(ret=ret_from_np)
+ for u, v in zip(ret_np, ret_from_np):
+ assert u.dtype == v.dtype
+ assert u.shape == v.shape
+
+
+# not_equal
@handle_frontend_method(
class_tree=CLASS_TREE,
init_tree="torch.tensor",
- method_name="new_empty",
+ method_name="not_equal",
dtype_and_x=helpers.dtype_and_values(
- available_dtypes=helpers.get_dtypes("numeric"),
- ),
- size=helpers.get_shape(
- min_num_dims=1,
- max_num_dims=3,
+ available_dtypes=helpers.get_dtypes("float"),
+ num_arrays=2,
+ min_value=-1e04,
+ max_value=1e04,
+ allow_inf=False,
),
)
-def test_torch_tensor_new_empty(
+def test_torch_not_equal(
dtype_and_x,
- size,
+ frontend,
frontend_method_data,
init_flags,
method_flags,
- frontend,
on_device,
backend_fw,
):
input_dtype, x = dtype_and_x
helpers.test_frontend_method(
- init_input_dtypes=[input_dtype[0]],
+ init_input_dtypes=input_dtype,
backend_to_test=backend_fw,
init_all_as_kwargs_np={
- "data": x,
+ "data": x[0],
},
- method_input_dtypes=[ivy.int32],
+ method_input_dtypes=input_dtype,
method_all_as_kwargs_np={
- "size": size,
+ "other": x[1],
},
frontend_method_data=frontend_method_data,
init_flags=init_flags,
method_flags=method_flags,
frontend=frontend,
+ atol_=1e-02,
on_device=on_device,
)
-# new_full
@handle_frontend_method(
class_tree=CLASS_TREE,
init_tree="torch.tensor",
- method_name="new_full",
- dtype_and_x=_fill_value_and_size(max_num_dims=3),
+ method_name="not_equal_",
+ dtype_and_x=helpers.dtype_and_values(
+ available_dtypes=helpers.get_dtypes("float"),
+ num_arrays=2,
+ min_value=-1e04,
+ max_value=1e04,
+ allow_inf=False,
+ ),
)
-def test_torch_tensor_new_full(
+def test_torch_not_equal_(
dtype_and_x,
+ frontend,
frontend_method_data,
init_flags,
method_flags,
- frontend,
on_device,
backend_fw,
):
input_dtype, x = dtype_and_x
helpers.test_frontend_method(
- init_input_dtypes=[input_dtype[0]],
+ init_input_dtypes=input_dtype,
backend_to_test=backend_fw,
init_all_as_kwargs_np={
"data": x[0],
},
- method_input_dtypes=[input_dtype[1]],
+ method_input_dtypes=input_dtype,
method_all_as_kwargs_np={
- "size": x[1],
- "fill_value": x[2],
+ "other": x[1],
},
frontend_method_data=frontend_method_data,
init_flags=init_flags,
method_flags=method_flags,
frontend=frontend,
+ atol_=1e-02,
on_device=on_device,
)
-# new_ones
+# numpy
@handle_frontend_method(
class_tree=CLASS_TREE,
init_tree="torch.tensor",
- method_name="new_ones",
- dtype_and_x=helpers.dtype_and_values(available_dtypes=helpers.get_dtypes("float")),
- size=helpers.get_shape(
- allow_none=False,
- min_num_dims=1,
- max_num_dims=5,
- min_dim_size=1,
- max_dim_size=10,
+ method_name="numpy",
+ dtype_and_x=helpers.dtype_and_values(
+ available_dtypes=helpers.get_dtypes("valid"),
),
- dtypes=_dtypes(),
- requires_grad=_requires_grad(),
)
-def test_torch_tensor_new_ones(
+def test_torch_numpy(
dtype_and_x,
- size,
- dtypes,
- requires_grad,
- on_device,
frontend_method_data,
init_flags,
method_flags,
frontend,
+ on_device,
backend_fw,
):
input_dtype, x = dtype_and_x
- helpers.test_frontend_method(
+ ret, frontend_ret = helpers.test_frontend_method(
init_input_dtypes=input_dtype,
backend_to_test=backend_fw,
init_all_as_kwargs_np={
"data": x[0],
},
- method_input_dtypes=dtypes,
- method_all_as_kwargs_np={
- "size": size,
- "dtype": dtypes[0],
- "requires_grad": requires_grad,
- "device": on_device,
- },
+ method_input_dtypes=[],
+ method_all_as_kwargs_np={},
frontend_method_data=frontend_method_data,
init_flags=init_flags,
method_flags=method_flags,
frontend=frontend,
on_device=on_device,
+ test_values=False,
+ )
+ # manual testing required as function return is numpy frontend
+ helpers.value_test(
+ ret_np_flat=helpers.flatten_and_to_np(ret=ret),
+ ret_np_from_gt_flat=frontend_ret[0],
+ ground_truth_backend="torch",
)
-# new_tensor
+# permute
@handle_frontend_method(
class_tree=CLASS_TREE,
init_tree="torch.tensor",
- method_name="new_tensor",
- dtype_and_x=helpers.dtype_and_values(
- available_dtypes=helpers.get_dtypes("numeric"),
- num_arrays=2,
+ method_name="permute",
+ dtype_values_axis=_array_idxes_n_dtype(
+ available_dtypes=helpers.get_dtypes("float"),
),
)
-def test_torch_tensor_new_tensor(
- dtype_and_x,
+def test_torch_permute(
+ dtype_values_axis,
frontend_method_data,
init_flags,
method_flags,
@@ -10143,18 +10353,27 @@ def test_torch_tensor_new_tensor(
on_device,
backend_fw,
):
- input_dtype, x = dtype_and_x
+ x, idxes, dtype = dtype_values_axis
+ unpack_dims = True
+ if unpack_dims:
+ method_flags.num_positional_args = len(idxes) + 1
+ dims = {}
+ i = 0
+ for x_ in idxes:
+ dims[f"x{i}"] = x_
+ i += 1
+ else:
+ dims = {
+ "dims": tuple(idxes),
+ }
helpers.test_frontend_method(
- init_input_dtypes=[input_dtype[0]],
+ init_input_dtypes=dtype,
backend_to_test=backend_fw,
init_all_as_kwargs_np={
"data": x[0],
},
- method_input_dtypes=[input_dtype[1]],
- method_all_as_kwargs_np={
- "data": x[1],
- "dtype": input_dtype[1],
- },
+ method_input_dtypes=dtype,
+ method_all_as_kwargs_np=dims,
frontend_method_data=frontend_method_data,
init_flags=init_flags,
method_flags=method_flags,
@@ -10163,47 +10382,41 @@ def test_torch_tensor_new_tensor(
)
-# new_zeros
+# pow
@handle_frontend_method(
class_tree=CLASS_TREE,
init_tree="torch.tensor",
- method_name="new_zeros",
- dtype_and_x=helpers.dtype_and_values(available_dtypes=helpers.get_dtypes("valid")),
- size=helpers.get_shape(
- allow_none=False,
- min_num_dims=1,
- max_num_dims=5,
- min_dim_size=1,
- max_dim_size=10,
+ method_name="pow",
+ dtype_and_x=helpers.dtype_and_values(
+ available_dtypes=helpers.get_dtypes("numeric"),
+ num_arrays=2,
+ min_value=-1e04,
+ max_value=1e04,
+ allow_inf=False,
),
- dtypes=_dtypes(),
- requires_grad=_requires_grad(),
)
-def test_torch_tensor_new_zeros(
+def test_torch_pow(
dtype_and_x,
- size,
- dtypes,
- requires_grad,
- on_device,
frontend_method_data,
init_flags,
method_flags,
frontend,
+ on_device,
backend_fw,
):
input_dtype, x = dtype_and_x
+ dtype = input_dtype[0]
+ if "int" in dtype:
+ x[1] = ivy.abs(x[1])
helpers.test_frontend_method(
init_input_dtypes=input_dtype,
backend_to_test=backend_fw,
init_all_as_kwargs_np={
"data": x[0],
},
- method_input_dtypes=dtypes,
+ method_input_dtypes=input_dtype,
method_all_as_kwargs_np={
- "size": size,
- "dtype": dtypes[0],
- "requires_grad": requires_grad,
- "device": on_device,
+ "exponent": x[1],
},
frontend_method_data=frontend_method_data,
init_flags=init_flags,
@@ -10213,17 +10426,19 @@ def test_torch_tensor_new_zeros(
)
-# nonzero
+# pow_
@handle_frontend_method(
class_tree=CLASS_TREE,
init_tree="torch.tensor",
- method_name="nonzero",
- dtype_and_values=helpers.dtype_and_values(
+ method_name="pow_",
+ dtype_and_x=helpers.dtype_and_values(
available_dtypes=helpers.get_dtypes("numeric"),
+ num_arrays=2,
),
+ test_inplace=st.just(True),
)
-def test_torch_tensor_nonzero(
- dtype_and_values,
+def test_torch_pow_(
+ dtype_and_x,
frontend_method_data,
init_flags,
method_flags,
@@ -10231,7 +10446,10 @@ def test_torch_tensor_nonzero(
on_device,
backend_fw,
):
- input_dtype, x = dtype_and_values
+ input_dtype, x = dtype_and_x
+ dtype = input_dtype[0]
+ if "int" in dtype:
+ x[1] = ivy.abs(x[1])
helpers.test_frontend_method(
init_input_dtypes=input_dtype,
backend_to_test=backend_fw,
@@ -10239,7 +10457,9 @@ def test_torch_tensor_nonzero(
"data": x[0],
},
method_input_dtypes=input_dtype,
- method_all_as_kwargs_np={},
+ method_all_as_kwargs_np={
+ "exponent": x[1],
+ },
frontend_method_data=frontend_method_data,
init_flags=init_flags,
method_flags=method_flags,
@@ -10248,19 +10468,30 @@ def test_torch_tensor_nonzero(
)
-# norm
+# prod
@handle_frontend_method(
class_tree=CLASS_TREE,
init_tree="torch.tensor",
- method_name="norm",
- p_dtype_x_axis=_get_axis_and_p(),
- keepdim=st.booleans(),
- dtype=helpers.get_dtypes("valid", full=False),
+ method_name="prod",
+ dtype_x_axis=helpers.dtype_values_axis(
+ available_dtypes=helpers.get_dtypes("numeric"),
+ min_num_dims=1,
+ max_num_dims=5,
+ valid_axis=True,
+ allow_neg_axes=False,
+ max_axes_size=1,
+ force_int_axis=True,
+ large_abs_safety_factor=10,
+ small_abs_safety_factor=10,
+ safety_factor_scale="log",
+ ),
+ dtype=helpers.get_dtypes("float", none=True, full=False),
+ keepdims=st.booleans(),
)
-def test_torch_tensor_norm(
- p_dtype_x_axis,
- keepdim,
+def test_torch_prod(
+ dtype_x_axis,
dtype,
+ keepdims,
frontend,
frontend_method_data,
init_flags,
@@ -10268,42 +10499,38 @@ def test_torch_tensor_norm(
on_device,
backend_fw,
):
- p, values = p_dtype_x_axis
- input_dtype, x, axis = values
+ input_dtype, x, axis = dtype_x_axis
+ if ivy.current_backend_str() == "torch":
+ init_flags.as_variable = [False]
+ method_flags.as_variable = [False]
helpers.test_frontend_method(
init_input_dtypes=input_dtype,
backend_to_test=backend_fw,
init_all_as_kwargs_np={"data": x[0]},
method_input_dtypes=input_dtype,
method_all_as_kwargs_np={
- "p": p,
"dim": axis,
- "keepdim": keepdim,
+ "keepdim": keepdims,
"dtype": dtype[0],
},
- frontend=frontend,
frontend_method_data=frontend_method_data,
init_flags=init_flags,
method_flags=method_flags,
+ frontend=frontend,
on_device=on_device,
)
-# normal_
@handle_frontend_method(
class_tree=CLASS_TREE,
init_tree="torch.tensor",
- method_name="normal_",
- dtype_and_x=helpers.dtype_and_values(
- available_dtypes=helpers.get_dtypes("float"),
- ),
- mean=helpers.floats(min_value=-1, max_value=1),
- std=helpers.floats(min_value=0, max_value=1),
+ method_name="quantile",
+ dtype_and_x=_quantile_helper().filter(lambda x: "bfloat16" not in x[0]),
+ keepdims=st.booleans(),
)
-def test_torch_tensor_normal_(
+def test_torch_quantile(
dtype_and_x,
- mean,
- std,
+ keepdims,
frontend,
frontend_method_data,
init_flags,
@@ -10311,55 +10538,45 @@ def test_torch_tensor_normal_(
on_device,
backend_fw,
):
- dtype, x = dtype_and_x
-
- def call():
- return helpers.test_frontend_method(
- init_input_dtypes=dtype,
- backend_to_test=backend_fw,
- init_all_as_kwargs_np={"data": x[0]},
- method_input_dtypes=dtype,
- method_all_as_kwargs_np={
- "mean": mean,
- "std": std,
- },
- frontend_method_data=frontend_method_data,
- init_flags=init_flags,
- method_flags=method_flags,
- frontend=frontend,
- on_device=on_device,
- test_values=False,
- )
-
- ret = call()
-
- if not ivy.exists(ret):
- return
-
- ret_np, ret_from_np = ret
- ret_np = helpers.flatten_and_to_np(ret=ret_np)
- ret_from_np = helpers.flatten_and_to_np(ret=ret_from_np)
- for u, v in zip(ret_np, ret_from_np):
- assert u.dtype == v.dtype
- assert u.shape == v.shape
+ input_dtype, x, axis, interpolation, q = dtype_and_x
+ if type(axis) is tuple:
+ axis = axis[0]
+ helpers.test_frontend_method(
+ init_input_dtypes=input_dtype,
+ backend_to_test=backend_fw,
+ init_all_as_kwargs_np={
+ "data": x[0],
+ },
+ method_input_dtypes=input_dtype,
+ method_all_as_kwargs_np={
+ "q": q,
+ "dim": axis,
+ "keepdim": keepdims,
+ "interpolation": interpolation[0],
+ },
+ frontend=frontend,
+ frontend_method_data=frontend_method_data,
+ init_flags=init_flags,
+ method_flags=method_flags,
+ on_device=on_device,
+ )
-# not_equal
+# rad2deg
@handle_frontend_method(
class_tree=CLASS_TREE,
init_tree="torch.tensor",
- method_name="not_equal",
+ method_name="rad2deg",
dtype_and_x=helpers.dtype_and_values(
- available_dtypes=helpers.get_dtypes("valid"),
- num_arrays=2,
+ available_dtypes=helpers.get_dtypes("float"),
),
)
-def test_torch_tensor_not_equal(
+def test_torch_rad2deg(
dtype_and_x,
- frontend,
frontend_method_data,
init_flags,
method_flags,
+ frontend,
on_device,
backend_fw,
):
@@ -10371,71 +10588,72 @@ def test_torch_tensor_not_equal(
"data": x[0],
},
method_input_dtypes=input_dtype,
- method_all_as_kwargs_np={
- "other": x[1],
- },
+ method_all_as_kwargs_np={},
frontend_method_data=frontend_method_data,
init_flags=init_flags,
method_flags=method_flags,
frontend=frontend,
- atol_=1e-02,
on_device=on_device,
)
-# numpy
+# random_
@handle_frontend_method(
class_tree=CLASS_TREE,
init_tree="torch.tensor",
- method_name="numpy",
+ method_name="random_",
dtype_and_x=helpers.dtype_and_values(
- available_dtypes=helpers.get_dtypes("valid"),
+ available_dtypes=helpers.get_dtypes("float_and_integer"),
+ min_value=1,
+ max_value=5,
+ min_num_dims=1,
+ max_num_dims=5,
),
+ to=helpers.ints(min_value=1, max_value=100),
+ test_inplace=st.just(True),
)
-def test_torch_tensor_numpy(
+def test_torch_random_(
dtype_and_x,
+ to,
+ frontend,
frontend_method_data,
init_flags,
method_flags,
- frontend,
on_device,
backend_fw,
):
input_dtype, x = dtype_and_x
- ret, frontend_ret = helpers.test_frontend_method(
+ helpers.test_frontend_method(
init_input_dtypes=input_dtype,
backend_to_test=backend_fw,
+ method_input_dtypes=input_dtype,
+ frontend_method_data=frontend_method_data,
init_all_as_kwargs_np={
"data": x[0],
},
- method_input_dtypes=[],
- method_all_as_kwargs_np={},
- frontend_method_data=frontend_method_data,
+ method_all_as_kwargs_np={
+ "to": to,
+ },
init_flags=init_flags,
method_flags=method_flags,
frontend=frontend,
on_device=on_device,
test_values=False,
)
- # manual testing required as function return is numpy frontend
- helpers.value_test(
- ret_np_flat=helpers.flatten_and_to_np(ret=ret),
- ret_np_from_gt_flat=frontend_ret[0],
- ground_truth_backend="torch",
- )
-# permute
+# ravel
@handle_frontend_method(
class_tree=CLASS_TREE,
init_tree="torch.tensor",
- method_name="permute",
- dtype_values_axis=_array_idxes_n_dtype(
- available_dtypes=helpers.get_dtypes("float"),
+ method_name="ravel",
+ dtype_value=helpers.dtype_and_values(
+ available_dtypes=helpers.get_dtypes("valid"),
+ shape=st.shared(helpers.get_shape(min_num_dims=1), key="shape"),
),
)
-def test_torch_tensor_permute(
- dtype_values_axis,
+def test_torch_ravel(
+ dtype_value,
frontend_method_data,
init_flags,
method_flags,
@@ -10443,27 +10661,15 @@ def test_torch_tensor_permute(
on_device,
backend_fw,
):
- x, idxes, dtype = dtype_values_axis
- unpack_dims = True
- if unpack_dims:
- method_flags.num_positional_args = len(idxes) + 1
- dims = {}
- i = 0
- for x_ in idxes:
- dims[f"x{i}"] = x_
- i += 1
- else:
- dims = {
- "dims": tuple(idxes),
- }
+ input_dtype, x = dtype_value
helpers.test_frontend_method(
- init_input_dtypes=dtype,
+ init_input_dtypes=input_dtype,
backend_to_test=backend_fw,
init_all_as_kwargs_np={
"data": x[0],
},
- method_input_dtypes=dtype,
- method_all_as_kwargs_np=dims,
+ method_input_dtypes=input_dtype,
+ method_all_as_kwargs_np={},
frontend_method_data=frontend_method_data,
init_flags=init_flags,
method_flags=method_flags,
@@ -10472,20 +10678,31 @@ def test_torch_tensor_permute(
)
-# pow
+@given(
+ dtype_x=helpers.dtype_and_values(
+ available_dtypes=helpers.get_dtypes("complex", prune_function=False)
+ ).filter(lambda x: "bfloat16" not in x[0]),
+)
+def test_torch_real(dtype_x, backend_fw):
+ ivy.set_backend(backend_fw)
+ _, data = dtype_x
+ x = Tensor(data[0])
+ x.ivy_array = data[0]
+ ivy.utils.assertions.check_equal(x.real, ivy.real(data[0]))
+ ivy.previous_backend()
+
+
+# reciprocal
@handle_frontend_method(
class_tree=CLASS_TREE,
init_tree="torch.tensor",
- method_name="pow",
+ method_name="reciprocal",
dtype_and_x=helpers.dtype_and_values(
- available_dtypes=helpers.get_dtypes("numeric"),
- num_arrays=2,
- min_value=-1e04,
- max_value=1e04,
- allow_inf=False,
+ available_dtypes=helpers.get_dtypes("float"),
+ min_value=1,
),
)
-def test_torch_tensor_pow(
+def test_torch_reciprocal(
dtype_and_x,
frontend_method_data,
init_flags,
@@ -10495,9 +10712,6 @@ def test_torch_tensor_pow(
backend_fw,
):
input_dtype, x = dtype_and_x
- dtype = input_dtype[0]
- if "int" in dtype:
- x[1] = ivy.abs(x[1])
helpers.test_frontend_method(
init_input_dtypes=input_dtype,
backend_to_test=backend_fw,
@@ -10505,9 +10719,7 @@ def test_torch_tensor_pow(
"data": x[0],
},
method_input_dtypes=input_dtype,
- method_all_as_kwargs_np={
- "exponent": x[1],
- },
+ method_all_as_kwargs_np={},
frontend_method_data=frontend_method_data,
init_flags=init_flags,
method_flags=method_flags,
@@ -10516,18 +10728,18 @@ def test_torch_tensor_pow(
)
-# pow_
+# reciprocal_
@handle_frontend_method(
class_tree=CLASS_TREE,
init_tree="torch.tensor",
- method_name="pow_",
+ method_name="reciprocal_",
dtype_and_x=helpers.dtype_and_values(
- available_dtypes=helpers.get_dtypes("numeric"),
- num_arrays=2,
+ available_dtypes=helpers.get_dtypes("valid"),
+ min_value=1,
),
test_inplace=st.just(True),
)
-def test_torch_tensor_pow_(
+def test_torch_reciprocal_(
dtype_and_x,
frontend_method_data,
init_flags,
@@ -10537,9 +10749,6 @@ def test_torch_tensor_pow_(
backend_fw,
):
input_dtype, x = dtype_and_x
- dtype = input_dtype[0]
- if "int" in dtype:
- x[1] = ivy.abs(x[1])
helpers.test_frontend_method(
init_input_dtypes=input_dtype,
backend_to_test=backend_fw,
@@ -10547,9 +10756,7 @@ def test_torch_tensor_pow_(
"data": x[0],
},
method_input_dtypes=input_dtype,
- method_all_as_kwargs_np={
- "exponent": x[1],
- },
+ method_all_as_kwargs_np={},
frontend_method_data=frontend_method_data,
init_flags=init_flags,
method_flags=method_flags,
@@ -10558,51 +10765,34 @@ def test_torch_tensor_pow_(
)
-# prod
+# relu
@handle_frontend_method(
class_tree=CLASS_TREE,
init_tree="torch.tensor",
- method_name="prod",
- dtype_x_axis=helpers.dtype_values_axis(
- available_dtypes=helpers.get_dtypes("numeric"),
- min_num_dims=1,
- max_num_dims=5,
- valid_axis=True,
- allow_neg_axes=False,
- max_axes_size=1,
- force_int_axis=True,
- large_abs_safety_factor=10,
- small_abs_safety_factor=10,
- safety_factor_scale="log",
+ method_name="relu",
+ dtype_and_x=helpers.dtype_and_values(
+ available_dtypes=helpers.get_dtypes("float"),
+ allow_inf=False,
),
- dtype=helpers.get_dtypes("float", none=True, full=False),
- keepdims=st.booleans(),
)
-def test_torch_tensor_prod(
- dtype_x_axis,
- dtype,
- keepdims,
- frontend,
+def test_torch_relu(
+ dtype_and_x,
frontend_method_data,
init_flags,
method_flags,
+ frontend,
on_device,
backend_fw,
):
- input_dtype, x, axis = dtype_x_axis
- if ivy.current_backend_str() == "torch":
- init_flags.as_variable = [False]
- method_flags.as_variable = [False]
+ input_dtype, x = dtype_and_x
helpers.test_frontend_method(
init_input_dtypes=input_dtype,
backend_to_test=backend_fw,
- init_all_as_kwargs_np={"data": x[0]},
- method_input_dtypes=input_dtype,
- method_all_as_kwargs_np={
- "dim": axis,
- "keepdim": keepdims,
- "dtype": dtype[0],
+ init_all_as_kwargs_np={
+ "data": x[0],
},
+ method_input_dtypes=input_dtype,
+ method_all_as_kwargs_np={},
frontend_method_data=frontend_method_data,
init_flags=init_flags,
method_flags=method_flags,
@@ -10611,26 +10801,29 @@ def test_torch_tensor_prod(
)
+# remainder
@handle_frontend_method(
class_tree=CLASS_TREE,
init_tree="torch.tensor",
- method_name="quantile",
- dtype_and_x=_quantile_helper().filter(lambda x: "bfloat16" not in x[0]),
- keepdims=st.booleans(),
+ method_name="remainder",
+ dtype_and_x=helpers.dtype_and_values(
+ available_dtypes=helpers.get_dtypes("float"),
+ large_abs_safety_factor=2.5,
+ small_abs_safety_factor=2.5,
+ shared_dtype=True,
+ num_arrays=2,
+ ),
)
-def test_torch_tensor_quantile(
+def test_torch_remainder(
dtype_and_x,
- keepdims,
- frontend,
frontend_method_data,
init_flags,
method_flags,
+ frontend,
on_device,
backend_fw,
):
- input_dtype, x, axis, interpolation, q = dtype_and_x
- if type(axis) is tuple:
- axis = axis[0]
+ input_dtype, x = dtype_and_x
helpers.test_frontend_method(
init_input_dtypes=input_dtype,
backend_to_test=backend_fw,
@@ -10639,41 +10832,38 @@ def test_torch_tensor_quantile(
},
method_input_dtypes=input_dtype,
method_all_as_kwargs_np={
- "q": q,
- "dim": axis,
- "keepdim": keepdims,
- "interpolation": interpolation[0],
+ "other": x[1],
},
- frontend=frontend,
frontend_method_data=frontend_method_data,
init_flags=init_flags,
method_flags=method_flags,
+ frontend=frontend,
on_device=on_device,
)
-# random_
+# remainder_
@handle_frontend_method(
class_tree=CLASS_TREE,
init_tree="torch.tensor",
- method_name="random_",
+ method_name="remainder_",
dtype_and_x=helpers.dtype_and_values(
- available_dtypes=helpers.get_dtypes("float_and_integer"),
- min_value=1,
- max_value=5,
- min_num_dims=1,
- max_num_dims=5,
+ available_dtypes=helpers.get_dtypes("valid"),
+ min_value=-1e04,
+ max_value=1e04,
+ large_abs_safety_factor=2.5,
+ small_abs_safety_factor=2.5,
+ shared_dtype=True,
+ num_arrays=2,
),
- to=helpers.ints(min_value=1, max_value=100),
test_inplace=st.just(True),
)
-def test_torch_tensor_random_(
+def test_torch_remainder_(
dtype_and_x,
- to,
- frontend,
frontend_method_data,
init_flags,
method_flags,
+ frontend,
on_device,
backend_fw,
):
@@ -10681,34 +10871,32 @@ def test_torch_tensor_random_(
helpers.test_frontend_method(
init_input_dtypes=input_dtype,
backend_to_test=backend_fw,
- method_input_dtypes=input_dtype,
- frontend_method_data=frontend_method_data,
init_all_as_kwargs_np={
"data": x[0],
},
+ method_input_dtypes=input_dtype,
method_all_as_kwargs_np={
- "to": to,
+ "other": x[1],
},
+ frontend_method_data=frontend_method_data,
init_flags=init_flags,
method_flags=method_flags,
frontend=frontend,
on_device=on_device,
- test_values=False,
)
-# ravel
+# repeat
@handle_frontend_method(
class_tree=CLASS_TREE,
init_tree="torch.tensor",
- method_name="ravel",
- dtype_value=helpers.dtype_and_values(
- available_dtypes=helpers.get_dtypes("valid"),
- shape=st.shared(helpers.get_shape(min_num_dims=1), key="shape"),
- ),
+ method_name="repeat",
+ dtype_x_repeats=_repeat_helper(),
+ unpack_repeat=st.booleans(),
)
-def test_torch_tensor_ravel(
- dtype_value,
+def test_torch_repeat(
+ dtype_x_repeats,
+ unpack_repeat,
frontend_method_data,
init_flags,
method_flags,
@@ -10716,7 +10904,14 @@ def test_torch_tensor_ravel(
on_device,
backend_fw,
):
- input_dtype, x = dtype_value
+ input_dtype, x, repeats = dtype_x_repeats
+ repeat = {
+ "repeats": repeats,
+ }
+ if unpack_repeat:
+ method_flags.num_positional_args = len(repeat["repeats"]) + 1
+ for i, x_ in enumerate(repeat["repeats"]):
+ repeat[f"x{i}"] = x_
helpers.test_frontend_method(
init_input_dtypes=input_dtype,
backend_to_test=backend_fw,
@@ -10724,7 +10919,7 @@ def test_torch_tensor_ravel(
"data": x[0],
},
method_input_dtypes=input_dtype,
- method_all_as_kwargs_np={},
+ method_all_as_kwargs_np=repeat,
frontend_method_data=frontend_method_data,
init_flags=init_flags,
method_flags=method_flags,
@@ -10735,30 +10930,37 @@ def test_torch_tensor_ravel(
@given(
dtype_x=helpers.dtype_and_values(
- available_dtypes=helpers.get_dtypes("complex", prune_function=False)
- ).filter(lambda x: "bfloat16" not in x[0]),
+ available_dtypes=helpers.get_dtypes("valid", prune_function=False),
+ ),
+ requires_grad=st.booleans(),
)
-def test_torch_tensor_real(dtype_x, backend_fw):
+def test_torch_requires_grad(dtype_x, requires_grad, backend_fw):
ivy.set_backend(backend_fw)
_, data = dtype_x
- x = Tensor(data[0])
- x.ivy_array = data[0]
- ivy.utils.assertions.check_equal(x.real, ivy.real(data[0]))
+ x = Tensor(data[0], requires_grad=requires_grad)
+ ivy.utils.assertions.check_equal(x.requires_grad, requires_grad, as_array=False)
+ x.requires_grad = not requires_grad
+ ivy.utils.assertions.check_equal(x.requires_grad, not requires_grad, as_array=False)
ivy.previous_backend()
-# reciprocal
@handle_frontend_method(
class_tree=CLASS_TREE,
init_tree="torch.tensor",
- method_name="reciprocal",
- dtype_and_x=helpers.dtype_and_values(
- available_dtypes=helpers.get_dtypes("float"),
- min_value=1,
+ method_name="reshape",
+ dtype_x=helpers.dtype_and_values(
+ available_dtypes=helpers.get_dtypes("valid"),
+ shape=st.shared(helpers.get_shape(), key="value_shape"),
+ ),
+ shape=helpers.reshape_shapes(
+ shape=st.shared(helpers.get_shape(), key="value_shape")
),
+ unpack_shape=st.booleans(),
)
-def test_torch_tensor_reciprocal(
- dtype_and_x,
+def test_torch_reshape(
+ dtype_x,
+ shape,
+ unpack_shape,
frontend_method_data,
init_flags,
method_flags,
@@ -10766,7 +10968,16 @@ def test_torch_tensor_reciprocal(
on_device,
backend_fw,
):
- input_dtype, x = dtype_and_x
+ input_dtype, x = dtype_x
+ shape = {
+ "shape": shape,
+ }
+ if unpack_shape:
+ method_flags.num_positional_args = len(shape["shape"]) + 1
+ i = 0
+ for x_ in shape["shape"]:
+ shape[f"x{i}"] = x_
+ i += 1
helpers.test_frontend_method(
init_input_dtypes=input_dtype,
backend_to_test=backend_fw,
@@ -10774,7 +10985,7 @@ def test_torch_tensor_reciprocal(
"data": x[0],
},
method_input_dtypes=input_dtype,
- method_all_as_kwargs_np={},
+ method_all_as_kwargs_np=shape,
frontend_method_data=frontend_method_data,
init_flags=init_flags,
method_flags=method_flags,
@@ -10783,19 +10994,17 @@ def test_torch_tensor_reciprocal(
)
-# reciprocal_
+# reshape_as
@handle_frontend_method(
class_tree=CLASS_TREE,
init_tree="torch.tensor",
- method_name="reciprocal_",
- dtype_and_x=helpers.dtype_and_values(
- available_dtypes=helpers.get_dtypes("valid"),
- min_value=1,
+ method_name="reshape_as",
+ dtype_x=helpers.dtype_and_values(
+ available_dtypes=helpers.get_dtypes("valid"), num_arrays=2
),
- test_inplace=st.just(True),
)
-def test_torch_tensor_reciprocal_(
- dtype_and_x,
+def test_torch_reshape_as(
+ dtype_x,
frontend_method_data,
init_flags,
method_flags,
@@ -10803,7 +11012,7 @@ def test_torch_tensor_reciprocal_(
on_device,
backend_fw,
):
- input_dtype, x = dtype_and_x
+ input_dtype, x = dtype_x
helpers.test_frontend_method(
init_input_dtypes=input_dtype,
backend_to_test=backend_fw,
@@ -10811,7 +11020,9 @@ def test_torch_tensor_reciprocal_(
"data": x[0],
},
method_input_dtypes=input_dtype,
- method_all_as_kwargs_np={},
+ method_all_as_kwargs_np={
+ "other": x[1],
+ },
frontend_method_data=frontend_method_data,
init_flags=init_flags,
method_flags=method_flags,
@@ -10820,18 +11031,19 @@ def test_torch_tensor_reciprocal_(
)
-# relu
+# round
@handle_frontend_method(
class_tree=CLASS_TREE,
init_tree="torch.tensor",
- method_name="relu",
+ method_name="round",
dtype_and_x=helpers.dtype_and_values(
available_dtypes=helpers.get_dtypes("float"),
- allow_inf=False,
),
+ decimals=st.integers(min_value=0, max_value=5),
)
-def test_torch_tensor_relu(
+def test_torch_round(
dtype_and_x,
+ decimals,
frontend_method_data,
init_flags,
method_flags,
@@ -10847,7 +11059,9 @@ def test_torch_tensor_relu(
"data": x[0],
},
method_input_dtypes=input_dtype,
- method_all_as_kwargs_np={},
+ method_all_as_kwargs_np={
+ "decimals": decimals,
+ },
frontend_method_data=frontend_method_data,
init_flags=init_flags,
method_flags=method_flags,
@@ -10856,21 +11070,20 @@ def test_torch_tensor_relu(
)
-# remainder
+# round_
@handle_frontend_method(
class_tree=CLASS_TREE,
init_tree="torch.tensor",
- method_name="remainder",
+ method_name="round_",
dtype_and_x=helpers.dtype_and_values(
available_dtypes=helpers.get_dtypes("float"),
- large_abs_safety_factor=2.5,
- small_abs_safety_factor=2.5,
- shared_dtype=True,
- num_arrays=2,
),
+ decimals=st.integers(min_value=0, max_value=5),
+ test_inplace=st.just(True),
)
-def test_torch_tensor_remainder(
+def test_torch_round_(
dtype_and_x,
+ decimals,
frontend_method_data,
init_flags,
method_flags,
@@ -10887,7 +11100,7 @@ def test_torch_tensor_remainder(
},
method_input_dtypes=input_dtype,
method_all_as_kwargs_np={
- "other": x[1],
+ "decimals": decimals,
},
frontend_method_data=frontend_method_data,
init_flags=init_flags,
@@ -10897,23 +11110,16 @@ def test_torch_tensor_remainder(
)
-# remainder_
+# rsqrt
@handle_frontend_method(
class_tree=CLASS_TREE,
init_tree="torch.tensor",
- method_name="remainder_",
+ method_name="rsqrt",
dtype_and_x=helpers.dtype_and_values(
- available_dtypes=helpers.get_dtypes("valid"),
- min_value=-1e04,
- max_value=1e04,
- large_abs_safety_factor=2.5,
- small_abs_safety_factor=2.5,
- shared_dtype=True,
- num_arrays=2,
+ available_dtypes=helpers.get_dtypes("float"),
),
- test_inplace=st.just(True),
)
-def test_torch_tensor_remainder_(
+def test_torch_rsqrt(
dtype_and_x,
frontend_method_data,
init_flags,
@@ -10930,9 +11136,7 @@ def test_torch_tensor_remainder_(
"data": x[0],
},
method_input_dtypes=input_dtype,
- method_all_as_kwargs_np={
- "other": x[1],
- },
+ method_all_as_kwargs_np={},
frontend_method_data=frontend_method_data,
init_flags=init_flags,
method_flags=method_flags,
@@ -10941,17 +11145,18 @@ def test_torch_tensor_remainder_(
)
-# repeat
+# rsqrt_
@handle_frontend_method(
class_tree=CLASS_TREE,
init_tree="torch.tensor",
- method_name="repeat",
- dtype_x_repeats=_repeat_helper(),
- unpack_repeat=st.booleans(),
+ method_name="rsqrt_",
+ dtype_and_x=helpers.dtype_and_values(
+ available_dtypes=helpers.get_dtypes("float"),
+ ),
+ test_inplace=st.just(True),
)
-def test_torch_tensor_repeat(
- dtype_x_repeats,
- unpack_repeat,
+def test_torch_rsqrt_(
+ dtype_and_x,
frontend_method_data,
init_flags,
method_flags,
@@ -10959,243 +11164,250 @@ def test_torch_tensor_repeat(
on_device,
backend_fw,
):
- input_dtype, x, repeats = dtype_x_repeats
- repeat = {
- "repeats": repeats,
- }
- if unpack_repeat:
- method_flags.num_positional_args = len(repeat["repeats"]) + 1
- for i, x_ in enumerate(repeat["repeats"]):
- repeat[f"x{i}"] = x_
+ input_dtype, x = dtype_and_x
helpers.test_frontend_method(
init_input_dtypes=input_dtype,
backend_to_test=backend_fw,
init_all_as_kwargs_np={
"data": x[0],
},
- method_input_dtypes=input_dtype,
- method_all_as_kwargs_np=repeat,
+ method_input_dtypes=input_dtype,
+ method_all_as_kwargs_np={},
+ frontend_method_data=frontend_method_data,
+ init_flags=init_flags,
+ method_flags=method_flags,
+ frontend=frontend,
+ on_device=on_device,
+ )
+
+
+# scatter
+@handle_frontend_method(
+ class_tree=CLASS_TREE,
+ init_tree="torch.tensor",
+ method_name="scatter",
+ args=put_along_axis_helper(),
+)
+def test_torch_scatter(
+ args,
+ frontend,
+ frontend_method_data,
+ init_flags,
+ method_flags,
+ on_device,
+ backend_fw,
+):
+ input_dtypes, x, indices, values, axis = args
+ helpers.test_frontend_method(
+ init_input_dtypes=[input_dtypes[0]],
+ backend_to_test=backend_fw,
+ init_all_as_kwargs_np={
+ "data": x,
+ },
+ method_input_dtypes=["int64", input_dtypes[0]],
+ method_all_as_kwargs_np={
+ "dim": axis,
+ "index": indices,
+ "src": values,
+ },
+ frontend=frontend,
frontend_method_data=frontend_method_data,
init_flags=init_flags,
method_flags=method_flags,
- frontend=frontend,
on_device=on_device,
)
-@given(
- dtype_x=helpers.dtype_and_values(
- available_dtypes=helpers.get_dtypes("valid", prune_function=False),
- ),
- requires_grad=st.booleans(),
-)
-def test_torch_tensor_requires_grad(dtype_x, requires_grad, backend_fw):
- ivy.set_backend(backend_fw)
- _, data = dtype_x
- x = Tensor(data[0], requires_grad=requires_grad)
- ivy.utils.assertions.check_equal(x.requires_grad, requires_grad, as_array=False)
- x.requires_grad = not requires_grad
- ivy.utils.assertions.check_equal(x.requires_grad, not requires_grad, as_array=False)
- ivy.previous_backend()
-
-
+# scatter_
@handle_frontend_method(
class_tree=CLASS_TREE,
init_tree="torch.tensor",
- method_name="reshape",
- dtype_x=helpers.dtype_and_values(
- available_dtypes=helpers.get_dtypes("valid"),
- shape=st.shared(helpers.get_shape(), key="value_shape"),
- ),
- shape=helpers.reshape_shapes(
- shape=st.shared(helpers.get_shape(), key="value_shape")
- ),
- unpack_shape=st.booleans(),
+ method_name="scatter_",
+ args=put_along_axis_helper(),
+ reduce=st.sampled_from(["add", "multiply"]),
)
-def test_torch_tensor_reshape(
- dtype_x,
- shape,
- unpack_shape,
+def test_torch_scatter_(
+ args,
+ reduce,
+ frontend,
frontend_method_data,
init_flags,
method_flags,
- frontend,
on_device,
backend_fw,
):
- input_dtype, x = dtype_x
- shape = {
- "shape": shape,
- }
- if unpack_shape:
- method_flags.num_positional_args = len(shape["shape"]) + 1
- i = 0
- for x_ in shape["shape"]:
- shape[f"x{i}"] = x_
- i += 1
+ input_dtypes, x, indices, values, axis = args
helpers.test_frontend_method(
- init_input_dtypes=input_dtype,
+ init_input_dtypes=[input_dtypes[0]],
backend_to_test=backend_fw,
init_all_as_kwargs_np={
- "data": x[0],
+ "data": x,
},
- method_input_dtypes=input_dtype,
- method_all_as_kwargs_np=shape,
+ method_input_dtypes=["int64", input_dtypes[0]],
+ method_all_as_kwargs_np={
+ "dim": axis,
+ "index": indices,
+ "src": values,
+ "reduce": reduce,
+ },
+ frontend=frontend,
frontend_method_data=frontend_method_data,
init_flags=init_flags,
method_flags=method_flags,
- frontend=frontend,
on_device=on_device,
)
-# reshape_as
+# scatter_add
@handle_frontend_method(
class_tree=CLASS_TREE,
init_tree="torch.tensor",
- method_name="reshape_as",
- dtype_x=helpers.dtype_and_values(
- available_dtypes=helpers.get_dtypes("valid"), num_arrays=2
- ),
+ method_name="scatter_add",
+ args=put_along_axis_helper(),
)
-def test_torch_tensor_reshape_as(
- dtype_x,
+def test_torch_scatter_add(
+ args,
+ frontend,
frontend_method_data,
init_flags,
method_flags,
- frontend,
on_device,
backend_fw,
):
- input_dtype, x = dtype_x
+ input_dtypes, x, indices, values, axis = args
helpers.test_frontend_method(
- init_input_dtypes=input_dtype,
+ init_input_dtypes=[input_dtypes[0]],
backend_to_test=backend_fw,
init_all_as_kwargs_np={
- "data": x[0],
+ "data": x,
},
- method_input_dtypes=input_dtype,
+ method_input_dtypes=["int64", input_dtypes[0]],
method_all_as_kwargs_np={
- "other": x[1],
+ "dim": axis,
+ "index": indices,
+ "src": values,
},
+ frontend=frontend,
frontend_method_data=frontend_method_data,
init_flags=init_flags,
method_flags=method_flags,
- frontend=frontend,
on_device=on_device,
)
-# round
+# scatter_add_
@handle_frontend_method(
class_tree=CLASS_TREE,
init_tree="torch.tensor",
- method_name="round",
- dtype_and_x=helpers.dtype_and_values(
- available_dtypes=helpers.get_dtypes("float"),
- ),
- decimals=st.integers(min_value=0, max_value=5),
+ method_name="scatter_add_",
+ args=put_along_axis_helper(),
)
-def test_torch_tensor_round(
- dtype_and_x,
- decimals,
+def test_torch_scatter_add_(
+ args,
+ frontend,
frontend_method_data,
init_flags,
method_flags,
- frontend,
on_device,
backend_fw,
):
- input_dtype, x = dtype_and_x
+ input_dtypes, x, indices, values, axis = args
helpers.test_frontend_method(
- init_input_dtypes=input_dtype,
+ init_input_dtypes=[input_dtypes[0]],
backend_to_test=backend_fw,
init_all_as_kwargs_np={
- "data": x[0],
+ "data": x,
},
- method_input_dtypes=input_dtype,
+ method_input_dtypes=["int64", input_dtypes[0]],
method_all_as_kwargs_np={
- "decimals": decimals,
+ "dim": axis,
+ "index": indices,
+ "src": values,
},
+ frontend=frontend,
frontend_method_data=frontend_method_data,
init_flags=init_flags,
method_flags=method_flags,
- frontend=frontend,
on_device=on_device,
)
-# round_
+# scatter_reduce
@handle_frontend_method(
class_tree=CLASS_TREE,
init_tree="torch.tensor",
- method_name="round_",
- dtype_and_x=helpers.dtype_and_values(
- available_dtypes=helpers.get_dtypes("float"),
- ),
- decimals=st.integers(min_value=0, max_value=5),
- test_inplace=st.just(True),
+ method_name="scatter_reduce",
+ args=put_along_axis_helper(),
+ mode=st.sampled_from(["sum", "prod", "amin", "amax"]),
)
-def test_torch_tensor_round_(
- dtype_and_x,
- decimals,
+def test_torch_scatter_reduce(
+ args,
+ mode,
+ frontend,
frontend_method_data,
init_flags,
method_flags,
- frontend,
on_device,
backend_fw,
):
- input_dtype, x = dtype_and_x
+ input_dtypes, x, indices, values, axis = args
helpers.test_frontend_method(
- init_input_dtypes=input_dtype,
+ init_input_dtypes=[input_dtypes[0]],
backend_to_test=backend_fw,
init_all_as_kwargs_np={
- "data": x[0],
+ "data": x,
},
- method_input_dtypes=input_dtype,
+ method_input_dtypes=["int64", input_dtypes[0]],
method_all_as_kwargs_np={
- "decimals": decimals,
+ "dim": axis,
+ "index": indices,
+ "src": values,
+ "reduce": mode,
},
+ frontend=frontend,
frontend_method_data=frontend_method_data,
init_flags=init_flags,
method_flags=method_flags,
- frontend=frontend,
on_device=on_device,
)
-# rsqrt
+# scatter_reduce_
@handle_frontend_method(
class_tree=CLASS_TREE,
init_tree="torch.tensor",
- method_name="rsqrt",
- dtype_and_x=helpers.dtype_and_values(
- available_dtypes=helpers.get_dtypes("float"),
- ),
+ method_name="scatter_reduce_",
+ args=put_along_axis_helper(),
+ mode=st.sampled_from(["sum", "prod", "amin", "amax"]),
)
-def test_torch_tensor_rsqrt(
- dtype_and_x,
+def test_torch_scatter_reduce_(
+ args,
+ mode,
+ frontend,
frontend_method_data,
init_flags,
method_flags,
- frontend,
on_device,
backend_fw,
):
- input_dtype, x = dtype_and_x
+ input_dtypes, x, indices, values, axis = args
helpers.test_frontend_method(
- init_input_dtypes=input_dtype,
+ init_input_dtypes=[input_dtypes[0]],
backend_to_test=backend_fw,
init_all_as_kwargs_np={
- "data": x[0],
+ "data": x,
},
- method_input_dtypes=input_dtype,
- method_all_as_kwargs_np={},
+ method_input_dtypes=["int64", input_dtypes[0]],
+ method_all_as_kwargs_np={
+ "dim": axis,
+ "index": indices,
+ "src": values,
+ "reduce": mode,
+ },
+ frontend=frontend,
frontend_method_data=frontend_method_data,
init_flags=init_flags,
method_flags=method_flags,
- frontend=frontend,
on_device=on_device,
)
@@ -11206,7 +11418,7 @@ def test_torch_tensor_rsqrt(
ret_shape=True,
).filter(lambda x: "bfloat16" not in x[0]),
)
-def test_torch_tensor_shape(dtype_x, backend_fw):
+def test_torch_shape(dtype_x, backend_fw):
ivy.set_backend(backend_fw)
dtype, data, shape = dtype_x
x = Tensor(data[0])
@@ -11228,7 +11440,7 @@ def test_torch_tensor_shape(dtype_x, backend_fw):
allow_inf=False,
),
)
-def test_torch_tensor_short(
+def test_torch_short(
dtype_and_x,
frontend_method_data,
init_flags,
@@ -11263,7 +11475,7 @@ def test_torch_tensor_short(
available_dtypes=helpers.get_dtypes("float"),
),
)
-def test_torch_tensor_sigmoid(
+def test_torch_sigmoid(
dtype_x,
frontend_method_data,
init_flags,
@@ -11299,7 +11511,7 @@ def test_torch_tensor_sigmoid(
),
test_inplace=st.just(True),
)
-def test_torch_tensor_sigmoid_(
+def test_torch_sigmoid_(
dtype_x,
frontend_method_data,
init_flags,
@@ -11334,7 +11546,7 @@ def test_torch_tensor_sigmoid_(
available_dtypes=helpers.get_dtypes("valid"),
),
)
-def test_torch_tensor_sign(
+def test_torch_sign(
dtype_x,
frontend,
frontend_method_data,
@@ -11368,7 +11580,7 @@ def test_torch_tensor_sign(
),
test_inplace=st.just(True),
)
-def test_torch_tensor_sign_(
+def test_torch_sign_(
dtype_x,
frontend,
frontend_method_data,
@@ -11402,7 +11614,7 @@ def test_torch_tensor_sign_(
allow_inf=False,
),
)
-def test_torch_tensor_sin(
+def test_torch_sin(
dtype_and_x,
frontend_method_data,
init_flags,
@@ -11439,7 +11651,7 @@ def test_torch_tensor_sin(
),
test_inplace=st.just(True),
)
-def test_torch_tensor_sin_(
+def test_torch_sin_(
dtype_and_x,
frontend_method_data,
init_flags,
@@ -11465,6 +11677,78 @@ def test_torch_tensor_sin_(
)
+@handle_frontend_method(
+ class_tree=CLASS_TREE,
+ init_tree="torch.tensor",
+ method_name="sinc",
+ dtype_and_x=helpers.dtype_and_values(
+ available_dtypes=helpers.get_dtypes("float"),
+ ),
+)
+def test_torch_sinc(
+ *,
+ dtype_and_x,
+ frontend,
+ backend_fw,
+ frontend_method_data,
+ init_flags,
+ method_flags,
+ on_device,
+):
+ input_dtype, x = dtype_and_x
+ helpers.test_frontend_method(
+ init_input_dtypes=input_dtype,
+ init_all_as_kwargs_np={
+ "data": x[0],
+ },
+ method_input_dtypes=input_dtype,
+ method_all_as_kwargs_np={},
+ frontend_method_data=frontend_method_data,
+ init_flags=init_flags,
+ method_flags=method_flags,
+ frontend=frontend,
+ backend_to_test=backend_fw,
+ on_device=on_device,
+ )
+
+
+# sinc_
+@handle_frontend_method(
+ class_tree=CLASS_TREE,
+ init_tree="torch.tensor",
+ method_name="sinc_",
+ dtype_and_x=helpers.dtype_and_values(
+ available_dtypes=helpers.get_dtypes("valid"),
+ ),
+ test_inplace=st.just(True),
+)
+def test_torch_sinc_(
+ *,
+ dtype_and_x,
+ frontend,
+ backend_fw,
+ frontend_method_data,
+ init_flags,
+ method_flags,
+ on_device,
+):
+ input_dtype, x = dtype_and_x
+ helpers.test_frontend_method(
+ init_input_dtypes=input_dtype,
+ init_all_as_kwargs_np={
+ "data": x[0],
+ },
+ method_input_dtypes=input_dtype,
+ method_all_as_kwargs_np={},
+ frontend_method_data=frontend_method_data,
+ init_flags=init_flags,
+ method_flags=method_flags,
+ frontend=frontend,
+ backend_to_test=backend_fw,
+ on_device=on_device,
+ )
+
+
# sinh
@handle_frontend_method(
class_tree=CLASS_TREE,
@@ -11475,7 +11759,7 @@ def test_torch_tensor_sin_(
allow_inf=False,
),
)
-def test_torch_tensor_sinh(
+def test_torch_sinh(
dtype_and_x,
frontend_method_data,
init_flags,
@@ -11512,7 +11796,7 @@ def test_torch_tensor_sinh(
