-
Notifications
You must be signed in to change notification settings - Fork 0
/
Copy pathpart1.Rmd
501 lines (311 loc) · 18 KB
/
part1.Rmd
1
2
3
4
5
6
7
8
9
10
11
12
13
14
15
16
17
18
19
20
21
22
23
24
25
26
27
28
29
30
31
32
33
34
35
36
37
38
39
40
41
42
43
44
45
46
47
48
49
50
51
52
53
54
55
56
57
58
59
60
61
62
63
64
65
66
67
68
69
70
71
72
73
74
75
76
77
78
79
80
81
82
83
84
85
86
87
88
89
90
91
92
93
94
95
96
97
98
99
100
101
102
103
104
105
106
107
108
109
110
111
112
113
114
115
116
117
118
119
120
121
122
123
124
125
126
127
128
129
130
131
132
133
134
135
136
137
138
139
140
141
142
143
144
145
146
147
148
149
150
151
152
153
154
155
156
157
158
159
160
161
162
163
164
165
166
167
168
169
170
171
172
173
174
175
176
177
178
179
180
181
182
183
184
185
186
187
188
189
190
191
192
193
194
195
196
197
198
199
200
201
202
203
204
205
206
207
208
209
210
211
212
213
214
215
216
217
218
219
220
221
222
223
224
225
226
227
228
229
230
231
232
233
234
235
236
237
238
239
240
241
242
243
244
245
246
247
248
249
250
251
252
253
254
255
256
257
258
259
260
261
262
263
264
265
266
267
268
269
270
271
272
273
274
275
276
277
278
279
280
281
282
283
284
285
286
287
288
289
290
291
292
293
294
295
296
297
298
299
300
301
302
303
304
305
306
307
308
309
310
311
312
313
314
315
316
317
318
319
320
321
322
323
324
325
326
327
328
329
330
331
332
333
334
335
336
337
338
339
340
341
342
343
344
345
346
347
348
349
350
351
352
353
354
355
356
357
358
359
360
361
362
363
364
365
366
367
368
369
370
371
372
373
374
375
376
377
378
379
380
381
382
383
384
385
386
387
388
389
390
391
392
393
394
395
396
397
398
399
400
401
402
403
404
405
406
407
408
409
410
411
412
413
414
415
416
417
418
419
420
421
422
423
424
425
426
427
428
429
430
431
432
433
434
435
436
437
438
439
440
441
442
443
444
445
446
447
448
449
450
451
452
453
454
455
456
457
458
459
460
461
462
463
464
465
466
467
468
469
470
471
472
473
474
475
476
477
478
479
480
481
482
483
484
485
486
487
488
489
490
491
492
493
494
495
496
497
498
499
500
501
---
title: "R Crash Course"
author: "Mark Dunning"
date: '`r format(Sys.time(), "Last modified: %d %b %Y")`'
output:
html_notebook:
toc: yes
toc_float: yes
css: stylesheets/styles.css
editor_options:
chunk_output_type: inline
---
<img src="images/logo-sm.png" style="position:absolute;top:40px;right:10px;" width="200" />
# Acknowledgement
If you find these materials useful for your research, please consider acknowledging the Sheffield Bioinformatics Core in any outputs that arise from having learnt R. You can contact us for support on specific projects using:- `[email protected]`
# Disclaimer
<div class="warning">
Although R is well-regarded as a tool for performing statistical analysis, this workshop will not explicitly teach stats. Instead we give introduce the tools that we allow you to manipulate and interrogate your data into a form with which you can execute statistical tests.
</div>
# Setup
If you are following these notes independently (outside one of our workshops)
From the RStudio menus, Choose the **File** -> **New Project** option and select New Directory from the new window

Then for the **Project Type** pick New Project.

It will ask you to pick a new Directory name, and where to create that directory (e.g. your Home directory or directory where you usually save your work)

RStudio should now refresh itself. You can now download the data required for the workshop by copying and pasting the following into the R console (as shown in the screenshot)
```{r}
download.file("https://github.com/sheffield-bioinformatics-core/r-online/raw/master/CourseData.zip", destfile = "CourseData.zip")
```

The files from the zip file can be extracted using the command:-
```{r}
unzip("CourseData.zip")
```
Your RStudio screen should look like:-

You will need to install some R packages and download some data before you start. You can install the packages by copying and pasting the following into an R console and pressing ENTER
```{r}
install.packages("dplyr")
install.packages("ggplot2")
install.packages("readr")
install.packages("rmarkdown")
install.packages("tidyr")
```
You can check that this worked by copying and pasting the following:-
```{r}
source("https://raw.githubusercontent.com/sheffield-bioinformatics-core/r-online/master/check_packages.R")
```
If you want to follow along with the R code on this webpage, you can open the file `part1.Rmd` from the bottom-right corner of RStudio

There are equivalent markdown files (`part2.Rmd`, `part3.Rmd`) for the other sections of the course. Enjoy!
