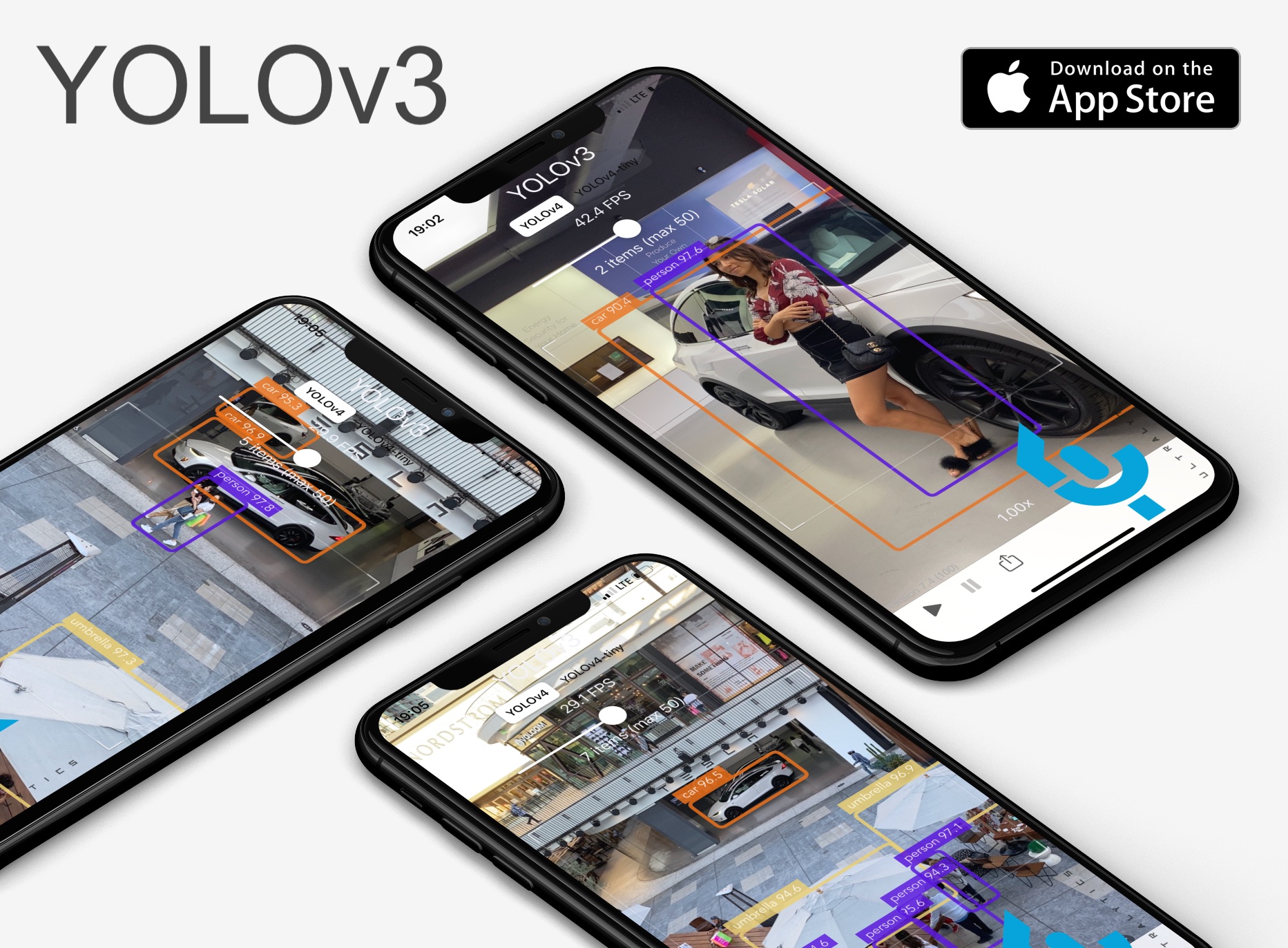
This repo contains Ultralytics inference and training code for YOLOv3 in PyTorch. The code works on Linux, MacOS and Windows. Credit to Joseph Redmon for YOLO https://pjreddie.com/darknet/yolo/.