),
test_inplace=st.just(True),
)
-def test_torch_tensor_sinh_(
+def test_torch_sinh_(
dtype_and_x,
frontend_method_data,
init_flags,
@@ -11552,7 +11836,7 @@ def test_torch_tensor_sinh_(
force_int=True,
),
)
-def test_torch_tensor_size(
+def test_torch_size(
dtype_and_x,
dim,
frontend_method_data,
@@ -11595,7 +11879,7 @@ def test_torch_tensor_size(
),
dtype=helpers.get_dtypes("float", full=False),
)
-def test_torch_tensor_softmax(
+def test_torch_softmax(
dtype_x_and_axis,
dtype,
frontend_method_data,
@@ -11641,7 +11925,7 @@ def test_torch_tensor_softmax(
),
descending=st.booleans(),
)
-def test_torch_tensor_sort(
+def test_torch_sort(
dtype_value,
dim,
descending,
@@ -11690,7 +11974,7 @@ def test_torch_tensor_sort(
key="target_axis",
),
)
-def test_torch_tensor_split(
+def test_torch_split(
dtype_value,
split_size,
dim,
@@ -11730,7 +12014,7 @@ def test_torch_tensor_split(
available_dtypes=helpers.get_dtypes("numeric"),
),
)
-def test_torch_tensor_sqrt(
+def test_torch_sqrt(
dtype_x,
frontend,
frontend_method_data,
@@ -11764,7 +12048,7 @@ def test_torch_tensor_sqrt(
),
test_inplace=st.just(True),
)
-def test_torch_tensor_sqrt_(
+def test_torch_sqrt_(
dtype_x,
frontend,
frontend_method_data,
@@ -11797,7 +12081,43 @@ def test_torch_tensor_sqrt_(
available_dtypes=helpers.get_dtypes("float"),
),
)
-def test_torch_tensor_square(
+def test_torch_square(
+ dtype_x,
+ frontend,
+ frontend_method_data,
+ init_flags,
+ method_flags,
+ on_device,
+ backend_fw,
+):
+ input_dtype, x = dtype_x
+ helpers.test_frontend_method(
+ init_input_dtypes=input_dtype,
+ backend_to_test=backend_fw,
+ init_all_as_kwargs_np={"data": x[0]},
+ method_input_dtypes=input_dtype,
+ method_all_as_kwargs_np={},
+ frontend_method_data=frontend_method_data,
+ init_flags=init_flags,
+ method_flags=method_flags,
+ frontend=frontend,
+ on_device=on_device,
+ )
+
+
+# square_
+@handle_frontend_method(
+ class_tree=CLASS_TREE,
+ init_tree="torch.tensor",
+ method_name="square_",
+ dtype_x=helpers.dtype_and_values(
+ available_dtypes=helpers.get_dtypes("valid"),
+ allow_inf=False,
+ max_value=1e04,
+ min_value=-1e04,
+ ),
+)
+def test_torch_square_(
dtype_x,
frontend,
frontend_method_data,
@@ -11834,7 +12154,7 @@ def test_torch_tensor_square(
shape=st.shared(helpers.get_shape(min_num_dims=1), key="shape"),
),
)
-def test_torch_tensor_squeeze(
+def test_torch_squeeze(
dtype_value_axis,
frontend_method_data,
init_flags,
@@ -11876,7 +12196,7 @@ def test_torch_tensor_squeeze(
),
test_inplace=st.just(True),
)
-def test_torch_tensor_squeeze_(
+def test_torch_squeeze_(
dtype_value_axis,
frontend_method_data,
init_flags,
@@ -11911,7 +12231,7 @@ def test_torch_tensor_squeeze_(
method_name="std",
dtype_and_x=_statistical_dtype_values(function="std"),
)
-def test_torch_tensor_std(
+def test_torch_std(
dtype_and_x,
frontend,
frontend_method_data,
@@ -11948,7 +12268,7 @@ def test_torch_tensor_std(
force_int_axis=True,
),
)
-def test_torch_tensor_stride(
+def test_torch_stride(
dtype_value_axis,
frontend,
frontend_method_data,
@@ -11986,7 +12306,7 @@ def test_torch_tensor_stride(
),
alpha=st.floats(min_value=-1e04, max_value=1e04, allow_infinity=False),
)
-def test_torch_tensor_sub(
+def test_torch_sub(
dtype_and_x,
alpha,
frontend,
@@ -12028,7 +12348,7 @@ def test_torch_tensor_sub(
),
test_inplace=st.just(True),
)
-def test_torch_tensor_subtract_(
+def test_torch_subtract_(
dtype_and_x,
frontend_method_data,
init_flags,
@@ -12067,7 +12387,7 @@ def test_torch_tensor_subtract_(
),
keepdim=st.booleans(),
)
-def test_torch_tensor_sum(
+def test_torch_sum(
dtype_x_dim,
keepdim,
frontend_method_data,
@@ -12114,7 +12434,7 @@ def test_torch_tensor_sum(
some=st.booleans(),
compute_uv=st.booleans(),
)
-def test_torch_tensor_svd(
+def test_torch_svd(
dtype_and_x,
some,
compute_uv,
@@ -12183,7 +12503,7 @@ def test_torch_tensor_svd(
shape=helpers.get_shape(min_num_dims=2, max_num_dims=2),
),
)
-def test_torch_tensor_t(
+def test_torch_t(
dtype_and_x,
frontend_method_data,
init_flags,
@@ -12223,7 +12543,7 @@ def test_torch_tensor_t(
indices_same_dims=True,
),
)
-def test_torch_tensor_take_along_dim(
+def test_torch_take_along_dim(
dtype_indices_axis,
frontend_method_data,
init_flags,
@@ -12262,7 +12582,7 @@ def test_torch_tensor_take_along_dim(
allow_inf=False,
),
)
-def test_torch_tensor_tan(
+def test_torch_tan(
dtype_and_x,
frontend_method_data,
init_flags,
@@ -12299,7 +12619,7 @@ def test_torch_tensor_tan(
),
test_inplace=st.just(True),
)
-def test_torch_tensor_tan_(
+def test_torch_tan_(
dtype_and_x,
frontend_method_data,
init_flags,
@@ -12335,7 +12655,7 @@ def test_torch_tensor_tan_(
allow_inf=False,
),
)
-def test_torch_tensor_tanh(
+def test_torch_tanh(
dtype_and_x,
frontend_method_data,
init_flags,
@@ -12372,7 +12692,80 @@ def test_torch_tensor_tanh(
),
test_inplace=st.just(True),
)
-def test_torch_tensor_tanh_(
+def test_torch_tanh_(
+ dtype_and_x,
+ frontend_method_data,
+ init_flags,
+ method_flags,
+ frontend,
+ on_device,
+ backend_fw,
+):
+ input_dtype, x = dtype_and_x
+ helpers.test_frontend_method(
+ init_input_dtypes=input_dtype,
+ backend_to_test=backend_fw,
+ init_all_as_kwargs_np={
+ "data": x[0],
+ },
+ method_input_dtypes=input_dtype,
+ method_all_as_kwargs_np={},
+ frontend_method_data=frontend_method_data,
+ init_flags=init_flags,
+ method_flags=method_flags,
+ frontend=frontend,
+ on_device=on_device,
+ )
+
+
+# corrcoef
+@handle_frontend_method(
+ class_tree=CLASS_TREE,
+ init_tree="torch.tensor",
+ method_name="corrcoef",
+ dtype_and_x=helpers.dtype_and_values(
+ available_dtypes=helpers.get_dtypes("valid"),
+ ),
+)
+def test_torch_tensor_corrcoef(
+ dtype_and_x,
+ frontend,
+ backend_fw,
+ frontend_method_data,
+ init_flags,
+ method_flags,
+ on_device,
+):
+ input_dtype, x = dtype_and_x
+ helpers.test_frontend_method(
+ init_input_dtypes=input_dtype,
+ init_all_as_kwargs_np={
+ "data": x[0],
+ },
+ method_input_dtypes=input_dtype,
+ method_all_as_kwargs_np={},
+ frontend_method_data=frontend_method_data,
+ init_flags=init_flags,
+ method_flags=method_flags,
+ frontend=frontend,
+ backend_to_test=backend_fw,
+ on_device=on_device,
+ )
+
+
+# positive
+@handle_frontend_method(
+ class_tree=CLASS_TREE,
+ init_tree="torch.tensor",
+ method_name="positive",
+ dtype_and_x=helpers.dtype_and_values(
+ available_dtypes=helpers.get_dtypes("float"),
+ min_value=-1e04,
+ max_value=1e04,
+ allow_inf=False,
+ ),
+)
+def test_torch_tensor_positive(
dtype_and_x,
frontend_method_data,
init_flags,
@@ -12419,7 +12812,7 @@ def test_torch_tensor_tanh_(
),
method_num_positional_args=st.just(1),
)
-def test_torch_tensor_tensor_split(
+def test_torch_tensor_split(
dtype_value,
indices_or_sections,
dim,
@@ -12463,7 +12856,7 @@ def test_torch_tensor_tensor_split(
allow_neg=False,
),
)
-def test_torch_tensor_tile(
+def test_torch_tile(
dtype_and_values,
reps,
frontend,
@@ -12503,7 +12896,7 @@ def test_torch_tensor_tile(
method_name="to",
args_kwargs=_to_helper(),
)
-def test_torch_tensor_to(
+def test_torch_to(
args_kwargs,
frontend_method_data,
init_flags,
@@ -12541,7 +12934,7 @@ def test_torch_tensor_to(
largest=st.booleans(),
sorted=st.booleans(),
)
-def test_torch_tensor_topk(
+def test_torch_topk(
dtype_x_axis_k,
largest,
sorted,
@@ -12593,7 +12986,7 @@ def test_torch_tensor_topk(
force_int=True,
),
)
-def test_torch_tensor_transpose(
+def test_torch_transpose(
dtype_value,
dim0,
dim1,
@@ -12641,7 +13034,7 @@ def test_torch_tensor_transpose(
force_int=True,
),
)
-def test_torch_tensor_transpose_(
+def test_torch_transpose_(
dtype_value,
dim0,
dim1,
@@ -12683,7 +13076,7 @@ def test_torch_tensor_transpose_(
),
diagonal=st.integers(min_value=-100, max_value=100),
)
-def test_torch_tensor_tril(
+def test_torch_tril(
dtype_and_values,
diagonal,
frontend_method_data,
@@ -12724,7 +13117,7 @@ def test_torch_tensor_tril(
diagonal=st.integers(min_value=-100, max_value=100),
test_inplace=st.just(True),
)
-def test_torch_tensor_tril_(
+def test_torch_tril_(
dtype_and_values,
diagonal,
frontend_method_data,
@@ -12753,6 +13146,90 @@ def test_torch_tensor_tril_(
)
+# triu
+@handle_frontend_method(
+ class_tree=CLASS_TREE,
+ init_tree="torch.tensor",
+ method_name="triu",
+ dtype_x=helpers.dtype_and_values(
+ available_dtypes=helpers.get_dtypes("numeric"),
+ min_num_dims=2,
+ max_num_dims=5,
+ min_dim_size=1,
+ max_dim_size=5,
+ ),
+ diagonal=st.integers(
+ min_value=-4,
+ max_value=4,
+ ),
+)
+def test_torch_triu(
+ dtype_x,
+ diagonal,
+ frontend,
+ frontend_method_data,
+ init_flags,
+ method_flags,
+ on_device,
+ backend_fw,
+):
+ input_dtype, x = dtype_x
+ helpers.test_frontend_method(
+ init_input_dtypes=input_dtype,
+ backend_to_test=backend_fw,
+ init_all_as_kwargs_np={"data": x[0]},
+ method_input_dtypes=input_dtype,
+ method_all_as_kwargs_np={"diagonal": diagonal},
+ frontend_method_data=frontend_method_data,
+ init_flags=init_flags,
+ method_flags=method_flags,
+ frontend=frontend,
+ on_device=on_device,
+ )
+
+
+# triu_
+@handle_frontend_method(
+ class_tree=CLASS_TREE,
+ init_tree="torch.tensor",
+ method_name="triu_",
+ dtype_x=helpers.dtype_and_values(
+ available_dtypes=helpers.get_dtypes("valid"),
+ min_num_dims=2,
+ max_num_dims=5,
+ min_dim_size=1,
+ max_dim_size=5,
+ ),
+ diagonal=st.integers(
+ min_value=-4,
+ max_value=4,
+ ),
+)
+def test_torch_triu_(
+ dtype_x,
+ diagonal,
+ frontend,
+ frontend_method_data,
+ init_flags,
+ method_flags,
+ on_device,
+ backend_fw,
+):
+ input_dtype, x = dtype_x
+ helpers.test_frontend_method(
+ init_input_dtypes=input_dtype,
+ backend_to_test=backend_fw,
+ init_all_as_kwargs_np={"data": x[0]},
+ method_input_dtypes=input_dtype,
+ method_all_as_kwargs_np={"diagonal": diagonal},
+ frontend_method_data=frontend_method_data,
+ init_flags=init_flags,
+ method_flags=method_flags,
+ frontend=frontend,
+ on_device=on_device,
+ )
+
+
# true_divide_
@handle_frontend_method(
class_tree=CLASS_TREE,
@@ -12767,7 +13244,7 @@ def test_torch_tensor_tril_(
),
test_inplace=st.just(True),
)
-def test_torch_tensor_true_divide_(
+def test_torch_true_divide_(
dtype_and_x,
frontend,
frontend_method_data,
@@ -12805,7 +13282,7 @@ def test_torch_tensor_true_divide_(
shape=st.shared(helpers.get_shape(min_num_dims=1), key="shape"),
),
)
-def test_torch_tensor_trunc(
+def test_torch_trunc(
dtype_value,
frontend_method_data,
init_flags,
@@ -12842,7 +13319,7 @@ def test_torch_tensor_trunc(
),
test_inplace=st.just(True),
)
-def test_torch_tensor_trunc_(
+def test_torch_trunc_(
dtype_value,
frontend_method_data,
init_flags,
@@ -12878,7 +13355,7 @@ def test_torch_tensor_trunc_(
),
dtype=helpers.get_dtypes("valid", full=False),
)
-def test_torch_tensor_type(
+def test_torch_type(
dtype_and_x,
dtype,
frontend_method_data,
@@ -12917,8 +13394,83 @@ def test_torch_tensor_type(
num_arrays=2,
),
)
-def test_torch_tensor_type_as(
- dtype_and_x,
+def test_torch_type_as(
+ dtype_and_x,
+ frontend_method_data,
+ init_flags,
+ method_flags,
+ frontend,
+ on_device,
+ backend_fw,
+):
+ input_dtype, x = dtype_and_x
+ helpers.test_frontend_method(
+ init_input_dtypes=input_dtype,
+ backend_to_test=backend_fw,
+ init_all_as_kwargs_np={
+ "data": x[0],
+ },
+ method_input_dtypes=input_dtype,
+ method_all_as_kwargs_np={
+ "other": x[1],
+ },
+ frontend_method_data=frontend_method_data,
+ init_flags=init_flags,
+ method_flags=method_flags,
+ frontend=frontend,
+ on_device=on_device,
+ )
+
+
+# unbind
+@handle_frontend_method(
+ class_tree=CLASS_TREE,
+ init_tree="torch.tensor",
+ method_name="unbind",
+ dtype_value_axis=helpers.dtype_values_axis(
+ available_dtypes=helpers.get_dtypes("numeric"),
+ min_num_dims=1,
+ valid_axis=True,
+ force_int_axis=True,
+ ),
+)
+def test_torch_unbind(
+ dtype_value_axis,
+ frontend_method_data,
+ init_flags,
+ method_flags,
+ frontend,
+ on_device,
+ backend_fw,
+):
+ input_dtypes, x, axis = dtype_value_axis
+ helpers.test_frontend_method(
+ init_input_dtypes=input_dtypes,
+ backend_to_test=backend_fw,
+ init_all_as_kwargs_np={
+ "data": x[0],
+ },
+ method_input_dtypes=input_dtypes,
+ method_all_as_kwargs_np={
+ "dim": axis,
+ },
+ frontend_method_data=frontend_method_data,
+ init_flags=init_flags,
+ method_flags=method_flags,
+ frontend=frontend,
+ on_device=on_device,
+ )
+
+
+# unfold
+@handle_frontend_method(
+ class_tree=CLASS_TREE,
+ init_tree="torch.tensor",
+ method_name="unfold",
+ dtype_values_args=_unfold_args(),
+)
+def test_torch_unfold(
+ dtype_values_args,
frontend_method_data,
init_flags,
method_flags,
@@ -12926,16 +13478,19 @@ def test_torch_tensor_type_as(
on_device,
backend_fw,
):
- input_dtype, x = dtype_and_x
+ input_dtype, x, axis, size, step = dtype_values_args
+ print(axis, size, step)
helpers.test_frontend_method(
init_input_dtypes=input_dtype,
backend_to_test=backend_fw,
init_all_as_kwargs_np={
- "data": x[0],
+ "data": x,
},
method_input_dtypes=input_dtype,
method_all_as_kwargs_np={
- "other": x[1],
+ "dimension": axis,
+ "size": size,
+ "step": step,
},
frontend_method_data=frontend_method_data,
init_flags=init_flags,
@@ -12945,36 +13500,42 @@ def test_torch_tensor_type_as(
)
-# unbind
+# unique
@handle_frontend_method(
class_tree=CLASS_TREE,
init_tree="torch.tensor",
- method_name="unbind",
- dtype_value_axis=helpers.dtype_values_axis(
- available_dtypes=helpers.get_dtypes("numeric"),
- min_num_dims=1,
+ method_name="unique",
+ dtype_x_axis=helpers.dtype_values_axis(
+ available_dtypes=helpers.get_dtypes("valid"),
valid_axis=True,
force_int_axis=True,
),
+ sorted=st.booleans(),
+ return_inverse=st.booleans(),
+ return_counts=st.booleans(),
)
-def test_torch_tensor_unbind(
- dtype_value_axis,
+def test_torch_unique(
+ dtype_x_axis,
+ sorted,
+ return_inverse,
+ return_counts,
+ frontend,
frontend_method_data,
init_flags,
method_flags,
- frontend,
on_device,
backend_fw,
):
- input_dtypes, x, axis = dtype_value_axis
+ input_dtype, x, axis = dtype_x_axis
helpers.test_frontend_method(
- init_input_dtypes=input_dtypes,
+ init_input_dtypes=input_dtype,
backend_to_test=backend_fw,
- init_all_as_kwargs_np={
- "data": x[0],
- },
- method_input_dtypes=input_dtypes,
+ init_all_as_kwargs_np={"data": x[0]},
+ method_input_dtypes=input_dtype,
method_all_as_kwargs_np={
+ "sorted": sorted,
+ "return_inverse": return_inverse,
+ "return_counts": return_counts,
"dim": axis,
},
frontend_method_data=frontend_method_data,
@@ -12985,35 +13546,42 @@ def test_torch_tensor_unbind(
)
-# unfold
+# unique_consecutive
@handle_frontend_method(
class_tree=CLASS_TREE,
init_tree="torch.tensor",
- method_name="unfold",
- dtype_values_args=_unfold_args(),
+ method_name="unique_consecutive",
+ dtype_x_axis=helpers.dtype_values_axis(
+ available_dtypes=helpers.get_dtypes("valid"),
+ min_num_dims=2,
+ min_dim_size=2,
+ force_int_axis=True,
+ valid_axis=True,
+ ),
+ return_inverse=st.booleans(),
+ return_counts=st.booleans(),
)
-def test_torch_tensor_unfold(
- dtype_values_args,
+def test_torch_unique_consecutive(
+ dtype_x_axis,
+ return_inverse,
+ return_counts,
+ frontend,
frontend_method_data,
init_flags,
method_flags,
- frontend,
on_device,
backend_fw,
):
- input_dtype, x, axis, size, step = dtype_values_args
- print(axis, size, step)
+ input_dtype, x, axis = dtype_x_axis
helpers.test_frontend_method(
init_input_dtypes=input_dtype,
backend_to_test=backend_fw,
- init_all_as_kwargs_np={
- "data": x,
- },
+ init_all_as_kwargs_np={"data": x[0]},
method_input_dtypes=input_dtype,
method_all_as_kwargs_np={
- "dimension": axis,
- "size": size,
- "step": step,
+ "return_inverse": return_inverse,
+ "return_counts": return_counts,
+ "dim": axis,
},
frontend_method_data=frontend_method_data,
init_flags=init_flags,
@@ -13038,7 +13606,7 @@ def test_torch_tensor_unfold(
force_int=True,
),
)
-def test_torch_tensor_unsqueeze(
+def test_torch_unsqueeze(
dtype_value,
dim,
frontend_method_data,
@@ -13083,7 +13651,7 @@ def test_torch_tensor_unsqueeze(
),
test_inplace=st.just(True),
)
-def test_torch_tensor_unsqueeze_(
+def test_torch_unsqueeze_(
dtype_value,
dim,
frontend_method_data,
@@ -13123,7 +13691,7 @@ def test_torch_tensor_unsqueeze_(
),
keepdim=st.booleans(),
)
-def test_torch_tensor_var(
+def test_torch_var(
dtype_and_x,
keepdim,
frontend,
@@ -13165,7 +13733,7 @@ def test_torch_tensor_var(
shape=st.shared(helpers.get_shape(min_num_dims=1), key="value_shape")
),
)
-def test_torch_tensor_view(
+def test_torch_view(
dtype_x,
shape,
frontend_method_data,
@@ -13205,7 +13773,7 @@ def test_torch_tensor_view(
num_arrays=2,
),
)
-def test_torch_tensor_view_as(
+def test_torch_view_as(
dtype_x,
frontend_method_data,
init_flags,
@@ -13250,7 +13818,7 @@ def test_torch_tensor_view_as(
is_mod_split=True,
),
)
-def test_torch_tensor_vsplit(
+def test_torch_vsplit(
dtype_value,
indices_or_sections,
frontend_method_data,
@@ -13284,7 +13852,7 @@ def test_torch_tensor_vsplit(
method_name="where",
broadcastables=_broadcastable_trio(),
)
-def test_torch_tensor_where(
+def test_torch_where(
broadcastables,
frontend_method_data,
init_flags,
@@ -13327,7 +13895,7 @@ def test_torch_tensor_where(
shared_dtype=True,
),
)
-def test_torch_tensor_xlogy(
+def test_torch_xlogy(
dtype_and_x,
frontend,
backend_fw,
@@ -13370,7 +13938,7 @@ def test_torch_tensor_xlogy(
),
test_inplace=st.just(True),
)
-def test_torch_tensor_xlogy_(
+def test_torch_xlogy_(
dtype_and_x,
frontend,
backend_fw,
@@ -13409,7 +13977,7 @@ def test_torch_tensor_xlogy_(
),
test_inplace=st.just(True),
)
-def test_torch_tensor_zero_(
+def test_torch_zero_(
dtype_and_x,
frontend_method_data,
init_flags,
@@ -13433,178 +14001,3 @@ def test_torch_tensor_zero_(
frontend=frontend,
on_device=on_device,
)
-
-
-# triu
-@handle_frontend_method(
- class_tree=CLASS_TREE,
- init_tree="torch.tensor",
- method_name="triu",
- dtype_x=helpers.dtype_and_values(
- available_dtypes=helpers.get_dtypes("numeric"),
- min_num_dims=2,
- max_num_dims=5,
- min_dim_size=1,
- max_dim_size=5,
- ),
- diagonal=st.integers(
- min_value=-4,
- max_value=4,
- ),
-)
-def test_torch_triu(
- dtype_x,
- diagonal,
- frontend,
- frontend_method_data,
- init_flags,
- method_flags,
- on_device,
- backend_fw,
-):
- input_dtype, x = dtype_x
- helpers.test_frontend_method(
- init_input_dtypes=input_dtype,
- backend_to_test=backend_fw,
- init_all_as_kwargs_np={"data": x[0]},
- method_input_dtypes=input_dtype,
- method_all_as_kwargs_np={"diagonal": diagonal},
- frontend_method_data=frontend_method_data,
- init_flags=init_flags,
- method_flags=method_flags,
- frontend=frontend,
- on_device=on_device,
- )
-
-
-# triu_
-@handle_frontend_method(
- class_tree=CLASS_TREE,
- init_tree="torch.tensor",
- method_name="triu_",
- dtype_x=helpers.dtype_and_values(
- available_dtypes=helpers.get_dtypes("valid"),
- min_num_dims=2,
- max_num_dims=5,
- min_dim_size=1,
- max_dim_size=5,
- ),
- diagonal=st.integers(
- min_value=-4,
- max_value=4,
- ),
-)
-def test_torch_triu_(
- dtype_x,
- diagonal,
- frontend,
- frontend_method_data,
- init_flags,
- method_flags,
- on_device,
- backend_fw,
-):
- input_dtype, x = dtype_x
- helpers.test_frontend_method(
- init_input_dtypes=input_dtype,
- backend_to_test=backend_fw,
- init_all_as_kwargs_np={"data": x[0]},
- method_input_dtypes=input_dtype,
- method_all_as_kwargs_np={"diagonal": diagonal},
- frontend_method_data=frontend_method_data,
- init_flags=init_flags,
- method_flags=method_flags,
- frontend=frontend,
- on_device=on_device,
- )
-
-
-# unique
-@handle_frontend_method(
- class_tree=CLASS_TREE,
- init_tree="torch.tensor",
- method_name="unique",
- dtype_x_axis=helpers.dtype_values_axis(
- available_dtypes=helpers.get_dtypes("valid"),
- valid_axis=True,
- force_int_axis=True,
- ),
- sorted=st.booleans(),
- return_inverse=st.booleans(),
- return_counts=st.booleans(),
-)
-def test_torch_unique(
- dtype_x_axis,
- sorted,
- return_inverse,
- return_counts,
- frontend,
- frontend_method_data,
- init_flags,
- method_flags,
- on_device,
- backend_fw,
-):
- input_dtype, x, axis = dtype_x_axis
- helpers.test_frontend_method(
- init_input_dtypes=input_dtype,
- backend_to_test=backend_fw,
- init_all_as_kwargs_np={"data": x[0]},
- method_input_dtypes=input_dtype,
- method_all_as_kwargs_np={
- "sorted": sorted,
- "return_inverse": return_inverse,
- "return_counts": return_counts,
- "dim": axis,
- },
- frontend_method_data=frontend_method_data,
- init_flags=init_flags,
- method_flags=method_flags,
- frontend=frontend,
- on_device=on_device,
- )
-
-
-# unique_consecutive
-@handle_frontend_method(
- class_tree=CLASS_TREE,
- init_tree="torch.tensor",
- method_name="unique_consecutive",
- dtype_x_axis=helpers.dtype_values_axis(
- available_dtypes=helpers.get_dtypes("valid"),
- min_num_dims=2,
- min_dim_size=2,
- force_int_axis=True,
- valid_axis=True,
- ),
- return_inverse=st.booleans(),
- return_counts=st.booleans(),
-)
-def test_torch_unique_consecutive(
- dtype_x_axis,
- return_inverse,
- return_counts,
- frontend,
- frontend_method_data,
- init_flags,
- method_flags,
- on_device,
- backend_fw,
-):
- input_dtype, x, axis = dtype_x_axis
- helpers.test_frontend_method(
- init_input_dtypes=input_dtype,
- backend_to_test=backend_fw,
- init_all_as_kwargs_np={"data": x[0]},
- method_input_dtypes=input_dtype,
- method_all_as_kwargs_np={
- "return_inverse": return_inverse,
- "return_counts": return_counts,
- "dim": axis,
- },
- frontend_method_data=frontend_method_data,
- init_flags=init_flags,
- method_flags=method_flags,
- frontend=frontend,
- on_device=on_device,
- )
diff --git a/ivy_tests/test_ivy/test_frontends/test_torch/test_utilities.py b/ivy_tests/test_ivy/test_frontends/test_torch/test_utilities.py
index 6956ffa3f2a68..dfb129e991ba2 100644
--- a/ivy_tests/test_ivy/test_frontends/test_torch/test_utilities.py
+++ b/ivy_tests/test_ivy/test_frontends/test_torch/test_utilities.py
@@ -50,7 +50,7 @@ def _elemwise_helper(draw):
# ------------ #
-# ToDo: Fix this test after torch overide of assert is implemented
+# ToDo: Fix this test after torch override of assert is implemented
# @handle_frontend_test(
# fn_tree="torch._assert",
# dtype_and_x=helpers.dtype_and_values(
diff --git a/ivy_tests/test_ivy/test_frontends/test_torchvision/__init__.py b/ivy_tests/test_ivy/test_frontends/test_torchvision/__init__.py
new file mode 100644
index 0000000000000..e69de29bb2d1d
diff --git a/ivy_tests/test_ivy/test_frontends/test_torchvision/conftest.py b/ivy_tests/test_ivy/test_frontends/test_torchvision/conftest.py
new file mode 100644
index 0000000000000..81d643e34538a
--- /dev/null
+++ b/ivy_tests/test_ivy/test_frontends/test_torchvision/conftest.py
@@ -0,0 +1,6 @@
+import pytest
+
+
+@pytest.fixture(scope="session")
+def frontend():
+ return "torchvision"
diff --git a/ivy_tests/test_ivy/test_frontends/test_torchvision/test_ops.py b/ivy_tests/test_ivy/test_frontends/test_torchvision/test_ops.py
new file mode 100644
index 0000000000000..919c05a30274f
--- /dev/null
+++ b/ivy_tests/test_ivy/test_frontends/test_torchvision/test_ops.py
@@ -0,0 +1,283 @@
+# global
+import numpy as np
+from hypothesis import strategies as st
+
+
+# local
+import ivy_tests.test_ivy.helpers as helpers
+from ivy_tests.test_ivy.helpers import handle_frontend_test
+
+
+# --- Helpers --- #
+# --------------- #
+
+
+@st.composite
+def _nms_helper(draw, batched=False):
+ img_width = draw(st.integers(250, 1250))
+ img_height = draw(st.integers(250, 1250))
+ num_boxes = draw(st.integers(5, 50))
+ bbox = {}
+ for _ in range(num_boxes):
+ x1 = draw(st.integers(0, img_width - 20))
+ w = draw(st.integers(5, img_width - x1))
+ y1 = draw(st.integers(0, img_height - 20))
+ h = draw(st.integers(5, img_height - y1))
+ bbox[(x1, y1, x1 + w, y1 + h)] = draw(st.floats(0.1, 0.7))
+ iou_threshold = draw(st.floats(0.2, 0.5))
+ idxs = None
+ if batched:
+ bbox_len = len(bbox)
+ num_of_categories = draw(st.integers(1, max(bbox_len // 2, 2)))
+ idxs = np.arange(num_of_categories)
+ idxs = np.random.choice(idxs, size=bbox_len)
+ return (
+ ["float32", "float32"],
+ np.array(list(bbox.keys()), dtype=np.float32),
+ np.array(list(bbox.values()), dtype=np.float32),
+ iou_threshold,
+ idxs,
+ )
+
+
+@st.composite
+def _roi_align_helper(draw):
+ dtype = draw(helpers.get_dtypes("valid"))[0]
+ N = draw(st.integers(1, 5))
+ C = draw(st.integers(1, 5))
+ H = W = draw(st.integers(5, 20))
+
+ img_width = img_height = draw(st.integers(50, 100))
+
+ spatial_scale = H / img_height
+
+ output_size = draw(st.integers(H - 2, H + 5))
+
+ sampling_ratio = draw(st.one_of(st.just(-1), st.integers(1, 3)))
+
+ aligned = draw(st.booleans())
+ input = draw(
+ helpers.array_values(
+ dtype=dtype,
+ shape=(N, C, H, W),
+ min_value=-3,
+ max_value=3,
+ )
+ )
+ bbox = {}
+ for i in range(N):
+ num_boxes = draw(st.integers(1, 5))
+ for _ in range(num_boxes):
+ x1 = draw(st.integers(0, img_width - 20))
+ w = draw(st.integers(5, img_width - x1))
+ y1 = draw(st.integers(0, img_height - 20))
+ h = draw(st.integers(5, img_height - y1))
+ bbox[(i, x1, y1, x1 + w, y1 + h)] = 1
+
+ return (
+ [dtype],
+ input,
+ np.array(list(bbox.keys()), dtype=dtype).reshape((-1, 5)),
+ output_size,
+ spatial_scale,
+ sampling_ratio,
+ aligned,
+ )
+
+
+# --- Main --- #
+# ------------ #
+
+
+# batched_nms
+@handle_frontend_test(
+ fn_tree="torchvision.ops.batched_nms",
+ dts_boxes_scores_iou_idxs=_nms_helper(batched=True),
+ test_with_out=st.just(False),
+)
+def test_torchvision_batched_nms(
+ *,
+ dts_boxes_scores_iou_idxs,
+ on_device,
+ fn_tree,
+ frontend,
+ test_flags,
+ backend_fw,
+):
+ dts, boxes, scores, iou, idxs = dts_boxes_scores_iou_idxs
+ helpers.test_frontend_function(
+ input_dtypes=dts,
+ backend_to_test=backend_fw,
+ frontend=frontend,
+ test_flags=test_flags,
+ fn_tree=fn_tree,
+ on_device=on_device,
+ boxes=boxes,
+ scores=scores,
+ idxs=idxs,
+ iou_threshold=iou,
+ )
+
+
+# box_area
+@handle_frontend_test(
+ fn_tree="torchvision.ops.box_area",
+ boxes=helpers.dtype_and_values(
+ available_dtypes=helpers.get_dtypes("float"),
+ shape=st.tuples(helpers.ints(min_value=1, max_value=5), st.just(4)),
+ ),
+)
+def test_torchvision_box_area(
+ *,
+ boxes,
+ on_device,
+ fn_tree,
+ frontend,
+ test_flags,
+ backend_fw,
+):
+ dtype, boxes = boxes
+ helpers.test_frontend_function(
+ input_dtypes=dtype,
+ backend_to_test=backend_fw,
+ frontend=frontend,
+ test_flags=test_flags,
+ fn_tree=fn_tree,
+ on_device=on_device,
+ boxes=boxes[0],
+ )
+
+
+@handle_frontend_test(
+ fn_tree="torchvision.ops.clip_boxes_to_image",
+ boxes=helpers.dtype_and_values(
+ available_dtypes=helpers.get_dtypes("valid"),
+ shape=st.tuples(helpers.ints(min_value=1, max_value=5), st.just(4)),
+ ),
+ size=st.tuples(
+ helpers.ints(min_value=1, max_value=256),
+ helpers.ints(min_value=1, max_value=256),
+ ),
+)
+def test_torchvision_clip_boxes_to_image(
+ *,
+ boxes,
+ size,
+ on_device,
+ fn_tree,
+ frontend,
+ test_flags,
+ backend_fw,
+):
+ dtype, boxes = boxes
+ helpers.test_frontend_function(
+ input_dtypes=dtype,
+ backend_to_test=backend_fw,
+ frontend=frontend,
+ test_flags=test_flags,
+ fn_tree=fn_tree,
+ on_device=on_device,
+ boxes=boxes[0],
+ size=size,
+ )
+
+
+# nms
+@handle_frontend_test(
+ fn_tree="torchvision.ops.nms",
+ dts_boxes_scores_iou=_nms_helper(),
+ test_with_out=st.just(False),
+)
+def test_torchvision_nms(
+ *,
+ dts_boxes_scores_iou,
+ on_device,
+ fn_tree,
+ frontend,
+ test_flags,
+ backend_fw,
+):
+ dts, boxes, scores, iou, _ = dts_boxes_scores_iou
+ helpers.test_frontend_function(
+ input_dtypes=dts,
+ backend_to_test=backend_fw,
+ frontend=frontend,
+ test_flags=test_flags,
+ fn_tree=fn_tree,
+ on_device=on_device,
+ boxes=boxes,
+ scores=scores,
+ iou_threshold=iou,
+ )
+
+
+# remove_small_boxes
+@handle_frontend_test(
+ fn_tree="torchvision.ops.remove_small_boxes",
+ boxes=helpers.dtype_and_values(
+ available_dtypes=helpers.get_dtypes("valid"),
+ shape=st.tuples(helpers.ints(min_value=1, max_value=5), st.just(4)),
+ ),
+ min_size=helpers.floats(
+ min_value=0.0,
+ max_value=10,
+ small_abs_safety_factor=2,
+ large_abs_safety_factor=2,
+ safety_factor_scale="log",
+ ),
+)
+def test_torchvision_remove_small_boxes(
+ *,
+ boxes,
+ min_size,
+ on_device,
+ fn_tree,
+ frontend,
+ test_flags,
+ backend_fw,
+):
+ dtype, boxes = boxes
+ helpers.test_frontend_function(
+ input_dtypes=dtype,
+ backend_to_test=backend_fw,
+ frontend=frontend,
+ test_flags=test_flags,
+ fn_tree=fn_tree,
+ on_device=on_device,
+ boxes=boxes[0],
+ min_size=min_size,
+ )
+
+
+# roi_align
+@handle_frontend_test(
+ fn_tree="torchvision.ops.roi_align",
+ inputs=_roi_align_helper(),
+ test_with_out=st.just(False),
+)
+def test_torchvision_roi_align(
+ *,
+ inputs,
+ on_device,
+ fn_tree,
+ frontend,
+ test_flags,
+ backend_fw,
+):
+ dtypes, input, boxes, output_size, spatial_scale, sampling_ratio, aligned = inputs
+ helpers.test_frontend_function(
+ input_dtypes=dtypes,
+ backend_to_test=backend_fw,
+ frontend=frontend,
+ test_flags=test_flags,
+ fn_tree=fn_tree,
+ on_device=on_device,
+ input=input,
+ boxes=boxes,
+ output_size=output_size,
+ spatial_scale=spatial_scale,
+ sampling_ratio=sampling_ratio,
+ aligned=aligned,
+ rtol=1e-5,
+ atol=1e-5,
+ )
diff --git a/ivy_tests/test_ivy/test_functional/test_core/test_creation.py b/ivy_tests/test_ivy/test_functional/test_core/test_creation.py
index 81a2e92cba0bc..99a105e454651 100644
--- a/ivy_tests/test_ivy/test_functional/test_core/test_creation.py
+++ b/ivy_tests/test_ivy/test_functional/test_core/test_creation.py
@@ -185,6 +185,7 @@ def test_arange(
x_dtype_x_and_dtype=_asarray_helper(),
test_gradients=st.just(False),
test_instance_method=st.just(False),
+ test_with_copy=st.just(True),
)
def test_asarray(
*,
@@ -218,6 +219,7 @@ def test_asarray(
fn_tree="functional.ivy.copy_array",
dtype_and_x=helpers.dtype_and_values(available_dtypes=helpers.get_dtypes("valid")),
to_ivy_array_bool=st.booleans(),
+ test_with_copy=st.just(True),
)
def test_copy_array(
*,
diff --git a/ivy_tests/test_ivy/test_functional/test_core/test_device.py b/ivy_tests/test_ivy/test_functional/test_core/test_device.py
index ec6c8b6a84351..016373f599baa 100644
--- a/ivy_tests/test_ivy/test_functional/test_core/test_device.py
+++ b/ivy_tests/test_ivy/test_functional/test_core/test_device.py
@@ -258,7 +258,7 @@ def test_dev_util(backend_fw):
devices = _get_possible_devices()
for device in devices:
# The internally called psutil.cpu_percent() has a unique behavior where it
- # returns 0 as usageΒ when run the second time in same line so simple
+ # returns 0 as usage when run the second time in same line so simple
# assert psutil.cpu_percent() ==Β ivy.dev_util(device) isn't possible
if "cpu" in device:
assert 100 >= ivy_backend.dev_util(device) >= 0
@@ -460,7 +460,7 @@ def test_print_all_ivy_arrays_on_dev(
del item
# Apply the regex search
- assert all([re.match(regex, line) for line in written])
+ assert all(re.match(regex, line) for line in written)
# profiler
diff --git a/ivy_tests/test_ivy/test_functional/test_core/test_dtype.py b/ivy_tests/test_ivy/test_functional/test_core/test_dtype.py
index 8d1fd1f442b4a..576ab01a6e393 100644
--- a/ivy_tests/test_ivy/test_functional/test_core/test_dtype.py
+++ b/ivy_tests/test_ivy/test_functional/test_core/test_dtype.py
@@ -132,8 +132,8 @@ def test_as_ivy_dtype(
assert isinstance(res, str)
return
- assert isinstance(input_dtype, ivy_backend.Dtype) or isinstance(
- input_dtype, str
+ assert isinstance(
+ input_dtype, (ivy_backend.Dtype, str)
), f"input_dtype={input_dtype!r}, but should be str or ivy.Dtype"
assert isinstance(res, str), f"result={res!r}, but should be str"
@@ -155,8 +155,8 @@ def test_as_native_dtype(
assert isinstance(res, ivy_backend.NativeDtype)
return
- assert isinstance(input_dtype, ivy_backend.Dtype) or isinstance(
- input_dtype, str
+ assert isinstance(
+ input_dtype, (ivy_backend.Dtype, str)
), f"input_dtype={input_dtype!r}, but should be str or ivy.Dtype"
assert isinstance(
res, ivy_backend.NativeDtype
@@ -168,6 +168,7 @@ def test_as_native_dtype(
fn_tree="functional.ivy.astype",
dtype_and_x_and_cast_dtype=astype_helper(),
test_gradients=st.just(False),
+ test_with_copy=st.just(True),
)
def test_astype(
*, dtype_and_x_and_cast_dtype, test_flags, backend_fw, fn_name, on_device
@@ -274,11 +275,9 @@ def test_closest_valid_dtype(
with BackendHandler.update_backend(backend_fw) as ivy_backend:
input_dtype = input_dtype[0]
res = ivy_backend.closest_valid_dtype(input_dtype)
- assert isinstance(input_dtype, ivy_backend.Dtype) or isinstance(
- input_dtype, str
- )
- assert isinstance(res, ivy_backend.Dtype) or isinstance(
- res, str
+ assert isinstance(input_dtype, (ivy_backend.Dtype, str))
+ assert isinstance(
+ res, (ivy_backend.Dtype, str)
), f"result={res!r}, but should be str or ivy.Dtype"
@@ -302,11 +301,14 @@ def test_default_complex_dtype(
complex_dtype=complex_dtype[0],
as_native=as_native,
)
- assert (
- isinstance(res, ivy_backend.Dtype)
- or isinstance(res, typing.get_args(ivy_backend.NativeDtype))
- or isinstance(res, ivy_backend.NativeDtype)
- or isinstance(res, str)
+ assert isinstance(
+ res,
+ (
+ ivy_backend.Dtype,
+ typing.get_args(ivy_backend.NativeDtype),
+ ivy_backend.NativeDtype,
+ str,
+ ),
)
assert (
ivy_backend.default_complex_dtype(
@@ -336,10 +338,8 @@ def test_default_dtype(
with BackendHandler.update_backend(backend_fw) as ivy_backend:
input_dtype = input_dtype[0]
res = ivy_backend.default_dtype(dtype=input_dtype, as_native=as_native)
- assert (
- isinstance(input_dtype, ivy_backend.Dtype)
- or isinstance(input_dtype, str)
- or isinstance(input_dtype, ivy_backend.NativeDtype)
+ assert isinstance(
+ input_dtype, (ivy_backend.Dtype, str, ivy_backend.NativeDtype)
)
assert isinstance(res, ivy_backend.Dtype) or isinstance(
input_dtype, str
@@ -366,11 +366,14 @@ def test_default_float_dtype(
float_dtype=float_dtype[0],
as_native=as_native,
)
- assert (
- isinstance(res, ivy_backend.Dtype)
- or isinstance(res, typing.get_args(ivy_backend.NativeDtype))
- or isinstance(res, ivy_backend.NativeDtype)
- or isinstance(res, str)
+ assert isinstance(
+ res,
+ (
+ ivy_backend.Dtype,
+ typing.get_args(ivy_backend.NativeDtype),
+ ivy_backend.NativeDtype,
+ str,
+ ),
)
assert (
ivy_backend.default_float_dtype(
@@ -405,11 +408,14 @@ def test_default_int_dtype(
int_dtype=int_dtype[0],
as_native=as_native,
)
- assert (
- isinstance(res, ivy_backend.Dtype)
- or isinstance(res, typing.get_args(ivy_backend.NativeDtype))
- or isinstance(res, ivy_backend.NativeDtype)
- or isinstance(res, str)
+ assert isinstance(
+ res,
+ (
+ ivy_backend.Dtype,
+ typing.get_args(ivy_backend.NativeDtype),
+ ivy_backend.NativeDtype,
+ str,
+ ),
)
assert (
ivy_backend.default_int_dtype(input=None, int_dtype=None, as_native=False)
@@ -623,7 +629,7 @@ def test_function_dtype_versioning_frontend(
var[backend_fw] = key2
fn = getattr(
_import_mod.import_module(
- "ivy.functional.frontends." + backend_fw
+ f"ivy.functional.frontends.{backend_fw}"
),
key1,
)
diff --git a/ivy_tests/test_ivy/test_functional/test_core/test_elementwise.py b/ivy_tests/test_ivy/test_functional/test_core/test_elementwise.py
index 1bf00a9e0628d..bb2fd1c5695bb 100644
--- a/ivy_tests/test_ivy/test_functional/test_core/test_elementwise.py
+++ b/ivy_tests/test_ivy/test_functional/test_core/test_elementwise.py
@@ -465,7 +465,7 @@ def test_bitwise_invert(*, dtype_and_x, test_flags, backend_fw, fn_name, on_devi
def test_bitwise_left_shift(*, dtype_and_x, test_flags, backend_fw, fn_name, on_device):
input_dtype, x = dtype_and_x
# negative shifts will throw an exception
- # shifts >= dtype witdth produce backend-defined behavior
+ # shifts >= dtype width produce backend-defined behavior
dtype = np.promote_types(input_dtype[0], input_dtype[1])
bit_cap = (
np.iinfo(dtype).bits
@@ -532,7 +532,7 @@ def test_bitwise_right_shift(
input_dtype, x = dtype_and_x
# negative shifts will throw an exception
- # shifts >= dtype witdth produce backend-defined behavior
+ # shifts >= dtype width produce backend-defined behavior
x[1] = np.asarray(
np.clip(x[1], 0, np.iinfo(input_dtype[1]).bits - 1), dtype=input_dtype[1]
)
@@ -1502,6 +1502,7 @@ def test_multiply(*, dtype_and_x, test_flags, backend_fw, fn_name, on_device):
posinf=st.floats(min_value=5e100, max_value=5e100),
neginf=st.floats(min_value=-5e100, max_value=-5e100),
test_gradients=st.just(False),
+ test_with_copy=st.just(True),
)
def test_nan_to_num(
*,
@@ -1916,10 +1917,9 @@ def test_tanh(*, dtype_and_x, complex_mode, test_flags, backend_fw, fn_name, on_
backend_to_test=backend_fw,
fn_name=fn_name,
on_device=on_device,
- rtol_=1e-1,
- atol_=1e-2,
x=x[0],
complex_mode=complex_mode,
+ atol_=1e-02, # for `test_flags.test_gradients and 'bfloat16' in input_dtype`
)
diff --git a/ivy_tests/test_ivy/test_functional/test_core/test_general.py b/ivy_tests/test_ivy/test_functional/test_core/test_general.py
index 8f7ffa0c81ede..7170cb1196af0 100644
--- a/ivy_tests/test_ivy/test_functional/test_core/test_general.py
+++ b/ivy_tests/test_ivy/test_functional/test_core/test_general.py
@@ -1068,6 +1068,7 @@ def test_get_all_arrays_in_memory():
test_gradients=st.just(False),
test_instance_method=st.just(False),
container_flags=st.just([False]),
+ test_with_copy=st.just(True),
)
def test_get_item(
dtypes_x_query,
@@ -1640,6 +1641,7 @@ def test_set_inplace_mode(mode):
test_gradients=st.just(False),
test_instance_method=st.just(False),
container_flags=st.just([False]),
+ test_with_copy=st.just(True),
)
def test_set_item(
dtypes_x_query_val,
@@ -1760,7 +1762,7 @@ def test_stable_pow(
*, dtypes_and_xs, min_base, test_flags, backend_fw, fn_name, on_device
):
dtypes, xs = dtypes_and_xs
- assume(all(["bfloat16" not in x for x in dtypes]))
+ assume(all("bfloat16" not in x for x in dtypes))
helpers.test_function(
input_dtypes=dtypes,
test_flags=test_flags,
@@ -1848,6 +1850,7 @@ def test_to_list(x0_n_x1_n_res, test_flags, backend_fw, fn_name, on_device):
copy=st.booleans(),
test_with_out=st.just(False),
test_gradients=st.just(False),
+ test_with_copy=st.just(True),
)
def test_to_numpy(*, dtype_x, copy, test_flags, backend_fw, fn_name, on_device):
dtype, x = dtype_x
@@ -1878,6 +1881,7 @@ def test_to_numpy(*, dtype_x, copy, test_flags, backend_fw, fn_name, on_device):
),
test_with_out=st.just(False),
test_gradients=st.just(False),
+ test_with_copy=st.just(True),
)
def test_to_scalar(x0_n_x1_n_res, test_flags, backend_fw, fn_name, on_device):
dtype, x = x0_n_x1_n_res
diff --git a/ivy_tests/test_ivy/test_functional/test_core/test_gradients.py b/ivy_tests/test_ivy/test_functional/test_core/test_gradients.py
index 38a1553183f6e..5556747a2f82b 100644
--- a/ivy_tests/test_ivy/test_functional/test_core/test_gradients.py
+++ b/ivy_tests/test_ivy/test_functional/test_core/test_gradients.py
@@ -239,9 +239,7 @@ def func(xs):
@pytest.mark.parametrize("nth", [1, 2, 3])
def test_grad(x, dtype, func, backend_fw, nth):
# ToDo: Remove skipping for paddle and jax for nth > 1
- if backend_fw == "numpy" or (
- (backend_fw == "paddle" or backend_fw == "jax") and nth > 1
- ):
+ if backend_fw == "numpy" or (backend_fw in ["paddle", "jax"] and nth > 1):
return
with BackendHandler.update_backend(backend_fw) as ivy_backend:
diff --git a/ivy_tests/test_ivy/test_functional/test_core/test_manipulation.py b/ivy_tests/test_ivy/test_functional/test_core/test_manipulation.py
index 902ed255922ce..e76b88adddc49 100644
--- a/ivy_tests/test_ivy/test_functional/test_core/test_manipulation.py
+++ b/ivy_tests/test_ivy/test_functional/test_core/test_manipulation.py
@@ -33,7 +33,7 @@ def _arrays_idx_n_dtypes(draw):
size=num_arrays,
)
)
- xs = list()
+ xs = []
input_dtypes = draw(
helpers.array_dtypes(available_dtypes=draw(helpers.get_dtypes("float")))
)
@@ -346,6 +346,7 @@ def test_constant_pad(
axis=helpers.get_axis(
shape=st.shared(helpers.get_shape(), key="value_shape"),
),
+ test_with_copy=st.just(True),
)
def test_expand_dims(*, dtype_value, axis, test_flags, backend_fw, fn_name, on_device):
dtype, value = dtype_value
@@ -379,6 +380,7 @@ def test_expand_dims(*, dtype_value, axis, test_flags, backend_fw, fn_name, on_d
max_size=1,
force_int=True,
),
+ test_with_copy=st.just(True),
)
def test_flip(*, dtype_value, axis, test_flags, backend_fw, fn_name, on_device):
dtype, value = dtype_value
@@ -402,6 +404,7 @@ def test_flip(*, dtype_value, axis, test_flags, backend_fw, fn_name, on_device):
shape=st.shared(helpers.get_shape(min_num_dims=1), key="value_shape"),
),
permutation=_permute_dims_helper(),
+ test_with_copy=st.just(True),
)
def test_permute_dims(
*, dtype_value, permutation, test_flags, backend_fw, fn_name, on_device
