-
Notifications
You must be signed in to change notification settings - Fork 360
Commit
This commit does not belong to any branch on this repository, and may belong to a fork outside of the repository.
- Loading branch information
1 parent
5bc43f0
commit da5fe3f
Showing
14 changed files
with
548 additions
and
188 deletions.
There are no files selected for viewing
This file contains bidirectional Unicode text that may be interpreted or compiled differently than what appears below. To review, open the file in an editor that reveals hidden Unicode characters.
Learn more about bidirectional Unicode characters
Original file line number | Diff line number | Diff line change |
---|---|---|
@@ -0,0 +1,31 @@ | ||
name: Pre-commit Check | ||
|
||
on: | ||
push: | ||
paths: | ||
- 'sql_agent/**' # 检测sql_agent 目录下所有文件 | ||
|
||
jobs: | ||
pre-commit-check: | ||
name: Pre-commit Check | ||
runs-on: ubuntu-latest | ||
|
||
steps: | ||
- name: Checkout code | ||
uses: actions/checkout@v2 | ||
|
||
- name: Set up Python | ||
uses: actions/setup-python@v2 | ||
with: | ||
python-version: '3.10' # 你的项目所需的 Python 版本 | ||
|
||
- name: Install pre-commit | ||
run: pip install pre-commit | ||
|
||
- name: Run pre-commit | ||
run: pre-commit run --all-files | ||
|
||
# 如果检查失败,你可以选择终止 workflow 并将状态设置为失败 | ||
# - name: Fail workflow on pre-commit check failure | ||
# if: ${{ failure() }} | ||
# run: exit 1 |
This file contains bidirectional Unicode text that may be interpreted or compiled differently than what appears below. To review, open the file in an editor that reveals hidden Unicode characters.
Learn more about bidirectional Unicode characters
Original file line number | Diff line number | Diff line change |
---|---|---|
|
@@ -8,7 +8,7 @@ | |
# Env | ||
.env | ||
venv/ | ||
|
||
build/ | ||
test/ | ||
# project | ||
poetry.lock |
This file contains bidirectional Unicode text that may be interpreted or compiled differently than what appears below. To review, open the file in an editor that reveals hidden Unicode characters.
Learn more about bidirectional Unicode characters
Original file line number | Diff line number | Diff line change |
---|---|---|
@@ -0,0 +1,32 @@ | ||
# .readthedocs.yaml | ||
# Read the Docs configuration file | ||
# See https://docs.readthedocs.io/en/stable/config-file/v2.html for details | ||
|
||
# Required | ||
version: 2 | ||
|
||
# Set the OS, Python version and other tools you might need | ||
build: | ||
os: ubuntu-22.04 | ||
tools: | ||
python: "3.12" | ||
# You can also specify other tool versions: | ||
# nodejs: "19" | ||
# rust: "1.64" | ||
# golang: "1.19" | ||
|
||
# Build documentation in the "docs/" directory with Sphinx | ||
sphinx: | ||
configuration: docs/source/conf.py | ||
|
||
# Optionally build your docs in additional formats such as PDF and ePub | ||
# formats: | ||
# - epub | ||
|
||
# Optional but recommended, declare the Python requirements required | ||
# to build your documentation | ||
# See https://docs.readthedocs.io/en/stable/guides/reproducible-builds.html | ||
python: | ||
install: | ||
- requirements: docs/requirements.txt |
This file contains bidirectional Unicode text that may be interpreted or compiled differently than what appears below. To review, open the file in an editor that reveals hidden Unicode characters.
