-
Notifications
You must be signed in to change notification settings - Fork 627
New issue
Have a question about this project? Sign up for a free GitHub account to open an issue and contact its maintainers and the community.
By clicking “Sign up for GitHub”, you agree to our terms of service and privacy statement. We’ll occasionally send you account related emails.
Already on GitHub? Sign in to your account
DeosGCF implementation #594
Open
deklanw
wants to merge
2
commits into
RUCAIBox:master
Choose a base branch
from
deklanw:deosgcf
base: master
Could not load branches
Branch not found: {{ refName }}
Loading
Could not load tags
Nothing to show
Loading
Are you sure you want to change the base?
Some commits from the old base branch may be removed from the timeline,
and old review comments may become outdated.
Open
Changes from all commits
Commits
Show all changes
2 commits
Select commit
Hold shift + click to select a range
File filter
Filter by extension
Conversations
Failed to load comments.
Loading
Jump to
Jump to file
Failed to load files.
Loading
Diff view
Diff view
There are no files selected for viewing
This file contains bidirectional Unicode text that may be interpreted or compiled differently than what appears below. To review, open the file in an editor that reveals hidden Unicode characters.
Learn more about bidirectional Unicode characters
This file contains bidirectional Unicode text that may be interpreted or compiled differently than what appears below. To review, open the file in an editor that reveals hidden Unicode characters.
Learn more about bidirectional Unicode characters
Original file line number | Diff line number | Diff line change |
---|---|---|
@@ -0,0 +1,213 @@ | ||
import scipy.sparse as sp | ||
import numpy as np | ||
import torch | ||
|
||
from recbole.utils import InputType | ||
from recbole.model.abstract_recommender import GeneralRecommender | ||
from recbole.model.loss import BPRLoss, EmbLoss | ||
from recbole.model.init import xavier_uniform_initialization | ||
|
||
|
||
def scipy_to_sparse_tensor(A): | ||
# https://stackoverflow.com/a/50665264/7367514 | ||
C = A.tocoo() | ||
|
||
values = C.data | ||
indices = np.vstack((C.row, C.col)) | ||
|
||
i = torch.LongTensor(indices) | ||
v = torch.FloatTensor(values) | ||
shape = C.shape | ||
|
||
return torch.sparse.FloatTensor(i, v, torch.Size(shape)) | ||
|
||
|
||
def adjacency_of_bipartite(B): | ||
m, n = B.shape | ||
|
||
Z1 = sp.coo_matrix((m, m)) | ||
Z2 = sp.coo_matrix((n, n)) | ||
|
||
A = sp.bmat([[Z1, B], [B.T, Z2]]) | ||
|
||
return A | ||
|
||
|
||
def get_symmetric_normalized(A): | ||
# add epsilon to degree sums to suppress warning about division by zero | ||
degree_sums = A.sum(axis=1).getA1() + 1e-7 | ||
|
||
D = sp.diags(np.power(degree_sums, -1/2)) | ||
|
||
return D * A * D | ||
|
||
|
||
def get_symm_norm_tensor(A): | ||
return scipy_to_sparse_tensor(get_symmetric_normalized(A)) | ||
|
||
|
||
|
||
class SparseDropout(torch.nn.Module): | ||
""" | ||
This is a Module that execute Dropout on Pytorch sparse tensor. | ||
""" | ||
|
||
def __init__(self, p=0.5): | ||
super().__init__() | ||
# p is ratio of dropout | ||
# convert to keep probability | ||
self.kprob = 1 - p | ||
|
||
def forward(self, x): | ||
if not self.training: | ||
return x | ||
|
||
mask = ((torch.rand(x._values().size()) + | ||
self.kprob).floor()).type(torch.bool) | ||
rc = x._indices()[:, mask] | ||
val = x._values()[mask] * (1.0 / self.kprob) | ||
return torch.sparse.FloatTensor(rc, val, x.shape) | ||
|
||
|
||
class DeosGCF(GeneralRecommender): | ||
input_type = InputType.PAIRWISE | ||
|
||