@@ -475,6 +478,7 @@ def test_repeat(
),
order=st.sampled_from(["C", "F"]),
allowzero=st.booleans(),
+ test_with_copy=st.just(True),
)
def test_reshape(
*,
@@ -581,6 +585,7 @@ def test_roll(*, dtype_value, shift, axis, test_flags, backend_fw, fn_name, on_d
with_remainder=st.booleans(),
num_or_size_splits=_get_splits(),
test_with_out=st.just(False),
+ test_with_copy=st.just(True),
)
def test_split(
*,
@@ -621,6 +626,7 @@ def test_split(
shape=st.shared(helpers.get_shape(), key="value_shape"),
),
axis=_squeeze_helper(),
+ test_with_copy=st.just(True),
)
def test_squeeze(*, dtype_value, axis, test_flags, backend_fw, fn_name, on_device):
dtype, value = dtype_value
@@ -672,6 +678,7 @@ def test_stack(*, dtypes_arrays, axis, test_flags, backend_fw, fn_name, on_devic
axis1=helpers.get_axis(
shape=st.shared(helpers.get_shape(min_num_dims=2), key="shape"), force_int=True
),
+ test_with_copy=st.just(True),
)
def test_swapaxes(
*, dtype_value, axis0, axis1, test_flags, backend_fw, fn_name, on_device
@@ -733,6 +740,7 @@ def test_tile(*, dtype_value, repeat, test_flags, backend_fw, fn_name, on_device
),
keepdims=st.booleans(),
test_with_out=st.just(False),
+ test_with_copy=st.just(True),
)
def test_unstack(
*, x_n_dtype_axis, keepdims, test_flags, backend_fw, fn_name, on_device
diff --git a/ivy_tests/test_ivy/test_functional/test_core/test_meta.py b/ivy_tests/test_ivy/test_functional/test_core/test_meta.py
index bc6b5d524a1b4..a0b3cc5723e1c 100644
--- a/ivy_tests/test_ivy/test_functional/test_core/test_meta.py
+++ b/ivy_tests/test_ivy/test_functional/test_core/test_meta.py
@@ -104,7 +104,7 @@ def outer_cost_fn(batch_in, v):
batch_np = batch.cont_map(lambda x, kc: ivy_backend.to_numpy(x))
# true gradient
- all_outer_grads = list()
+ all_outer_grads = []
for sub_batch in batch_np.cont_unstack_conts(0, True, num_tasks):
all_outer_grads.append(
[
@@ -118,10 +118,10 @@ def outer_cost_fn(batch_in, v):
)
if average_across_steps:
true_weight_grad = (
- sum([sum(og) / len(og) for og in all_outer_grads]) / num_tasks
+ sum(sum(og) / len(og) for og in all_outer_grads) / num_tasks
)
else:
- true_weight_grad = sum([og[-1] for og in all_outer_grads]) / num_tasks
+ true_weight_grad = sum(og[-1] for og in all_outer_grads) / num_tasks
# true latent gradient
true_latent_grad = np.array(
@@ -275,10 +275,10 @@ def loss_grad_fn(sub_batch_in, w_in, outer=False):
)
# true gradient
- true_outer_grads = list()
+ true_outer_grads = []
for sub_batch in batch_np.cont_unstack_conts(0, True, num_tasks):
- ws = list()
- grads = list()
+ ws = []
+ grads = []
ws.append(latent_np)
for step in range(inner_grad_steps):
update_grad = loss_grad_fn(sub_batch, ws[-1])
@@ -468,7 +468,7 @@ def outer_cost_fn(batch_in, v):
batch_np = batch.cont_map(lambda x, kc: ivy_backend.to_numpy(x))
# true gradient
- all_outer_grads = list()
+ all_outer_grads = []
for sub_batch in batch_np.cont_unstack_conts(0, True, num_tasks):
all_outer_grads.append(
[
@@ -482,10 +482,10 @@ def outer_cost_fn(batch_in, v):
)
if average_across_steps:
true_weight_grad = (
- sum([sum(og) / len(og) for og in all_outer_grads]) / num_tasks
+ sum(sum(og) / len(og) for og in all_outer_grads) / num_tasks
)
else:
- true_weight_grad = sum([og[-1] for og in all_outer_grads]) / num_tasks
+ true_weight_grad = sum(og[-1] for og in all_outer_grads) / num_tasks
# true cost
true_cost_dict = {
@@ -632,7 +632,7 @@ def outer_cost_fn(batch_in, v):
batch_np = batch.cont_map(lambda x, kc: ivy_backend.to_numpy(x))
# true weight gradient
- all_outer_grads = list()
+ all_outer_grads = []
for sub_batch in batch_np.cont_unstack_conts(0, True, num_tasks):
all_outer_grads.append(
[
@@ -650,10 +650,10 @@ def outer_cost_fn(batch_in, v):
)
if average_across_steps:
true_weight_grad = (
- sum([sum(og) / len(og) for og in all_outer_grads]) / num_tasks
+ sum(sum(og) / len(og) for og in all_outer_grads) / num_tasks
)
else:
- true_weight_grad = sum([og[-1] for og in all_outer_grads]) / num_tasks
+ true_weight_grad = sum(og[-1] for og in all_outer_grads) / num_tasks
# true latent gradient
true_latent_grad = np.array(
@@ -816,30 +816,28 @@ def update_grad_fn(w_init, sub_batch_in, num_steps, average=False):
collection_of_terms.append([t for t in terms])
if average:
return [
- sum(
- [
+ (
+ sum(
t * inner_learning_rate ** (num_steps - i)
for i, t in enumerate(tms)
- ]
+ )
+ * w_init.latent
)
- * w_init.latent
for tms in collection_of_terms
]
return (
sum(
- [
- t * inner_learning_rate ** (num_steps - i)
- for i, t in enumerate(terms)
- ]
+ t * inner_learning_rate ** (num_steps - i)
+ for i, t in enumerate(terms)
)
* w_init.latent
)
# true gradient
- true_outer_grads = list()
+ true_outer_grads = []
for sub_batch in batch_np.cont_unstack_conts(0, True, num_tasks):
- ws = list()
- grads = list()
+ ws = []
+ grads = []
ws.append(variables_np)
for step in range(inner_grad_steps):
update_grad = loss_grad_fn(sub_batch, ws[-1])
@@ -857,15 +855,16 @@ def update_grad_fn(w_init, sub_batch_in, num_steps, average=False):
# true outer grad
if average_across_steps:
true_outer_grad = sum(
- [
- ig.latent * ug
- for ig, ug in zip(
- grads,
- update_grad_fn(
- variables_np, sub_batch, inner_grad_steps, average=True
- ),
- )
- ]
+ ig.latent * ug
+ for ig, ug in zip(
+ grads,
+ update_grad_fn(
+ variables_np,
+ sub_batch,
+ inner_grad_steps,
+ average=True,
+ ),
+ )
) / len(grads)
else:
true_outer_grad = ivy_backend.multiply(
@@ -1040,7 +1039,7 @@ def outer_cost_fn(batch_in, v):
batch_np = batch.cont_map(lambda x, kc: ivy_backend.to_numpy(x))
# true gradient
- all_outer_grads = list()
+ all_outer_grads = []
for sub_batch in batch_np.cont_unstack_conts(0, True, num_tasks):
all_outer_grads.append(
[
@@ -1058,10 +1057,10 @@ def outer_cost_fn(batch_in, v):
)
if average_across_steps:
true_outer_grad = (
- sum([sum(og) / len(og) for og in all_outer_grads]) / num_tasks
+ sum(sum(og) / len(og) for og in all_outer_grads) / num_tasks
)
else:
- true_outer_grad = sum([og[-1] for og in all_outer_grads]) / num_tasks
+ true_outer_grad = sum(og[-1] for og in all_outer_grads) / num_tasks
# true cost
true_cost_dict = {
@@ -1185,10 +1184,10 @@ def loss_grad_fn(sub_batch_in, w_in):
return -2 * sub_batch_in["x"][0] * w_in
# true gradient
- true_outer_grads = list()
+ true_outer_grads = []
for sub_batch in batch_np.cont_unstack_conts(0, True, num_tasks):
- ws = list()
- grads = list()
+ ws = []
+ grads = []
ws.append(latent_np)
for step in range(inner_grad_steps):
update_grad = loss_grad_fn(sub_batch, ws[-1])
diff --git a/ivy_tests/test_ivy/test_functional/test_core/test_nest.py b/ivy_tests/test_ivy/test_functional/test_core/test_nest.py
index fbdbbac9158f5..be259bb20e3ff 100644
--- a/ivy_tests/test_ivy/test_functional/test_core/test_nest.py
+++ b/ivy_tests/test_ivy/test_functional/test_core/test_nest.py
@@ -241,7 +241,7 @@ def mnais(n, idxs, vs):
)
def test_multi_index_nest(nest, multi_indices):
rets = ivy.multi_index_nest(nest, multi_indices)
- true_rets = list()
+ true_rets = []
for indices in multi_indices:
true_ret = nest
for i in indices:
diff --git a/ivy_tests/test_ivy/test_functional/test_core/test_set.py b/ivy_tests/test_ivy/test_functional/test_core/test_set.py
index 1b58a73ef003a..69f9be2fbf241 100644
--- a/ivy_tests/test_ivy/test_functional/test_core/test_set.py
+++ b/ivy_tests/test_ivy/test_functional/test_core/test_set.py
@@ -78,9 +78,9 @@ def test_unique_counts(*, dtype_and_x, test_flags, backend_fw, fn_name, on_devic
test_with_out=st.just(False),
test_gradients=st.just(False),
)
-def test_unique_inverse(*, dtype_and_x, test_flags, backend_fw, fn_name, on_device):
- dtype, x = dtype_and_x
- assume(not np.any(np.isclose(x, 0.0)))
+def test_unique_inverse(*, dtype_x_axis, test_flags, backend_fw, fn_name, on_device):
+ dtype, x, axis = dtype_x_axis
+ assume(not np.any(np.isclose(x, 0.0), axis=axis))
helpers.test_function(
input_dtypes=dtype,
@@ -88,6 +88,7 @@ def test_unique_inverse(*, dtype_and_x, test_flags, backend_fw, fn_name, on_devi
on_device=on_device,
backend_to_test=backend_fw,
fn_name=fn_name,
+ axis=axis,
x=x[0],
)
diff --git a/ivy_tests/test_ivy/test_functional/test_core/test_sorting.py b/ivy_tests/test_ivy/test_functional/test_core/test_sorting.py
index 76d4e9b2e9b33..8a6f5476a3812 100644
--- a/ivy_tests/test_ivy/test_functional/test_core/test_sorting.py
+++ b/ivy_tests/test_ivy/test_functional/test_core/test_sorting.py
@@ -104,6 +104,7 @@ def test_argsort(
max_value=100,
),
test_gradients=st.just(False),
+ test_with_copy=st.just(True),
)
def test_msort(dtype_and_x, test_flags, backend_fw, fn_name, on_device):
dtype, x = dtype_and_x
@@ -173,6 +174,7 @@ def test_searchsorted(
descending=st.booleans(),
stable=st.booleans(),
test_gradients=st.just(False),
+ test_with_copy=st.just(True),
)
def test_sort(
*, dtype_x_axis, descending, stable, test_flags, backend_fw, fn_name, on_device
diff --git a/ivy_tests/test_ivy/test_functional/test_core/test_statistical.py b/ivy_tests/test_ivy/test_functional/test_core/test_statistical.py
index bd1cedb889106..87b230fdf9649 100644
--- a/ivy_tests/test_ivy/test_functional/test_core/test_statistical.py
+++ b/ivy_tests/test_ivy/test_functional/test_core/test_statistical.py
@@ -1,4 +1,5 @@
"""Collection of tests for statistical functions."""
+
# global
import numpy as np
from hypothesis import strategies as st, assume
@@ -62,6 +63,10 @@ def _statistical_dtype_values(draw, *, function, min_value=None, max_value=None)
shape = values[0].shape
size = values[0].size
max_correction = np.min(shape)
+ if "complex" in dtype[0]:
+ # TODO skip complex median test until added ?
+ # because it is not supported in tensorflow (ground truth backend)
+ dtype = ["float32"]
if any(ele in function for ele in ["std", "var"]):
if size == 1:
correction = 0
@@ -327,6 +332,7 @@ def test_std(*, dtype_and_x, keep_dims, test_flags, backend_fw, fn_name, on_devi
fn_tree="functional.ivy.sum",
dtype_x_axis_castable=_get_castable_dtype(),
keep_dims=st.booleans(),
+ test_gradients=st.just(False),
)
def test_sum(
*, dtype_x_axis_castable, keep_dims, test_flags, backend_fw, fn_name, on_device
@@ -337,6 +343,9 @@ def test_sum(
if "torch" in backend_fw:
assume(not test_flags.as_variable[0])
assume(not test_flags.test_gradients)
+ if "jax" in backend_fw and castable_dtype in ["complex64", "complex128"]:
+ assume(not test_flags.test_gradients)
+
helpers.test_function(
input_dtypes=[input_dtype],
test_flags=test_flags,
diff --git a/ivy_tests/test_ivy/test_functional/test_experimental/test_core/test_creation.py b/ivy_tests/test_ivy/test_functional/test_experimental/test_core/test_creation.py
index 694601fe0c45e..151cc3fcb344d 100644
--- a/ivy_tests/test_ivy/test_functional/test_experimental/test_core/test_creation.py
+++ b/ivy_tests/test_ivy/test_functional/test_experimental/test_core/test_creation.py
@@ -406,6 +406,38 @@ def test_ndindex(dtype_x_shape):
assert index1 == index2
+# polyval
+@handle_test(
+ fn_tree="functional.ivy.experimental.polyval",
+ dtype_and_coeffs=helpers.dtype_and_values(
+ available_dtypes=helpers.get_dtypes("valid"),
+ min_num_dims=1,
+ ),
+ dtype_and_x=helpers.dtype_and_values(
+ available_dtypes=helpers.get_dtypes("valid"),
+ min_num_dims=0,
+ ),
+ test_with_out=st.just(False),
+ test_gradients=st.just(False),
+ test_instance_method=st.just(False),
+)
+def test_polyval(
+ *, dtype_and_coeffs, dtype_and_x, test_flags, backend_fw, fn_name, on_device
+):
+ coeffs_dtype, coeffs = dtype_and_coeffs
+ x_dtype, x = dtype_and_x
+
+ helpers.test_function(
+ input_dtypes=coeffs_dtype + x_dtype,
+ test_flags=test_flags,
+ on_device=on_device,
+ backend_to_test=backend_fw,
+ fn_name=fn_name,
+ coeffs=coeffs,
+ x=x,
+ )
+
+
@handle_test(
fn_tree="functional.ivy.experimental.random_cp",
data=_random_cp_data(),
@@ -767,6 +799,33 @@ def test_trilu(*, dtype_and_x, k, upper, test_flags, backend_fw, fn_name, on_dev
)
+@handle_test(
+ fn_tree="functional.ivy.experimental.unsorted_segment_mean",
+ d_x_n_s=valid_unsorted_segment_min_inputs(),
+ test_with_out=st.just(False),
+ test_gradients=st.just(False),
+)
+def test_unsorted_segment_mean(
+ *,
+ d_x_n_s,
+ test_flags,
+ backend_fw,
+ fn_name,
+ on_device,
+):
+ dtypes, data, num_segments, segment_ids = d_x_n_s
+ helpers.test_function(
+ input_dtypes=dtypes,
+ test_flags=test_flags,
+ on_device=on_device,
+ backend_to_test=backend_fw,
+ fn_name=fn_name,
+ data=data,
+ segment_ids=segment_ids,
+ num_segments=num_segments,
+ )
+
+
# unsorted_segment_min
@handle_test(
fn_tree="functional.ivy.experimental.unsorted_segment_min",
diff --git a/ivy_tests/test_ivy/test_functional/test_experimental/test_core/test_elementwise.py b/ivy_tests/test_ivy/test_functional/test_experimental/test_core/test_elementwise.py
index bc47684cd014e..24517040cf225 100644
--- a/ivy_tests/test_ivy/test_functional/test_experimental/test_core/test_elementwise.py
+++ b/ivy_tests/test_ivy/test_functional/test_experimental/test_core/test_elementwise.py
@@ -231,6 +231,72 @@ def test_allclose(
)
+@handle_test(
+ fn_tree="functional.ivy.experimental.amax",
+ dtype_and_x=helpers.dtype_values_axis(
+ available_dtypes=helpers.get_dtypes("valid"),
+ large_abs_safety_factor=2,
+ small_abs_safety_factor=2,
+ safety_factor_scale="log",
+ min_num_dims=1,
+ max_num_dims=5,
+ min_dim_size=2,
+ valid_axis=True,
+ allow_neg_axes=True,
+ min_axes_size=1,
+ min_value=None,
+ max_value=None,
+ allow_nan=False,
+ ),
+ keep_dims=st.booleans(),
+)
+def test_amax(*, dtype_and_x, keep_dims, test_flags, backend_fw, fn_name, on_device):
+ input_dtype, x, axis = dtype_and_x
+ helpers.test_function(
+ input_dtypes=input_dtype,
+ test_flags=test_flags,
+ backend_to_test=backend_fw,
+ fn_name=fn_name,
+ on_device=on_device,
+ x=x[0],
+ axis=axis,
+ keepdims=keep_dims,
+ )
+
+
+@handle_test(
+ fn_tree="functional.ivy.experimental.amin",
+ dtype_and_x=helpers.dtype_values_axis(
+ available_dtypes=helpers.get_dtypes("valid"),
+ large_abs_safety_factor=2,
+ small_abs_safety_factor=2,
+ safety_factor_scale="log",
+ min_num_dims=1,
+ max_num_dims=5,
+ min_dim_size=2,
+ valid_axis=True,
+ allow_neg_axes=True,
+ min_axes_size=1,
+ min_value=None,
+ max_value=None,
+ allow_nan=False,
+ ),
+ keep_dims=st.booleans(),
+)
+def test_amin(*, dtype_and_x, keep_dims, test_flags, backend_fw, fn_name, on_device):
+ input_dtype, x, axis = dtype_and_x
+ helpers.test_function(
+ input_dtypes=input_dtype,
+ test_flags=test_flags,
+ backend_to_test=backend_fw,
+ fn_name=fn_name,
+ on_device=on_device,
+ x=x[0],
+ axis=axis,
+ keepdims=keep_dims,
+ )
+
+
@handle_test(
fn_tree="functional.ivy.experimental.binarizer",
dtype_and_x=helpers.dtype_and_values(
@@ -544,7 +610,7 @@ def test_frexp(*, dtype_and_x, test_flags, backend_fw, fn_name, on_device):
@handle_test(
fn_tree="functional.ivy.experimental.gradient",
dtype_n_x_n_axis=helpers.dtype_values_axis(
- available_dtypes=("float32", "float16", "float64"),
+ available_dtypes=helpers.get_dtypes("valid"),
min_num_dims=1,
max_num_dims=3,
min_dim_size=2,
@@ -556,11 +622,19 @@ def test_frexp(*, dtype_and_x, test_flags, backend_fw, fn_name, on_device):
min_value=-3,
max_value=3,
),
+ edge_order=st.sampled_from([1, 2]),
test_with_out=st.just(False),
test_gradients=st.just(False),
)
def test_gradient(
- *, dtype_n_x_n_axis, spacing, test_flags, backend_fw, fn_name, on_device
+ *,
+ dtype_n_x_n_axis,
+ spacing,
+ test_flags,
+ backend_fw,
+ fn_name,
+ on_device,
+ edge_order,
):
input_dtype, x, axis = dtype_n_x_n_axis
helpers.test_function(
@@ -572,6 +646,7 @@ def test_gradient(
x=x[0],
spacing=spacing,
axis=axis,
+ edge_order=edge_order,
)
diff --git a/ivy_tests/test_ivy/test_functional/test_experimental/test_core/test_gradients.py b/ivy_tests/test_ivy/test_functional/test_experimental/test_core/test_gradients.py
index d5758cc2d34cd..f34b3513a8290 100644
--- a/ivy_tests/test_ivy/test_functional/test_experimental/test_core/test_gradients.py
+++ b/ivy_tests/test_ivy/test_functional/test_experimental/test_core/test_gradients.py
@@ -7,6 +7,44 @@
from ivy_tests.test_ivy.helpers.pipeline_helper import BackendHandler
+# --- Helpers --- #
+# --------------- #
+
+
+def _get_primals_and_tangents(x_, dtype, ivy_backend, primals_cont, tangents_cont):
+ if primals_cont:
+ primals = ivy_backend.Container(
+ {
+ "l": {
+ "a": ivy_backend.array(x_[0][0], dtype=dtype),
+ "b": ivy_backend.array(x_[0][1], dtype=dtype),
+ }
+ }
+ )
+ else:
+ primals = ivy_backend.array(x_[0], dtype=dtype)
+
+ if tangents_cont:
+ tangents = ivy_backend.Container(
+ {
+ "l": {
+ "a": ivy_backend.array([t[0] for t in x_[1]], dtype=dtype),
+ "b": ivy_backend.array([t[0] for t in x_[1]], dtype=dtype),
+ }
+ }
+ )
+ else:
+ if primals_cont:
+ tangents = ivy_backend.array([t[0] for t in x_[1]], dtype=dtype)
+ else:
+ tangents = ivy_backend.array(x_[1], dtype=dtype).T
+ return primals, tangents
+
+
+# --- Main --- #
+# ------------ #
+
+
# bind_custom_gradient_function
@pytest.mark.parametrize(
"x_", [[[4.6, 2.1, 5], [2.8, 1.3, 6.2]], [[4.6, 2.1], [5, 2.8], [1.3, 6.2]]]
@@ -61,3 +99,153 @@ def func(x):
for grad, grad_from_gt in zip(grad_np, grad_np_from_gt):
assert grad.shape == grad_from_gt.shape
assert np.allclose(grad, grad_from_gt)
+
+
+# write a test for jvp
+@pytest.mark.parametrize(
+ "x_", [[[[4.6, 2.1, 5], [2.8, 1.3, 6.2]], [[4.6, 2.1], [5, 2.8], [1.3, 6.2]]]]
+)
+@pytest.mark.parametrize("dtype", ["float32", "float64"])
+@pytest.mark.parametrize("func_str", ["square", "cos"])
+@pytest.mark.parametrize(
+ "nested_structs", ["nested_input_nested_output", "nested_input_flat_output", "none"]
+)
+def test_jvp(x_, dtype, func_str, backend_fw, nested_structs):
+ if backend_fw in ["numpy", "paddle", "mxnet"]:
+ pytest.skip()
+
+ with BackendHandler.update_backend(backend_fw) as ivy_backend:
+ base_func = ivy_backend.__dict__[func_str]
+ if nested_structs == "none":
+ primals, tangents = _get_primals_and_tangents(
+ x_, dtype, ivy_backend, False, False
+ )
+ func = base_func
+ elif nested_structs == "nested_input_nested_output":
+ primals, tangents = _get_primals_and_tangents(
+ x_, dtype, ivy_backend, True, True
+ )
+ func = base_func
+ elif nested_structs == "nested_input_flat_output":
+ primals, tangents = _get_primals_and_tangents(
+ x_, dtype, ivy_backend, True, True
+ )
+
+ def func(x):
+ return base_func(x["l"]["a"]) + base_func(x["l"]["b"])
+
+ primals = (primals,)
+ tangents = (tangents,)
+ primals_out, jvp = ivy_backend.jvp(func, primals, tangents)
+ flat_primals_np = helpers.flatten_and_to_np(ret=primals_out, backend=backend_fw)
+ jvp_np = helpers.flatten_and_to_np(ret=jvp, backend=backend_fw)
+ assert jvp_np != []
+
+ with BackendHandler.update_backend("jax") as gt_backend:
+ base_func = gt_backend.__dict__[func_str]
+ if nested_structs == "none":
+ primals, tangents = _get_primals_and_tangents(
+ x_, dtype, gt_backend, False, False
+ )
+ func = base_func
+ elif nested_structs == "nested_input_nested_output":
+ primals, tangents = _get_primals_and_tangents(
+ x_, dtype, gt_backend, True, True
+ )
+ func = base_func
+ elif nested_structs == "nested_input_flat_output":
+ primals, tangents = _get_primals_and_tangents(
+ x_, dtype, gt_backend, True, True
+ )
+
+ def func(x):
+ return base_func(x["l"]["a"]) + base_func(x["l"]["b"])
+
+ # func = base_func
+
+ primals = (primals,)
+ tangents = (tangents,)
+ primals_out_gt, jvp = gt_backend.jvp(func, primals, tangents)
+ flat_primals_np_from_gt = helpers.flatten_and_to_np(
+ ret=primals_out_gt, backend="jax"
+ )
+ jvp_np_from_gt = helpers.flatten_and_to_np(ret=jvp, backend="jax")
+ assert jvp_np_from_gt != []
+
+ assert np.allclose(flat_primals_np, flat_primals_np_from_gt)
+ assert np.allclose(jvp_np, jvp_np_from_gt)
+
+
+# write a test for vjp
+@pytest.mark.parametrize(
+ "x_", [[[[4.6, 2.1, 5], [2.8, 1.3, 6.2]], [[4.6, 2.1], [5, 2.8], [1.3, 6.2]]]]
+)
+@pytest.mark.parametrize("dtype", ["float32", "float64"])
+@pytest.mark.parametrize("func_str", ["square", "cos"])
+@pytest.mark.parametrize(
+ "nested_structs", ["nested_input_nested_output", "nested_input_flat_output", "none"]
+)
+def test_vjp(x_, dtype, func_str, backend_fw, nested_structs):
+ if backend_fw == "numpy":
+ pytest.skip()
+
+ with BackendHandler.update_backend(backend_fw) as ivy_backend:
+ base_func = ivy_backend.__dict__[func_str]
+ if nested_structs == "none":
+ primals, tangents = _get_primals_and_tangents(
+ x_, dtype, ivy_backend, False, False
+ )
+ func = base_func
+ elif nested_structs == "nested_input_nested_output":
+ primals, tangents = _get_primals_and_tangents(
+ x_, dtype, ivy_backend, True, True
+ )
+ func = base_func
+ elif nested_structs == "nested_input_flat_output":
+ primals, tangents = _get_primals_and_tangents(
+ x_, dtype, ivy_backend, True, False
+ )
+
+ def func(x):
+ return base_func(x["l"]["a"]) + base_func(x["l"]["b"])
+
+ primals = (primals,)
+ tangents = (tangents,)
+ primals_out, vjp_fn = ivy_backend.vjp(func, *primals)
+ vjp = vjp_fn(*tangents)
+ flat_primals_np = helpers.flatten_and_to_np(ret=primals_out, backend=backend_fw)
+ vjp_np = helpers.flatten_and_to_np(ret=vjp, backend=backend_fw)
+ assert vjp_np != []
+
+ with BackendHandler.update_backend("jax") as gt_backend:
+ base_func = gt_backend.__dict__[func_str]
+ if nested_structs == "none":
+ primals, tangents = _get_primals_and_tangents(
+ x_, dtype, gt_backend, False, False
+ )
+ func = base_func
+ elif nested_structs == "nested_input_nested_output":
+ primals, tangents = _get_primals_and_tangents(
+ x_, dtype, gt_backend, True, True
+ )
+ func = base_func
+ elif nested_structs == "nested_input_flat_output":
+ primals, tangents = _get_primals_and_tangents(
+ x_, dtype, gt_backend, True, False
+ )
+
+ def func(x):
+ return base_func(x["l"]["a"]) + base_func(x["l"]["b"])
+
+ primals = (primals,)
+ tangents = (tangents,)
+ primals_out_gt, vjp_fn = gt_backend.vjp(func, *primals)
+ vjp = vjp_fn(*tangents)
+ flat_primals_np_from_gt = helpers.flatten_and_to_np(
+ ret=primals_out_gt, backend="jax"
+ )
+ vjp_np_from_gt = helpers.flatten_and_to_np(ret=vjp, backend="jax")
+ assert vjp_np_from_gt != []
+
+ assert np.allclose(flat_primals_np, flat_primals_np_from_gt)
+ assert np.allclose(vjp_np, vjp_np_from_gt)
diff --git a/ivy_tests/test_ivy/test_functional/test_experimental/test_core/test_linalg.py b/ivy_tests/test_ivy/test_functional/test_experimental/test_core/test_linalg.py
index df1bb66f5792f..fe2f805d4759b 100644
--- a/ivy_tests/test_ivy/test_functional/test_experimental/test_core/test_linalg.py
+++ b/ivy_tests/test_ivy/test_functional/test_experimental/test_core/test_linalg.py
@@ -1,6 +1,7 @@
# global
import math
from hypothesis import strategies as st
+from hypothesis import assume
import numpy as np
import pytest
import itertools
@@ -309,6 +310,48 @@ def _generate_multi_dot_dtype_and_arrays(draw):
return input_dtype, [matrix_1[1][0], matrix_2[1][0], matrix_3[1][0]]
+# solve_triangular
+@st.composite
+def _generate_solve_triangular_args(draw):
+ shape = draw(
+ st.lists(st.integers(min_value=1, max_value=3), min_size=2, max_size=5)
+ )
+ shape_b = list(shape)
+ shape_a = list(shape)
+ shape_a[-1] = shape_a[-2] # Make square
+
+ dtype_a, a = draw(
+ helpers.dtype_and_values(
+ shape=shape_a,
+ available_dtypes=helpers.get_dtypes("float"),
+ min_value=-10,
+ max_value=10,
+ )
+ )
+
+ dtype_b, b = draw(
+ helpers.dtype_and_values(
+ shape=shape_b,
+ available_dtypes=helpers.get_dtypes("float"),
+ min_value=-10,
+ max_value=10,
+ )
+ )
+
+ dtype_a = dtype_a[0]
+ dtype_b = dtype_b[0]
+ a = a[0]
+ b = b[0]
+ upper = draw(st.booleans())
+ adjoint = draw(st.booleans())
+ unit_diagonal = draw(st.booleans())
+
+ for i in range(shape_a[-2]):
+ a[ivy.abs(a[..., i, i]) < 0.01, i, i] = 0.01 # Make diagonals non-zero
+
+ return upper, adjoint, unit_diagonal, [dtype_a, dtype_b], [a, b]
+
+
@st.composite
def _get_dtype_value1_value2_cov(
draw,
@@ -415,7 +458,7 @@ def _higher_order_moment_data(draw):
return dtype, x[0], order
-# intialize tucker
+# initialize tucker
@st.composite
def _initialize_tucker_data(draw):
x_dtype, x, shape = draw(
@@ -671,6 +714,30 @@ def _partial_tucker_data(draw):
)
+# tensor train
+@st.composite
+def _tensor_train_data(draw):
+ x_dtype, x, shape = draw(
+ helpers.dtype_and_values(
+ available_dtypes=helpers.get_dtypes("float"),
+ min_value=0.1,
+ max_value=10,
+ min_num_dims=2,
+ max_num_dims=5,
+ min_dim_size=2,
+ max_dim_size=5,
+ ret_shape=True,
+ ).filter(lambda x: "float16" not in x[0] and "bfloat16" not in x[0])
+ )
+ dims = len(shape)
+ rank = [1]
+ for i in range(dims - 1):
+ rank.append(draw(helpers.ints(min_value=1, max_value=shape[i])))
+ rank.append(1)
+
+ return x_dtype, x[0], rank
+
+
# truncated svd
@st.composite
def _truncated_svd_data(draw):
@@ -1225,7 +1292,7 @@ def test_khatri_rao(*, data, test_flags, backend_fw, fn_name, on_device):
# The following two tests have been adapted from TensorLy
# https://github.com/tensorly/tensorly/blob/main/tensorly/tenalg/tests/test_khatri_rao.py
-@pytest.mark.parametrize("columns, rows", [(4, [3, 4, 2])])
+@pytest.mark.parametrize(("columns", "rows"), [(4, [3, 4, 2])])
def test_khatri_rao_tensorly_1(columns, rows):
columns = columns
rows = rows
@@ -1239,7 +1306,7 @@ def test_khatri_rao_tensorly_1(columns, rows):
@pytest.mark.parametrize(
- "t1, t2, true_res",
+ ("t1", "t2", "true_res"),
[
(
[[1, 2, 3], [4, 5, 6], [7, 8, 9]],
@@ -1410,7 +1477,7 @@ def test_mode_dot(*, data, test_flags, backend_fw, fn_name, on_device):
@pytest.mark.parametrize(
- "X, U, true_res",
+ ("X", "U", "true_res"),
[
(
[
@@ -1478,7 +1545,7 @@ def test_multi_mode_dot(*, data, test_flags, backend_fw, fn_name, on_device):
# The following 2 tests have been adapted from TensorLy
# https://github.com/tensorly/tensorly/blob/main/tensorly/tenalg/tests/test_n_mode_product.py#L81
@pytest.mark.parametrize(
- "X, U, true_res",
+ ("X", "U", "true_res"),
[
([[1, 2], [0, -1]], [[2, 1], [-1, 1]], [1]),
],
@@ -1489,7 +1556,12 @@ def test_multi_mode_dot_tensorly_1(X, U, true_res):
assert np.allclose(true_res, res)
-@pytest.mark.parametrize("shape", ((3, 5, 4, 2),))
+@pytest.mark.parametrize(
+ "shape",
+ [
+ (3, 5, 4, 2),
+ ],
+)
def test_multi_mode_dot_tensorly_2(shape):
print(shape)
X = ivy.ones(shape)
@@ -1557,7 +1629,7 @@ def test_partial_tucker(*, data, test_flags, backend_fw, fn_name, on_device):
# test adapted from TensorLy
# https://github.com/tensorly/tensorly/blob/main/tensorly/decomposition/tests/test_tucker.py#L24
@pytest.mark.parametrize(
- "tol_norm_2, tol_max_abs, modes, shape",
+ ("tol_norm_2", "tol_max_abs", "modes", "shape"),
[
(
10e-3,
@@ -1620,6 +1692,32 @@ def test_partial_tucker_tensorly(tol_norm_2, tol_max_abs, modes, shape):
np.allclose(factor1, factor2)
+@handle_test(
+ fn_tree="functional.ivy.experimental.solve_triangular",
+ data=_generate_solve_triangular_args(),
+ test_instance_method=st.just(False),
+)
+def test_solve_triangular(*, data, test_flags, backend_fw, fn_name, on_device):
+ # Temporarily ignore gradients on paddlepaddle backend
+ # See: https://github.com/unifyai/ivy/pull/25917
+ assume(not (backend_fw == "paddle" and test_flags.test_gradients))
+ upper, adjoint, unit_diagonal, input_dtypes, x = data
+ helpers.test_function(
+ backend_to_test=backend_fw,
+ test_flags=test_flags,
+ fn_name=fn_name,
+ on_device=on_device,
+ rtol_=1e-3,
+ atol_=1e-3,
+ input_dtypes=input_dtypes,
+ x1=x[0],
+ x2=x[1],
+ upper=upper,
+ adjoint=adjoint,
+ unit_diagonal=unit_diagonal,
+ )
+
+
@handle_test(
fn_tree="functional.ivy.experimental.svd_flip",
uv=helpers.dtype_and_values(
@@ -1647,6 +1745,101 @@ def test_svd_flip(*, uv, u_based_decision, test_flags, backend_fw, fn_name, on_d
)
+@handle_test(
+ fn_tree="functional.ivy.experimental.tensor_train",
+ data=_tensor_train_data(),
+ # TODO: add support for more modes
+ svd=st.just("truncated_svd"),
+ test_with_out=st.just(False),
+ test_gradients=st.just(False),
+)
+def test_tensor_train(*, data, svd, test_flags, backend_fw, fn_name, on_device):
+ input_dtype, x, rank = data
+ results = helpers.test_function(
+ backend_to_test=backend_fw,
+ test_flags=test_flags,
+ fn_name=fn_name,
+ on_device=on_device,
+ input_dtypes=input_dtype,
+ input_tensor=x,
+ rank=rank,
+ svd=svd,
+ test_values=False,
+ )
+
+ ret_np, ret_from_gt_np = results
+
+ factors = helpers.flatten_and_to_np(ret=ret_np, backend=backend_fw)
+ factors_gt = helpers.flatten_and_to_np(
+ ret=ret_from_gt_np, backend=test_flags.ground_truth_backend
+ )
+
+ for f, f_gt in zip(factors, factors_gt):
+ assert np.prod(f.shape) == np.prod(f_gt.shape)
+
+
+# The following 3 tests have been adapted from TensorLy
+# https://github.com/tensorly/tensorly/blob/main/tensorly/decomposition/tests/test_tt_decomposition.py
+@pytest.mark.parametrize(
+ ("shape", "rank"), [((3, 4, 5, 6, 2, 10), (1, 3, 3, 4, 2, 2, 1))]
+)
+def test_tensor_train_tensorly_1(shape, rank):
+ tensor = ivy.random_uniform(shape=shape)
+ tensor_shape = tensor.shape
+ factors = ivy.tensor_train(tensor, rank)
+
+ assert len(factors) == 6, "Number of factors should be 6, currently has " + str(
+ len(factors)
+ )
+
+ r_prev_iteration = 1
+ for k in range(6):
+ (r_prev_k, n_k, r_k) = factors[k].shape
+ assert tensor_shape[k] == n_k, (
+ "Mode 1 of factor "
+ + str(k)
+ + "needs "
+ + str(tensor_shape[k])
+ + " dimensions, currently has "
+ + str(n_k)
+ )
+ assert r_prev_k == r_prev_iteration, " Incorrect ranks of factors "
+ r_prev_iteration = r_k
+
+
+@pytest.mark.parametrize(
+ ("shape", "rank"), [((3, 4, 5, 6, 2, 10), (1, 5, 4, 3, 8, 10, 1))]
+)
+def test_tensor_train_tensorly_2(shape, rank):
+ tensor = ivy.random_uniform(shape=shape)
+ factors = ivy.tensor_train(tensor, rank)
+
+ for k in range(6):
+ (r_prev, n_k, r_k) = factors[k].shape
+
+ first_error_message = (
+ "TT rank " + str(k) + " is greater than the maximum allowed "
+ )
+ first_error_message += str(r_prev) + " > " + str(rank[k])
+ assert r_prev <= rank[k], first_error_message
+
+ first_error_message = (
+ "TT rank " + str(k + 1) + " is greater than the maximum allowed "
+ )
+ first_error_message += str(r_k) + " > " + str(rank[k + 1])
+ assert r_k <= rank[k + 1], first_error_message
+
+
+@pytest.mark.parametrize(("shape", "rank", "tol"), [((3, 3, 3), (1, 3, 3, 1), (10e-5))])
+def test_tensor_train_tensorly_3(shape, rank, tol):
+ tensor = ivy.random_uniform(shape=shape)
+ factors = ivy.tensor_train(tensor, rank)
+ reconstructed_tensor = ivy.TTTensor.tt_to_tensor(factors)
+ error = ivy.vector_norm(ivy.matrix_norm(tensor - reconstructed_tensor, ord=2))
+ error /= ivy.vector_norm(ivy.matrix_norm(tensor, ord=2))
+ np.testing.assert_(error < tol, "norm 2 of reconstruction higher than tol")
+
+
@handle_test(
fn_tree="functional.ivy.experimental.truncated_svd",
data=_truncated_svd_data(),
@@ -1805,7 +1998,8 @@ def test_tucker(*, data, test_flags, backend_fw, fn_name, on_device):
# test adapted from tensorly
# https://github.com/tensorly/tensorly/blob/main/tensorly/decomposition/tests/test_tucker.py#L71
@pytest.mark.parametrize(
- "tol_norm_2, tol_max_abs, shape, ranks", [(10e-3, 10e-1, (3, 4, 3), [2, 3, 1])]
+ ("tol_norm_2", "tol_max_abs", "shape", "ranks"),
+ [(10e-3, 10e-1, (3, 4, 3), [2, 3, 1])],
)
def test_tucker_tensorly(tol_norm_2, tol_max_abs, shape, ranks):
tensor = ivy.random_uniform(shape=shape)
diff --git a/ivy_tests/test_ivy/test_functional/test_experimental/test_core/test_manipulation.py b/ivy_tests/test_ivy/test_functional/test_experimental/test_core/test_manipulation.py
index b69a12d923fed..c008dcb0b4cf6 100644
--- a/ivy_tests/test_ivy/test_functional/test_experimental/test_core/test_manipulation.py
+++ b/ivy_tests/test_ivy/test_functional/test_experimental/test_core/test_manipulation.py
@@ -505,6 +505,7 @@ def st_tuples(elements, *, min_size=0, max_size=None, unique_by=None, unique=Fal
test_with_out=st.just(False),
test_gradients=st.just(False),
ground_truth_backend="numpy",
+ test_with_copy=st.just(True),
)
def test_as_strided(*, all_args, test_flags, backend_fw, fn_name, on_device):
dtype, x, shape, strides = all_args
@@ -555,6 +556,7 @@ def test_associative_scan(
),
test_with_out=st.just(False),
test_gradients=st.just(False),
+ test_with_copy=st.just(True),
)
def test_atleast_1d(dtype_and_x, test_flags, backend_fw, fn_name, on_device):
input_dtypes, arrays = dtype_and_x
@@ -581,6 +583,7 @@ def test_atleast_1d(dtype_and_x, test_flags, backend_fw, fn_name, on_device):
),
test_with_out=st.just(False),
test_gradients=st.just(False),
+ test_with_copy=st.just(True),
)
def test_atleast_2d(*, dtype_and_x, test_flags, backend_fw, fn_name, on_device):
input_dtypes, arrays = dtype_and_x
@@ -607,6 +610,7 @@ def test_atleast_2d(*, dtype_and_x, test_flags, backend_fw, fn_name, on_device):
),
test_with_out=st.just(False),
test_gradients=st.just(False),
+ test_with_copy=st.just(True),
)
def test_atleast_3d(*, dtype_and_x, test_flags, backend_fw, fn_name, on_device):
input_dtypes, arrays = dtype_and_x
@@ -675,7 +679,7 @@ def test_column_stack(*, arrays_dtypes, test_flags, backend_fw, fn_name, on_devi
test_instance_method=st.just(False),
)
def test_concat_from_sequence(
- *, dtypes_arrays_axis, new_axis, test_flags, backend_fw, fn_name, on_device
+ *, dtypes_arrays_axis, new_axis, test_flags, backend_fw, fn_name, on_device: str
):
dtypes, arrays, axis = dtypes_arrays_axis
@@ -685,7 +689,7 @@ def test_concat_from_sequence(
backend_to_test=backend_fw,
fn_name=fn_name,
on_device=on_device,
- input_sequence=arrays,
+ *arrays,
new_axis=new_axis,
axis=axis,
)
@@ -694,13 +698,7 @@ def test_concat_from_sequence(
# dsplit
@handle_test(
fn_tree="functional.ivy.experimental.dsplit",
- dtype_and_x=helpers.dtype_and_values(
- available_dtypes=helpers.get_dtypes("valid"),
- shape=st.shared(helpers.get_shape(min_num_dims=3), key="value_shape"),
- ),
- indices_or_sections=_get_splits(allow_none=False, min_num_dims=3, axis=2),
- test_gradients=st.just(False),
- test_with_out=st.just(False),
+ test_with_copy=st.just(True),
)
def test_dsplit(
dtype_and_x, indices_or_sections, test_flags, backend_fw, fn_name, on_device
@@ -708,10 +706,10 @@ def test_dsplit(
input_dtype, x = dtype_and_x
helpers.test_function(
input_dtypes=input_dtype,
+ on_device=on_device,
test_flags=test_flags,
backend_to_test=backend_fw,
fn_name=fn_name,
- on_device=on_device,
x=x[0],
indices_or_sections=indices_or_sections,
)
@@ -770,6 +768,7 @@ def test_dstack(*, dtype_and_x, test_flags, backend_fw, fn_name, on_device):
container_flags=st.just([False]),
test_instance_method=st.just(False),
test_gradients=st.just(False),
+ test_with_copy=st.just(True),
)
def test_expand(*, dtype_and_x, shape, test_flags, backend_fw, fn_name, on_device):
dtype, x = dtype_and_x
@@ -831,6 +830,7 @@ def test_fill_diagonal(
@handle_test(
fn_tree="functional.ivy.experimental.flatten",
data=_flatten_data_helper(),
+ test_with_copy=st.just(True),
)
def test_flatten(
*,
@@ -858,10 +858,11 @@ def test_flatten(
@handle_test(
fn_tree="functional.ivy.experimental.fliplr",
dtype_and_m=helpers.dtype_and_values(
- available_dtypes=helpers.get_dtypes("numeric"),
+ available_dtypes=helpers.get_dtypes("valid"),
min_num_dims=2,
),
test_gradients=st.just(False),
+ test_with_copy=st.just(True),
)
def test_fliplr(*, dtype_and_m, test_flags, backend_fw, fn_name, on_device):
input_dtype, m = dtype_and_m
@@ -879,7 +880,7 @@ def test_fliplr(*, dtype_and_m, test_flags, backend_fw, fn_name, on_device):
@handle_test(
fn_tree="functional.ivy.experimental.flipud",
dtype_and_m=helpers.dtype_and_values(
- available_dtypes=helpers.get_dtypes("float"),
+ available_dtypes=helpers.get_dtypes("valid"),
min_value=-100,
max_value=100,
min_num_dims=1,
@@ -888,6 +889,7 @@ def test_fliplr(*, dtype_and_m, test_flags, backend_fw, fn_name, on_device):
max_dim_size=3,
),
test_gradients=st.just(False),
+ test_with_copy=st.just(True),
)
def test_flipud(*, dtype_and_m, test_flags, backend_fw, fn_name, on_device):
input_dtype, m = dtype_and_m
@@ -962,6 +964,7 @@ def test_heaviside(*, dtype_and_x, test_flags, backend_fw, fn_name, on_device):
indices_or_sections=_get_splits(allow_none=False, min_num_dims=2, axis=1),
test_gradients=st.just(False),
test_with_out=st.just(False),
+ test_with_copy=st.just(True),
)
def test_hsplit(
dtype_and_x, indices_or_sections, test_flags, backend_fw, fn_name, on_device
@@ -1059,7 +1062,7 @@ def test_matricize(*, data, test_flags, backend_fw, fn_name, on_device):
@handle_test(
fn_tree="functional.ivy.experimental.moveaxis",
dtype_and_a=helpers.dtype_and_values(
- available_dtypes=helpers.get_dtypes("float"),
+ available_dtypes=helpers.get_dtypes("valid"),
min_value=-100,
max_value=100,
shape=st.shared(
@@ -1103,6 +1106,7 @@ def test_matricize(*, data, test_flags, backend_fw, fn_name, on_device):
force_int=True,
),
test_gradients=st.just(False),
+ test_with_copy=st.just(True),
)
def test_moveaxis(
*, dtype_and_a, source, destination, test_flags, backend_fw, fn_name, on_device
@@ -1283,13 +1287,14 @@ def test_put_along_axis(
@handle_test(
fn_tree="functional.ivy.experimental.rot90",
dtype_m_k_axes=_get_dtype_values_k_axes_for_rot90(
- available_dtypes=helpers.get_dtypes("numeric"),
+ available_dtypes=helpers.get_dtypes("valid"),
min_num_dims=1,
max_num_dims=5,
min_dim_size=1,
max_dim_size=10,
),
test_gradients=st.just(False),
+ test_with_copy=st.just(True),
)
def test_rot90(dtype_m_k_axes, test_flags, backend_fw, fn_name, on_device):
input_dtype, m, k, axes = dtype_m_k_axes
@@ -1324,11 +1329,49 @@ def test_soft_thresholding(*, data, test_flags, backend_fw, fn_name, on_device):
)
+@handle_test(
+ fn_tree="functional.ivy.experimental.take",
+ dtype_x_indices_axis=helpers.array_indices_axis(
+ array_dtypes=helpers.get_dtypes("valid"),
+ indices_dtypes=["int32", "int64"],
+ min_num_dims=1,
+ max_num_dims=3,
+ min_dim_size=1,
+ max_dim_size=5,
+ indices_same_dims=False,
+ valid_bounds=False,
+ ),
+ mode=st.sampled_from(["clip", "wrap", "fill"]),
+ ground_truth_backend="jax",
+)
+def test_take(
+ *,
+ dtype_x_indices_axis,
+ mode,
+ test_flags,
+ backend_fw,
+ fn_name,
+ on_device,
+):
+ dtypes, x, indices, axis, _ = dtype_x_indices_axis
+ helpers.test_function(
+ input_dtypes=dtypes,
+ test_flags=test_flags,
+ backend_to_test=backend_fw,
+ fn_name=fn_name,
+ on_device=on_device,
+ x=x,
+ indices=indices,
+ axis=axis,
+ mode=mode,
+ )
+
+
# take_along_axis
@handle_test(
fn_tree="functional.ivy.experimental.take_along_axis",
dtype_x_indices_axis=helpers.array_indices_axis(
- array_dtypes=helpers.get_dtypes("numeric"),
+ array_dtypes=helpers.get_dtypes("valid"),
indices_dtypes=["int32", "int64"],
min_num_dims=1,
max_num_dims=5,
@@ -1396,6 +1439,37 @@ def test_top_k(
)
+@handle_test(
+ fn_tree="trim_zeros",
+ dt_a=helpers.dtype_and_values(
+ available_dtypes=helpers.get_dtypes("numeric"),
+ num_arrays=1,
+ min_num_dims=1,
+ max_num_dims=1,
+ min_value=-100,
+ max_value=100,
+ ),
+ test_with_out=st.just(False),
+)
+def test_trim_zeros(
+ *,
+ dt_a,
+ test_flags,
+ backend_fw,
+ fn_name,
+ on_device,
+):
+ dt, a = dt_a
+ helpers.test_function(
+ input_dtypes=dt,
+ test_flags=test_flags,
+ on_device=on_device,
+ fw=backend_fw,
+ fn_name=fn_name,
+ a=a[0],
+ )
+
+
@handle_test(
fn_tree="functional.ivy.experimental.unfold",
dtype_values_axis=helpers.dtype_values_axis(
@@ -1465,6 +1539,7 @@ def test_unique_consecutive(
indices_or_sections=_get_splits(allow_none=False, min_num_dims=2, axis=0),
test_gradients=st.just(False),
test_with_out=st.just(False),
+ test_with_copy=st.just(True),
)
def test_vsplit(
dtype_and_x, indices_or_sections, test_flags, backend_fw, fn_name, on_device
diff --git a/ivy_tests/test_ivy/test_functional/test_experimental/test_core/test_statistical.py b/ivy_tests/test_ivy/test_functional/test_experimental/test_core/test_statistical.py
index 716ff0ae7353c..6ceea7b2c08a1 100644
--- a/ivy_tests/test_ivy/test_functional/test_experimental/test_core/test_statistical.py
+++ b/ivy_tests/test_ivy/test_functional/test_experimental/test_core/test_statistical.py
@@ -19,6 +19,9 @@
def _get_castable_float_dtype_nan(draw, min_value=None, max_value=None):
available_dtypes = helpers.get_dtypes("float")
shape = draw(helpers.get_shape(min_num_dims=1, max_num_dims=4, max_dim_size=6))
+ dtype3, where = draw(
+ helpers.dtype_and_values(available_dtypes=["bool"], shape=shape)
+ )
dtype, values = draw(
helpers.dtype_and_values(
available_dtypes=available_dtypes,
@@ -36,7 +39,7 @@ def _get_castable_float_dtype_nan(draw, min_value=None, max_value=None):
dtype1, values, dtype2 = draw(
helpers.get_castable_dtype(draw(available_dtypes), dtype[0], values[0])
)