Learn more about bidirectional Unicode characters
Original file line number | Diff line number | Diff line change |
---|---|---|
@@ -1,180 +1,72 @@ | ||
# SQLAGENT | ||
|
||
<img src="/asset/robot.jpeg" width = "300" height = "300" alt="图片名称" align=center /> | ||
|
||
## 总览 | ||
SQLAgent 是一个 **开源的(Open source)、大模型驱动的(LLM-Powered)、专注于私有化部署的Text2SQL 智能体(Agent)** 项目(Project),我们的目标是提供产品级的Text2SQL解决方案,致力于解决Text2SQL在实际应用中遇到的各种问题如模型私有化部署、面向Text2SQL任务的RAG最佳方案等等。为此,我们将持续探索什么是Text2SQL在实际应用中的最佳实践。 | ||
> SQLAgent可以简单的理解为Text2SQL + LLM-Powered Agent,是大模型驱动的面向Text2SQL任务的智能体。基于SQLAgent,你可以通过只调用几个服务接口来快速的开发属于自己的Text2SQL产品,且你只需要专注于你的业务应用,SQLAgent会以一种经过实验数据证明是最合理的方式来帮你解决那些关于Text2SQL的技术难题。 | ||
> Text2SQL(Text-to-SQL),顾名思义,即将自然语言转化为SQL,更为学术的定义是将以自然语言表达的数据库领域的问题转化为可执行的结构化查询语句。 | ||
> Agent,在本项目中更准确的叫法是LLM-Powered Agent,即⼤语⾔模型驱动的智能代理,是以LLM 作为⼤脑,可感知环境,具备任务规划、记忆、工具调用等能力的一组计算机程序(参考 https://lilianweng.github.io/posts/2023-06-23-agent/ )。 | ||
## 为什么选择SQLAgent | ||
与那些Text2SQL开发框架(Text2SQL Framework)、具备大模型聊天功能的数据库客户端等项目不同的是,SQLAgent项目的目标是一个支持完全私有化部署、专门面向Text2SQL任务的智能体,这意味着基于SQLAgent来开发产品你将 **无需收集数据来微调模型、无需开展繁琐的Prompt工程、无需关心如何组织数据来实现RAG、更无需关心大模型的Token费用等等问题** ,因为这一切都是由SQLAgent来提供。你可以将SQLAgent当做一个真正SQL专家,尽管问它问题即可! | ||
|
||
| 项目 | 举例 | 目标 | | ||
|----|----|----------------------------------------------------------------------------------------------------------------------------------------------------------------------------------------------------------| | ||
| Text2SQL 开发框架 | DB-GPT、Vanna-AI等 | 面向Text2SQL任务提供一系列开发工具集以便利产品开发。这类项目通常提供: <br/>- 调用各种LLM的接口 <br/>- 调用各种Embedding模型的接口 <br/> - 调用各种向量数据库的接口 <br/> - 访问各种数据库的接口 <br/>... | | ||
| 具备大模型聊天功能的数据库客户端 | Sqlchat、chat2db等 | 在数据库客户端的基础上叠加大模型对话功能,这类项目通常提供: <br/> - 调用各种LLM的接口 <br/>- 访问各种数据库的接口以及用户界面 <br/>... | | ||
| SQLAgent | SQLAgent | 提供产品级的Text2SQL解决方案,我们提供: <br/> - 经过专门为生成SQL而微调LLM(langcode-sql-coder-34B),不仅仅是LLM接口! <br/>- 针对表结构、Gold SQL、指标计算公式等数据对象的高性能RAG的算法,百万检索小于1s,召回率大于95%!不仅仅是对接向量数据库的接口! <br/>- 基于vLL的多卡推理的解决方案! <br/>... | | ||
|
||
## 架构设计 | ||
|
||
## 特性 | ||
- **Text2SQL LLM**:基于CodeLlama-34b-Instruct模型进行微调得到的专门用于SQL生成的模型langcode-sql-coder-34B。 | ||
- **RAG**:针对表结构、Gold SQL、指标计算公式等数据对象的高性能RAG的算法,百万数据检索小于1s,召回率大于95%。 | ||
- **多卡推理**:基于vLL的多卡推理的部署方案。 | ||
- **意图理解**:通过引导式问答来理解用户意图。 | ||
- ... | ||
|
||
## 未来的工作 | ||
- **SQL验证器**:对生成的SQL进行语法检查,对生成错误的SQL会根据错误信息让LLM重新生成。 | ||
