- Object Detection using Deep Learning Model (YOLOv5)
- Training YOLOv5 with custom dataset
- Object location coordinate transformation ( image > Work space )
- Arduino control using Object location
- we creating cutom dataset using roboflow
- Training YOLOv5-s model using colab
- YOLOv5 Github Link : https://github.com/ultralytics/yolov5
%cd /content !git clone https://github.com/ultralytics/yolov5.git !python train.py --img 416 --batch 16 --epochs 100 --data /content/dataset/data.yaml --cfg ./models/yolov5s.yaml --weights yolov5s.pt
- real-time object detection
So we converted object location coordinate(pixel) within the image to (theta1, theta2)
[Web cam to Real place]
Web cam[px] | Real Value[cm] | Scale factor[px/cm] | |
---|---|---|---|
1 | (245, 270) | (26.5, 20) | (0.1082, 0.0741) |
2 | (195, 345) | (21.5, 25) | (0.1102, 0.0724) |
3 | (297, 198) | (31.5, 15) | (0.1060, 0.0758) |
4 | (135, 198) | (16.5, 15) | (0.1222, 0.0758) |
So we get average scale factor (0.1116, 0.0745)
[ Real place to Polar]
[Polar to Arduino]
Radius(r) | Theta2(degree) | |
---|---|---|
1 | 52.5 | 92 |
2 | 48.5 | 93 |
3 | 15.5 | 94 |
4 | 41.5 | 95 |
5 | 39.5 | 96 |
6 | 35.8 | 97 |
7 | 30.5 | 100 |
8 | 29.5 | 101 |
9 | 26.5 | 103 |
10 | 23.3 | 106 |
11 | 20.0 | 109 |
12 | 17.7 | 112 |
13 | 14.5 | 117 |
14 | 11.0 | 125 |
15 | 9.3 | 130 |
16 | 7.4 | 135 |
17 | 4.7 | 145 |
18 | 2.3 | 155 |
19 | 0.0 | 175 |
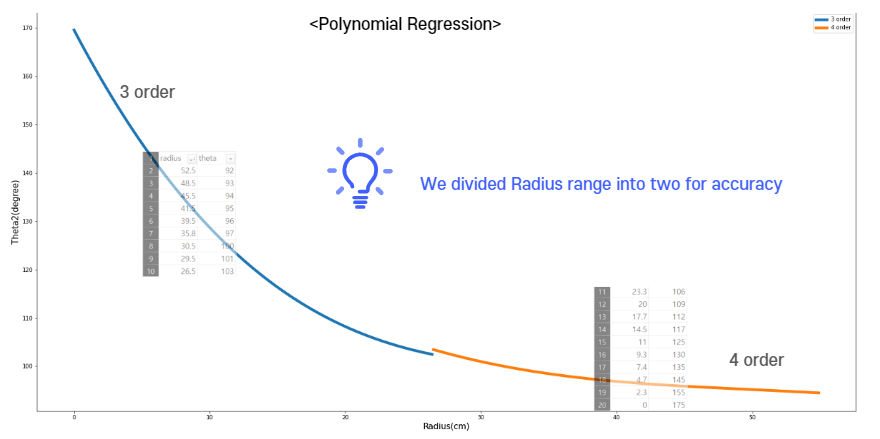
import numpy as np
# pixel to cm
real_x = (pixel_x) * 0.11165
real_y = (pixel_y) * 0.0745
# cartesian to polar
R = (real_x**2 + real_y**2)**0.5 - 6.5
theta1 = 115 - np.degrees(np.arctan(real_y/real_x))
# r to theta2 (Regression)
if R >= 26.5:
# 4 order polynomial regression
theta2 = (-0.0000001)*(R**4) - (0.0004)*(R**3) + (0.0611)*(R**2) -3.1901*(R) + 152.57
else:
# 3 order polynomial regression
theta2 = (-0.0012)*(R**3) + (0.1378)*(R**2) -5.3419*(R) + 169.53