- return dtype1, [values], axis, dtype2
+ return dtype1, [values], axis, dtype2, dtype3, where
@st.composite
@@ -493,7 +496,7 @@ def test_cummin(
# - Error description: typo that throws unintended exceptions when using both
# weights and multiple axis.
# - fixed in TFP 0.20 release.
-# - Test helper needs to be modified to handle this case in older verions.
+# - Test helper needs to be modified to handle this case in older versions.
@handle_test(
fn_tree="functional.ivy.experimental.histogram",
values=_histogram_helper(),
@@ -590,7 +593,7 @@ def test_median(*, dtype_x_axis, keep_dims, test_flags, backend_fw, fn_name, on_
fn_tree="functional.ivy.experimental.nanmean",
dtype_x_axis=_statistical_dtype_values(function="nanmean"),
keep_dims=st.booleans(),
- dtype=helpers.get_dtypes("float", full=False),
+ dtype=helpers.get_dtypes("valid", full=False),
test_gradients=st.just(False),
)
def test_nanmean(
@@ -616,7 +619,7 @@ def test_nanmean(
fn_tree="functional.ivy.experimental.nanmedian",
dtype_x_axis=_statistical_dtype_values(function="nanmedian"),
keep_dims=st.booleans(),
- dtype=helpers.get_dtypes("float", full=False),
+ dtype=helpers.get_dtypes("valid", full=False),
overwriteinput=st.booleans(),
test_gradients=st.just(False),
)
@@ -646,6 +649,41 @@ def test_nanmedian(
)
+@handle_test(
+ fn_tree="functional.ivy.experimental.nanmin",
+ dtype_x_axis_castable=_get_castable_float_dtype_nan(),
+ test_gradients=st.just(False),
+ initial=st.integers(min_value=-5, max_value=5),
+ keep_dims=st.booleans(),
+)
+def test_nanmin(
+ *,
+ dtype_x_axis_castable,
+ initial,
+ keep_dims,
+ test_flags,
+ backend_fw,
+ fn_name,
+ on_device,
+):
+ input_dtype, x, axis, castable_dtype, dtype3, where = dtype_x_axis_castable
+ x = x[0]
+ helpers.test_function(
+ input_dtypes=[input_dtype, dtype3[0]],
+ test_flags=test_flags,
+ rtol_=1e-1,
+ atol_=1e-1,
+ backend_to_test=backend_fw,
+ fn_name=fn_name,
+ on_device=on_device,
+ a=x,
+ axis=axis,
+ keepdims=keep_dims,
+ initial=initial,
+ where=where[0],
+ )
+
+
@handle_test(
fn_tree="functional.ivy.experimental.nanprod",
dtype_x_axis_castable=_get_castable_float_dtype_nan(),
diff --git a/ivy_tests/test_ivy/test_functional/test_experimental/test_nn/test_activations.py b/ivy_tests/test_ivy/test_functional/test_experimental/test_nn/test_activations.py
index c1a12da9ab139..5a79ef33ad3cf 100644
--- a/ivy_tests/test_ivy/test_functional/test_experimental/test_nn/test_activations.py
+++ b/ivy_tests/test_ivy/test_functional/test_experimental/test_nn/test_activations.py
@@ -6,11 +6,41 @@
from ivy_tests.test_ivy.helpers import handle_test
+# celu
+@handle_test(
+ fn_tree="functional.ivy.experimental.celu",
+ dtype_and_x=helpers.dtype_and_values(
+ available_dtypes=helpers.get_dtypes("float_and_complex"),
+ large_abs_safety_factor=8,
+ small_abs_safety_factor=8,
+ safety_factor_scale="log",
+ ),
+ alpha=st.floats(min_value=0.1, max_value=1.0),
+ complex_mode=st.sampled_from(["jax", "split", "magnitude"]),
+)
+def test_celu(
+ *, dtype_and_x, alpha, complex_mode, test_flags, backend_fw, fn_name, on_device
+):
+ dtype, x = dtype_and_x
+ helpers.test_function(
+ input_dtypes=dtype,
+ backend_to_test=backend_fw,
+ test_flags=test_flags,
+ fn_name=fn_name,
+ on_device=on_device,
+ rtol_=1e-2,
+ atol_=1e-2,
+ x=x[0],
+ alpha=alpha,
+ complex_mode=complex_mode,
+ )
+
+
# elu
@handle_test(
fn_tree="functional.ivy.experimental.elu",
dtype_and_x=helpers.dtype_and_values(
- available_dtypes=helpers.get_dtypes("float"),
+ available_dtypes=helpers.get_dtypes("valid"),
large_abs_safety_factor=8,
small_abs_safety_factor=8,
safety_factor_scale="log",
@@ -40,6 +70,34 @@ def test_elu(
)
+# hardshrink
+@handle_test(
+ fn_tree="functional.ivy.experimental.hardshrink",
+ dtype_and_x=helpers.dtype_and_values(
+ available_dtypes=helpers.get_dtypes("valid"),
+ large_abs_safety_factor=8,
+ small_abs_safety_factor=8,
+ safety_factor_scale="log",
+ ),
+ threshold=st.one_of(
+ st.floats(min_value=0.0, max_value=1e30),
+ ),
+)
+def test_hardshrink(
+ *, dtype_and_x, threshold, test_flags, backend_fw, fn_name, on_device
+):
+ dtype, x = dtype_and_x
+ helpers.test_function(
+ input_dtypes=dtype,
+ backend_to_test=backend_fw,
+ test_flags=test_flags,
+ fn_name=fn_name,
+ on_device=on_device,
+ x=x[0],
+ lambd=threshold,
+ )
+
+
# hardtanh
@handle_test(
fn_tree="functional.ivy.experimental.hardtanh",
@@ -178,6 +236,36 @@ def test_relu6(
)
+# scaled_tanh
+@handle_test(
+ fn_tree="functional.ivy.experimental.scaled_tanh",
+ dtype_and_x=helpers.dtype_and_values(
+ available_dtypes=helpers.get_dtypes("valid"),
+ min_dim_size=1,
+ min_num_dims=1,
+ ),
+ alpha=st.floats(min_value=0.1, max_value=5.0),
+ beta=st.floats(min_value=0.1, max_value=5.0),
+ ground_truth_backend="paddle",
+)
+def test_scaled_tanh(
+ *, dtype_and_x, alpha, beta, test_flags, backend_fw, fn_name, on_device
+):
+ dtype, x = dtype_and_x
+ helpers.test_function(
+ input_dtypes=dtype,
+ backend_to_test=backend_fw,
+ test_flags=test_flags,
+ fn_name=fn_name,
+ on_device=on_device,
+ rtol_=1e-5,
+ atol_=1e-5,
+ x=x[0],
+ alpha=alpha,
+ beta=beta,
+ )
+
+
# selu
@handle_test(
fn_tree="functional.ivy.experimental.selu",
@@ -206,7 +294,7 @@ def test_selu(*, dtype_and_input, test_flags, backend_fw, fn_name, on_device):
@handle_test(
fn_tree="functional.ivy.experimental.silu",
dtype_and_x=helpers.dtype_and_values(
- available_dtypes=helpers.get_dtypes("float"),
+ available_dtypes=helpers.get_dtypes("valid"),
large_abs_safety_factor=8,
small_abs_safety_factor=8,
safety_factor_scale="log",
@@ -226,6 +314,90 @@ def test_silu(*, dtype_and_x, test_flags, backend_fw, fn_name, on_device):
)
+# softshrink
+@handle_test(
+ fn_tree="functional.ivy.experimental.softshrink",
+ dtype_and_x=helpers.dtype_and_values(
+ available_dtypes=helpers.get_dtypes("valid"),
+ large_abs_safety_factor=8,
+ small_abs_safety_factor=8,
+ safety_factor_scale="log",
+ ),
+ threshold=st.one_of(
+ st.floats(min_value=0.0, max_value=1e30),
+ ),
+)
+def test_softshrink(
+ *, dtype_and_x, threshold, test_flags, backend_fw, fn_name, on_device
+):
+ dtype, x = dtype_and_x
+ helpers.test_function(
+ input_dtypes=dtype,
+ backend_to_test=backend_fw,
+ test_flags=test_flags,
+ fn_name=fn_name,
+ on_device=on_device,
+ x=x[0],
+ lambd=threshold,
+ )
+
+
+# tanhshrink
+@handle_test(
+ fn_tree="functional.ivy.experimental.tanhshrink",
+ dtype_and_x=helpers.dtype_and_values(
+ available_dtypes=helpers.get_dtypes("float"),
+ large_abs_safety_factor=8,
+ small_abs_safety_factor=8,
+ safety_factor_scale="log",
+ ),
+)
+def test_tanhshrink(*, dtype_and_x, test_flags, backend_fw, fn_name, on_device):
+ dtype, x = dtype_and_x
+ helpers.test_function(
+ input_dtypes=dtype,
+ backend_to_test=backend_fw,
+ test_flags=test_flags,
+ fn_name=fn_name,
+ on_device=on_device,
+ rtol_=1e-02,
+ atol_=1e-02,
+ x=x[0],
+ )
+
+
+# threshold
+@handle_test(
+ fn_tree="functional.ivy.experimental.threshold",
+ dtype_and_x=helpers.dtype_and_values(
+ available_dtypes=helpers.get_dtypes("valid"),
+ large_abs_safety_factor=8,
+ small_abs_safety_factor=8,
+ safety_factor_scale="log",
+ ),
+ threshold=st.one_of(
+ st.floats(min_value=-1e30, max_value=1e30),
+ ),
+ value=st.one_of(
+ st.floats(min_value=-1e30, max_value=1e30),
+ ),
+)
+def test_threshold(
+ *, dtype_and_x, threshold, value, test_flags, backend_fw, fn_name, on_device
+):
+ dtype, x = dtype_and_x
+ helpers.test_function(
+ input_dtypes=dtype,
+ backend_to_test=backend_fw,
+ test_flags=test_flags,
+ fn_name=fn_name,
+ on_device=on_device,
+ x=x[0],
+ threshold=threshold,
+ value=value,
+ )
+
+
# thresholded_relu
@handle_test(
fn_tree="functional.ivy.experimental.thresholded_relu",
diff --git a/ivy_tests/test_ivy/test_functional/test_experimental/test_nn/test_layers.py b/ivy_tests/test_ivy/test_functional/test_experimental/test_nn/test_layers.py
index 7e139b27b6b72..1840881cf128c 100644
--- a/ivy_tests/test_ivy/test_functional/test_experimental/test_nn/test_layers.py
+++ b/ivy_tests/test_ivy/test_functional/test_experimental/test_nn/test_layers.py
@@ -31,31 +31,31 @@ def _interp_args(draw, mode=None, mode_list=None):
"nearest",
"nearest-exact",
"area",
+ "bicubic",
]
-
tf_modes = [
"linear",
"bilinear",
"trilinear",
"nearest-exact",
"tf_area",
- "bicubic_tensorflow",
+ "tf_bicubic",
"lanczos3",
"lanczos5",
"mitchellcubic",
"gaussian",
]
-
jax_modes = [
"linear",
"bilinear",
"trilinear",
"nearest-exact",
- "bicubic_tensorflow",
+ "tf_bicubic",
"lanczos3",
"lanczos5",
]
-
+ if mode_list == "torch":
+ mode_list = torch_modes
if not mode and not mode_list:
if curr_backend == "torch" and not mixed_fn_compos:
mode = draw(st.sampled_from(torch_modes))
@@ -74,7 +74,7 @@ def _interp_args(draw, mode=None, mode_list=None):
"nearest-exact",
"area",
"tf_area",
- "bicubic_tensorflow",
+ "tf_bicubic",
"lanczos3",
"lanczos5",
"mitchellcubic",
@@ -84,14 +84,11 @@ def _interp_args(draw, mode=None, mode_list=None):
)
elif mode_list:
mode = draw(st.sampled_from(mode_list))
- align_corners = draw(st.one_of(st.booleans(), st.none()))
- if (curr_backend == "tensorflow" or curr_backend == "jax") and not mixed_fn_compos:
- align_corners = False
if mode == "linear":
num_dims = 3
elif mode in [
"bilinear",
- "bicubic_tensorflow",
+ "tf_bicubic",
"bicubic",
"mitchellcubic",
"gaussian",
@@ -113,7 +110,6 @@ def _interp_args(draw, mode=None, mode_list=None):
)
+ 2
)
- align_corners = None
if curr_backend == "tensorflow" and not mixed_fn_compos:
num_dims = 3
dtype, x = draw(
@@ -125,47 +121,36 @@ def _interp_args(draw, mode=None, mode_list=None):
max_num_dims=num_dims,
min_dim_size=2,
max_dim_size=5,
- large_abs_safety_factor=50,
- small_abs_safety_factor=50,
- safety_factor_scale="log",
+ max_value=1e04,
+ min_value=-1e04,
+ abs_smallest_val=1e-04,
)
)
+ align_corners = draw(st.booleans())
if draw(st.booleans()):
- scale_factor = draw(
- st.one_of(
- helpers.lists(
- x=helpers.floats(
- min_value=1.0, max_value=2.0, mixed_fn_compos=mixed_fn_compos
- ),
- min_size=num_dims - 2,
- max_size=num_dims - 2,
- ),
- helpers.floats(
- min_value=1.0, max_value=2.0, mixed_fn_compos=mixed_fn_compos
- ),
+ if draw(st.booleans()):
+ scale_factor = draw(
+ st.floats(min_value=max([1 / d for d in x[0].shape[2:]]), max_value=3)
)
- )
+ else:
+ scale_factor = []
+ for s in x[0].shape[2:]:
+ scale_factor += [draw(st.floats(min_value=1 / s, max_value=3))]
recompute_scale_factor = draw(st.booleans())
size = None
else:
size = draw(
st.one_of(
- helpers.lists(
- x=helpers.ints(
- min_value=1, max_value=3, mixed_fn_compos=mixed_fn_compos
- ),
+ st.lists(
+ st.integers(min_value=1, max_value=3 * max(x[0].shape)),
min_size=num_dims - 2,
max_size=num_dims - 2,
),
- st.integers(min_value=1, max_value=3),
+ st.integers(min_value=1, max_value=3 * max(x[0].shape)),
)
)
- recompute_scale_factor = False
+ recompute_scale_factor = None
scale_factor = None
- if (curr_backend == "tensorflow" or curr_backend == "jax") and not mixed_fn_compos:
- if not recompute_scale_factor:
- recompute_scale_factor = True
-
return (dtype, x, mode, size, align_corners, scale_factor, recompute_scale_factor)
@@ -385,6 +370,29 @@ def _x_and_ifftn(draw):
return dtype, x, s, axes, norm
+@st.composite
+def _x_and_rfft(draw):
+ min_fft_points = 2
+ dtype = draw(helpers.get_dtypes("numeric"))
+ x_dim = draw(
+ helpers.get_shape(
+ min_dim_size=2, max_dim_size=100, min_num_dims=1, max_num_dims=4
+ )
+ )
+ x = draw(
+ helpers.array_values(
+ dtype=dtype[0],
+ shape=tuple(x_dim),
+ min_value=-1e-10,
+ max_value=1e10,
+ )
+ )
+ axis = draw(st.integers(1 - len(list(x_dim)), len(list(x_dim)) - 1))
+ norm = draw(st.sampled_from(["backward", "forward", "ortho"]))
+ n = draw(st.integers(min_fft_points, 256))
+ return dtype, x, axis, norm, n
+
+
@st.composite
def _x_and_rfftn(draw):
min_rfftn_points = 2
@@ -1051,13 +1059,10 @@ def test_ifftn(
@handle_test(
fn_tree="functional.ivy.experimental.interpolate",
dtype_x_mode=_interp_args(),
- antialias=st.just(False),
test_gradients=st.just(False),
number_positional_args=st.just(2),
)
-def test_interpolate(
- dtype_x_mode, antialias, test_flags, backend_fw, fn_name, on_device
-):
+def test_interpolate(dtype_x_mode, test_flags, backend_fw, fn_name, on_device):
(
input_dtype,
x,
@@ -1074,12 +1079,11 @@ def test_interpolate(
fn_name=fn_name,
on_device=on_device,
rtol_=1e-01,
- atol_=1e-01,
+ atol_=1e-03,
x=x[0],
size=size,
mode=mode,
align_corners=align_corners,
- antialias=antialias,
scale_factor=scale_factor,
recompute_scale_factor=recompute_scale_factor,
)
@@ -1114,7 +1118,7 @@ def test_max_pool1d(
data_format = "NCW" if data_format == "channel_first" else "NWC"
assume(not (isinstance(pad, str) and (pad.upper() == "VALID") and ceil_mode))
# TODO: Remove this once the paddle backend supports dilation
- assume(not (backend_fw == "paddle" and max(list(dilation)) > 1))
+ assume(backend_fw != "paddle" or max(list(dilation)) <= 1)
helpers.test_function(
input_dtypes=dtype,
@@ -1175,7 +1179,7 @@ def test_max_pool2d(
data_format = "NCHW" if data_format == "channel_first" else "NHWC"
assume(not (isinstance(pad, str) and (pad.upper() == "VALID") and ceil_mode))
# TODO: Remove this once the paddle backend supports dilation
- assume(not (backend_fw == "paddle" and max(list(dilation)) > 1))
+ assume(backend_fw != "paddle" or max(list(dilation)) <= 1)
helpers.test_function(
input_dtypes=dtype,
@@ -1225,7 +1229,7 @@ def test_max_pool3d(
data_format = "NCDHW" if data_format == "channel_first" else "NDHWC"
assume(not (isinstance(pad, str) and (pad.upper() == "VALID") and ceil_mode))
# TODO: Remove this once the paddle backend supports dilation
- assume(not (backend_fw == "paddle" and max(list(dilation)) > 1))
+ assume(backend_fw != "paddle" or max(list(dilation)) <= 1)
helpers.test_function(
input_dtypes=dtype,
@@ -1302,6 +1306,35 @@ def test_reduce_window(*, all_args, test_flags, backend_fw, fn_name, on_device):
)
+@handle_test(
+ fn_tree="functional.ivy.experimental.rfft",
+ dtype_x_axis_norm_n=_x_and_rfft(),
+ ground_truth_backend="numpy",
+)
+def test_rfft(
+ *,
+ dtype_x_axis_norm_n,
+ test_flags,
+ backend_fw,
+ fn_name,
+ on_device,
+):
+ dtype, x, axis, norm, n = dtype_x_axis_norm_n
+ helpers.test_function(
+ input_dtypes=dtype,
+ test_flags=test_flags,
+ backend_to_test=backend_fw,
+ on_device=on_device,
+ fn_name=fn_name,
+ rtol_=1e-2,
+ atol_=1e-2,
+ x=x,
+ n=n,
+ axis=axis,
+ norm=norm,
+ )
+
+
@handle_test(
fn_tree="functional.ivy.experimental.rfftn",
d_x_d_s_n=_x_and_rfftn(),
diff --git a/ivy_tests/test_ivy/test_functional/test_experimental/test_nn/test_losses.py b/ivy_tests/test_ivy/test_functional/test_experimental/test_nn/test_losses.py
index dc35af8445ee6..2161ee814304b 100644
--- a/ivy_tests/test_ivy/test_functional/test_experimental/test_nn/test_losses.py
+++ b/ivy_tests/test_ivy/test_functional/test_experimental/test_nn/test_losses.py
@@ -280,6 +280,7 @@ def test_poisson_nll_loss(
),
beta=helpers.floats(min_value=0.0, max_value=1.0),
reduction=st.sampled_from(["none", "sum", "mean"]),
+ ground_truth_backend="torch",
)
def test_smooth_l1_loss(
dtype_and_input,
diff --git a/ivy_tests/test_ivy/test_functional/test_nn/test_activations.py b/ivy_tests/test_ivy/test_functional/test_nn/test_activations.py
index 8c47ca2f40447..83a1d3985c6ff 100644
--- a/ivy_tests/test_ivy/test_functional/test_nn/test_activations.py
+++ b/ivy_tests/test_ivy/test_functional/test_nn/test_activations.py
@@ -208,8 +208,6 @@ def test_sigmoid(
test_flags=test_flags,
fn_name=fn_name,
on_device=on_device,
- rtol_=1e-2,
- atol_=1e-2,
x=x[0],
complex_mode=complex_mode,
)
diff --git a/ivy_tests/test_ivy/test_functional/test_nn/test_layers.py b/ivy_tests/test_ivy/test_functional/test_nn/test_layers.py
index c844f9b5583fa..988d6e7ac78fa 100644
--- a/ivy_tests/test_ivy/test_functional/test_nn/test_layers.py
+++ b/ivy_tests/test_ivy/test_functional/test_nn/test_layers.py
@@ -307,7 +307,7 @@ def _mha_helper(draw, same_pre_embed_dim=False, batch_second=False):
average_attention_weights = draw(st.booleans())
if len(q.shape) == 3 and not batch_first:
- q, k, v = (np.swapaxes(x, 0, 1) if x is not None else x for x in [q, k, v])
+ q, k, v = [np.swapaxes(x, 0, 1) if x is not None else x for x in [q, k, v]]
ret = (
q,
@@ -516,7 +516,7 @@ def _x_and_filters(
else:
filter_shape = filter_shape + (input_channels,)
channel_first = True
- if data_format == "NHWC" or data_format == "NWC" or data_format == "NDHWC":
+ if data_format in ["NHWC", "NWC", "NDHWC"]:
x_shape = (batch_size,) + x_dim + (input_channels,)
channel_first = False
else:
@@ -1460,6 +1460,7 @@ def test_multi_head_attention(
inputs=_nms_helper(),
test_instance_method=st.just(False),
test_with_out=st.just(False),
+ test_gradients=st.just(False),
)
def test_nms(
*,
diff --git a/ivy_tests/test_ivy/test_misc/test_assertions.py b/ivy_tests/test_ivy/test_misc/test_assertions.py
index b6e4c948c1dea..986190041034d 100644
--- a/ivy_tests/test_ivy/test_misc/test_assertions.py
+++ b/ivy_tests/test_ivy/test_misc/test_assertions.py
@@ -23,7 +23,7 @@
check_shape,
check_shapes_broadcastable,
check_true,
- check_unsorted_segment_min_valid_params,
+ check_unsorted_segment_valid_params,
)
from ivy.utils.assertions import _check_jax_x64_flag
import ivy
@@ -64,7 +64,7 @@ def test_check_all(results):
@pytest.mark.parametrize(
- "args, fn, type, limit",
+ ("args", "fn", "type", "limit"),
[
# INVALID CASES
((1, 2, 0), ivy.array, "all", [3]),
@@ -198,7 +198,7 @@ def test_check_dimensions(x):
@pytest.mark.parametrize(
- "elem, list, inverse",
+ ("elem", "list", "inverse"),
[
(1, [1, 2], False),
("a", [1, 2], False),
@@ -240,7 +240,7 @@ def test_check_elem_in_list(elem, list, inverse):
@pytest.mark.parametrize(
- "x1, x2, inverse",
+ ("x1", "x2", "inverse"),
[
(5, 10, False),
(10, 10, False),
@@ -283,7 +283,7 @@ def test_check_equal(x1, x2, inverse):
@pytest.mark.parametrize(
- "x, inverse",
+ ("x", "inverse"),
[(None, False), ([], False), (None, True), ("abc", True)],
)
def test_check_exists(x, inverse):
@@ -356,7 +356,7 @@ def test_check_false(expression):
@pytest.mark.parametrize(
- "params, indices, axis, batch_dims",
+ ("params", "indices", "axis", "batch_dims"),
[
# INVALID CASES
(ivy.array([1, 2, 3]), ivy.array([1]), 2, 3),
@@ -402,7 +402,7 @@ def test_check_gather_input_valid(params, indices, axis, batch_dims):
@pytest.mark.parametrize(
- "params, indices, batch_dims",
+ ("params", "indices", "batch_dims"),
[
# INVALID CASES
(ivy.array([1, 2, 3]), ivy.array([1]), 2),
@@ -450,7 +450,7 @@ def test_check_gather_nd_input_valid(params, indices, batch_dims):
@pytest.mark.parametrize(
- "x1, x2, allow_equal",
+ ("x1", "x2", "allow_equal"),
[
(5, 10, False),
(10, 5, False),
@@ -488,7 +488,7 @@ def test_check_greater(x1, x2, allow_equal):
@pytest.mark.parametrize(
- "var, data",
+ ("var", "data"),
[
# INVALID CASES
(ivy.array([1]), ivy.array([1, 2])),
@@ -528,7 +528,7 @@ def test_check_inplace_sizes_valid(var, data):
@pytest.mark.parametrize(
- "x, allowed_types",
+ ("x", "allowed_types"),
[(5.0, float), (ivy.array(5), type(ivy.array(8))), (5, float), ([5, 10], tuple)],
)
def test_check_isinstance(x, allowed_types):
@@ -602,7 +602,7 @@ def test_check_jax_x64_flag(dtype):
@pytest.mark.parametrize(
- "kernel_size, padding_size",
+ ("kernel_size", "padding_size"),
[
# INVALID CASES
(((2, 2), ((2, 2), (1, 1)))),
@@ -642,7 +642,7 @@ def test_check_kernel_padding_size(kernel_size, padding_size):
@pytest.mark.parametrize(
- "x1, x2, allow_equal",
+ ("x1", "x2", "allow_equal"),
[
(5, 10, False),
(10, 5, False),
@@ -680,7 +680,7 @@ def test_check_less(x1, x2, allow_equal):
@pytest.mark.parametrize(
- "x1, x2",
+ ("x1", "x2"),
[
(ivy.array([1, 2, 3]), ivy.array([4, 5, 6])),
(ivy.array([1.0, 2.0, 3.0]), ivy.array([4, 5, 6])),
@@ -718,7 +718,7 @@ def test_check_same_dtype(x1, x2):
@pytest.mark.parametrize(
- "x1, x2",
+ ("x1", "x2"),
[
(ivy.array([1, 2, 3]), ivy.array([[4, 5, 6], [2, 3, 1]])),
(ivy.array([[1.0, 2.0], [3.0, 4.0]]), ivy.array([4, 5, 6])),
@@ -757,7 +757,7 @@ def test_check_shape(x1, x2):
@pytest.mark.parametrize(
- "var, data",
+ ("var", "data"),
[
# INVALID CASES
((2, 1), (1, 2, 1)),
@@ -832,7 +832,7 @@ def test_check_true(expression):
@pytest.mark.parametrize(
- "data, segment_ids, num_segments",
+ ("data", "segment_ids", "num_segments"),
[
# INVALID CASES
(ivy.array([1, 2, 3]), ivy.array([0, 1, 0], dtype=ivy.int32), 2.0),
@@ -852,7 +852,7 @@ def test_check_true(expression):
(ivy.array([1, 2, 3]), ivy.array([0, 1, 0], dtype=ivy.int32), ivy.array([2])),
],
)
-def test_check_unsorted_segment_min_valid_params(data, segment_ids, num_segments):
+def test_check_unsorted_segment_valid_params(data, segment_ids, num_segments):
filename = "except_out.txt"
orig_stdout = sys.stdout
@@ -860,7 +860,7 @@ def test_check_unsorted_segment_min_valid_params(data, segment_ids, num_segments
sys.stdout = f
lines = ""
try:
- check_unsorted_segment_min_valid_params(data, segment_ids, num_segments)
+ check_unsorted_segment_valid_params(data, segment_ids, num_segments)
local_vars = {**locals()}
except Exception as e:
local_vars = {**locals()}
diff --git a/ivy_tests/test_ivy/test_misc/test_backend_utils/test_backend_handler.py b/ivy_tests/test_ivy/test_misc/test_backend_utils/test_backend_handler.py
index a839509b8284a..f44835ada15d6 100644
--- a/ivy_tests/test_ivy/test_misc/test_backend_utils/test_backend_handler.py
+++ b/ivy_tests/test_ivy/test_misc/test_backend_utils/test_backend_handler.py
@@ -88,32 +88,33 @@ def test_current_backend(backend, array_type):
@pytest.mark.parametrize(
- "middle_backend,end_backend", [(a, b) for a in backends for b in backends if a != b]
+ ["middle_backend", "end_backend"],
+ [(a, b) for a in backends for b in backends if (a != b and "mxnet" not in [a, b])],
)
def test_dynamic_backend_all_combos(middle_backend, end_backend):
# create an ivy array, container and native container
a = ivy.array([1, 2, 3])
b = ivy.array([4, 5, 6])
ivy_cont = ivy.Container({"w": a, "b": b})
- nativ_cont = ivy.Container(
- {"w": tf.Variable([1, 2, 3]), "b": tf.Variable([4, 5, 6])}
- )
# clear the backend stack after initialization of inputs
ivy.unset_backend()
# set dynamic_backend to false for all objects
ivy_cont.dynamic_backend = False
- nativ_cont.dynamic_backend = False
a.dynamic_backend = False
b.dynamic_backend = False
# set the middle backend
ivy.set_backend(middle_backend, dynamic=True)
-
+ var_cont = ivy.Container(
+ {
+ "w": ivy.gradients._variable(ivy.array([10, 20, 30])),
+ "b": ivy.gradients._variable(ivy.array([40, 50, 60])),
+ }
+ )
# set dynamic_backend to true for all objects
ivy_cont.dynamic_backend = True
- nativ_cont.dynamic_backend = True
a.dynamic_backend = True
b.dynamic_backend = True
@@ -123,20 +124,14 @@ def test_dynamic_backend_all_combos(middle_backend, end_backend):
# add the necessary asserts to check if the data
# of the objects are in the correct format
- assert isinstance(a.data, ivy.current_backend().NativeArray)
- assert isinstance(ivy_cont["b"].data, ivy.current_backend().NativeArray)
+ assert isinstance(a.data, ivy.NativeArray)
+ assert isinstance(ivy_cont["b"].data, ivy.NativeArray)
- if end_backend == "numpy":
- assert isinstance(nativ_cont["b"].data, np.ndarray)
- elif end_backend == "jax":
- assert isinstance(nativ_cont["b"].data, jax.Array)
-
- if middle_backend not in ("jax", "numpy") and end_backend not in ("jax", "numpy"):
+ if set(["numpy", "jax"]).intersection([middle_backend, end_backend]):
# these frameworks don't support native variables
- assert ivy.current_backend().gradients.is_variable(nativ_cont["b"].data)
-
+ assert isinstance(var_cont["b"].data, ivy.NativeArray)
else:
- assert isinstance(nativ_cont["b"].data, ivy.current_backend().NativeArray)
+ assert ivy.gradients._is_variable(var_cont["b"])
def test_dynamic_backend_context_manager():
diff --git a/ivy_tests/test_ivy/test_misc/test_container.py b/ivy_tests/test_ivy/test_misc/test_container.py
index 9c90d2ac39b67..ebdea48aa8e94 100644
--- a/ivy_tests/test_ivy/test_misc/test_container.py
+++ b/ivy_tests/test_ivy/test_misc/test_container.py
@@ -1116,9 +1116,9 @@ def worker_fn(in_queue, out_queue, load_size, worker_id):
}
)
- workers = list()
- in_queues = list()
- out_queues = list()
+ workers = []
+ in_queues = []
+ out_queues = []
queue_load_sizes = [1, 2, 1]
for i, queue_load_size in enumerate(queue_load_sizes):
input_queue = multiprocessing.Queue()
@@ -3221,10 +3221,10 @@ def test_container_try_kc(on_device):
def test_container_unify(on_device):
# on_devices and containers
- on_devices = list()
+ on_devices = []
dev0 = on_device
on_devices.append(dev0)
- conts = dict()
+ conts = {}
conts[dev0] = Container(
{
"a": ivy.array([1], device=dev0),
diff --git a/ivy_tests/test_ivy/test_misc/test_exceptions.py b/ivy_tests/test_ivy/test_misc/test_exceptions.py
index d08a3c3fffa81..68520ed026efb 100644
--- a/ivy_tests/test_ivy/test_misc/test_exceptions.py
+++ b/ivy_tests/test_ivy/test_misc/test_exceptions.py
@@ -30,20 +30,18 @@ def test_trace_modes(backend_fw, trace_mode, show_func_wrapper):
ivy.set_backend(backend_fw)
filename = "excep_out.txt"
orig_stdout = sys.stdout
- f = open(filename, "w")
- sys.stdout = f
- ivy.set_exception_trace_mode(trace_mode)
- ivy.set_show_func_wrapper_trace_mode(show_func_wrapper)
- x = ivy.array([])
- y = ivy.array([1.0, 3.0, 4.0])
- lines = ""
- try:
- ivy.divide(x, y)
- except Exception as e:
- print(e)
- sys.stdout = orig_stdout
- f.close()
-
+ with open(filename, "w") as f:
+ sys.stdout = f
+ ivy.set_exception_trace_mode(trace_mode)
+ ivy.set_show_func_wrapper_trace_mode(show_func_wrapper)
+ x = ivy.array([])
+ y = ivy.array([1.0, 3.0, 4.0])
+ lines = ""
+ try:
+ ivy.divide(x, y)
+ except Exception as e:
+ print(e)
+ sys.stdout = orig_stdout
with open(filename) as f:
lines += f.read()
@@ -59,16 +57,16 @@ def test_trace_modes(backend_fw, trace_mode, show_func_wrapper):
if backend_fw.current_backend_str() not in ["torch", "numpy"]:
assert "/dist-packages" in lines
- if (trace_mode == "ivy" or trace_mode == "frontend") and not show_func_wrapper:
- assert "/func_wrapper.py" not in lines
- assert "/dist-packages" not in lines
-
- if (trace_mode == "ivy" or trace_mode == "frontend") and show_func_wrapper:
- if trace_mode == "ivy":
- assert "/func_wrapper.py" in lines
+ if trace_mode in ["ivy", "frontend"]:
+ if not show_func_wrapper:
+ assert "/func_wrapper.py" not in lines
assert "/dist-packages" not in lines
- if trace_mode == "frontend":
- assert "/ivy/functional/backends" not in lines
+
+ if show_func_wrapper:
+ if trace_mode == "frontend":
+ assert "/ivy/functional/backends" not in lines
+ else:
+ assert "/func_wrapper.py" in lines
assert "/dist-packages" not in lines
with contextlib.suppress(FileNotFoundError):
diff --git a/ivy_tests/test_ivy/test_misc/test_factorized_tensor/test_cp_tensor.py b/ivy_tests/test_ivy/test_misc/test_factorized_tensor/test_cp_tensor.py
index 97b70949ae5a0..526ce9761b152 100644
--- a/ivy_tests/test_ivy/test_misc/test_factorized_tensor/test_cp_tensor.py
+++ b/ivy_tests/test_ivy/test_misc/test_factorized_tensor/test_cp_tensor.py
@@ -5,7 +5,7 @@
@pytest.mark.parametrize(
- "shape, rank",
+ ("shape", "rank"),
[
(
(3, 4, 5),
@@ -28,7 +28,7 @@ def test_cp_flip_sign(shape, rank):
@pytest.mark.parametrize(
- "shape, rank",
+ ("shape", "rank"),
[
(
(8, 5, 6, 4),
@@ -64,7 +64,7 @@ def test_cp_lstsq_grad(shape, rank):
@pytest.mark.parametrize(
- "shape, rank",
+ ("shape", "rank"),
[
(
(5, 4, 6),
@@ -78,7 +78,7 @@ def test_cp_mode_dot(shape, rank):
# matrix for mode 1
matrix = ivy.random_uniform(shape=(7, shape[1]))
# vec for mode 2
- vec = ivy.random_uniform(shape=(shape[2]))
+ vec = ivy.random_uniform(shape=shape[2])
# Test cp_mode_dot with matrix
res = ivy.CPTensor.cp_mode_dot(cp_ten, matrix, mode=1, copy=True)
@@ -101,7 +101,7 @@ def test_cp_mode_dot(shape, rank):
@pytest.mark.parametrize(
- "shape, rank, tol",
+ ("shape", "rank", "tol"),
[
(
(8, 5, 6, 4),
@@ -123,7 +123,7 @@ def test_cp_norm(shape, rank, tol):
@pytest.mark.parametrize(
- "shape, rank",
+ ("shape", "rank"),
[
(
(3, 4, 5),
@@ -145,7 +145,7 @@ def test_cp_normalize(shape, rank):
@pytest.mark.parametrize(
- "shapeU1, shapeU2, shapeU3, shapeU4, true_res, columns, rows",
+ ("shapeU1", "shapeU2", "shapeU3", "shapeU4", "true_res", "columns", "rows"),
[
(
(3, 3),
@@ -201,7 +201,7 @@ def test_cp_to_tensor(shapeU1, shapeU2, shapeU3, shapeU4, true_res, columns, row
matrices.insert(i, U_i)
-@pytest.mark.parametrize("shape, expected", [((2, 2), [[-2, -2], [6, 10]])])
+@pytest.mark.parametrize(("shape", "expected"), [((2, 2), [[-2, -2], [6, 10]])])
def test_cp_to_tensor_with_weights(shape, expected):
A = ivy.reshape(ivy.arange(1, 5, dtype=float), shape)
B = ivy.reshape(ivy.arange(5, 9, dtype=float), shape)
@@ -222,7 +222,7 @@ def test_cp_to_tensor_with_weights(shape, expected):
@pytest.mark.parametrize(
- "shapeU1, shapeU2, shapeU3, shapeU4", [((3, 3), (4, 3), (2, 3), (2, 3))]
+ ("shapeU1", "shapeU2", "shapeU3", "shapeU4"), [((3, 3), (4, 3), (2, 3), (2, 3))]
)
def test_cp_to_unfolded(shapeU1, shapeU2, shapeU3, shapeU4):
U1 = ivy.reshape(ivy.arange(1, 10, dtype=float), shapeU1)
@@ -243,7 +243,7 @@ def test_cp_to_unfolded(shapeU1, shapeU2, shapeU3, shapeU4):
@pytest.mark.parametrize(
- "shapeU1, shapeU2, shapeU3, shapeU4", [((3, 3), (4, 3), (2, 3), (2, 3))]
+ ("shapeU1", "shapeU2", "shapeU3", "shapeU4"), [((3, 3), (4, 3), (2, 3), (2, 3))]
)
def test_cp_to_vec(shapeU1, shapeU2, shapeU3, shapeU4):
"""Test for cp_to_vec."""