- **日志服务**:独立的日志服务。 | ||
- **图表生成**:根据SQL查询的结果动态生成图表。 | ||
|
||
## 推理性能 | ||
 | ||
|
||
## 在线演示系统 | ||
即将上线、敬请期待..... | ||
|
||
## 如何开始 | ||
### SQLAgent部署 | ||
#### 资源要求 | ||
CPU:8核 | ||
内存:32GB+ | ||
#### 环境要求 | ||
项目存储依赖mongodb,在开始安装之前需要提前安装 | ||
#### 源码部署 | ||
|
||
> SQLAgent依赖Python >= 3.10 | ||
1、[可选] 创建conda环境 | ||
|
||
conda create -n sqlagent python=3.10 | ||
conda activate sqlagent | ||
|
||
2、可以通过运行以下命令来下载源码和安装依赖: | ||
|
||
#源码下载 | ||
git clone https://github.com/hitsz-ids/SQLAgent.git`` | ||
cd SQLAgent | ||
# 安装依赖 | ||
安装poetry | ||
python -m pip install poetry | ||
安装依赖 | ||
poetry install | ||
|
||
|
||
|
||
3、创建``.env``文件,可以从``.env_template``文件复制 | ||
|
||
cp .env_template .env | ||
|
||
4、请根据实际情况对以下变量进行配置 | ||
|
||
# mongodb 配置 | ||
MONGODB_URI="mongodb://localhost:27017" | ||
MONGODB_DB_NAME='sql_agent' | ||
MONGODB_DB_USERNAME='' | ||
MONGODB_DB_PASSWORD='' | ||
# OpenAI 配置,留空即可 | ||
OPENAI_KEY='' | ||
MODEL_NAME='' | ||
# [可选]embedding 模型名称 默认使用 infgrad/stella-large-zh-v2 | ||
EMBEDDINGS_MODEL_NAME='infgrad/stella-large-zh-v2' | ||
# [可选]embedding 模型名称 默认使用 infgrad/stella-large-zh-v2 | ||
EMBEDDINGS_MODEL_NAME='infgrad/stella-large-zh-v2' | ||
|
||
5、启动服务 | ||
|
||
python startup.py | ||
|
||
#### Docker部署 | ||
|
||
1、构建镜像 | ||
|
||
bash docker/build.sh | ||
|
||
2、创建embedding模型存储目录 | ||
|
||
mkdir -p /data/huggingface | ||
|
||
3、启动容器 | ||
|
||
docker run -idt --privileged=true \ | ||
-p 8888:8888 \ | ||
-v /data/huggingface:/root/.cache/huggingface \ | ||
-e LLM_SQL_ORIGIN=http://xxx.xxx.xxx.xxx:8000 \ # 按实际情况替换 | ||
-e MONGODB_URI=mongodb://xxx.xxx.xxx.xxx:27017 \ # 按实际情况替换 | ||
-e MONGODB_DB_NAME=sqlagent \ | ||
-e MONGODB_DB_USERNAME=xxx \ # 按实际情况替换 | ||
-e MONGODB_DB_PASSWORD=xxx \ # 按实际情况替换 | ||
-e EMBEDDINGS_MODEL_NAME=infgrad/stella-large-zh-v2 \ | ||
--restart always \ | ||
--name sqlagent \ | ||
langcode/sqlagent:Alpha | ||
|
||
## 📖 介绍 | ||
DataAgent是面向数据分析的多智能体,能够理解数据开发和数据分析需求、理解数据、生成面向数据查询、数据可视化、机器学习等任务的SQL和Python代码。 | ||
### 特性: | ||
|
||
- **精准数据检索**:DataAgent具有强大的数据处理和搜索能力,可以从成百上千张表中精准找数,满足您在大数据环境下的数据查找需求。 | ||
- **业务知识理解**:DataAgent不仅能处理数据,还深入理解数据指标、计算公式等业务知识,为您提供更深层次、更具业务价值的数据分析。 | ||
- **多智能体协同工作**:DataAgent采用面向数据分析需求的多轮对话设计,多智能体可以协同工作,进行数据分析代码的self-debug,提升分析效率,降低错误率。 | ||
- **数据可视化**:DataAgent可以将复杂的数据通过可视化的方式呈现,让数据分析结果更易于理解,帮助您更好地做出决策。 | ||