def __init__(self, config, dataset): | ||
super().__init__(config, dataset) | ||
|
||
# load dataset info | ||
self.interaction_matrix = dataset.inter_matrix( | ||
form='coo').astype(np.float32) | ||
|
||
# load parameters info | ||
self.latent_dim = config['embedding_size'] | ||
self.n_layers = config['n_layers'] | ||
self.reg_weight = config['reg_weight'] | ||
self.dropout_prob = config['dropout_prob'] | ||
|
||
# define layers and loss | ||
self.user_embedding = torch.nn.Embedding( | ||
num_embeddings=self.n_users, embedding_dim=self.latent_dim) | ||
self.item_embedding = torch.nn.Embedding( | ||
num_embeddings=self.n_items, embedding_dim=self.latent_dim) | ||
|
||
# will get a warning about this ParameterList ".training" attr getting set until this goes through | ||
# https://github.com/pytorch/pytorch/pull/48315 | ||
self.la_layer_params = torch.nn.ParameterList([torch.nn.Parameter( | ||
torch.ones(self.n_users + self.n_items)) for _ in range(self.n_layers)]) | ||
|
||
# xavier init the LA layer params | ||
# not sure this even makes sense, but imitating the existing TF implementation | ||
# https://github.com/JimLiu96/DeosciRec/blob/b3575da96908d062fcf23a0d4fd5f3e3f082573d/DGCF_osci.py#L146 | ||
# since there is no defined "fanout" have to do this manually | ||
# i.e., "Fan in and fan out can not be computed for tensor with fewer than 2 dimensions" | ||
uniform_range = np.sqrt(6/(self.n_users+self.n_items+1)) | ||
for weights in self.la_layer_params: | ||
torch.nn.init.uniform_(weights, -uniform_range, uniform_range) | ||
|
||
self.sparse_dropout = SparseDropout(p=self.dropout_prob) | ||
|
||
self.mf_loss = BPRLoss() | ||
self.reg_loss = EmbLoss() | ||
|
||
# storage variables for full sort evaluation acceleration | ||
self.restore_user_e = None | ||
self.restore_item_e = None | ||
|
||
# generate intermediate data | ||
A = adjacency_of_bipartite(self.interaction_matrix) | ||
norm_adj_matrix = get_symm_norm_tensor(A).to(self.device) | ||
norm_crosshop_matrix = get_symm_norm_tensor(A**2).to(self.device) | ||
self.A_hat = norm_adj_matrix + norm_crosshop_matrix | ||
There was a problem hiding this comment. Choose a reason for hiding this commentThe reason will be displayed to describe this comment to others. Learn more. |
||
|
||
# parameters initialization | ||
self.apply(xavier_uniform_initialization) | ||
|
||
def get_ego_embeddings(self): | ||
r"""Get the embedding of users and items and combine to an embedding matrix. | ||
|
||
Returns: | ||
Tensor of the embedding matrix. Shape of [n_items+n_users, embedding_dim] | ||
""" | ||
user_embeddings = self.user_embedding.weight | ||
item_embeddings = self.item_embedding.weight | ||
ego_embeddings = torch.cat([user_embeddings, item_embeddings], dim=0) | ||
return ego_embeddings | ||
|
||
def forward(self): | ||
all_embeddings = self.get_ego_embeddings() | ||
embeddings_list = [all_embeddings] | ||
|
||
dropped_out_A_hat = self.sparse_dropout(self.A_hat) | ||
|
||
for layer_idx in range(self.n_layers): | ||
la_params = self.la_layer_params[layer_idx] | ||
la_diag_matrix = torch.diag(torch.sigmoid(la_params)) | ||
weighted_embedding = torch.mm(la_diag_matrix, all_embeddings) | ||
|
||
all_embeddings = torch.sparse.mm( | ||
dropped_out_A_hat, weighted_embedding) | ||
|
||
embeddings_list.append(all_embeddings) | ||
|
||
stacked_embeddings = torch.stack(embeddings_list, dim=1) | ||
mean_embeddings = torch.mean(stacked_embeddings, dim=1) | ||
|
||
user_all_embeddings, item_all_embeddings = torch.split( | ||
mean_embeddings, [self.n_users, self.n_items]) | ||
|
||
return user_all_embeddings, item_all_embeddings | ||
|
||