@@ -267,7 +267,7 @@ def test_cp_to_vec(shapeU1, shapeU2, shapeU3, shapeU4):
@pytest.mark.parametrize(
- "shape, rank",
+ ("shape", "rank"),
[
(
(10, 10, 10, 4),
@@ -280,7 +280,7 @@ def test_unfolding_dot_khatri_rao(shape, rank):
weights, factors = ivy.random_cp(shape, rank, full=False, normalise_factors=True)
for mode in range(4):
- # Version forming explicitely the khatri-rao product
+ # Version forming explicitly the khatri-rao product
unfolded = ivy.unfold(tensor, mode)
kr_factors = ivy.khatri_rao(factors, weights=weights, skip_matrix=mode)
true_res = ivy.matmul(unfolded, kr_factors)
@@ -307,7 +307,7 @@ def test_validate_cp_rank(size):
@pytest.mark.parametrize(
- "true_shape, true_rank",
+ ("true_shape", "true_rank"),
[
(
(3, 4, 5),
diff --git a/ivy_tests/test_ivy/test_misc/test_factorized_tensor/test_parafac2_tensor.py b/ivy_tests/test_ivy/test_misc/test_factorized_tensor/test_parafac2_tensor.py
index 11769f5719785..8992e92efa2f6 100644
--- a/ivy_tests/test_ivy/test_misc/test_factorized_tensor/test_parafac2_tensor.py
+++ b/ivy_tests/test_ivy/test_misc/test_factorized_tensor/test_parafac2_tensor.py
@@ -5,7 +5,7 @@
@pytest.mark.parametrize(
- "weights, factors, projections, true_res",
+ ("weights", "factors", "projections", "true_res"),
[
(
(2, 3),
@@ -29,7 +29,7 @@ def test_apply_parafac2_projections(weights, factors, projections, true_res):
@pytest.mark.parametrize(
- "shape, rank",
+ ("shape", "rank"),
[
(
[(4, 5)] * 3,
@@ -54,7 +54,7 @@ def test_parafac2_normalise(shape, rank):
@pytest.mark.parametrize(
- "weights, factors, projections, true_res",
+ ("weights", "factors", "projections", "true_res"),
[
(
(2, 3),
@@ -82,7 +82,7 @@ def test_parafac2_to_slices(weights, factors, projections, true_res):
@pytest.mark.parametrize(
- "weights, factors, projections, true_res",
+ ("weights", "factors", "projections", "true_res"),
[
(
(2, 3),
@@ -98,12 +98,11 @@ def test_parafac2_to_tensor(weights, factors, projections, true_res):
projections = [ivy.array(p) for p in projections]
true_res = ivy.array(true_res)
res = ivy.Parafac2Tensor.parafac2_to_tensor((weights, factors, projections))
- (true_res, res)
assert np.allclose(res, true_res)
@pytest.mark.parametrize(
- "shape, rank",
+ ("shape", "rank"),
[
(
[(4, 5)] * 3,
@@ -122,7 +121,7 @@ def test_parafac2_to_unfolded(shape, rank):
@pytest.mark.parametrize(
- "shape, rank",
+ ("shape", "rank"),
[
(
[(4, 5)] * 3,
@@ -140,7 +139,7 @@ def test_parafac2_to_vec(shape, rank):
@pytest.mark.parametrize(
- "true_shape, true_rank",
+ ("true_shape", "true_rank"),
[
(
[(4, 5)] * 3,
diff --git a/ivy_tests/test_ivy/test_misc/test_factorized_tensor/test_tr_tensor.py b/ivy_tests/test_ivy/test_misc/test_factorized_tensor/test_tr_tensor.py
index e48dca6ccb866..d7923f83e6cf6 100644
--- a/ivy_tests/test_ivy/test_misc/test_factorized_tensor/test_tr_tensor.py
+++ b/ivy_tests/test_ivy/test_misc/test_factorized_tensor/test_tr_tensor.py
@@ -5,7 +5,7 @@
@pytest.mark.parametrize(
- "shape1, shape2, shape3",
+ ("shape1", "shape2", "shape3"),
[
(
(2, 4, 3),
@@ -30,7 +30,7 @@ def test_tr_to_tensor(shape1, shape2, shape3):
@pytest.mark.parametrize(
- "rank1, rank2",
+ ("rank1", "rank2"),
[((2, 3, 4, 2), (2, 3, 4, 2, 3))],
)
def test_validate_tr_rank(rank1, rank2):
@@ -60,7 +60,7 @@ def test_validate_tr_rank(rank1, rank2):
@pytest.mark.parametrize(
- "true_shape, true_rank",
+ ("true_shape", "true_rank"),
[
(
(6, 4, 5),
diff --git a/ivy_tests/test_ivy/test_misc/test_factorized_tensor/test_tt_tensor.py b/ivy_tests/test_ivy/test_misc/test_factorized_tensor/test_tt_tensor.py
index 3aacfa4485500..273e697b927de 100644
--- a/ivy_tests/test_ivy/test_misc/test_factorized_tensor/test_tt_tensor.py
+++ b/ivy_tests/test_ivy/test_misc/test_factorized_tensor/test_tt_tensor.py
@@ -42,7 +42,7 @@ def test_pad_tt_rank(n_pad):
@pytest.mark.parametrize(
- "shape, rank",
+ ("shape", "rank"),
[((4, 5, 4, 8, 5), (1, 3, 2, 2, 4, 1))],
)
def test_tt_n_param(shape, rank):
@@ -53,7 +53,7 @@ def test_tt_n_param(shape, rank):
@pytest.mark.parametrize(
- "n1, n2, n3, shape1, shape2, shape3",
+ ("n1", "n2", "n3", "shape1", "shape2", "shape3"),
[(3, 4, 2, (1, 3, 2), (2, 4, 2), (2, 2, 1))],
)
def test_tt_to_tensor(n1, n2, n3, shape1, shape2, shape3):
@@ -109,7 +109,7 @@ def test_validate_tt_rank(coef):
@pytest.mark.parametrize(
- "true_shape, true_rank",
+ ("true_shape", "true_rank"),
[
(
(3, 4, 5),
diff --git a/ivy_tests/test_ivy/test_misc/test_factorized_tensor/test_tucker_tensor.py b/ivy_tests/test_ivy/test_misc/test_factorized_tensor/test_tucker_tensor.py
index 3601d740e4b51..bb03df0116781 100644
--- a/ivy_tests/test_ivy/test_misc/test_factorized_tensor/test_tucker_tensor.py
+++ b/ivy_tests/test_ivy/test_misc/test_factorized_tensor/test_tucker_tensor.py
@@ -4,7 +4,7 @@
import pytest
-@pytest.mark.parametrize("shape, rank", [((5, 4, 6), (3, 2, 3))])
+@pytest.mark.parametrize(("shape", "rank"), [((5, 4, 6), (3, 2, 3))])
def test_n_param_tucker(shape, rank):
tucker_tensor = ivy.random_tucker(shape, rank)
true_n_param = ivy.prod(ivy.shape(tucker_tensor[0])) + ivy.sum(
@@ -14,7 +14,7 @@ def test_n_param_tucker(shape, rank):
assert np.allclose(n_param, true_n_param)
-@pytest.mark.parametrize("shape, rank", [((3, 4, 5), 4)])
+@pytest.mark.parametrize(("shape", "rank"), [((3, 4, 5), 4)])
def test_tucker_copy(shape, rank):
tucker_tensor = ivy.random_tucker(shape, rank)
core, factors = tucker_tensor
@@ -28,14 +28,14 @@ def test_tucker_copy(shape, rank):
)
-@pytest.mark.parametrize("shape, ranks", [((5, 4, 6), (3, 2, 3))])
+@pytest.mark.parametrize(("shape", "ranks"), [((5, 4, 6), (3, 2, 3))])
def test_tucker_mode_dot(shape, ranks):
tucker_ten = ivy.random_tucker(shape, ranks, full=False)
full_tensor = ivy.TuckerTensor.tucker_to_tensor(tucker_ten)
# matrix for mode 1
matrix = ivy.random_uniform(shape=(7, shape[1]))
# vec for mode 2
- vec = ivy.random_uniform(shape=(shape[2]))
+ vec = ivy.random_uniform(shape=shape[2])
# Test tucker_mode_dot with matrix
res = ivy.TuckerTensor.tucker_mode_dot(tucker_ten, matrix, mode=1, copy=True)
@@ -57,7 +57,7 @@ def test_tucker_mode_dot(shape, ranks):
assert np.allclose(true_res, res)
-@pytest.mark.parametrize("shape, rank", [((3, 4, 5), (3, 2, 4))])
+@pytest.mark.parametrize(("shape", "rank"), [((3, 4, 5), (3, 2, 4))])
def test_tucker_normalize(shape, rank):
tucker_ten = ivy.random_tucker(shape, rank)
core, factors = ivy.TuckerTensor.tucker_normalize(tucker_ten)
@@ -71,7 +71,7 @@ def test_tucker_normalize(shape, rank):
@pytest.mark.parametrize(
- "X, ranks, true_res",
+ ("X", "ranks", "true_res"),
[
(
[
@@ -107,7 +107,7 @@ def test_tucker_to_tensor(X, ranks, true_res):
assert np.allclose(true_res, res)
-@pytest.mark.parametrize("shape, ranks", [((4, 3, 5, 2), (2, 2, 3, 4))])
+@pytest.mark.parametrize(("shape", "ranks"), [((4, 3, 5, 2), (2, 2, 3, 4))])
def test_tucker_to_unfolded(shape, ranks):
G = ivy.random_uniform(shape=shape)
U = [ivy.random_uniform(shape=(ranks[i], G.shape[i])) for i in range(4)]
@@ -126,7 +126,7 @@ def test_tucker_to_unfolded(shape, ranks):
)
-@pytest.mark.parametrize("shape, ranks", [((4, 3, 5, 2), (2, 2, 3, 4))])
+@pytest.mark.parametrize(("shape", "ranks"), [((4, 3, 5, 2), (2, 2, 3, 4))])
def test_tucker_to_vec(shape, ranks):
G = ivy.random_uniform(shape=shape)
ranks = [2, 2, 3, 4]
@@ -191,7 +191,7 @@ def test_validate_tucker_rank(tol):
# These tests have been adapted from TensorLy
# https://github.com/tensorly/tensorly/blob/main/tensorly/tests/test_tucker_tensor.py
-@pytest.mark.parametrize("true_shape, true_rank", [((3, 4, 5), (3, 2, 4))])
+@pytest.mark.parametrize(("true_shape", "true_rank"), [((3, 4, 5), (3, 2, 4))])
def test_validate_tucker_tensor(true_shape, true_rank):
core, factors = ivy.random_tucker(true_shape, true_rank)
diff --git a/ivy_tests/test_ivy/test_misc/test_handle_exceptions.py b/ivy_tests/test_ivy/test_misc/test_handle_exceptions.py
index 79cfe53b1a547..2c379e20e8b51 100644
--- a/ivy_tests/test_ivy/test_misc/test_handle_exceptions.py
+++ b/ivy_tests/test_ivy/test_misc/test_handle_exceptions.py
@@ -30,7 +30,7 @@ def func(e):
@pytest.mark.parametrize(
"e",
- (
+ [
IvyError,
IvyNotImplementedException,
IvyBroadcastShapeError,
@@ -43,7 +43,7 @@ def func(e):
IvyDeviceError,
IvyInvalidBackendException,
IvyDtypePromotionError,
- ),
+ ],
)
def test_ivy_errors_raising(e):
with pytest.raises(e):
@@ -55,7 +55,7 @@ def test_no_exception():
@pytest.mark.parametrize(
- "e, to_be_raised",
+ ("e", "to_be_raised"),
_non_ivy_exceptions_mapping.items(),
)
def test_non_ivy_errors_mapping(e, to_be_raised):
diff --git a/ivy_tests/test_ivy/test_misc/test_shape.py b/ivy_tests/test_ivy/test_misc/test_shape.py
index a9687daedb24a..f20c34bb3a386 100644
--- a/ivy_tests/test_ivy/test_misc/test_shape.py
+++ b/ivy_tests/test_ivy/test_misc/test_shape.py
@@ -448,6 +448,7 @@ def test_shape__mul__(
helpers.test_method(
on_device=on_device,
ground_truth_backend=ground_truth_backend,
+ backend_to_test=backend_fw,
init_flags=init_flags,
method_flags=method_flags,
init_all_as_kwargs_np={"data": x[0]},
@@ -497,7 +498,7 @@ def test_shape__radd__(
@handle_method(
- method_tree="Shape.__rdivmod__",
+ method_tree="Shape.__rdiv__",
dtype_and_x=helpers.dtype_and_values(
available_dtypes=helpers.get_dtypes("numeric"),
num_arrays=2,
@@ -507,7 +508,7 @@ def test_shape__radd__(
shared_dtype=True,
),
)
-def test_shape__rdivmod__(
+def test_shape__rdiv__(
dtype_and_x,
method_name,
class_name,
@@ -588,6 +589,7 @@ def test_shape__rmul__(
method_name,
class_name,
ground_truth_backend,
+ backend_fw,
init_flags,
method_flags,
on_device,
@@ -596,6 +598,7 @@ def test_shape__rmul__(
helpers.test_method(
on_device=on_device,
ground_truth_backend=ground_truth_backend,
+ backend_to_test=backend_fw,
init_flags=init_flags,
method_flags=method_flags,
init_all_as_kwargs_np={"data": x[0]},
@@ -644,43 +647,6 @@ def test_shape__rsub__(
)
-@handle_method(
- method_tree="Shape.__rtruediv__",
- dtype_and_x=helpers.dtype_and_values(
- available_dtypes=helpers.get_dtypes("numeric"),
- num_arrays=2,
- large_abs_safety_factor=2.5,
- small_abs_safety_factor=2.5,
- safety_factor_scale="log",
- shared_dtype=True,
- ),
-)
-def test_shape__rtruediv__(
- dtype_and_x,
- method_name,
- class_name,
- ground_truth_backend,
- backend_fw,
- init_flags,
- method_flags,
- on_device,
-):
- dtype, x = dtype_and_x
- helpers.test_method(
- on_device=on_device,
- ground_truth_backend=ground_truth_backend,
- backend_to_test=backend_fw,
- init_flags=init_flags,
- method_flags=method_flags,
- init_all_as_kwargs_np={"shape": x[0]},
- init_input_dtypes=dtype,
- method_input_dtypes=dtype,
- method_all_as_kwargs_np={"other": x[1]},
- class_name=class_name,
- method_name=method_name,
- )
-
-
@handle_method(
method_tree="Shape.__sub__",
dtype_and_x=helpers.dtype_and_values(
@@ -716,40 +682,3 @@ def test_shape__sub__(
class_name=class_name,
method_name=method_name,
)
-
-
-@handle_method(
- method_tree="Shape.__truediv__",
- dtype_and_x=helpers.dtype_and_values(
- available_dtypes=helpers.get_dtypes("numeric"),
- num_arrays=2,
- large_abs_safety_factor=2.5,
- small_abs_safety_factor=2.5,
- safety_factor_scale="log",
- shared_dtype=True,
- ),
-)
-def test_shape__truediv__(
- dtype_and_x,
- method_name,
- class_name,
- ground_truth_backend,
- backend_fw,
- init_flags,
- method_flags,
- on_device,
-):
- dtype, x = dtype_and_x
- helpers.test_method(
- on_device=on_device,
- ground_truth_backend=ground_truth_backend,
- backend_to_test=backend_fw,
- init_flags=init_flags,
- method_flags=method_flags,
- init_all_as_kwargs_np={"shape": x[0]},
- init_input_dtypes=dtype,
- method_input_dtypes=dtype,
- method_all_as_kwargs_np={"other": x[1]},
- class_name=class_name,
- method_name=method_name,
- )
diff --git a/ivy_tests/test_ivy/test_stateful/test_activations.py b/ivy_tests/test_ivy/test_stateful/test_activations.py
index 638aaee6fbe80..6a1892b165c6f 100644
--- a/ivy_tests/test_ivy/test_stateful/test_activations.py
+++ b/ivy_tests/test_ivy/test_stateful/test_activations.py
@@ -32,12 +32,14 @@ def test_elu(
class_name,
method_name,
ground_truth_backend,
+ backend_fw,
init_flags,
method_flags,
on_device,
):
input_dtype, x = dtype_and_x
helpers.test_method(
+ backend_to_test=backend_fw,
ground_truth_backend=ground_truth_backend,
init_flags=init_flags,
method_flags=method_flags,
@@ -167,12 +169,14 @@ def test_hardswish(
class_name,
method_name,
ground_truth_backend,
+ backend_fw,
init_flags,
method_flags,
on_device,
):
input_dtype, x = dtype_and_x
helpers.test_method(
+ backend_to_test=backend_fw,
ground_truth_backend=ground_truth_backend,
init_flags=init_flags,
method_flags=method_flags,
@@ -307,12 +311,14 @@ def test_logit(
class_name,
method_name,
ground_truth_backend,
+ backend_fw,
init_flags,
method_flags,
on_device,
):
input_dtype, x = dtype_and_x
helpers.test_method(
+ backend_to_test=backend_fw,
ground_truth_backend=ground_truth_backend,
init_flags=init_flags,
method_flags=method_flags,
@@ -351,12 +357,14 @@ def test_logsigmoid(
class_name,
method_name,
ground_truth_backend,
+ backend_fw,
init_flags,
method_flags,
on_device,
):
input_dtype, x = dtype_and_x
helpers.test_method(
+ backend_to_test=backend_fw,
ground_truth_backend=ground_truth_backend,
init_flags=init_flags,
method_flags=method_flags,
@@ -437,12 +445,14 @@ def test_prelu(
class_name,
method_name,
ground_truth_backend,
+ backend_fw,
init_flags,
method_flags,
on_device,
):
input_dtype, x = dtype_and_x
helpers.test_method(
+ backend_to_test=backend_fw,
ground_truth_backend=ground_truth_backend,
init_flags=init_flags,
method_flags=method_flags,
@@ -521,12 +531,14 @@ def test_relu6(
class_name,
method_name,
ground_truth_backend,
+ backend_fw,
init_flags,
method_flags,
on_device,
):
input_dtype, x = dtype_and_x
helpers.test_method(
+ backend_to_test=backend_fw,
ground_truth_backend=ground_truth_backend,
init_flags=init_flags,
method_flags=method_flags,
@@ -563,12 +575,14 @@ def test_selu(
class_name,
method_name,
ground_truth_backend,
+ backend_fw,
init_flags,
method_flags,
on_device,
):
input_dtype, x = dtype_and_x
helpers.test_method(
+ backend_to_test=backend_fw,
ground_truth_backend=ground_truth_backend,
init_flags=init_flags,
method_flags=method_flags,
@@ -605,12 +619,14 @@ def test_sigmoid(
class_name,
method_name,
ground_truth_backend,
+ backend_fw,
init_flags,
method_flags,
on_device,
):
input_dtype, x = dtype_and_x
helpers.test_method(
+ backend_to_test=backend_fw,
ground_truth_backend=ground_truth_backend,
init_flags=init_flags,
method_flags=method_flags,
@@ -781,12 +797,14 @@ def test_tanh(
class_name,
method_name,
ground_truth_backend,
+ backend_fw,
init_flags,
method_flags,
on_device,
):
input_dtype, x = dtype_and_x
helpers.test_method(
+ backend_to_test=backend_fw,
ground_truth_backend=ground_truth_backend,
init_flags=init_flags,
method_flags=method_flags,
diff --git a/ivy_tests/test_ivy/test_stateful/test_converters.py b/ivy_tests/test_ivy/test_stateful/test_converters.py
index dcc100a29cf45..037ca20c0fd84 100644
--- a/ivy_tests/test_ivy/test_stateful/test_converters.py
+++ b/ivy_tests/test_ivy/test_stateful/test_converters.py
@@ -85,14 +85,15 @@
paddle.optimizer.SGD = SimpleNamespace
paddle.nn.L1Loss = SimpleNamespace
+
FROM_CONVERTERS = {
- "torch": ivy.Module.from_torch_module,
+ "torch": "from_torch_module",
"jax": {
- "haiku": ivy.Module.from_haiku_module,
- "flax": ivy.Module.from_flax_module,
+ "haiku": "from_haiku_module",
+ "flax": "from_flax_module",
},
- "tensorflow": ivy.Module.from_keras_module,
- "paddle": ivy.Module.from_paddle_module,
+ "tensorflow": "from_keras_module",
+ "paddle": "from_paddle_module",
}
@@ -219,134 +220,173 @@ def forward(self, x):
return paddle.nn.functional.tanh(self._linear2(x))[0]
+def get_converter(ivy_backend, converter):
+ return getattr(ivy_backend.Module, converter)
+
+
@pytest.mark.parametrize("bs_ic_oc", [([1, 2], 4, 5)])
@pytest.mark.parametrize("from_class_and_args", [True, False])
-def test_from_backend_module(bs_ic_oc, from_class_and_args):
+def test_from_backend_module(bs_ic_oc, from_class_and_args, backend_fw):
# smoke test
- if ivy.current_backend_str() in ["numpy", "jax"]:
+ if backend_fw in ["numpy", "jax"]:
# Converters not implemented in numpy
pytest.skip()
+
batch_shape, input_channels, output_channels = bs_ic_oc
- x = ivy.astype(
- ivy.linspace(ivy.zeros(batch_shape), ivy.ones(batch_shape), input_channels),
- "float32",
- )
- native_module_class = NATIVE_MODULES[ivy.current_backend_str()]
- module_converter = FROM_CONVERTERS[ivy.current_backend_str()]
-
- if from_class_and_args:
- ivy_module = module_converter(
- native_module_class,
- instance_args=[x],
- constructor_kwargs={"in_size": input_channels, "out_size": output_channels},
+
+ # using ivy_backend.utils.backend.ContextManager instead of update_backend,
+ # because with_backend doesn't work here
+ with ivy.utils.backend.ContextManager(backend_fw) as ivy_backend:
+ x = ivy_backend.astype(
+ ivy_backend.linspace(
+ ivy_backend.zeros(batch_shape),
+ ivy_backend.ones(batch_shape),
+ input_channels,
+ ),
+ "float32",
)
- else:
- if ivy.current_backend_str() == "tensorflow":
- native_module = native_module_class(
- in_size=input_channels, out_size=output_channels
+ native_module_class = NATIVE_MODULES[ivy_backend.current_backend_str()]
+ module_converter = get_converter(
+ ivy_backend, FROM_CONVERTERS[ivy_backend.current_backend_str()]
+ )
+
+ if from_class_and_args:
+ ivy_module = module_converter(
+ native_module_class,
+ instance_args=[x],
+ constructor_kwargs={
+ "in_size": input_channels,
+ "out_size": output_channels,
+ },
)
- native_module.build((input_channels,))
else:
- native_module = native_module_class(
- in_size=input_channels, out_size=output_channels
- )
-
- fw_kwargs = {}
- ivy_module = module_converter(native_module, **fw_kwargs)
-
- def loss_fn(v_=None):
- out = ivy_module(x, v=v_)
- return ivy.mean(out)
-
- # train
- loss_tm1 = 1e12
- loss = None
- grads = None
- loss_fn() # for on-call mode
-
- for i in range(10):
- loss, grads = ivy.execute_with_gradients(loss_fn, ivy_module.v)
- w = ivy.gradient_descent_update(ivy_module.v, grads, 1e-3)
- ivy.inplace_update(ivy_module.v, w)
- assert loss < loss_tm1
- loss_tm1 = loss
-
- # type test
- assert ivy.is_array(loss)
- assert isinstance(grads, ivy.Container)
- # cardinality test
- assert loss.shape == ()
- # value test
- assert (abs(grads).max() > 0).cont_all_true()
+ if ivy_backend.current_backend_str() == "tensorflow":
+ native_module = native_module_class(
+ in_size=input_channels, out_size=output_channels
+ )
+ native_module.build((input_channels,))
+ else:
+ native_module = native_module_class(
+ in_size=input_channels, out_size=output_channels
+ )
+
+ fw_kwargs = {}
+ ivy_module = module_converter(native_module, **fw_kwargs)
+
+ def loss_fn(v_=None):
+ out = ivy_module(x, v=v_)
+ return ivy_backend.mean(out)
+
+ # train
+ loss_tm1 = 1e12
+ loss = None
+ grads = None
+ loss_fn() # for on-call mode
+
+ for i in range(10):
+ loss, grads = ivy_backend.execute_with_gradients(loss_fn, ivy_module.v)
+ w = ivy_backend.gradient_descent_update(ivy_module.v, grads, 1e-3)
+ ivy_backend.inplace_update(ivy_module.v, w)
+ assert loss <= loss_tm1
+ loss_tm1 = loss
+
+ # type test
+ assert ivy_backend.is_array(loss)
+ assert isinstance(grads, ivy_backend.Container)
+ # cardinality test
+ assert loss.shape == ()
+ # value test
+ assert (abs(grads).max() > 0).cont_all_true()
@pytest.mark.parametrize("bs_ic_oc", [([1, 2], 4, 5)])
@pytest.mark.parametrize("from_class_and_args", [True, False])
@pytest.mark.parametrize("module_type", ["haiku", "flax"])
-def test_from_jax_module(bs_ic_oc, from_class_and_args, module_type):
+def test_from_jax_module(bs_ic_oc, from_class_and_args, module_type, backend_fw):
# smoke test
- if ivy.current_backend_str() not in ["jax"]:
+ if backend_fw not in ["jax"]:
# Converters not implemented in numpy
pytest.skip()
+
batch_shape, input_channels, output_channels = bs_ic_oc
- x = ivy.astype(
- ivy.linspace(ivy.zeros(batch_shape), ivy.ones(batch_shape), input_channels),
- "float32",
- )
- native_module_class = NATIVE_MODULES[ivy.current_backend_str()][module_type]
- module_converter = FROM_CONVERTERS[ivy.current_backend_str()][module_type]
-
- if from_class_and_args:
- ivy_module = module_converter(
- native_module_class,
- instance_args=[x],
- constructor_kwargs={"in_size": input_channels, "out_size": output_channels},
- )
- else:
- if module_type == "haiku":
- def forward_fn(*a, **kw):
- model = native_module_class(input_channels, output_channels)
- return model(ivy.to_native(x))
+ # using ivy_backend.utils.backend.ContextManager instead of update_backend,
+ # because with_backend doesn't work here
+ with ivy.utils.backend.ContextManager(backend_fw) as ivy_backend:
+ x = ivy_backend.astype(
+ ivy_backend.linspace(
+ ivy_backend.zeros(batch_shape),
+ ivy_backend.ones(batch_shape),
+ input_channels,
+ ),
+ "float32",
+ )
+ native_module_class = NATIVE_MODULES[ivy_backend.current_backend_str()][
+ module_type
+ ]
+ module_converter = FROM_CONVERTERS[ivy_backend.current_backend_str()][
+ module_type
+ ]
+ module_converter = get_converter(
+ ivy_backend, FROM_CONVERTERS[ivy_backend.current_backend_str()][module_type]
+ )
- native_module = hk.transform(forward_fn)
- else:
- native_module = native_module_class(
- in_size=input_channels, out_size=output_channels
+ if from_class_and_args:
+ ivy_module = module_converter(
+ native_module_class,
+ instance_args=[x],
+ constructor_kwargs={
+ "in_size": input_channels,
+ "out_size": output_channels,
+ },
)
-
- fw_kwargs = {}
- if module_type == "haiku":
- fw_kwargs["params_hk"] = native_module.init(0, x)
else:
- fw_kwargs["params_fx"] = native_module.init(
- jax.random.PRNGKey(0), ivy.to_native(x)
+ if module_type == "haiku":
+
+ def forward_fn(*a, **kw):
+ model = native_module_class(input_channels, output_channels)
+ return model(ivy_backend.to_native(x))
+
+ native_module = hk.transform(forward_fn)
+ else:
+ native_module = native_module_class(
+ in_size=input_channels, out_size=output_channels
+ )
+
+ fw_kwargs = {}
+ if module_type == "haiku":
+ fw_kwargs["params_hk"] = native_module.init(0, x)
+ else:
+ fw_kwargs["params_fx"] = native_module.init(
+ jax.random.PRNGKey(0), ivy_backend.to_native(x)
+ )
+ ivy_module = module_converter(native_module, **fw_kwargs)
+
+ def loss_fn(v_=None):
+ out = ivy_module(x, v=v_)
+ return ivy_backend.mean(out)
+
+ # train
+ loss_tm1 = 1e12
+ loss = None
+ grads = None
+ loss_fn() # for on-call mode
+
+ for i in range(10):
+ loss, grads = ivy_backend.execute_with_gradients(loss_fn, ivy_module.v)
+ ivy_module.v = ivy_backend.gradient_descent_update(
+ ivy_module.v, grads, 1e-3
)
- ivy_module = module_converter(native_module, **fw_kwargs)
-
- def loss_fn(v_=None):
- out = ivy_module(x, v=v_)
- return ivy.mean(out)
-
- # train
- loss_tm1 = 1e12
- loss = None
- grads = None
- loss_fn() # for on-call mode
-
- for i in range(10):
- loss, grads = ivy.execute_with_gradients(loss_fn, ivy_module.v)
- ivy_module.v = ivy.gradient_descent_update(ivy_module.v, grads, 1e-3)
- assert loss < loss_tm1
- loss_tm1 = loss
-
- # type test
- assert ivy.is_array(loss)
- assert isinstance(grads, ivy.Container)
- # cardinality test
- assert loss.shape == ()
- # value test
- assert (abs(grads).max() > 0).cont_all_true()
+ assert loss < loss_tm1
+ loss_tm1 = loss
+
+ # type test
+ assert ivy_backend.is_array(loss)
+ assert isinstance(grads, ivy_backend.Container)
+ # cardinality test
+ assert loss.shape == ()
+ # value test
+ assert (abs(grads).max() > 0).cont_all_true()
NATIVE_MODULES = {
diff --git a/ivy_tests/test_ivy/test_stateful/test_losses.py b/ivy_tests/test_ivy/test_stateful/test_losses.py
index c8efbe04c1295..2849ab86f7989 100644
--- a/ivy_tests/test_ivy/test_stateful/test_losses.py
+++ b/ivy_tests/test_ivy/test_stateful/test_losses.py
@@ -58,6 +58,7 @@ def test_binary_cross_entropy_loss(
dtype_and_true,
dtype_and_pred,
dtype_and_pos,
+ backend_fw,
from_logits,
reduction,
axis,
@@ -75,6 +76,7 @@ def test_binary_cross_entropy_loss(
if from_logits:
helpers.test_method(
+ backend_to_test=backend_fw,
ground_truth_backend=ground_truth_backend,
init_flags=init_flags,
method_flags=method_flags,
@@ -98,6 +100,7 @@ def test_binary_cross_entropy_loss(
)
else:
helpers.test_method(
+ backend_to_test=backend_fw,
ground_truth_backend=ground_truth_backend,
init_flags=init_flags,
method_flags=method_flags,
@@ -158,6 +161,7 @@ def test_cross_entropy_loss(
axis,
reduction,
class_name,
+ backend_fw,
method_name,
ground_truth_backend,
init_flags,
@@ -167,6 +171,7 @@ def test_cross_entropy_loss(
targets_dtype, targets = dtype_and_targets
log_input_dtype, log_input = dtype_and_log_input
helpers.test_method(
+ backend_to_test=backend_fw,
ground_truth_backend=ground_truth_backend,
init_flags=init_flags,
method_flags=method_flags,
@@ -224,6 +229,7 @@ def test_log_poisson_loss(
axis,
reduction,
class_name,
+ backend_fw,
method_name,
ground_truth_backend,
init_flags,
@@ -233,6 +239,7 @@ def test_log_poisson_loss(
targets_dtype, targets = dtype_and_targets
log_input_dtype, log_input = dtype_and_log_input
helpers.test_method(
+ backend_to_test=backend_fw,
ground_truth_backend=ground_truth_backend,
init_flags=init_flags,
method_flags=method_flags,
diff --git a/ivy_tests/test_ivy/test_stateful/test_modules.py b/ivy_tests/test_ivy/test_stateful/test_modules.py
index 14e66a53d3b00..1a361cba5fa42 100644
--- a/ivy_tests/test_ivy/test_stateful/test_modules.py
+++ b/ivy_tests/test_ivy/test_stateful/test_modules.py
@@ -159,15 +159,16 @@ def _forward():
]
)
)
-def test_get_buffers(buffer):
- module = ModuleWithBuffer()
- buffers = {}
- for item in buffer:
- buffers.update(item)
- for key in item:
- module.register_buffer(key, item[key])
+def test_get_buffers(buffer, backend_fw):
+ with ivy.utils.backend.ContextManager(backend_fw):
+ module = ModuleWithBuffer()
+ buffers = {}
+ for item in buffer:
+ buffers.update(item)
+ for key in item:
+ module.register_buffer(key, item[key])
- assert module.buffers == buffers
+ assert module.buffers == buffers
# check submod returns
@@ -179,89 +180,93 @@ def test_get_buffers(buffer):
output_channels=st.integers(min_value=2, max_value=5),
)
def test_module_check_submod_rets(
- batch_shape, input_channels, output_channels, on_device
+ batch_shape, input_channels, output_channels, on_device, backend_fw
):
# smoke test
- if ivy.current_backend_str() == "numpy":
+ if backend_fw == "numpy":
# NumPy does not support gradients
return
- x = ivy.astype(
- ivy.linspace(ivy.zeros(batch_shape), ivy.ones(batch_shape), input_channels),
- "float32",
- )
- module = WithNestedModules(input_channels, output_channels, device=on_device)
-
- # depth 1
- ret = module(x, track_submod_rets=True, submod_depth=1)
- assert ret.shape == tuple(list(batch_shape) + [64])
- sm_rets = module.submod_rets
- module(x, expected_submod_rets=sm_rets)
- sm_rets.random_uniform(map_sequences=True)
- try:
- module(x, expected_submod_rets=sm_rets.random_uniform(map_sequences=True))
- raise Exception(
- "forward pass succeeded despite passing random expected_submod_rets, "
- "assertion error expected."
+ with ivy.utils.backend.ContextManager(backend_fw):
+ x = ivy.astype(
+ ivy.linspace(ivy.zeros(batch_shape), ivy.ones(batch_shape), input_channels),
+ "float32",
)
- except ivy.utils.exceptions.IvyException:
- pass
+ module = WithNestedModules(input_channels, output_channels, device=on_device)
- # depth 2 (full)
- ret = module(x, track_submod_rets=True)
- assert ret.shape == tuple(list(batch_shape) + [64])
- sm_rets = module.submod_rets
- module(x, expected_submod_rets=sm_rets)
- try:
- module(x, expected_submod_rets=sm_rets.random_uniform(map_sequences=True))
- raise Exception(
- "forward pass succeeded despite passing random expected_submod_rets, "
- "assertion error expected."
+ # depth 1
+ ret = module(x, track_submod_rets=True, submod_depth=1)
+ assert ret.shape == tuple(list(batch_shape) + [64])
+ sm_rets = module.submod_rets
+ module(x, expected_submod_rets=sm_rets)
+ sm_rets.random_uniform(map_sequences=True)
+ try:
+ module(x, expected_submod_rets=sm_rets.random_uniform(map_sequences=True))
+ raise Exception(
+ "forward pass succeeded despite passing random expected_submod_rets, "
+ "assertion error expected."
+ )
+ except ivy.utils.exceptions.IvyException:
+ pass
+
+ # depth 2 (full)
+ ret = module(x, track_submod_rets=True)
+ assert ret.shape == tuple(list(batch_shape) + [64])
+ sm_rets = module.submod_rets
+ module(x, expected_submod_rets=sm_rets)
+ try:
+ module(x, expected_submod_rets=sm_rets.random_uniform(map_sequences=True))
+ raise Exception(
+ "forward pass succeeded despite passing random expected_submod_rets, "
+ "assertion error expected."
+ )
+ except ivy.utils.exceptions.IvyException:
+ pass
+
+ # partial submodules
+ ret = module(
+ x, track_submod_rets=True, submods_to_track=[module._dl1, module._dl0._l0]
)
- except ivy.utils.exceptions.IvyException:
- pass
-
- # partial submodules
- ret = module(
- x, track_submod_rets=True, submods_to_track=[module._dl1, module._dl0._l0]
- )
- assert ret.shape == tuple(list(batch_shape) + [64])
- sm_rets = module.submod_rets
- module(x, expected_submod_rets=sm_rets)
- try:
- module(x, expected_submod_rets=sm_rets.random_uniform(map_sequences=True))
- raise Exception(
- "forward pass succeeded despite passing random expected_submod_rets, "
- "assertion error expected."
+ assert ret.shape == tuple(list(batch_shape) + [64])
+ sm_rets = module.submod_rets
+ module(x, expected_submod_rets=sm_rets)
+ try:
+ module(x, expected_submod_rets=sm_rets.random_uniform(map_sequences=True))
+ raise Exception(
+ "forward pass succeeded despite passing random expected_submod_rets, "
+ "assertion error expected."
+ )
+ except ivy.utils.exceptions.IvyException:
+ pass
+
+ # with tolerances
+ ret = module(x, track_submod_rets=True)
+ assert ret.shape == tuple(list(batch_shape) + [64])
+ sm_rets_orig = module.submod_rets
+ sm_rets = ivy.Container(
+ {
+ k: {"val": v, "atol": [1e-8] * len(v), "rtol": [1e-5] * len(v)}
+ for k, v in sm_rets_orig.items()
+ },
+ **sm_rets_orig._config,
)
- except ivy.utils.exceptions.IvyException:
- pass
-
- # with tolerances
- ret = module(x, track_submod_rets=True)
- assert ret.shape == tuple(list(batch_shape) + [64])
- sm_rets_orig = module.submod_rets
- sm_rets = ivy.Container(
- {
- k: {"val": v, "atol": [1e-8] * len(v), "rtol": [1e-5] * len(v)}
- for k, v in sm_rets_orig.items()
- },
- **sm_rets_orig._config,
- )
- module(x, expected_submod_rets=sm_rets)
- sm_rets = ivy.Container(
- {k: {"val": v, "atol": 1e-8, "rtol": 1e-5} for k, v in sm_rets_orig.items()},
- **sm_rets_orig._config,
- )
- module(x, expected_submod_rets=sm_rets)
- try:
- module(x, expected_submod_rets=sm_rets.random_uniform(map_sequences=True))
- raise Exception(
- "forward pass succeeded despite passing random expected_submod_rets, "
- "assertion error expected."
+ module(x, expected_submod_rets=sm_rets)
+ sm_rets = ivy.Container(
+ {
+ k: {"val": v, "atol": 1e-8, "rtol": 1e-5}
+ for k, v in sm_rets_orig.items()
+ },
+ **sm_rets_orig._config,
)
- except ivy.utils.exceptions.IvyException:
- pass
+ module(x, expected_submod_rets=sm_rets)
+ try:
+ module(x, expected_submod_rets=sm_rets.random_uniform(map_sequences=True))
+ raise Exception(
+ "forward pass succeeded despite passing random expected_submod_rets, "
+ "assertion error expected."