# Entering commands in R
The traditional way to enter R commands is via the Terminal, or using the console in RStudio (bottom-left panel when RStudio opens for first time). This doesn’t automatically keep track of the steps you did.
We'll be working in an [**R Notebook**](https://bookdown.org/yihui/rmarkdown/notebook.html). These file are an [R Markdown](https://bookdown.org/yihui/rmarkdown/) document type, which allow us to **combine R code with** [markdown](https://pandoc.org/MANUAL.html#pandocs-markdown), **a documentation language**, providing a framework for [literate programming](https://en.wikipedia.org/wiki/Literate_programming). In an R Notebook, R code chunks can be executed independently and interactively, with output visible immediately beneath the input.
Let’s try this now!
```{r}
print("Hello World")
```
R can be used as a calculator to compute simple sums
```{r}
2 + 2
2 - 2
4 * 3
10 / 2
```
The answer is displayed at the console with a `[1]` in front of it. The `1` inside the square brackets is a place-holder to signify how many values were in the answer (in this case only one). We will talk about dealing with lists of numbers shortly...
In the case of expressions involving multiple operations, R respects the [BODMAS](https://en.wikipedia.org/wiki/Order_of_operations#Mnemonics) system to decide the order in which operations should be performed.
```{r}
2 + 2 *3
2 + (2 * 3)
(2 + 2) * 3
```
R is capable of more complicated arithmetic such as trigonometry and logarithms; like you would find on a fancy scientific calculator. Of course, R also has a plethora of statistical operations as we will see.
```{r}
pi
sin (pi/2)
cos(pi)
tan(2)
log(1)
```
We can only go so far with performing simple calculations like this. Eventually we will need to store our results for later use. For this, we need to make use of *variables*.
## Variables
A variable is a letter or word which takes (or contains) a value. We
use the assignment 'operator', `<-` to create a variable and store some value in it.
```{r}
x <- 10
x
myNumber <- 25
myNumber
```
We also can perform arithmetic on variables using functions:
```{r}
sqrt(myNumber)
```
We can add variables together:
```{r}
x + myNumber
```
We can change the value of an existing variable:
```{r}
x <- 21
x
```
We can set one variable to equal the value of another variable:
```{r}
x <- myNumber
x
```
When we are feeling lazy we might give our variables short names (`x`, `y`, `i`...etc), but a better practice would be to give them meaningful names. There are some restrictions on creating variable names. They cannot start with a number or contain characters such as `.` and '-'. Naming variables the same as in-built functions in R, such as `c`, `T`, `mean` should also be avoided.
Naming variables is a matter of taste. Some [conventions](http://adv-r.had.co.nz/Style.html) exist such as a separating words with `-` or using *c*amel*C*aps. Whatever convention you decided, stick with it!
## Functions
**Functions** in R perform operations on **arguments** (the inputs(s) to the function). We have already used:
```{r}
sin(x)
```
this returns the sine of x. In this case the function has one argument: **x**. Arguments are always contained in parentheses -- curved brackets, **()** -- separated by commas.
Arguments can be named or unnamed, but if they are unnamed they must be ordered (we will see later how to find the right order). The names of the arguments are determined by the author of the function and can be found in the help page for the function. When testing code, it is easier and safer to name the arguments. `seq` is a function for generating a numeric sequence *from* and *to* particular numbers. Type `?seq` to get the help page for this function.
```{r}
seq(from = 3, to = 20, by = 4)
seq(3, 20, 4)
```
Arguments can have *default* values, meaning we do not need to specify values for these in order to run the function.