Python 3.8 or later with all requirements.txt dependencies installed, including torch>=1.6
. To install run:
$ pip install -r requirements.txt
- Notebook
- Train Custom Data << highly recommended
- GCP Quickstart
- Docker Quickstart Guide
- A TensorRT Implementation of YOLOv3 and YOLOv4
Start Training: python3 train.py
to begin training after downloading COCO data with data/get_coco2017.sh
. Each epoch trains on 117,263 images from the train and validate COCO sets, and tests on 5000 images from the COCO validate set.
Resume Training: python3 train.py --resume
to resume training from weights/last.pt
.
Plot Training: from utils import utils; utils.plot_results()
datasets.py
applies OpenCV-powered (https://opencv.org/) augmentation to the input image. We use a mosaic dataloader to increase image variability during training.
https://cloud.google.com/deep-learning-vm/
Machine type: preemptible n1-standard-8 (8 vCPUs, 30 GB memory)
CPU platform: Intel Skylake
GPUs: K80 ($0.14/hr), T4 ($0.11/hr), V100 ($0.74/hr) CUDA with Nvidia Apex FP16/32
HDD: 300 GB SSD
Dataset: COCO train 2014 (117,263 images)
Model: yolov3-spp.cfg
Command: python3 train.py --data coco2017.data --img 416 --batch 32
GPU | n | --batch-size |
img/s | epoch time |
epoch cost |
---|---|---|---|---|---|
K80 | 1 | 32 x 2 | 11 | 175 min | $0.41 |
T4 | 1 2 |
32 x 2 64 x 1 |
41 61 |
48 min 32 min |
$0.09 $0.11 |
V100 | 1 2 |
32 x 2 64 x 1 |
122 178 |
16 min 11 min |
$0.21 $0.28 |
2080Ti | 1 2 |
32 x 2 64 x 1 |
81 140 |
24 min 14 min |
- - |
python3 detect.py --source ...
- Image:
--source file.jpg
- Video:
--source file.mp4
- Directory:
--source dir/
- Webcam:
--source 0
- RTSP stream:
--source rtsp://170.93.143.139/rtplive/470011e600ef003a004ee33696235daa
- HTTP stream:
--source http://112.50.243.8/PLTV/88888888/224/3221225900/1.m3u8
YOLOv3: python3 detect.py --cfg cfg/yolov3.cfg --weights yolov3.pt
YOLOv3-tiny: python3 detect.py --cfg cfg/yolov3-tiny.cfg --weights yolov3-tiny.pt
YOLOv3-SPP: python3 detect.py --cfg cfg/yolov3-spp.cfg --weights yolov3-spp.pt
Download from: https://drive.google.com/open?id=1LezFG5g3BCW6iYaV89B2i64cqEUZD7e0
$ git clone https://github.com/ultralytics/yolov3 && cd yolov3
# convert darknet cfg/weights to pytorch model
$ python3 -c "from models import *; convert('cfg/yolov3-spp.cfg', 'weights/yolov3-spp.weights')"
Success: converted 'weights/yolov3-spp.weights' to 'weights/yolov3-spp.pt'
# convert cfg/pytorch model to darknet weights
$ python3 -c "from models import *; convert('cfg/yolov3-spp.cfg', 'weights/yolov3-spp.pt')"
Success: converted 'weights/yolov3-spp.pt' to 'weights/yolov3-spp.weights'
Size | COCO mAP @0.5...0.95 |
COCO mAP @0.5 |
|
---|---|---|---|
YOLOv3-tiny YOLOv3 YOLOv3-SPP YOLOv3-SPP-ultralytics |
320 | 14.0 28.7 30.5 37.7 |
29.1 51.8 52.3 56.8 |
YOLOv3-tiny YOLOv3 YOLOv3-SPP YOLOv3-SPP-ultralytics |
416 | 16.0 31.2 33.9 41.2 |
33.0 55.4 56.9 60.6 |
YOLOv3-tiny YOLOv3 YOLOv3-SPP YOLOv3-SPP-ultralytics |
512 | 16.6 32.7 35.6 42.6 |
34.9 57.7 59.5 62.4 |
YOLOv3-tiny YOLOv3 YOLOv3-SPP YOLOv3-SPP-ultralytics |
608 | 16.6 33.1 37.0 43.1 |
35.4 58.2 60.7 62.8 |