+ )
+ except ivy.utils.exceptions.IvyException:
+ pass
# module depth
@@ -272,26 +277,29 @@ def test_module_check_submod_rets(
input_channels=st.integers(min_value=2, max_value=5),
output_channels=st.integers(min_value=2, max_value=5),
)
-def test_module_depth(batch_shape, input_channels, output_channels, on_device):
+def test_module_depth(
+ batch_shape, input_channels, output_channels, on_device, backend_fw
+):
# smoke test
- if ivy.current_backend_str() == "numpy":
+ if backend_fw == "numpy":
# NumPy does not support gradients
return
- module = WithNestedModules(input_channels, output_channels, device=on_device)
+ with ivy.utils.backend.ContextManager(backend_fw):
+ module = WithNestedModules(input_channels, output_channels, device=on_device)
- # depth 0
- assert module.mod_depth() == 0
+ # depth 0
+ assert module.mod_depth() == 0
- # depth 1
- assert module._dl0.mod_depth() == 1
- assert module._dl1.mod_depth() == 1
+ # depth 1
+ assert module._dl0.mod_depth() == 1
+ assert module._dl1.mod_depth() == 1
- # depth 2
- assert module._dl0._l0.mod_depth() == 2
- assert module._dl0._l1.mod_depth() == 2
- assert module._dl1._l0.mod_depth() == 2
- assert module._dl1._l1.mod_depth() == 2
+ # depth 2
+ assert module._dl0._l0.mod_depth() == 2
+ assert module._dl0._l1.mod_depth() == 2
+ assert module._dl1._l0.mod_depth() == 2
+ assert module._dl1._l1.mod_depth() == 2
# module height
@@ -302,26 +310,29 @@ def test_module_depth(batch_shape, input_channels, output_channels, on_device):
input_channels=st.integers(min_value=2, max_value=5),
output_channels=st.integers(min_value=2, max_value=5),
)
-def test_module_height(batch_shape, input_channels, output_channels, on_device):
+def test_module_height(
+ batch_shape, input_channels, output_channels, on_device, backend_fw
+):
# smoke test
- if ivy.current_backend_str() == "numpy":
+ if backend_fw == "numpy":
# NumPy does not support gradients
return
- module = WithNestedModules(input_channels, output_channels, device=on_device)
+ with ivy.utils.backend.ContextManager(backend_fw):
+ module = WithNestedModules(input_channels, output_channels, device=on_device)
- # height 2
- assert module.mod_height() == 2
+ # height 2
+ assert module.mod_height() == 2
- # height 1
- assert module._dl0.mod_height() == 1
- assert module._dl1.mod_height() == 1
+ # height 1
+ assert module._dl0.mod_height() == 1
+ assert module._dl1.mod_height() == 1
- # height 0
- assert module._dl0._l0.mod_height() == 0
- assert module._dl0._l1.mod_height() == 0
- assert module._dl1._l0.mod_height() == 0
- assert module._dl1._l1.mod_height() == 0
+ # height 0
+ assert module._dl0._l0.mod_height() == 0
+ assert module._dl0._l1.mod_height() == 0
+ assert module._dl1._l0.mod_height() == 0
+ assert module._dl1._l1.mod_height() == 0
@given(
@@ -332,75 +343,80 @@ def test_module_height(batch_shape, input_channels, output_channels, on_device):
output_channels=st.integers(min_value=2, max_value=5),
)
def test_module_save_and_load_as_pickled(
- batch_shape, input_channels, output_channels, on_device
+ batch_shape, input_channels, output_channels, on_device, backend_fw
):
save_filepath = "module.pickled"
# smoke test
- if ivy.current_backend_str() == "numpy":
+ if backend_fw == "numpy":
# NumPy does not support gradients
return
- x = ivy.astype(
- ivy.linspace(ivy.zeros(batch_shape), ivy.ones(batch_shape), input_channels),
- "float32",
- )
- module = TrainableModule(input_channels, output_channels, device=on_device)
- def loss_fn(v_):
- out = module(x, v=v_)
- return ivy.mean(out)
+ with ivy.utils.backend.ContextManager(backend_fw):
+ x = ivy.astype(
+ ivy.linspace(ivy.zeros(batch_shape), ivy.ones(batch_shape), input_channels),
+ "float32",
+ )
+ module = TrainableModule(input_channels, output_channels, device=on_device)
+
+ def loss_fn(v_):
+ out = module(x, v=v_)
+ return ivy.mean(out)
- module.save(save_filepath)
- assert os.path.exists(save_filepath)
- loaded_module = ivy.Module.load(save_filepath)
+ module.save(save_filepath)
+ assert os.path.exists(save_filepath)
+ loaded_module = ivy.Module.load(save_filepath)
- # train
- loss, grads = ivy.execute_with_gradients(loss_fn, module.v)
- module.v = ivy.gradient_descent_update(module.v, grads, 1e-3)
+ # train
+ loss, grads = ivy.execute_with_gradients(loss_fn, module.v)
+ module.v = ivy.gradient_descent_update(module.v, grads, 1e-3)
- loaded_loss, loaded_grads = ivy.execute_with_gradients(loss_fn, loaded_module.v)
- loaded_module.v = ivy.gradient_descent_update(loaded_module.v, loaded_grads, 1e-3)
+ loaded_loss, loaded_grads = ivy.execute_with_gradients(loss_fn, loaded_module.v)
+ loaded_module.v = ivy.gradient_descent_update(
+ loaded_module.v, loaded_grads, 1e-3
+ )
- # type test
- assert ivy.is_array(loaded_loss)
- assert isinstance(loaded_grads, ivy.Container)
- # cardinality test
- assert loaded_loss.shape == ()
- # value test
- assert ivy.all_equal(loaded_loss == loss)
- assert ivy.Container.all(loaded_module.v == module.v).cont_all_true()
+ # type test
+ assert ivy.is_array(loaded_loss)
+ assert isinstance(loaded_grads, ivy.Container)
+ # cardinality test
+ assert loaded_loss.shape == ()
+ # value test
+ assert ivy.all_equal(loaded_loss == loss)
+ assert ivy.Container.all(loaded_module.v == module.v).cont_all_true()
- os.remove(save_filepath)
+ os.remove(save_filepath)
@given(dummy=st.booleans())
-def test_module_to_device(dummy, on_device):
- model = TrainableModule(5, 5)
- model.to_device(on_device)
-
- def assertion(x, on_device):
- if x != on_device:
- print(f"{x} is not equal to {on_device}")
- raise AssertionError
-
- def model_assert(mod, on_device):
- for key, obj in mod.v.items():
- if isinstance(obj, ivy.Module):
- return model_assert(obj, on_device)
- if isinstance(obj, ivy.Container) or isinstance(obj, dict):
- for item1, item2 in obj.items():
- assertion(item2.device, on_device)
-
- else:
- assertion(obj.device, on_device)
- if getattr(mod, "buffers", None):
- for key, obj in mod.buffers.items():
- if isinstance(obj, ivy.Container) or isinstance(obj, dict):
- ivy.nested_map(lambda x: assertion(x.device, on_device), obj)
+def test_module_to_device(dummy, on_device, backend_fw):
+ with ivy.utils.backend.ContextManager(backend_fw):
+ model = TrainableModule(5, 5)
+ model.to_device(on_device)
+
+ def assertion(x, on_device):
+ if x != on_device:
+ print(f"{x} is not equal to {on_device}")
+ raise AssertionError
+
+ def model_assert(mod, on_device):
+ for key, obj in mod.v.items():
+ if isinstance(obj, ivy.Module):
+ return model_assert(obj, on_device)
+ if isinstance(obj, (ivy.Container, dict)):
+ for item1, item2 in obj.items():
+ assertion(item2.device, on_device)
+
else:
assertion(obj.device, on_device)
+ if getattr(mod, "buffers", None):
+ for key, obj in mod.buffers.items():
+ if isinstance(obj, (ivy.Container, dict)):
+ ivy.nested_map(lambda x: assertion(x.device, on_device), obj)
+ else:
+ assertion(obj.device, on_device)
- model_assert(model, on_device)
+ model_assert(model, on_device)
# track submod call order
@@ -412,160 +428,169 @@ def model_assert(mod, on_device):
output_channels=st.integers(min_value=2, max_value=5),
)
def test_module_track_submod_call_order(
- batch_shape, input_channels, output_channels, on_device
+ batch_shape, input_channels, output_channels, on_device, backend_fw
):
# smoke test
- if ivy.current_backend_str() == "numpy":
+ if backend_fw == "numpy":
# NumPy does not support gradients
return
- x = ivy.astype(
- ivy.linspace(ivy.zeros(batch_shape), ivy.ones(batch_shape), input_channels),
- "float32",
- )
- module = WithNestedModules(input_channels, output_channels, device=on_device)
+ with ivy.utils.backend.ContextManager(backend_fw):
+ x = ivy.astype(
+ ivy.linspace(ivy.zeros(batch_shape), ivy.ones(batch_shape), input_channels),
+ "float32",
+ )
+ module = WithNestedModules(input_channels, output_channels, device=on_device)
- root_key_0 = ivy.Container.cont_flatten_key_chain(module.__repr__(), "_") + "_0"
+ root_key_0 = ivy.Container.cont_flatten_key_chain(module.__repr__(), "_") + "_0"
- dl0_key_0 = ivy.Container.cont_flatten_key_chain(module._dl0.__repr__(), "_") + "_0"
- dl1_key_0 = ivy.Container.cont_flatten_key_chain(module._dl1.__repr__(), "_") + "_0"
- dl1_key_1 = ivy.Container.cont_flatten_key_chain(module._dl1.__repr__(), "_") + "_1"
+ dl0_key_0 = (
+ ivy.Container.cont_flatten_key_chain(module._dl0.__repr__(), "_") + "_0"
+ )
+ dl1_key_0 = (
+ ivy.Container.cont_flatten_key_chain(module._dl1.__repr__(), "_") + "_0"
+ )
+ dl1_key_1 = (
+ ivy.Container.cont_flatten_key_chain(module._dl1.__repr__(), "_") + "_1"
+ )
- dl0_l0_key_0 = (
- ivy.Container.cont_flatten_key_chain(module._dl0._l0.__repr__(), "_") + "_0"
- )
- dl0_l1_key_0 = (
- ivy.Container.cont_flatten_key_chain(module._dl0._l1.__repr__(), "_") + "_0"
- )
- dl1_l0_key_0 = (
- ivy.Container.cont_flatten_key_chain(module._dl1._l0.__repr__(), "_") + "_0"
- )
- dl1_l1_key_0 = (
- ivy.Container.cont_flatten_key_chain(module._dl1._l1.__repr__(), "_") + "_0"
- )
+ dl0_l0_key_0 = (
+ ivy.Container.cont_flatten_key_chain(module._dl0._l0.__repr__(), "_") + "_0"
+ )
+ dl0_l1_key_0 = (
+ ivy.Container.cont_flatten_key_chain(module._dl0._l1.__repr__(), "_") + "_0"
+ )
+ dl1_l0_key_0 = (
+ ivy.Container.cont_flatten_key_chain(module._dl1._l0.__repr__(), "_") + "_0"
+ )
+ dl1_l1_key_0 = (
+ ivy.Container.cont_flatten_key_chain(module._dl1._l1.__repr__(), "_") + "_0"
+ )
- # depth 1
- ret = module(x, track_submod_call_order=True, submod_depth=1)
- assert ret.shape == tuple(list(batch_shape) + [64])
+ # depth 1
+ ret = module(x, track_submod_call_order=True, submod_depth=1)
+ assert ret.shape == tuple(list(batch_shape) + [64])
- sm_co = module.submod_call_order
+ sm_co = module.submod_call_order
- assert root_key_0 in sm_co
+ assert root_key_0 in sm_co
- assert dl0_key_0 in sm_co[root_key_0]
- assert dl1_key_0 in sm_co[root_key_0]
- assert dl1_key_1 in sm_co[root_key_0]
+ assert dl0_key_0 in sm_co[root_key_0]
+ assert dl1_key_0 in sm_co[root_key_0]
+ assert dl1_key_1 in sm_co[root_key_0]
- assert ivy.Container.cont_identical(
- [
- sm_co[root_key_0][dl0_key_0],
- module._dl0.v.cont_flatten_key_chains().to_numpy(),
- ]
- )
- assert ivy.Container.cont_identical(
- [
- sm_co[root_key_0][dl1_key_0],
- module._dl1.v.cont_flatten_key_chains().to_numpy(),
- ]
- )
- assert ivy.Container.cont_identical(
- [
- sm_co[root_key_0][dl1_key_1],
- module._dl1.v.cont_flatten_key_chains().to_numpy(),
- ]
- )
+ assert ivy.Container.cont_identical(
+ [
+ sm_co[root_key_0][dl0_key_0],
+ module._dl0.v.cont_flatten_key_chains().to_numpy(),
+ ]
+ )
+ assert ivy.Container.cont_identical(
+ [
+ sm_co[root_key_0][dl1_key_0],
+ module._dl1.v.cont_flatten_key_chains().to_numpy(),
+ ]
+ )
+ assert ivy.Container.cont_identical(
+ [
+ sm_co[root_key_0][dl1_key_1],
+ module._dl1.v.cont_flatten_key_chains().to_numpy(),
+ ]
+ )
- # depth 2 (full)
- ret = module(x, track_submod_call_order=True)
- assert ret.shape == tuple(list(batch_shape) + [64])
+ # depth 2 (full)
+ ret = module(x, track_submod_call_order=True)
+ assert ret.shape == tuple(list(batch_shape) + [64])
- sm_co = module.submod_call_order
+ sm_co = module.submod_call_order
- assert root_key_0 in sm_co
+ assert root_key_0 in sm_co
- assert dl0_key_0 in sm_co[root_key_0]
- assert dl1_key_0 in sm_co[root_key_0]
- assert dl1_key_1 in sm_co[root_key_0]
+ assert dl0_key_0 in sm_co[root_key_0]
+ assert dl1_key_0 in sm_co[root_key_0]
+ assert dl1_key_1 in sm_co[root_key_0]
- assert dl0_l0_key_0 in sm_co[root_key_0][dl0_key_0]
- assert dl0_l1_key_0 in sm_co[root_key_0][dl0_key_0]
- assert dl1_l0_key_0 in sm_co[root_key_0][dl1_key_0]
- assert dl1_l1_key_0 in sm_co[root_key_0][dl1_key_0]
- assert dl1_l0_key_0 in sm_co[root_key_0][dl1_key_1]
- assert dl1_l1_key_0 in sm_co[root_key_0][dl1_key_1]
+ assert dl0_l0_key_0 in sm_co[root_key_0][dl0_key_0]
+ assert dl0_l1_key_0 in sm_co[root_key_0][dl0_key_0]
+ assert dl1_l0_key_0 in sm_co[root_key_0][dl1_key_0]
+ assert dl1_l1_key_0 in sm_co[root_key_0][dl1_key_0]
+ assert dl1_l0_key_0 in sm_co[root_key_0][dl1_key_1]
+ assert dl1_l1_key_0 in sm_co[root_key_0][dl1_key_1]
- assert ivy.Container.cont_identical(
- [
- sm_co[root_key_0][dl0_key_0][dl0_l0_key_0],
- module._dl0._l0.v.cont_flatten_key_chains().to_numpy(),
- ]
- )
- assert ivy.Container.cont_identical(
- [
- sm_co[root_key_0][dl0_key_0][dl0_l1_key_0],
- module._dl0._l1.v.cont_flatten_key_chains().to_numpy(),
- ]
- )
- assert ivy.Container.cont_identical(
- [
- sm_co[root_key_0][dl1_key_0][dl1_l0_key_0],
- module._dl1._l0.v.cont_flatten_key_chains().to_numpy(),
- ]
- )
- assert ivy.Container.cont_identical(
- [
- sm_co[root_key_0][dl1_key_0][dl1_l1_key_0],
- module._dl1._l1.v.cont_flatten_key_chains().to_numpy(),
- ]
- )
- assert ivy.Container.cont_identical(
- [
- sm_co[root_key_0][dl1_key_1][dl1_l0_key_0],
- module._dl1._l0.v.cont_flatten_key_chains().to_numpy(),
- ]
- )
- assert ivy.Container.cont_identical(
- [
- sm_co[root_key_0][dl1_key_1][dl1_l1_key_0],
- module._dl1._l1.v.cont_flatten_key_chains().to_numpy(),
- ]
- )
+ assert ivy.Container.cont_identical(
+ [
+ sm_co[root_key_0][dl0_key_0][dl0_l0_key_0],
+ module._dl0._l0.v.cont_flatten_key_chains().to_numpy(),
+ ]
+ )
+ assert ivy.Container.cont_identical(
+ [
+ sm_co[root_key_0][dl0_key_0][dl0_l1_key_0],
+ module._dl0._l1.v.cont_flatten_key_chains().to_numpy(),
+ ]
+ )
+ assert ivy.Container.cont_identical(
+ [
+ sm_co[root_key_0][dl1_key_0][dl1_l0_key_0],
+ module._dl1._l0.v.cont_flatten_key_chains().to_numpy(),
+ ]
+ )
+ assert ivy.Container.cont_identical(
+ [
+ sm_co[root_key_0][dl1_key_0][dl1_l1_key_0],
+ module._dl1._l1.v.cont_flatten_key_chains().to_numpy(),
+ ]
+ )
+ assert ivy.Container.cont_identical(
+ [
+ sm_co[root_key_0][dl1_key_1][dl1_l0_key_0],
+ module._dl1._l0.v.cont_flatten_key_chains().to_numpy(),
+ ]
+ )
+ assert ivy.Container.cont_identical(
+ [
+ sm_co[root_key_0][dl1_key_1][dl1_l1_key_0],
+ module._dl1._l1.v.cont_flatten_key_chains().to_numpy(),
+ ]
+ )
- # partial submodules
- ret = module(
- x, track_submod_call_order=True, submods_to_track=[module._dl1, module._dl0._l0]
- )
- assert ret.shape == tuple(list(batch_shape) + [64])
+ # partial submodules
+ ret = module(
+ x,
+ track_submod_call_order=True,
+ submods_to_track=[module._dl1, module._dl0._l0],
+ )
+ assert ret.shape == tuple(list(batch_shape) + [64])
- sm_co = module.submod_call_order
+ sm_co = module.submod_call_order
- assert root_key_0 in sm_co
+ assert root_key_0 in sm_co
- assert dl0_key_0 in sm_co[root_key_0]
- assert dl1_key_0 in sm_co[root_key_0]
- assert dl1_key_1 in sm_co[root_key_0]
+ assert dl0_key_0 in sm_co[root_key_0]
+ assert dl1_key_0 in sm_co[root_key_0]
+ assert dl1_key_1 in sm_co[root_key_0]
- assert dl0_l0_key_0 in sm_co[root_key_0][dl0_key_0]
- assert dl0_l1_key_0 not in sm_co[root_key_0][dl0_key_0]
- assert ivy.Container.cont_identical(
- [
- sm_co[root_key_0][dl1_key_0],
- module._dl1.v.cont_flatten_key_chains().to_numpy(),
- ]
- )
- assert ivy.Container.cont_identical(
- [
- sm_co[root_key_0][dl1_key_1],
- module._dl1.v.cont_flatten_key_chains().to_numpy(),
- ]
- )
+ assert dl0_l0_key_0 in sm_co[root_key_0][dl0_key_0]
+ assert dl0_l1_key_0 not in sm_co[root_key_0][dl0_key_0]
+ assert ivy.Container.cont_identical(
+ [
+ sm_co[root_key_0][dl1_key_0],
+ module._dl1.v.cont_flatten_key_chains().to_numpy(),
+ ]
+ )
+ assert ivy.Container.cont_identical(
+ [
+ sm_co[root_key_0][dl1_key_1],
+ module._dl1.v.cont_flatten_key_chains().to_numpy(),
+ ]
+ )
- assert ivy.Container.cont_identical(
- [
- sm_co[root_key_0][dl0_key_0][dl0_l0_key_0],
- module._dl0._l0.v.cont_flatten_key_chains().to_numpy(),
- ]
- )
+ assert ivy.Container.cont_identical(
+ [
+ sm_co[root_key_0][dl0_key_0][dl0_l0_key_0],
+ module._dl0._l0.v.cont_flatten_key_chains().to_numpy(),
+ ]
+ )
# track submod returns
@@ -577,62 +602,70 @@ def test_module_track_submod_call_order(
output_channels=st.integers(min_value=2, max_value=5),
)
def test_module_track_submod_rets(
- batch_shape, input_channels, output_channels, on_device
+ batch_shape, input_channels, output_channels, on_device, backend_fw
):
# smoke test
- if ivy.current_backend_str() == "numpy":
+ if backend_fw == "numpy":
# NumPy does not support gradients
return
- x = ivy.astype(
- ivy.linspace(ivy.zeros(batch_shape), ivy.ones(batch_shape), input_channels),
- "float32",
- )
- module = WithNestedModules(input_channels, output_channels, device=on_device)
-
- # depth 1
- ret = module(x, track_submod_rets=True, submod_depth=1)
- assert ret.shape == tuple(list(batch_shape) + [64])
- sm_rets = module.submod_rets
- for submod in [module._dl0, module._dl1]:
- for ret in sm_rets[submod.get_mod_key()]:
- assert isinstance(ret, np.ndarray)
- assert ret.shape == tuple(list(batch_shape) + [64])
- for submod in [module._dl0._l0, module._dl0._l1, module._dl1._l0, module._dl1._l1]:
- assert (
- ivy.Container.cont_flatten_key_chain(submod.__repr__(), "_") not in sm_rets
+ with ivy.utils.backend.ContextManager(backend_fw):
+ x = ivy.astype(
+ ivy.linspace(ivy.zeros(batch_shape), ivy.ones(batch_shape), input_channels),
+ "float32",
)
+ module = WithNestedModules(input_channels, output_channels, device=on_device)
- # depth 2 (full)
- ret = module(x, track_submod_rets=True)
- assert ret.shape == tuple(list(batch_shape) + [64])
- sm_rets = module.submod_rets
- for submod in [
- module._dl0,
- module._dl1,
- module._dl0._l0,
- module._dl0._l1,
- module._dl1._l0,
- module._dl1._l1,
- ]:
- for ret in sm_rets[submod.get_mod_key()]:
- assert isinstance(ret, np.ndarray)
- assert ret.shape == tuple(list(batch_shape) + [64])
-
- # partial submodules
- ret = module(
- x, track_submod_rets=True, submods_to_track=[module._dl1, module._dl0._l0]
- )
- assert ret.shape == tuple(list(batch_shape) + [64])
- sm_rets = module.submod_rets
- for submod in [module._dl1, module._dl0._l0]:
- for ret in sm_rets[submod.get_mod_key()]:
- assert isinstance(ret, np.ndarray)
- assert ret.shape == tuple(list(batch_shape) + [64])
- for submod in [module._dl0, module._dl0._l1, module._dl1._l0, module._dl1._l1]:
- assert (
- ivy.Container.cont_flatten_key_chain(submod.__repr__(), "_") not in sm_rets
+ # depth 1
+ ret = module(x, track_submod_rets=True, submod_depth=1)
+ assert ret.shape == tuple(list(batch_shape) + [64])
+ sm_rets = module.submod_rets
+ for submod in [module._dl0, module._dl1]:
+ for ret in sm_rets[submod.get_mod_key()]:
+ assert isinstance(ret, np.ndarray)
+ assert ret.shape == tuple(list(batch_shape) + [64])
+ for submod in [
+ module._dl0._l0,
+ module._dl0._l1,
+ module._dl1._l0,
+ module._dl1._l1,
+ ]:
+ assert (
+ ivy.Container.cont_flatten_key_chain(submod.__repr__(), "_")
+ not in sm_rets
+ )
+
+ # depth 2 (full)
+ ret = module(x, track_submod_rets=True)
+ assert ret.shape == tuple(list(batch_shape) + [64])
+ sm_rets = module.submod_rets
+ for submod in [
+ module._dl0,
+ module._dl1,
+ module._dl0._l0,
+ module._dl0._l1,
+ module._dl1._l0,
+ module._dl1._l1,
+ ]:
+ for ret in sm_rets[submod.get_mod_key()]:
+ assert isinstance(ret, np.ndarray)
+ assert ret.shape == tuple(list(batch_shape) + [64])
+
+ # partial submodules
+ ret = module(
+ x, track_submod_rets=True, submods_to_track=[module._dl1, module._dl0._l0]
)
+ assert ret.shape == tuple(list(batch_shape) + [64])
+ sm_rets = module.submod_rets
+ for submod in [module._dl1, module._dl0._l0]:
+ for ret in sm_rets[submod.get_mod_key()]:
+ assert isinstance(ret, np.ndarray)
+ assert ret.shape == tuple(list(batch_shape) + [64])
+ for submod in [module._dl0, module._dl0._l1, module._dl1._l0, module._dl1._l1]:
+ assert (
+ ivy.Container.cont_flatten_key_chain(submod.__repr__(), "_")
+ not in sm_rets
+ )
# module training
@@ -643,47 +676,51 @@ def test_module_track_submod_rets(
input_channels=st.integers(min_value=2, max_value=5),
output_channels=st.integers(min_value=2, max_value=5),
)
-def test_module_training(batch_shape, input_channels, output_channels, on_device):
+def test_module_training(
+ batch_shape, input_channels, output_channels, on_device, backend_fw
+):
# smoke test
- if ivy.current_backend_str() == "numpy":
+ if backend_fw == "numpy":
# NumPy does not support gradients
return
- x = ivy.astype(
- ivy.linspace(ivy.zeros(batch_shape), ivy.ones(batch_shape), input_channels),
- "float32",
- )
- module = TrainableModule(input_channels, output_channels, device=on_device)
- def loss_fn(v_):
- out = module(x, v=v_)
- return ivy.mean(out)
-
- # train
- loss_tm1 = 1e12
- loss = None
- grads = None
- for i in range(10):
- loss, grads = ivy.execute_with_gradients(loss_fn, module.v)
- module.v = ivy.gradient_descent_update(module.v, grads, 1e-3)
- assert loss < loss_tm1
- loss_tm1 = loss
-
- # type test
- assert ivy.is_array(loss)
- assert isinstance(grads, ivy.Container)
- # cardinality test
- assert loss.shape == ()
- # value test
- assert ivy.max(ivy.abs(grads.linear0.b)) > 0
- assert ivy.max(ivy.abs(grads.linear0.w)) > 0
- assert ivy.max(ivy.abs(grads.linear1.b)) > 0
- assert ivy.max(ivy.abs(grads.linear1.w)) > 0
- assert ivy.max(ivy.abs(grads.linear2.b)) > 0
- assert ivy.max(ivy.abs(grads.linear2.w)) > 0
- # tracing test
- if ivy.current_backend_str() == "torch":
- # pytest scripting does not support **kwargs
- return
+ with ivy.utils.backend.ContextManager(backend_fw):
+ x = ivy.astype(
+ ivy.linspace(ivy.zeros(batch_shape), ivy.ones(batch_shape), input_channels),
+ "float32",
+ )
+ module = TrainableModule(input_channels, output_channels, device=on_device)
+
+ def loss_fn(v_):
+ out = module(x, v=v_)
+ return ivy.mean(out)
+
+ # train
+ loss_tm1 = 1e12
+ loss = None
+ grads = None
+ for i in range(10):
+ loss, grads = ivy.execute_with_gradients(loss_fn, module.v)
+ module.v = ivy.gradient_descent_update(module.v, grads, 1e-3)
+ assert loss < loss_tm1
+ loss_tm1 = loss
+
+ # type test
+ assert ivy.is_array(loss)
+ assert isinstance(grads, ivy.Container)
+ # cardinality test
+ assert loss.shape == ()
+ # value test
+ assert ivy.max(ivy.abs(grads.linear0.b)) > 0
+ assert ivy.max(ivy.abs(grads.linear0.w)) > 0
+ assert ivy.max(ivy.abs(grads.linear1.b)) > 0
+ assert ivy.max(ivy.abs(grads.linear1.w)) > 0
+ assert ivy.max(ivy.abs(grads.linear2.b)) > 0
+ assert ivy.max(ivy.abs(grads.linear2.w)) > 0
+ # tracing test
+ if backend_fw == "torch":
+ # pytest scripting does not support **kwargs
+ return
# module training with duplicate
@@ -694,44 +731,49 @@ def loss_fn(v_):
channels=st.integers(min_value=1, max_value=64),
same_layer=st.booleans(),
)
-def test_module_training_with_duplicate(batch_shape, channels, same_layer, on_device):
+def test_module_training_with_duplicate(
+ batch_shape, channels, same_layer, on_device, backend_fw
+):
# smoke test
- if ivy.current_backend_str() == "numpy":
+ if backend_fw == "numpy":
# NumPy does not support gradients
return
- x = ivy.astype(
- ivy.linspace(ivy.zeros(batch_shape), ivy.ones(batch_shape), channels), "float32"
- )
- module = TrainableModuleWithDuplicate(channels, same_layer, device=on_device)
- def loss_fn(v_):
- out = module(x, v=v_)
- return ivy.mean(out)
-
- # train
- loss_tm1 = 1e12
- loss = None
- grads = None
- for i in range(10):
- loss, grads = ivy.execute_with_gradients(loss_fn, module.v)
- module.v = ivy.gradient_descent_update(module.v, grads, 1e-3)
- assert loss < loss_tm1
- loss_tm1 = loss
-
- # type test
- assert ivy.is_array(loss)
- assert isinstance(grads, ivy.Container)
- # cardinality test
- assert loss.shape == ()
- # value test
- assert ivy.max(ivy.abs(grads.linear0.b)) > 0
- assert ivy.max(ivy.abs(grads.linear0.w)) > 0
- if not same_layer:
- assert ivy.max(ivy.abs(grads.linear1.b)) > 0
- # tracing test
- if ivy.current_backend_str() == "torch":
- # pytest scripting does not support **kwargs
- return
+ with ivy.utils.backend.ContextManager(backend_fw):
+ x = ivy.astype(
+ ivy.linspace(ivy.zeros(batch_shape), ivy.ones(batch_shape), channels),
+ "float32",
+ )
+ module = TrainableModuleWithDuplicate(channels, same_layer, device=on_device)
+
+ def loss_fn(v_):
+ out = module(x, v=v_)
+ return ivy.mean(out)
+
+ # train
+ loss_tm1 = 1e12
+ loss = None
+ grads = None
+ for i in range(10):
+ loss, grads = ivy.execute_with_gradients(loss_fn, module.v)
+ module.v = ivy.gradient_descent_update(module.v, grads, 1e-3)
+ assert loss < loss_tm1
+ loss_tm1 = loss
+
+ # type test
+ assert ivy.is_array(loss)
+ assert isinstance(grads, ivy.Container)
+ # cardinality test
+ assert loss.shape == ()
+ # value test
+ assert ivy.max(ivy.abs(grads.linear0.b)) > 0
+ assert ivy.max(ivy.abs(grads.linear0.w)) > 0
+ if not same_layer:
+ assert ivy.max(ivy.abs(grads.linear1.b)) > 0
+ # tracing test
+ if backend_fw == "torch":
+ # pytest scripting does not support **kwargs
+ return
# module with dict training
@@ -743,48 +785,52 @@ def loss_fn(v_):
output_channels=st.integers(min_value=2, max_value=5),
)
def test_module_w_dict_training(
- batch_shape, input_channels, output_channels, on_device
+ batch_shape, input_channels, output_channels, on_device, backend_fw
):
# smoke test
- if ivy.current_backend_str() == "numpy":
+ if backend_fw == "numpy":
# NumPy does not support gradients
return
- x = ivy.astype(
- ivy.linspace(ivy.zeros(batch_shape), ivy.ones(batch_shape), input_channels),
- "float32",
- )
- module = TrainableModuleWithDict(input_channels, output_channels, device=on_device)
- def loss_fn(v_):
- out = module(x, v=v_)
- return ivy.mean(out)
+ with ivy.utils.backend.ContextManager(backend_fw):
+ x = ivy.astype(
+ ivy.linspace(ivy.zeros(batch_shape), ivy.ones(batch_shape), input_channels),
+ "float32",
+ )
+ module = TrainableModuleWithDict(
+ input_channels, output_channels, device=on_device
+ )
- # train
- loss_tm1 = 1e12
- loss = None
- grads = None
- for i in range(10):
- loss, grads = ivy.execute_with_gradients(loss_fn, module.v)
- module.v = ivy.gradient_descent_update(module.v, grads, 1e-3)
- assert loss < loss_tm1
- loss_tm1 = loss
-
- # type test
- assert ivy.is_array(loss)
- assert isinstance(grads, ivy.Container)
- # cardinality test
- assert loss.shape == ()
- # value test
- assert ivy.max(ivy.abs(grads.layers.linear0.b)) > 0
- assert ivy.max(ivy.abs(grads.layers.linear0.w)) > 0
- assert ivy.max(ivy.abs(grads.layers.linear1.b)) > 0
- assert ivy.max(ivy.abs(grads.layers.linear1.w)) > 0
- assert ivy.max(ivy.abs(grads.layers.linear2.b)) > 0
- assert ivy.max(ivy.abs(grads.layers.linear2.w)) > 0
- # tracing test
- if ivy.current_backend_str() == "torch":
- # pytest scripting does not support **kwargs
- return
+ def loss_fn(v_):
+ out = module(x, v=v_)
+ return ivy.mean(out)
+
+ # train
+ loss_tm1 = 1e12
+ loss = None
+ grads = None
+ for i in range(10):
+ loss, grads = ivy.execute_with_gradients(loss_fn, module.v)
+ module.v = ivy.gradient_descent_update(module.v, grads, 1e-3)
+ assert loss < loss_tm1
+ loss_tm1 = loss
+
+ # type test
+ assert ivy.is_array(loss)
+ assert isinstance(grads, ivy.Container)
+ # cardinality test
+ assert loss.shape == ()
+ # value test
+ assert ivy.max(ivy.abs(grads.layers.linear0.b)) > 0
+ assert ivy.max(ivy.abs(grads.layers.linear0.w)) > 0
+ assert ivy.max(ivy.abs(grads.layers.linear1.b)) > 0
+ assert ivy.max(ivy.abs(grads.layers.linear1.w)) > 0
+ assert ivy.max(ivy.abs(grads.layers.linear2.b)) > 0
+ assert ivy.max(ivy.abs(grads.layers.linear2.w)) > 0
+ # tracing test
+ if backend_fw == "torch":
+ # pytest scripting does not support **kwargs
+ return
# module with list training
@@ -796,48 +842,52 @@ def loss_fn(v_):
output_channels=st.integers(min_value=2, max_value=5),
)
def test_module_w_list_training(
- batch_shape, input_channels, output_channels, on_device
+ batch_shape, input_channels, output_channels, on_device, backend_fw
):
# smoke test
- if ivy.current_backend_str() == "numpy":
+ if backend_fw == "numpy":
# NumPy does not support gradients
return
- x = ivy.astype(
- ivy.linspace(ivy.zeros(batch_shape), ivy.ones(batch_shape), input_channels),
- "float32",
- )
- module = TrainableModuleWithList(input_channels, output_channels, device=on_device)
- def loss_fn(v_):
- out = module(x, v=v_)
- return ivy.mean(out)
+ with ivy.utils.backend.ContextManager(backend_fw):
+ x = ivy.astype(
+ ivy.linspace(ivy.zeros(batch_shape), ivy.ones(batch_shape), input_channels),
+ "float32",
+ )
+ module = TrainableModuleWithList(
+ input_channels, output_channels, device=on_device
+ )
- # train
- loss_tm1 = 1e12
- loss = None
- grads = None
- for i in range(10):
- loss, grads = ivy.execute_with_gradients(loss_fn, module.v)
- module.v = ivy.gradient_descent_update(module.v, grads, 1e-3)
- assert loss < loss_tm1
- loss_tm1 = loss
-
- # type test
- assert ivy.is_array(loss)
- assert isinstance(grads, ivy.Container)
- # cardinality test
- assert loss.shape == ()
- # value test
- assert ivy.max(ivy.abs(grads.layers.v0.b)) > 0
- assert ivy.max(ivy.abs(grads.layers.v0.w)) > 0
- assert ivy.max(ivy.abs(grads.layers.v1.b)) > 0
- assert ivy.max(ivy.abs(grads.layers.v1.w)) > 0
- assert ivy.max(ivy.abs(grads.layers.v2.b)) > 0
- assert ivy.max(ivy.abs(grads.layers.v2.w)) > 0
- # tracing test
- if ivy.current_backend_str() == "torch":
- # pytest scripting does not support **kwargs
- return
+ def loss_fn(v_):
+ out = module(x, v=v_)
+ return ivy.mean(out)
+
+ # train
+ loss_tm1 = 1e12
+ loss = None
+ grads = None
+ for i in range(10):
+ loss, grads = ivy.execute_with_gradients(loss_fn, module.v)
+ module.v = ivy.gradient_descent_update(module.v, grads, 1e-3)
+ assert loss < loss_tm1
+ loss_tm1 = loss
+
+ # type test
+ assert ivy.is_array(loss)
+ assert isinstance(grads, ivy.Container)
+ # cardinality test
+ assert loss.shape == ()
+ # value test
+ assert ivy.max(ivy.abs(grads.layers.v0.b)) > 0
+ assert ivy.max(ivy.abs(grads.layers.v0.w)) > 0
+ assert ivy.max(ivy.abs(grads.layers.v1.b)) > 0
+ assert ivy.max(ivy.abs(grads.layers.v1.w)) > 0
+ assert ivy.max(ivy.abs(grads.layers.v2.b)) > 0
+ assert ivy.max(ivy.abs(grads.layers.v2.w)) > 0
+ # tracing test
+ if backend_fw == "torch":
+ # pytest scripting does not support **kwargs
+ return
# module with none attribute
@@ -849,19 +899,20 @@ def loss_fn(v_):
output_channels=st.integers(min_value=2, max_value=5),
)
def test_module_w_none_attribute(
- batch_shape, input_channels, output_channels, on_device
+ batch_shape, input_channels, output_channels, on_device, backend_fw
):
# smoke test
- if ivy.current_backend_str() == "numpy":
+ if backend_fw == "numpy":
# NumPy does not support gradients
return
- x = ivy.astype(
- ivy.linspace(ivy.zeros(batch_shape), ivy.ones(batch_shape), input_channels),
- "float32",
- )
- module = ModuleWithNoneAttribute(device=on_device)
- module(x)
+ with ivy.utils.backend.ContextManager(backend_fw):
+ x = ivy.astype(
+ ivy.linspace(ivy.zeros(batch_shape), ivy.ones(batch_shape), input_channels),
+ "float32",
+ )
+ module = ModuleWithNoneAttribute(device=on_device)
+ module(x)
# module with partial v
@@ -872,65 +923,73 @@ def test_module_w_none_attribute(
input_channels=st.integers(min_value=2, max_value=5),
output_channels=st.integers(min_value=2, max_value=5),
)
-def test_module_w_partial_v(batch_shape, input_channels, output_channels, on_device):
+def test_module_w_partial_v(
+ batch_shape, input_channels, output_channels, on_device, backend_fw
+):
# smoke test
- if ivy.current_backend_str() == "numpy":
+ if backend_fw == "numpy":
# NumPy does not support gradients
-
return
- x = ivy.astype(
- ivy.linspace(ivy.zeros(batch_shape), ivy.ones(batch_shape), input_channels),
- "float32",
- )
- v = ivy.Container(
- {
- "linear0": {
- "b": _variable(ivy.random_uniform(shape=[64])),
- "w": _variable(ivy.random_uniform(shape=[64, 4])),
- },
- "linear1": {
- "b": _variable(ivy.random_uniform(shape=[64])),
- "w": _variable(ivy.random_uniform(shape=[64, 64])),
- "extra": _variable(ivy.random_uniform(shape=[64, 64])),
- },
- "linear2": {
- "b": _variable(ivy.random_uniform(shape=[5])),
- "w": _variable(ivy.random_uniform(shape=[5, 64])),
- },
- }
- )
- try:
- TrainableModule(
- input_channels, output_channels, device=on_device, v=v, with_partial_v=True
+ with ivy.utils.backend.ContextManager(backend_fw):
+ x = ivy.astype(
+ ivy.linspace(ivy.zeros(batch_shape), ivy.ones(batch_shape), input_channels),
+ "float32",
+ )
+ v = ivy.Container(
+ {
+ "linear0": {
+ "b": _variable(ivy.random_uniform(shape=[64])),
+ "w": _variable(ivy.random_uniform(shape=[64, 4])),
+ },
+ "linear1": {
+ "b": _variable(ivy.random_uniform(shape=[64])),
+ "w": _variable(ivy.random_uniform(shape=[64, 64])),
+ "extra": _variable(ivy.random_uniform(shape=[64, 64])),
+ },
+ "linear2": {
+ "b": _variable(ivy.random_uniform(shape=[5])),
+ "w": _variable(ivy.random_uniform(shape=[5, 64])),
+ },
+ }
)
- raise Exception(
- "TrainableModule did not raise exception desipite being passed "
- "with wrongly shaped variables."
+ try:
+ TrainableModule(
+ input_channels,
+ output_channels,
+ device=on_device,
+ v=v,
+ with_partial_v=True,
+ )
+ raise Exception(
+ "TrainableModule did not raise exception despite being passed "
+ "with wrongly shaped variables."
+ )
+ except ivy.utils.exceptions.IvyException:
+ pass
+ v = ivy.Container(
+ {
+ "linear0": {
+ "b": _variable(ivy.random_uniform(shape=[64])),
+ },
+ "linear1": {"w": _variable(ivy.random_uniform(shape=[64, 64]))},
+ "linear2": {
+ "b": _variable(ivy.random_uniform(shape=[output_channels]))
+ },
+ }
)
- except ivy.utils.exceptions.IvyException:
- pass
- v = ivy.Container(
- {
- "linear0": {
- "b": _variable(ivy.random_uniform(shape=[64])),
- },
- "linear1": {"w": _variable(ivy.random_uniform(shape=[64, 64]))},
- "linear2": {"b": _variable(ivy.random_uniform(shape=[output_channels]))},
- }
- )
- try:
- TrainableModule(input_channels, output_channels, device=on_device, v=v)
- raise Exception(
- "TrainableModule did not raise exception desipite being passed "
- "with wrongly shaped variables."
+ try:
+ TrainableModule(input_channels, output_channels, device=on_device, v=v)
+ raise Exception(
+ "TrainableModule did not raise exception despite being passed "
+ "with wrongly shaped variables."