`rnorm` is a function that will generate a series of values from a *normal distribution*. In order to use the function, we need to tell R how many values we want
```{r}
## this will produce a random set of numbers, so everyone will get a different set of numbers
rnorm(n=10)
```
The normal distribution is defined by a *mean* (average) and *standard deviation* (spread). However, in the above example we didn't tell R what mean and standard deviation we wanted. So how does R know what to do? All arguments to a function and their default values are listed in the help page
(*N.B sometimes help pages can describe more than one function*)
```{r}
?rnorm
```
In this case, we see that the defaults for mean and standard deviation are 0 and 1. We can change the function to generate values from a distribution with a different mean and standard deviation using the `mean` and `sd` *arguments*. It is important that we get the spelling of these arguments exactly right, otherwise R will an error message, or (worse?) do something unexpected.
```{r}
rnorm(n=10, mean=2,sd=3)
rnorm(10, 2, 3)
```
In the examples above, `seq` and `rnorm` were both outputting a series of numbers, which is called a *vector* in R and is the most-fundamental data-type.
Just as we can save single numbers as a variable, we can also save a vector. In fact a single number is still a vector.
```{r}
my_seq <- seq(from = 3, to = 20, by = 4)
```
The arithmetic operations we have seen can be applied to these vectors; exactly the same as a single number.
```{r}
my_seq + 2
```
```{r}
my_seq * 2
```
******
******
******
### Exercise
<div class="exercise">
- What is the value of `pi` to 3 decimal places?
+ see the help for `round` `?round`
- How can we a create a sequence from 2 to 20 comprised of 5 equally-spaced numbers?
+ i.e. not specifying the `by` argument and getting R to work-out the intervals
+ check the help page for seq `?seq`
- Create a *variable* containing 1000 random numbers with a *mean* of 2 and a *standard deviation* of 3
+ what is the maximum and minimum of these numbers?
+ what is the average?
+ HINT: see the help pages for functions `min`, `max` and `mean`
</div>
```{r}
## Type your code to answer the exercises in here
```
******
******
******
## Saving your notebook
If you want to re-visit your code at any point, you will need to save a copy.
<div class="information">
**File > Save > **
</div>
## Packages in R
So far we have used functions that are available with the *base* distribution of R; the functions you get with a clean install of R. The open-source nature of R encourages others to write their own functions for their particular data-type or analyses.
Packages are distributed through *repositories*. The most-common ones are CRAN and Bioconductor. CRAN alone has many thousands of packages.
<div class="information">
- The [meta cran](https://www.r-pkg.org/) website can be used to browse packages available in CRAN
- Bioconductor packages can be browsed [here](http://bioconductor.org/packages/release/BiocViews.html#___Software)
</div>
CRAN and Bioconductor have some level of curation so should be the first place to look. Researchers sometimes make their packages available on [github](www.github.com). However, there is no straightforward way of searching github for a particular package and no guarentee of quality.
The **Packages** tab in the bottom-right panel of RStudio lists all packages that you currently have installed. Clicking on a package name will show a list of functions that available once that package has been loaded.
There are functions for installing packages within R. If your package is part of the main **CRAN** repository, you can use `install.packages`.
We will be using a set of `tidyverse` R packages in this practical. To install them, we would do.
```{r eval=FALSE}
## You should already have installed these as part of the course setup
install.packages("readr")
install.packages("ggplot2")
install.packages("dplyr")
# to install the entire set of tidyverse packages, we can do install.packages("tidyverse"). But this will take some time
```
A package may have several *dependencies*; other R packages from which it uses functions or data types (re-using code from other packages is strongly-encouraged). If this is the case, the other R packages will be located and installed too.
**So long as you stick with the same version of R, you won't need to repeat this install process.**
Once a package is installed, the `library` function is used to load a package and make it's functions / data available in your current R session. *You need to do this every time you load a new RStudio session*. Let's go ahead and load the `readr` so we can import some data.
```{r message=FALSE}
## readr is a packages to import spreadsheets into R
library(readr)
```
# Dealing with data
The [***tidyverse***](https://www.tidyverse.org/) is an eco-system of packages that provides a consistent, intuitive system for data manipulation and visualisation in R.