- [email protected] run at
--iou-thr 0.5
, [email protected] run at--iou-thr 0.7
- Darknet results: https://arxiv.org/abs/1804.02767
$ python3 test.py --cfg yolov3-spp.cfg --weights yolov3-spp-ultralytics.pt --img 640 --augment
Namespace(augment=True, batch_size=16, cfg='cfg/yolov3-spp.cfg', conf_thres=0.001, data='coco2014.data', device='', img_size=640, iou_thres=0.6, save_json=True, single_cls=False, task='test', weights='weight
Using CUDA device0 _CudaDeviceProperties(name='Tesla V100-SXM2-16GB', total_memory=16130MB)
Class Images Targets P R [email protected] F1: 100%|█████████| 313/313 [03:00<00:00, 1.74it/s]
all 5e+03 3.51e+04 0.375 0.743 0.64 0.492
Average Precision (AP) @[ IoU=0.50:0.95 | area= all | maxDets=100 ] = 0.456
Average Precision (AP) @[ IoU=0.50 | area= all | maxDets=100 ] = 0.647
Average Precision (AP) @[ IoU=0.75 | area= all | maxDets=100 ] = 0.496
Average Precision (AP) @[ IoU=0.50:0.95 | area= small | maxDets=100 ] = 0.263
Average Precision (AP) @[ IoU=0.50:0.95 | area=medium | maxDets=100 ] = 0.501
Average Precision (AP) @[ IoU=0.50:0.95 | area= large | maxDets=100 ] = 0.596
Average Recall (AR) @[ IoU=0.50:0.95 | area= all | maxDets= 1 ] = 0.361
Average Recall (AR) @[ IoU=0.50:0.95 | area= all | maxDets= 10 ] = 0.597
Average Recall (AR) @[ IoU=0.50:0.95 | area= all | maxDets=100 ] = 0.666
Average Recall (AR) @[ IoU=0.50:0.95 | area= small | maxDets=100 ] = 0.492
Average Recall (AR) @[ IoU=0.50:0.95 | area=medium | maxDets=100 ] = 0.719
Average Recall (AR) @[ IoU=0.50:0.95 | area= large | maxDets=100 ] = 0.810
Speed: 17.5/2.3/19.9 ms inference/NMS/total per 640x640 image at batch-size 16
Run commands below. Training takes about one week on a 2080Ti per model.
$ python train.py --data coco2014.data --weights '' --batch-size 16 --cfg yolov3-spp.cfg
$ python train.py --data coco2014.data --weights '' --batch-size 32 --cfg yolov3-tiny.cfg
To access an up-to-date working environment (with all dependencies including CUDA/CUDNN, Python and PyTorch preinstalled), consider a:
- GCP Deep Learning VM with $300 free credit offer: See our GCP Quickstart Guide
- Google Colab Notebook with 12 hours of free GPU time.
- Docker Image https://hub.docker.com/r/ultralytics/yolov3. See Docker Quickstart Guide
Ultralytics is a U.S.-based particle physics and AI startup with over 6 years of expertise supporting government, academic and business clients. We offer a wide range of vision AI services, spanning from simple expert advice up to delivery of fully customized, end-to-end production solutions, including:
- Cloud-based AI systems operating on hundreds of HD video streams in realtime.
- Edge AI integrated into custom iOS and Android apps for realtime 30 FPS video inference.
- Custom data training, hyperparameter evolution, and model exportation to any destination.
For business inquiries and professional support requests please visit us at https://www.ultralytics.com.
Issues should be raised directly in the repository. For business inquiries or professional support requests please visit https://www.ultralytics.com or email Glenn Jocher at [email protected].