+ )
+ except ivy.utils.exceptions.IvyException:
+ pass
+ module = TrainableModule(
+ input_channels, output_channels, device=on_device, v=v, with_partial_v=True
)
- except ivy.utils.exceptions.IvyException:
- pass
- module = TrainableModule(
- input_channels, output_channels, device=on_device, v=v, with_partial_v=True
- )
- module(x)
+ module(x)
# sub modules
@@ -941,32 +1000,35 @@ def test_module_w_partial_v(batch_shape, input_channels, output_channels, on_dev
input_channels=st.integers(min_value=2, max_value=5),
output_channels=st.integers(min_value=2, max_value=5),
)
-def test_sub_modules(batch_shape, input_channels, output_channels, on_device):
+def test_sub_modules(
+ batch_shape, input_channels, output_channels, on_device, backend_fw
+):
# smoke test
- if ivy.current_backend_str() == "numpy":
+ if backend_fw == "numpy":
# NumPy does not support gradients
return
- module = WithNestedModules(input_channels, output_channels, device=on_device)
+ with ivy.utils.backend.ContextManager(backend_fw):
+ module = WithNestedModules(input_channels, output_channels, device=on_device)
- # depth 0
- sub_mods = module.sub_mods(depth=0)
- assert module.v is sub_mods
+ # depth 0
+ sub_mods = module.sub_mods(depth=0)
+ assert module.v is sub_mods
- # depth 1
- sub_mods = module.sub_mods(depth=1)
- for v in [module._dl0.v, module._dl1.v]:
- assert v in sub_mods
+ # depth 1
+ sub_mods = module.sub_mods(depth=1)
+ for v in [module._dl0.v, module._dl1.v]:
+ assert v in sub_mods
- # depth 2 (full)
- sub_mods = module.sub_mods()
- for v in [
- module._dl0._l0.v,
- module._dl0._l1.v,
- module._dl1._l0.v,
- module._dl1._l1.v,
- ]:
- assert v in sub_mods
+ # depth 2 (full)
+ sub_mods = module.sub_mods()
+ for v in [
+ module._dl0._l0.v,
+ module._dl0._l1.v,
+ module._dl1._l0.v,
+ module._dl1._l1.v,
+ ]:
+ assert v in sub_mods
# top module
@@ -977,28 +1039,31 @@ def test_sub_modules(batch_shape, input_channels, output_channels, on_device):
input_channels=st.integers(min_value=2, max_value=5),
output_channels=st.integers(min_value=2, max_value=5),
)
-def test_top_module(batch_shape, input_channels, output_channels, on_device):
+def test_top_module(
+ batch_shape, input_channels, output_channels, on_device, backend_fw
+):
# smoke test
- if ivy.current_backend_str() == "numpy":
+ if backend_fw == "numpy":
# NumPy does not support gradients
return
- module = WithNestedModules(input_channels, output_channels, device=on_device)
+ with ivy.utils.backend.ContextManager(backend_fw):
+ module = WithNestedModules(input_channels, output_channels, device=on_device)
- # full depth
- assert module._dl0.top_mod() is module
- assert module._dl1.top_mod() is module
+ # full depth
+ assert module._dl0.top_mod() is module
+ assert module._dl1.top_mod() is module
- assert module._dl0._l0.top_mod() is module
- assert module._dl0._l1.top_mod() is module
- assert module._dl1._l0.top_mod() is module
- assert module._dl1._l1.top_mod() is module
+ assert module._dl0._l0.top_mod() is module
+ assert module._dl0._l1.top_mod() is module
+ assert module._dl1._l0.top_mod() is module
+ assert module._dl1._l1.top_mod() is module
- # depth 1
- assert module._dl0._l0.top_mod(1) is module._dl0
- assert module._dl0._l1.top_mod(1) is module._dl0
- assert module._dl1._l0.top_mod(1) is module._dl1
- assert module._dl1._l1.top_mod(1) is module._dl1
+ # depth 1
+ assert module._dl0._l0.top_mod(1) is module._dl0
+ assert module._dl0._l1.top_mod(1) is module._dl0
+ assert module._dl1._l0.top_mod(1) is module._dl1
+ assert module._dl1._l1.top_mod(1) is module._dl1
# top variables
@@ -1009,46 +1074,51 @@ def test_top_module(batch_shape, input_channels, output_channels, on_device):
input_channels=st.integers(min_value=2, max_value=5),
output_channels=st.integers(min_value=2, max_value=5),
)
-def test_top_variables(batch_shape, input_channels, output_channels, on_device):
+def test_top_variables(
+ batch_shape, input_channels, output_channels, on_device, backend_fw
+):
# smoke test
- if ivy.current_backend_str() == "numpy":
+ if backend_fw == "numpy":
# NumPy does not support gradients
return
- module = WithNestedModules(input_channels, output_channels, device=on_device)
- for key_chain in [
- "dl0",
- "dl0/l0",
- "dl0/l1",
- "dl0/l0/b",
- "dl0/l0/w",
- "dl0/l1/b",
- "dl0/l1/w",
- "dl1",
- "dl1/l0",
- "dl1/l1",
- "dl1/l0/b",
- "dl1/l0/w",
- "dl1/l1/b",
- "dl1/l1/w",
- ]:
- # depth 1
- assert key_chain in module._dl0.top_v()
- assert key_chain in module._dl1.top_v()
- # depth 2
- assert key_chain in module._dl0._l0.top_v()
- assert key_chain in module._dl0._l1.top_v()
- assert key_chain in module._dl1._l0.top_v()
- assert key_chain in module._dl1._l1.top_v()
+ with ivy.utils.backend.ContextManager(backend_fw):
+ module = WithNestedModules(input_channels, output_channels, device=on_device)
+ for key_chain in [
+ "dl0",
+ "dl0/l0",
+ "dl0/l1",
+ "dl0/l0/b",
+ "dl0/l0/w",
+ "dl0/l1/b",
+ "dl0/l1/w",
+ "dl1",
+ "dl1/l0",
+ "dl1/l1",
+ "dl1/l0/b",
+ "dl1/l0/w",
+ "dl1/l1/b",
+ "dl1/l1/w",
+ ]:
+ # depth 1
+ assert key_chain in module._dl0.top_v()
+ assert key_chain in module._dl1.top_v()
+
+ # depth 2
+ assert key_chain in module._dl0._l0.top_v()
+ assert key_chain in module._dl0._l1.top_v()
+ assert key_chain in module._dl1._l0.top_v()
+ assert key_chain in module._dl1._l1.top_v()
@given(mode=st.booleans())
-def test_train_eval(mode):
- cls = ModuleWithTrainEval()
- cls.train(mode)
- assert mode == cls.training
- cls.eval()
- assert not cls.training
+def test_train_eval(mode, backend_fw):
+ with ivy.utils.backend.ContextManager(backend_fw):
+ cls = ModuleWithTrainEval()
+ cls.train(mode)
+ assert mode == cls.training
+ cls.eval()
+ assert not cls.training
# v with top v key chains
@@ -1060,61 +1130,62 @@ def test_train_eval(mode):
output_channels=st.integers(min_value=2, max_value=5),
)
def test_v_with_top_v_key_chains(
- batch_shape, input_channels, output_channels, on_device
+ batch_shape, input_channels, output_channels, on_device, backend_fw
):
# smoke test
- if ivy.current_backend_str() == "numpy":
+ if backend_fw == "numpy":
# NumPy does not support gradients
return
- module = WithNestedModules(input_channels, output_channels, device=on_device)
+ with ivy.utils.backend.ContextManager(backend_fw):
+ module = WithNestedModules(input_channels, output_channels, device=on_device)
- # full depth
- v = module._dl0.v_with_top_v_key_chains()
- assert "dl0" in v
- assert v.dl0 is module._dl0.v
+ # full depth
+ v = module._dl0.v_with_top_v_key_chains()
+ assert "dl0" in v
+ assert v.dl0 is module._dl0.v
- v = module._dl1.v_with_top_v_key_chains()
- assert "dl1" in v
- assert v.dl1 is module._dl1.v
+ v = module._dl1.v_with_top_v_key_chains()
+ assert "dl1" in v
+ assert v.dl1 is module._dl1.v
- v = module._dl0._l0.v_with_top_v_key_chains()
- assert "dl0" in v
- assert "l0" in v.dl0
- assert v.dl0.l0 is module._dl0._l0.v
+ v = module._dl0._l0.v_with_top_v_key_chains()
+ assert "dl0" in v
+ assert "l0" in v.dl0
+ assert v.dl0.l0 is module._dl0._l0.v
- v = module._dl0._l1.v_with_top_v_key_chains()
- assert "dl0" in v
- assert "l1" in v.dl0
- assert v.dl0.l1 is module._dl0._l1.v
+ v = module._dl0._l1.v_with_top_v_key_chains()
+ assert "dl0" in v
+ assert "l1" in v.dl0
+ assert v.dl0.l1 is module._dl0._l1.v
- v = module._dl1._l0.v_with_top_v_key_chains()
- assert "dl1" in v
- assert "l0" in v.dl1
- assert v.dl1.l0 is module._dl1._l0.v
+ v = module._dl1._l0.v_with_top_v_key_chains()
+ assert "dl1" in v
+ assert "l0" in v.dl1
+ assert v.dl1.l0 is module._dl1._l0.v
- v = module._dl1._l1.v_with_top_v_key_chains()
- assert "dl1" in v
- assert "l1" in v.dl1
- assert v.dl1.l1 is module._dl1._l1.v
+ v = module._dl1._l1.v_with_top_v_key_chains()
+ assert "dl1" in v
+ assert "l1" in v.dl1
+ assert v.dl1.l1 is module._dl1._l1.v
- # depth 1
+ # depth 1
- v = module._dl0._l0.v_with_top_v_key_chains(depth=1)
- assert "l0" in v
- assert v.l0 is module._dl0._l0.v
+ v = module._dl0._l0.v_with_top_v_key_chains(depth=1)
+ assert "l0" in v
+ assert v.l0 is module._dl0._l0.v
- v = module._dl0._l1.v_with_top_v_key_chains(depth=1)
- assert "l1" in v
- assert v.l1 is module._dl0._l1.v
+ v = module._dl0._l1.v_with_top_v_key_chains(depth=1)
+ assert "l1" in v
+ assert v.l1 is module._dl0._l1.v
- v = module._dl1._l0.v_with_top_v_key_chains(depth=1)
- assert "l0" in v
- assert v.l0 is module._dl1._l0.v
+ v = module._dl1._l0.v_with_top_v_key_chains(depth=1)
+ assert "l0" in v
+ assert v.l0 is module._dl1._l0.v
- v = module._dl1._l1.v_with_top_v_key_chains(depth=1)
- assert "l1" in v
- assert v.l1 is module._dl1._l1.v
+ v = module._dl1._l1.v_with_top_v_key_chains(depth=1)
+ assert "l1" in v
+ assert v.l1 is module._dl1._l1.v
# with custom var structure
@@ -1126,13 +1197,17 @@ def test_v_with_top_v_key_chains(
output_channels=st.integers(min_value=2, max_value=5),
)
def test_with_custom_var_structure(
- batch_shape, input_channels, output_channels, on_device
+ batch_shape, input_channels, output_channels, on_device, backend_fw
):
# smoke test
- if ivy.current_backend_str() == "numpy":
+ if backend_fw == "numpy":
# NumPy does not support gradients
return
- module = WithCustomVarStructure(input_channels, output_channels, device=on_device)
- assert "x" in module.v
- assert "y" in module.v
- assert "z" in module.v
+
+ with ivy.utils.backend.ContextManager(backend_fw):
+ module = WithCustomVarStructure(
+ input_channels, output_channels, device=on_device
+ )
+ assert "x" in module.v
+ assert "y" in module.v
+ assert "z" in module.v
diff --git a/ivy_tests/test_ivy/test_stateful/test_optimizers.py b/ivy_tests/test_ivy/test_stateful/test_optimizers.py
index 9b566531a7e73..f936fb3d526fe 100644
--- a/ivy_tests/test_ivy/test_stateful/test_optimizers.py
+++ b/ivy_tests/test_ivy/test_stateful/test_optimizers.py
@@ -75,6 +75,73 @@ def test_adam_optimizer(
)
+# AdamW
+@handle_method(
+ method_tree="AdamW._step",
+ dtype_x_lr=get_gradient_arguments_with_lr(
+ min_value=1e-05,
+ max_value=1e08,
+ num_arrays=2,
+ float_lr=True,
+ large_abs_safety_factor=2,
+ small_abs_safety_factor=2,
+ ),
+ beta1_n_beta2_n_epsilon=helpers.list_of_size(
+ x=helpers.floats(min_value=1e-1, max_value=1),
+ size=3,
+ ),
+ weight_decay=helpers.floats(min_value=0, max_value=1e-1),
+ inplace=st.booleans(),
+ stop_gradients=st.booleans(),
+ test_gradients=st.just(True),
+)
+def test_adamw_optimizer(
+ dtype_x_lr,
+ beta1_n_beta2_n_epsilon,
+ weight_decay,
+ inplace,
+ stop_gradients,
+ on_device,
+ class_name,
+ method_name,
+ backend_fw,
+ ground_truth_backend,
+ test_gradients,
+ init_flags,
+ method_flags,
+):
+ input_dtype, x, lr = dtype_x_lr
+ beta1, beta2, epsilon = beta1_n_beta2_n_epsilon
+ xs_grad_idxs = [[0, 0]] if method_flags.num_positional_args else [[1, "v"]]
+ helpers.test_method(
+ backend_to_test=backend_fw,
+ ground_truth_backend=ground_truth_backend,
+ init_flags=init_flags,
+ method_flags=method_flags,
+ init_all_as_kwargs_np={
+ "lr": lr,
+ "beta1": beta1,
+ "beta2": beta2,
+ "epsilon": epsilon,
+ "weight_decay": weight_decay,
+ "inplace": inplace,
+ "stop_gradients": stop_gradients,
+ },
+ method_input_dtypes=input_dtype,
+ method_all_as_kwargs_np={
+ "v": x[0],
+ "grads": x[1],
+ },
+ class_name=class_name,
+ method_name=method_name,
+ rtol_=1e-1,
+ atol_=1e-1,
+ test_gradients=test_gradients,
+ xs_grad_idxs=xs_grad_idxs,
+ on_device=on_device,
+ )
+
+
# lamb
@handle_method(
method_tree="LAMB._step",
diff --git a/priority_tests/ivy.txt b/priority_tests/ivy.txt
deleted file mode 100644
index 1089e98d13c33..0000000000000
--- a/priority_tests/ivy.txt
+++ /dev/null
@@ -1,77 +0,0 @@
-ivy/ivy_tests/test_ivy/test_functional/test_core/test_creation.py::test_asarray
-ivy/ivy_tests/test_ivy/test_functional/test_core/test_manipulation.py::test_reshape
-ivy/ivy_tests/test_ivy/test_functional/test_nn/test_layers.py::test_linear
-ivy/ivy_tests/test_ivy/test_functional/test_core/test_manipulation.py::test_permute_dims
-ivy/ivy_tests/test_ivy/test_functional/test_core/test_elementwise.py::test_add
-ivy/ivy_tests/test_ivy/test_functional/test_experimental/test_nn/test_layers.py::test_dropout
-ivy/ivy_tests/test_ivy/test_functional/test_core/test_elementwise.py::test_multiply
-ivy/ivy_tests/test_ivy/test_functional/test_nn/test_layers.py::test_conv
-ivy/ivy_tests/test_ivy/test_functional/test_core/test_manipulation.py::test_swapaxes
-ivy/ivy_tests/test_ivy/test_functional/test_core/test_general.py::test_array
-ivy/ivy_tests/test_ivy/test_functional/test_nn/test_norms.py::test_layer_norm
-ivy/ivy_tests/test_ivy/test_functional/test_core/test_linalg.py::test_matmul
-ivy/ivy_tests/test_ivy/test_misc/test_array.py::test_array__getitem__
-ivy/ivy_tests/test_ivy/test_misc/test_array.py::test_array__setitem__
-ivy/ivy_tests/test_ivy/test_functional/test_experimental/test_nn/test_norms.py::test_batch_norm
-ivy/ivy_tests/test_ivy/test_functional/test_nn/test_activations.py::test_gelu
-ivy/ivy_tests/test_ivy/test_functional/test_nn/test_activations.py::test_softmax
-ivy/ivy_tests/test_ivy/test_functional/test_core/test_elementwise.py::test_divide
-ivy/ivy_tests/test_ivy/test_functional/test_experimental/test_nn/test_activations.py::test_relu
-ivy/ivy_tests/test_ivy/test_functional/test_nn/test_activations.py::test_sigmoid
-ivy/ivy_tests/test_ivy/test_misc/test_array.py::test_array__iadd__
-ivy/ivy_tests/test_ivy/test_functional/test_core/test_general.py::test_get_item
-ivy/ivy_tests/test_ivy/test_functional/test_core/test_searching.py::test_where
-ivy/ivy_tests/test_ivy/test_functional/test_core/test_dtype.py::test_astype
-ivy/ivy_tests/test_ivy/test_functional/test_core/test_elementwise.py::test_pow
-ivy/ivy_tests/test_ivy/test_functional/test_experimental/test_core/test_manipulation.py::test_flatten
-ivy/ivy_tests/test_ivy/test_functional/test_experimental/test_core/test_manipulation.py::test_pad
-ivy/ivy_tests/test_ivy/test_functional/test_experimental/test_nn/test_norms.py::test_group_norm
-ivy/ivy_tests/test_ivy/test_functional/test_core/test_statistical.py::test_mean
-ivy/ivy_tests/test_ivy/test_functional/test_core/test_manipulation.py::test_expand_dims
-ivy/ivy_tests/test_ivy/test_functional/test_experimental/test_nn/test_layers.py::test_adaptive_avg_pool2d
-ivy/ivy_tests/test_ivy/test_functional/test_core/test_general.py::test_inplace_update
-ivy/ivy_tests/test_ivy/test_misc/test_array.py::test_array__mul__
-ivy/ivy_tests/test_ivy/test_functional/test_core/test_elementwise.py::test_subtract
-ivy/ivy_tests/test_ivy/test_functional/test_core/test_manipulation.py::test_concat
-ivy/ivy_tests/test_ivy/test_functional/test_core/test_elementwise.py::test_less
-ivy/ivy_tests/test_ivy/test_functional/test_core/test_device.py::test_split
-ivy/ivy_tests/test_ivy/test_functional/test_core/test_elementwise.py::test_greater
-ivy/ivy_tests/test_ivy/test_functional/test_core/test_elementwise.py::test_sqrt
-ivy/ivy_tests/test_ivy/test_functional/test_core/test_elementwise.py::test_tanh
-ivy/ivy_tests/test_ivy/test_functional/test_core/test_statistical.py::test_sum
-ivy/ivy_tests/test_ivy/test_functional/test_core/test_manipulation.py::test_roll
-ivy/ivy_tests/test_ivy/test_functional/test_core/test_elementwise.py::test_reciprocal
-ivy/ivy_tests/test_ivy/test_functional/test_experimental/test_nn/test_layers.py::test_embedding
-ivy/ivy_tests/test_ivy/test_functional/test_core/test_manipulation.py::test_expand
-ivy/ivy_tests/test_ivy/test_functional/test_core/test_elementwise.py::test_abs
-ivy/ivy_tests/test_ivy/test_functional/test_core/test_elementwise.py::test_maximum
-ivy/ivy_tests/test_ivy/test_functional/test_core/test_creation.py::test_zeros
-ivy/ivy_tests/test_ivy/test_functional/test_core/test_elementwise.py::test_equal
-ivy/ivy_tests/test_ivy/test_functional/test_core/test_statistical.py::test_einsum
-ivy/ivy_tests/test_ivy/test_functional/test_experimental/test_nn/test_activations.py::test_relu6
-ivy/ivy_tests/test_ivy/test_functional/test_core/test_elementwise.py::test_not_equal
-ivy/ivy_tests/test_ivy/test_functional/test_core/test_linalg.py::test_vector_norm
-ivy/ivy_tests/test_ivy/test_functional/test_core/test_creation.py::test_arange
-ivy/ivy_tests/test_ivy/test_functional/test_experimental/test_nn/test_layers.py::test_interpolate
-ivy/ivy_tests/test_ivy/test_functional/test_core/test_general.py::test_shape
-ivy/ivy_tests/test_ivy/test_functional/test_core/test_elementwise.py::test_negative
-ivy/ivy_tests/test_ivy/test_functional/test_core/test_elementwise.py::test_cos
-ivy/ivy_tests/test_ivy/test_functional/test_core/test_elementwise.py::test_sin
-ivy/ivy_tests/test_ivy/test_functional/test_core/test_manipulation.py::test_zero_pad
-ivy/ivy_tests/test_ivy/test_functional/test_core/test_linalg.py::test_outer
-ivy/ivy_tests/test_ivy/test_functional/test_core/test_elementwise.py::test_erf
-ivy/ivy_tests/test_ivy/test_functional/test_core/test_manipulation.py::test_tile
-ivy/ivy_tests/test_ivy/test_functional/test_core/test_creation.py::test_full
-ivy/ivy_tests/test_ivy/test_functional/test_core/test_statistical.py::test_cumsum
-ivy/ivy_tests/test_ivy/test_functional/test_experimental/test_nn/test_layers.py::test_max_pool2d
-ivy/ivy_tests/test_ivy/test_functional/test_experimental/test_nn/test_layers.py::test_adaptive_avg_pool1d
-ivy/ivy_tests/test_ivy/test_functional/test_core/test_creation.py::test_ones
-ivy/ivy_tests/test_ivy/test_functional/test_core/test_elementwise.py::test_minimum
-ivy/ivy_tests/test_ivy/test_functional/test_core/test_creation.py::test_log
-ivy/ivy_tests/test_ivy/test_functional/test_core/test_creation.py::test_full_like
-ivy/ivy_tests/test_ivy/test_functional/test_core/test_creation.py::test_zeros_like
-ivy/ivy_tests/test_ivy/test_functional/test_core/test_manipulation.py::test_stack
-ivy/ivy_tests/test_ivy/test_functional/test_experimental/test_nn/test_layers.py::test_avg_pool2d
-ivy/ivy_tests/test_ivy/test_functional/test_core/test_elementwise.py::test_less_equal
-ivy/ivy_tests/test_ivy/test_functional/test_core/test_searching.py::test_argmax
-ivy/ivy_tests/test_ivy/test_functional/test_core/test_elementwise.py::test_bitwise_invert
diff --git a/priority_tests/torch.txt b/priority_tests/torch.txt
deleted file mode 100644
index 7d49d9d583c85..0000000000000
--- a/priority_tests/torch.txt
+++ /dev/null
@@ -1,101 +0,0 @@
-ivy/ivy_tests/test_ivy/test_frontends/test_torch/test_nn/test_functional/test_linear_functions.py::test_torch_linear
-ivy/ivy_tests/test_ivy/test_frontends/test_torch/test_miscellaneous_ops.py::test_torch_view
-ivy/ivy_tests/test_ivy/test_frontends/test_torch/test_tensor.py::test_torch___add__
-ivy/ivy_tests/test_ivy/test_frontends/test_torch/test_indexing_slicing_joining_mutating_ops.py::test_torch_permute
-ivy/ivy_tests/test_ivy/test_frontends/test_torch/test_tensor.py::test_torch_size
-ivy/ivy_tests/test_ivy/test_frontends/test_torch/test_nn/test_functional/test_dropout_functions.py::test_torch_dropout
-ivy/ivy_tests/test_ivy/test_frontends/test_torch/test_nn/test_functional/test_convolution_functions.py::test_torch_conv2d
-ivy/ivy_tests/test_ivy/test_frontends/test_torch/test_indexing_slicing_joining_mutating_ops.py::test_torch_transpose
-ivy/ivy_tests/test_ivy/test_frontends/test_torch/test_nn/test_functional/test_norms.py::test_torch_layer_norm
-ivy/ivy_tests/test_ivy/test_frontends/test_torch/test_tensor.py::test_torch___mul__
-ivy/ivy_tests/test_ivy/test_frontends/test_torch/test_tensor.py::test_torch_contiguous
-ivy/ivy_tests/test_ivy/test_frontends/test_torch/test_blas_and_lapack_ops.py::test_torch_matmul
-ivy/ivy_tests/test_ivy/test_frontends/test_torch/test_nn/test_functional/test_norms.py::test_torch_batch_norm
-ivy/ivy_tests/test_ivy/test_frontends/test_torch/test_nn/test_functional/test_non_linear_activation_functions.py::test_torch_gelu
-ivy/ivy_tests/test_ivy/test_frontends/test_torch/test_nn/test_functional/test_non_linear_activation_functions.py::test_torch_softmax
-ivy/ivy_tests/test_ivy/test_frontends/test_torch/test_indexing_slicing_joining_mutating_ops.py::test_torch_reshape
-ivy/ivy_tests/test_ivy/test_frontends/test_torch/test_nn/test_functional/test_non_linear_activation_functions.py::test_torch_relu
-ivy/ivy_tests/test_ivy/test_frontends/test_torch/test_tensor.py::test_torch___truediv__
-ivy/ivy_tests/test_ivy/test_frontends/test_torch/test_nn/test_functional/test_non_linear_activation_functions.py::test_torch_silu
-ivy/ivy_tests/test_ivy/test_frontends/test_torch/test_blas_and_lapack_ops.py::test_torch_bmm
-ivy/ivy_tests/test_ivy/test_frontends/test_torch/test_tensor.py::test_torch___rmul__
-ivy/ivy_tests/test_ivy/test_frontends/test_torch/test_miscellaneous_ops.py::test_torch_flatten
-ivy/ivy_tests/test_ivy/test_frontends/test_torch/test_nn/test_functional/test_vision_functions.py::test_torch_pad
-ivy/ivy_tests/test_ivy/test_frontends/test_torch/test_nn/test_functional/test_norms.py::test_torch_group_norm
-ivy/ivy_tests/test_ivy/test_frontends/test_torch/test_comparison_ops.py::test_torch_to
-ivy/ivy_tests/test_ivy/test_frontends/test_torch/test_indexing_slicing_joining_mutating_ops.py::test_torch_unsqueeze
-ivy/ivy_tests/test_ivy/test_frontends/test_torch/test_reduction_ops.py::test_torch_mean
-ivy/ivy_tests/test_ivy/test_frontends/test_torch/test_nn/test_functional/test_non_linear_activation_functions.py::test_torch_sigmoid
-ivy/ivy_tests/test_ivy/test_frontends/test_torch/test_nn/test_functional/test_pooling_functions.py::test_torch_adaptive_avg_pool2d
-ivy/ivy_tests/test_ivy/test_frontends/test_torch/test_indexing_slicing_joining_mutating_ops.py::test_torch_cat
-ivy/ivy_tests/test_ivy/test_frontends/test_torch/test_nn/test_functional/test_non_linear_activation_functions.py::test_torch_hardtanh
-ivy/ivy_tests/test_ivy/test_frontends/test_torch/test_tensor.py::test_torch___radd__
-ivy/ivy_tests/test_ivy/test_frontends/test_torch/test_pointwise_ops.py::test_torch_pow
-ivy/ivy_tests/test_ivy/test_frontends/test_torch/test_tensor.py::test_torch___sub__
-ivy/ivy_tests/test_ivy/test_frontends/test_torch/test_linalg.py::test_torch_mul
-ivy/ivy_tests/test_ivy/test_frontends/test_torch/test_pointwise_ops.py::test_torch_masked_fill
-ivy/ivy_tests/test_ivy/test_frontends/test_torch/test_nn/test_functional/test_non_linear_activation_functions.py::test_torch_tanh
-ivy/ivy_tests/test_ivy/test_frontends/test_torch/test_blas_and_lapack_ops.py::test_torch_addmm
-ivy/ivy_tests/test_ivy/test_frontends/test_torch/test_tensor.py::test_torch_reshape_as
-ivy/ivy_tests/test_ivy/test_frontends/test_torch/test_miscellaneous_ops.py::test_torch_roll
-ivy/ivy_tests/test_ivy/test_frontends/test_torch/test_pointwise_ops.py::test_torch_float
-ivy/ivy_tests/test_ivy/test_frontends/test_torch/test_pointwise_ops.py::test_torch_rsqrt
-ivy/ivy_tests/test_ivy/test_frontends/test_torch/test_nn/test_functional/test_sparse_functions.py::test_torch_embedding
-ivy/ivy_tests/test_ivy/test_frontends/test_torch/test_pointwise_ops.py::test_torch_pow
-ivy/ivy_tests/test_ivy/test_frontends/test_torch/test_tensor.py::test_torch___matmul__
-ivy/ivy_tests/test_ivy/test_frontends/test_torch/test_indexing_slicing_joining_mutating_ops.py::test_torch_split
-ivy/ivy_tests/test_ivy/test_frontends/test_torch/test_nn/test_functional/test_non_linear_activation_functions.py::test_torch_normalize
-ivy/ivy_tests/test_ivy/test_frontends/test_torch/test_tensor.py::test_torch_type_as
-ivy/ivy_tests/test_ivy/test_frontends/test_torch/test_tensor.py::test_torch___eq__
-ivy/ivy_tests/test_ivy/test_frontends/test_torch/test_tensor.py::test_torch___rsub__
-ivy/ivy_tests/test_ivy/test_frontends/test_torch/test_miscellaneous_ops.py::test_torch_einsum
-ivy/ivy_tests/test_ivy/test_frontends/test_torch/test_nn/test_functional/test_non_linear_activation_functions.py::test_torch_hardswish
-ivy/ivy_tests/test_ivy/test_frontends/test_torch/test_tensor.py::test_torch_expand
-ivy/ivy_tests/test_ivy/test_frontends/test_torch/test_creation_ops.py::test_torch_zeros
-ivy/ivy_tests/test_ivy/test_frontends/test_torch/test_indexing_slicing_joining_mutating_ops.py::test_torch_split
-ivy/ivy_tests/test_ivy/test_frontends/test_torch/test_tensor.py::test_torch___ne__
-ivy/ivy_tests/test_ivy/test_frontends/test_torch/test_creation_ops.py::test_torch_arange
-ivy/ivy_tests/test_ivy/test_frontends/test_torch/test_nn/test_functional/test_vision_functions.py::test_torch_interpolate
-ivy/ivy_tests/test_ivy/test_frontends/test_torch/test_nn/test_functional/test_non_linear_activation_functions.py::test_torch_norm
-ivy/ivy_tests/test_ivy/test_frontends/test_torch/test_pointwise_ops.py::test_torch_sqrt
-ivy/ivy_tests/test_ivy/test_frontends/test_torch/test_tensor.py::test_torch_expand_as
-ivy/ivy_tests/test_ivy/test_frontends/test_torch/test_nn/test_functional/test_non_linear_activation_functions.py::test_torch_softmax
-ivy/ivy_tests/test_ivy/test_frontends/test_torch/test_reduction_ops.py::test_torch_sum
-ivy/ivy_tests/test_ivy/test_frontends/test_torch/test_pointwise_ops.py::test_torch_neg
-ivy/ivy_tests/test_ivy/test_frontends/test_torch/test_indexing_slicing_joining_mutating_ops.py::test_torch_chunk
-ivy/ivy_tests/test_ivy/test_frontends/test_torch/test_nn/test_functional/test_convolution_functions.py::test_torch_conv1d
-ivy/ivy_tests/test_ivy/test_frontends/test_torch/test_nn/test_functional/test_distance_functions.py::test_torch_cos
-ivy/ivy_tests/test_ivy/test_frontends/test_torch/test_pointwise_ops.py::test_torch_sin
-ivy/ivy_tests/test_ivy/test_frontends/test_torch/test_tensor.py::test_torch___lt__
-ivy/ivy_tests/test_ivy/test_frontends/test_torch/test_blas_and_lapack_ops.py::test_torch_outer
-ivy/ivy_tests/test_ivy/test_frontends/test_torch/test_pointwise_ops.py::test_torch_erf
-ivy/ivy_tests/test_ivy/test_frontends/test_torch/test_miscellaneous_ops.py::test_torch_repeat
-ivy/ivy_tests/test_ivy/test_frontends/test_torch/test_miscellaneous_ops.py::test_torch_flatten
-ivy/ivy_tests/test_ivy/test_frontends/test_torch/test_creation_ops.py::test_torch_full
-ivy/ivy_tests/test_ivy/test_frontends/test_torch/test_nn/test_functional/test_pooling_functions.py::test_torch_max_pool2d
-ivy/ivy_tests/test_ivy/test_frontends/test_torch/test_nn/test_functional/test_convolution_functions.py::test_torch_unfold
-ivy/ivy_tests/test_ivy/test_frontends/test_torch/test_nn/test_functional/test_convolution_functions.py::test_torch_fold
-ivy/ivy_tests/test_ivy/test_frontends/test_torch/test_nn/test_functional/test_pooling_functions.py::test_torch_adaptive_avg_pool1d
-ivy/ivy_tests/test_ivy/test_frontends/test_torch/test_creation_ops.py::test_torch_ones
-ivy/ivy_tests/test_ivy/test_frontends/test_torch/test_comparison_ops.py::test_torch_min
-ivy/ivy_tests/test_ivy/test_frontends/test_torch/test_reduction_ops.py::test_torch_mean
-ivy/ivy_tests/test_ivy/test_frontends/test_torch/test_pointwise_ops.py::test_torch_ne
-ivy/ivy_tests/test_ivy/test_frontends/test_torch/test_nn/test_functional/test_vision_functions.py::test_torch_int
-ivy/ivy_tests/test_ivy/test_frontends/test_torch/test_miscellaneous_ops.py::test_torch_cumsum
-ivy/ivy_tests/test_ivy/test_frontends/test_torch/test_tensor.py::test_torch_long
-ivy/ivy_tests/test_ivy/test_frontends/test_torch/test_reduction_ops.py::test_torch_sum
-ivy/ivy_tests/test_ivy/test_frontends/test_torch/test_blas_and_lapack_ops.py::test_torch_log
-ivy/ivy_tests/test_ivy/test_frontends/test_torch/test_creation_ops.py::test_torch_full_like
-ivy/ivy_tests/test_ivy/test_frontends/test_torch/test_indexing_slicing_joining_mutating_ops.py::test_torch_where
-ivy/ivy_tests/test_ivy/test_frontends/test_torch/test_creation_ops.py::test_torch_zeros_like
-ivy/ivy_tests/test_ivy/test_frontends/test_torch/test_nn/test_functional/test_non_linear_activation_functions.py::test_torch_norm
-ivy/ivy_tests/test_ivy/test_frontends/test_torch/test_blas_and_lapack_ops.py::test_torch_t
-ivy/ivy_tests/test_ivy/test_frontends/test_torch/test_miscellaneous_ops.py::test_torch_cumsum
-ivy/ivy_tests/test_ivy/test_frontends/test_torch/test_indexing_slicing_joining_mutating_ops.py::test_torch_stack
-ivy/ivy_tests/test_ivy/test_frontends/test_torch/test_nn/test_functional/test_pooling_functions.py::test_torch_avg_pool2d
-ivy/ivy_tests/test_ivy/test_frontends/test_torch/test_tensor.py::test_torch_new_zeros
-ivy/ivy_tests/test_ivy/test_frontends/test_torch/test_miscellaneous_ops.py::test_torch_clone
-ivy/ivy_tests/test_ivy/test_frontends/test_torch/test_tensor.py::test_torch___gt__
-ivy/ivy_tests/test_ivy/test_frontends/test_torch/test_pointwise_ops.py::test_torch_abs
-ivy/ivy_tests/test_ivy/test_frontends/test_torch/test_reduction_ops.py::test_torch_argmax
-ivy/ivy_tests/test_ivy/test_frontends/test_torch/test_tensor.py::test_torch___invert__
diff --git a/project.toml b/project.toml
index 374b58cbf4636..f41dc8bb153c2 100644
--- a/project.toml
+++ b/project.toml
@@ -1,6 +1,7 @@
[build-system]
requires = [
"setuptools>=42",
- "wheel"
+ "wheel",
+ "pip"
]
build-backend = "setuptools.build_meta"
diff --git a/requirements/optional.txt b/requirements/optional.txt
index d142483f67d23..e34585650624e 100644
--- a/requirements/optional.txt
+++ b/requirements/optional.txt
@@ -10,7 +10,6 @@ jax[cpu] # unpinned, we test for latest version now, mod_name=jax
jaxlib # unpinned, we test for latest version now
paddlepaddle # unpinned , mod_name=paddle
tensorflow-cpu # unpinned, we test for latest version now, mod_name=tensorflow
-tensorflow-probability # unpinned, we test for latest version now, mod_name=tensorflow_probability
torch # unpinned, we test for latest version now
# torch-scatter # unpinned, mod_name=torch_scatter
functorch # unpinned, we test for latest version now
@@ -18,7 +17,6 @@ scipy # unpinned
dm-haiku # unpinned mod_name=haiku
flax
pydriller
-tqdm
coverage
scikit-learn # mod_name=sklearn
pandas
diff --git a/requirements/optional_apple_silicon_2.txt b/requirements/optional_apple_silicon_2.txt
index 42a07692d8343..7a3325ede80c3 100644
--- a/requirements/optional_apple_silicon_2.txt
+++ b/requirements/optional_apple_silicon_2.txt
@@ -5,7 +5,6 @@ dm-haiku # mod_name=haiku
flax
protobuf
pydriller
-tqdm
coverage
scikit-learn # mod_name=sklearn
pandas
diff --git a/requirements/optional_apple_silicon_gpu_2.txt b/requirements/optional_apple_silicon_gpu_2.txt
index 42a07692d8343..7a3325ede80c3 100644
--- a/requirements/optional_apple_silicon_gpu_2.txt
+++ b/requirements/optional_apple_silicon_gpu_2.txt
@@ -5,7 +5,6 @@ dm-haiku # mod_name=haiku
flax
protobuf
pydriller
-tqdm
coverage
scikit-learn # mod_name=sklearn
pandas
diff --git a/requirements/optional_gpu.txt b/requirements/optional_gpu.txt
index 84635358d95e8..0810e836b9ba1 100644
--- a/requirements/optional_gpu.txt
+++ b/requirements/optional_gpu.txt
@@ -9,7 +9,6 @@ opencv-python # unpinned , mod_name=cv2
jax # unpinned, we test for latest version now
jaxlib # unpinned, we test for latest version now
tensorflow # unpinned, we test for latest version now
-tensorflow-probability # unpinned, we test for latest version now, mod_name=tensorflow_probability
torch # unpinned, we test for latest version now
torch-scatter # unpinned, mod_name=torch_scatter
functorch # unpinned, we test for latest version now
@@ -17,7 +16,6 @@ scipy # unpinned
dm-haiku # unpinned mod_name=haiku
flax
pydriller
-tqdm
coverage
scikit-learn # mod_name=sklearn
pandas
diff --git a/requirements/requirements.txt b/requirements/requirements.txt
index b1cbd4c78209c..375a11f7bbeaa 100644
--- a/requirements/requirements.txt
+++ b/requirements/requirements.txt
@@ -13,3 +13,6 @@ pyvis
dill
astunparse
ml-dtypes # mod_name=ml_dtypes
+cloudpickle
+gast
+tqdm
diff --git a/run_tests.py b/run_tests.py
deleted file mode 100644
index 2be91b96115b7..0000000000000
--- a/run_tests.py
+++ /dev/null
@@ -1,224 +0,0 @@
-# Run Tests
-import os
-import sys
-from pymongo import MongoClient
-import requests
-from run_tests_CLI.get_all_tests import BACKENDS
-
-
-submodules = (
- "test_paddle",
- "test_tensorflow",
- "test_torch",
- "test_jax",
- "test_numpy",
- "test_functional",
- "test_experimental",
- "test_stateful",
- "test_misc",
- "test_scipy",
- "test_pandas",
- "test_mindspore",
- "test_onnx",
- "test_sklearn",
- "test_xgboost",
-)
-db_dict = {
- "test_functional/test_core": ["core", 10],
- "test_experimental/test_core": ["exp_core", 11],
- "test_functional/test_nn": ["nn", 12],
- "test_experimental/test_nn": ["exp_nn", 13],
- "test_stateful": ["stateful", 14],
- "test_torch": ["torch", 15],
- "test_jax": ["jax", 16],
- "test_tensorflow": ["tensorflow", 17],
- "test_numpy": ["numpy", 18],
- "test_misc": ["misc", 19],
- "test_paddle": ["paddle", 20],
- "test_scipy": ["scipy", 21],
- "test_pandas": ["pandas", 22],
- "test_mindspore": ["mindspore", 23],
- "test_onnx": ["onnx", 24],
- "test_sklearn": ["sklearn", 25],
- "test_xgboost": ["xgboost", 26],
-}
-result_config = {
- "success": "https://img.shields.io/badge/-success-success",
- "failure": "https://img.shields.io/badge/-failure-red",
-}
-
-
-def get_latest_package_version(package_name):
- try:
- url = f"https://pypi.org/pypi/{package_name}/json"
- response = requests.get(url)
- response.raise_for_status()
- package_info = response.json()
- return package_info["info"]["version"]
- except requests.exceptions.RequestException:
- print(f"Error: Failed to fetch package information for {package_name}.")
- return None
-
-
-def make_clickable(url, name):
- return (
- f'
'
- )
-
-
-def get_submodule(test_path):
- test_path = test_path.split("/")
- for name in submodules:
- if name in test_path:
- if name == "test_functional":
- if len(test_path) > 3 and test_path[3] == "test_experimental":
- coll = db_dict[f"test_experimental/{test_path[4]}"]
- else:
- coll = db_dict[f"test_functional/{test_path[-2]}"]
- else:
- coll = db_dict[name]
- break
- submod_test = test_path[-1]
- submod, test_fn = submod_test.split("::")
- submod = submod.replace("test_", "").replace(".py", "")
- return coll, submod, test_fn
-
-
-def update_individual_test_results(
- collection,
- id,
- submod,
- backend,
- test,
- result,
- backend_version=None,
- frontend_version=None,
- device=None,
-):
- key = f"{submod}.{backend}"
- if backend_version is not None:
- backend_version = backend_version.replace(".", "_")
- key += f".{backend_version}"
- if frontend_version is not None:
- frontend_version = frontend_version.replace(".", "_")
- key += f".{frontend_version}"
- key += f".{test}"
- if device:
- key += f".{device}"
- collection.update_one(
- {"_id": id},
- {"$set": {key: result}},
- upsert=True,
- )
-
-
-if __name__ == "__main__":
- redis_url = sys.argv[1]
- redis_pass = sys.argv[2]
- mongo_key = sys.argv[3]
- version_flag = sys.argv[4]
- gpu_flag = sys.argv[5]
- workflow_id = sys.argv[6]
- priority_flag = sys.argv[7]
- if len(sys.argv) > 8 and sys.argv[8] != "null":
- run_id = sys.argv[8]
- else:
- run_id = f"https://github.com/unifyai/ivy/actions/runs/{workflow_id}"
- failed = False
- # GPU Testing
- with_gpu = False
- if gpu_flag == "true":
- with_gpu = True
- if priority_flag == "true":
- priority_flag = True
- else:
- priority_flag = False
- cluster = MongoClient(
- f"mongodb+srv://deep-ivy:{mongo_key}@cluster0.qdvf8q3.mongodb.net/?retryWrites=true&w=majority" # noqa
- )
- db = cluster["Ivy_tests_multi_gpu"]
- db_priority = cluster["Ivy_tests_priority"]
- if with_gpu:
- os.system("docker pull unifyai/multicuda:base_and_requirements")
- with open("tests_to_run") as f:
- for line in f:
- test, backend = line.split(",")
- coll, submod, test_fn = get_submodule(test)
- print(f"\n{'*' * 100}")
- print(f"{line[:-1]}")
- print(f"{'*' * 100}\n")
- backend_version = "latest-stable"
- sys.stdout.flush()
- if version_flag == "true":
- backends = [backend.strip()]
- [backend_name, backend_version] = backend.split("/")
- other_backends = [
- fw for fw in BACKENDS if (fw != backend_name and fw != "paddle")
- ]
- for backend in other_backends:
- backends.append(backend + "/" + get_latest_package_version(backend))
- print("Backends:", backends)
- ret = os.system(
- f"docker run --rm --env REDIS_URL={redis_url} --env"
- f' REDIS_PASSWD={redis_pass} -v "$(pwd)":/ivy -v'
- ' "$(pwd)"/.hypothesis:/.hypothesis unifyai/multiversion:latest'
- ' /bin/bash -c "cd docker;python'
- f" multiversion_framework_directory.py {' '.join(backends)};cd"
- f' ..;pytest --tb=short {test} --backend={backend}"'
- )
- else:
- if with_gpu:
- ret = os.system(
- f"docker run --rm --gpus all --env REDIS_URL={redis_url} --env"
- f' REDIS_PASSWD={redis_pass} -v "$(pwd)":/ivy -v'
- ' "$(pwd)"/.hypothesis:/.hypothesis'
- " unifyai/multicuda:base_and_requirements python3 -m pytest"
- f" --tb=short {test} --device=gpu:0 -B={backend}"
- # noqa
- )
- else:
- ret = os.system(
- f"docker run --rm --env REDIS_URL={redis_url} --env"
- f' REDIS_PASSWD={redis_pass} -v "$(pwd)":/ivy -v'
- ' "$(pwd)"/.hypothesis:/.hypothesis unifyai/ivy:latest python3'
- f" -m pytest --tb=short {test} --backend {backend}"
- # noqa
- )
- if ret != 0:
- res = make_clickable(run_id, result_config["failure"])
- failed = True
- else:
- res = make_clickable(run_id, result_config["success"])
- frontend_version = None
- if coll[0] in ["numpy", "jax", "tensorflow", "torch", "paddle"]:
- frontend_version = "latest-stable"
- if priority_flag:
- print("Updating Priority DB")
- update_individual_test_results(
- db_priority[coll[0]],
- coll[1],
- submod,
- backend,
- test_fn,
- res,
- "latest-stable",
- frontend_version,
- "gpu" if with_gpu else "cpu",
- )
- else:
- print(backend_version)
- update_individual_test_results(
- db[coll[0]],
- coll[1],
- submod,
- backend,
- test_fn,
- res,
- backend_version,
- frontend_version,
- "gpu" if with_gpu else "cpu",
- )
-
- if failed:
- exit(1)
diff --git a/run_tests_CLI/array_api_run_tests_pr.py b/run_tests_CLI/array_api_run_tests_pr.py
deleted file mode 100644
index 1852c0a936267..0000000000000
--- a/run_tests_CLI/array_api_run_tests_pr.py
+++ /dev/null
@@ -1,41 +0,0 @@
-# Run Array API Tests for PRs
-import os
-import subprocess
-import sys
-
-BACKENDS = ["numpy", "jax", "tensorflow", "torch"]
-
-
-def main():
- failed = False
- k_flag = {}
- subprocess.run(
- ["python3", "ivy_tests/array_api_testing/write_array_api_tests_k_flag.py"],
- check=True,
- )
- for backend in BACKENDS:
- k_flag_file = f"ivy_tests/array_api_testing/.array_api_tests_k_flag_{backend}"
- with open(k_flag_file) as f:
- array_api_tests_k_flag = f.read().strip()
- if backend == "torch":
- array_api_tests_k_flag += " and not (uint16 or uint32 or uint64)"
- k_flag[backend] = array_api_tests_k_flag
-
- with open("tests_to_run") as f:
- for line in f:
- test, backend = line.split(",")
- backend = backend.strip("\n")
- command = f'docker run --rm --env IVY_BACKEND={backend} --env ARRAY_API_TESTS_MODULE="ivy" -v "$(pwd)":/ivy -v "$(pwd)"/.hypothesis:/.hypothesis unifyai/ivy:latest timeout 30m python3 -m pytest {test} -k "{k_flag[backend]}" --tb=short -vv' # noqa
- print(f"\n{'*' * 100}")
- print(f"{line[:-1]}")
- print(f"{'*' * 100}\n")
- sys.stdout.flush()
- ret = os.system(command)
- if ret != 0:
- failed = True
- if failed:
- exit(1)
-
-
-if __name__ == "__main__":
- main()
diff --git a/run_tests_CLI/cron_tests_multi_version.py b/run_tests_CLI/cron_tests_multi_version.py
deleted file mode 100644
index a3ad33eaa3f74..0000000000000
--- a/run_tests_CLI/cron_tests_multi_version.py
+++ /dev/null
@@ -1,109 +0,0 @@
-import os
-import sys
-
-# BACKENDS = ["numpy", "jax", "tensorflow", "torch"]
-torch_req = [
- # "torch/1.4.0",
- # "torch/1.5.0",
- # "torch/1.10.1",
- # "torch/1.10.2",
- # "torch/2.0.1",
- # "torch/1.12.0",
- "torch/1.12.1",
- "torch/1.13.0",
-]
-tensorflow_req = [
- # "tensorflow/2.2.0",
- # "tensorflow/2.2.1",
- # "tensorflow/2.2.2",
- # "tensorflow/2.4.4",
- # "tensorflow/2.9.0",
- # "tensorflow/2.12.0",
- "tensorflow/2.12.0",
- "tensorflow/2.9.2",
-]
-jax_only_req = [
- # "jax/0.1.60",
- # "jax/0.1.61",
- # "jax/0.3.10",
- # "jax/0.3.13",
- # "jax/0.4.10",
- # "jax/0.4.10",
- # "jax/0.3.15",
- "jax/0.3.16",
- "jax/0.3.17",
-]
-jaxlib_req = [
- # "jaxlib/0.1.50",
- # "jaxlib/0.1.60",
- # "jaxlib/0.1.61",
- # "jaxlib/0.3.10",
- # "jaxlib/0.4.10",
- # "jaxlib/0.3.15",
- "jaxlib/0.3.20",
- "jaxlib/0.3.22",
-]
-numpy_req = [
- # "numpy/1.17.3",
- # "numpy/1.17.4",
- # "numpy/1.23.1",
- # "numpy/1.24.0",
- "numpy/1.24.1",
- "numpy/1.24.2",
-]
-jax_req = [
- f"{jax_ver}/{jaxlib_ver}" for jax_ver in jax_only_req for jaxlib_ver in jaxlib_req
-]
-
-framework_versions = {
- "numpy": numpy_req,
- "torch": torch_req,
- "jax": jax_req,
- "tensorflow": tensorflow_req,
-}
-
-run_iter = int(sys.argv[1])
-os.system(
- "docker run -v `pwd`:/ivy -v `pwd`/.hypothesis:/.hypothesis unifyai/ivy:latest python3 -m pytest --disable-pytest-warnings ivy_tests/test_ivy --my_test_dump true > test_names" # noqa
-)
-test_names_without_backend = []
-test_names = []
-with open("test_names") as f:
- for line in f:
- if "ERROR" in line:
- break
- if not line.startswith("ivy_tests"):
- continue
- test_name = line[:-1]
- pos = test_name.find("[")
- if pos != -1:
- test_name = test_name[:pos]
- test_names_without_backend.append(test_name)
-
-for test_name in test_names_without_backend:
- for backend_versions in framework_versions.values():
- for backend_version in backend_versions:
- test_backend = f"{test_name},{backend_version}"
- if "test_frontends" in test_name:
- frontend = test_name[39:]
- frontend = frontend[: frontend.find("/")]
- frontend_versions = framework_versions.get(frontend, [])
- for frontend_version in frontend_versions:
- test_names.append(f"{test_backend};{frontend_version}")
- else:
- test_names.append(test_backend)
-
-test_names = sorted(set(test_names))
-# Run 150 tests in each iteration of the cron job
-num_tests = len(test_names)
-print(num_tests)
-tests_per_run = 150
-start = run_iter * tests_per_run
-end = (run_iter + 1) * tests_per_run
-print("Running Tests:")
-with open("tests_to_run", "w") as f:
- for i in range(start, end):
- i = i % num_tests
- test = test_names[i]
- print(test)
- f.write(test + "\n")
diff --git a/run_tests_CLI/setup_priority_tests.py b/run_tests_CLI/setup_priority_tests.py
deleted file mode 100644
index 1b0bd932cbf37..0000000000000
--- a/run_tests_CLI/setup_priority_tests.py
+++ /dev/null
@@ -1,17 +0,0 @@
-import sys
-from get_all_tests import BACKENDS
-
-
-def main():
- with open("tests_to_run", "w") as write_file:
- with open(sys.argv[1]) as f:
- for test in f:
- test = test.strip()
- if test.startswith("ivy/"):
- test = test[4:]
- for backend in BACKENDS:
- write_file.write(f"{test},{backend}\n")
-
-
-if __name__ == "__main__":
- main()
diff --git a/scripts/backend_generation/generate.py b/scripts/backend_generation/generate.py
index c68757a53b8d4..152bd5801cdd5 100644
--- a/scripts/backend_generation/generate.py
+++ b/scripts/backend_generation/generate.py
@@ -94,7 +94,7 @@ def _get_user_input(fn, *args, **kwargs):
break
except KeyboardInterrupt:
print("Aborted.")