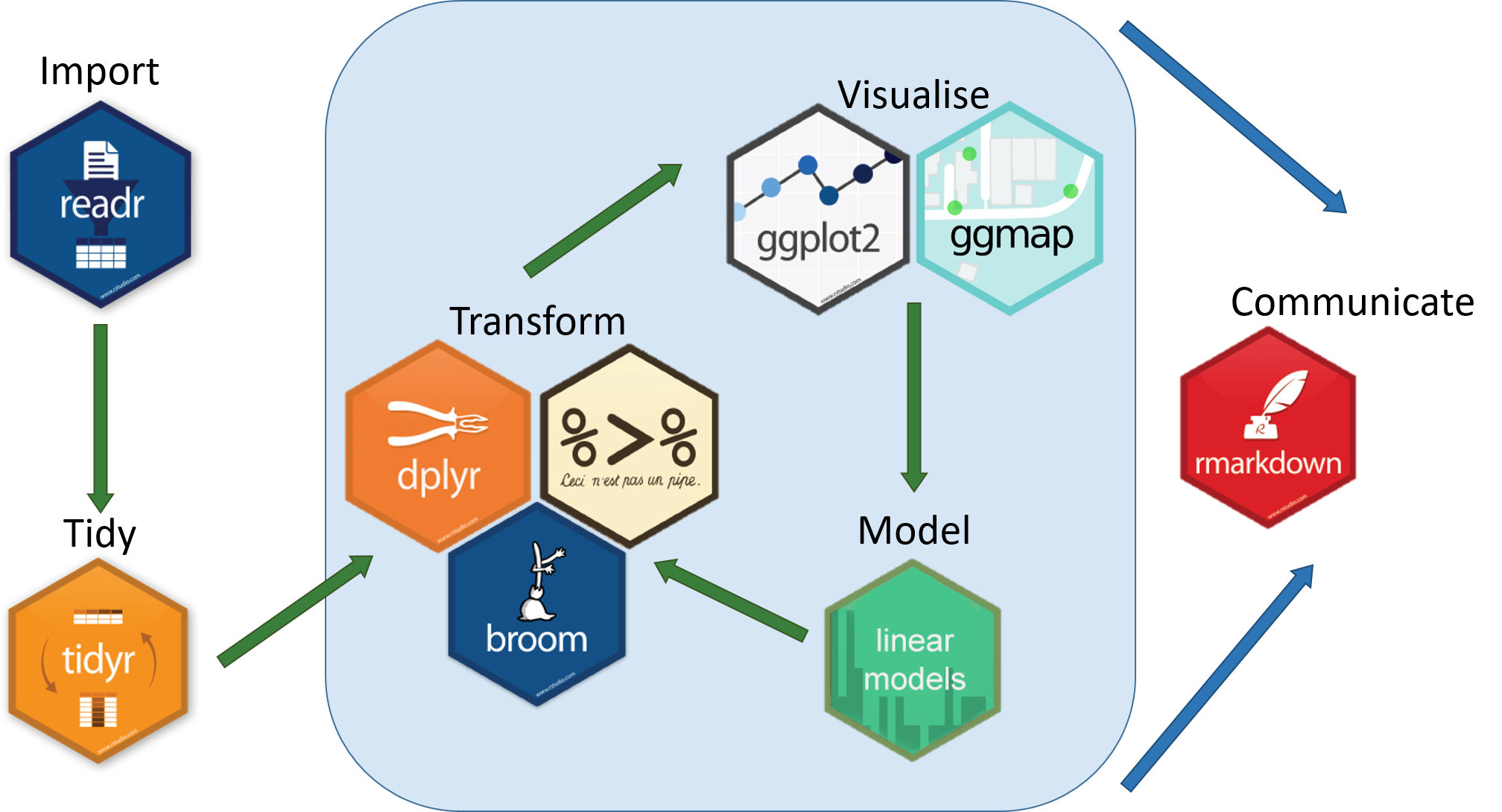
_Image Credit:_ [***Aberdeen Study Group***](https://aberdeenstudygroup.github.io/studyGroup/lessons/SG-T2-JointWorkshop/PopulationChangeSpeciesOccurrence/)
We are going to explore some of the basic features of the `tidyverse` using data from the [gapminder](https://www.gapminder.org/data/) project, which have been bundled into an [R package](https://github.com/jennybc/gapminder). These data give various indicator variables for different countries around the world (life expectancy, population and Gross Domestic Product). We have saved these data as a `.csv` file called `gapminder.csv` in a sub-directory called `raw_data/` to demonstrate how to import data into R.
## Reading in data
Any `.csv` file can be imported into R by supplying the path to the file to `readr` function `read_csv` and assigning it to a new object to store the result. A useful sanity check is the `file.exists` function which will print `TRUE` is the file can be found in the working directory.
```{r}
gapminder_path <- "raw_data/gapminder.csv"
file.exists(gapminder_path)
```
Assuming the file can be found, we can use `read_csv` to import. Other functions can be used to read tab-delimited files (`read_delim`) or a generic `read.table` function. A data frame object is created.