### DataAgent工作流程: | ||
**1. 需求确认:**DataAgent与用户建立对话,理解用户的需求。在这一阶段,DataAgent会提出一系列问题,以便更准确地了解用户的需求。 | ||
**2. 任务规划:**DataAgent会根据最终确认的需求内容为用户制定任务规划。这个规划包括一系列步骤,DataAgent会按照这些步骤来为用户提供服务。 | ||
**3. 任务执行:**DataAgent将规划好的任务分配给不同的智能体,如数据查找智能体、SQL生成智能体、代码生成智能体、可视化分析智能体等。每个智能体负责其专业领域的任务执行,协同工作以确保任务的高效完成。 | ||
**4.应用生成:**DataAgent根据用户需求任务将结果数据转化为应用成果,如指标大屏展示、数据API服务和数据应用等,这些成果能够以可视化的形式展示关键数据指标,提供API接口供其他系统或服务调用,以及根据用户需求生成具体的应用程序。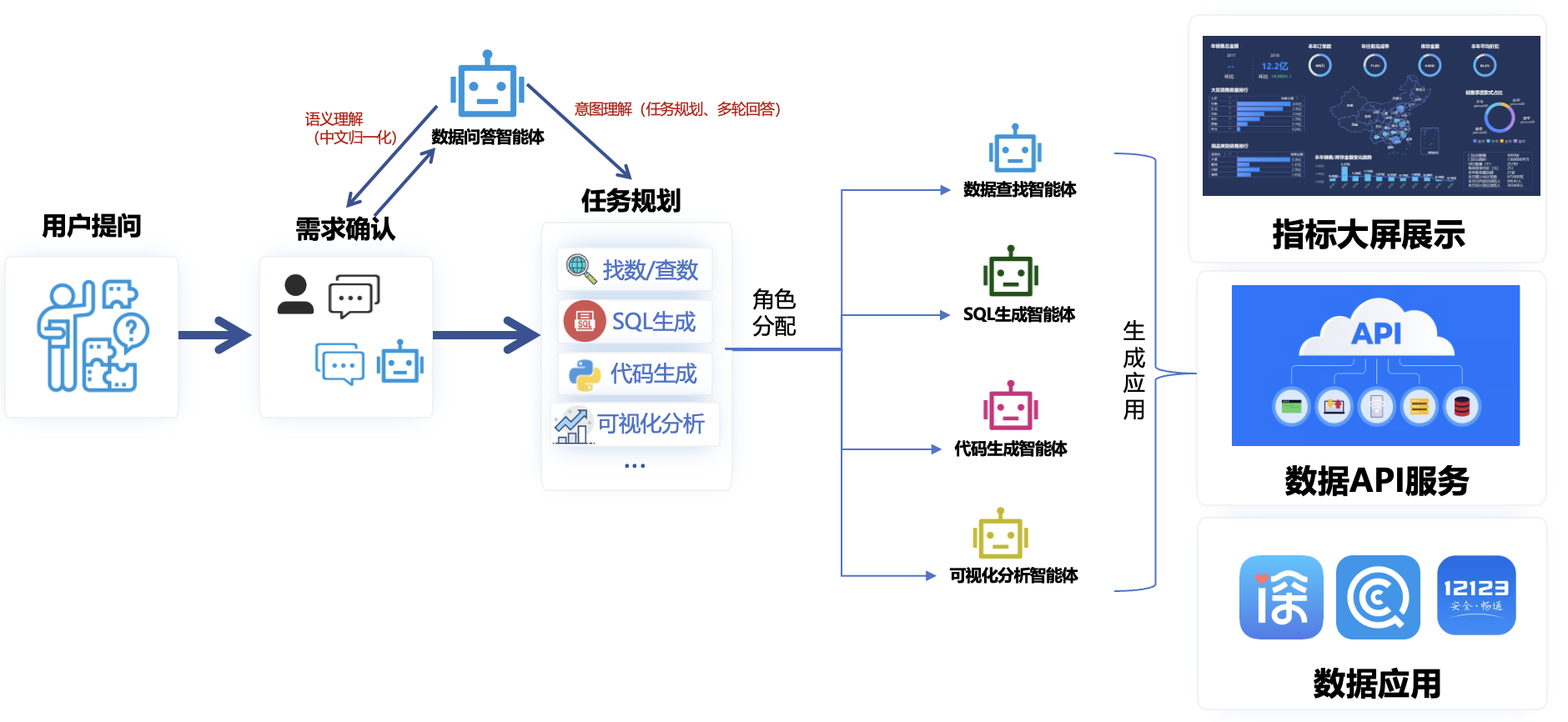 | ||
DataAgent的工作流程图 | ||
### 完成进度: | ||
|
||
- [x] SQL生成 | ||
- [x] 数据接入 | ||
- [x] 知识库 | ||
- [ ] 语料库 | ||
- [ ] 图表生成 | ||
- [ ] 任务规划 | ||
|
||
## ✨ 快速开始 | ||
### DataAgent部署 | ||
[https://www.yuque.com/biehuitou/dasgwp/gxii4gkkvudskf4k?singleDoc#](https://www.yuque.com/biehuitou/dasgwp/gxii4gkkvudskf4k?singleDoc#) 《DataAgent部署》 | ||
### 模型部署 | ||
#### 资源要求 | ||
- CPU:8核 | ||
- 内存:32GB+ | ||
- GPU:80G | ||
|
||
1、下载模型文件 | ||
> 下载地址,敬请期待 | ||
2、部署模型 | ||
|
||
这里展示使用FastChat来部署模型服务 | ||
> 需要根据现实情况手动修改下列配置项: | ||
{PATH_TO_SQL_MODEL_DIR} 需要替换成步骤1模型文件的下载地址 | ||
{MODEL_NAME} 是你希望暴露的模型调用的名称,使用接口调用部署了多个模型的服务时,可用此参数区分 | ||
{HOST} 暴露服务的地址,没有特殊需求可以写 0.0.0.0 | ||
{PORT} 暴露服务的端口 | ||
|
||
#[可选] 创建conda环境 | ||
conda create -n llmserver python=3.9 | ||
conda activate llmserver | ||
#安装依赖 | ||
pip3 install "fschat[model_worker,webui]" vllm | ||
#启动服务 | ||
# 启动控制器模块 | ||
python -m fastchat.serve.controller | ||
# 启动模型模型 | ||
CUDA_VISIBLE_DEVICES=0 python -m fastchat.serve.vllm_worker --model-path {PATH_TO_SQL_MODEL_DIR} --model-names {MODEL_NAME} --controller-address http://localhost:21001 --port 31002 --worker-address http://localhost:31002 --limit-worker-concurrency 10 --conv-template llama-2 | ||
# 启动server 模块 | ||
python -m fastchat.serve.openai_api_server --host {HOST} --port {PORT} | ||
|
||
|
||
[https://www.yuque.com/biehuitou/dasgwp/nhvzgnpyq7cmy590?singleDoc#](https://www.yuque.com/biehuitou/dasgwp/nhvzgnpyq7cmy590?singleDoc#) 《模型部署》 | ||
### 对你的数据库进行提问 | ||
1、训练你的数据库 | ||
|
||
curl -X 'POST' \ | ||
'http://localhost/v1/instruction/sync' \ | ||
-H 'accept: application/json' \ | ||
-H 'Content-Type: application/json' \ | ||
-d '{ | ||
"datasource_id": "datasource_id", | ||
"table_names": ["table_name"] | ||
}' | ||
|
||
``` | ||
curl -X 'POST' \ | ||
'http://localhost/v1/instruction/sync' \ | ||