def calculate_loss(self, interaction): | ||
# clear the storage variable when training | ||
if self.restore_user_e is not None or self.restore_item_e is not None: | ||
self.restore_user_e, self.restore_item_e = None, None | ||
|
||
user = interaction[self.USER_ID] | ||
pos_item = interaction[self.ITEM_ID] | ||
neg_item = interaction[self.NEG_ITEM_ID] | ||
|
||
user_all_embeddings, item_all_embeddings = self.forward() | ||
u_embeddings = user_all_embeddings[user] | ||
posi_embeddings = item_all_embeddings[pos_item] | ||
negi_embeddings = item_all_embeddings[neg_item] | ||
|
||
# calculate BPR Loss | ||
pos_scores = torch.mul(u_embeddings, posi_embeddings).sum(dim=1) | ||
neg_scores = torch.mul(u_embeddings, negi_embeddings).sum(dim=1) | ||
mf_loss = self.mf_loss(pos_scores, neg_scores) | ||
|
||
# calculate BPR Loss | ||
u_ego_embeddings = self.user_embedding(user) | ||
posi_ego_embeddings = self.item_embedding(pos_item) | ||
negi_ego_embeddings = self.item_embedding(neg_item) | ||
|
||
reg_loss = self.reg_loss( | ||
u_ego_embeddings, posi_ego_embeddings, negi_ego_embeddings) | ||
loss = mf_loss + self.reg_weight * reg_loss | ||
|
||
return loss | ||
|
||
def predict(self, interaction): | ||
user = interaction[self.USER_ID] | ||
item = interaction[self.ITEM_ID] | ||
|
||
user_all_embeddings, item_all_embeddings = self.forward() | ||
|
||
u_embeddings = user_all_embeddings[user] | ||
i_embeddings = item_all_embeddings[item] | ||
scores = torch.mul(u_embeddings, i_embeddings).sum(dim=1) | ||
return scores | ||
|
||
def full_sort_predict(self, interaction): | ||
user = interaction[self.USER_ID] | ||
if self.restore_user_e is None or self.restore_item_e is None: | ||
self.restore_user_e, self.restore_item_e = self.forward() | ||
# get user embedding from storage variable | ||
u_embeddings = self.restore_user_e[user] | ||
|
||
# dot with all item embedding to accelerate | ||
scores = torch.matmul( | ||
u_embeddings, self.restore_item_e.transpose(0, 1)) | ||
|
||
return scores.view(-1) |
This file contains bidirectional Unicode text that may be interpreted or compiled differently than what appears below. To review, open the file in an editor that reveals hidden Unicode characters.
Learn more about bidirectional Unicode characters
Original file line number | Diff line number | Diff line change |
---|---|---|
@@ -0,0 +1,4 @@ | ||
embedding_size: 64 | ||
n_layers: 2 | ||
reg_weight: 1e-02 | ||
dropout_prob: 0.1 |
Add this suggestion to a batch that can be applied as a single commit.
This suggestion is invalid because no changes were made to the code.
Suggestions cannot be applied while the pull request is closed.
Suggestions cannot be applied while viewing a subset of changes.
Only one suggestion per line can be applied in a batch.
Add this suggestion to a batch that can be applied as a single commit.
Applying suggestions on deleted lines is not supported.
You must change the existing code in this line in order to create a valid suggestion.
Outdated suggestions cannot be applied.
This suggestion has been applied or marked resolved.
Suggestions cannot be applied from pending reviews.
Suggestions cannot be applied on multi-line comments.
Suggestions cannot be applied while the pull request is queued to merge.
Suggestion cannot be applied right now. Please check back later.
There was a problem hiding this comment.
Choose a reason for hiding this comment
The reason will be displayed to describe this comment to others. Learn more.
There is a high-pass filtering on the corss-hop metrix which greatly improves the efficiency. It should also be implemented.
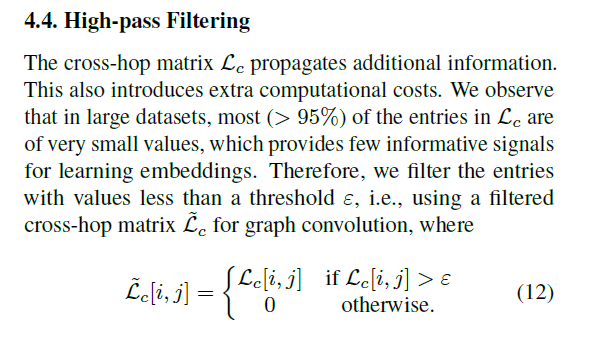