- exit()
+ sys.exit()
def _update_native_config_value(key):
@@ -102,14 +102,14 @@ def _update_native_config_value(key):
ret = input(
"\nPress ENTER to skip, use full namespace\n"
f"Enter a value for {Style.BRIGHT + key + Style.NORMAL} "
- "(case sensistive) "
+ "(case sensitive) "
f"default: '{Style.BRIGHT}{config_natives[key]['name']}{Style.NORMAL}': "
)
if ret != "" and _imported_backend is not None:
parsed = ret.strip().rpartition(".")
try:
if parsed[1] == "":
- # Primitve type
+ # primitive type
try:
obj = __builtins__.__dict__[parsed[-1]]
except KeyError:
@@ -264,7 +264,7 @@ def _update_valid_config_value(key):
print(f"Select items to remove from list {Style.BRIGHT}{key}:\n")
for i, item in enumerate(config_valids[key]):
print(f"{i}. {item}")
- ret = input("\nPress ENTER to skip. Enter numbers (space seperated): ")
+ ret = input("\nPress ENTER to skip. Enter numbers (space separated): ")
ret = ret.strip("")
if ret == "":
return True
@@ -344,11 +344,10 @@ def _call_generate_tree(config_name: str):
pprint.pprint(config_natives, sort_dicts=False)
# Print valids
- for key in config_valids.keys():
- if key.startswith("in"):
- continue
- valid_items = config_valids[key]
- invalid_items = config_valids[f"in{key}"]
+ for key, valid_itesm in config_valids.items():
+ if not key.startswith("in"):
+ valid_items = config_valids[key]
+ invalid_items = config_valids[f"in{key}"]
print("\n:: " + key.partition("_")[-1])
print(f"{Fore.GREEN}valid > {valid_items.__str__()}")
print(f"{Fore.RED}invalid > {invalid_items.__str__()}")
diff --git a/scripts/backend_generation/tree_generation.py b/scripts/backend_generation/tree_generation.py
index 95e25b6a7b376..c763af66cce48 100644
--- a/scripts/backend_generation/tree_generation.py
+++ b/scripts/backend_generation/tree_generation.py
@@ -208,7 +208,7 @@ def _copy_tree(backend_reference_path: str, backend_generation_path: str):
def _create_type_mapping(config: dict, reference_backend_init_path: str):
- with open(reference_backend_init_path) as file:
+ with open(reference_backend_init_path, "r") as file:
file_src = file.read()
init_tree = ast.parse(file_src)
@@ -232,7 +232,7 @@ def _create_type_mapping(config: dict, reference_backend_init_path: str):
def generate(config_file):
global _config
- with open(config_file) as file:
+ with open(config_file, "r") as file:
_config = json.load(file)
global _target_backend
@@ -269,11 +269,11 @@ def generate(config_file):
"valid_uint_dtypes",
]
for key in valids:
- params[key + "_dict"] = {
- "None": tuple(["ivy." + x for x in _config[key]])
+ params[f"{key}_dict"] = {
+ "None": tuple(f"ivy.{x}" for x in _config[key])
}.__str__()
- params["in" + key + "_dict"] = {
- "None": tuple(["ivy." + x for x in _config["in" + key]])
+ params[f"in{key}_dict"] = {
+ "None": tuple(f"ivy.{x}" for x in _config[f"in{key}"])
}.__str__()
InitFileTransformer(params).visit(tree_to_write)
except Exception as e:
diff --git a/run_tests_CLI/array_api_det_coverage.py b/scripts/determine_tests/array_api_det_coverage.py
similarity index 80%
rename from run_tests_CLI/array_api_det_coverage.py
rename to scripts/determine_tests/array_api_det_coverage.py
index 741b964217745..0315a04139e79 100644
--- a/run_tests_CLI/array_api_det_coverage.py
+++ b/scripts/determine_tests/array_api_det_coverage.py
@@ -1,4 +1,5 @@
import os
+import sys
import subprocess
from pydriller import Repository
from tqdm import tqdm
@@ -8,26 +9,28 @@
def main():
BACKENDS = ["numpy", "jax", "tensorflow", "torch"]
+ N = 4
+ run_iter = int(sys.argv[1]) - 1
test_names = []
func_folder = "ivy_tests/array_api_testing/array_api_methods_to_test"
func_fnames = os.listdir(func_folder)
func_fnames.sort()
framework_tests_to_run = {
- "jax": list(),
- "numpy": list(),
- "torch": list(),
- "tensorflow": list(),
+ "jax": [],
+ "numpy": [],
+ "torch": [],
+ "tensorflow": [],
}
# add from each filepath
for fname in func_fnames:
fpath = os.path.join(func_folder, fname)
- with open(fpath) as file:
+ with open(fpath, "r") as file:
contents = file.read()
contents = [line.replace("__", "") for line in contents.split("\n")]
for framework in framework_tests_to_run:
- tests_to_run = list()
+ tests_to_run = []
for s in contents:
if s == "":
continue
@@ -62,7 +65,7 @@ def main():
)
for backend in BACKENDS:
k_flag_file = f"ivy_tests/array_api_testing/.array_api_tests_k_flag_{backend}"
- with open(k_flag_file) as f:
+ with open(k_flag_file, "r") as f:
array_api_tests_k_flag = f.read().strip()
if backend == "torch":
@@ -77,9 +80,20 @@ def main():
x for x in directories if not (x.endswith("__pycache__") or "hypothesis" in x)
]
directories = set(directories_filtered)
- for test_backend in tqdm(test_names):
+ num_tests = len(test_names)
+ tests_per_run = num_tests // N
+ start = run_iter * tests_per_run
+ end = num_tests if run_iter == N - 1 else (run_iter + 1) * tests_per_run
+ for test_backend in tqdm(test_names[start:end]):
test_name, backend = test_backend.split(",")
- command = f'docker run --rm --env IVY_BACKEND={backend} --env ARRAY_API_TESTS_MODULE="ivy" -v "$(pwd)":/ivy unifyai/ivy:latest timeout 30m /bin/bash -c "coverage run --source=ivy,ivy_tests -m pytest {test_name} -k \\"{k_flag[backend]}\\" --disable-warnings --tb=short -vv > coverage_output;coverage annotate > coverage_output" ' # noqa
+ command = (
+ f"docker run --rm --env IVY_BACKEND={backend} --env "
+ 'ARRAY_API_TESTS_MODULE="ivy" -v "$(pwd)":/ivy unifyai/ivy:latest '
+ 'timeout 30m /bin/bash -c "coverage run --source=ivy,ivy_tests -m pytest '
+ f'{test_name} -k \\"{k_flag[backend]}\\" --disable-warnings --tb=short '
+ "--hypothesis-max-examples 5 -vv > coverage_output;coverage annotate > "
+ 'coverage_output"'
+ )
os.system(command)
for directory in directories:
for file_name in os.listdir(directory):
diff --git a/run_tests_CLI/array_api_determine_tests.py b/scripts/determine_tests/array_api_determine_tests.py
similarity index 99%
rename from run_tests_CLI/array_api_determine_tests.py
rename to scripts/determine_tests/array_api_determine_tests.py
index fade279139968..cfe818105903b 100644
--- a/run_tests_CLI/array_api_determine_tests.py
+++ b/scripts/determine_tests/array_api_determine_tests.py
@@ -42,7 +42,7 @@ def determine_tests_line(_tests_file, _line, _tests_to_run):
for file in modified_files:
try:
file_name = f"{file.new_path},cover"
- except: # noqa
+ except Exception:
continue
if file_name not in tests.keys():
continue
diff --git a/determine_test_coverage.py b/scripts/determine_tests/determine_test_coverage.py
similarity index 92%
rename from determine_test_coverage.py
rename to scripts/determine_tests/determine_test_coverage.py
index 588399a9ab8e5..098e0a61b9fb7 100644
--- a/determine_test_coverage.py
+++ b/scripts/determine_tests/determine_test_coverage.py
@@ -5,7 +5,7 @@
from tqdm import tqdm
import bz2
import _pickle as cPickle
-from run_tests_CLI.get_all_tests import get_all_tests
+from get_all_tests import get_all_tests
# Shared Map
@@ -39,7 +39,7 @@
test_name, backend = test_backend.split(",")
command = (
f'docker run -v "$(pwd)":/ivy unifyai/ivy:latest timeout 30m /bin/bash -c "coverage run --source=ivy,' # noqa
- f"ivy_tests -m pytest {test_name} --backend {backend} --disable-warnings > coverage_output;coverage " # noqa
+ f"ivy_tests -m pytest {test_name} --num-examples 5 --backend {backend} --disable-warnings > coverage_output;coverage " # noqa
f'annotate > coverage_output" '
)
os.system(command)
diff --git a/determine_tests.py b/scripts/determine_tests/determine_tests.py
similarity index 97%
rename from determine_tests.py
rename to scripts/determine_tests/determine_tests.py
index c422d2bee1e2e..ef60b4d28c335 100644
--- a/determine_tests.py
+++ b/scripts/determine_tests/determine_tests.py
@@ -6,7 +6,7 @@
import bz2
import _pickle as cPickle
import sys
-from run_tests_CLI.get_all_tests import get_all_tests
+from get_all_tests import get_all_tests
MAX_TESTS = 10
@@ -45,7 +45,7 @@ def main():
for file in modified_files:
try:
file_name = f"{file.new_path},cover"
- except: # noqa
+ except Exception: # noqa
continue
if file_name not in tests.keys():
continue
@@ -138,7 +138,7 @@ def main():
directories_filtered = [
x
for x in directories
- if not (x.endswith("__pycache__") or "hypothesis" in x)
+ if not x.endswith("__pycache__") and "hypothesis" not in x
]
directories = set(directories_filtered)
for test_backend in new_tests[old_num_tests:num_tests]:
@@ -155,7 +155,7 @@ def main():
for directory in directories:
for file_name in os.listdir(directory):
if file_name.endswith("cover"):
- file_name = directory + "/" + file_name
+ file_name = f"{directory}/{file_name}"
if file_name not in tests:
tests[file_name] = []
with open(file_name) as f:
diff --git a/run_tests_CLI/get_all_tests.py b/scripts/determine_tests/get_all_tests.py
similarity index 92%
rename from run_tests_CLI/get_all_tests.py
rename to scripts/determine_tests/get_all_tests.py
index c2c95d720672f..122c78ccafd3a 100644
--- a/run_tests_CLI/get_all_tests.py
+++ b/scripts/determine_tests/get_all_tests.py
@@ -2,7 +2,7 @@
import random
import ast
-BACKENDS = ["numpy", "jax", "tensorflow", "torch", "paddle"]
+BACKENDS = ["jax", "numpy", "tensorflow", "torch", "paddle"]
def is_test_function(node):
@@ -12,7 +12,7 @@ def is_test_function(node):
def extract_tests_from_file(filename):
- with open(filename) as file:
+ with open(filename, "r") as file:
try:
module = ast.parse(file.read())
except SyntaxError:
diff --git a/duplicate.py b/scripts/duplicate.py
similarity index 96%
rename from duplicate.py
rename to scripts/duplicate.py
index bd084f4ca411b..589183e308222 100644
--- a/duplicate.py
+++ b/scripts/duplicate.py
@@ -1,12 +1,13 @@
import importlib
import os
+import sys
import glob
def get_all_functions_from_directory(root_dir, startswith="test"):
if not os.path.exists(root_dir):
print("Invalid directory")
- exit(1)
+ sys.exit(1)
functions_names = []
for filename in glob.iglob(f"{root_dir}/**/*.py", recursive=True):
if len(filename) >= 2 and filename[:2] == "./":
@@ -40,4 +41,4 @@ def check_duplicate():
common_set = check_duplicate()
if len(common_set) != 0:
print("This function already exists in the functional API.")
- exit(1)
+ sys.exit(1)
diff --git a/generate_intelligent_tests_workflow.py b/scripts/generate_intelligent_tests_workflow.py
similarity index 92%
rename from generate_intelligent_tests_workflow.py
rename to scripts/generate_intelligent_tests_workflow.py
index e7f2e66ea181a..6b8b8a3a301cb 100644
--- a/generate_intelligent_tests_workflow.py
+++ b/scripts/generate_intelligent_tests_workflow.py
@@ -70,12 +70,12 @@
print(" touch .ivy/key.pem")
print(" echo -n ${{ secrets.USER_API_KEY }} > .ivy/key.pem")
if i == 1:
- print(" python determine_tests.py extra")
+ print(" python scripts/determine_tests/determine_tests.py extra")
else:
- print(" python determine_tests.py")
+ print(" python scripts/determine_tests/determine_tests.py")
print(" set -o pipefail")
print(
- f" python run_tests_pr.py new_failures_{i}.txt | tee"
+ f" python scripts/run_tests/run_tests_pr.py new_failures_{i}.txt | tee"
f" test_results_{i}.txt"
)
print(" continue-on-error: true")
diff --git a/scripts/rename_wheels.py b/scripts/rename_wheels.py
new file mode 100644
index 0000000000000..656cb01c68f8c
--- /dev/null
+++ b/scripts/rename_wheels.py
@@ -0,0 +1,14 @@
+import os
+
+if __name__ == "__main__":
+ tag = os.environ["TAG"]
+ python_tag, abi_tag, plat_name = tag.split("-")
+ if os.path.exists("dist"):
+ for file in os.listdir("dist"):
+ old_name = f"{python_tag}-none-{plat_name}.whl"
+ new_name = f"{python_tag}-{abi_tag}-{plat_name}.whl"
+ if file.endswith(old_name):
+ os.rename(
+ os.path.join("dist", file),
+ os.path.join("dist", file[: -len(old_name)] + new_name),
+ )
diff --git a/run_tests_CLI/array_api_run_tests.py b/scripts/run_tests/array_api_run_tests.py
similarity index 97%
rename from run_tests_CLI/array_api_run_tests.py
rename to scripts/run_tests/array_api_run_tests.py
index e19136484b3f5..6417b24e46444 100644
--- a/run_tests_CLI/array_api_run_tests.py
+++ b/scripts/run_tests/array_api_run_tests.py
@@ -74,13 +74,13 @@ def main():
)
for backend in BACKENDS:
k_flag_file = f"ivy_tests/array_api_testing/.array_api_tests_k_flag_{backend}"
- with open(k_flag_file) as f:
+ with open(k_flag_file, "r") as f:
array_api_tests_k_flag = f.read().strip()
if backend == "torch":
array_api_tests_k_flag += " and not (uint16 or uint32 or uint64)"
k_flag[backend] = array_api_tests_k_flag
- with open("tests_to_run") as f:
+ with open("tests_to_run", "r") as f:
for line in f:
test, backend = line.split(",")
backend = backend.strip("\n")
@@ -106,7 +106,7 @@ def main():
"latest-stable",
)
if failed:
- exit(1)
+ sys.exit(1)
if __name__ == "__main__":
diff --git a/scripts/run_tests/array_api_run_tests_pr.py b/scripts/run_tests/array_api_run_tests_pr.py
new file mode 100644
index 0000000000000..72c774b85a0e6
--- /dev/null
+++ b/scripts/run_tests/array_api_run_tests_pr.py
@@ -0,0 +1,43 @@
+# Run Array API Tests for PRs
+import os
+import subprocess
+import sys
+
+BACKENDS = ["numpy", "jax", "tensorflow", "torch"]
+
+
+def main():
+ failed = False
+ k_flag = {}
+ subprocess.run(
+ ["python3", "ivy_tests/array_api_testing/write_array_api_tests_k_flag.py"],
+ check=True,
+ )
+ for backend in BACKENDS:
+ k_flag_file = f"ivy_tests/array_api_testing/.array_api_tests_k_flag_{backend}"
+ with open(k_flag_file, "r") as f:
+ array_api_tests_k_flag = f.read().strip()
+ if backend == "torch":
+ array_api_tests_k_flag += " and not (uint16 or uint32 or uint64)"
+ k_flag[backend] = array_api_tests_k_flag
+
+ with open(sys.argv[1], "w") as f_write:
+ with open("tests_to_run", "r") as f:
+ for line in f:
+ test, backend = line.split(",")
+ backend = backend.strip("\n")
+ command = f'docker run --rm --env IVY_BACKEND={backend} --env ARRAY_API_TESTS_MODULE="ivy" -v "$(pwd)":/ivy -v "$(pwd)"/.hypothesis:/.hypothesis unifyai/ivy:latest timeout 30m python3 -m pytest {test} -k "{k_flag[backend]}" --tb=short -vv' # noqa
+ print(f"\n{'*' * 100}")
+ print(f"{line[:-1]}")
+ print(f"{'*' * 100}\n")
+ sys.stdout.flush()
+ ret = os.system(command)
+ if ret != 0:
+ failed = True
+ f_write.write(line)
+ if failed:
+ sys.exit(1)
+
+
+if __name__ == "__main__":
+ main()
diff --git a/scripts/run_tests/get_all_tests.py b/scripts/run_tests/get_all_tests.py
new file mode 100644
index 0000000000000..122c78ccafd3a
--- /dev/null
+++ b/scripts/run_tests/get_all_tests.py
@@ -0,0 +1,49 @@
+import os
+import random
+import ast
+
+BACKENDS = ["jax", "numpy", "tensorflow", "torch", "paddle"]
+
+
+def is_test_function(node):
+ if isinstance(node, ast.FunctionDef):
+ return node.name.startswith("test_")
+ return False
+
+
+def extract_tests_from_file(filename):
+ with open(filename, "r") as file:
+ try:
+ module = ast.parse(file.read())
+ except SyntaxError:
+ print(f"Syntax error in file: {filename}")
+ return []
+
+ return [
+ f"{filename}::{node.name}" for node in module.body if is_test_function(node)
+ ]
+
+
+def extract_tests_from_dir(directory):
+ test_files = []
+ for root, _, files in os.walk(directory):
+ for file in files:
+ if file.endswith(".py") and "helpers" not in root:
+ full_path = os.path.join(root, file)
+ test_files.extend(extract_tests_from_file(full_path))
+
+ return test_files
+
+
+def get_all_tests():
+ test_names_without_backend = extract_tests_from_dir("ivy_tests/test_ivy")
+ test_names_without_backend = sorted(set(test_names_without_backend))
+ random.Random(4).shuffle(test_names_without_backend)
+
+ test_names = []
+ for test_name in test_names_without_backend:
+ for backend in BACKENDS:
+ test_backend = f"{test_name},{backend}"
+ test_names.append(test_backend)
+
+ return test_names
diff --git a/scripts/run_tests/old_run_test_helpers.py b/scripts/run_tests/old_run_test_helpers.py
new file mode 100644
index 0000000000000..29a7a6338a525
--- /dev/null
+++ b/scripts/run_tests/old_run_test_helpers.py
@@ -0,0 +1,95 @@
+submodules = (
+ "test_paddle",
+ "test_tensorflow",
+ "test_torch",
+ "test_jax",
+ "test_numpy",
+ "test_functional",
+ "test_experimental",
+ "test_stateful",
+ "test_misc",
+ "test_scipy",
+ "test_pandas",
+ "test_mindspore",
+ "test_onnx",
+ "test_sklearn",
+ "test_xgboost",
+)
+
+db_dict = {
+ "test_functional/test_core": ["core", 10],
+ "test_experimental/test_core": ["exp_core", 11],
+ "test_functional/test_nn": ["nn", 12],
+ "test_experimental/test_nn": ["exp_nn", 13],
+ "test_stateful": ["stateful", 14],
+ "test_torch": ["torch", 15],
+ "test_jax": ["jax", 16],
+ "test_tensorflow": ["tensorflow", 17],
+ "test_numpy": ["numpy", 18],
+ "test_misc": ["misc", 19],
+ "test_paddle": ["paddle", 20],
+ "test_scipy": ["scipy", 21],
+ "test_pandas": ["pandas", 22],
+ "test_mindspore": ["mindspore", 23],
+ "test_onnx": ["onnx", 24],
+ "test_sklearn": ["sklearn", 25],
+ "test_xgboost": ["xgboost", 26],
+}
+
+result_config = {
+ "success": "https://img.shields.io/badge/-success-success",
+ "failure": "https://img.shields.io/badge/-failure-red",
+}
+
+
+def make_clickable(url, name):
+ return (
+ f'
'
+ )
+
+
+def get_submodule(test_path):
+ test_path = test_path.split("/")
+ for name in submodules:
+ if name in test_path:
+ if name == "test_functional":
+ if len(test_path) > 3 and test_path[3] == "test_experimental":
+ coll = db_dict[f"test_experimental/{test_path[4]}"]
+ else:
+ coll = db_dict[f"test_functional/{test_path[-2]}"]
+ else:
+ coll = db_dict[name]
+ break
+ submod_test = test_path[-1]
+ submod, test_fn = submod_test.split("::")
+ submod = submod.replace("test_", "").replace(".py", "")
+ return coll, submod, test_fn
+
+
+def update_individual_test_results(
+ collection,
+ id,
+ submod,
+ backend,
+ test,
+ result,
+ backend_version=None,
+ frontend_version=None,
+ device=None,
+):
+ key = f"{submod}.{backend}"
+ if backend_version is not None:
+ backend_version = backend_version.replace(".", "_")
+ key += f".{backend_version}"
+ if frontend_version is not None:
+ frontend_version = frontend_version.replace(".", "_")
+ key += f".{frontend_version}"
+ key += f".{test}"
+ if device:
+ key += f".{device}"
+ collection.update_one(
+ {"_id": id},
+ {"$set": {key: result}},
+ upsert=True,
+ )
diff --git a/scripts/run_tests/run_tests.py b/scripts/run_tests/run_tests.py
new file mode 100644
index 0000000000000..1dadc0d123b45
--- /dev/null
+++ b/scripts/run_tests/run_tests.py
@@ -0,0 +1,301 @@
+# Run Tests
+import os
+import sys
+from pymongo import MongoClient
+from pymongo.errors import WriteError
+import requests
+import json
+import old_run_test_helpers as old_helpers
+from get_all_tests import BACKENDS
+
+
+def get_latest_package_version(package_name):
+ try:
+ url = f"https://pypi.org/pypi/{package_name}/json"
+ response = requests.get(url)
+ response.raise_for_status()
+ package_info = response.json()
+ return package_info["info"]["version"]
+ except requests.exceptions.RequestException:
+ print(f"Error: Failed to fetch package information for {package_name}.")
+ return None
+
+
+def get_submodule_and_function_name(test_path, is_frontend_test=False):
+ submodule_test = test_path.split("/")[-1]
+ submodule, test_function = submodule_test.split("::")
+ submodule = submodule.replace("test_", "").replace(".py", "")
+
+ with open(test_path.split("::")[0]) as test_file:
+ test_file_content = test_file.read()
+ test_function_idx = test_file_content.find(f"def {test_function}")
+ test_function_block_idx = test_file_content[:test_function_idx].rfind("\n\n")
+ if test_function_block_idx == -1:
+ return submodule, None
+ relevant_file_content = test_file_content[
+ test_function_block_idx:test_function_idx
+ ]
+ fn_tree_idx = relevant_file_content.rfind('fn_tree="')
+
+ # frontend test
+ if is_frontend_test:
+ function_name = relevant_file_content[fn_tree_idx + 9 :].split('"')[0]
+
+ # instance method test
+ if fn_tree_idx == -1:
+ class_tree_idx = test_file_content.find('CLASS_TREE = "')
+ method_name_idx = relevant_file_content.rfind('method_name="')
+ if class_tree_idx == -1 or method_name_idx == -1:
+ return submodule, None
+ class_tree = test_file_content[class_tree_idx + 14 :].split('"')[0]
+ class_name = ".".join(class_tree.split(".")[3:])
+ method_name = relevant_file_content[method_name_idx + 13 :].split('"')[
+ 0
+ ]
+ function_name = f"{class_name}.{method_name}"
+
+ # ivy test
+ else:
+ function_name = test_function[5:]
+
+ # instance method test
+ if fn_tree_idx == -1:
+ method_name_idx = relevant_file_content.rfind('method_tree="')
+ if method_name_idx != -1:
+ method_name = relevant_file_content[method_name_idx + 13 :].split(
+ '"'
+ )[0]
+ function_name = f"ivy.{method_name}"
+ else:
+ return submodule, None
+
+ return submodule, function_name
+
+
+if __name__ == "__main__":
+ redis_url = sys.argv[1]
+ redis_pass = sys.argv[2]
+ mongo_key = sys.argv[3]
+ version_flag = sys.argv[4]
+ gpu_flag = sys.argv[5]
+ workflow_id = sys.argv[6]
+ priority_flag = sys.argv[7]
+
+ if len(sys.argv) > 8 and sys.argv[8] != "null":
+ run_id = sys.argv[8]
+ else:
+ run_id = f"https://github.com/unifyai/ivy/actions/runs/{workflow_id}"
+
+ device = "cpu"
+ if gpu_flag == "true":
+ device = "gpu"
+
+ cluster = MongoClient(
+ f"mongodb+srv://deep-ivy:{mongo_key}@cluster0.qdvf8q3.mongodb.net/?retryWrites=true&w=majority" # noqa
+ )
+ db = cluster["ci_dashboard"]
+
+ # old
+ if priority_flag == "true":
+ priority_flag = True
+ else:
+ priority_flag = False
+ failed = False
+ old_db = cluster["Ivy_tests_multi_gpu"]
+ old_db_priority = cluster["Ivy_tests_priority"]
+
+ # pull gpu image for gpu testing
+ if device == "gpu":
+ os.system("docker pull unifyai/ivy:latest-gpu")
+
+ # read the tests to be run
+ with open("tests_to_run", "r") as f:
+ for line in f:
+ print(f"\n{'*' * 100}")
+ print(f"{line[:-1]}")
+ print(f"{'*' * 100}\n")
+
+ # get the test, submodule, backend and version
+ test_path, backend = line.strip().split(",")
+ is_frontend_test = "test_frontends" in test_path
+ collection = db["frontend_tests"] if is_frontend_test else db["ivy_tests"]
+ submodule, function_name = get_submodule_and_function_name(
+ test_path, is_frontend_test
+ )
+ version = get_latest_package_version(backend).replace(".", "_")
+
+ # old
+ coll, submod, test_fn = old_helpers.get_submodule(test_path)
+ backend_version = "latest-stable"
+
+ # multi-version tests
+ if version_flag == "true":
+ backends = [backend.strip()]
+ backend_name, backend_version = backend.split("/")
+ other_backends = [
+ fw for fw in BACKENDS if (fw != backend_name and fw != "paddle")
+ ]
+ for other_backend in other_backends:
+ backends.append(
+ other_backend + "/" + get_latest_package_version(other_backend)
+ )
+ print("Backends:", backends)
+ os.system(
+ 'docker run --name test-container -v "$(pwd)":/ivy/ivy '
+ f"-e REDIS_URL={redis_url} -e REDIS_PASSWD={redis_pass} "
+ "-itd unifyai/multiversion:latest /bin/bash -c"
+ f'python multiversion_framework_directory.py {" ".join(backends)};'
+ )
+ os.system(
+ "docker exec test-container cd ivy; python3 -m pytest --tb=short "
+ f"{test_path} --backend={backend.strip()}"
+ )
+ backend = backend.split("/")[0] + "\n"
+ backend_version = backend_version.strip()
+
+ else:
+ device_str = ""
+ image = "unifyai/ivy:latest"
+
+ # gpu tests
+ if device == "gpu":
+ image = "unifyai/ivy:latest-gpu"
+ device_str = " --device=gpu:0"
+
+ os.system(
+ 'docker run --name test-container -v "$(pwd)":/ivy -v '
+ f'"$(pwd)"/.hypothesis:/.hypothesis -e REDIS_URL={redis_url} '
+ f"-e REDIS_PASSWD={redis_pass} -itd {image}"
+ )
+ command = (
+ "docker exec test-container python3 -m pytest --tb=short"
+ f" {test_path} {device_str} --backend {backend}"
+ )
+ os.system(command)
+
+ # run the test
+ sys.stdout.flush()
+ failed = bool(os.system(command))
+
+ # old (populate the old database with results)
+ if not failed:
+ res = old_helpers.make_clickable(
+ run_id, old_helpers.result_config["success"]
+ )
+ else:
+ res = old_helpers.make_clickable(
+ run_id, old_helpers.result_config["failure"]
+ )
+ failed = True
+ frontend_version = None
+ if coll[0] in ["numpy", "jax", "tensorflow", "torch", "paddle"]:
+ frontend_version = "latest-stable"
+ try:
+ if priority_flag:
+ print("Updating Priority DB")
+ old_helpers.update_individual_test_results(
+ old_db_priority[coll[0]],
+ coll[1],
+ submod,
+ backend,
+ test_fn,
+ res,
+ "latest-stable",
+ frontend_version,
+ device,
+ )
+ else:
+ print(backend_version)
+ old_helpers.update_individual_test_results(
+ old_db[coll[0]],
+ coll[1],
+ submod,
+ backend,
+ test_fn,
+ res,
+ backend_version,
+ frontend_version,
+ device,
+ )
+ except WriteError:
+ print("Old DB Write Error")
+
+ # skip updating db for instance methods as of now
+ # run transpilation tests if the test passed
+ if not failed and function_name:
+ print(f"\n{'*' * 100}")
+ print(f"{line[:-1]} --> transpilation tests")
+ print(f"{'*' * 100}\n")
+ command = f"{command} --num-examples 5 --with-transpile"
+ sys.stdout.flush()
+ os.system(command)
+ os.system(
+ "docker cp test-container:/ivy/report.json"
+ f" {__file__[: __file__.rfind(os.sep)]}/report.json"
+ )
+
+ # load data from report if generated
+ report_path = os.path.join(
+ __file__[: __file__.rfind(os.sep)], "report.json"
+ )
+ report_content = {}
+ if os.path.exists(report_path):
+ report_content = json.load(open(report_path))
+
+ # create a prefix str for the update query for frontend tests
+ # (with frontend version)
+ test_info = {}
+ prefix_str = ""
+ if is_frontend_test:
+ frontend = test_path[test_path.find("test_frontends") :].split(os.sep)[
+ 1
+ ][5:]
+ frontend_version = get_latest_package_version(frontend).replace(
+ ".", "_"
+ )
+ test_info["frontend"] = frontend
+ prefix_str = f"{frontend_version}."
+
+ # initialize test information for ci_dashboard db
+ # format of the last 2 keys
+ # ....
+ # ...
+ # for frontend tests and ivy tests respectively
+ test_info = {
+ "_id": function_name,
+ "test_path": test_path,
+ "submodule": submodule,
+ f"{prefix_str}{backend}.{version}.status.{device}": not failed,
+ f"{prefix_str}{backend}.{version}.workflow.{device}": run_id,
+ }
+
+ # add transpilation metrics if report generated
+ if not failed and report_content:
+ if is_frontend_test:
+ test_info = {
+ **test_info,
+ "fw_time": report_content["fw_time"],
+ "ivy_nodes": report_content["ivy_nodes"],
+ }
+ transpilation_metrics = {
+ "nodes": report_content["nodes"][backend],
+ "time": report_content["time"][backend],
+ "args": report_content["args"][backend],
+ "kwargs": report_content["kwargs"][backend],
+ }
+ for metric, value in transpilation_metrics.items():
+ test_info[f"{prefix_str}{backend}.{version}.{metric}"] = value
+
+ # populate the ci_dashboard db, skip instance methods
+ if function_name:
+ id = test_info.pop("_id")
+ print(
+ collection.update_one({"_id": id}, {"$set": test_info}, upsert=True)
+ )
+
+ # delete the container
+ os.system("docker rm -f test-container")
+
+ # if any tests fail, the workflow fails
+ if failed:
+ sys.exit(1)
diff --git a/run_tests_pr.py b/scripts/run_tests/run_tests_pr.py
similarity index 97%
rename from run_tests_pr.py
rename to scripts/run_tests/run_tests_pr.py
index 2b64b1a33fde7..3dd5a0a7a3d29 100644
--- a/run_tests_pr.py
+++ b/scripts/run_tests/run_tests_pr.py
@@ -55,7 +55,7 @@ def get_mod_submod_test(test_path):
if __name__ == "__main__":
failed = False
with open(sys.argv[1], "w") as f_write:
- with open("tests_to_run") as f:
+ with open("tests_to_run", "r") as f:
for line in f:
test, backend = line.split(",")
print(f"\n{'*' * 100}")
@@ -79,4 +79,4 @@ def get_mod_submod_test(test_path):
f_write.write(line)
if failed:
- exit(1)
+ sys.exit(1)
diff --git a/run_tests_CLI/clone-mapping.py b/scripts/setup_tests/clone-mapping.py
similarity index 98%
rename from run_tests_CLI/clone-mapping.py
rename to scripts/setup_tests/clone-mapping.py
index bb9df2316da46..ac2347c35c184 100644
--- a/run_tests_CLI/clone-mapping.py
+++ b/scripts/setup_tests/clone-mapping.py
@@ -1,4 +1,5 @@
import os
+import sys
import git
import bz2
import _pickle as cPickle
@@ -9,7 +10,7 @@
# Check if the directory exists
if not os.path.exists(mapping_dir):
print(f"Directory does not exist: {mapping_dir}")
- exit(1)
+ sys.exit(1)
# Create a Repo object to interact with the Git repositories
current_repo = git.Repo("ivy/")
diff --git a/run_tests_CLI/cron_tests.py b/scripts/setup_tests/cron_tests.py
similarity index 100%
rename from run_tests_CLI/cron_tests.py
rename to scripts/setup_tests/cron_tests.py
diff --git a/scripts/setup_tests/cron_tests_multi_version.py b/scripts/setup_tests/cron_tests_multi_version.py
new file mode 100644
index 0000000000000..051472dc21567
--- /dev/null
+++ b/scripts/setup_tests/cron_tests_multi_version.py
@@ -0,0 +1,47 @@
+import sys
+from get_all_tests import get_all_tests
+
+torch_req = ["torch/2.0.0", "torch/2.0.1"]
+tensorflow_req = [
+ "tensorflow/2.13.0",
+ "tensorflow/2.14.0",
+]
+jax_req = [
+ "jax/0.4.10",
+ "jax/0.4.14",
+]
+numpy_req = [
+ "numpy/1.25.0",
+ "numpy/1.24.0",
+]
+framework_versions = {
+ "numpy": numpy_req,
+ "torch": torch_req,
+ "jax": jax_req,
+ "tensorflow": tensorflow_req,
+}
+
+run_iter = int(sys.argv[1])
+all_tests = get_all_tests()
+test_names_without_backend = [test.split(",")[0].strip() for test in all_tests]
+test_names = []
+for test_name in test_names_without_backend:
+ for backend, backend_versions in framework_versions.items():
+ for backend_version in backend_versions:
+ test_backend = test_name + "," + backend_version
+ test_names.append(test_backend)
+
+# Run 150 tests in each iteration of the cron job
+num_tests = len(test_names)
+tests_per_run = 5
+start = run_iter * tests_per_run
+end = (run_iter + 1) * tests_per_run
+print("Running Tests:")
+with open("tests_to_run", "w") as f:
+ for i in range(start, end):
+ i = i % num_tests
+ test = test_names[i]
+ if "test_frontends" in test:
+ continue # skip frontend tests (No support from testing)
+ print(test)
+ f.write(test + "\n")
diff --git a/run_tests_CLI/filter_tests.py b/scripts/setup_tests/filter_tests.py
similarity index 100%
rename from run_tests_CLI/filter_tests.py
rename to scripts/setup_tests/filter_tests.py
diff --git a/scripts/setup_tests/get_all_tests.py b/scripts/setup_tests/get_all_tests.py
new file mode 100644
index 0000000000000..122c78ccafd3a
--- /dev/null
+++ b/scripts/setup_tests/get_all_tests.py
@@ -0,0 +1,49 @@
+import os
+import random
+import ast
+
+BACKENDS = ["jax", "numpy", "tensorflow", "torch", "paddle"]
+
+
+def is_test_function(node):
+ if isinstance(node, ast.FunctionDef):
+ return node.name.startswith("test_")
+ return False
+
+
+def extract_tests_from_file(filename):
+ with open(filename, "r") as file:
+ try:
+ module = ast.parse(file.read())
+ except SyntaxError:
+ print(f"Syntax error in file: {filename}")
+ return []
+
+ return [
+ f"{filename}::{node.name}" for node in module.body if is_test_function(node)
+ ]
+
+
+def extract_tests_from_dir(directory):
+ test_files = []
+ for root, _, files in os.walk(directory):
+ for file in files:
+ if file.endswith(".py") and "helpers" not in root:
+ full_path = os.path.join(root, file)
+ test_files.extend(extract_tests_from_file(full_path))
+
+ return test_files
+
+
+def get_all_tests():
+ test_names_without_backend = extract_tests_from_dir("ivy_tests/test_ivy")
+ test_names_without_backend = sorted(set(test_names_without_backend))
+ random.Random(4).shuffle(test_names_without_backend)
+
+ test_names = []
+ for test_name in test_names_without_backend:
+ for backend in BACKENDS:
+ test_backend = f"{test_name},{backend}"
+ test_names.append(test_backend)
+
+ return test_names
diff --git a/run_tests_CLI/run_ivy_core_test.py b/scripts/setup_tests/run_ivy_core_test.py
similarity index 96%
rename from run_tests_CLI/run_ivy_core_test.py
rename to scripts/setup_tests/run_ivy_core_test.py
index a1eb8e29cda79..c05646373fc75 100644
--- a/run_tests_CLI/run_ivy_core_test.py
+++ b/scripts/setup_tests/run_ivy_core_test.py
@@ -25,7 +25,7 @@
M = len(submodules)
num_tests = N * M
-run = run % num_tests
+run %= num_tests
i = run // M
j = run % M
diff --git a/run_tests_CLI/run_ivy_nn_test.py b/scripts/setup_tests/run_ivy_nn_test.py
similarity index 100%
rename from run_tests_CLI/run_ivy_nn_test.py
rename to scripts/setup_tests/run_ivy_nn_test.py
diff --git a/run_tests_CLI/run_ivy_stateful_test.py b/scripts/setup_tests/run_ivy_stateful_test.py
similarity index 96%
rename from run_tests_CLI/run_ivy_stateful_test.py
rename to scripts/setup_tests/run_ivy_stateful_test.py
index 1ef9a28d4b820..7651e1fba8601 100644
--- a/run_tests_CLI/run_ivy_stateful_test.py
+++ b/scripts/setup_tests/run_ivy_stateful_test.py
@@ -19,7 +19,7 @@
M = len(submodules)
num_tests = N * M
-run = run % num_tests
+run %= num_tests
i = run // M
j = run % M
diff --git a/scripts/setup_tests/setup_priority_tests.py b/scripts/setup_tests/setup_priority_tests.py
new file mode 100644
index 0000000000000..509b641289804
--- /dev/null
+++ b/scripts/setup_tests/setup_priority_tests.py
@@ -0,0 +1,47 @@
+import sys
+from pymongo import MongoClient
+from get_all_tests import BACKENDS
+
+
+def main():
+ # connect to the database
+ mongo_key = sys.argv[1]
+ cluster = MongoClient(
+ f"mongodb+srv://deep-ivy:{mongo_key}@cluster0.qdvf8q3.mongodb.net/?retryWrites=true&w=majority" # noqa
+ )
+ ci_dashboard_db = cluster["ci_dashboard"]
+ ivy_tests_collection = ci_dashboard_db["ivy_tests"]
+ frontend_tests_collection = ci_dashboard_db["frontend_tests"]
+ demos_collection = ci_dashboard_db["demos"]
+
+ # iterate over demos and collect ivy and frontend functions used
+ demos = demos_collection.find()
+ ivy_functions, frontend_functions = [], []
+ for demo in demos:
+ ivy_functions += demo.get("ivy_functions", [])
+ frontend_functions += demo.get("frontend_functions", [])
+ ivy_functions = list(set(ivy_functions))
+ frontend_functions = list(set(frontend_functions))
+
+ # find corresponding test paths for those functions
+ ivy_test_paths = []
+ frontend_test_paths = []
+ for function in ivy_functions:
+ result = ivy_tests_collection.find_one({"_id": function})
+ if result:
+ ivy_test_paths.append(result["test_path"])
+ for function in frontend_functions:
+ result = frontend_tests_collection.find_one({"_id": function})
+ if result:
+ frontend_test_paths.append(result["test_path"])
+
+ # add those paths to the tests_to_run
+ with open("tests_to_run", "w") as write_file:
+ for test_path in ivy_test_paths + frontend_test_paths:
+ test_path = test_path.strip()
+ for backend in BACKENDS:
+ write_file.write(f"{test_path},{backend}\n")
+
+
+if __name__ == "__main__":
+ main()
diff --git a/setup_tests.py b/scripts/setup_tests/setup_tests.py
similarity index 86%
rename from setup_tests.py
rename to scripts/setup_tests/setup_tests.py
index 9f2a357d9a829..f3a10c1bdc8d8 100644
--- a/setup_tests.py
+++ b/scripts/setup_tests/setup_tests.py
@@ -1,5 +1,5 @@
import sys
-from run_tests_CLI.get_all_tests import BACKENDS
+from get_all_tests import BACKENDS
def main():
diff --git a/run_tests_CLI/synchronize_db.py b/scripts/setup_tests/synchronize_db.py
similarity index 90%
rename from run_tests_CLI/synchronize_db.py
rename to scripts/setup_tests/synchronize_db.py
index 0de0455a8d0c4..fa5d53e924b0d 100644
--- a/run_tests_CLI/synchronize_db.py
+++ b/scripts/setup_tests/synchronize_db.py
@@ -16,6 +16,7 @@
"misc": "test_misc",
"paddle": "test_frontends/test_paddle",
"scipy": "test_frontends/test_scipy",
+ "torchvision": "test_frontends/test_torchvision",
}
@@ -24,19 +25,18 @@ def keys_to_delete_from_db(all_tests, module, data, current_key=""):
keys_for_deletion = []
for key, value in data.items():
- new_key = current_key + "." + key if current_key else key
+ new_key = f"{current_key}.{key}" if current_key else key
# If this is a dictionary, recurse deeper
if isinstance(value, dict):
keys_for_deletion.extend(
keys_to_delete_from_db(all_tests, module, value, new_key)
)
- # If the new_key is not in keys_to_keep, mark it for deletion
elif key != "_id":
components = new_key.split(".")
submodule = components[0]
function = components[-2]
- test = module + "/" + submodule + "::" + function
+ test = f"{module}/{submodule}::{function}"
if test not in all_tests:
keys_for_deletion.append(".".join(components[:-1]))
@@ -59,6 +59,7 @@ def keys_to_delete_from_db(all_tests, module, data, current_key=""):
"test_onnx",
"test_sklearn",
"test_xgboost",
+ "test_torchvision",
)
db_dict = {
"test_functional/test_core": ["core", 10],
@@ -78,6 +79,7 @@ def keys_to_delete_from_db(all_tests, module, data, current_key=""):
"test_onnx": ["onnx", 24],
"test_sklearn": ["sklearn", 25],
"test_xgboost": ["xgboost", 26],
+ "test_torchvision": ["torchvision", 27],
}
@@ -87,9 +89,9 @@ def get_submodule(test_path):
if name in test_path:
if name == "test_functional":
if test_path[3] == "test_experimental":
- coll = db_dict["test_experimental/" + test_path[4]]
+ coll = db_dict[f"test_experimental/{test_path[4]}"]
else:
- coll = db_dict["test_functional/" + test_path[-2]]
+ coll = db_dict[f"test_functional/{test_path[-2]}"]
else:
coll = db_dict[name]
break
@@ -101,7 +103,7 @@ def get_submodule(test_path):
def process_test(test):
coll, submod, test_fn = get_submodule(test)
- return coll[0] + "/" + submod + "::" + test_fn
+ return f"{coll[0]}/{submod}::{test_fn}"
def remove_empty_objects(document, key_prefix=""):
@@ -114,7 +116,7 @@ def remove_empty_objects(document, key_prefix=""):
for key, value in document.items():
# Generate the full key path
- full_key = key_prefix + "." + key if key_prefix else key
+ full_key = f"{key_prefix}.{key}" if key_prefix else key
# If the value is a dictionary, recursively check for empty objects
if isinstance(value, dict):
diff --git a/clone_mapping.sh b/scripts/shell/clone_mapping.sh
similarity index 90%
rename from clone_mapping.sh
rename to scripts/shell/clone_mapping.sh
index 5f8a5f5e01ea0..4a94c56ef55fb 100755
--- a/clone_mapping.sh
+++ b/scripts/shell/clone_mapping.sh
@@ -1,5 +1,5 @@
-USER_EMAIL="rashul.chutani@gmail.com"
-USER_NAME="Rashul Chutani"
+USER_EMAIL="ivy.branch@lets-unify.ai"
+USER_NAME="ivy-branch"
TARGET_BRANCH=$1
CLONE_DIR=$(mktemp -d)
GITHUB_SERVER="github.com"
diff --git a/scripts/shell/deploy_pypi.sh b/scripts/shell/deploy_pypi.sh
new file mode 100644
index 0000000000000..720d60f39bfb4
--- /dev/null
+++ b/scripts/shell/deploy_pypi.sh
@@ -0,0 +1,6 @@
+jq -c '.compiler[]' available_configs.json | while read config; do
+ export TAG=${config:1:${#config}-2}
+ python -m build
+done
+python3 scripts/rename_wheels.py
+python3 -m twine upload dist/* -u "__token__" -p "$PYPI_PASSWORD" --verbose
diff --git a/merge_with_upstream.sh b/scripts/shell/merge_with_upstream.sh
similarity index 100%
rename from merge_with_upstream.sh
rename to scripts/shell/merge_with_upstream.sh
diff --git a/run_tests_CLI/run_tests.sh b/scripts/shell/run_tests.sh
similarity index 100%
rename from run_tests_CLI/run_tests.sh
rename to scripts/shell/run_tests.sh
diff --git a/stash_pull.sh b/scripts/shell/stash_pull.sh
similarity index 100%
rename from stash_pull.sh
rename to scripts/shell/stash_pull.sh
diff --git a/run_tests_CLI/test_array_api.sh b/scripts/shell/test_array_api.sh
similarity index 100%
rename from run_tests_CLI/test_array_api.sh
rename to scripts/shell/test_array_api.sh
diff --git a/run_tests_CLI/test_dependencies.sh b/scripts/shell/test_dependencies.sh
similarity index 100%
rename from run_tests_CLI/test_dependencies.sh
rename to scripts/shell/test_dependencies.sh
diff --git a/run_tests_CLI/test_experimental_core.sh b/scripts/shell/test_experimental_core.sh
similarity index 100%
rename from run_tests_CLI/test_experimental_core.sh
rename to scripts/shell/test_experimental_core.sh
diff --git a/run_tests_CLI/test_experimental_nn.sh b/scripts/shell/test_experimental_nn.sh
similarity index 100%
rename from run_tests_CLI/test_experimental_nn.sh
rename to scripts/shell/test_experimental_nn.sh
diff --git a/run_tests_CLI/test_ivy_core.sh b/scripts/shell/test_ivy_core.sh
similarity index 100%
rename from run_tests_CLI/test_ivy_core.sh
rename to scripts/shell/test_ivy_core.sh
diff --git a/run_tests_CLI/test_ivy_nn.sh b/scripts/shell/test_ivy_nn.sh
similarity index 100%
rename from run_tests_CLI/test_ivy_nn.sh
rename to scripts/shell/test_ivy_nn.sh
diff --git a/run_tests_CLI/test_ivy_stateful.sh b/scripts/shell/test_ivy_stateful.sh
similarity index 100%
rename from run_tests_CLI/test_ivy_stateful.sh
rename to scripts/shell/test_ivy_stateful.sh
diff --git a/run_tests_CLI/test_jax_frontend.sh b/scripts/shell/test_jax_frontend.sh
similarity index 100%
rename from run_tests_CLI/test_jax_frontend.sh
rename to scripts/shell/test_jax_frontend.sh
diff --git a/run_tests_CLI/test_numpy_frontend.sh b/scripts/shell/test_numpy_frontend.sh
similarity index 100%
rename from run_tests_CLI/test_numpy_frontend.sh
rename to scripts/shell/test_numpy_frontend.sh
diff --git a/run_tests_CLI/test_tensorflow_frontend.sh b/scripts/shell/test_tensorflow_frontend.sh
similarity index 100%
rename from run_tests_CLI/test_tensorflow_frontend.sh
rename to scripts/shell/test_tensorflow_frontend.sh
diff --git a/run_tests_CLI/test_torch_frontend.sh b/scripts/shell/test_torch_frontend.sh
similarity index 100%
rename from run_tests_CLI/test_torch_frontend.sh
rename to scripts/shell/test_torch_frontend.sh
diff --git a/run_tests_CLI/test_dependencies.py b/scripts/test_dependencies.py
similarity index 99%
rename from run_tests_CLI/test_dependencies.py
rename to scripts/test_dependencies.py
index 929f284fc1ed1..02bb80e9c2d0c 100644
--- a/run_tests_CLI/test_dependencies.py
+++ b/scripts/test_dependencies.py
@@ -58,7 +58,7 @@ def test_imports(fname, assert_version, update_versions):
PRINT_MSG += msg
ERROR_MSG += msg
WARN_MSG += msg
- with open(fname) as f:
+ with open(fname, "r") as f:
file_lines = f.readlines()
mod_names_n_versions = [parse(req) for req in file_lines]
for line_num, (mod_name, expected_version, expected_op) in enumerate(
diff --git a/setup.py b/setup.py
index fd739df0e6761..ae87c18254b6b 100644
--- a/setup.py
+++ b/setup.py
@@ -44,13 +44,18 @@ def _strip(line):
# Download all relevant binaries in binaries.json
-all_tags = list(tags.sys_tags())
binaries_dict = json.load(open("binaries.json"))
available_configs = json.load(open("available_configs.json"))
binaries_paths = _get_paths_from_binaries(binaries_dict)
version = os.environ["VERSION"] if "VERSION" in os.environ else "main"
terminate = False
-
+fixed_tag = os.environ["TAG"] if "TAG" in os.environ else None
+all_tags = list(tags.sys_tags())
+python_tag, plat_name, options = None, None, None
+if fixed_tag:
+ python_tag, _, plat_name = str(fixed_tag).split("-")
+ options = {"bdist_wheel": {"python_tag": python_tag, "plat_name": plat_name}}
+ all_tags = [fixed_tag]
# download binaries for the tag with highest precedence
for tag in all_tags:
@@ -119,12 +124,13 @@ def _strip(line):
include_package_data=True,
packages=setuptools.find_packages(),
install_requires=[
- _strip(line) for line in open("requirements/requirements.txt", encoding="utf-8")
+ _strip(line)
+ for line in open("requirements/requirements.txt", "r", encoding="utf-8")
],
- python_requires="==3.10.*",
+ python_requires=">=3.8,<=3.11",
classifiers=[
"License :: OSI Approved :: Apache Software License",
- "Programming Language :: Python :: 3.10",
],
license="Apache 2.0",
+ options=options,
)