-H 'accept: application/json' \ | ||
-H 'Content-Type: application/json' \ | ||
-d '{ | ||
"db_connection_id": "db_connection_id", | ||
"table_names": ["table_name"] | ||
}' | ||
``` | ||
2、训练你的知识库 | ||
|
||
curl -X 'POST' \ | ||
'http://localhost/v1/knowledge/train' \ | ||
-H 'accept: application/json' \ | ||
-H 'Content-Type: application/json' \ | ||
-d '{ | ||
"file_id": "file_id", | ||
"file_name": "file_name", | ||
"file":File | ||
}' | ||
|
||
``` | ||
curl -X 'POST' \ | ||
'http://localhost/v1/knowledge/train' \ | ||
-H 'accept: application/json' \ | ||
-H 'Content-Type: application/json' \ | ||
-d '{ | ||
"file_id": "file_id", | ||
"file_name": "file_name", | ||
"file":File | ||
}' | ||
``` | ||
3、通过自然语言查询数据 | ||
|
||
curl -X 'POST' \ | ||
'http://localhost/v1/chat/completions' \ | ||
-H 'accept: application/json' \ | ||
-H 'Content-Type: application/json' \ | ||
-d '{ | ||
"model": "sql_model", | ||
"messages": [{"role":"user","content":"自然语言问题"}], | ||
``` | ||
curl -X 'POST' \ | ||
'http://localhost/v1/chat/completions' \ | ||
-H 'accept: application/json' \ | ||
-H 'Content-Type: application/json' \ | ||
-d '{ | ||
"model": "sql_model", | ||
"messages": [{"role":"user","content":"自然语言问题"}], | ||
``` | ||
## 👏 贡献 | ||
我们欢迎各种贡献和建议,共同努力,使本项目更上一层楼!麻烦遵循以下步骤: | ||
|
||
- **步骤1:** 如果您想添加任何额外的功能、增强功能或在使用过程中遇到任何问题,请发布一个 [问题](https://github.com/hitsz-ids/SQLAgent/issues) 。如果您能遵循 [问题模板](https://github.com/hitsz-ids/SQLAgent/issues/1) 我们将不胜感激。问题将在那里被讨论和分配。 | ||
- **步骤2:** 无论何时,当一个问题被分配后,您都可以按照 [PR模板](https://github.com/hitsz-ids/SQLAgent/pulls) 创建一个 [拉取请求](https://github.com/hitsz-ids/SQLAgent/pulls) 进行贡献。您也可以认领任何公开的问题。共同努力,我们可以使DataAgents变得更好! | ||
- **步骤3:** 在审查和讨论后,PR将被合并或迭代。感谢您的贡献! | ||
|
||
在您开始之前,我们强烈建议您花一点时间检查 [这里](https://github.com/hitsz-ids/SQLAgent/blob/developing/CONTRIBUTING.md) 再进行贡献。 | ||
## 📖 文档 | ||
请在[这里](https://dataagent.readthedocs.io/zh/latest/index.html#)查看完整文档,将随着demo更改和代码发布更新。 |
This file contains bidirectional Unicode text that may be interpreted or compiled differently than what appears below. To review, open the file in an editor that reveals hidden Unicode characters.
Learn more about bidirectional Unicode characters
Original file line number | Diff line number | Diff line change |
---|---|---|
@@ -0,0 +1,20 @@ | ||