```{r}
library(readr)
gapminder_path <- "raw_data/gapminder.csv"
gapminder <- read_csv(gapminder_path)
```
<div class="warning">
**Why would specifying `gapminder_path` as **
`Users/Anna/Documents/workflows/workshops/r-crash-course/raw_data/gapminder.csv` **be a bad idea?** Would you be able to re-run the analysis on another machine?
</div>
<div class="information">
You can also read excel (`.xls` or `.xlsx`) files into R, but you will have to use the `readxl` package instead.
```{r eval=FALSE}
install.packages("readxl")
library(readxl)
## Replace PATH_TO_MY_XLS with the name of the file you want to read
data <- read_xls(PATH_TO_MY_XLS)
## Replace PATH_TO_MY_XLSX with the name of the file you want to read
data <- read_xlsx(PATH_TO_MY_XLSX)
```
</div>
<div class="information">
If you get *really* stuck importing data, there is a File -> Import Dataset option that should guide you through the process. It will also show the corresponding R code that you can use in future.
</div>
The data frame object in R allows us to work with **"tabular" data**, like we might be used to dealing with in Excel, where our data can be thought of having **rows and columns**. The values in **each column** have to all be of the **same type** (i.e. all numbers or all text).
In Rstudio, you can **view the contents of the data frame** we have just created using function `View()`. This is useful for interactive exploration of the data, but not so useful for automation, scripting and analyses.
```{r eval=FALSE}
## Make sure that you use a capital letter V
View(gapminder)
```
```{r, echo = FALSE}
gapminder
```
We should **always check the data frame that we have created**. Sometimes R will happily read data using an inappropriate function and create an object without raising an error. However, the data might be unusable. Consider:-
```{r}
test <- read_table(gapminder_path)
```
```{r, eval=F}
View(test)
```
```{r, echo = FALSE}
test
```
Quick sanity checks can also be performed by inspecting details in the environment tab. A useful check in RStudio is to use the `head` function, which prints the first 6 rows of the data frame to the screen.
```{r}
head(gapminder)
```
<div class="warning">
We have used a nice, clean, dataset as our example for the workshop. Other datasets out in the wild might not be so ameanable for analysis in R. If your data look like this, you might have problems:-
<img src="https://datacarpentry.org/2015-05-29-great-plains/spreadsheet-ecology/fig/2_datasheet_example.jpg"/>
We recommend the Data Carpentry materials on spreadsheet organisation for an overview of common pitfalls - and how to address them
- https://datacarpentry.org/spreadsheet-ecology-lesson/
</div>
## Accessing data in columns
In the next section we will explore in more detail how to control the columns and rows from a data frame that are displayed in RStudio. For now, accessing all the observations from a particular column can be achieved by typing the `$` symbol after the name of the data frame followed by the name of a column you are interested in.
RStudio is able to *"tab-complete"* the column name, so typing the following and pressing the **TAB** key will bring-up a list of possible columns. The contents of the column that you select are then printed to the screen.
```{r eval=FALSE}
gapminder$c
```
Rather than merely printing to the screen we can also create a variable
```{r}
years <- gapminder$year
```
We can then use some of the functions we have seen before
```{r}
min(years)
max(years)
median(years)
```
Although we don't have to save the values in the column as a variable first
```{r}
min(gapminder$year)
```
## Creating a new R notebook
You will probably want to create a new *R notebook* file to perform your analysis. This can be done by following the menus:-
<div class="information">
**File -> New File -> R notebook**
</div>
A new pane should open that includes some example code. You can delete everything **apart from lines 1 to 4**

You can now insert R code chunks using the insert menu.
Before generating a report you will need to save the file with the menu **File -> Save**. You will then be able to create a report using the Preview button. N.B. you may need to install extra software before doing this.
******
******
******
### Exercise before the next session
<div class="exercise">
- Create a new R notebook using the instructions above and create a code chunk to read the `gapminder.csv` file. Answer the following questions and generate a report
+ The function `tail` is similar to `head` except it prints the *last* lines in a file. Use this function to print the last *10* lines in the data frame (you will have to consult the help on `tail` to see how to change the default arguments.)
+ What is the largest observed population?
+ What is the lowest life expectancy
</div>
******
******
******
<div class="exercise">
(Optional): Familiarise yourself with the contents of the data frame. What numerical summaries can you produce from the dataset (e.g. average life expectancy per-year, countries that are most wealthy etc) and what plots might be of interest? Discuss with your neighbours
</div>