# Minimal makefile for Sphinx documentation | ||
# | ||
|
||
# You can set these variables from the command line, and also | ||
# from the environment for the first two. | ||
SPHINXOPTS ?= | ||
SPHINXBUILD ?= sphinx-build | ||
SOURCEDIR = source | ||
BUILDDIR = build | ||
|
||
# Put it first so that "make" without argument is like "make help". | ||
help: | ||
@$(SPHINXBUILD) -M help "$(SOURCEDIR)" "$(BUILDDIR)" $(SPHINXOPTS) $(O) | ||
|
||
.PHONY: help Makefile | ||
|
||
# Catch-all target: route all unknown targets to Sphinx using the new | ||
# "make mode" option. $(O) is meant as a shortcut for $(SPHINXOPTS). | ||
%: Makefile | ||
@$(SPHINXBUILD) -M $@ "$(SOURCEDIR)" "$(BUILDDIR)" $(SPHINXOPTS) $(O) |
This file contains bidirectional Unicode text that may be interpreted or compiled differently than what appears below. To review, open the file in an editor that reveals hidden Unicode characters.
Learn more about bidirectional Unicode characters
Original file line number | Diff line number | Diff line change |
---|---|---|
@@ -0,0 +1,29 @@ | ||
# SQLAgent API docs | ||
|
||
## Online docs | ||
|
||
Typically, our [latest API document](https://data-agent.readthedocs.io/en/latest/) can be accessed via readthedocs. | ||
|
||
## Build docs locally | ||
|
||
You can build the docs on your own computer. | ||
|
||
Step 1: Install docs dependencies | ||
|
||
``` | ||
pip install -e .[docs] | ||
``` | ||
|
||
Step 2: Build docs | ||
|
||
``` | ||
cd docs && make html | ||
``` | ||
|
||
Step 3 (Optional): deploy a local http server to view the docs | ||
|
||
``` | ||
cd build/html && python -m http.server | ||
``` | ||
|
||
Then access http://localhost:8000 for docs. |
This file contains bidirectional Unicode text that may be interpreted or compiled differently than what appears below. To review, open the file in an editor that reveals hidden Unicode characters.
Learn more about bidirectional Unicode characters
Original file line number | Diff line number | Diff line change |
---|---|---|
@@ -0,0 +1,6 @@ | ||
Sphinx | ||
sphinxemoji | ||
sphinx-copybutton | ||
sphinx-contributors | ||
git+https://github.com/SuperKogito/sphinxcontrib-pdfembed | ||
furo |
Loading
Sorry, something went wrong. Reload?
Sorry, we cannot display this file.
Sorry, this file is invalid so it cannot be displayed.
Oops, something went wrong.